- 1Third Institute of Oceanography, Ministry of Natural Resources, Xiamen, China
- 2College of Ocean and Earth Sciences, Xiamen University, Xiamen, China
- 3Hainan Academy of Ocean and Fisheries Sciences, Haikou, China
- 4Key Laboratory of Marine Ecological Conservation and Restoration, Ministry of Natural Resources, Xiamen, China
- 5Fujian Provincial Key Laboratory of Marine Ecological Conservation and Restoration, Xiamen, China
- 6Fisheries College, Jimei University, Xiamen, China
Global change has generated challenges for oceans, from individuals to the entire ecosystem, and has raised contemporary issues related to ocean conservation and management. Specifically, coral reef ecosystems have been exposed to various environmental and human disturbances. In this study, the Ecopath with Ecosim model was used to explore the impacts of ocean warming and fishing on Xisha Islands coral reef ecosystem in the South China Sea. The variables in this model included two ocean warming scenarios and three fishing scenarios. The model consisted of 23 functional groups including algae, coral, sea birds, and sharks. Our results showed that by the middle of the century, ocean warming and fishing led to a 3.79% and 4.74% decrease in total catch compared with 2009, respectively. In addition, the combined effects of ocean warming and fishing caused a 4.79% decrease in total catch, and the mean trophic level of catch was predicted to decrease by 6.01% under the SSP585-High fishing scenario. Reducing the fishing effort mitigates the effects of ocean warming on some species, such as large carnivorous fish and medium carnivorous fish; however, under low fishing effort, some functional groups, such as small carnivorous and omnivorous fish, have low biomass because of higher predation mortality.
1 Introduction
Coral reefs are ecosystems with rich biodiversity and high service value, and are referred to as “marine rainforests.” (Smith, 1978; Reaka-Kudla., 1997; Miloslavich et al., 2005; Hughes et al., 2017; Williams et al., 2019). The coral reef ecosystem provides food, medicine, tourism, aesthetics and coastal zone protection, and it is of great significance for the socio-economic, cultural, and security of the country and region, playing an irreplaceable role in regulating the global climate and ecosystem balance (Pilling et al., 2017; Zheng et al., 2021). Although coral reefs only account for approximately 0.2% of the world’s ocean area (Spalding et al., 2001; Fisher et al., 2015), they support nearly one-third of the world’s marine fish species and approximately 10% of the marine fishery catch (Smith, 1978; Zheng et al., 2021).
However, in recent decades, coral reefs worldwide have faced various threats, and a series of climate change impacts and human activities have led to the degradation of coral reefs, with 75% of global coral reefs seriously threatened (Bruno et al., 2007; Hu et al., 2020). The service value of coral reef ecosystems is declining at an alarming rate owing to global and local pressures (Eddy et al., 2021). Moreover, some studies have shown that 50% of tropical coral reefs will lose their basic ecological and fishery functions by 2050 (Bellwood et al., 2004; Bruno et al., 2007). Ocean warming and fishing are the most important threat factors that affect the coral reef ecosystem, which may cause widespread degradation of the ecosystem (David et al., 2011), reducing the stability of tropical coral reefs (Zhang et al., 2017; Gibert, 2019; Inagaki et al., 2020), and increasing ecosystem vulnerability (Hughes et al., 2003; Pandolfi et al., 2003; Bellwood et al., 2006; Hughes et al., 2007).
Ecosystem models are generally used to examine ecological issues and one of their applications is assessing the impacts of climate change. These include the Ecopath with Ecosim (EwE) model (Christensen et al., 2014), OSMOSE (Shin and Cury, 2001), Atlantis model (Fulton et al., 2011), and linear inverse model (Legendre et al., 2013). One of the most widely used ecosystem model and food web network analysis tools for marine, estuarine, and other ecosystems worldwide is EwE (Christensen et al., 2008). Ecopath was originally used to build coral reef ecosystems (Polovina, 1984) and was later developed into the EwE software (Christensen et al., 1992; Christensen et al., 2005). Although there are more than 500 models which used the EwE model, less than 70 EwE models have focused on the coral reef ecosystem owing to its complexity. Most previous studies focus on the static structure of the ecosystem, with a lesser focus on the impact of a single factor on the coral reef ecosystem, and the comprehensive impact of multiple factors is not well examined (Colléter et al., 2015; Argüelles-Jiménez et al., 2020). For example, by the end of the century, ocean warming would lead to a reduction in the total standing biomass of 1.00%, 8.00%, and 44.00% in RCP2.6 (low greenhouse gas emissions), RCP4.5 (medium greenhouse gas emissions), and RCP8.5 (high greenhouse gas emissions) in the coral reef ecosystem in Brazil, respectively, and change the ecosystem structure to promote low trophic level functional groups, and cause algal increase during coral decrease (Capitani et al., 2022). The biomass of the Red Sea coral reef ecosystem would decrease with an increase in fishing effort, except for those developed by beach seines, consisting of low trophic level fishes (Tesfamichael et al., 2016). The coral-algal phase shifts on coral reef ecosystem would result in reduced biodiversity and ecosystem maturity in Raja Ampat Archipelago in Eastern Indonesia, and the landing of traditional targeted species would greatly reduce (Answorth et al., 2015). In recent years, some studies have reported the combined impacts of overfishing and climate change on large marine ecosystems or estuarine ecosystems. For example, large-scale declines in biomass under climate change in the South China Sea (SCS), and a 99.00% decline in biomass compared to the levels in 2000 of 17 functional groups were predicted under baseline fishing and the RCP8.5 scenario (Teh et al., 2019). The combined effects of climate change and fishing will reduce the biomass in the Pearl River Estuary (PRE) ecosystem (Zeng et al., 2019). However, there is a lack of studies on the combined effects of ocean warming and fishing on coral reef ecosystems, which hinders our forward-looking management.
Xisha Islands are located in the north-central part of the SCS and at the northern edge of the “coral triangle” with the highest biodiversity worldwide (Allen, 2008; Huang et al., 2008). Coral reefs of Xisha Islands are a typical oceanic worldwide, as well as the oldest and most precious coral reefs in China (Huang et al., 2008), and one of the main fishing grounds for reef fisheries in the SCS (Li et al., 2007). Recently, Xisha Islands coral reef (XICR) ecosystem has faced threats of ocean warming and overfishing (Shi et al., 2008; Liu et al., 2021; Wang et al., 2022), leading to a 16.28% reduction in coral reef coverage (Zuo et al., 2020), a decline in fish productivity, diversity, and large fish populations (Li, 2020), and an explosion in the crown of thorns (Acanthaster planci) (Li et al., 2019). In addition, the sea surface temperature (SST) of Xisha Islands rises faster than that of the Zhongsha and Nansha Islands (Zuo et al., 2015), and the ecosystem here has weak resistance to external disturbances (Hong et al., 2021). Although a few studies have examined the effects of global warming on corals in the SCS, for example, the potential threat of global warming to coral growth was explored in Xisha Islands and Nansha Islands (Shi et al., 2008), and the remote sensing image records of coral reefs in the SCS responding to climate warming in the past 40 years were analyzed (Liu, 2020), the fate of coral reef ecosystems in the SCS under climate change and fishing remains unknown.
In this study, we developed the Ecopath and Ecosim models using continuous underwater monitoring data for Xisha Islands from 2009 to 2020, and projected trends under different scenarios up to the 2050s. The main objectives were to (1) explore the food web structure of coral reef ecosystem in the SCS and its differences compared to other regions (2) study the combined effects of ocean warming and fishing on the coral reef ecosystem and identify factors exerting greater influence. This study is the first to examine the effects of global change on coral reef ecosystems in the SCS. Through this study, we aimed to provide a scientific basis for the conservation and management of coral reef ecosystems in the SCS in the context of global change in the future.
2 Materials and methods
2.1 Study area
Xisha Islands are located at 15°46′N−17°08′N and 111°11′E−112°54′E (Figure 1). It is one of the archipelagos with the largest land area (approximately 10 km2) and the largest number of islands in the SCS (Li et al., 2002). It comprises Yongle and Xuande islands, with 26 islands and sandbanks, 11 underwater reefs, shoal reefs, and sandy beaches. The climate is tropical monsoon, with an annual average SST of 27.50°C. The highest temperature is from May to September (average 29.90°C), and the lowest is from December to February of the following year (average 24.80°C). The transparency of seawater is usually greater than 15 m and can even exceed 30 m. The environmental conditions are suitable for the growth of coral communities (Zuo et al., 2020). The biological resources on Xisha Island are very rich and this region serves as important fishing grounds for coral reef fisheries in the SCS (Li et al., 2007). The island also supports high biodiversity, with 643 fish species, 409 coral species, and 1570 benthic species (Li et al., 2002; Huang et al., 2018; Qiu et al., 2022).
2.2 Food web modeling
The Ecopath with Ecosim (EwE) model (Christensen and Pauly, 1992; Walters et al., 1997; Walters et al., 2000) was used to estimate the model for XICR ecosystem. This model combines a static (Ecopath) and a time dynamic model (Ecosim). The Ecopath model can be used to describe the state of an ecosystem at a given time, and Ecosim is used to simulate the temporal dynamics of a food web under environmental disturbances and fishing (Christensen et al., 2005).
2.2.1 Ecopath
To reduce the complexity of the food web, individuals or groups of species were assigned to similar functional groups or guilds, which share similar ecological parameters, such as growth and consumption rates, diet composition, and predators. Ecopath assumes that the production of each functional group is equal to the consumption due to predation, fishing mortality, biomass accumulation, and migration. The following equations describe the Ecopath model:
where Bi is the biomass of the prey species (i) of group i; (P/B)i is the production/biomass ratio for i; EEi is the ratio of production to consumption for group i; Ei stands for the net emigration of I; Bj is the biomass of consumers (j) of group i; (Q/B)j is the ratio of consumption to biomass per unit of j; DCji is the proportion of i in j’s diet; Yi is the fishery catch of group I; and BAi is the biomass accumulation of group I.
To satisfy the energy flow balance among the functional groups of the ecosystem, each functional group should satisfy the second equation, where Q is consumption, P is production, R is respiration, and U is unassimilated food.
2.2.2 Ecosim
Ecosim enables dynamic simulation at the ecosystem level by inheriting key parameters from Ecopath (Christensen et al., 2008). The following equation describes the Ecosim model.
where dBi/dt is the growth rate of the biomass of group i in time interval dt; gi is the estimated net growth efficiency based on P/B and Q/B; MOi is the estimated natural mortality based on the ecotrophic efficiency; ei is the emigration rate; Ii is the immigration rate. The two summations of Equation (3) estimate consumption rates, the first is the total consumption of group i and the second is predation by its predators on group i.
2.3 Model parameterization
The Ecopath model was built to simulate the coral reef ecosystem of Xisha Islands and was used as the basis for Ecosim. The aquatic ecosystem is very complex, aggregating species into function group is a way to reduce the complex of food web, each function group is conformed according to species traits that are most relevant to define their ecological role. Twenty-three functional groups were defined according to the ecology, taxonomy, feeding or phylogeny characteristics of organisms in the coral reef ecosystem in Xisha Islands, the latest literature in the same area (Hong et al., 2021), and the fish resource survey conducted in Xisha Islands (Sun et al., 2005; Wang et al., 2011), ranging from primary producers to herbivorous fish and Sharks, which basically covered the energy flow process of each trophic level of the ecosystem. The biomass of fish and benthos functional groups were estimated using diving data from Underwater Visual Census (UVC) conducted in 2009, whereas the biomass of other functional groups was got from studies in the SCS (Huang et al., 2009; Hong et al., 2021) and other coral reef model (Du et al., 2020). The P/B, Q/B and EE values of each functional group were mainly acquired from the Qilianyu coral reef model, which lies in the same region (Hong et al., 2021). Diet composition was derived from our feeding studies, stable isotope analysis, and FishBase (https://www.fishbase.se/). The catch data was obtained from comprehensive estimation through interviews and discussions with fishers. Detailed parameters are listed in Table 1.
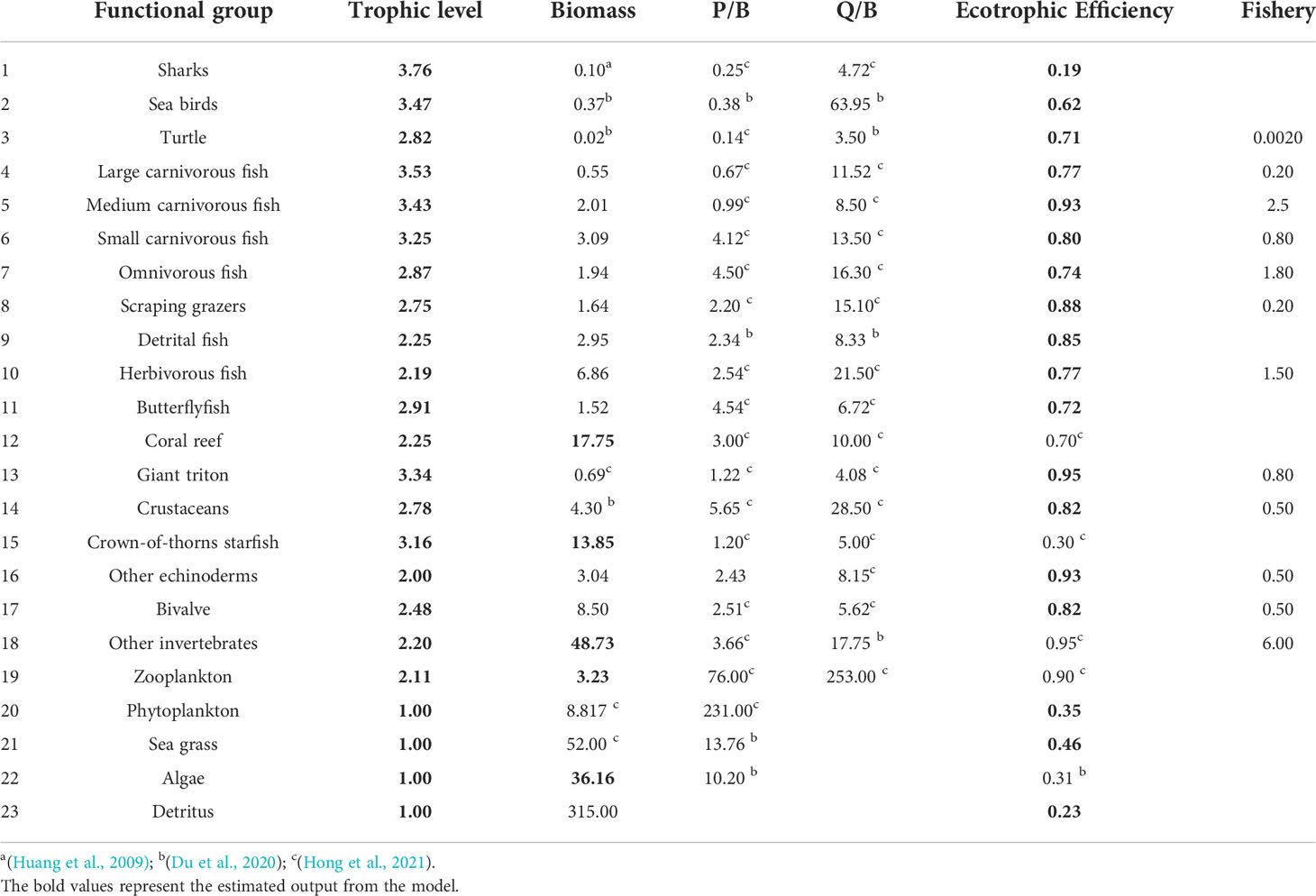
Table 1 Basic input and estimated (bold) parameters for the functional groups in the coral reef model of the XICR ecosystem.
2.4 Addressing uncertainty in input data
The Ecosampler module in EwE model is used to analyze the impact of uncertainty of input parameters on model output parameters (Steenbeek et al., 2018). We used the Monte Carlo sampling method built in Ecosampler to set a 20% uncertainty for the B, P/B, Q/B and EE parameters of the functional group, and randomly generate 500 rebalanced XICR Ecopath models.
2.5 Simulating ocean warming effects in Ecosim
Ecosim allows us to explore the effects of climate change and human activities on ecosystems over long time scales by fitting time series data. In this study, the time series contained reference data and driving data. The reference data consisting of the biomass of eight fish functional groups was obtained through diving surveys in 2009−2020. The driving data consisted of data on chlorophyll a concentrations, and was obtained from National Oceanic and Atmospheric Administration (https://www.noaa.gov/). In the EwE model, the forcing function was used to express the species’ responses to temperature change. It was assumed that the predicted temperature preferences of the species conform to a Gaussian distribution. The Gaussian function was the best statistical fit for the temperature survival curve of fish eggs in the North Atlantic (Dahlke et al., 2020). The species has the highest growth rate at the preferred temperature. Temperatures lower or higher than the optimal will cause a decrease in biomass and productivity (Zeng et al., 2019). In this study, the preferred temperatures of species in Xisha Islands ecosystem were calculated according to previous studies (Day et al., 2018) and FishBase (https://www.fishbase.se/).
SST was obtained from the GFDL-ESM4 (https://esgf-node.llnl.gov/search/cmip6/). It contains historical data (1990–2014) and future simulation data (2015–2100). This study used SSP126 (low radiation forcing scenario) and SSP585 (high radiation forcing scenario) as two climate scenarios. SST selected the r1i1p1f1 model, the spatial resolution contained 15°46′−17°08′ N, 111°11′−112°54′ E, the accuracy was 1°×1°, and the time resolution was monthly. To improve the credibility of future forecast data, the deviation of the climate model data was corrected according to the retrieved SST based on MODIS data. The retrieved SST data were obtained from the National Oceanic and Atmospheric Administration (https://www.noaa.gov/). The average value of the processed temperature was considered the final SST. The SST in Xisha Islands is projected to increase by approximately 0.47 °C and 1.17 °C under SSP126 and SSP585 in 2050, respectively (Figure 2). Based on this, normalized preference indices for different environmental temperatures were calculated, and biomass dynamics were simulated based on the forcing function and projected SST from 2009 to 2050.
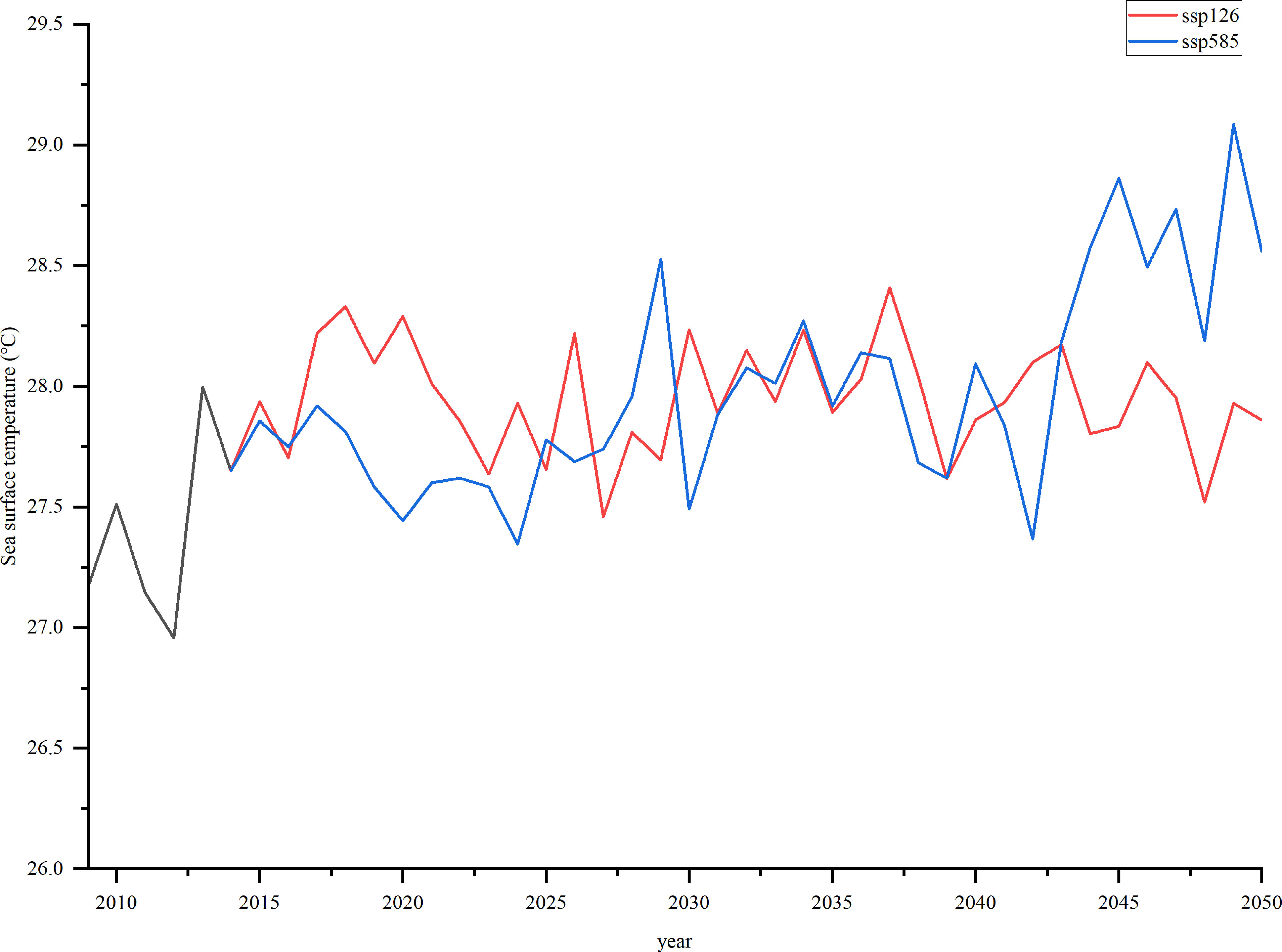
Figure 2 Sea surface temperature (°C) in Xisha Islands for the two scenarios (SSP126 and SSP585) in 2009–2050.
2.6 Simulating fishing effects in Ecosim
Three fishing scenarios were simulated to explore the potential effects of fishing on the Xisha Reef ecosystem. Given that the current global fishing capacity may be 1.5 to 2.5 times the level required to maximize sustainable fishing (Porter, 1998; Sumaila et al., 2012), fishing efforts may need to be reduced by 40 to 60% (World Bank, 2017). In the study of the SCS, the fishing effort in 2100 was simulated to be 50% lower than that in 2010, and the rate of decline was approximately 0.56% per year (Teh et al., 2019). According to this rate, the fishing effort is expected to decline by approximately 20% by 2050. Considering factors such as population and economic constraints, the amount of fishing effort cannot be reduced suddenly, and referring to the study conducted on the Pearl River Estuary (Zeng et al., 2019), we chose the strategy of year by year reduction, to reduce the fishing effort by 20% in 2050 compared with 2009. At the same time, in order to explore the impact of increased effort on the ecosystem, we increased the fishing effort by 20% in the same manner. Thus, the fishing effort in 2009 was set to 100%, and the relative effort in 2050 was set to 80%, 100%, and 120% of 2009, which represented the low, medium, and high fishing scenarios, respectively.
3 Results
3.1 Ecopath model outputs
The sensitivity analysis of the input parameters of the Ecopath model is based on 500 Monte Carlo simulations of Ecosampler embedded in the software. It showed that the average perturbations of EE, P/B, and Q/B were less than 10%, and the disturbance of biomass was less than 12% (Figure 3). The Ecopath model output showed that the trophic level (TL: refers to the level of organisms in the food chain of the ecosystem.) of the XICR ecosystem was 1–3.76, and cartilaginous fish had the highest TL (3.76), followed by large carnivorous fish (3.53), and the level of the coral reef fish functional groups was 2.19–3.53. The energy flows of 23 functional groups in the XICR ecosystem were merged into four TLs through trophic level aggregation, and the total flow of each trophic level decreased with an increase in the nutrient level. The total energy flow of TL I and II accounts for 66.05% and 27.51% of the total energy flow, respectively, and the total energy flow of TL III–IV accounts for 6.44%, conforming to the ecological energy pyramid law. In the XICR ecosystem, the food web comprises grazing and detritus food chains, with 40.00% of the total energy directly derived from detritus and 60.00% from primary producers. A grazing food chain dominates the current ecosystem.
3.2 Projected changes in biomass
Non-producer biomass through the food web increased from 2009 to 2050 for SSP126 and SSP585 in the low and medium fishing scenarios, while the biomass of non-producers projected a decrease for the two ocean warming scenarios in the high fishing scenarios (Figure 4). In the SSP126 scenario, the model expected that the biomass of non-producers would increase by 1.80% and 0.46% for low and medium fishing intensities, respectively, while it would decrease by 1.52% for high fishing effort. In the SSP585 scenario, the biomass of non-producers was predicted to increase by 1.65% and 0.29% for low and medium fishing intensities, respectively, while the predicted ocean warming would lead to a 1.32% decrease in the high fishing effort between 2009 and 2050. Neither SSP126 nor SSP585 caused more than a 2.00% change in the biomass of non-producers in all fishing scenarios. The increase in high trophic level functional groups led to an increase in non-producer biomass, such as large carnivorous fish, medium-carnivorous fish, and sea birds. However, the fishing intensity was predicted to be the primary factor determining changes in biomass.
3.3 Projected changes in catch
Ecosim predicted a decline in fishery catches from 2009 to 2050 for SSP126 and SSP585 across all fishing efforts (Figure 5). In the SSP126 scenario, compared with the baseline scenario, fishery catches were expected to decrease by 11.53, 2.62, and 4.73% for low, medium, and high fishing efforts by 2050, respectively. In the SSP585 scenario, compared with the baseline, the model projected that the fishery catches of the XICR ecosystem would decrease by 12.26, 3.78, and 4.78% for low, medium, and high fishing efforts by 2050, respectively. Fishing intensity was predicted to be the main factor determining the future catches of the XICR ecosystem. The results showed that catches decreased more at low fishing intensity than at medium and high fishing intensities. Simultaneously, ocean warming was predicted to change the total fishery catch by no more than 1.20% in either the SSP126 or SSP585 scenarios.
3.4 Projected changes in the food web structure
Unlike the biomass of non-producers, the transfer efficiency of the coral reef ecosystem of Xisha Islands decreased for all scenarios, but the magnitude was different (Figure 6). The model assumed that ocean warming (SSP126 and SSP585) had little effect on the transfer efficiency because there was no significant difference between SSP126 and SSP585 in each fishing intensity scenario. In contrast, the fishing intensity was predicted to be a major determinant of future transfer efficiency because there were obvious differences between low, medium, and high fishing intensity settings. In the low fishing scenario, compared to 2009, SSP126 and SSP585 decreased by 0.49% and 0.43%, respectively, whereas in the medium fishing effort scenario, the transfer efficiency was predicted to decrease by 0.37% and 0.43%, and in the high fishing intensity scenario, it decreased by 2.41% and 2.35% under SSP126 and SSP585, respectively. There was a slight difference between the low and medium fishing scenarios. However, in the high fishing scenario, the transfer efficiency for SSP126 and SSP585 decreased by 2.41% and 2.35%, respectively.
In the SSP126 scenario, the change in trophic level of catch (TLC) was projected to decrease by 0.42% and 5.74% under medium and high fishing intensity, respectively (Figure 7). However, under low fishing intensity, it would increase by 1.94% by 2050 compared with the baseline. The model expected that the change in the trophic level of the community (TLcom) would increase under low and medium fishing intensity, while TLcom was predicted to decrease (0.32%) under high fishing intensity. In the SSP585 scenario, TLC would increase (1.64%) under low fishing intensity while decreasing by 0.84% and 6.01% under medium and high fishing efforts, respectively. Notably, the model projected that the impact of fishing intensities on TLC and TLcom was more prominent than that of ocean warming.
In the SSP126 scenario, changes in Kempton’s Q were more evident than those in the Shannon index (Figure 8). The model expected that by 2050, the fishing intensity would lead to an increase by 9.90% and 0.18%, higher than the baseline under low and medium fishing efforts, respectively. However, there was a decrease (24.73%) under high-intensity irradiation. The Shannon index was not expected to change by > 1% in all settings. The results showed that it would increase by 0.72% and 0.45% under low and medium fishing intensity, respectively, whereas it would decrease by 0.27% under high fishing effort. The changes in Kempton’s Q and Shannon index in the SSP585 scenario were similar to those in SSP126; however, the degree of change was higher than that in the SSP126 scenario. The model predicted that by 2050, Kempton’s Q in the SSP585 would increase by 11.96% compared with the baseline under low fishing intensity, whereas it would decrease by 0.47% and 26.50% under medium and high fishing efforts, respectively. The Shannon index was projected to increase by 0.49% and 0.18% for low and medium fishing intensities, respectively, whereas it decreased slightly from 100.00% to 99.51% under high fishing effort. Fishing intensities were predicted to be the most important factor in determining future changes in Kempton’s Q and Shannon indices.
The total standing biomass through the food web varied for the different scenarios (Figure 9). In SSP126, the total standing biomass was projected to decrease by 0.27% under medium fishing intensity and by 1.19% under high fishing effort, whereas it would increase by 0.43% under low fishing intensity. In the SSP585 scenario, the total ecosystem standing biomass was predicted to increase by 0.33%, 0.07%, and 0.80% for low, medium, and high fishing efforts, respectively.
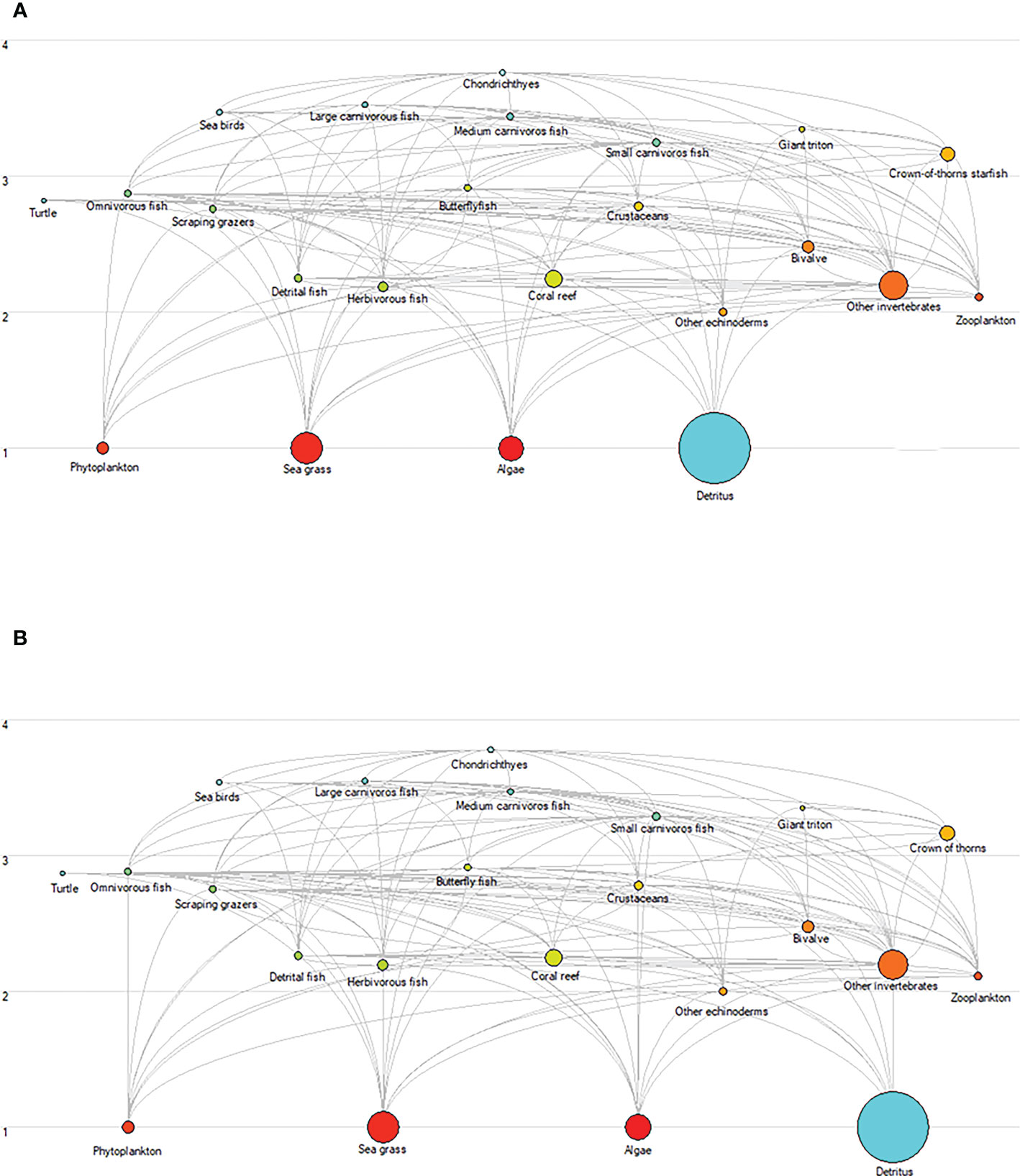
Figure 9 Changes in biomass and energy flows within the XICR ecosystem food web for ocean warming and fishing. (A), food web of baseline ecosystem (2009). (B), food web of the Xisha ecosystem for the SSP585-High fishing scenario (2050). Each circle represents a functional group, and its size represents the biomass. Functional groups of food webs are represented by trophic levels and are linked by relationships between predators and prey.
4 Discussion
The biomass of this study area is similar to that in the East Brazil Large Marine Ecosystem (Freire et al., 2008), slightly higher than that of Qilianyu Islands (Hong et al., 2021) and Lembeh Strait (Chen et al., 2009), but much lower than that of the Abrolhos region (Rocha et al., 2007). The study area of Abrolhos has been protected since 1983 and is expected to have higher biomass. The total system throughput was 7937 t/km2/year, similar to the study in Qilianyu Islands coral reef ecosystem but lower than that of other coral reef ecosystems in Table 2. However, the study area of Xisha Islands is larger than that of the other study areas, with an area of approximately 5×105 km2. The System Omnivory Index (SOI) of the XICR ecosystem was 0.24, whereas it was 0.22 in the Qilainyu model, indicating the TL of functional groups in the XICR did not change obviously, showing that the connection between organisms in XICR food web is more complex. Moreover, the mean trophic level of the catch of XICR is lower than other ecosystems, indicating that the area is greatly affected by fishing. In addition, the total primary production/total respiration (TPP/TR) is an indicator of ecosystem maturity (Odum, 1969; Christensen, 1995), with a range of 0.80–3.2, indicated more maturity when near 1 (Christensen and Pauly, 1992). TPP/TR of XICR ecosystem was 2.47, while the values of the Lembeh Strait and East Brazil Large Marine Ecosystem were 1.48 and 6.60, respectively (Chen et al., 2009; Freire et al., 2008). However, TPP/TR has great variability, it needs more indicators such as macro-descriptors or development attributes to measure the maturity (Argüelles-Jiménez et al., 2020; Arguelles-Jimenez et al., 2021).
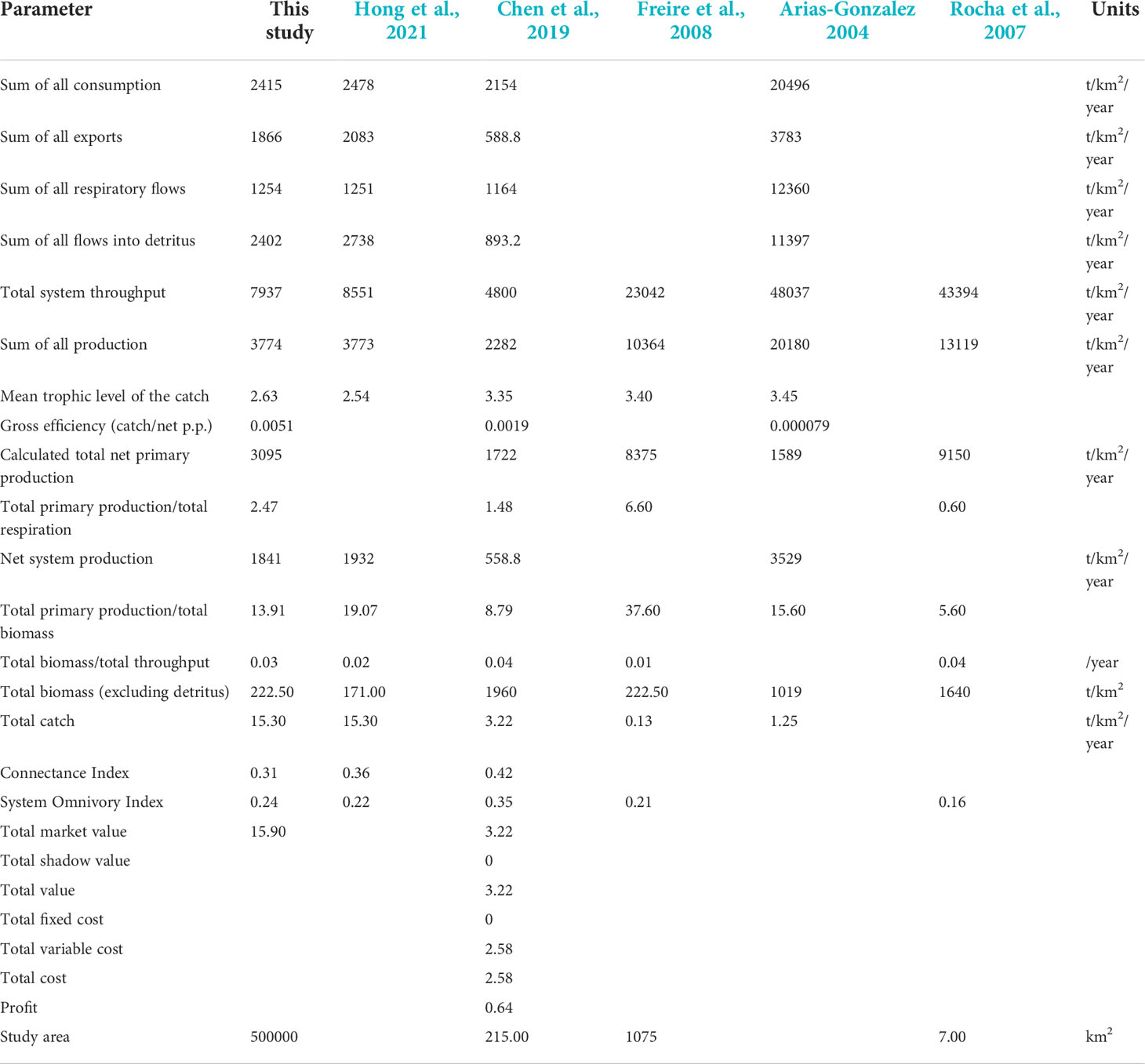
Table 2 System statistics were obtained from the Ecopath model of XICR and other models of coral reef ecosystems.
Ocean warming can influence the ingestion, digestion, absorption, and assimilation of marine organisms because it requires extra energy to regain homeostasis and endure extreme temperature events (Volkoff et al., 2020; Shahjahan, 2022). In addition, ocean warming can reduce the reproductive capacity of fishes (Rummer et al., 2017). Our results showed that by 2050, the total biomass of fish would decrease, and the biomass of fish with low thermal niches would decrease under the high emission scenario. This phenomenon may be because marine fishes respond to ocean warming by changing their distribution, usually to higher latitudes and deeper waters (Cheung et al., 2013). Ocean warming also directly affects coral reefs, possibly because coral reefs usually live close to the upper limits of their thermal tolerance, and even small temperature increases can lead to bleaching and mortality (DeCarlo et al., 2019). Coral biomass decreased by 0.23% under SSP585, and when coral was lost, algal biomass increased, leading to a decrease in biodiversity and ecosystem maturity; additionally, the biomass of small-bodied fish would increase, implying that there would be higher productivity on reefs, although the total fish landed would be reduced (Ainsworth et al., 2015). In our study, the total production was predicted to increase, whereas the total catch showed a decrease in all scenarios.
The XICR ecosystem has also been affected by ocean warming. The evenness of the ecosystem was projected to change by 2050, and the evenness of the high trophic species would also shift, especially in the high emission scenario. The Shannon index would not shift considerably, but Kempton’s Q index would be influenced by ocean warming, consistent with the study on the Pearl River Estuary (PRE). A study on PRE ecosystem predicted changes in the ecosystem under the dual pressure of climate change and fishing using the EwE model (Zeng et al., 2021). The results indicated that the Shannon index was slightly influenced by climate change, whereas Kempton’s Q index was predicted to show a different pattern (Zeng et al., 2019). Ocean variables, including ocean warming, net primary production, dissolved oxygen, and ocean acidification, were predicted to shift the Shannon index during small events, while ocean warming was forecasted to cause a large decrease in the Kempton's Q index (Zeng et al., 2019). These changes indicate that the XICR ecosystem structure will change by 2050, leading to a reduction in the resilience of the coral reef ecosystem. Changes in the ecosystem structure can alter the function of coral reef ecosystems (Dulvy et al., 2004; Inagaki et al., 2020). One of the main indicators of the degeneration of coral reef ecosystems is a significant increase in the biomass of macroalgae (Jackson et al., 2001; Kramer, 2003; Acosta et al., 2013), which confirms our findings. Our results predicted that coral biomass would decrease whereas algal biomass would increase in the SSP585 scenario.
Overfishing can affect the biomass of coral reef ecosystems, especially the biomass of large fish and scraping grazers, which are indicators of the health of the ecosystem (McClanahan et al., 2014; Lefcheck et al., 2021). Reducing large predators and competitors alters nutrient profiles, often increasing the number of prey species (Steneck, 1998). In our model, the results predicted that when the fishing effort was reduced (in the low fishing scenario), there would be a small increase in biomass (0.33%), similar to that observed in a previous study in the East China Sea (ECS). Research in the ECS, including 24 functional groups, simulated the impact of the seasonal fishing moratorium (SFM) from 1997 to 2018 (Xu et al., 2022). Compared with no SFM, the biomass of the ECS increased by 67.12% by 2018 with SFM. The fishing policy of the ECS is seasonal and more reasonable, which may be the reason for the larger gap between the growth amplitudes of the XICR and ECS. A significant increase (SOI increased from 0.13 to 0.18) in the ECS ecosystem maturity indicates a slow recovery of the ECS ecosystem in the past two decades (Xu et al., 2022). In our study, the SOI was predicted to decrease from 0.25 (high fishing intensity) to 0.24 (low fishing intensity). This suggests that the ecosystem can recover to some extent by reducing fishing intensity. Furthermore, the ecosystem can be repaired better through seasonal fishing moratoriums.
Overfishing can also cause ecological problems. With the increase in fishing effort, the biomass of crown of thorns starfish, which is a large predator of coral reefs, has increased by 1.71% under high fishing effort. In healthy coral reef ecosystems, the density of thorn of crowns usually does not exceed one per hectare (Wabnitz et al., 2010). One crown of thorns can eat approximately 0.1 m2 of healthy coral on average every day (Babcock et al., 2016; Li et al., 2019). When the density exceeds 30 per hectare, the coral biomass cannot meet the predation demand of the crown of thorns, leading to an imbalance in the coral reef ecosystem (Wabnitz et al., 2010). Coral reefs damaged by the crown of thorns cannot recover to their pre-outbreak levels, even over a long period (Li et al., 2019). Therefore, fishing effort should be controlled to keep the biomass of crown of thorns from exploding in large numbers.
Our findings also suggest that there is a risk of ecosystem degeneration due to ocean warming and overfishing, consistent with the findings of recent studies (Burke et al., 2011; Blowes et al., 2019; Bryndum et al., 2019), especially in coral reef ecosystems where human activities are exacerbating the degradation caused by ocean warming (Hughes et al., 2017; Beyer et al., 2018; Capitani et al., 2022). In this case, TPP/TR would decrease by 0.40%, and SOI would decrease by 4.17% in the SSP585-High fishing scenario, indicating that the maturity of the XICR ecosystem would continue to decrease with increasing dual pressure of ocean warming and fishing.
Reducing fishing intensities can ease the impact of ocean warming on most fish functional groups (McClanahan et al., 2014). However, the biomass of some species will also decrease under low fishing effort because they may have higher fishing mortality (Zeng et al., 2019). In this study, the total biomass of the XICR ecosystem was 220.7 t/km2 in the SSP585-high fishing scenario, whereas it was 224.2 t/km2 in the SSP585-low fishing scenario, and the increase in fish biomass was mainly concentrated in functional groups composed of non-economic fish, such as butterflyfish and detrital fish. Several studies have also suggested that fishing activities could aggravate the effects of ocean warming and prolong the recovery time of species (Brander, 2007; Kirby et al., 2009; McClanahan et al., 2014; Zeng et al., 2019). In addition, fishing can remove large and older species, increasing age-truncated or juvenile populations in ecosystems (McOwen et al., 2015; Zeng et al., 2019), which can increase the sensitivity of ecosystems to ocean warming (Zeng et al., 2019). One study found that there are mainly small fish species in the XICR ecosystem, and the density of grouper and parrotfish with high food value is low (Yang et al., 2018), which aggravates the impact of climate warming on the ecosystem.
It should be noted that there were some uncertainties in this study. First, the tradeoff between simplicity and complexity is a source of uncertainty. To solve the variability in functional groups and reduce uncertainty, each species is classified into functional groups based on traits, such as individual size, diet, and habitat (Milessi et al., 2010). EwE is advised to divide species into multi-stanza groups (sub-groups), making their dynamics more realistic and providing insights into stock-recruitment relationships, especially the top predators (Christensen et al., 2008; Du et al., 2020). However, we did not define the groups representing life stages or stanzas for species with complex trophic ontogeny in this study owing to a lack of data, which may be uncertain in this model. Twenty-three functional groups are far from enough for complex coral reef ecosystem, the future research can combine diving survey data and net fishing data through cooperation with other fisheries research institute, in order to obtain more biodiversity data and more functional groups, not only the Chondrichthyes, but also the multi-stanza of the main functional groups. Second, some input parameters derived from other ecosystems are relatively more uncertain. For example, the biomass of sharks and phytoplankton was based on previous studies in the SCS (Huang et al., 2009; Hong et al., 2021). Sensitivity analysis showed that biomass has more influence on Ecosim results. To improve the accuracy of the model prediction, historical comparison data were used to reduce the differences between the historical observations and model predictions (Christensen et al., 2008). However, in this study, full historical comparison data were only available for a small number of functional groups, and the normalized change in chlorophyll in Xisha from 2009 to 2021 was applied in our model. The third uncertainty is associated with an incomplete understanding of species’ response to ocean warming and their interaction with fishing. In this model, the forcing function used in the ocean warming simulations were linearized and simplified, and the major impacts were related to the growth of the species. The impacts of ocean warming are more complex and extensive because of the complexity of the relationship between coral and temperature (Hughes et al., 2007). Coral bleaching and mortality were not considered in this study, which is another uncertainty in our study. This study was a preliminary attempt to understand the impact of ocean warming and fishing on the coral reef ecosystem of Xisha Islands. To make the study more comprehensive and representative, more environmental parameters, such as pH, dissolved oxygen, and net primary productivity, will be considered in future studies, and the fishing scenario will be revised according to existing fishing policies and trends in fishing effort.
5 Conclusions
We simulated the impacts of environmental stressors (ocean warming) and human activity (fishing) on the XICR ecosystem in the SCS. We identified four reliable ecosystem trends under the impacts of ocean warming and fishing. (1) In the XICR ecosystem, the grazing food chain had a higher energy source than the detrital food chain. (2) Ocean warming will seriously affect tropical reef ecosystems through biomass decline. (3) Fishing has a greater impact on the Xisha coral reef ecosystem than that of ocean warming. (4) The impact of ocean warming can be mitigated by reducing the fishing intensity.
Data availability statement
The original contributions presented in the study are included in the article/supplementary material. Further inquiries can be directed to the corresponding authors.
Author contributions
XZ: Conceptualization, methodology, software, writing. YL: Investigation, data curation. JD: Conceptualization, funding acquisition, methodology, writing, supervision. SQ: Investigation, data curation. BX: Investigation, data curation. WC: Investigation, data curation. JW: Investigation, data curation. WH: Formal analysis, data curation. ZW: Investigation, data curation. BC: Resources, data curation, supervision. All authors contributed to the article and approved the submitted version.
Funding
This work was financially supported by the National Natural Science Foundation of China (grant numbers 42176153 and 41676096), Xiamen Youth Innovation Fund (grant number 3502Z20206096).
Acknowledgments
We thank Lingyan Xu, Qian Peng, and Qifang Wang for the help in the process of this study, and we thank the two reviewers for their constructive comments which greatly improved this paper.
Conflict of interest
The authors declare that the research was conducted in the absence of any commercial or financial relationships that could be construed as a potential conflict of interest.
Publisher’s note
All claims expressed in this article are solely those of the authors and do not necessarily represent those of their affiliated organizations, or those of the publisher, the editors and the reviewers. Any product that may be evaluated in this article, or claim that may be made by its manufacturer, is not guaranteed or endorsed by the publisher.
References
Acosta G., Rodriguez F. A., Hernandez R. C., Arias J. E. (2013). Additive diversity partitioning of fish in a Caribbean coral reef undergoing shift transition. PloS One 8, 11. doi: 10.1371/journal.pone.0065665
Ainsworth C. H., Mumby P. J. (2015). Coral–algal phase shifts alter fish communities and reduce fisheries production. Global Change Biol. 21, 165–172. doi: 10.1111/gcb.12667
Allen G. R. (2008). Conservation hotspots of biodiversity and endemism for indo-pacific coral reef fishes. Aquat. Conservation-Marine Freshw. Ecosystems. 18, 541–556. doi: 10.1002/aqc.880
Arguelles-Jimenez J., Alva-Basurto J. C., Perea-Espana H., Zetina-rejon M. J., Arias-Gonzalez J. E. (2020). The measurement of ecosystem development in Caribbean coral reefs through topological indices. Ecol. Indicators 110, 11. doi: 10.1016/j.ecolind.2019.105866
Arguelles-Jimenez J., Rodriguez-Zaragoza F. A., Gonzalez-Gandara C., Alva-Basurto J. C., Arias-Gonzalez J. E., Hernandez-Landa R., et al. (2021). Functional developmental states of the greater Caribbean coral reefs. Ecol. Indicators 121, 107170. doi: 10.1016/j.ecolind.2019.105866
Arias-Gonzalez J. E., Nunez-Lara E., Gonzalez-Salas C., Galzin R (2004). Trophic models for investigation of fishing effect on coral reef ecosystems. Ecological Modelling. 172, 197–212. doi: 10.1016/j.ecolmodel.2003.09.007
Babcock R. C., Dambacher J. M., Morello E. B., Plaganyi E. E., Hayes K. R., Sweatman H. P. A., et al. (2016). Assessing different causes of crown-of-Thorns starfish outbreaks and appropriate responses for management on the great barrier reef. PloS One 11 (12), e0169048. doi: 10.1371/journal.pone.0169048
Bellwood D. R., Hughes T. P., Folke C., Nystrom M. (2004). Confronting the coral reef crisis. Nature. 429, 827–833. doi: 10.1038/NATURE02691
Bellwood D. R., Hughes T. P., Hoey A. S. (2006). Sleeping functional group drives coral-reef recovery. Curr. Biol. 16, 2434–2439. doi: 10.1016/j.cub.2006.10.030
Beyer H. L., Kennedy E. V., Beger M., ChenN C. A., Cinner J. E., Darling E. S., et al. (2018). Risk-sensitive planning for conserving coral reefs under rapid climate change. Conserv. Letters. 11, 10. doi: 10.1111/conl.12587
Blowes S. A., Supp S. R., Antao L. H., Bates A., Bruelheide H., Chase J. M., et al. (2019). The geography of biodiversity changes in marine and terrestrial assemblages. Science. 366, 339. doi: 10.1126/science.aaw1620
Brander K. M. (2007). Global fish production and climate change. Proc. Natl. Acad. Sci. U S A. 104, 19709–19714. doi: 10.1073/pnas.0702059104
Bruno J. F., Selig E. R. (2007). Regional decline of coral cover in the indo-pacific: Timing, extent, and subregional comparisons. PloS One 2(8), e711. doi: 10.1371/journal.pone.0000711
Bryndum A., Tittensor D. P., Blanchard J. L., Cheung W. W. L., Coll M., Galbraith E. D., et al. (2019). Twenty-first-century climate change impacts on marine animal biomass and ecosystem structure across ocean basins. Global Change Biol. 25, 459–472. doi: 10.1111/gcb.14512
Burke L. M., Reytar K., Spalding M., Perry A. L. (2011). Coral reefs at risk. Ethics Medics. 83 (12), 130. doi: 10.1029/EO083i012p00130-03
Capitani L., De Araujo J. N., Vieira E. A., Angelini R., Longo G. O. (2022). Ocean warming will reduce standing biomass in a tropical Western Atlantic reef ecosystem. Ecosystems. 25, 843–857. doi: 10.1007/s10021-021-00691-z
Chen B. H., Zhou Q. L., Yang S. Y. (2009). Impacts of climate changes on marine biodiversity. J. Oceanography Taiwan Strait. 28 (3), 437–444.
Cheung W. W. L., Watson R., Pauly D. (2013). Signature of ocean warming in global fisheries catch. Nature. 497, 365. doi: 10.1038/nature12156
Christensen V. (1995). ECOSYSTEM MATURITY - TOWARDS QUANTIFICATION. Ecol. Modelling. 77, 3–32. doi: 10.1016/0304-3800(93)E0073-C
Christensen V., Coll M., Piroddi C., Steenbeek J., Buszowski J., Pauly D. (2014). A century of fish biomass decline in the ocean. Mar. Ecol. Prog. Series. 512, 155–166. doi: 10.3354/meps10946
Christensen V., Pauly D. (1992). Ecopath II–a software for balancing steady-state ecosystem models and calculating network characteristics. Ecol. Model. 61 (3-4), 169–185. doi: 10.1016/0304-3800(92)90016-8
Christensen V., Walters C. J., Pauly D. (2005). Ecopath with ecosim: A user’s guide. fish. centre. univ. br. Columbia. Vancouver. 154, 31.
Christensen V., Walters C. J., Pauly D., Forrest R. (2008). Ecopath with ecosim version 6 user guide. Lenfest Ocean Futures Project. 235.
Colléter M., Valls A., Guitton J., Gascuel D., Pauly D., Christensen V. (2015). Global overview of the applications of the ecopath with ecosim modeling approach using the EcoBase models repository. Ecol. Modelling. 302, 42–53. doi: 10.1016/j.ecolmodel.2015.01.025
Dahlke F. T., Wohlrab S., Butzin M., Portner H. O. (2020). Thermal bottlenecks in the life cycle define climate vulnerability of fish. Science 369, 65. doi: 10.1126/science.aaz3658
David D., Degani G. (2011). Temperature affects brain and pituitary gene expression related to reproduction and growth in the Male blue gouramis, trichogaster trichopterus. J. Exp. Zoology Part a-Ecological Integr. Physiol. 315A, 203–214. doi: 10.1002/jez.663
Day P. B., Stuart R. D., Edgar G. J., Bates A. E. (2018). Species' thermal ranges predict changes in reef fish community structure during 8 years of extreme temperature variation. Diversity Distributions. 24, 1036–1046. doi: 10.1111/ddi.12753
DeCarlo T. M., Harrison H. B., Gajdzik L., Alaguarda D., Rodolfo-Metalpa R., D'Olivo J., et al. (2019). Acclimatization of massive reef-building corals to consecutive heatwaves. Proc. R. Soc. B-Biological Sci. 286, 7. doi: 10.1098/rspb.2019.0235
Dulvy N. K., Freckleton R. P., Polunin N. V. C. (2004). Coral reef cascades and the indirect effects of predator removal by exploitation. Ecol. Letters. 7, 410–416. doi: 10.1111/j.1461-0248.2004.00593.x
Du J. G., Makatipu P. C., Tao L. S. R., Pauly D., Cheung W. W. L., Peristiwady T., et al. (2020). Comparing trophic levels estimated from a tropical marine food web using an ecosystem model and stable isotopes. Estuar. Coast. Shelf Science. 233, 13. doi: 10.1016/j.ecss.2019.106518
Eddy T. D., Bernhardt J. R., Blanchard J. L., Cheung W. W. L., Colleter M., Pontavice H., et al. (2021). Energy flow through marine ecosystems: Confronting transfer efficiency. Trends Ecol. Evo. 36 (1), 76–86. doi: 10.1016/j.tree.2020.09.006
Fisher R., O'Leary R. A., Low-Choy S., Mengersen K., Knowlton N., Brainard R. E., et al. (2015). Species richness on coral reefs and the pursuit of convergent global estimates. Curr. Biol. 25, 500–505. doi: 10.1016/j.cub.2014.12.022
Freire K. M. F., Christensen V., Pauly D. (2008). Description of the East Brazil Large marine ecosystem using a trophic model. Scientia Marina. 72, 477–491.
Fulton E. A., Link J. S., Kaplan I. C., Savina-Rolland M., Johnson P., Ainsworth C., et al. (2011). Lessons in modeling and management of marine ecosystems: the Atlantis experience. Fish Fisheries. 12, 171–188. doi: 10.1111/j.1467-2979.2011.00412.x
Gibert J. P. (2019). Temperature directly and indirectly influences food web structure. Sci. Rep. 9, 5312. doi: 10.1038/s41598-019-41783-0
Hong X., Chen Z., Zhang J., Jiang Y., Gong Y., Cai Y., et al. (2021). Analysis of ecological carrying capacity of reefs organisms enhancement in qilanyu islands based on ecopath model. Trop. Oceanography. 41 (01), 15–27. doi: 10.11978/2020156(in Chinese with English abstract
Huang Z., Chen Z., Zeng X. (2009). Species composition and resources density of Chondrichthyes in the continental shelf of northern south China Sea. Ocean ographyin Taiwan Strait. 28 (1), 38–44. doi: 10.3969/j.issn.1000-8160.2009.01.007(in Chinese with English abstract
Huang H., Dong Z., Lian J. (2008). Establishment of nature reserve of coral reef ecosystem on the xisha islands. Trop. Geography. 28 (6), 540–544. doi: 10.3969/j.issn.1001-5221.2008.06.010(in Chinese with English abstract
Hu W., Chen B., Arnupap P., Zhang D., Liu X., Gu H., et al. (2020). Ecological vulnerability assessment of coral reef: A case study of Si Chang island, Thailand. Chin. J. Ecology. 39 (03), 979–989. doi: 10.13292/j.1000-4890.202003.029(in Chinese with English abstract
Hughes T. P., Baird A. H., Bellwood D. R., Card M., Connolly S. R., Folke C., et al. (2003). Climate change, human impacts, and the resilience of coral reefs. Science. 301, 929–933. doi: 10.1126/science.1085046
Hughes T. P., Barnes M. L., Bellwood D. R., Cinner J. E., Cumming G. S., Jackson J. B. C., et al. (2017). Coral reefs in the anthropocene. Nature. 546, 82–90. doi: 10.1038/nature22901
Hughes T. P., Rodrigues M. J., Bellwood D. R., Ceccarelli D., Hoegh O., Mccook L., et al. (2007). Phase shifts, herbivory, and the resilience of coral reefs to climate change. Curr. Biol. 17, 360–365. doi: 10.1016/j.cub.2006.12.049
Inagaki K. Y., Pennino M. G., Floeter S. R., Hay M. E., Longo G. O. (2020). Trophic interactions will expand geographically but be less intense as oceans warm. Global Change Biol. 26, 6805–6812. doi: 10.1111/gcb.15346
Jackson J. B. C., Kirby M. X., Berger W. H., Bjorndal K. A., Boysford L. W., Bourque B. J., et al. (2001). Historical overfishing and the recent collapse of coastal ecosystems. Science. 293, 629–638. doi: 10.1126/science.1059199
Kirby R. R., Beaugrand G., Lindley J. A. (2009). Synergistic effects of climate and fishing in a marine ecosystem. Ecosystems 12, 548–561. doi: 10.1007/s10021-009-9241-9
Kramer P. A. (2003). Synthesis of coral reef health indicators for the western Atlantic: Results of the AGRRA progra-2000). Atoll Res. Bul., 496, 1–58. doi: 10.5479/si.00775630.496-3.1
Lefcheck J. S., Edgar G. J., Stuart-Smith R. D., Bates A. E., Waldock C., Brandl S. J., et al. (2021). Species richness and identity both determine the biomass of global reef fish communities. Nat. Commun. 12 (1), 1. doi: 10.1038/s41467-021-27212-9
Legendre L., Niquil N. (2013). Large-Scale regional comparisons of ecosystem processes: Methods and approaches. J. Marine. Syst. 109, 4–21. doi: 10.1016/j.jmarsys.2011.11.021
Li Y. (2020). Biodiversity and biological characteristics of dominant fish species of typical reef fishes in south China sea (Shanghai, China: Shanghai Ocean University).
Li Y., Jia X., Chen G. (2007). Coral reef fish resources in the south China Sea (Beijing: Ocean Press).
Liu J. (2020). Response of coral reefs and islands in the south China sea to climate warming in the past 40 years recorded by remote sensing images (Guangxi, China: Guangxi University).
Liu X., Shi Q., Tao S., Yang H., Zhang X., Zhou S. (2021). The growth rate of coral porites at the zhongbei ansha of the zhongsha atoll and its response to seawater temperature change in the past 165 years. Trop. Oceanogr. 41(5), 64–73. doi: 10.11978/2021159
Li X., Wang Y. (2002). Comparison between benthos of nansha islands and xisha islands ,south china sea. Studia Marina Sinica. 44, 74–79.
Li Y., Wu Z., Liang J., Chen S., Zhao M. (2019). Analysis on the outbreak period and cause of acanthaster planci in xisha islands in recent 15 years. Sci. Bulletin. 64 (33), 3478–3484. doi: 10.1360/TB-2019-0152(in Chinese with English abstract
McClanahan T. R., Graham N. A. J., Darling E. S. (2014). Coral reefs in a crystal ball: predicting the future from the vulnerability of corals and reef fishes to multiple stressors. Curr. Opin. Environ. Sustainability. 7, 59–64. doi: 10.1016/j.cosust.2013.11.028
McOwen C. J., Cheung W. W. L., Rykaczewski R. R., Watson R. A., Wood L. J. (2015). Is fisheries production within Large marine ecosystems determined by bottom-up or top-down forcing? Fish Fisheries. 16, 623–632. doi: 10.1111/faf.12082
Milessi A. C., Danilo C., Laura R. G., Daniel C., Sellanes J., Rodriguze L. (2010). Trophic mass-balance model of a subtropical coastal lagoon, including a comparison with a stable isotope analysis of the food-web. Ecol. Modelling. 221, 2859–2869. doi: 10.1016/j.ecolmodel.2010.08.037
Miloslavich P., Klein E. (2005). Caribbean Marine biodiversity: the known and unknown Lancaster, PA: Des Tech Publishers Press
Odum E. P. (1969). The strategy of ecosystem development. Science. 164, 262–270. doi: 10.1126/science.164.3877.262
Pandolfi J. M., Bradbury R. H., Sala E., Hughes T. P., Bjorndal K. A., Cooke R. G., et al. (2003). Global trajectories of the long-term decline of coral reef ecosystems. Science. 301, 955–958. doi: 10.1126/science.1085706
Pilling G., Sheppard C., Davy S., Grahamet N. (2017). The biology of coral reefs (Second edition) (Oxford: Oxford University Press).
Polovina J. J. (1984). Model of a coral reef ecosystem i. the ECOPATH model and its application to French frigate shoals. Coral reefs. 3, 1–11. doi: 10.1007/BF00306135
Qiu S., Liu X., Chen B., Wang Y., Liao J., Du J. (2022). Reef fish diversity and community pattern of the xisha islands, south China Sea. Mar. Environ. Science. 41 (3), 395–401. doi: 10.13634/j.cnki.mes.2022.03.012(in Chinese with English abstract
Reaka-Kudla M. L. (1997). The global biodiversity of coral reefs: A comparison with rain forests (Washington, D.C: Joseph Henry Press).
Rocha G., Rossi-Wongtschowski C., Pires-Vanin A., Soares L. (2007). Trophic models of são sebastião channel and continental shelf systems, SE Brazil. Panam J. Aquat Sci. 2, 149–162.
Rummer J. L., Munday P. L. (2017). Climate change and the evolution of reef fishes: past and future. Fish Fisheries. 18, 22–39. doi: 10.1111/faf.12164
Shahjahan M., Islam M. J., Hossain M. T., Mishu M. A., Hasan J., Brown C. (2022). Blood biomarkers as diagnostic tools: An overview of climate-driven stress responses in fish. Sci. Total Environment. 843, 156910. doi: 10.1016/j.scitotenv.2022.156910
Shi X., Liu Y., Chen T., Yu K. (2008). The potential threats of global warming on corals living in the xisha islands. Trophical Geography. 04, 342–345, 368. doi: ckni:sun:rddd.0.2008-04-011(in Chinese with English abstract
Shin Y. J., Cury P. (2001). Exploring fish community dynamics through size-dependent trophic interactions using a spatialized individual-based model. Aquat. Living Resour. 14, 65–80. doi: 10.1016/S0990-7440(01)01106-8
Simth S. V. (1978). Coral-reef area and the contributions of reefs to processes and resources of the world's oceans. Nature. 273, 225–226. doi: 10.1038/273225a0
Spalding M., Ravilous C. R., Green E. P. (2001). World atlas of coral reefs; California: University of California Press.
Steenbeek J., Corrales X., Platts M., Coll M. (2018). Ecosampler: A new approach to assessing parameter uncertainty in ecopath with ecosim. Softwarex. 7, e40542 198–204. doi: 10.1016/j.softx.2018.06.004
Steneck R. S. (1988). Herbivory on coral reefs: A synthesis. Queensland: James Cook University Press.
Sumaila U. R., Cheung W., Dyck A., Gueye K., Huang L., Lam V., et al. (2012). Benefits of rebuilding global marine fisheries outweigh costs. PloS One 7 (7). doi: 10.1371/journal.pone.0040542
Sun D., Lin Z., Qiu Y., Wang X. (2005). Fish fauna of coral reef waters of the xisha islands. South China Fisheries Science. 1 (5), 18–25.
Teh L. S. L., Cashion T., Alava Saltos J. J., Cheung W. W. L., Sumaila U. R. (2019). Status, trends, and the future of fisheries in the East and south China seas. Fisheries Centre Res. Rep. 27 (1), 101.
Tesfamichael D., Pauly D. (2016). An exploration of ecosystem-based approaches for the management of red Sea fisheries. Springer Netherlands., 111–113. doi: 10.1007/978-94-017-7435-2_9
Volkoff H., Ronnestad I. (2020). Effects of temperature on feeding and digestive processes in fish. Temperature (Austin Tex.). 7, 307–320. doi: 10.1080/23328940.2020.1765950
Wabnitz C. C. C., Balazs G., Beavers S., Bjorndal K. A., Bolten A. B., Christensen V., et al. (2010). Ecosystem structure and processes at kaloko honokohau, focusing on the role of herbivores, including the green sea turtle chelonia mydas, in reef resilience. Mar. Ecol. Prog. Series. 420, 27–U392. doi: 10.3354/meps08846
Walters C., Christensen V., Pauly D. (1997). Structuring dynamic models of exploited ecosystems from trophic mass-balance assessments. Rev. Fish Biol. Fisheries. 7, 139–172. doi: 10.1023/A:1018479526149
Walters C., Pauly D., Christensen V., Kitchell J. F. (2000). Representing density dependent consequences of life history strategies in aquatic ecosystems: EcoSim II. Ecosystems. 3, 70–83. doi: 10.1007/s100210000011
Wang X., Du F., Lin Z., Sun D., Qiu Y., Huang S. (2011). Fish species diversity and community pattern in coral reefs of the xisha islands, south China Sea. Biodiversity Science. 19 (4), 463–469.
Wang T., Liu Y., Quan Q., Xioa Y., Wu P., Li C. (2022). Species composition characteristics analysis of qilianyu reef fishes of xisha islands. J. Fishery Sci. China 29 (1), 102–117. doi: 10.12264/JFSC2021-0122(in Chinese with English abstract
Williams G. J., Graham N. A. J. (2019). Rethinking coral reef functional futures. Funct. Ecology. 33, 942–947. doi: 10.1111/1365-2435.13374
World bank (2017). The sunken billions revisited: progress and challenges in global marine fisheries (Washington, D.C: WB Press).
Xu L., Song P., Wang Y., Xie B., Huang L., LI Y., et al. (2022). Estimating the impact of a seasonal fishing moratorium on the East China Sea ecosystem from 1997 to 2018. Front. Mar. Science. 9. doi: 10.3389/fmars.2022.865645
Yang W., Hu J., Lin B., Huang H., Liu M. (2018). Species diversity of coral reef fishes in zhaoshu island waters, xisha islands. J. Xiamen Univ. (Natural Science) 57 (6), 819–825. doi: 10.6043/j.issn.0438-0479,201807002(in Chinese with English abstract
Zeng Z., Cheung W. W. L., Li S. Y., Hu J. T., Wang Y. (2019). Effects of climate change and fishing on the pearl river estuary ecosystem and fisheries. Rev. Fish Biol. Fisheries. 29, 861–875. doi: 10.1007/s11160-019-09574-y
Zhang L., Takahashi D., Hartvig M., Andersen K. H. (2017). Food-web dynamics under climate change. Proc. R. Soc. B: Biol. Sci. 284, 20171772. doi: 10.1098/rspb.2017.1772
Zheng X., Zhang H., Chen B., Yu W. (2021). Advance of indicator system for the evaluation of coral reef restoration effectiveness. Appl. Oceanography. 40 (1), 126–139. doi: 10.3969/J
Zuo X., Su F., Wang Q., Wang C., Jiang H., Shi W. (2020). Thermal stress temporary refugia under global change for coral reefs in the south China Sea islands. Scientia Geographica Sinica. 40 (5), 814–822. doi: 10.13249/j.cnki.sgs.2020.05.016
Keywords: climate change, fishing effort, Xisha Islands coral reef ecosystem, food web simulations, Ecopath with Ecosim
Citation: Zhang X, Li Y, Du J, Qiu S, Xie B, Chen W, Wang J, Hu W, Wu Z and Chen B (2022) Effects of ocean warming and fishing on the coral reef ecosystem: A case study of Xisha Islands, South China Sea. Front. Mar. Sci. 9:1046106. doi: 10.3389/fmars.2022.1046106
Received: 16 September 2022; Accepted: 28 October 2022;
Published: 14 November 2022.
Edited by:
Juan D. Gaitan-Espitia, The University of Hong Kong, Hong Kong SAR, ChinaReviewed by:
Jimmy Argüelles, Universidad Veracruzana, MexicoStuart James Kininmonth, The University of Queensland, Australia
Copyright © 2022 Zhang, Li, Du, Qiu, Xie, Chen, Wang, Hu, Wu and Chen. This is an open-access article distributed under the terms of the Creative Commons Attribution License (CC BY). The use, distribution or reproduction in other forums is permitted, provided the original author(s) and the copyright owner(s) are credited and that the original publication in this journal is cited, in accordance with accepted academic practice. No use, distribution or reproduction is permitted which does not comply with these terms.
*Correspondence: Jianguo Du, ZHVqaWFuZ3VvQHRpby5vcmcuY24=; Bin Chen, Y2hlbmJpbkB0aW8ub3JnLmNu