- 1Guangdong Provincial Key Laboratory of Marine Disaster Prediction and Prevention, and Guangdong Provincial Key Laboratory of Marine Biotechnology, Shantou University, Shantou, China
- 2Southern Marine Science and Engineering Guangdong Laboratory (Guangzhou), Guangzhou, China
Pharmaceuticals and personal care products (PPCPs) are contaminants of emerging concern. PPCPs have been detected in various environmental matrices, posing potential threat to human health and environment quality. Thus far, there are no water quality guidelines (WQGs) established for PPCPs for the protection of freshwater organisms and ecosystems. In this study, we used the species sensitivity distribution (SSD) and assessment factor (AF) approaches to derive the 5% hazardous concentrations (HC5) of 35 selected PPCPs using acute and chronic toxicity data. The predicted no-effect concentrations (PNECs) and the acute-to-chronic ratios (ACRs) of chemicals were inherently computed to support the derivation of WQGs and for ecological risk assessment. Among these, endocrine-disrupting chemicals and antipsychotics were shown to pose a greater threat to the freshwater environment and organisms. The highest PNEC was recorded for chloramphenicol (3,620 μg/L) and the lowest for fluoxetine (0.0000291 μg/L), which could have significant ecological risks. In addition, the commonly used default ACRs do not seem to provide adequate support for the prediction of chronic toxicity thresholds and WQGs, as the highest ACRs of these drugs reached 39,100 (e.g., chloramphenicol). The findings of this study provide critical scientific information regarding the development of WQGs for environmental management and the risk control of PPCPs.
Highlights
● SSD and AF approaches were used for PNEC derivations
● PNECs were derived for 35 selected PPCPs in freshwater ecosystem
● Thirteen ACRs were computed for conservatism
● Probabilistic approaches can provide more profound protection for risk assessment
Introduction
Aquatic contamination by emerging pollutants has raised serious ecological concerns due to their negative impacts on the environment. The most commonly used emerging pollutants are pesticides, pharmaceutical and personal care products (PPCPs), fragrances, plasticizers, hormones, flame retardants, nanoparticles, perfluoroalkyl compounds, chlorinated paraffin, siloxanes, algal toxins, and various trace elements (Sauve and Desrosiers, 2014). PPCPs are classified among emerging pseudo-persistent organic pollutants because they are considered a major public health issue of global importance. The global consumption of PPCPs exceeds 10,000 t/year (Wilkinson et al., 2017), and a large number of these PPCPs have been detected in various environmental matrices, posing a potential threat to human and ecological health.
Pharmaceuticals include products commonly used to cure human and animal diseases. PPCPs comprise a new type of pollutants that have been receiving special attention in recent years. The extensive use of PPCPs in humans and animals resulted in their direct or indirect release as parent compounds through sewage discharge into the aquatic environment. Various compounds (e.g., paracetamol, ibuprofen, and oxazepam) associated with pharmaceuticals have been detected in freshwaters worldwide (Ebele et al., 2017). The reported occurrence of PPCPs in wastewater treatment plants (WWTPs) indicated that they cannot be completely removed by the wastewater treatment process; thus, in residual form, PPCPs are finally emptied into the aquatic environment (Dai et al., 2014; Sun et al., 2014). There are more than 5,000 substances related to pharmaceuticals that are synthesized and available for human and animal use; therefore, there is an urgent need to assess the potential hazard of PPCPs to aquatic organisms. However, the major concern related to pharmaceuticals is their long-term regular discharge, leading to their persistent contamination in aquatic environments that induces chronic effects on aquatic ecosystems (Wang et al., 2021a). Currently, the most common pharmaceuticals detected in freshwater are: non-steroidal anti-inflammatory drugs (NSAIDs), antibiotics, endocrine-disrupting chemicals (EDCs; e.g., hormones), β-receptor blockers, stimulants, and antipsychotics (Patel et al., 2020).
Various studies have reported the environmental impacts of these pharmaceuticals, particularly their toxicity to aquatic organisms such as Anabaena variabilis, Danio rerio, and Daphnia magna. It has been observed that these pharmaceuticals confer serious threats to aquatic ecology and induce adverse effects on aquatic organisms, including abnormal metabolism and survival (Wang et al., 2021a). For example, under long-term exposure, tetracycline affected the reproductive system of D. magna by disrupting the energy-related physiological function at a threshold concentration of 0.01 mg/L (Kim et al., 2014). Additionally, some of these pharmaceuticals can cause sublethal and long-term effects on aquatic organisms at very low concentrations. Likewise, the morphology of Catostomus commersoni was significantly affected after a 180-day exposure to ethinylestradiol at a concentration of 6.1 × 10−6 mg/L (Palace et al., 2009). However, although studies have shown the harmful effects of these drugs on the environment, at present, there are only a few corresponding water quality standards to effectively protect the environment (Peters et al., 2022). Therefore, deriving predicted no-effect concentration (PNEC) values for these drugs, developing water quality standards, and introducing corresponding management methods are crucial steps.
Large amounts of data on emerging contaminants are available on a global scale, including lethal and sublethal end points such as mortality, metabolism, growth, and development, which reflect the adverse effects of these pollutants on aquatic organisms in the laboratory. Specifically, the toxicity data of PPCPs could be used to derive the risk thresholds (e.g., PNEC for ecological risk assessment and ecosystem protection). However, the PNECs for aquatic organisms are generally utilized as the water quality guideline (WQG) values in risk assessment (Wang et al., 2014a). Estimation of the PNECs is mainly based on two methods: traditional assessment factor (AF) method and the probabilistic species sensitivity distribution (SSD) approach (Lei et al., 2010; Jin et al., 2012). In the derivation of PNECs, the acute-to-chronic ratio (ACR) can be applied if adequate chronic data are unavailable. However, for better development, it is necessary to establish the WQGs for these potentially harmful drugs. At present, multiple WQGs for emerging pollutants have been formulated worldwide, but only a few of them focused on PPCPs for the protection of freshwater organisms are available. Most of these WQGs are for drinking water and sewage plant discharge standards. Although some studies have been conducted, they are still far from meeting the urgent requirements of a sound environmental management of the emerging contaminants, such as PPCPs, across the world (Moermond and Smit, 2016). This study was conducted to develop PNECs for the frequently detected PPCPs in aquatic environment, which can support their environmental risk assessment and the derivation of sound WQGs. Briefly, the PNECs of 17 PPCPs were derived using the SSD method, while the those of the remaining 18 PPCPs were derived with the AF method. To obtain more robust values, both the acute and the chronic 5% hazardous concentration (HC5) were calculated using a probabilistic SSD method. Acute and chronic PNECs were derived based on HC5. This approach could support a comprehensive environmental risk assessment and the management of the target PPCPs and ACRs. Additionally, it can also provide a reference for the extrapolation of chronic toxicity data and for future PNEC derivation.
Methods
Data mining
The toxicity data of 35 PPCPs on freshwater species can be divided into five classes: NSAIDs, and antibiotics, EDCs (hormones), β-receptor blockers, and stimulants and antipsychotics. These data were obtained from the USEPA ECOTOX database (http://cfpub.epa.gov/ecotox/) and from peer-reviewed published literature (Supplementary Table S2). The toxicity data obtained from in vivo tests mainly focused on aquatic organisms, including algae, invertebrates, worms, crustaceans, insects, fish, amphibians, plants, and mollusks. The toxicity end points were as follows: accumulation, mortality, growth, biochemistry, physiology, injury, intoxication, morphology, hormone(s), enzyme(s), development, histology, behavior, genetics, reproduction, and population. The reliability, relevance, and the adequacy of all data were evaluated following Klimisch et al. (1997)). Toxicity data from different studies following standard testing guidelines recommended by international organizations were preferably chosen as data sources (e.g., OECD, 1992; Edition F., 2002; OECD, 2011). Toxicity data based on specific testing guidelines or closely related comparable operational parameters to the guideline methods were also incorporated. Values that were “greater than” or “less than” were considered not accurate and were excluded.
During the screening process, the toxicity data were divided into acute and chronic datasets. The classification criteria for the acute and chronic data followed those of Warne et al. (2018) based on the exposure duration and the standard toxicity test. For algae, an exposure duration of ≤1 or >1 day was considered as acute or chronic, respectively. For other species such as fish, invertebrates, worms, and crustaceans, an exposure duration of ≤4 or > 4 days was considered as acute or chronic, respectively. The preferred order of the statistical estimates of the toxicity data to calculate the guideline values are: no-effect concentration (NEC); effect/inhibition/lethal concentration (EC/IC/LCx), where x ≤ 10; bounded effect concentration (BEC10); EC/IC/LC15–20; and no observed effect concentration (NOEC). In cases where the EC/IC/LCx (where x ≤ 10), NEC, BEC10, EC/IC/LC15–20, and NOEC data are insufficient to derive a guideline value using the SSD method, the LC/IC/EC50, the lowest observed effect concentration (LOEC), and the maximum acceptable toxicant concentration (MATC) should be divided by 5, 2.5, and 2, respectively, in order to provide estimates of the NOEC/EC10 data (Warne et al., 2018). However, if multiple acute or chronic datasets for same compound are available, the geometric mean was used (Wang et al., 2014b).
Construction of species sensitivity distribution and calculation of hazardous concentrations
The methods used for SSD derivation were based on previous studies by Wang et al. (2014b) and Warne et al. (2018). To construct the SSD, each toxicity dataset should contain at least five species from three taxonomic groups (Warne et al., 2018). Briefly, the toxicity data were ranked in ascending order, and then percentiles were assigned based on the Weibull formula described in Eq. 1. The SSD curve for chemicals was fitted using the logarithmic value of toxicity concentration as the x-axis (independent variable) and the probability of the species being affected as the y-axis(dependent variable).
where i is ranked and assigned to the pharmaceutical based on the acute and chronic toxicity thresholds. For the same compound, the corresponding toxicity data of each species were obtained and the final results ranked from the lowest to the highest, with the lowest value of i being 1 and the largest being n, with n as the total number of data points. The percentage ranking served as the dependent variable in the probability distribution, whereas the concentrations were plotted as a function of log10 against their percentage ranking. The SSDs were constructed and fitted using a linear regression model for each distribution using SigmaPlot (version 14.0; Systat Software). HC5 was determined for each SSD using the parametric log-normal regression model. Corresponding 95% confidence intervals (95% CIs) were computed using Monte Carlo simulation (resampled 5,000 times). All computations were performed using the log-normal procedures available in the Statistical Analysis System package (SAS version 9.4, Cary, NC, USA).
Calculation of the predicted no-effect concentrations
The predicted no-effect concentrations were determined using the deterministic (AF) and probabilistic (SSD) approaches described in Eqs. 2 and 3, respectively. Subsequently, the pollutant concentration that results in observed effects in a predetermined percentage of the population can be derived by extrapolating the SSD curve. HC5, which is the pollutant concentration hazardous to 5% of the species, is recommended and commonly used in current PNEC derivations for conservancy around the globe.
The criteria for the selection of AFs under different methods and different toxicity end points were as follows: 1) for the SSD method, AFs of 5 and 1 were used for acute SSD and chronic SSD, respectively; 2) for the AF method, an AF of 10 was applied to the toxicity end points of NOEC/EC10/IC10/LC10, an AF of 100 was used when the end point was LOEC, and an AF of 1,000 was used when the end points were EC50/LC50/IC50 (REACH, 2008).
Calculations of the acute-to-chronic ratios
After obtaining the regression line for the SSDs, we obtained the HC5 values. ACRs were derived for each pharmaceutical by calculating the acute-to-chronic HC5 ratios (with 95% CIs) from the corresponding acute and chronic SSDs. The acute-to-chronic HC5 ratios and their 95% CIs for pairwise SSDs were calculated using the Monte Carlo simulation program (resampling 5,000 times; SAS version 9.4, Cary, NC, USA), and computation was performed using the log-normal model.
Results
Species sensitivity distributions and hazardous concentrations
After screening, 328 available toxicity data, including 180 acute and 148 chronic data, were obtained to construct the SSDs. A total of 108 species belonging to nine taxa were included in the analysis, which comprised 23 fish species, 31 algae species, 14 crustaceans, 15 invertebrates, 4 amphibians, 9 mollusks, 4 insects, 5 small plant species, and 3 species of worms. Majority of the toxicity datasets were focused on fish, algae, and crustaceans (Supplementary Table S2). Most of the species belonging to these taxa were used as model organisms in aquatic ecotoxicology and were included in standard testing methods. Generally, five toxicity datasets belonging to at least three taxa are required to build the SSD. In this study, for example, 12 acute and 9 chronic datasets were obtained for tetracycline, which belong to 7 and 5 taxa, respectively. Using Grubb’s or Tietjen–Moore tests, no outliers were identified among the datasets used for SSD construction. All datasets passed the Shapiro–Francia (normality of residuals; p > 0.05) and Anderson–Darling (p > 0.05) tests (Supplementary Table S3). The SSD curves for 17 drugs were constructed and demonstrated in Figures 1, 2, and the corresponding HC5 values with 95% CIs were computed using the log-normal model (Table 1). These HC5 values were divided by the different AFs to derive the corresponding PNEC for each PPCP. The HC5 values (and 95% CIs) were calculated for the antibiotics tetracyclines, quinolones, sulfonamides, macrolides, and chloramphenicols. The acute and chronic HC5 values for tetracyclines were 147 (95% CI = 13.2–392) and 3.49 μg/L (95% CI = 0.146–19.6), respectively, while those for oxytetracycline were 39.9 (95% CI = 28.7–54.4) and 1.32 μg/L (95% CI = 0.231–5.11), respectively. The 95% CIs of the other common pharmaceuticals, including the NSAIDs acetaminophen, diclofenac, and ibuprofen; the antipsychotics fluoxetine, carbamazepine, and diazepam; and the β-receptor blockers atenolol, metoprolol, and propranolol, were also calculated. The chronic HC5 values for fluoxetine and carbamazepine were estimated to be 0.0000291 (95% CI = 0.00000365–0.000113) and 0.00262 μg/L (95% CI = 0.000044–0.0188), respectively, which were much lower than those of others in this category. Among the hormones, only nonylphenol had sufficient toxicity data for the construction of the SSD curve. The acute and chronic HC5 values for nonylphenol were 0.154 (95% CI = 0.00654–0.713) and 0.0279 μg/L (95% CI = 0.000202–0.196), respectively.
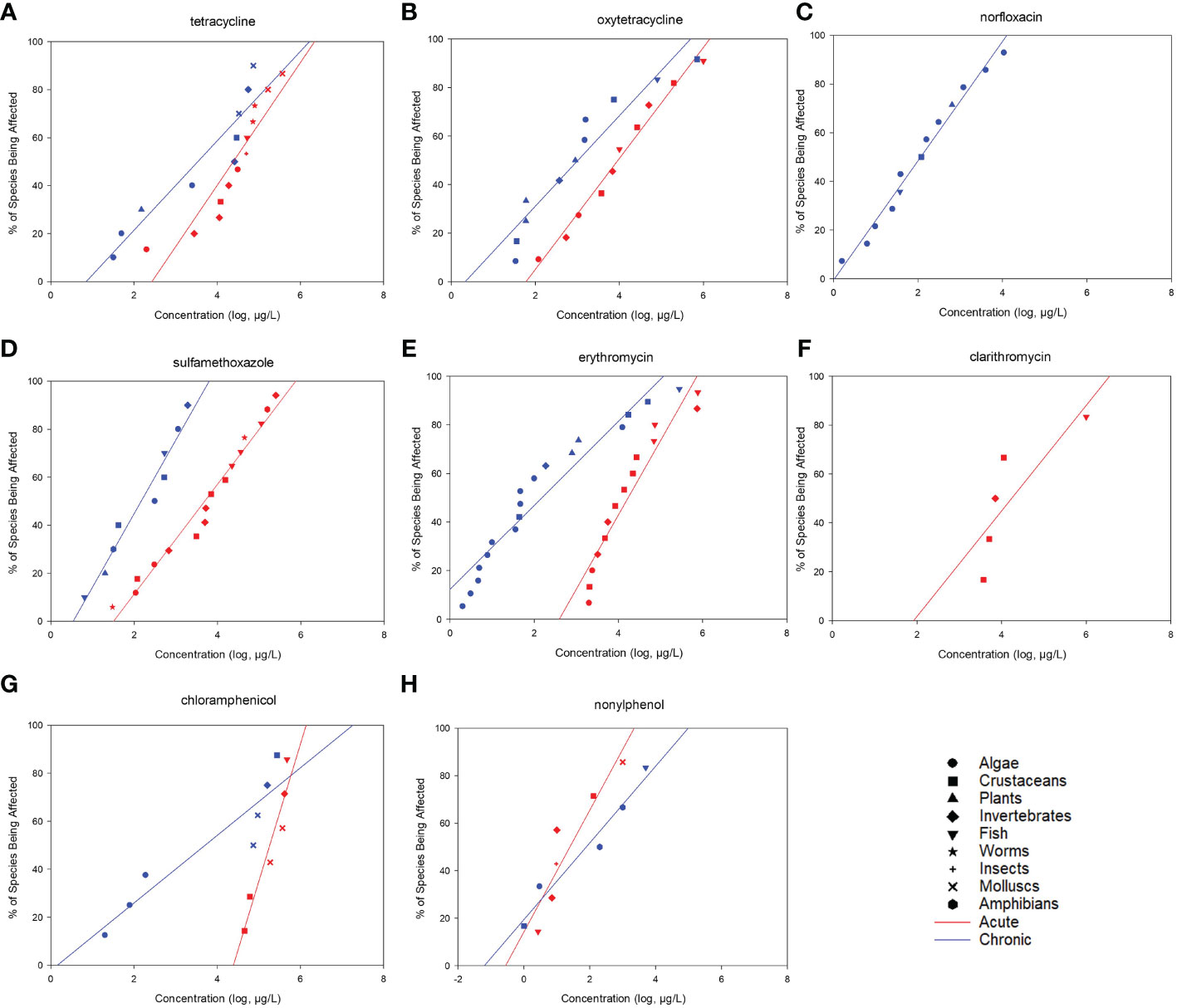
Figure 1 Freshwater acute (red) and chronic (blue) species sensitivity distributions (SSDs) for (A) tetracycline, (B) oxytetracycline, (C) norfloxacin, (D) sulfamethoxazole, (E) erythromycin, (F) clarithromycin, (G) chloramphenicol, (H) nonylphenol.
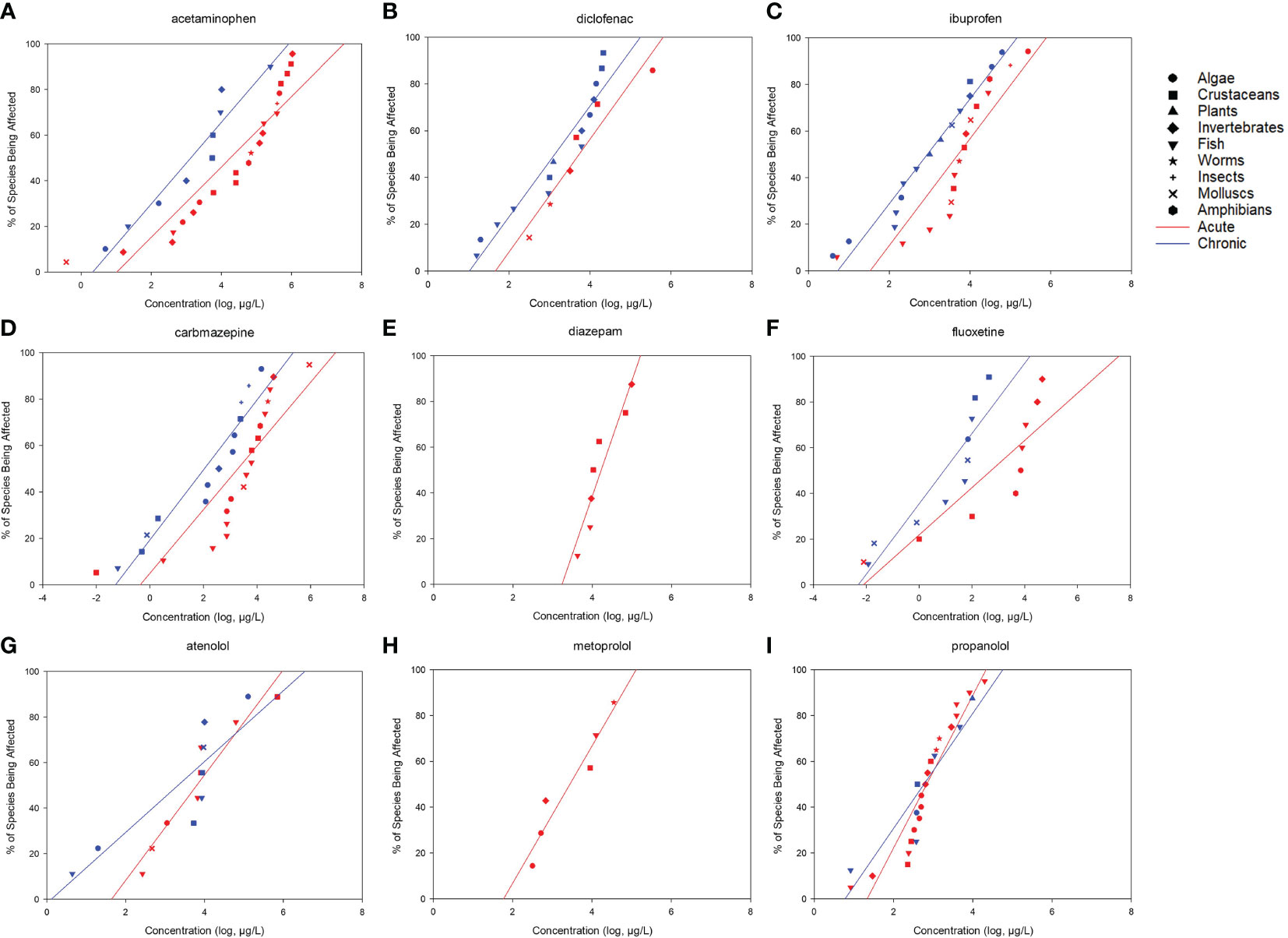
Figure 2 Freshwater acute (red) and chronic (blue) species sensitivity distributions (SSDs) for (A) acetaminophen, (B) diclofenac, (C) ibuprofen, (D) carbamazepine, (E) diazepam, (F) fluoxetine, (G) atenolol, (H) metoprolol, (I) propranolol.
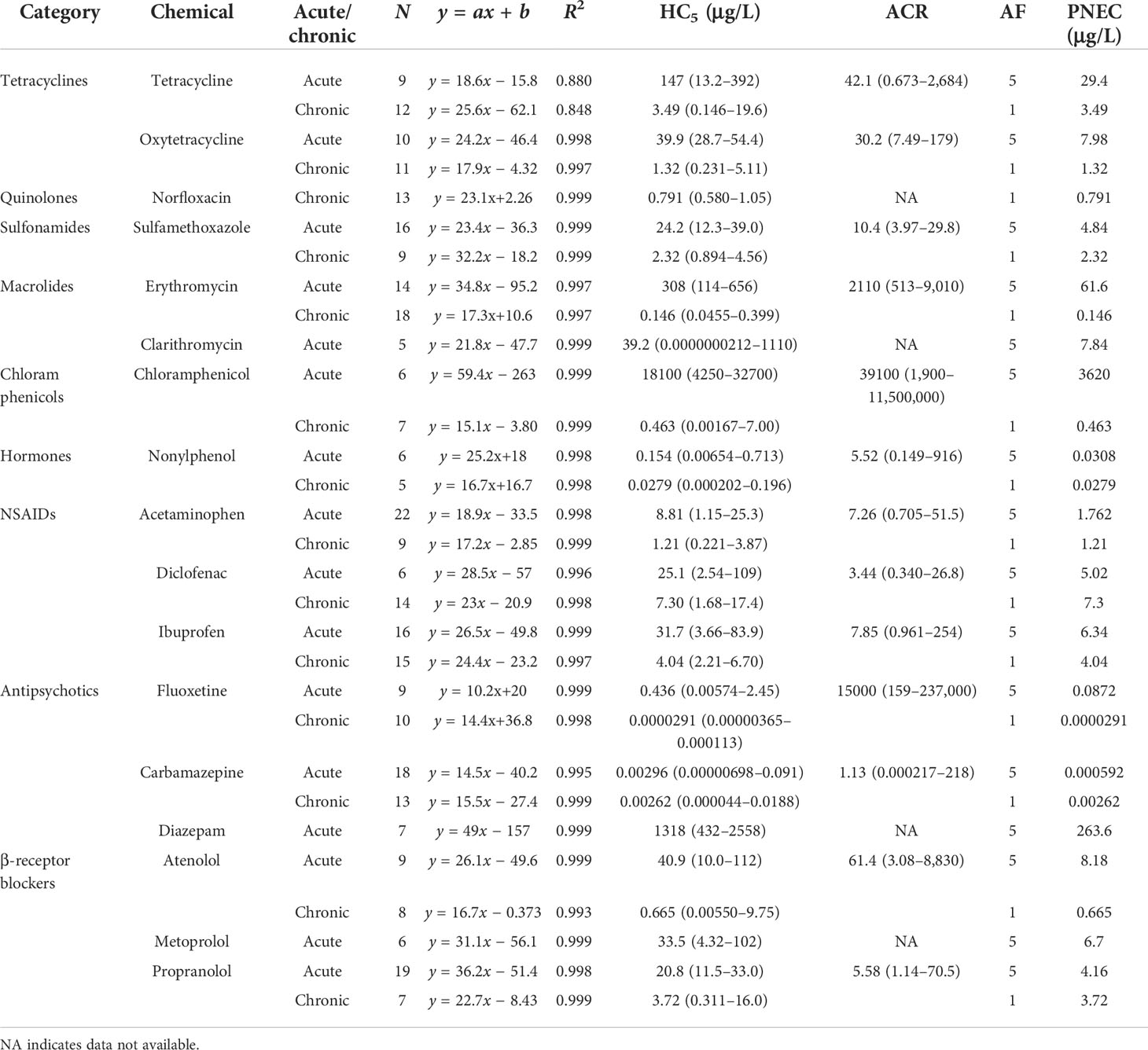
Table 1 Derived predicted no-effect concentrations (PNECs; in micrograms per liter) using species sensitivity distribution approaches and acute-to-chronic ratios (ACRs) extrapolated from corresponding acute and chronic 5% hazard concentration (HC5) ratios.
Predicted no-effect concentrations
The AF approach was used as a supplementary method to obtain the PNECs of the pharmaceuticals that did not meet the criteria for the construction of the SSDs. With this approach, 18 PNECs were obtained, with the data used to derive these PNEC containing 11 species in five major categories (Table 2). Of 18 drugs, 12 had toxicity data for algae and small aquatic plants such as Microcystis aeruginosa and Lemna gibba, while four drugs (estradiol, estrone, ethinylestradiol, and ketoprofen) had toxicity data only for fish. Algae and small plants are commonly used in toxicity tests since they are easy to test and are good respondents to these compounds, with adverse effects mainly on their population and reproduction. In contrast, most of the toxicity tests for the antibiotics on fish emphasized the toxic effects on their gene expression and enzyme activity; however, their ultimate effects on individuals remain uncertain.
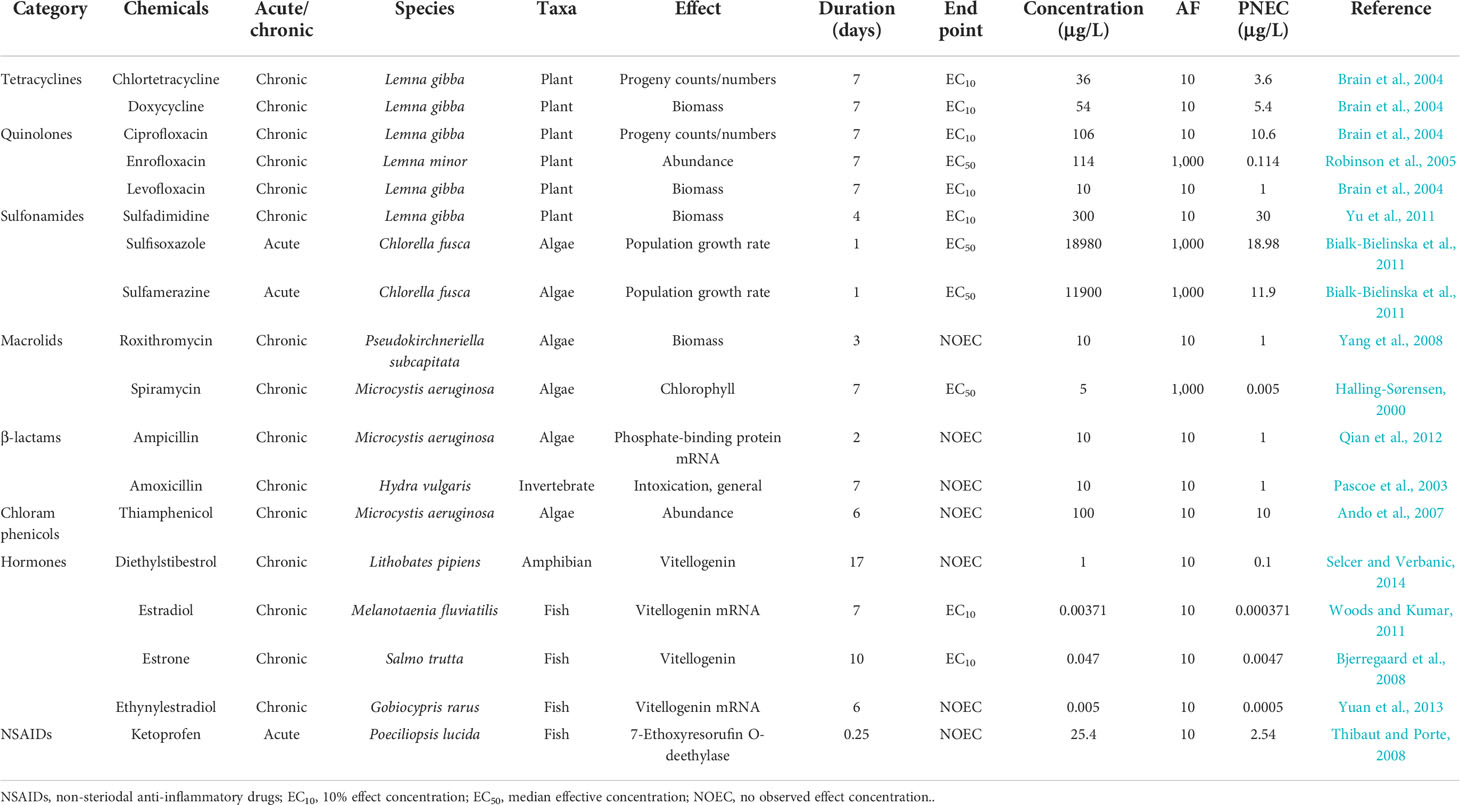
Table 2 Derived predicted no-effect concentrations (PNECs; in micrograms per liter) using the assessment factor (AF) approach.
Among the derived chronic PNECs (Tables 1, 2), the lowest value of 0.0000291 μg/L (using the SSD approach) was shown by fluoxetine, indicating that this antipsychotic might pose the highest risk potential to freshwater ecosystems. In contrast, sulfadimidine (a sulfonamide) had the biggest PNEC of 30 μg/L (using the AF approach), demonstrating its lowest risk potential to the ecosystem. The PNECs of the hormones were lower than those of pharmaceuticals such as diethylstilbestrol, estradiol, estrone, ethinylestradiol, and nonylphenol, with values of 0.1 (AF approach), 0.000371 (AF approach), 0.0047 (AF approach), 0.0005 (AF approach), and 0.0279 μg/L (SSD approach), respectively. Conversely, the tetracyclines and NSAIDs showed similar values to those of others in their class. The PNECs of chlortetracycline, doxycycline, tetracycline, and oxytetracycline were 3.6 (AF approach), 5.4 (AF approach), 3.49 (SSD approach), and 1.32 μg/L (SSD approach), respectively. The NSAIDs acetaminophen, diclofenac, ibuprofen, and ketoprofen had values of 1.21 (SSD approach), 7.3 (SSD approach), 4.04 (SSD approach), and 2.54 μg/L (AF approach), respectively. However, the PNECs of sulfonamides and sulfamethoxazole were 30 (AF approach) and 2.32 μg/L (SSD approach), respectively, which were a little higher than those of the other pharmaceuticals.
Acute-to-chronic ratios
The acute-to-chronic ratios based on the HC5 ratios with 95% CIs were calculated for those chemicals with corresponding acute and chronic SSDs. The ACRs and 95% CIs for 13 chemicals are presented in Table 1. The highest ACR of 39,100 was calculated for chloramphenicol (95% CI = 1,900–11,500,000), followed by the lowest at 1.13 for carbamazepine (95% CI = 0.000217–218). Those of erythromycin (ACR = 2110, 95% CI = 513–9,010) and fluoxetine (ACR = 15,000, 95% CI = 159–237,000) were much higher than the values of the other PPCPs. The ACRs of other normal pharmaceuticals ranged from 5.52 (95% CI = 0.149–916) for nonylphenol to 30.2 (95% CI = 7.49–179) for oxytetracycline. Generally, 10, 100, and 1,000 are used as default ACRs; however, according to our data-driven ACRs, the use of 10 as the default ACR might be underprotective for tetracycline, oxytetracycline, erythromycin, chloramphenicol, and fluoxetine. On the contrary, the use of 100 or 1,000 might lead to the overprotection of all PPCPs, except for erythromycin, chloramphenicol, and fluoxetine.
Discussion
In this study, all available chronic and acute toxicity data of 35 common PPCPs on freshwater species were collected from online databases and published literature. The high-quality results were mainly from the use of a single test species for either algae or fish, which are more representative model organisms and can reflect the toxic effects of a single chemical more accurately on numerous species. Therefore, considering the importance of a huge number of species in an ecosystem, toxicity tests on a single species do not provide sufficient information for the protection of the entire ecosystem. Thus, the range of test species should be expanded to improve the reliability and acceptability of the results. Species composition and the ecological status of the individual species vary in different ecosystems due to biodiversity, and priority should be given to numerous and more representative species. For example, in estuarine ecosystems, a number of benthic organisms such as sandworms can be used as a bioindicator of the pollution status (Zhang et al., 2021).
There is no uniform standard that specifies the end points used for toxicity testing. Most of the toxicity studies have used some of the traditional toxicity end points including mortality, development, and reproduction and other biomarkers such as enzyme activity and gene expression. However, these traditional toxicity end points may not be able to provide sufficient knowledge for ecosystem protection. It has been argued that the adverse effects may be observed at lower concentrations than those of the PNECs derived from traditional end points. Caldwell et al. (2008) reported that estrogen can cause the death of aquatic organisms at concentration levels of milligrams per liter; however, it could also lead to an irreversible toxic effect on the reproduction of vertebrates at levels of nanograms per liter. Therefore, more sensitive sublethal toxicity end points need to be determined. The study by Dang et al. (2011) using chronic toxicity tests of EDCs on fish showed that vitellogenin (VTG), fecundity, and gonad histology are the most sensitive end points for fathead minnow, medaka, and zebrafish in 21-day fish assays. In the context of such studies, more sensitive and appropriate toxicity end points should be selected for different drugs and test species. However, lethality and immobilization are the most commonly used end points in the toxicity tests of various toxicants. More sensitive parameters are still required to determine the sublethal acute effects. Despite some disadvantages, the feeding activity, respiration rate, and heart activity are still considered as valuable indicators of various stressors and may be used in ecotoxicological studies (Bownik, 2020). On the other hand, a single biomarker is only a small indicator of exposure, which makes linking the impacts at higher organization levels, e.g., life cycle traits or population dynamics, difficult (Monserrat et al., 2007). When predicting the responses of populations or ecosystems to toxic stress, reproductive outputs such as fecundity, gamete function, fertilization success, embryo development, or larval survivorship are more ecologically relevant to measure than adult physiological or biochemical changes. However, studies using reproductive end points are likely to be more informative of the higher-level effects as these changes can be linked directly to recruitment and are modeled to calculate the main demographic indices and other population statistics (Lewis and Watson, 2012; Wang et al., 2021b). We selected the most commonly used toxicity end points for use in this study.
In this study, the PNECs derived for the different drugs were significantly different from each other due to their different mechanisms of action. Among the PNECs obtained using the two different methods, those of the EDCs were much lower than the PNECs of the other classes of drugs. Estrone, estradiol, ethinylestradiol, nonylphenol, and diethylstilbestrol, as common EDCs, can act as substitutes for natural hormones by inhibiting or imitating their effects in humans and animals. Thus, they have the ability to regulate and feedback the hormone levels (Salehi et al., 2018). These characteristics have made it easy for them to interfere with the normal physiological activities of living organisms and induce adverse outcomes. In addition, the antipsychotic drugs carbamazepine, diazepam, and fluoxetine demonstrated relatively low PNECs (0.001, 0.0235, and 0.081 μg/L, respectively). Although these drugs potentially affect the human nervous system, they may not be exclusive to human targets. These drugs have also been found in other vertebrates and invertebrates due to the target receptors/molecules being similar and conserved during evolution (Fent et al., 2006). Several studies have shown that neuroactive compounds (antiepileptics and antidepressants) can modify the behavior of test organisms by causing hormonal dysfunction and neurotransmission disruption (Oggier et al., 2010). In fish, these compounds have also shown the ability to modify complex and ecologically relevant behaviors, including swimming performance, dark/light preference (scototaxis), thigmotaxis (i.e., tendency of animals to stay near the walls of an environment relative to the center), shoal cohesion, predator avoidance, feeding behavior, and growth (Gebauer et al., 2011; Riehl et al., 2011). In addition, they are usually present in the environment at very low concentrations of nanograms or micrograms per liter. For example, carbamazepine is commonly present in the aquatic environment, being one of the most frequently detected pharmaceuticals in drinking water (van den Brandhof and Montforts, 2010). It has also been found in surface waters near sewage treatment plant (STP) effluents at up to 0.65 μg/L (Metcalfe et al., 2003), which is much higher than the PNEC obtained in this study. Therefore, the ecological threat of these drugs is a major concern.
The PNECs for two other commonly used classes of drugs, i.e., NSAIDs and β-receptor blockers, were relatively high, but still are a threat. The NSAIDs diclofenac and ibuprofen have been prioritized as high-risk pharmaceuticals (Zhou et al., 2019). With growing hypertension and cardiopathy concerns worldwide, β-receptor blockers have been increasingly used and the most frequently detected PPCPs in the environment. The PNECs for antibiotics were generally higher, probably because they mainly target prokaryotes, but also showed low toxicity to eukaryotes. As demonstrated in Table 1, the lowest toxicity values of these antibiotics are overwhelmingly for algae and small plants. Although the antibiotics in freshwater ecosystems were of low concentrations, their continuous emission still poses a potential threat to the environment. Consequently, the long-term exposure of aquatic organisms to these substances can cause chronic and sublethal effects.
ACRs have been extensively used as extrapolation factors or uncertainty factors in ecological risk assessments to estimate the chronic toxicity thresholds of chemicals with absent or limited chronic toxicity. ACRs have been calculated typically for specific species and chemicals to reduce the uncertainty in extrapolating the chronic data from acute data. They are widely used in the US particularly for the derivation of the ambient water quality criteria (AWQC), as well as for water quality standards, screening values, and risk assessments in other countries (Barron and Wharton, 2005; Stephen, 2010). In most cases, the default ACR of 10 is commonly used for algae, invertebrates, and fish regardless of the species, chemical type, or mode of action (REACH, 2009). However, this situation can easily lead to uncertainties, such as underprotection or overprotection, in ecological risk assessment.
In this study, for ACR analysis, large numbers of species from different taxa were included, such as fish, algae, and invertebrates. Similar to our study, Raimondo et al. (2007) also computed the ACRs for multiple combinations of taxa, chemicals, and end points using linear regression with different model permutations and showed that the ACR for algae was consistently around 4 (ranging from 2.43 to 5.62). Therefore, they recommended using the ACR of 4 for algal toxins than the default value of 10. On the other hand, even for the same species, different drugs have different toxicity mechanisms and different mechanisms of action, more so for different species. Therefore, the toxicity end points for the final toxicity data obtained in this case are also very different, which is what needs to be considered when deriving the ACR. For example, Wang et al. (2020) classified the toxicity data of three fishes (Pimephales promelas, D. rerio, and Oryzias latipes) and one crustacean (D. magna) into four types according to their mechanisms of action and then calculated their ACRs. The results showed that, when considering the three conditions of toxicity end point, species, and mechanism of action—any one of them was different—the final ACRs obtained were significantly different. Therefore, the derivation of the ACR requires a combination of various aspects to be more reliable. The findings of this study also showed that the ACRs of different drugs varied greatly. The recommended default ACRs of 10, 100, and 1,000, which are commonly used in ecological risk assessments, may overlook different species, compounds, toxic effects, and test end points and are highly unrealistic and uncertain. However, the ACRs for individual chemicals derived by a data-driven method, as that in the present study, can reduce the uncertainty (e.g., overprotection or underprotection) caused by using default values. It may provide better and sufficient reference to establish the water quality standards of these pharmaceuticals.
Conclusion
In total, 35 PNECs for five common categories of PPCPs were derived using the SSD and AF methods. The EDCs and antipsychotics showed lower PNECs and are highly toxic to aquatic organisms; for instance, the PNEC of fluoxetine was only 0.0000291 μg/L. These PNECs can provide a theoretical basis and data reference for WQGs and for ecological risk assessment. On the other hand, 13 ACRs were derived from these PPCPs to reduce the uncertainty of using default values in risk assessment. For example, the ACR of chloramphenicol was 39,100 (95% CI = 1,900–11,500,000), which is much higher than the default value (ACR = 10, 100, or 1000). Both the current toxicity testing methods and the derivation of water quality benchmarks still have certain shortcomings. Most of the testing is based on a single compound, except in very few studies that focused on using the toxicity of two or more compounds. In contrast, in real environments, organisms are exposed to multiple compounds for long durations. Therefore, ecotoxicological studies dealing with multiple compounds and chronic toxicities should be prioritized in the future. In addition, the toxicity of compounds can also be affected by numerous environmental factors. To perform rapid toxicity testing with good results and to obtain toxicity test data, environmental factors are sufficient and more sensitive toxicity end points should be considered. This study provides critical scientific information regarding the ecological risks of long-term PPCP exposure and key insights for WQG development, risk assessment, and water quality control.
Data availability statement
The original contributions presented in the study are included in the article/Supplementary Material. Further inquiries can be directed to the corresponding author.
Author contributions
HF, XL: Investigation, methodology, writing—original draft. YW, SK, OO, YYW, and WL: Writing—review and editing. ZW: Conceptualization, supervision, funding acquisition, writing—review and editing. All authors contributed to the article and approved the submitted version.
Funding
This work was supported by the National Natural Science Foundation of China (42177264); 2020 Li Ka Shing Foundation Cross-Disciplinary Research Grant (2020LKSFG03E); Shantou University Scientific Research Foundation for Talents (NTF19044); and the Innovation and Entrepreneurship Project of Shantou (201112176541391).
Conflict of interest
The authors declare that the research was conducted in the absence of any commercial or financial relationships that could be construed as a potential conflict of interest.
Publisher’s note
All claims expressed in this article are solely those of the authors and do not necessarily represent those of their affiliated organizations, or those of the publisher, the editors and the reviewers. Any product that may be evaluated in this article, or claim that may be made by its manufacturer, is not guaranteed or endorsed by the publisher.
Supplementary material
The Supplementary Material for this article can be found online at: https://www.frontiersin.org/articles/10.3389/fmars.2022.1043792/full#supplementary-material
References
Ando T., Nagase H., Eguchi K., Hirooka T., Nakamura T., Miyamoto K., et al. (2007). A novel method using cyanobacteria for ecotoxicity test of veterinary antimicrobial agents. Environ. Toxicol. Chem. 26, 601–606. doi: 10.1897/06-195R.1
Barron M. G., Wharton S. R.. (2005). Survey of methodologies for developing media screening values for ecological risk assessment. Integr Environ Assess Manag 1, 320–332.
Bialk-Bielinska A., Stolte S., Arning J., Uebers U., Boschen A., Stepnowski P., et al. (2011). Ecotoxicity evaluation of selected sulfonamides. Chemosphere 85, 928–933. doi: 10.1016/j.chemosphere.2011.06.058
Bjerregaard P., Hansen P. R., Larsen K. J., Erratico C., Korsgaard B., Holbech H. (2008). Vitellogenin as a biomarker for estrogenic effects in brown trout, salmo trutta: laboratory and field investigations. Environ. Toxicol. Chem. 27, 2387–2396. doi: 10.1897/08-148.1
Bownik A. (2020). Physiological endpoints in daphnid acute toxicity tests. Sci. Total. Environ. 700, 134400. doi: 10.1016/j.scitotenv.2019.134400
Brain R. A., Johnson D. J., Richards S. M., Sanderson H., Sibley P. K., Solomon K. R. (2004). Effects of 25 pharmaceutical compounds to Lemna gibba using a seven-day static-renewal test. Environ. Toxicol. Chem. 23, 371–382. doi: 10.1897/02-576
Caldwell D. J., Mastrocco F., Hutchinson T. H., Lange R., Heijerick D., Janssen C., et al. (2008). Derivation of an aquatic predicted no-effect concentration for the synthetic hormone, 17 alpha-ethinyl estradiol. Environ. Sci. Technol. 42, 7046–7054. doi: 10.1021/es800633q
Dai G., Huang J., Chen W., Wang B., Yu G., Deng S. (2014). Major pharmaceuticals and personal care products (PPCPs) in wastewater treatment plant and receiving water in Beijing, China, and associated ecological risks. Bull. Environ. Contam. Toxicol. 92, 655–661. doi: 10.1007/s00128-014-1247-0
Dang Z., Li K., Yin H., Hakkert B., Vermeire T. (2011). Endpoint sensitivity in fish endocrine disruption assays: Regulatory implications. Toxicol. Lett. 202, 36–46. doi: 10.1016/j.toxlet.2011.01.016
Ebele A. J., Abou-Elwafa Abdallah M., Harrad S. (2017). Pharmaceuticals and personal care products (PPCPs) in the freshwater aquatic environment. Emerg. Contamin. 3, 1–16. doi: 10.1016/j.emcon.2016.12.004
Edition F (2002). Methods for measuring the acute toxicity of effluents and receiving waters to freshwater and marine organisms (Washington, DC, USA: US Environmental Protection Agency US EPA), p 1–266.
Fent K., Weston A. A., Caminada D. (2006). Ecotoxicology of human pharmaceuticals. Aquat. Toxicol. 76, 122–159. doi: 10.1016/j.aquatox.2005.09.009
Gebauer D. L., Pagnussat N., Piato A. L., Schaefer I. C., Bonan C. D., Lara D. R. (2011). Effects of anxiolytics in zebrafish: similarities and differences between benzodiazepines, buspirone and ethanol. Pharmacol. Biochem. Behav. 99, 480–486. doi: 10.1016/j.pbb.2011.04.021
Halling-Sørensen B. (2000). Algal toxicity of antibacterial agents used in intensive farming. Chemosphere 40, 731–739. doi: 10.1016/S0045-6535(99)00445-2
Jin X., Zha J., Xu Y., Giesy J. P., Richardson K. L., Wang Z. (2012). Derivation of predicted no effect concentrations (PNEC) for 2,4,6-trichlorophenol based on Chinese resident species. Chemosphere 86, 17–23. doi: 10.1016/j.chemosphere.2011.08.040
Kim H. Y., Yu S., Jeong T. Y., Kim S. D. (2014). Relationship between trans-generational effects of tetracycline on Daphnia magna at the physiological and whole organism level. Environ. pollut. 191, 111–118. doi: 10.1016/j.envpol.2014.04.022
Klimisch H. J., Andreae M., Tillmann U. (1997). A systematic approach for evaluating the quality of experimental toxicological and ecotoxicological data. Regul. Toxicol. Pharmacol. 25, 1–5. doi: 10.1006/rtph.1996.1076
Lei B. L., Huang S. B., Jin X. W., Wang Z. (2010). Deriving the aquatic predicted no-effect concentrations (PNECs) of three chlorophenols for the taihu lake, China. J. Environ. Sci. Health A. Tox. Hazard. Subst. Environ. Eng. 45, 1823–1831. doi: 10.1080/10934529.2010.520495
Lewis C., Watson G. J. (2012). Expanding the ecotoxicological toolbox: the inclusion of polychaete reproductive endpoints. Mar. Environ. Res. 75, 10–22. doi: 10.1016/j.marenvres.2011.08.002
Metcalfe C. D., Miao X. S., Koenig B. G., Struger J. (2003). Distribution of acidic and neutral drugs in surface waters near sewage treatment plants in the lower great lakes, Canada. Environ. Toxicol. Chem. 22, 2881–2889. doi: 10.1897/02-627
Moermond C. T., Smit C. E. (2016). Derivation of water quality standards for carbamazepine, metoprolol, and metformin and comparison with monitoring data. Environ. Toxicol. Chem. 35, 882–888. doi: 10.1002/etc.3178
Monserrat J. M., Martinez P. E., Geracitano L. A., Lund Amado L., Martinez Gaspar Martins C., Lopes Leaes Pinho G., et al. (2007). Pollution biomarkers in estuarine animals: critical review and new perspectives. Comp. Biochem. Physiol. C. Toxicol. Pharmacol. 146, 221–234. doi: 10.1016/j.cbpc.2006.08.012
OECD (1992). OECD guidelines for the testing of chemicals–fish, acute toxicity test. Organ. Econ. Coop. Dev., 1–9.
OECD (2011). OECD guidelines for the testing of chemicals–freshwater alga and cyanobacteria, growth inhibition test. Organ. Econ. Coop. Dev., 1–25.
Oggier D. M., Weisbrod C. J., Stoller A. M., Zenker A. K., Fent K. (2010). Effects of diazepam on gene expression and link to physiological effects in different life stages in zebrafish danio rerio. Environ. Sci. Technol. 44, 7685–7691. doi: 10.1021/es100980r
Palace V. P., MacLatchy D., Evans R. E., Wautier K. G., Mills K. H., Blanchfield P. J., et al. (2009). Interspecies differences in biochemical, histopathological, and population responses in four wild fish species exposed to ethynylestradiol added to a whole lakeThis paper is part of the series “Forty years of aquatic research at the experimental lakes area”. Can. J. Fisher. Aquat. Sci. 66, 1920–1935. doi: 10.1139/F09-125
Pascoe D., Karntanut W., Müller C. T. (2003). Do pharmaceuticals affect freshwater invertebrates? A. study. cnidarian. Hydra. vulgaris. Chemo. 51, 521–528.
Patel N., Khan M. Z. A., Shahane S., Rai D., Chauhan D., Kant C., et al. (2020). Emerging pollutants in aquatic environment: Source, effect, and challenges in biomonitoring and bioremediation- a review. Pollut 6, 99–113.
Peters A., Crane M., Merrington G., Ryan J. (2022). Environmental quality standards for diclofenac derived under the European water framework directive: 2. avian secondary poisoning. Environ. Sci. Europe. 34.
Qian H., Pan X., Chen J., Zhou D., Chen Z., Zhang L., et al. (2012). Analyses of gene expression and physiological changes in microcystis aeruginosa reveal the phytotoxicities of three environmental pollutants. Ecotoxicol 21, 847–859. doi: 10.1007/s10646-011-0845-4
Raimondo S., Montague B. J., Barron M. G. (2007). Determinants of variability in acute to chronic toxicity ratios for aquatic invertebrates and fish. Environ. Toxicol. Chem. 26, 2019–2023. doi: 10.1897/07-069R.1
REACH (2008). Guidance on information requirements and chemical safety assessment chapter R.10: Characterisation of dose-response for environment. ECHA version 2 Rev.:2.0.
REACH (2009). Guidance on information requirements and chemical safety assessment chapter R.15: Consumer exposure estimation. ECHA version 2 Rev.:2.0.
Riehl R., Kyzar E., Allain A., Green J., Hook M., Monnig L., et al. (2011). Behavioral and physiological effects of acute ketamine exposure in adult zebrafish. Neurotox. Teratol. 33, 658–667. doi: 10.1016/j.ntt.2011.05.011
Robinson A. A., Belden J. B., Lydy M. J. (2005). Toxicity of fluoroquinolone antibiotics to aquatic organisms. Environ. Toxicol. Chem. 24, 423–430. doi: 10.1897/04-210R.1
Salehi A. S. M., Yang S. O., Earl C. C., Shakalli Tang M. J., Porter Hunt J., Smith M. T., et al. (2018). Biosensing estrogenic endocrine disruptors in human blood and urine: A RAPID cell-free protein synthesis approach. Toxicol. Appl. Pharmacol. 345, 19–25. doi: 10.1016/j.taap.2018.02.016
Sauve S., Desrosiers M. (2014). A review of what is an emerging contaminant. Chem. Cent. J. 8, 15. doi: 10.1186/1752-153X-8-15
Selcer K. W., Verbanic J. D. (2014). Vitellogenin of the northern leopard frog (Rana pipiens): Development of an ELISA assay and evaluation of induction after immersion in xenobiotic estrogens. Chemosphere 112, 348–354. doi: 10.1016/j.chemosphere.2014.04.073
Stephen C. E., D. I. M., Hansen D. J., Gentile J. R., Chapman G. A., Brungs W. A. (2010). Guidelines for deriving numerical national water quality criteria for the protection of aquatic organisms and their uses. USEPA 277, PB85-227049–75.
Sun Q., Lv M., Hu A., Yang X., Yu C. P. (2014). Seasonal variation in the occurrence and removal of pharmaceuticals and personal care products in a wastewater treatment plant in xiamen, China. J. Hazard. Mater. 277, 69–75. doi: 10.1016/j.jhazmat.2013.11.056
Thibaut R., Porte C. (2008). Effects of fibrates, anti-inflammatory drugs and antidepressants in the fish hepatoma cell line PLHC-1: Cytotoxicity and interactions with cytochrome P450 1A. toxicol. In. Vitro 22, 1128–1135.
van den Brandhof E. J., Montforts M. (2010). Fish embryo toxicity of carbamazepine, diclofenac and metoprolol. Ecotoxicol. Environ. Saf. 73, 1862–1866.
Wang Z., Berninger J. P., You J., Brooks B. W. (2020). One uncertainty factor does not fit all: Identifying mode of action and species specific acute to chronic ratios for aquatic life. Environ. pollut. 262, 114262.
Wang Z., Kwok K. W., Lui G. C., Zhou G.-J., Lee J.-S., Lam M. H., et al. (2014b). The difference between temperate and tropical saltwater species’ acute sensitivity to chemicals is relatively small. Chemosphere 105, 31–43.
Wang Y. Y. L., Li P., Ohore O. E., Wang Y., Zhang D., Bai Y., et al. (2021b). Life stage and endpoint sensitivity differences of fathead minnow (Pimephales promelas) to chemicals with various modes of action. Environ. pollut. 290, 117995.
Wang Y., Wang J., Mu J., Wang Z., Yao Z., Lin Z. (2014a). Aquatic predicted no-effect concentration for three polycyclic aromatic hydrocarbons and probabilistic ecological risk assessment in liaodong bay of the bohai Sea, China. Environ. Sci. pollut. Res. Int. 21, 148–158.
Wang H., Xi H., Xu L., Jin M., Zhao W., Liu H. (2021a). Ecotoxicological effects, environmental fate and risks of pharmaceutical and personal care products in the water environment: A review. Sci. Total. Environ. 788, 147819.
Warne, Batley G., van Dam R., Chapman J., Fox D., Hickey C., Stauber J. (2018). Revised method for deriving Australian and new Zealand water quality guideline values for toxicants prepared for the revision of the Australian and new Zealand guidelines for fresh and marine water quality. department of science (Brisbane, Queensland: Information Technology and Innovation), p 1–p48.
Wilkinson J., Hooda P. S., Barker J., Barton S., Swinden J. (2017). Occurrence, fate and transformation of emerging contaminants in water: An overarching review of the field. Environ. Pollut. 231, 954–970.
Woods M., Kumar A. (2011). Vitellogenin induction by 17beta-estradiol and 17alpha-ethynylestradiol in Male Murray rainbowfish (Melanotaenia fluviatilis). Environ. Toxicol. Chem. 30, 2620–2627.
Yang L. H., Ying G. G., Su H. C., Stauber J. L., Adams M. S., Binet M. T. (2008). Growth-inhibiting effects of 12 antibacterial agents and their mixtures on the freshwater microalga pseudokirchneriella subcapitata. Environ. Toxicol. Chem. 27, 1201–1208.
Yuan C., Zhang Y., Hu G., Li M., Zheng Y., Gao J., et al. (2013). Expression of two zona pellucida genes is regulated by 17alpha-ethinylestradiol in adult rare minnow Gobiocypris rarus. Comp. Biochem. Physiol. C. Toxicol. Pharmacol. 158, 1–9.
Yu Z., Jiang L., Yin D. (2011). Behavior toxicity to Caenorhabditis elegans transferred to the progeny after exposure to sulfamethoxazole at environmentally relevant concentrations. J. Environ. Sci. 23, 294–300. doi: 10.1016/S1001-0742(10)60436-6
Zhang T., Liu K., Sun Y., Wang X., Fang T., Wu Y., et al. (2021). Effects of microplastics on physiological metabolism of Perinereis aibuhitensis. Asian J. Ecotoxicol. 16, 271–279.
Keywords: freshwater, pharmaceuticals and personal care products, predicted no-effect concentration, species sensitivity distribution, ecological risk assessment
Citation: Fan H, Wang Y, Liu X, Wang YYL, Kazmi SSUH, Ohore OE, Liu W and Wang Z (2022) Derivation of predicted no-effect concentrations for thirty-five pharmaceuticals and personal care products in freshwater ecosystem. Front. Mar. Sci. 9:1043792. doi: 10.3389/fmars.2022.1043792
Received: 14 September 2022; Accepted: 17 October 2022;
Published: 16 November 2022.
Edited by:
Qing Luo, Shenyang University, ChinaReviewed by:
Camilo Dias Seabra Pereira, Federal University of São Paulo, BrazilSara Long, RMIT University, Australia
Copyright © 2022 Fan, Wang, Liu, Wang, Kazmi, Ohore, Liu and Wang. This is an open-access article distributed under the terms of the Creative Commons Attribution License (CC BY). The use, distribution or reproduction in other forums is permitted, provided the original author(s) and the copyright owner(s) are credited and that the original publication in this journal is cited, in accordance with accepted academic practice. No use, distribution or reproduction is permitted which does not comply with these terms.
*Correspondence: Zhen Wang, zhenwang@stu.edu.cn
†These authors have contributed equally to this work