- 1School of Natural Sciences and Ryan Institute, University of Galway, Galway, Ireland
- 2Department of Chemistry, University of South Florida, Tampa, FL, United States
- 3School of Chemistry, University of Galway, Galway, Ireland
The extent to which any particular taxon supplies novel natural products depends on biological and evolutionary differences, and on decisions made by (or constraints on) biodiscovery scientists. The influences of different sources of variability on the biodiscovery process were examined in a study of the Cnidaria, a group recognised as an important source of novel marine natural products. The number of species with at least one novel metabolite within a genus was related to the number of species in the genus. This pattern implies that different genera do not differ in the probability of containing a species with novel natural products. Outlying points of this relationship were consistent with the ease of obtaining material through culturing organisms. The most productive five species were the sources of over 100 novel metabolites each. The distribution of novel metabolites across species showed no signs of exhausting novelty for the most productive source species. Novel metabolite drug likeness (ADMET-score) varied among genera. However, this pattern of variation was of the same degree as observed for molecular weights of metabolites, suggesting that differences among genera are generated by the decisions of analysts with different interests and do not reflect underlying biology. Biogeographic patterns of soft coral species with novel natural products were matched to regional species richness. Overall, the evidence for phylogenetic or spatial influences on the chance of finding novel metabolites was weak. The patterns are consistent with a constant chance of finding novel natural products across different species, with some constraints linked to ease of sampling or culturing and some reinforcement of biodiscovery in species that have previously been the source of novel metabolites.
1 Introduction
Marine species are important and continuing sources of chemical novelty with many realized and potential applications for marine natural products (Sigwart et al., 2021). Searching for new applications in the process of biodiscovery involves investments of time and effort. Ideally biodiscovery investments should be optimized, but this can be difficult when there are multiple potential targets and the pipeline involves a range of decisions including those taken for collection, choice of assays, and prioritization of extracts and fractions.
In considering which organisms to focus on for biodiscovery, there are essentially two alternative hypotheses: that potentially interesting molecules are randomly distributed across different species or that novelty is non-randomly distributed. The non-random hypothesis seems more likely, based on cases where species traits are associated with natural products, such as use of toxins (Kaas et al., 2012) or defensive chemicals (Lindquist et al., 2000). A non-random distribution of chemical novelty is supported when looking across marine invertebrate phyla: the average number of new natural products per species since 1990 was above 8 in Porifera and Cnidaria and below 5.4 in Mollusca, Echinodermata and Chordata (with other phyla being the source of few natural products Leal et al., 2012). Consequentially, sponges and cnidarians are repeatedly reported as among the most important invertebrate sources of marine natural products (Carroll et al., 2022).
Previous work on taxonomic associations with marine natural products has tended to collate information at higher levels such as phyla, subclass or order (although see details on families and selected genera in Leal et al. (2012); Leal et al. (2013) and Calado et al. (2022)). While analyses at genus or species level are rarer, these may provide more insight into the biological roles of natural products or improve the understanding of associations between natural products and species-specific traits. Alongside the number of natural products across genera, it is possible that the type of natural products varies: that different genera are the source of natural products with particular properties.
In addition to the distribution of natural products across phylogenies, there may also be biogeographic variation in chemical novelty. Such variation could simply be a passive reflection of species numbers, such that the number of natural products is positively associated with regional species richness (Leal et al., 2013; Calado et al., 2022). When the same number of species was assayed, different marine locations in Australia did not vary in assay activity, supporting the hypothesis that bioactivity per species is not higher in more diverse locations (Evans-Illidge et al., 2013). The discovery of spatial variation in chemical novelty may also be influenced by the distribution of funding for relevant programmes and other access constraints on research activity (Leal et al., 2012).
In the current study, we extend the taxonomic resolution for analysing patterns of natural products in the Cnidaria, the second largest invertebrate source phylum for marine natural products (Carroll et al., 2022). We tested the hypothesis that the number of species with a novel natural product is related to the number of species in a genus. Furthermore, the distributions of natural product molecular weight and a drug-likeness score were examined to evaluate whether different genera are likely to provide compounds with different properties. The relative distribution of novel compounds by species was also examined to further understand the biodiscovery process. With respect to biogeographic variation, we examined the global distribution for the proportion of recorded species having at least one MarinLit compound. Of particular interest is the order Alcyonacea, which dominates as a source of compounds in the MarinLit database of natural products. If there are no biogeographic effects, the proportion of alcyonacean species with a MarinLit compound should be constant.
2 Methods
The marine natural product (NP) database MarinLit (http://pubs.rsc.org/marinlit) was used to summarize the distribution of novel compounds across higher taxonomic levels (classes and orders) in Cnidaria (accessed 14/07/2022). MarinLit does not necessarily distinguish between metabolites associated with a species or one of its symbionts. For example, a group of diterpenoid acids, the talascortenes, are associated with a fungus Meng et al. (2020) but are listed under the host anemone in MarinLit. There are also some cases of out of date taxonomy in MarinLit. To avoid taxonomic confusion in analyses of species and genera, we used an recently compiled dataset (accessed December 2018, described in Laguionie-Marchais et al., 2022) where the taxonomy of the associated species had been checked using WoRMS (WoRMS Editorial Board, 2022), and with metabolites known to be from viral, microbial or bacterial symbionts removed from the dataset.
The hypothesis of a constant proportion of species with novel natural products (i.e. a random distribution of marine natural products) was tested using a regression of number of species against genus size. In the context of a constant discovery rate, a larger genus represents more species and therefore more chances to find a natural product. The expected relationship for a constant discovery rate is a straight line passing through the origin. Deviations from a constant rate could reflect analytical choices and related processes in the lab and/or biological variation among species.
When examining the number of compounds listed per species, a relevant null hypothesis is that the discovery of natural products is a random process occurring independently. This can be examined by comparing the number of novel compounds in different species. An alternative to randomness is that both species-specific biology and choices made during the biodiscovery processes may influence the pattern of discovery within species. For example, if analysts concentrated on under-explored species, this might produce a more even distribution of novel compounds per species. Conversely, familiarity with species, accessibility of samples, and other factors, including variations in metabolites, may cause the distribution of novel compounds to be heavily skewed toward certain species. In the context of possible declines in discovery rate (Calado et al., 2022), any flattening of the curve of compounds per species at the most productive tail of the novel compounds by species distribution might suggest that the limits of novelty within species are bring reached. Species-specific natural product distributions can be summarized with plots of the frequency of novel compounds by species rank. These types of plots are comparable to species abundance distributions common in ecological studies (Whittaker plots, e.g., Ulrich et al., 2010). The best descriptor for the observed distribution of MarinLit compounds was compared using a series of curves proposed for Whittaker plots: the null model (compounds randomly distributed across species), geometric series, lognormal, Zipf and Zipf-Mandelbrot distributions. The best fitting model was chosen by the lowest residual deviance and AIC with the radfit function of the vegan package in R (Oksanen et al., 2020). Models in radfit are based on Poisson error distributions (MarinLit compounds are counts). Conventional summaries of fit like r2 are not appropriate for Poisson models; a measure based on deviance residuals is reported instead (rD2, Cameron and Windmeijer, 1996).
The properties of MarinLit compounds found in different genera were compared using molecular weight and the ADMET-score, a drug-likeness measure based on 18 properties (e.g., carcinogenicity, oral toxicity and intestinal absorption) predicted from structure–activity relationships (Guan et al., 2019). ADMET-scores range between 0 (not favourable) and 1 (favourable), and are able to distinguish between approved and withdrawn drugs (Guan et al., 2019). Admet properties for each compound were generated using the web server admetSAR 2.0 http://lmmd.ecust.edu.cn/admetsar2/ (Cheng et al., 2012; Yang et al., 2018). The final ADMET‐score is the average of the scores for each property, weighted for the accuracy of the prediction, the clinical importance of the property, and the proportion of a set of reference drugs with the property (Guan et al., 2019). The ADMET-scores in the current study are the same data as used in Laguionie‐Marchais et al., 2022). A metabolite’s ADMET-score can be used to help screen molecules that are likely to fail during later stages of drug development and Cnidaria ADMET-scores correlate with other measures of drug-likeness, including a multivariate distance to drug-like chemical space, the relative drug likelihood (Yusof and Segall, 2013) and the number of exceptions to the rule of 5 (Laguionie‐Marchais et al., 2022). Molecular weight was used to estimate the role of chance in genus-level variability of observed properties. It seems very unlikely that differences in the molecular weight of metabolites reflect real differences in the mean sizes of compounds among genera. Differences in molecular weight of MarinLit compounds will reflect the choices of analysts, extraction protocols and other processes in the biodiscovery process. Hence, the test of distinct properties among genera is not based just on ADMET-score results. The patterns for ADMET-scores should be more striking than those for molecular weights to infer that any biological patterns are stronger than those generated by elements of the biodiscovery process subject to analytical choices.
Comparisons of mean properties among genera may not be independent, as some genera are more closely related (e.g., in same family) than others. These evolutionary relationships disrupt the assumed independence of observations in statistical models. Tests of the genus effect on metabolite properties were therefore caried out using phylogenetic generalized linear models (Freckleton et al., 2002). This approach includes a covariance matrix that models the dependence between observations associated with phylogeny. Branch lengths in the phylogeny are multiplied by a constant, Λ, which provides a weighting for the strength of the covariance, with the optimum value found using maximum likelihood. As Λ approaches 0, the observations approach statistical independence. Phylogenetic generalized linear models were fit to the molecular weight and ADMET-score data using the pgls function of the caper package in R (Orme et al., 2018). Initial branch lengths were set to 1 for each level of the hierarchy: class, subclass, order, family and genus as no complete set of branch lengths from molecular data is available.
Alcyonacea represent the cnidarian order with the largest number of MarinLit compounds (Leal et al., 2012). This group was used to examine the biogeographic pattern of discovery in more detail, using the relationship between species in an area and number of those species with at least one MarinLit compound. Bins of 3° x 3° were used for global coverage. Latitude and longitude do not produce equal-area samples, but hypotheses about the relative number of species with MarinLit compounds are not affected by this. The estimated global biodiversity patterns were derived from species records in the Global Biodiversity Information Facility, GBIF.org (accessed 31/11/2021 with data DOI https://doi.org/10.15468/dl.edsukx).
3 Results
The orders of Cnidaria have uneven proportions of MarinLit compounds (Table 1). A few orders of Anthozoa that contain fewer than 100 species have no records for natural products (Penicillaria, Spirularia, Corallimorpharia and Helioporacea). Other cnidarian orders have no or relatively few MarinLit compounds per species (Cubozoa, Hydrozoa, Myxozoa, Scyphozoa and Staurozoa). The Alcyonacea class (soft corals) dominate as a source of natural products. Scleractinia (stony corals) has the second largest number of MarinLit compounds, but this represents less than a tenth of the number of metabolites per species that Alcyonacea represents.
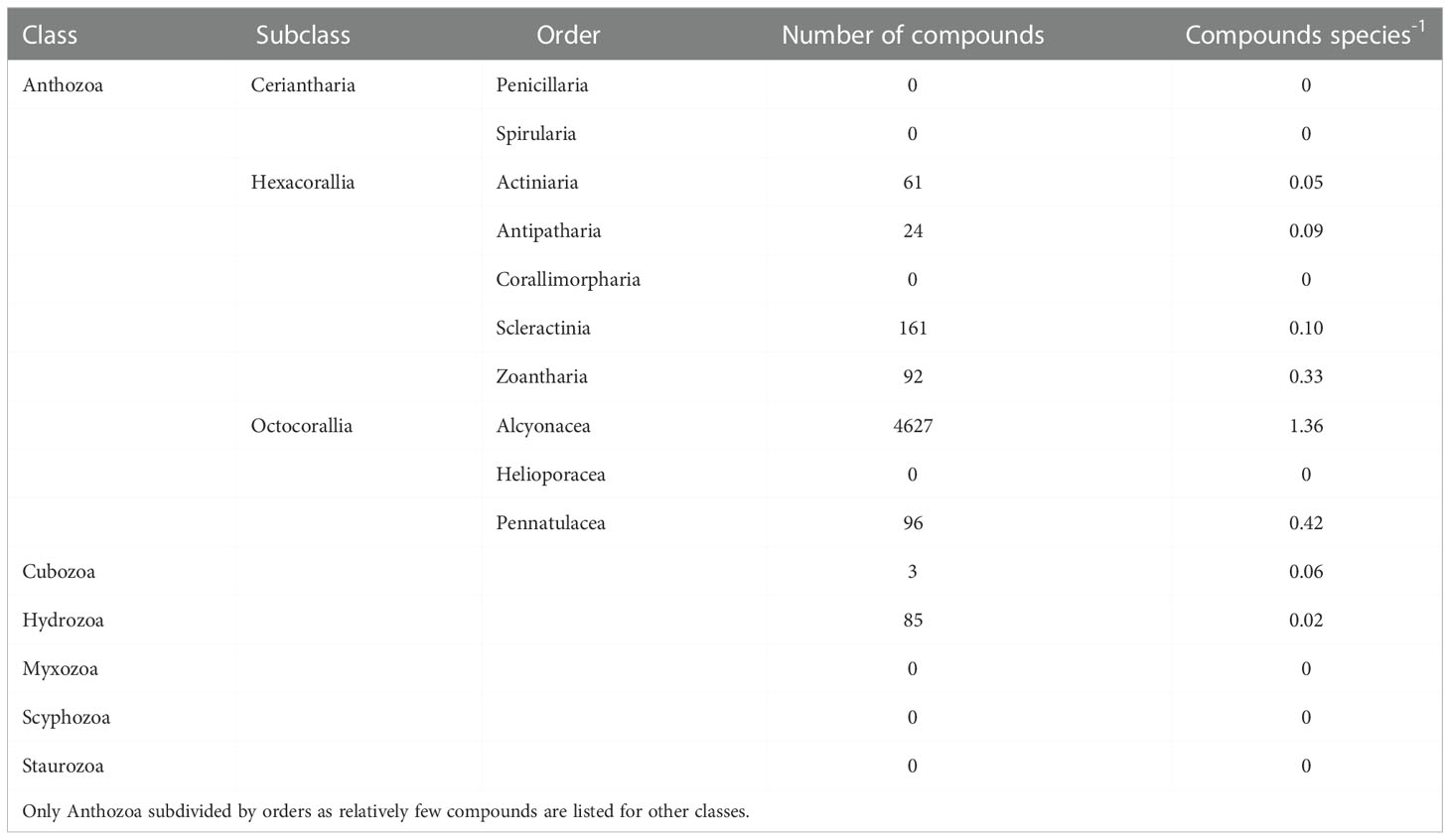
Table 1 Distribution of novel marine natural products recorded in the MarinLit database across cnidarian classes.
The number of species with at least one MarinLit compound was related to the size of the genus, consistent with a constant rate per species discovery among genera (Figure 1, slope = 0.095, p < 0.05, r2 39.5%, F1,365 = 238.3). The outliers on the right hand side of Figure 1 are Sinularia (above fitted line) and Dendronephthya (below fitted line). These are both conspicuous soft coral genera.
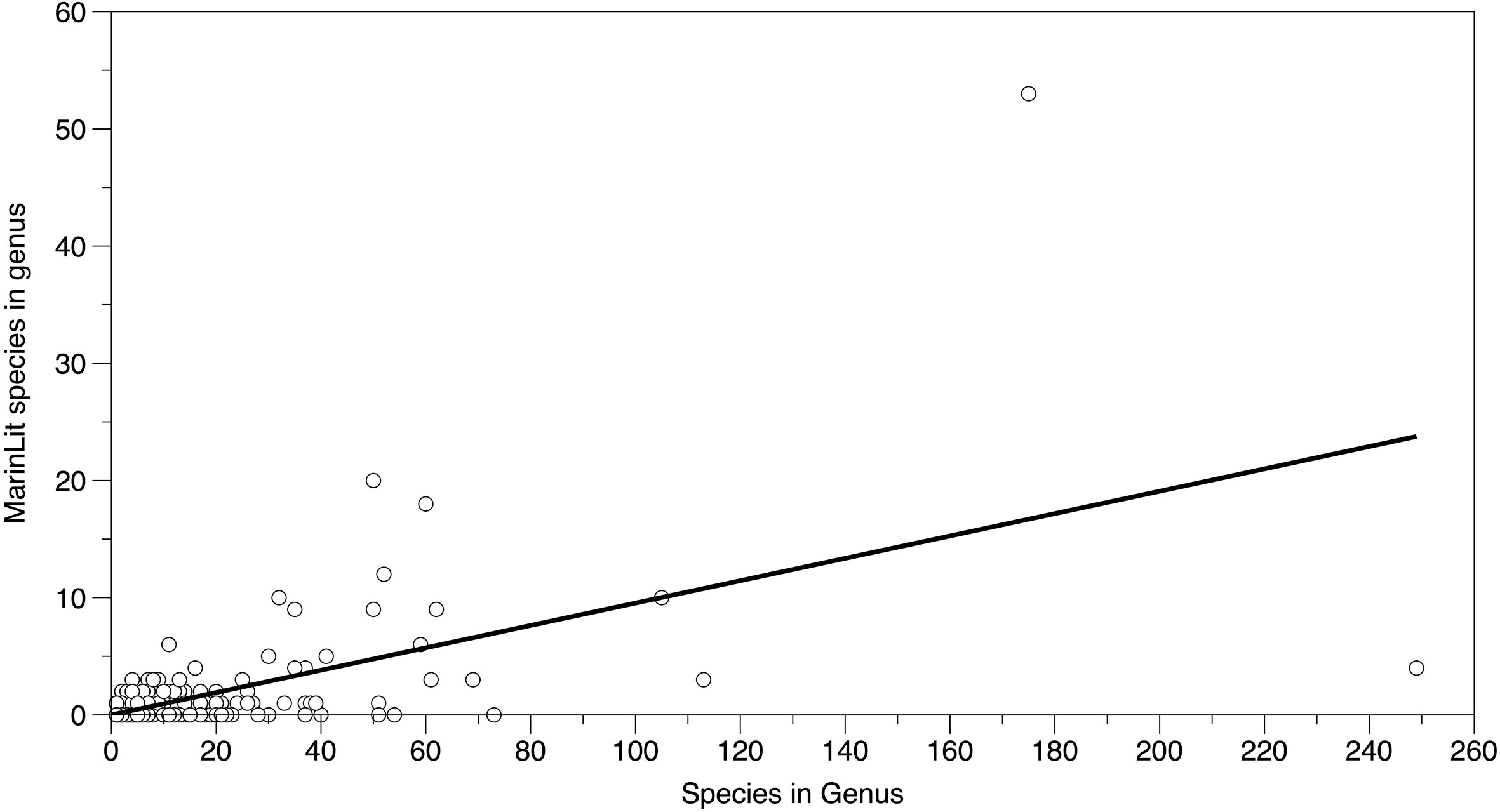
Figure 1 Number of species with at least one MarinLit compound recorded in different cnidarian genera. Fitted line is a regression passing through the origin (r2 39.5%).
The number of compounds per species was strongly skewed (Figure 2). Just considering species with at least one MarinLit entry, there was an average of 12.8 natural products per species. However, five species were each the source of over 100 natural products in MarinLit (Briareum stechei, Clavularia viridis, Briareum asbestinum, Sarcophyton glaucum, Antillogorgia elisabethae). There was no indication of a saturation or flattening of the discovery curve with species that have been the source of many compounds. There was also no flattening of the curve around the mean compounds per species as would be expected under a purely random process. Overall, a Zipf-Mandelbrot relationship described the observed distribution better than alternative models (AIC 1239.4, rD2 99%).
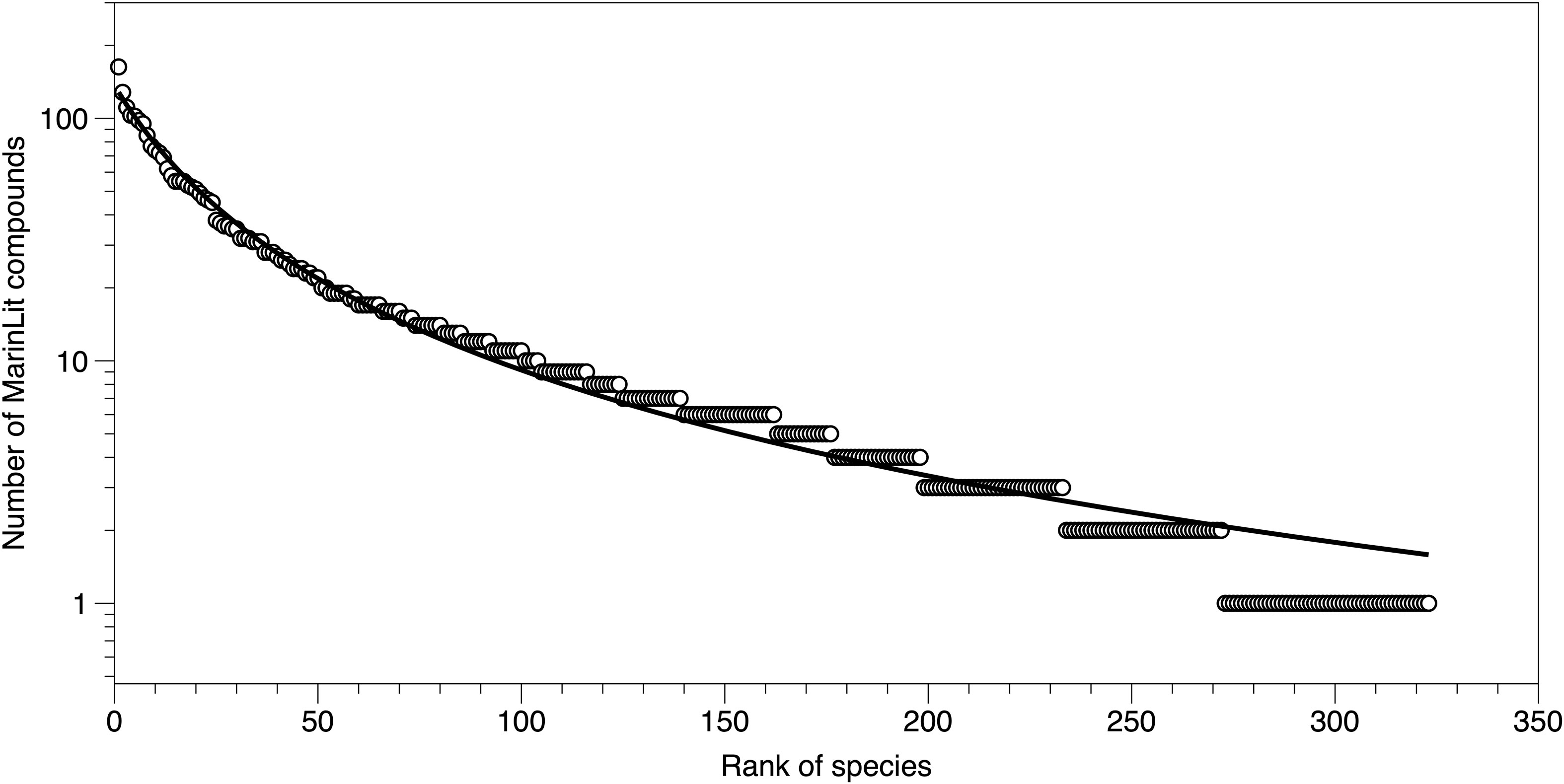
Figure 2 Number of compounds in different cnidarian species ranked from high count to low count species. The fitted line is a Zipf-Mandelbrot relationship (rD 99%), which described the pattern more closely than alternative statistical models.
Mean metabolite properties differed between genera (Figure 3). Genus explained just under a fifth (r2 19%, F97,225 = 1.795, p < 0.05) of the variability in the drug-likeness measure (ADMET-score). In contrast, genus was associated with one quarter of the variability in molecular weight of metabolites described in different genera (r2 24.9%, F97,225 = 2.101, p < 0.05). In both cases, the maximum likelihood estimate of the taxonomic covariance (λ) was 0, indicating no additional influences on molecular weight or ADMET-score associated with family, order or subclass taxonomic level.
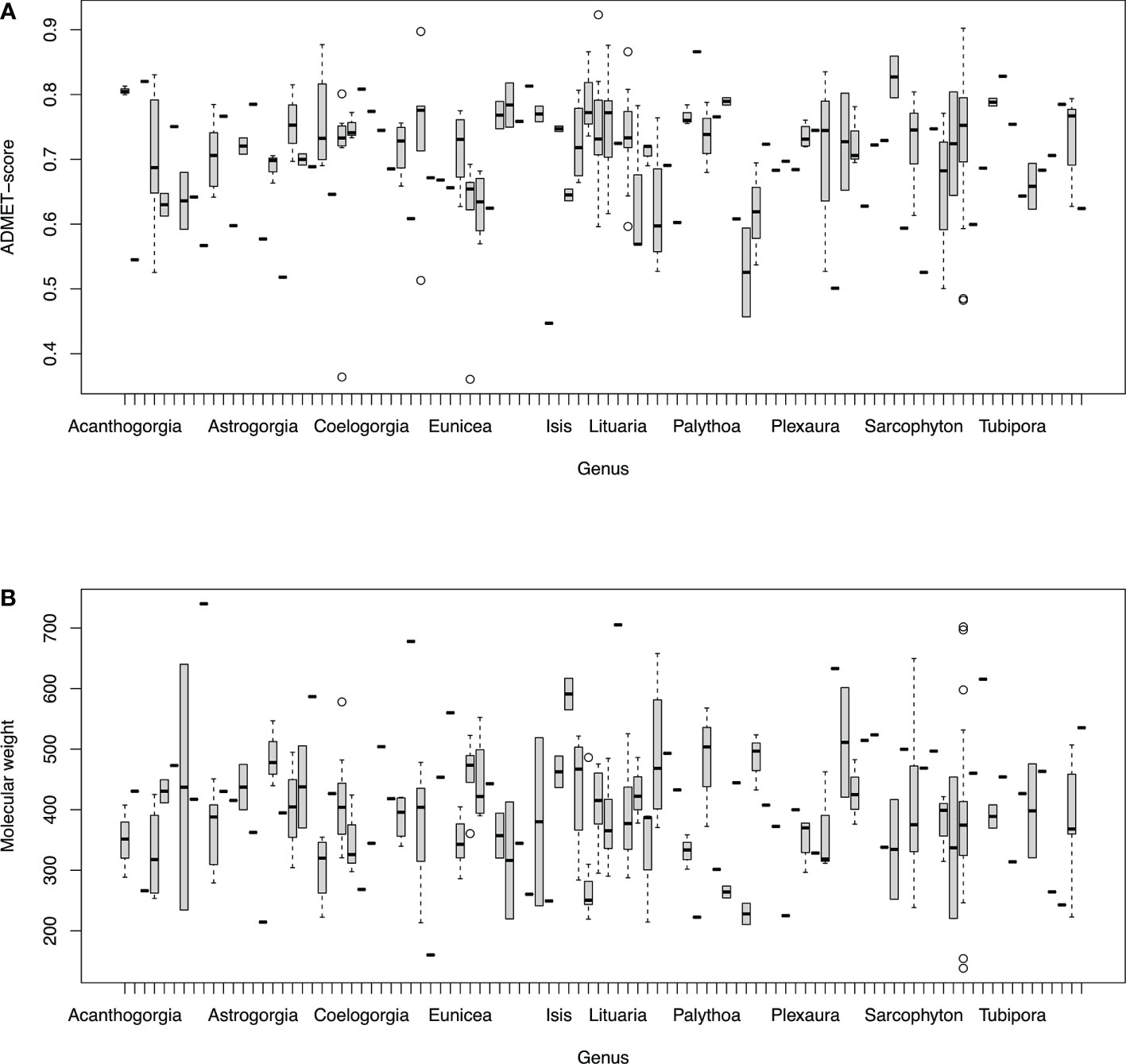
Figure 3 Box plots for (A) ADMET-score and (B) molecular weight of MarinLit compounds compared among genera. Full genus names (n = 98) are given in supplementary information.
Maps of species richness derived from GBIF data demonstrate some shortcomings of the available data (Figure 4A). While there are gaps in deeper water, the coastlines are generally covered. Relatively higher species richness is evident in areas like the great Barrier Reef off Australia and in the Caribbean. The number of species with at least one MarinLit compound in each 3° x 3° bin was positively related to the total number of species in the same area (Figure 4B, p < 0.05, r2 72.6%, F1,1207 = 3192). The remaining residual pattern indicates potential over- or under sampled areas (Figure 4C). Clusters of positive residuals (potentially more explored) areas are in the East and South China Seas, off the Australian coast, in the Caribbean and the Mediterranean. Potentially less explored 3° x 3° bins do not seem to show such distinct clustering, although areas adjacent to Florida, New Zealand and Hawaii may represent coherent areas of lower residuals.
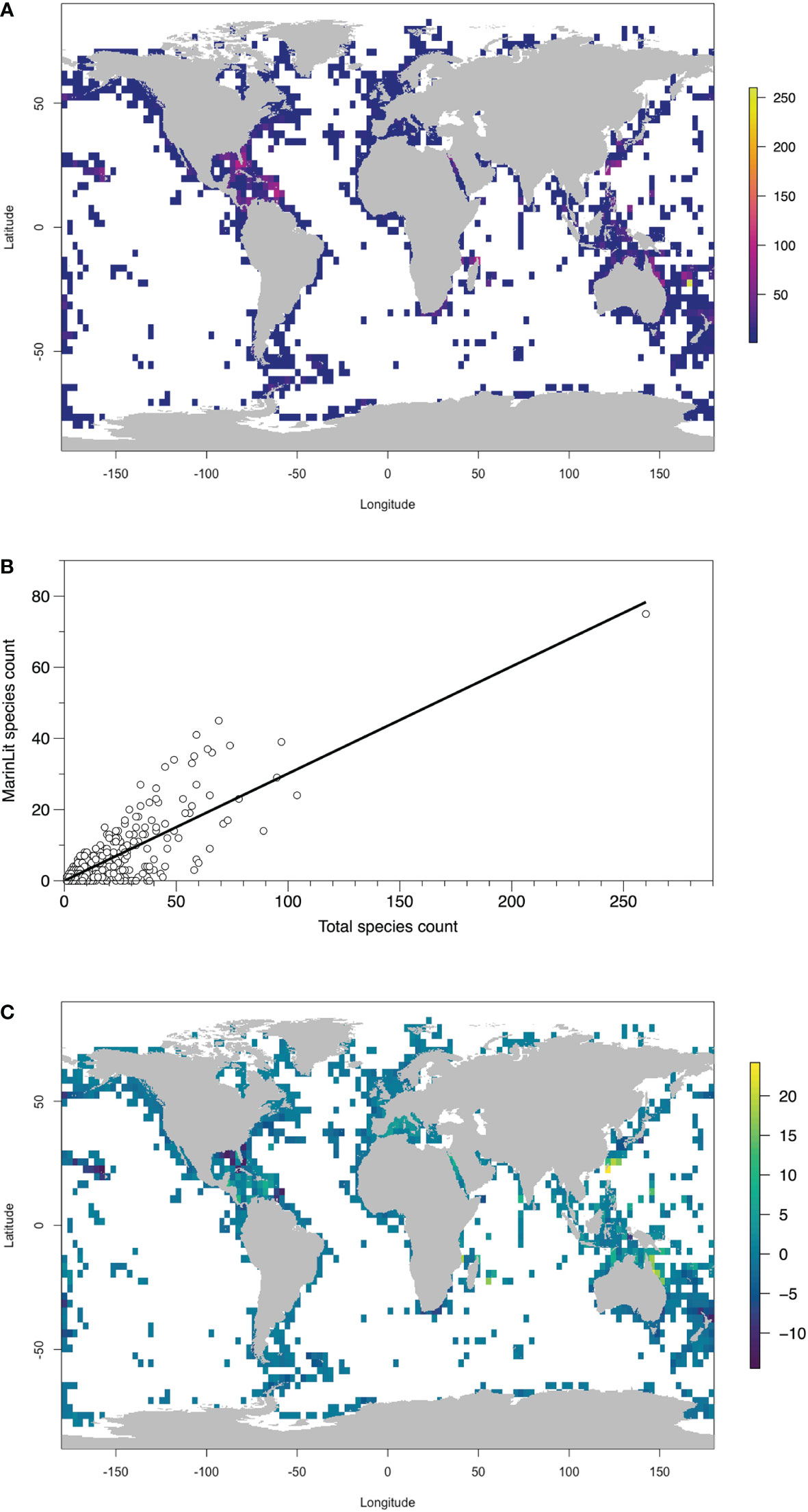
Figure 4 (A) Distribution of Alcyonacea species records in GBIF in 3° x 3° areas. (B) Relationship between number of species with at least one MarinLit compound and the total species count in each 3° x 3° area. Fitted line is linear regression through origin, r2 72.6%. (C) Pattern of residuals from panel B, indicating areas where the proportion of species with a MarinLit compound is higher or lower than expected.
4 Discussion
The patterns of MarinLit compounds in Cnidaria can mostly be explained in terms of the logistics of biodiscovery research practices rather than underlying biology. It is perhaps not surprising that there is a focus on species rich orders and taxa that grow with sufficient biomass for collection (Leal et al., 2012). The availability of biomass (e.g., where colonies are small and delicate) is likely to have influenced the relatively low number of natural products from Hydrozoa. Similarly, jellies in the Cubozoa, Scyphozoa and Staurozoa may be low in biomass or have unpredictable distributions. Myxozoa are generally microscopic parasites, so unlikely to be the focus of traditional biodiscovery. Other factors may affect the amount of work carried out in specific taxonomic groups. Ceriantharia are typically solitary burrowing anemones where sampling may require destructive digging. Similarly, many hard corals may need to be smashed or broken to collect biomass, with collectors potentially uncomfortable about sampling in very destructive ways. Further influences on the species that have been sampled may arise due to conservation and trade legislation. For example, the Convention on International Trade in Endangered Species of Wild Fauna and Flora (CITES) requires export controls on several Cnidaria groups, including black corals (Antipatharia spp.), stony corals (Scleractinia spp.), blue corals (Helioporidae spp.), organ-pipe corals (Tubiporidae spp.), Coralliidae (precious corals), fire corals (Milleporidae spp.), and lace corals (Stylasteridae spp.).
The hypothesis that species with at least one novel natural product are evenly distributed across genera was accepted, with genus size having a clear influence on MarinLit listing (Figure 1). The fitted line suggests an approximately 10% discovery rate of at least one natural product per species. Although the random discovery model was a statistically significant descriptor of the distribution of natural product associated species across genera, the r2 value was 39.5%, indicating that other factors may also have a role. Looking at the most extreme outliers, both Sinularia and Dendronephthya contain conspicuous species. However, Sinularia spp. (leather corals) are widely kept in aquaria and considered to be more easily cultured (e.g., compare Sinularia spp. kept for eight years in a flow through aquarium with no additional feeding Tsai et al. (2015), to comments on “difficult to maintain” Dendronephthya by Wijgerde (2015)). The ease of culturing may contribute to Sinularia spp. receiving more attention from biodiscovery studies, reflected in the positive outlier observed.
The distribution of natural products per species follows a scaling relationship seen in many phenomena, such as city size (e.g., Hackmann and Klarl, 2020), word frequency in languages (e.g., Meadow et al., 1993), and co-authorship networks (e.g., Ausloos, 2014). There is no indication that the discovery rate of natural products decreases for the most productive species. Macroalgae have a similar pattern of natural products per species, although no statistical model was fitted in Leal et al. (2013). Scaling relationships invite multiple explanations, but can be difficult to interpret. Influences on biodiscovery presumably reflect relative accessibility and ease of working with source biomass. Some of the top-ranked cnidarian species grow in shallow waters and are amenable to culturing. Other influences may also be relevant. For example, the same author occurs in over 50% of papers describing compounds from Briareum stechei, a different author is associated with over 50% of papers on MarinLit for Clavularia viridis. Scientific productivity is affected by many factors, but it is possible that success with identifying a novel natural product leads to more funding or interest in the source species or laboratory. This would have the effect of intensifying research on particular species, potentially producing the skewed distribution of novelty per species observed. Such reinforcement of success processes have long been recognized in the process of science as “Matthew effects” (Merton, 1968).
Although mean ADMET-score of natural products differed between genera, the observed signal was not as strong as that of molecular weight. The average molecular weight of natural products from different species could reflect biological influences or choices made by scientists. There may, for example, be evolutionary selection pressures for smaller metabolites if larger molecules performing the same function have a higher energetic cost of production. More information on metabolic pathways and function of metabolites, however, would be needed to explore any proposed selection pressures on average metabolite size. Other influences on observed MarinLit compound molecular weights may be introduced by analysts ignoring small metabolites likely to be volatile, or larger molecular weights that are less likely to be drug leads (Lipinski et al., 1997). Such decisions by analysts might be likely to narrow the range of molecular weights for MarinLit compounds. Without an obvious evolutionary hypothesis for the variation among genera in MarinLit compound molecular weight, the simplest hypothesis is that the observed differences reflect the analytical choices and interests of groups working on particular species. The observations made in the previous paragraph about scientists’ potential specialization on particular species offers various routes by which individual analytical choices can feed into different molecular weight compounds discovered in different genera. In this respect, the variance explained (as r2) for molecular weight is an estimate of the aggregate effect of many scientific choices. The corresponding r2 for among-genera variation in ADMET-score was no greater than what seems to have occurred for molecular weights during the biodiscovery process. Observations of ADMET-score are therefore consistent with the idea that differences reflect analytical choices rather than biology. The lack of a biologically-determined effect is also consistent with the lack of a covariance in metabolite properties at taxonomic levels above genus.
Using MarinLit compounds is a weak test of species-specific differences in natural product type. MarinLit records the first description of a natural product, but the same natural product may occur in other genera and such duplicated findings are less likely to be published (and are not included in the database). Metabolomic approaches, including standardised protocols and definition of metabolic ‘fingerprints’ (e.g., Mueller et al., 2020) would be a more powerful way of investigating evolutionary links to metabolite composition. Trait information (e.g., growth form) may also be a useful source of information to understand the prevalence of metabolites of interest across different groups once databases (e.g., Marine Species Traits editorial board, 2022) are complete enough for such analyses.
Further complexities with establishing taxonomic links to novel compounds arise through processes that cause the presence or concentration of metabolites to vary. Hence, a metabolite may be undetectable at the time of chemical analysis, even in species that are able to produce the compound. Such variability may occur due to ontogenetic changes, size (Maida et al., 1993), life history stage or sex (Fleury et al., 2016). Similarly, the presence of competitors may stimulate production of metabolites (Maida et al., 1993; Fleury et al., 2004; Singh and Thakur, 2016), with other biological interactions like infection or parasitism also likely to affect expressed levels of compounds. Different environmental conditions will influence metabolite concentrations (Kelman et al., 2000), these could be spatial or temporal (e.g., seasonal) influences on species’ biology (Marti et al., 2005).
The geographic distribution of biodiscovery in Alcyonacea is strongly related to underlying patterns of species richness. The process of science may have inflated the observed correlation as productive groups may submit more complete occurrence records to GBIF, while also being associated with biodiscovery labs (e.g., Evans-Illidge et al., 2013). However, the results are consistent with previous findings of biodiscovery associated with richness hotspots (Leal et al., 2012; Leal et al., 2013). Analysis of the residual variability suggested areas of slightly greater research intensity consistent with areas accessible to regions with historically greater funding (IOC-UNESCO, 2020) and also identified in Leal et al. (2012). As the GBIF data do not have fully global coverage, it was not possible to evaluate the intensity of biodiscovery in some regions (e.g., deep sea).
The overall picture of biodiscovery in Cnidaria is that, beyond an initial filtering of taxonomic groups unsuitable due to sampling and conservation considerations, exploration has been a passive process: following patterns of species richness in genera and among areas. This is perhaps a surprising conclusion, as individual analysts seem unlikely to have chosen study species at random. However, the role of individual choices is lost in an aggregated effort which leads to relatively consistent discovery rates for species containing at least one novel natural product, with outliers that seem to indicate genera that are harder or easier to work with.
The distribution of novel compounds by species reflects processes affecting lab productivity, but shows no sign of an upper limit to the number of novel compounds in a species. This is also a surprising conclusion as it has been suggested that potential exhaustion of chemical diversity is one of the possible reasons for a decline in the rate of novel metabolite description in marine invertebrates (Calado et al., 2022). The metabolomes of Cnidaria are not characterised, although presumably they contain many thousands of metabolites (a recent database for yeast includes 16042 compounds, Ramirez-Gaona et al., 2017), so the exhaustion of further novelty in Cnidaria is difficult to assess. However, the lack of a plateau in the species-specific natural product distribution implies that more novelty remains. Furthermore, instrumentation and analytical approaches are reducing the amount of material needed to identify novel natural products (Molinski, 2010; Freire et al., 2022).
At the moment, arguments could be advanced for both biodiscovery strategies proposed by Leal et al. (2012): a) focussing on well-studied genera or species that are amenable to biodiscovery work and have already yielded many new natural products or b) focussing on understudied taxonomic groups or regions. It may be possible to examine trade-offs between the two approaches by considering the size of the apparent deficit (as a negative residual in MarinLit species per genus, or negative residuals in fraction of MarinLit species in an area). The relatively high degree of variation in natural product discovery that reflects species richness (in genera or areas) implies that exploration of Cnidaria is at an early stage. The relationships examined in this study may become modified in the future, with evidence for specific phylogenetic influences on the patterns of novel natural products as metabolomes are explored further both within and between species.
Data availability statement
The datasets presented in this study can be found in online repositories. The names of the repository/repositories and accession number(s) can be found below: Average MW and ADMET scores by species, with taxonomic fields available at https://doi.org/10.6084/m9.figshare.20518275.v1. GBIF species distribution records https://doi.org/10.15468/dl.edsukx.
Author contributions
Conceptualisation, AA, MJ, CL-M, and BB. Methodology, CL-M, MJ. Resources, AA, MJ, BB. Data curation, CL-M, E-AC, KM. Writing—original draft preparation, MJ. Writing—review and editing, AA, MJ, CL-M, RY, E-AC, KM, BB. Supervision, CL-M, and BB. Project administration, AA. Funding acquisition, AA, MJ, and BB. All authors contributed to the article and approved the submitted version.
Funding
Research supported by a research grant from Science Foundation Ireland (SFI) and the Marine Institute under the Investigators Programme (grant no. SFI/15/1A/3100), co-funded under the European Regional Development Fund 2014–2020 to A.L.A.
Conflict of interest
The authors declare that the research was conducted in the absence of any commercial or financial relationships that could be construed as a potential conflict of interest.
Publisher’s note
All claims expressed in this article are solely those of the authors and do not necessarily represent those of their affiliated organizations, or those of the publisher, the editors and the reviewers. Any product that may be evaluated in this article, or claim that may be made by its manufacturer, is not guaranteed or endorsed by the publisher.
Supplementary material
The Supplementary Material for this article can be found online at: https://www.frontiersin.org/articles/10.3389/fmars.2022.1023518/full#supplementary-material
Supplementary Information | Mean property values by genus. Order is the same as the horizontal axis of Figure 3.
References
Ausloos M. (2014). Zipf–Mandelbrot–Pareto model for co-authorship popularity. Scientometrics 101, 1565–1586. doi: 10.1007/s11192-014-1302-y
Calado R., Mamede R., Cruz S., Leal M. C. (2022). Updated trends on the biodiscovery of new marine natural products from invertebrates. Mar. Drugs 20, 389. doi: 10.3390/md20060389
Cameron A. C., Windmeijer F. A. G. (1996). R-squared measures for count data regression models with applications to health-care utilization. J. Business Econ. Stat. 14 (2), 209–220. doi: 10.2307/1392433
Carroll A. R., Copp B. R., Davis R. A., Keyzers R. A., Prinsep M. R. (2022). Marine natural products. Nat. Prod. Rep. 39, 1122. doi: 10.1039/d1np00076d
Cheng F., Li W., Zhou Y., Shen J., Wu Z., Liu G., et al. (2012). admetSAR: a comprehensive source and free tool for assessment of chemical ADMET properties. J. Chem. Inf. Model. 52, 3099–3105. doi: 10.1021/ci300367a
Evans-Illidge E. A., Logan M., Doyle J., Fromont J., Battershill C. N., Ericson G., et al. (2013). Phylogeny drives large scale patterns in Australian marine bioactivity and provides a new chemical ecology rationale for future biodiscovery. PloS One 8 (9), e73800. doi: 10.1371/journal.pone.0073800
Fleury B., Coll J., Sammarco P. (2016). Complementary (secondary) metabolites in a soft coral: sex-specific variability, inter-clonal variability, and competition. Mar. Ecol.-.Evol. Persp 27, 204–218. doi: 10.1111/j.1439-0485.2006.00106.x
Fleury B. G., Coll J. C., Sammarco P. W., Tentori E., Duquesne S. (2004). Complementary (secondary) metabolites in an octocoral competing with a scleractinian coral: effects of varying nutrient regimes. J. Exp. Mar. Biol. Ecol. 303, 115–131. doi: 10.1016/j.jembe.2003.11.006
Freckleton R. P., Harvey P. H., Pagel M. (2002). Phylogenetic analysis and comparative data: A test and review of evidence. Am.Nat 160, 712–726. doi: 10.1086/343873
Freire V. F., Gubiani J. R., Spencer T. M., Hajdu E., Ferreira A. G., Ferreira D. A. S., et al. (2022). Feature-based molecular networking discovery of bromopyrrole alkaloids from the marine sponge agelas dispar. J. Nat. Prod 85, 1340–1350. doi: 10.1021/acs.jnatprod.2c00094
Guan L., Yang H., Cai Y., Sun L., Di P., Li W., et al. (2019). ADMET-score–a comprehensive scoring function for evaluation of chemical drug-likeness. MedChemComm 10, 148–157. doi: 10.1039/C8MD00472B
Hackmann A., Klarl T. (2020). The evolution of zipf's law for U.S. cities. Pap. Reg. Sci. 99, 841–852. doi: 10.1111/pirs.12498
IOC-UNESCO (2020). Global ocean science report 2020–charting capacity for ocean sustainability. Ed. Isensee K. (Paris: UNESCO Publishing).
Kaas Q., Yu R., Jin A.-H., Dutertre S., Craik D. J. (2012). ConoServer: updated content, knowledge, and discovery tools in the conopeptide database. Nucleic Acids Res. 40, D325–D330. doi: 10.1093/nar/gkr886
Kelman D., Benayahu Y., Kashman Y. (2000). Variation in secondary metabolite concentrations in yellow and grey morphs of the red Sea soft coral Parerythropodium fulvum fulvum: Possible ecological implications. J. Chem. Ecol. 26, 1123–1133. doi: 10.1023/A:1005423708904
Laguionie-Marchais C., Allcock A. L., Baker B. J., Conneely E.-A., Dietrick S. G., Kearns F., et al. (2022). Not drug-like, but like drugs: cnidaria natural products. Mar. Drugs 20 (1), 42. doi: 10.3390/md20010042
Leal M. C., Munro M. H. G., Blunt J. W., Puga J., Jesus B., Calado R., et al. (2013). Biogeography and biodiscovery hotspots of macroalgal marine natural products. Nat. Prod. Rep. 30, 1380. doi: 10.1039/c3np70057g
Leal M. C., Puga J., Serôdio J., Gomes N. C. M., Calado R. (2012). Trends in the discovery of new marine natural products from invertebrates over the last two decades – where and what are we bioprospecting? PloS One 7 (1), e30580. doi: 10.1371/journal.pone.0030580
Lindquist N., Shigematsu N., Pannell L. (2000). Corydendramines a and b, defensive natural products of the marine hydroid Corydendrium parasiticum. J. Nat. Prod. 63, 1290–1291. doi: 10.1021/np000050h
Lipinski C. A., Lombardo F., Dominy B. W., Feeney P. J. (1997). Experimental and computational approaches to estimate solubility and permeability in drug discovery and development settings. Adv. Drug Deliv. Rev. 23, 3–25. doi: 10.1016/S0169-409X(96)00423-1
Maida M., Carroll A. R., Coll J. C. (1993). Variability of terpene content in the soft coral Sinularia-flexibilis (Coelenterata, octocorallia), and its ecological implications. J. Chem. Ecol. 19, 2285–2296. doi: 10.1007/BF00979664
Marine Species Traits editorial board (2022) Marine species traits. Available at: http://www.marinespecies.org/traitson2022-07-21.
Marti R., Uriz M. J., Turon X. (2005). Spatial and temporal variation of natural toxicity in cnidarians, bryozoans and tunicates in Mediterranean caves. Scient. Mar. 69, 485–492. doi: 10.3989/scimar
Meadow C. T., Wang J., Stamboulie M. (1993). An analysis of zipf-Mandelbrot language measures and their application to artificial languages. J. Info. Sci. 19, 247–258. doi: 10.1177/016555159301900401
Meng L.-H., Li X.-M., Zhang F.-Z., Wang Y.-N., Wang B.-G. (2020). Talascortenes a–G, highly oxygenated diterpenoid acids from the sea-anemone-derived endozoic fungus Talaromyces scorteus AS242. J. Nat. Prod 83, 2528–2536. doi: 10.1021/acs.jnatprod.0c00628
Merton R. K. (1968). The Matthew effect in science. Science 159, (3810) 56–63. doi: 10.1126/science.159.3810.56
Molinski T. F. (2010). NMR of natural products at the ‘nanomole-scale’. Nat. Prod. Rep. 27, 321–329. doi: 10.1039/b920545b
Mueller C., Kremb S., Gonsior M., Brack-Werner R., Voolstra C. R., Schmitt-Kopplin P. (2020). Advanced identification of global bioactivity hotspots via screening of the metabolic fingerprint of entire ecosystems. Sci. Rep. 10, 1319. doi: 10.1038/s41598-020-57709-0
Oksanen J., Blanchet F. G., Friendly M., Kindt R., Legendre P., McGlinn D., et al. (2020) Vegan: Community ecology package. Available at: https://CRAN.R-project.org/package=vegan.
Orme D., Freckleton R., Thomas G., Petzoldt T., Fritz S., Isaac N., et al. (2018) Caper: Comparative analyses of phylogenetics and evolution in r. Available at: https://CRAN.R-project.org/package=caper.
Ramirez-Gaona M., Marcu A., Pon A., Guo A. C., Sajed T., Wishart N. A., et al. (2017). YMDB 2.0: a significantly expanded version of the yeast metabolome database. Nucleic Acids Res. 45 (D1), D440–D445. doi: 10.1093/nar/gkw1058
Sigwart J. D., Blasiak R., Jaspars M., Jouffray J.-B., Tasdemir D. (2021). Unlocking the potential of marine biodiscovery. Nat. Prod. Rep. 38, 1235. doi: 10.1039/d0np00067a
Singh A., Thakur N. L. (2016). Significance of investigating allelopathic interactions of marine organisms in the discovery and development of cytotoxic compounds. Chem. Biol. Interact. 243, 135–147. doi: 10.1016/j.cbi.2015.09.009
Tsai T.-C., Chen H.-U., Sheu J.-H., Chiang M.-Y., Wen Z. H., Dai C.-F., et al. (2015). Structural elucidation and structure–anti-inflammatory activity relationships of cembranoids from cultured soft corals Sinularia sandensis and sinularia flexibilis. J. Agric. Food Chem. 63 (32), 7211–7218. doi: 10.1021/acs.jafc.5b01931
Ulrich W., Ollik M., Ugland K. I. (2010). A meta-analysis of species–abundance distributions. Oikos 119 (7), 1149–1155. doi: 10.1111/j.l600-0706.2009.18236.x
Wijgerde T. (2015) Experimental aquaculture of dendronephthya corals. Available at: https://reefs.com/magazine/experimental-aquaculture-of-dendronephthya-corals/.
WoRMS Editorial Board (2022) World register of marine species. Available at: https://www.marinespecies.org (Accessed 2022-08-10).
Yang H., Sun L., Li W., Liu G., Tang Y. (2018). In silico prediction of chemical toxicity for drug design using machine learning methods and structural alerts. Front. Chem. 6. doi: 10.3389/fchem.2018.00030
Keywords: sampling, culture, access, ADMET-score, taxonomy, matthew effect
Citation: Johnson MP, Baker BJ, Conneely E-A, McKeever K, Young RM, Laguionie-Marchais C and Allcock AL (2022) Phylogenetic, species richness and logistic influences on the biodiscovery process in Cnidaria. Front. Mar. Sci. 9:1023518. doi: 10.3389/fmars.2022.1023518
Received: 19 August 2022; Accepted: 01 December 2022;
Published: 15 December 2022.
Edited by:
Bin Wu, Zhejiang University, ChinaReviewed by:
Narsinh L. Thakur, Council of Scientific and Industrial Research (CSIR), IndiaIsabella D’Ambra, University of Naples Federico II, Italy
Copyright © 2022 Johnson, Baker, Conneely, McKeever, Young, Laguionie-Marchais and Allcock. This is an open-access article distributed under the terms of the Creative Commons Attribution License (CC BY). The use, distribution or reproduction in other forums is permitted, provided the original author(s) and the copyright owner(s) are credited and that the original publication in this journal is cited, in accordance with accepted academic practice. No use, distribution or reproduction is permitted which does not comply with these terms.
*Correspondence: Mark P. Johnson, bWFyay5qb2huc29uQHVuaXZlcnNpdHlvZmdhbHdheS5pZQ==