- 1College of Plant Protection, Yangzhou University, Yangzhou, China
- 2State Key Laboratory of Estuarine and Coastal Research, Institute of Eco-Chongming, East China Normal University, Shanghai, China
- 3Yangtze Delta Estuarine Wetland Ecosystem Observation and Research Station, Ministry of Education and Shanghai Science and Technology Committee, Shanghai, China
- 4Conservation Biogeography Research Group, Institute of International Rivers and Eco-Security, Yunnan University, Kunming, Yunnan, China
- 5Yunnan Key Laboratory of International Rivers and Transboundary Eco-security, Yunnan University, Kunming, Yunnan, China
Governments and non-governmental organizations have widely recognized tidal wetland restoration as a sustainable instrument to lessen the threat of climate change, which is reflected by the expansion of the spatial scale of coastal restoration projects. However, approaches to large-scale spatial planning of tidal wetland restoration remain sparse. Previous studies on site selection for restoration planning have focused on the potential supply of ecosystem services (ES) or restoration feasibility with less emphasis on the mitigation of the status of regional ES supply and demand mismatches. We developed a five-step workflow based on systematic conservation planning to identify priority areas for tidal wetland restoration and applied it to the coastal reclaimed areas of Shanghai, China. With this workflow, we analyzed the changes in spatial distribution and the potential ecosystem services supply and restoration costs of priority areas between the two different scenarios of ES demand ignored and ES demand considered. Results showed that the potential restorable areas only accounted for 31.4% (425.2 km²) of the original reclaimed area because of other land use demands (e.g., permanent basic farmland conservation). We extracted 50% of the potential restorable areas as priority areas based on Aichi Target 15. Compared with the ES demand-ignored scenario, the ES demand scenario resulted in a substantial increase in the priority areas of Baoshan District (~177%) and Pudong New Area (~15%) and a small decrease in Chongming District (~4%). No significant change in the potential ES supply for all priority areas was observed between the two scenarios. However, the total restoration cost of the ES demand scenario is 10% higher than that of the ES demand-ignored scenario. Our study highlights the importance of considering the status of regional ES supply and demand (mis)matches in large-scale spatial planning for tidal wetland restoration.
1 Introduction
Coastal lowlands are frequently exposed to climate change-related risks (e.g., storm flooding), which evokes the need to explore new solutions for maintaining human well-being (Temmerman et al., 2013; Pittman et al., 2022). Healthy tidal wetlands can provide various valuable ecosystem services (ES) in a sustainable manner (e.g., coastal protection service; Liquete et al., 2013a; Temmerman and Kirwan, 2015; Zhu et al., 2020). Thus, restoring degraded tidal wetlands to strengthen the resilience of coastal zones has been well recognized by scientists in recent decades (Temmerman and Kirwan, 2015; Perillo et al., 2019). The spatial extent of coastal restoration projects has also gradually expanded recently (Gilby et al., 2021). Given that not all degraded tidal wetlands can be restored because of limited resources (e.g., the target area for restoration plans is often fixed; WWF, 2020), restoration actions must be prioritized in space (Wiens and Hobbs, 2015). To date, approaches for identifying priority areas for tidal wetland restoration at large spatial scales are still lacking (Adame et al., 2014; Liu et al., 2016; Gilby et al., 2021).
Target-based planning focuses on two types of conservation resource allocation problems: meeting the targets with the least cost (the minimum-set approach) and maximizing the number of targets met with a given resource (maximum coverage approach) (Moilanen, 2007). Systematic conservation planning (SCP), a typical target-based planning method, is widely considered the most influential paradigm for designing a protected area network (Kukkala and Moilanen, 2013). This method is composed of six stages: (1) collecting biodiversity data for the planning area, (2) setting conservation targets for the planning area, (3) assessing the conservation effectiveness of existing protected areas, (4) identifying additional protected areas, (5) implementing conservation actions, and (6) maintaining and monitoring protected areas (Margules and Pressey, 2000). Using the site selection algorithms (e.g., simulated annealing of MARXAN) of SCP to identify priority areas has more advantages than using scoring methods or expert opinions (McBride et al., 2010), such as reducing socio-economic investment and improving the connectivity of priority areas. Therefore, the SCP has been increasingly used to identify restoration priority areas for different ecosystems since 2006 (Crossman and Bryan, 2006; Qu et al., 2019; Costa et al., 2021; Gilby et al., 2021).
Studies applying SCP to the spatial planning of coastal ecosystem restoration have been conducted (e.g., Adame et al., 2014; Gilby et al., 2021), but they deserve improvement in at least two aspects. First, few studies have incorporated social and economic costs into the spatial planning of coastal restoration actions (Pittman et al., 2022; Su et al., 2022). For example, Adame et al. (2014) used a uniform indicator to represent the cost of hydrological restoration and afforestation. However, in practice, the cost of restoring the same ecosystem often varies considerably from site to site (Bayraktarov et al., 2016). Furthermore, Strassburg et al. (2020) confirmed that the cost-effectiveness of priority areas can be significantly improved by introducing a reduction in restoration costs as a key criterion for optimization. Therefore, mapping the spatial variation of costs and incorporating it into spatial optimization are two essential steps for identifying priority restoration areas, especially considering that economic feasibility is often the decisive factor in determining whether a restoration action can be implemented on the ground (Primack, 2010; Brancalion et al., 2019).
Second, previous studies used the biophysical supply of ES to represent restoration benefits (Strassburg et al., 2019; Strassburg et al., 2020) while ignoring that the supply of ES is not a good surrogate for the demand in many situations (Burkhard et al., 2012; Zhao et al., 2018; Cui et al., 2019). ES supply represents what is potentially available from ecosystem processes and functions, while ES demand represents the amount of ES consumed or expected to be obtained by human society (Villamagna et al., 2013; Peng et al., 2017). For example, the supply of carbon sequestration service is often quantified with the net primary productivity of natural ecosystems, while the demand for carbon sequestration service is expressed with the carbon dioxide emitted by socioeconomic systems (Shi et al., 2020). Currently, one of the important targets of ecological restoration is to improve human well-being (Gann et al., 2019; Peng et al., 2020). The maintenance of human well-being usually depends on the degree of coordination between ES supply and demand (Burkhard et al., 2012; Liquete et al., 2013b). Thus, the regional context of the degree of coordination between ES supply and demand serves as another important basis for guiding restoration action (Shi et al., 2020; Wang et al., 2021a). However, only a few studies have addressed the mitigation of regional ES supply and demand mismatches as an essential target of restoration planning (Costa et al., 2021; Goyette et al., 2021). A recent study by Costa et al. (2021) proved that ES demand should be integrated into forest landscape restoration to enhance local human well-being. However, Costa et al. (2021) did not examine the changes in restoration costs when improving the representativeness of ES demand in the priority area network. To the best of our knowledge, approaches for optimizing the allocation of restoration resources that simultaneously combine the status of regional ES supply–demand (mis)matches and restoration costs remain rare (Goyette et al., 2021).
China is one of the world’s largest resource countries in coastal wetlands (Perillo et al., 2019), but the country lost 58% of its coastal wetlands from 1950 to 2014 (Sun et al., 2015). Since 2000, China has gradually increased its investment in conserving coastal wetlands to reverse this situation (Sun et al., 2015; Liu et al., 2016; Wang et al., 2021b). China’s central government issued the “Master Plan for the Protection and Restoration of Important National Ecosystems (MPPRINE) (2021–2035)” in early June 2020 to promote ecological security and sustainability (Guan et al., 2021). In this plan, coastal ecological conservation and restoration are listed as one of the nine major national projects (Guan et al., 2021). MPPRINE has proposed general goals for restoration projects of different shore sections along the Chinese coast, such as improving the habitat quality of rare and endangered wildlife in the Yangtze River Estuary (Guan et al., 2021). MPPRINE does not provide site-selection methods to guide the deployment of ecological restoration projects. Soon after, China’s central government approved a national standard of Regulation Compiling Implementation Plan of Ecological Conservation and Restoration Project of Territorial Space (TD/T 1068–2022) to strengthen the top-level design of protection and restoration of multiple ecosystems in territorial space (http://gi.mnr.gov.cn/202207/t20220711_2742011.html). However, this document does not provide detailed information on the basis of site selection of coastal restoration projects (e.g., indicators for representing the restoration feasibility of hydrological connectivity). Therefore, the development of a systematic workflow and defensible indicators to guide the implementation of tidal wetland restoration planning is urgently needed (Liu et al., 2016).
In this study, we developed a framework for identifying priority areas for tidal wetland restoration and considered the reclaimed area of Shanghai coast as a case study. Our framework includes five steps: (1) removal of unsuitable restoration areas for delineating the planning area, (2) analysis of the status of regional ES supply and demand (mis)matches, (3) estimation of the potential ES supply to represent restoration benefits of the planning area, (4) construction of a concept model for quantifying restoration costs, and (5) identification of restoration priority areas based on the optimization algorithm of SCP. With this framework, we tested whether the introduction of regional ES supply and demand (mis)matches would significantly shift the spatial distribution of priority restoration areas and increase restoration costs. The results of this study would have important implications for optimizing spatial planning for coastal ecosystem restoration.
2 Materials and methods
2.1 Study area
The loss of tidal wetlands in Shanghai has been largely caused by coastal reclamation over the past 60 years (Wei et al., 2010; Lin et al., 2021). The remaining tidal wetlands cover a total area of 614 km², most of which are concentrated on Chongming Island and Jiuduansha Shoal (Cai et al., 2014; Figure 1). Annual average temperature, amount of precipitation, evaporation, and sunlight hours of Shanghai are 17.7°C, 1597.1 mm, 1027.9 mm, and 1668.6 h, respectively (SMSB and SONBS, 2017). The average and maximum tidal range are 2.67 and 4.62 m along Shanghai Coast, respectively (Zhang, 2015). The surface water salinity (> 1‰) in the north east of Chongming Island and the north bank of Hangzhou Bay is higher than that (< 0.45‰) along the coast of Baoshan District and part of southern Chongming Island (Zhang, 2015). The sediment types of Shanghai’s tidal wetlands are mainly sandy silt and silty sand (Wei et al., 2010). Common vegetation types of Shanghai’s tidal wetlands are Phragmites australis, Cyperaceae Juss., and Spartina alterniflora (Cai et al., 2014).
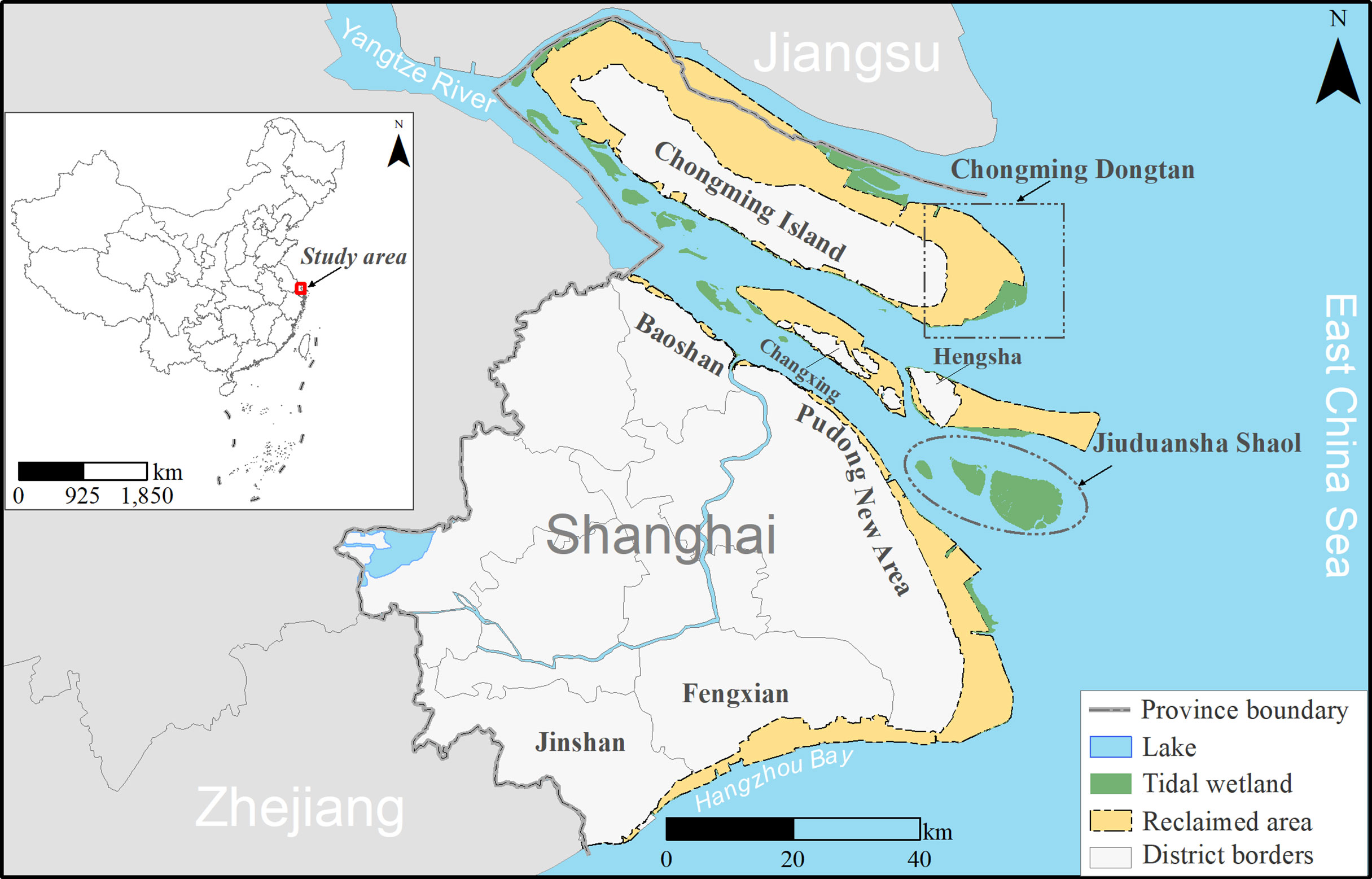
Figure 1 Map of the reclaimed area along Shanghai Coast between the 1950s and 2020. Inset shows the location of the study area in China (Left).
In this study, we focused on the reclamation area of Shanghai between the 1950s and 2020, including the northern part of Chongming Island, which belongs to the Jiangsu Province (Figure 1). Five administrative districts can be found along the Shanghai coast: Jinshan District, Fengxian District, Pudong New Area, Baoshan District, and Chongming District (Figure 1; SMSB and SONBS, 2017). Chongming District consists of three islands: Changxing Island, Hengsha Island, and Chongming Island (Figure 1). In the Shanghai Master Plan (2017–2035), the Shanghai Municipal People’s Government proposed the target of keeping the total amount of wetland resources unchanged (SUPLRAB, 2018). However, previous studies have shown that Shanghai’s tidal wetlands cannot be recovered to their historical maximum area through natural sedimentation, especially under the impacts of sediment declination, sea level rise, and coastal erosion (Ge et al., 2016; Luan et al., 2021). To promote the implementation of the Shanghai Master Plan (2017–2035), it is necessary to implement tidal wetland restoration in the reclaimed areas (SUPLRAB, 2018).
2.2 Overview of the framework
Based on the first four stages of the SCP paradigm (Margules and Pressey, 2000), we developed a five-step workflow to identify priority areas for tidal wetland restoration (Figure 2). The first step was to extract potential restorable areas from the reclaimed tidal wetlands. Multiple raster layers used for spatial prioritization were then produced through steps 2–4. In step 2, the status of regional ES supply and demand (mis)matches was assessed using the benefit transfer method and by analyzing the status of socioeconomic development. We calculated the ratio of ES demand to supply for each township. If this ratio is greater than one, then the ES in the township is in short supply. The potential benefits of the restored wetlands were estimated by combining the recovery rate, degree of land degradation, and ES value. Restoration costs were quantified by combining socio-economic costs and ecological feasibility. Finally, we input these layers into the SCP software and identified the priority areas for restoration under the two scenarios. In our workflow, steps 1–3 refer to stage 1 of the SCP, and steps 4–5 refer to stages 2 and 4 of the SCP.
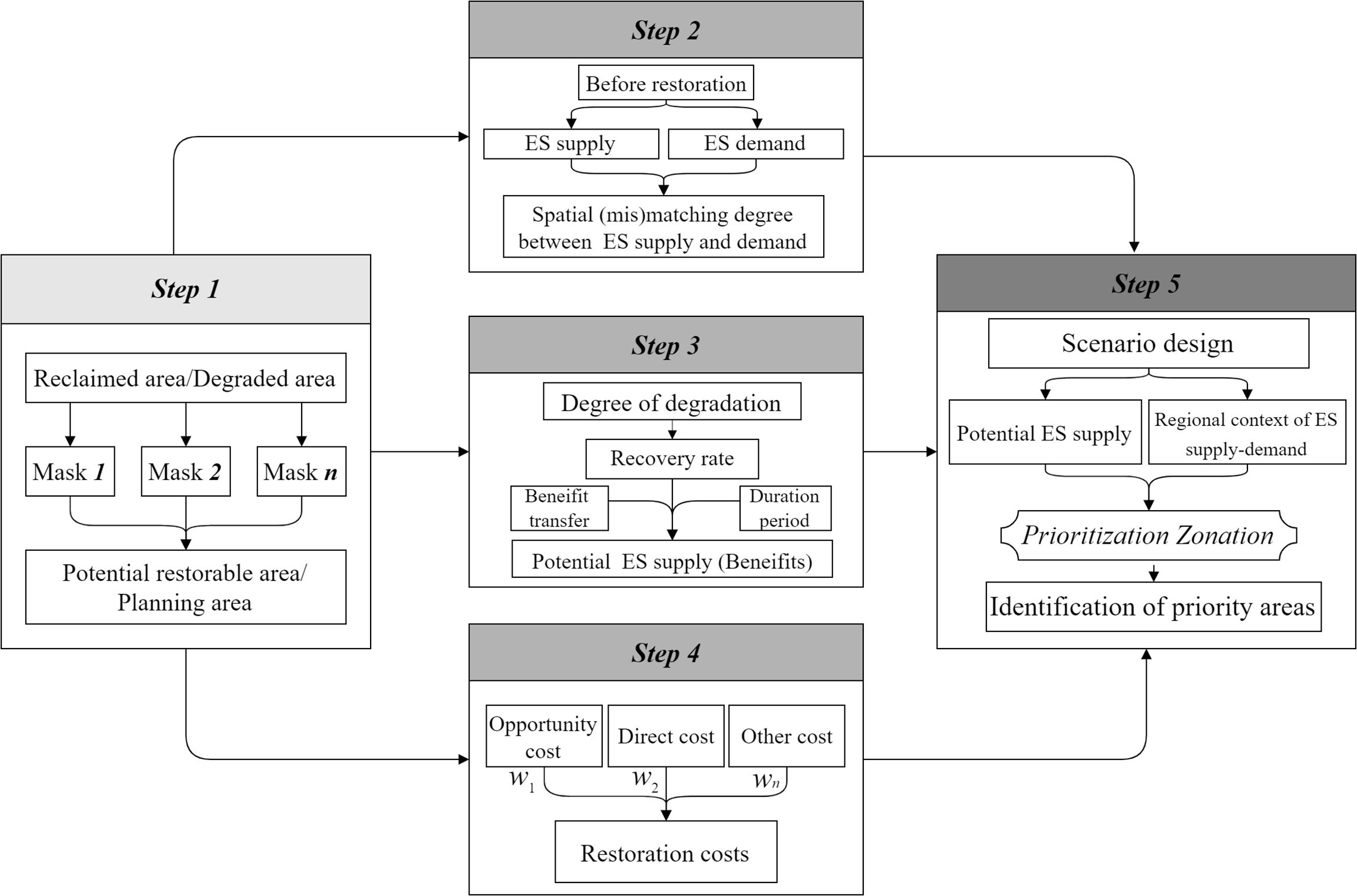
Figure 2 Workflow for identifying priority areas for tidal wetland restoration. wn represents the weight of the restoration cost n used for assessing the total restoration costs.
2.2.1 Extraction of the potential restorable areas
Ecological protection and restoration often face strong competition with other land-use demands such as coastal urbanization, food production, and low-carbon energy development (e.g., solar farms; Seddon, 2022). Therefore, we first eliminated reclaimed tidal wetlands that could not be restored for non-ecological reasons. Reclaimed areas from the 1950s to 2020 are regarded as degraded areas for tidal wetlands (Lin et al., 2021), which were digitized from the Shanghai Urban Geology (Wei et al., 2010) and Google Earth images. With reference to a previous study (Strassburg et al., 2019), we first excluded impervious surfaces (e.g., built-up land and roads) from the reclaimed area. Subsequently, several land use types used for social and economic development were erased from the map of reclaimed areas, including permanent basic farmland, urban drinking water sources, and advanced manufacturing bases. These land-use types are commonly known as “masks” in conservation planning (Groves, 2003). Among them, permanent basic farmland is determined in accordance with land use planning and is permanently protected and not allowed to be occupied for other uses, which has been implemented as a national policy for food security in China (Chen et al., 2017). Impervious surfaces were obtained from GlobeLand30 (V2020) (available at http://www.globallandcover.com/). The remaining “masks” were digitized based on the Atlas of the Shanghai Master Plan (2017–2035) (available at https://www.shanghai.gov.cn/nw42806/index.html).
2.2.2 Quantifying the spatial mismatch of ES supply and demand
We mapped ES supply and demand at the town scale according to Shi et al. (2020) to ensure a comparison of the results of different studies at the same spatial resolution. The economic value per unit area of ES was used to express the ES supply capacity of each town, which was calculated using the following equation:
where TESV represents the ES value per unit of each town in 2007$/ha/yr (same hereafter), wi is the area proportion of ecosystem i in the whole town, and ESVi is the ES value per unit area of ecosystem i. The composition data of the different ecosystems in each town were extracted from Globeland30 (V2020). We used the vector files of Shanghai’s dike in 2020 to divide “wetland” in Globeland30 (V2020) into two ecosystem types, namely, inland and tidal wetland. The ES values per unit area of different ecosystems were retrieved from a global database provided by Costanza et al. (2014). This database is one of the few that includes marine and terrestrial ES value data.
As recommended by previous studies (Peng et al., 2017; Zhao et al., 2018; Shou et al., 2020), we mapped ES demand by combining indicators representing land use intensity and socio-economic development:
where DIi is the ES demand index of town i, BAPi is the area proportion of built-up land in the town i, PDi is population density of town i in individuals/ha, and GDPi is the value per unit area of gross domestic product (commonly as GDP) of town i in CNY/ha. Population density and GDP data were derived from the 2017 statistical yearbook of each district.
We used the ES demand–supply ratio index ( DSRi hereinafter) to quantify the proximity between ES supply and demand. DSR can be calculated using the following equation:
where Si and Di are the ES supply and demand index of town i, respectively, using the min-max normalization method. The ES supply and demand of the town are more imbalanced when the DSRi value is higher.
2.2.3 Assessing benefits for tidal wetland restoration
In this study, we assumed that the potential ES supply of fully restored wetlands is consistent with global averages. The functional restoration of degraded tidal wetlands often takes a long time, ranging from 20 to 30 years (Bayraktarov et al., 2016). A global meta-analysis showed that the average annual recovery rate of degraded tidal wetlands is approximately 4% per year (Jones et al., 2018). The recovery rate of ecosystem properties is closely related to the degree of degradation before restoration (Ghazoul and Chazdon, 2017). Thus, we adjusted the recovery rate of different land cover types in potentially restorable areas according to their degree of degradation. Thus, the potential ES supply of tidal wetland restoration at the end of a pre-specified time can be calculated using the following equation:
where RBLCi represents the value per unit area of the potential ES supply of land cover type i at the end of year T, when it is used for restoration. In this study, we set T to 10 years according to the post-2020 outcome goals. ci is the coefficient used to adjust the recovery rate of land cover type i, which indicates the difference in the average degree of degradation for all land cover types in the reclaimed area. We used the ES value of each land cover type to represent the degree of degradation according to the concept of land degradation (IPBES, 2018). Thus, ci can be calculated using the following equation:
where ESVi is the ES value per unit area of land cover type i and is the average ES value per unit area for all land cover types in the potential restorable area.
2.2.4 Quantification of restoration costs
Restoration costs include opportunity costs, direct costs, contingency costs, transaction costs, external costs, and time lags (Wang et al., 2021a). Previous studies have used various indicators to represent monetary restoration costs, such as anthropogenic pressure (Qu et al., 2019; Goyette et al., 2021) and ecological restoration feasibility (Orsi and Geneletti, 2010). Considering that the available data on costs are often limited (Wang et al., 2022), we built a conceptual model for estimating restoration costs, which can be expressed by the following equation:
where RC is the restoration cost. NOC and NDC are the standardized indicators (based on the min-max normalization method) for opportunity cost and direct cost, respectively. w1 and w2 are the weights of NOC and NDC , respectively. In this study, both values were set to 0.5 in this study. Opportunity costs were represented by the housing prices of different districts in 2016, which were derived from Shanghai’s statistical yearbook (SMSB and SONBS, 2017).
The direct costs of restoration include investment in hydrological restoration, revegetation, sediment replenishment, raw material, and others (Wang et al., 2021a). Therefore, we assumed that direct costs were related to ecological feasibility. In this study, we propose the following equation to measure the direct costs:
where DC is the indicator for direct cost, and RFi is the normalized indicator for restoration feasibility i with the min-max normalization method. Based on previous studies and the availability of data (Widis et al., 2015; Liu et al., 2021), five key factors were adopted to assess restoration feasibility (Table 1). The methods and data sources for these indicators are listed in Table 1. The spatial extent of the tidal range and SSC data was restricted to offshore water. Therefore, we first divided the study area into multiple drainage basins using the hydrology tools of ArcGIS 10.2.1. The GEBCO_2021 Grid (with a spatial resolution of 15″; available at https://www.gebco.net/) was used to depict the drainage basins. Then, the average tidal range and SSC of the drainage basins intersected with coastal water were extracted from their original layers (Table 2) with the spatial analyst tools of ArcGIS 10.2.1. Finally, we assigned the values of the drainage basins intersecting with coastal water to those intersecting with or near the reclaimed area according to Tobler’s first law of geography (Tobler, 1970).
2.2.5 Identifying priority areas for tidal wetland restoration
Zonation can produce a priority scoring map by repeatedly calculating the importance values of all pixels across the planning region and excluding the pixels that have the lowest value until all pixels are excluded (Moilanen, 2007). Accordingly, the impacts of newly added features on the spatial distribution of priority ranking can be flexibly assessed. The additive benefit function (ABF) of Zonation was selected to address the spatial prioritization problem (Moilanen, 2007). The grid size of the three layers was resampled to 100 m × 100 m to ensure consistency with the units of the ES value.
In this study, two scenarios were designed to determine the impact of regional ES supply and demand (mis)matches on the distribution of priority areas. The first was “ES demand-ignored” scenario. In particular, the potential ES supply and restoration cost layers were inputted into Zonation 4.0. An edge removal function was used to improve the connectivity of the priority area network. The second scenario was the “ES demand” scenario. In particular, all three layers (potential ES supply, restoration costs, and ES demand-supply ratio) were inputted into the Zonation software and run under the same settings as in the first scenario. Referring to the methods of Costa et al. (2021), we extracted priority areas for tidal wetland restoration according to Aichi Biodiversity Target 15, which proposed the restoration of at least 15% of degraded ecosystems (IPBES, 2018). The potentially restorable areas may be much smaller than the original reclaimed areas. Thus, the threshold used for extracting priority areas should be modified according to potential restorable area ratio (Threshold = 0.15/potential restorable area ratio).
2.3 Statistical analysis
Kendall correlations were used to analyze the spatial association among the potential supply of ES, restoration costs, and the ES demand–supply ratio to explain the spatial characteristics of priority areas in different scenarios. The degree of spatial overlap of the priority areas generated from the two scenarios was evaluated. We then quantified the difference in priority areas for the different districts between the two scenarios. Changes in potential ES supply and restoration costs between the two scenarios were also calculated to analyze the impacts of incorporating the regional context of ES supply and demand into restoration planning.
3 Results
3.1 Spatial distribution of the potential restorable areas
From the 1950s to 2020, 1353 km² of tidal wetlands and coastal water along the Shanghai coast were reclaimed, among which 68.6% of the original reclaimed area was unsuitable for tidal wetland restoration because it had been converted into built-up land or other types for socio-economic development. The remaining 31.4% (425.2 km²) of the reclaimed area was considered suitable for ecological restoration (Figure 3), referred to as the potential restorable area hereinafter. Approximately 93.3% of the potential restorable areas were distributed in Pudong New Area and Chongming District (Figures 3A, B). Only 1.5% of the potential restorable areas were distributed in the Baoshan and Jinshan districts (Figures 3A, B). In terms of the proportion of different land cover types, cropland accounted for the largest proportion (72.3%) of the potential restorable areas, followed by water (15.2%), and inland wetlands (9.3%) (Figure 3C). Based on the method described in Section 2.2.5, we set the threshold for extracting priority areas to 50%.
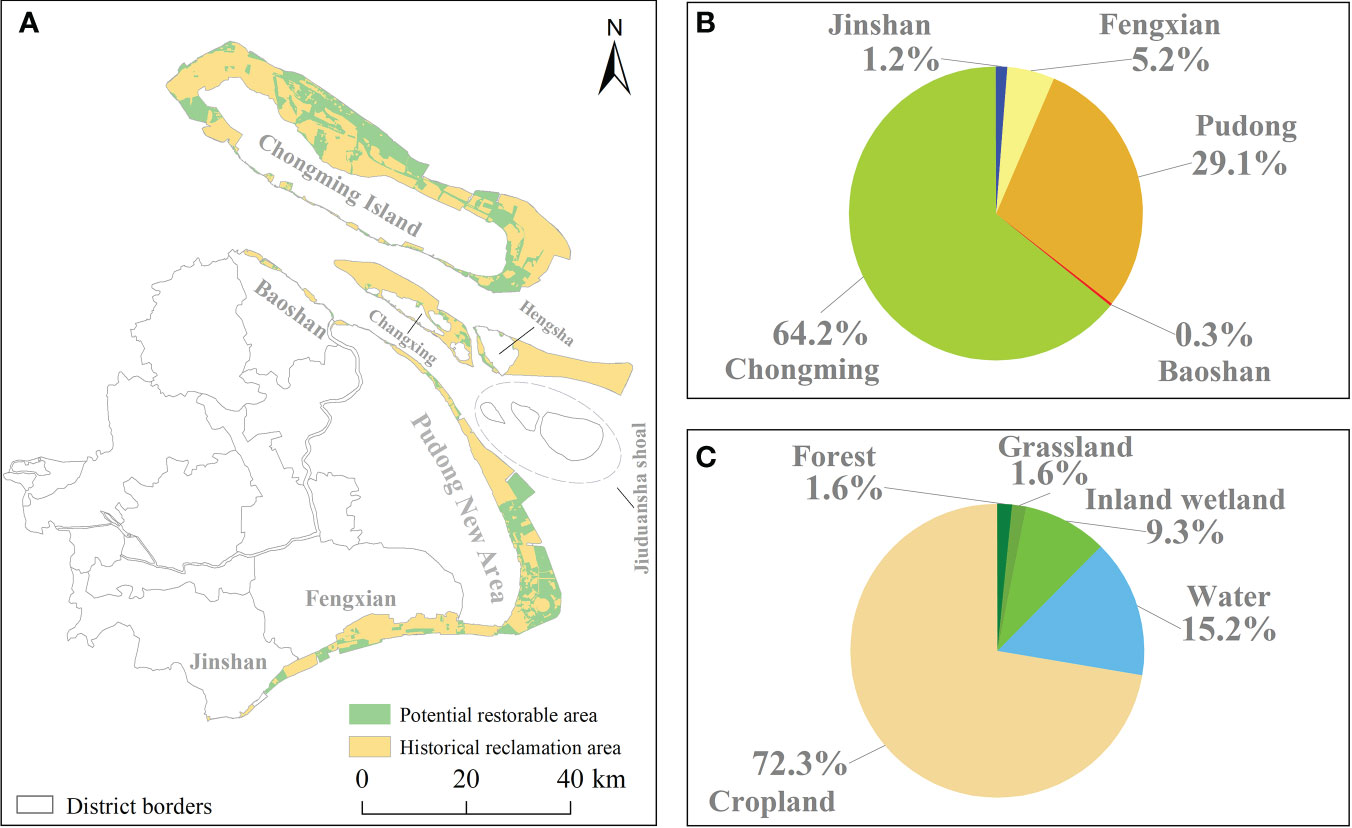
Figure 3 Spatial distribution of the potential restorable areas (A). The two pie charts were used to present the proportion of potential restorable areas contained in different districts (B) and the land cover composition of potential restorable areas (C), respectively.
3.2 Spatial relationships among restoration benefits, costs, and ES demand–supply ratio
The results showed that the ES demand–supply ratio in most potential restorable areas of Chongming District was lower than that of the other four districts (Figure 4A). Potential restorable areas with high ES demand–supply ratios were mainly distributed along the northeast coast of the Pudong New Area (Figure 4A). The estimation results showed that most potentially restorable areas had low ES values (Figure 4B). Only a few patches could provide high ES values after 10 years, such as the patches along the coast of the Jinshan District (Figure 4B). Restoration costs in Chongming District were significantly lower than those in the other districts (Figure 4C). The results of the Kendall correlation showed that the ES demand–supply ratio was significantly negatively correlated with the restoration costs (Figure 5, p < 0.05). The potential ES supply was insignificantly correlated with the ES demand–supply ratio and restoration costs (Figure 5, p > 0.05).
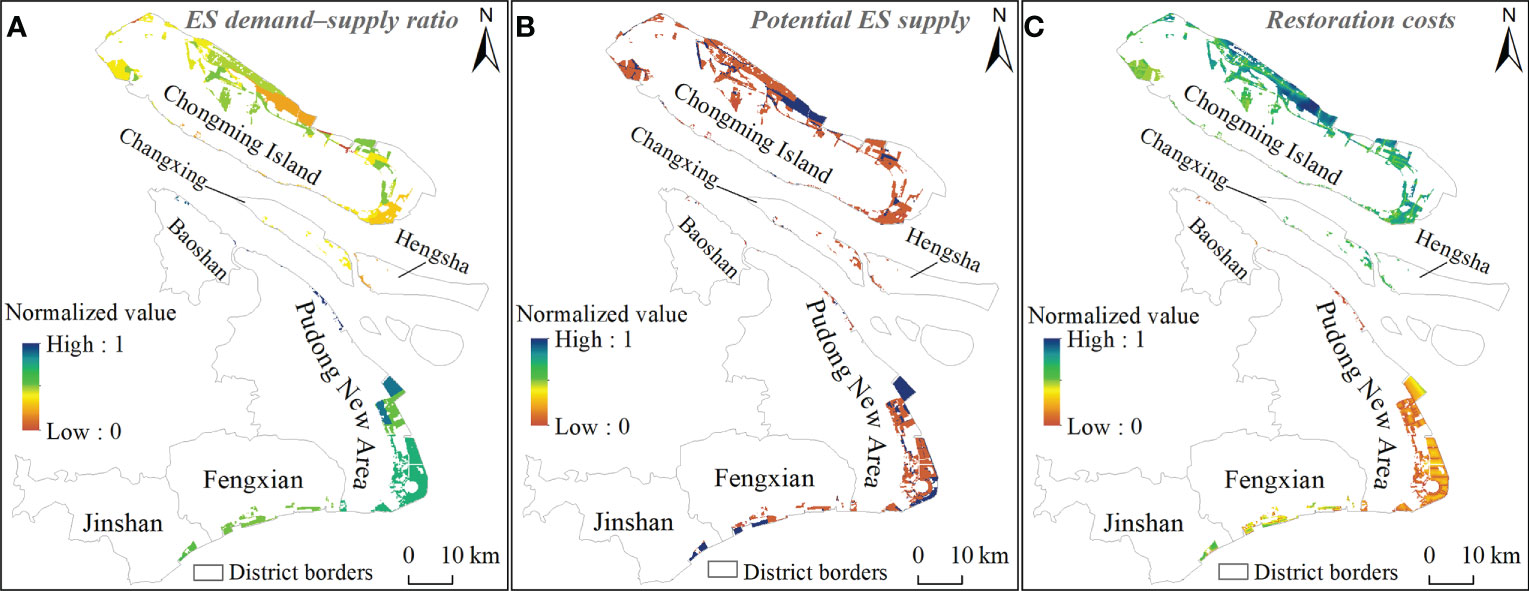
Figure 4 Spatial characteristics of ES demand–supply ratio (A), potential ES supply (B), and restoration costs (C). In (C), higher grid values correspond to lower restoration costs in reality.
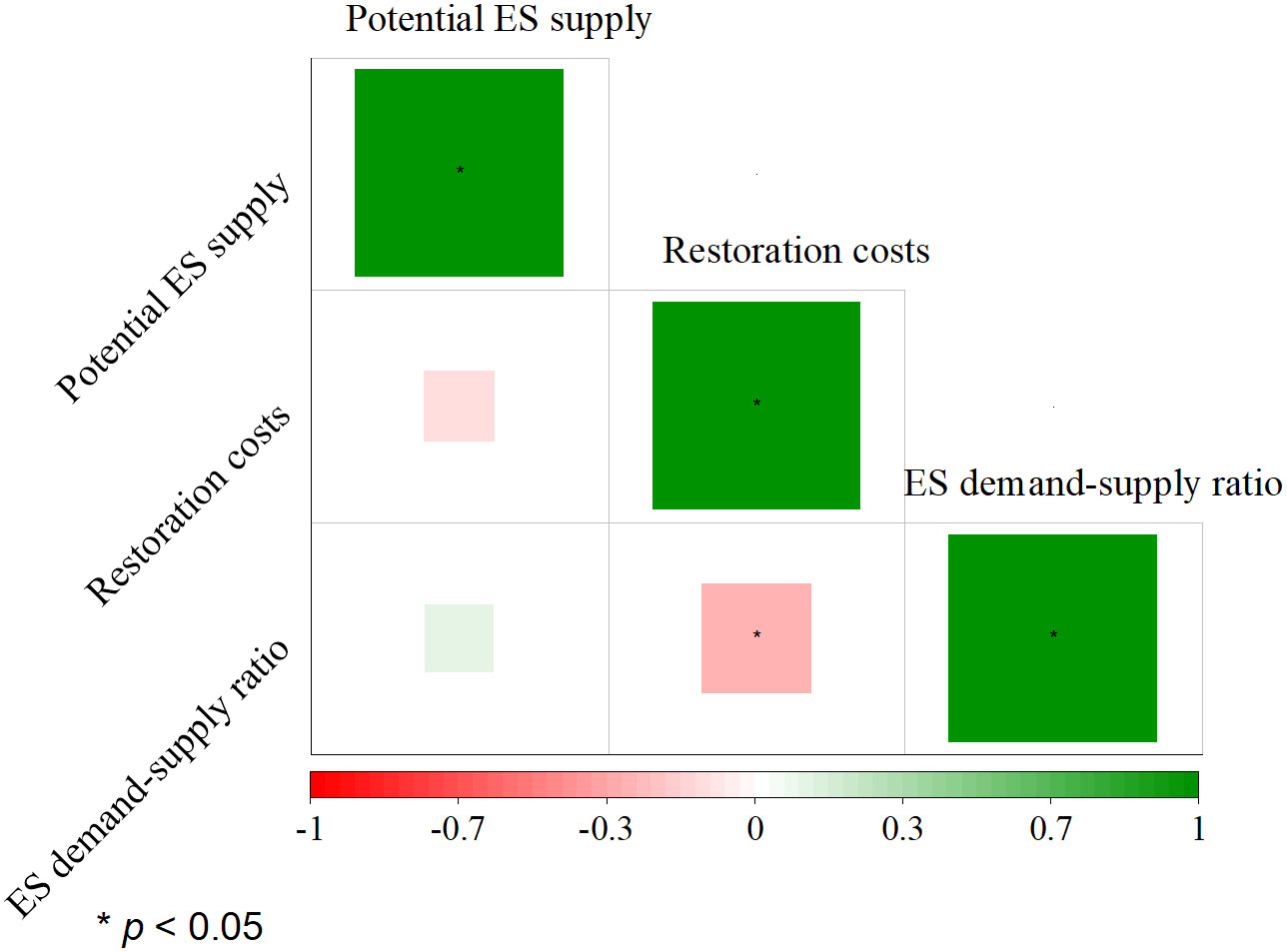
Figure 5 Correlation among the potential ES supply, restoration costs and ES demand–supply ratio. The size of the square represents the absolute value of the correlation coefficient. * means p < 0.05.
3.3 Spatial distribution of priority areas in different scenarios
The spatial distribution of the two priority ranking maps for the tidal wetlands varied greatly (Figure 6). The priority ranking of the northwest coast of Chongming Island in the ES demand scenario was lower than in the ES demand-ignored scenario (Figure 6C). In contrast, the priority ranking of many potential restorable areas in the central Pudong New Area was raised in the ES demand scenario compared with that in the ES demand-ignored scenario (Figure 6C). Among the five administrative districts, Fengxian and Chongming districts demonstrated declining performance in priority ranking, the two had average declines of 0.01 and 0.05, respectively (Figure 6C). The potential restorable areas of Baoshan District had the largest increase in priority ranking, on average (Figure 6C).
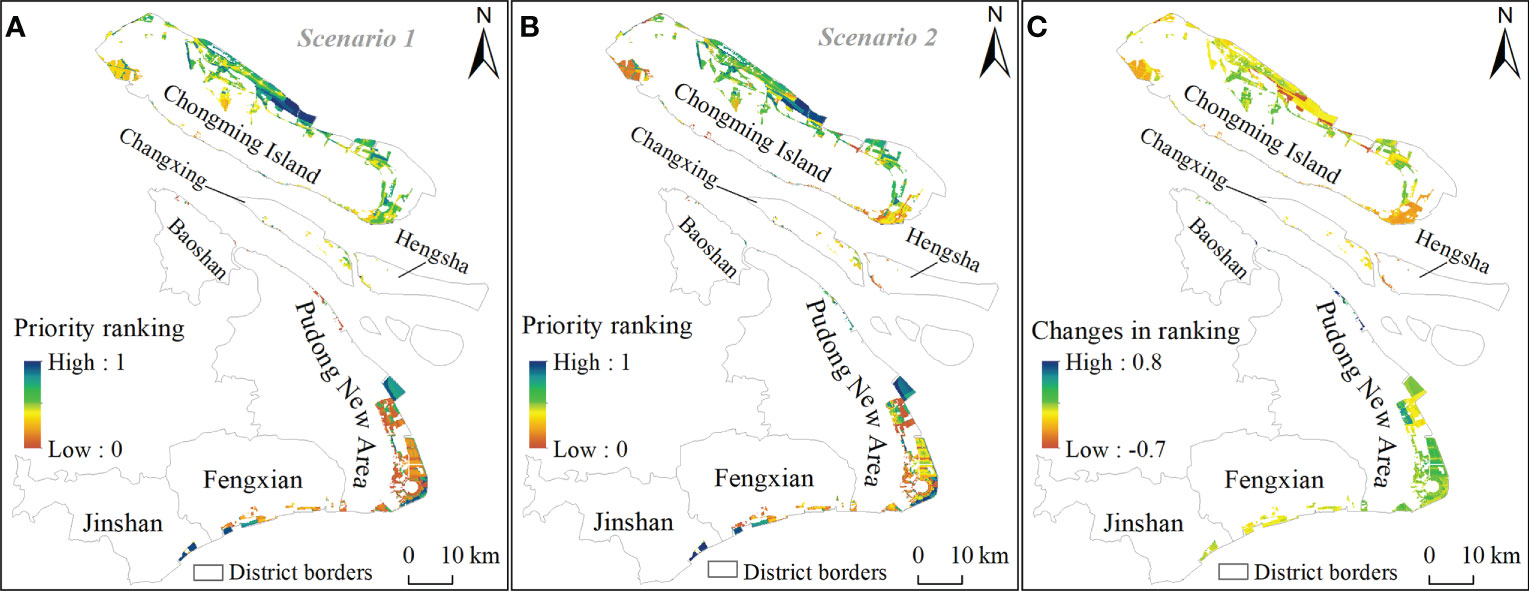
Figure 6 Spatial distribution of restoration priority ranking of potential restorable areas under ES demand-ignored (A) and ES demand scenario (B). (C) shows changes in the priority ranking between the two scenarios.
The total priority areas that we extracted accounted for approximately 45% of the potential restorable area instead of 50% (Table 2). This is because Zonation software requires all raster data to have the same number of rows and columns. However, the spatial resolution and format of the original data were different, which caused information loss during the data preparation. The overlapping area between the two scenarios accounted for 85% of the total priority areas. The priority areas of Chongming District in the ES demand scenario decreased by 3.9% compared with that in the ES demand-ignored scenario (Figure 7A and Table 2). For example, compared with the ES demand-ignored scenario, a larger number of potential restorable areas on eastern Chongming Island did not exist in the priority area network (Figure 7A). Conversely, many potential restorable patches along the coast of Baoshan District and the northern Pudong New Area were identified as priority areas for tidal wetland restoration only when combining the status of regional ES supply and demand (mis)matches (Figures 7B, C). For example, we found that restoration priority areas in Baoshan District increased by 1.8 times compared with the ES demand-ignored scenario (Table 2).
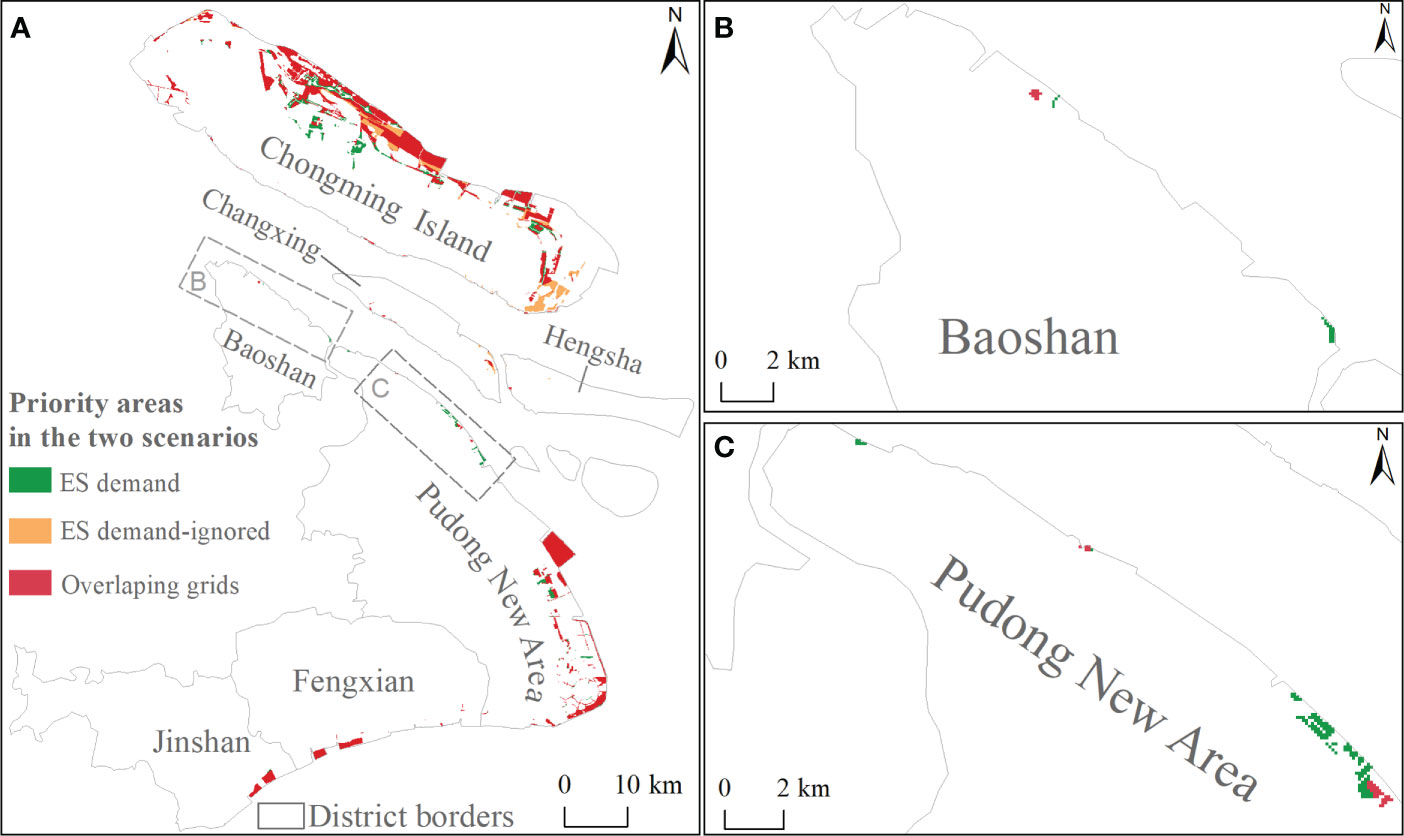
Figure 7 Spatial distribution of priority areas for tidal wetland restoration under different scenarios (A). (B) and (C) show the spatial distribution of priority areas along the coast of Baoshan District and the northern Pudong New Area, respectively.
3.4 Comparison of restoration benefits and costs between the two scenarios
Compared to the ES demand-ignored scenario, the total potential ES supply and restoration costs increased by 0.34% and 10%, respectively (Figure 8). Of the five administrative districts, only Chongming District showed a decrease in potential ES supply (2.3%) but an increase in restoration costs (3.0%) when accounting for the ES demand–supply ratio index (Figure 8). The change trend of the potential ES supply and restoration costs in the remaining four districts was consistent with that of the priority areas between the two scenarios. For example, the potential ES supply and restoration costs of Baoshan District increased by 57.6% and 161.1%, respectively, under the ES demand scenario (Figure 8).
4 Discussion
4.1 Availability of the potential restorable areas
Less than one-third of the original reclamation areas were potentially available for tidal wetland restoration along the Shanghai coast. This result demonstrates that previous studies may have overestimated the amount of land resources available for restoration because of the low availability of spatial data on the needs and interests of all relevant stakeholders (e.g., Qu et al., 2019; Strassburg et al., 2020). Excluding these specific land-use types from reclaimed areas is an essential step in the process of restoration planning. Many tidal wetlands are transformed to provide valuable services that cannot be offered by natural ecosystems, such as transport services provided by Shanghai Pudong International Airport and freshwater supply services provided by Qingcaosha Reservoir of Changxing Island (Chen et al., 2007). Similar projects are also being conducted abroad, such as the Eko Atlantic City project in Africa (Martín-Antón et al., 2016). Therefore, incorporating detailed information on competing land use demands into decision making by building a mechanism such as participatory land use planning is necessary to promote the benefits of ecological restoration (Gann et al., 2019).
4.2 Significance of incorporating ES demand–supply ratio index into restoration planning
In this study, trade-offs among the ES demand–supply ratio, potential ES supply, and restoration cost were mitigated using the ABF of Zonation. The ranking rule of the ABF is characterized by considering the overall high value for all the abovementioned features in a given planning unit instead of one feature that has the highest value. For this reason, we could explain why only considering the potential supply of ES and restoration costs would result in priority areas being concentrated in Chongming District (Figure 4A). Specifically, recent studies have shown that ES supply was lower than human demand in Baoshan District and the central Pudong New Area due to anthropogenic impacts (e.g., huge demand for land resources on industrial, residential, and commercial development; Chen et al., 2019; Jiang et al., 2020; Shi et al., 2020). The restoration costs in the two districts were much higher than those in Chongming District, which can be attributed to the high opportunity costs represented by local high housing prices (SMSB and SONBS, 2017). Given that the spatial distribution of potential ES supply was relatively homogeneous (Figure 4B), potential ES supply was not correlated with either the ES demand–supply ratio or restoration costs in this study. Thus, considering only the potential supply of ES and restoration costs would result in priority areas concentrated in districts with low economic development but relatively healthy ecosystems (e.g., Chongming District) (Figure 4A). Therefore, regional disparities in human well-being cannot be reduced by implementing ecological restoration projects.
The overlapping areas accounted for 85% of the priority areas in the two scenarios. However, the status of regional ES supply and demand (mis)matches cannot be neglected in restoration planning. Such a high proportion might have been caused by the high threshold for extracting priority areas in this study. In practice, the target of restoring 15% of degraded land is unlikely to be approved by the local government because of the scarcity of land resources in Shanghai (Chen et al., 2007). Discrepancies in the ranking score of many patches between the two scenarios were significant (Figure 6C), which means that patches with high ES demand–supply ratios but low restoration benefits or high restoration costs will be excluded from the priority area network as the threshold declines. For example, Wang et al. (2018) found that land subsidence exacerbates the threat of storm flooding to administrative districts with high housing prices (e.g., Baoshan District and northern Pudong New Area), which implies that coastal protection needs to be strengthened in these places. Restoring reclaimed tidal wetlands can attenuate wave energy, provide additional water storage, and improve the resilience of densely populated areas to flood risks (Temmerman and Kirwan, 2015). Coincidentally, parts of the local restorable areas only under the ES demand scenario could be included in the priority area network (Figures 7B, C). Future studies can design a scientific target for tidal wetland restoration based on the concept of ‘no net loss’ (He, 2019). For example, the total restoration area can be determined using the changes in the tidal wetland area over a certain period as a reference.
We used a conservative multiplier to calculate the benefits of restoration projects, which may have resulted in a low benefit-to-cost ratio in the first 10 years. However, a global meta-analysis found that the net benefits of restoration projects will ultimately outweigh the costs of that within a timeframe of approximately 70 years (Stewart-Sinclair et al., 2021). The case of the Yingwuzhou wetland restoration (located on the coast of Jinshan District) shows that the project investment can be recovered within 18 years according to the cost–benefit calculation of ES (Wu et al., 2020). Recent evidence also suggests that people are more inclined to pay for ES, which directly benefits them (Liu, 2020). Thus, tidal wetland restoration is worth implementing when ES is in high demand, even if restoration costs are high.
4.3 Applications for restoration planning
Regional ecosystem health deteriorates as the mismatch between ES demand and supply increases (Villamagna et al., 2013; Pan et al., 2021). Thus, restoration actions should be prioritized in areas with serious deficits in ES supply and demand to truly improve local human-being (Peng et al., 2020). In other words, if the information on regional supply and demand mismatches is ignored for spatial prioritization, it may lead to an increase in the overall potential ES supply only, but without the improvement in human well-being. Using our framework for implementing restoration projects can avoid this situation by addressing the trade-offs among the need to improve human well-being, the potential ES supply obtained from tidal wetland restoration, and restoration costs. In particular, our framework can guide the specific implementation of large-scale restoration projects such as China’s “MPPRINE (2021–2035)”. Many studies have quantified the spatial relationship between ES supply and demand to support environmental management in the Shanghai or Yangtze River Delta (e.g., Chen et al., 2019; Shi et al., 2020). However, they do not propose explicit spatial strategies to guide the deployment of restoration projects. For example, Shi et al. (2020) only recommended that priority areas for restoration should be placed in the central and suburbs of Shanghai City based on the analysis of ES flow. Priority areas identified by our framework not only provide essential ES to nearby people but are also highly cost-effective when they are implemented on the ground.
Our workflow has potential as a useful tool for informing restoration planning of other coastal ecosystems (e.g., mangroves and seagrass) by scientists and policymakers. First, most of the data and indicators used in this study also serve as an ecological and economic basis for the site selection of mangrove planting (e.g., tidal range; Worthington and Spalding, 2018). Second, the flexibility of our workflow allows the adjustment of indicators to adapt to different regional contexts. For instance, housing prices can be replaced by income from farming or aquaculture to represent the opportunity costs in tropical areas.
4.4 Refining the framework
Our framework has several limitations that should be addressed in the future. First, spatial (mis)matches of ES supply and demand were not assessed in a one-to-one manner because of the lack of unified methods for mapping ES demand (Zhao et al., 2018). Spatial mapping of ES demand is particularly scarce in coastal ES studies (Solé and Ariza, 2019). Second, the restoration cost equation can be further refined when the cost information on restoration engineering is available. For instance, external costs were ignored in this study because of the difficulty in defining the scope of the impact of external costs (Wang et al., 2021a). Finally, we used the global average recovery rate as the unique parameter of the reference system to estimate the potential ES supply, while ignoring the spatial heterogeneity of the environmental context. Future studies can build a reference ecosystem using the integrated database method, that is, a method derived from big data analysis (Pang et al., 2020).
5 Conclusions
In this study, a five-step framework was established to rank the spatial priorities of tidal wetland restoration. Compared with previous studies, we integrated the status of regional ES supply and demand (mis)matches into spatial prioritization to effectively improve human well-being through ecological restoration. We found that ignoring the comprehensive needs of socio-economic development (e.g., using reclaimed land to develop cutting-edge technology) can lead to the overestimation of potential restorable areas. The results showed that many potential restorable areas (e.g., the coast of Baoshan District and northern Pudong New Area) with a high demand for improving human well-being will be excluded from the priority area network unless the ES demand–supply ratio index is incorporated into spatial prioritization. Increasing the proportion of priority areas in districts with high ES demand–supply ratios increased the total restoration costs (up to 10%) and potential ES supply (approximately 0.3%) at the same time. This result was caused by the low spatial association between the status of regional ES supply and the demand matching degree before restoration and the potential ES supply thereafter. Overall, we advocate that information on the status of regional ES supply and demand (mis)matches should be incorporated into spatial planning to ensure the availability of restoration benefits to those who truly need them.
Data availability statement
The raw data supporting the conclusions of this article will be made available by the authors, without undue reservation.
Author contributions
SL designed the study, analyzed the data, and wrote the manuscript. SL and XL revised the manuscript. RW reviewed the manuscript and provided valuable feedback. YM, JW, and WZ contributed significantly to the data collection and manuscript preparation. All authors contributed to the article and approved the submitted version.
Funding
This work was supported by the National Natural Science Foundation of China (42141016) and Scientific Research Foundation for Advanced Talents, Yangzhou University (137012618).
Conflict of interest
The authors declare that the research was conducted in the absence of any commercial or financial relationships that could be construed as a potential conflict of interest.
Publisher’s note
All claims expressed in this article are solely those of the authors and do not necessarily represent those of their affiliated organizations, or those of the publisher, the editors and the reviewers. Any product that may be evaluated in this article, or claim that may be made by its manufacturer, is not guaranteed or endorsed by the publisher.
References
Adame M. F., Hermoso V., Perhans K., Lovelock C. E., Herrera-Silveira J. A. (2014). Selecting cost-effective areas for restoration of ecosystem services. Conserv. Biol. 29, 493–502. doi: 10.1111/cobi.12391
Bao D. Y., Zhu J. R. (2017). The effects of river regimes changes in the Changjiang Estuary on hydrodynamics and salinity intrusion in the past 60 years II. hydrodynamics. Acta Oceanologica Sin. 39, 1–15. doi: 10.3969/j.issn.0253-4193.2017.02.001
Bayraktarov E., Saunders M. I., Abdullah S., Mills M., Beher J., Possingham H. P., et al. (2016). The cost and feasibility of marine coastal restoration. Ecol. Appl. 26, 1055–1074. doi: 10.1890/15-1077
Brancalion P. H. S., Meli P., Tymus J. R. C., Lenti F. E. B., Benini R. M., Silva A. P. M., et al. (2019). What makes ecosystem restoration expensive? a systematic cost assessment of projects in Brazil. Biol. Conserv. 240, 108274. doi: 10.1016/j.biocon.2019.108274
Burkhard B., Kroll F., Nedkov S., Müller F. (2012). Mapping ecosystem service supply, demand and budgets. Ecol. Ind. 21, 17–29. doi: 10.1016/j.ecolind.2011.06.019
Cai Y. M., Zhou Y. X., Tian B. (2014). Shanghai wetlands. (Shanghai: Shanghai Scientific & Technical Publishers).
Chen J. Y., Cheng H. Q., Dai Z. J. (2007). Compatibility of utilization and protection of tidal flat and wetland: a case study in Shanghai area. Eng. Sci. 9, 11–17.
Chen J. Y., Jiang B., Bai Y., Xu X. B., Alatalo J. M. (2019). Quantifying ecosystem services supply and demand shortfalls and mismatches for management optimization. Sci. Total Environ. 650, 1426–1439. doi: 10.1016/j.scitotenv.2018.09.126
Chen C. Q., Jiang P. H., Cai L. Y., Shan J. X., Zhang Y. Q., Wang L. Y., et al. (2017). Delineation of a permanent basic farmland protection area around a city centre: case study of Changzhou city, China. Land Use Policy. 60, 73–89. doi: 10.1016/j.landusepol.2016.10.014
Costa T., Mazzochini G. G., Oliveira-Filho A. T., Ganade G., Carvalho A. R., Manhães A. P. (2021). Priority areas for restoring ecosystem services to enhance human well-being in a dry forest. Restor. Ecol. 29, e13426. doi: 10.1111/rec.13426
Costanza R., de Groot R., Sutton P., van der Poleg S., Anderson S. J., Kubiszewski I., et al. (2014). Changes in the global value of ecosystem services. Glob. Environ. Change. 26, 152–158. doi: 10.1016/j.gloenvcha.2014.04.002
Crossman N. D., Bryan B. A. (2006). Systematic landscape restoration using integer programming. Biol. Conserv. 128, 369–383. doi: 10.1016/j.biocon.2005.10.004
Cui F. Q., Tang H. P., Zhang Q., Wang B. J., Dai L. W. (2019). Integrating ecosystem services supply and demand into optimized management at different scales: a case study in Hulunbuir, China. Ecosyst. Serv. 39, 100984. doi: 10.1016/j.ecoser.2019.100984
Gann G. D., McDonald T., Walder B., Aronson J., Nelson C. R., Jonson J., et al. (2019). International principles and standards for the practice of ecological restoration. second edition. Restor. Ecol. 27, S1–S46. doi: 10.1111/rec.13035
Ge Z. M., Wang H., Cao H. B., Zhao B., Zhou X., Peltola H., et al. (2016). Responses of eastern Chinese coastal salt marshes to sea-level rise combined with vegetative and sedimentary processes. Sci. Rep. 6, 28466. doi: 10.1038/srep28466
Ghazoul J., Chazdon R. (2017). Degradation and recovery in changing forest landscapes: a multiscale conceptual framework. Annu. Rev. Environ. Resour. 42, 161–188. doi: 10.1146/annurev-environ-102016-060736
Gilby B. L., Olds A. D., Brown C. J., Connolly R. M., Henderson C. J., Maxwell P. S., et al. (2021). Applying systematic conservation planning to improve the allocation of restoration actions at multiple spatial scales. Restor. Ecol. 29, e13403. doi: 10.1111/rec.13403
Goyette J. O., Cimon-Morin J., Mendes P., Thériault M., Pellerin S., Poulin M. (2021). Planning wetland protection and restoration for the safeguard of ecosystem service flows to beneficiaries. Landsc. Ecol. 36, 2691–2706. doi: 10.1007/s10980-021-01267-x
Groves C. R. (2003). Drafting a conservation blueprint: a practitioner’s guide to planning for biodiversity. (Washington DC: Island Press).
Guan F. J., Liu L. H., Liu J. W., Fu Y., Wang L. Y., Wang F., et al. (2021). Systematically promoting the construction of natural ecological protection and governance capacity: experts comments on master plan for major projects of national important ecosystem protection and restoration, (2021-2035). J. Nat. Resour. 36, 290–299. doi: 10.31497/zrzyxb.20210202
He Q. (2019). Conservation: ‘no net loss’ of wetland quantity and quality. Curr. Biol. 29, R1070–R1093. doi: 10.1016/j.cub.2019.08.027
IPBES (2018). The IPBES assessment report on land degradation and restoration. (Bonn: Secretariat of the Intergovernmental Science-Policy Platform on Biodiversity and Ecosystem Services).
Jiang B., Bai Y., Chen J. Y., Alatalo J. M., Xu X. B., Liu G., et al. (2020). Land management to reconcile ecosystem services supply and demand mismatches-a case study in Shanghai municipality, China. Land Degrad. Dev. 31, 2684–2699. doi: 10.1002/ldr.3614
Jones H. P., Jones P. C., Barbier E. B., Blackburn R. C., Rey Benayas J. M., Holl K. D., et al. (2018). Restoration and repair of earth’s damaged ecosystems. Proc. Biol. Sci. 185, 20172577. doi: 10.1098/rspb.2017.2577
Kukkala A., Moilanen A. (2013). Core concepts of spatial prioritisation in systematic conservation planning. Biol. Rev. 88, 443–464. doi: 10.1111/brv.12008
Lin S. W., Li X. Z., Yang B., Ma Y. X., Jiang C., Xue L. M., et al. (2021). Systematic assessments of tidal wetlands loss and degradation in Shanghai, China: from the perspectives of area, composition and quality. Glob. Ecol. Conserv. 25, e01450. doi: 10.1016/j.gecco.2020.e01450
Liquete C., Piroddi C., Drakou E. G., Gurney L., Katsanevakis S., Charef A., et al. (2013a). Current status and future prospects for the assessment of marine and coastal ecosystem services: a systematic review. PloS One. 8, e67737. doi: 10.1371/journal.pone.0067737
Liquete C., Zulian G., Delgado I., Stips A., Maes J. (2013b). Assessment of coastal protection as an ecosystem service in Europe. Ecol. Indic. 30, 205–217. doi: 10.1016/j.ecolind.2013.02.013
Liu Y. X. (2020). The willingness to pay for ecosystem services on the Tibetan plateau of China. Geogr. Sustain. 1, 141–151. doi: 10.1016/j.geosus.2020.06.001
Liu Z. Z., Cui B. S., He Q. (2016). Shifting paradigms in coastal restoration: six decades’ lessons from China. Sci. Total Environ. 566, 205-214. doi: 10.1016/j.scitotenv.2016.05.049
Liu Z. Z., Fagherazzi S., Cui B. S. (2021). Success of coastal wetlands restoration is driven by sediment availability. Commun. Earth Environ. 2, 44. doi: 10.1038/s43247-021-00117-7
Luan H. L., Ding P. X., Yang S. L., Wang Z. B. (2021). Accretion-erosion conversion in the subaqueous Yangtze delta in response to fluvial sediment decline. Geomorphology. 382, 107680. doi: 10.1016/j.geomorph.2021.107680
Margules C. R., Pressey R. L. (2000). Systematic conservation planning. Nature. 405, 243–253. doi: 10.1038/35012251
Martín-Antón M., Negro V., del Campo J. M., López-Gutiérrez J. S., Esteban D. (2016). Review of coastal land reclamation situation in the world. J. Coast. Res. 75, 667–671. doi: 10.2112/si75-133.1
McBride M. F., Wilson K. A., Burger J., Fang Y. C., Lulow M., Olson D., et al. (2010). Mathematical problem definition for ecological restoration planning. Ecol. Model. 211, 2243–2250. doi: 10.1016/j.ecolmodel.2010.04.012
Moilanen A. (2007). Landscape zonation, benefit functions and target-based planning: unifying reserve selection strategies. Biol. Conserv. 134, 571–579. doi: 10.1016/j.biocon.2006.09.008
Orsi F., Geneletti D. (2010). Identifying priority areas for forest landscape restoration in Chiapas (Mexico): an operational approach combining ecological and socioeconomic criteria. Landsc. Urban Plan. 94, 20–30. doi: 10.1016/j.landurbplan.2009.07.014
Pang B., Cui B. S., Cai Y. Z., Xie T., Wang Q., Ning Z. H. (2020). Studies on selection method of reference condition for ecological restoration of coastal wetlands in China. Environ. Ecol. 2 (1-9), 25.
Pan L. Y., Guo B. Y. (2020). Remote sensing research of suspended sediment concentration in Zhoushan archipelago sea area based on Landsat8 data. Ocean Dev. Manage. 37, 82–90. doi: 10.20016/j.cnki.hykfygl.2020.09.014
Pan Z. Z., He J. H., Liu D. F., Wang J. W., Guo X. N. (2021). Ecosystem health assessment based on ecological integrity and ecosystem services demand in the middle reaches of the Yangtze river economic belt, China. Sci. Total Environ. 774, 144837. doi: 10.1016/j.scitotenv.2020.144837
Peng J., Li B., Dong J. Q., Liu Y. X., Lü D. N., Du Y. Y., et al. (2020). Basic logic of territorial ecological restoration. China Land Sci. 34, 18–26. doi: 10.11994/zgtdkx.20200427.124442
Peng J., Yang Y., Xie P., Liu Y. X. (2017). Zoning for the construction of green space ecological networks in Guangdong province based on the supply and demand of ecosystem services. Acta Ecol. Sin. 37, 4562–4572. doi: 10.5846/stxb201601020007
Perillo G. M. E., Wolanski E., Cahoon D. R., Hopkinson C. H. (2019). Coastal wetlands: an integrated ecosystem approach (2th). (Netherlands: Elsevier).
Pittman S. J., Stamoulis K. A., Antonopoulou M., Das H. S., Shahid M., Delevaux J. M. S., et al. (2022). Rapid site selection to prioritize coastal seascapes for nature-based solutions with multiple benefits. Front. Mar. Sci. 9, 832480. doi: 10.3389/fmars.2022.832480
Qu Y., Sun G. Q., Luo C. Y., Zeng X. Y., Zhang H. Q., Murray N. J., et al. (2019). Identifying restoration priorities for wetlands based on historical distributions of biodiversity features and restoration suitability. J. Environ. Manage. 231, 1222–1231. doi: 10.1016/j.jenvman.2018.10.057
Seddon N. (2022). Harnessing the potential of nature-based solutions for mitigating and adapting to climate change. Science. 376, 1410–1416. doi: 10.1126/science.abn9668
Shanghai Municipal Statistics Bureau (SMSB) and Survey office of the National Bureau of Statistics in Shanghai (SONBS) (2017). Shanghai statistical yearbook 2016. (Beijing: China Statistics Press).
Shanghai Urban Planning and Land Resource Administration Bureau (SUPLRAB) (2018). Shanghai master plan (2017-2035): string for the excellent global city. (Shanghai: Shanghai Urban Planning and Land Resource Administration Bureau).
Shi Y. S., Shi D. H., Zhou L. L., Fang R. B. (2020). Identification of ecosystem services supply and demand areas and simulation of ecosystem service flows in Shanghai. Ecol. Indic. 115, 106418. doi: 10.1016/j.ecolind.2020.106418
Shou F. Y., Li Z. F., Huang L., Huang S. R., Yan L. J. (2020). Spatial differentiation and ecological patterns of urban agglomeration based on evaluations of supply and demand of ecosystem services: a case study on the Yangtze river delta. Acta Ecol. Sin. 40, 2813–2826. doi: 10.5846/stxb201904120734
Solé L., Ariza E. (2019). A wider view of assessments of ecosystem services in coastal areas: the perspective of social-ecological complexity. Ecol. Soc. 24, 24. doi: 10.5751/ES-10883-240224
Stewart-Sinclair P. J., Klein C. J., Bateman I., Lovelock C. E. (2021). Spatial cost-benefit analysis of blue restoration and factors driving net benefits globally. Conserv. Biol. 35, 1850–1860. doi: 10.1111/cobi.13742
Strassburg B. B. N., Beyer H. L., Crouzeilles R., Iribarrem A., Barros F., de Siqueira M. F., et al. (2019). Strategic approaches to restoring ecosystems can triple conservation gains and halve costs. Nat. Ecol. Evol. 3, 62–70. doi: 10.1038/s41559-018-0743-8
Strassburg B. B. N., Iribarrem A., Beyer H. L., Cordeiro C. L., Crouzeilles C. L., Jakovac C. C., et al. (2020). Global priority areas for ecosystem restoration. Nature. 586, 724–729. doi: 10.1038/s41586-020-2784-9
Sun Z. G., Sun W. G., Tong C., Zeng C. S., Yu X., Mou X. J. (2015). China’s coastal wetlands: conservation history, implementation efforts, existing issues and strategies for future improvement. Environ. Int. 79, 25–41. doi: 10.1016/j.envint.2015.02.017
Su J., Yin B. C., Chen L. Z., Gasparatos A. (2022). Priority areas for mixed-species mangrove restoration: the suitable species in the right sites. Environ. Res. Lett. 17, 065001. doi: 10.1088/1748-9326/ac6b48
Temmerman S., Kirwan M. L. (2015). Building land with a rising sea: cost-efficient nature-based solutions can help to sustain coastal societies. Science. 349, 588–589. doi: 10.1126/science.aac8312
Temmerman S., Meire P., Bouma T. J., Herman P. M. J., Ysebaert T., De Vriend H. J. (2013). Ecosystem-based coastal defence in the face of global change. Nature. 504, 79–83. doi: 10.1038/nature12859
Tobler W. R. (1970). A computer movie simulating urban growth in the Detroit region. Econ. Geogr. 46 (Supp1), 234–240. doi: 10.2307/143141
Villamagna A. M., Angermeier P. L., Bennett E. M. (2013). Capacity, pressure, demand, and flow: a conceptual framework for analyzing ecosystem service provision and delivery. Ecol. Complex. 15, 114–121. doi: 10.1016/j.ecocom.2013.07.004
Wang J. J., Li X. Z., Lin. S. W., Ma Y. X. (2022). Economic evaluation and systematic review of salt marsh restoration projects at a global scale. Front. Ecol. Evol. 10, 865516. doi: 10.3389/fevo.2022.865516
Wang C. X., Liu Y. X., Yu C. Y., Liu X. Q. (2021a). Research progress on the arrangement of territorial ecological restoration. Prog. Geogr. 40, 1925–1941. doi: 10.18306/dlkxjz.2021.11.011
Wang X. X., Xiao X. M., Xu X., Zou Z. H., Chen B. Q., Qin Y. W., et al. (2021b). Rebound in China’s coastal wetlands following conservation and restoration. Nat. Sustain. 4, 1076–1083. doi: 10.1038/s41893-021-00793-5
Wang J., Yi S., Li M. Y., Wang L., Song C. C. (2018). Effects of sea level rise, land subsidence, bathymetric change and typhoon tracks on storm flooding in the coastal areas of Shanghai. Sci. Total Environ. 62, 228–234. doi: 10.1016/j.scitotenv.2017.11.224
Wei Z. X., Di G. Y., Yan X. X., Fang Z., Yang J. G., He Z. F., et al. (2010). Shanghai urban geology. (Beijing: Geological Publishing House).
Widis D. C., BenDor T. K., Deegan M. (2015). Prioritizing wetland restoration sites: a review and application to a large-scale coastal restoration program. Ecol. Restor. 33, 358–377. doi: 10.3368/er.33.4.358
Wiens J. A., Hobbs R. J. (2015). Integrating conservation and restoration in a changing world. Biosci. 65, 302–312. doi: 10.1093/biosci/biu235
Worthington T., Spalding M. (2018). Mangrove restoration potential: a global map highlighting a critical opportunity. (Cambridge: University of Cambridge).
Wu W., Li C. X., Chen X. C. (2020). Evaluation of the effectiveness of a coastal ecological restoration project based on ecosystem services: a case study on Yingwuzhou wetland, China. J. East China Normal Univ. (Nat Sci.). 211, 98–108. doi: 10.3969/j.issn.1000-5641.201941027
WWF (2020) The Bezos Earth Fund & WWF: investment in community and climate. Available at: https://www.worldwildlife.org/pages/the-bezos-earth-fund-wwfinvestment-in-community-and-climate.
Zhang J. (2015). Ecological continuum from the Changjiang (Yangtze river) watersheds to the East China Sea continental margin. (Switzerland: Springer International Publishing).
Zhao W. W., Liu Y., Feng Q., Wang Y. P., Yang S. Q. (2018). Ecosystem services for coupled human and environment systems. Prog. Geogr. 37, 139–151. doi: 10.18306/dlkxjz.2018.01.015
Keywords: tidal wetland, priority areas, ecological restoration, systematic conservation planning, reclaimed area, Shanghai, ecosystem service, supply and demand
Citation: Lin S, Li X, Wu R, Ma Y, Zhao W and Wang J (2022) Identifying priority areas for tidal wetland restoration by integrating ecosystem services supply and demand mismatches. Front. Mar. Sci. 9:1019619. doi: 10.3389/fmars.2022.1019619
Received: 15 August 2022; Accepted: 03 November 2022;
Published: 18 November 2022.
Edited by:
Zeng Zhou, Hohai University, ChinaReviewed by:
Robin Kundis Craig, University of Southern California, United StatesQin Zhu, Southern Marine Science and Engineering Guangdong Laboratory (Guangzhou), China
Copyright © 2022 Lin, Li, Wu, Ma, Zhao and Wang. This is an open-access article distributed under the terms of the Creative Commons Attribution License (CC BY). The use, distribution or reproduction in other forums is permitted, provided the original author(s) and the copyright owner(s) are credited and that the original publication in this journal is cited, in accordance with accepted academic practice. No use, distribution or reproduction is permitted which does not comply with these terms.
*Correspondence: Xiuzhen Li, eHpsaUBza2xlYy5lY251LmVkdS5jbg==