- 1Institute of Coastal Systems - Analysis and Modeling, Helmholtz-Zentrum Hereon, Geesthacht, Germany
- 2Center for Earth System Sustainability, Institute of Oceanography, Universität Hamburg, Hamburg, Germany
A novel 3-dimensional numerical model resolving dynamic interactions between environmental drivers and benthic fauna was applied to an idealized domain as analogous to typical tidal embayments. The aim is to derive insights into the role of benthic fauna in guiding long-term (decadal to centennial) coastal morphological evolution at a system scale. Three major functions by benthic fauna on sediment dynamics, namely bio-destabilization, bio-deposition and bio-stabilization, were incorporated. Results indicate that each of the three functions is able to guide a unique and profound long-term change of the embayment morphology. Bioturbation-induced sediment mixing and bio-destabilization may result in net sediment export out of the embayment, whilst bio-deposition and bio-stabilization tend to alter the embayment toward a net sediment import environment. Benthic fauna is able to modify large-scale hydro-morphology toward a state favorable for living. A combined effect of the three functions is not just a simple neutralization of the opposing impacts between sediment stabilization and destabilization. Rather, it leads to a unique response of the embayment morphology due to interactions between different benthic functional groups. Comparison with a real tidal embayment (Jade Bay from the Wadden Sea) justified a general validity of the model results in terms of statistics in both morphology and benthic fauna, and suggested an equal importance of interactions between benthic fauna and bed morphology and between different benthic functional groups in guiding morphological development of complex coastal systems.
Introduction
Morphological evolution of coastal systems is jointly controlled by physical, biological and anthropogenic processes (Angamuthu et al., 2018). While the impacts of physical and anthropogenic drivers on coastal morphological development have long been acknowledged and extensively studied, the role of biota in guiding evolution of coastal landscapes is often overlooked and has become another focal point only until recent decades (Fagherazzi et al., 2004; Murray et al., 2008; Wang and Temmerman, 2013; Shi et al., 2020; Viles, 2020; Chen et al., 2021). The interaction between biota and environment is twofold. On one hand, coastal morphology, associated environmental forcing (e.g. tides, waves) and food availability exert a first-order control on the type of habitats as well as abundance and trait expression of biota (Murray et al., 2008; Holzhauer et al., 2019; Shi et al., 2021). On the other hand, biota in turn actively modify their environment to attain an optimized fitness for their living conditions (Jones et al., 1994; Hastings et al., 2007; Li et al., 2021). Understanding such dynamic interactions between environmental parameters and biota is essential in management and optimization of many coastal systems against present and future climate and anthropogenic threats (Murray et al., 2008; Viles, 2020).
Biota in coastal systems include flora and fauna. The former is well recognized to exert predominantly stabilizing effects on sediments and coastal morphology. In coastal lands and marshes, for instance, plants play an antagonist role to erosion by dampening tidal currents and waves, and trapping sediment (Möller et al., 2014; Zhang et al., 2015; Leonardi et al., 2018). Benthic fauna incorporates all animals living on or within the sea floor. Benthic faunal behaviors are highly complex and variable (Murray et al., 2014; Murray et al., 2017; Dairain et al., 2019), and can influence the stability of coastal morphology in either positive or negative ways depending on a variety of parameters such as age, biomass, community composition, sediment type, hydrodynamics (Arlinghaus et al., 2021). Benthic fauna is known to actively rework sediments and promote soil formation at small spatial scales (from millimeters to meters) within the habitat (Craft, 2000; Valdemarsen et al., 2018), which is via four main functions, namely (1) biomixing which transports and mixes sediment particles horizontally and vertically through foraging and sheltering behaviors (Kristensen et al., 2012; Lindqvist et al., 2016), (2) mediating particle flux over chemical and compositional gradients and grain transformation through processing of food (Andersen and Pejrup, 2011), (3) change of sea bed roughness through allogenic and autogenic structures (Alves et al., 2017), and (4) coating on sediment grain surface by biofilm (Stal, 2010).
An understanding of how small-scale benthic faunal behaviors may accumulatively lead to long-term and large-scale (km-scale) coastal morphological change is not trivial since benthic fauna may cause sediment deposition and erosion of the same order of magnitude as changes caused by natural physical drivers such as tides, waves, and sediment supply (Wood and Widdows, 2003). It requires an extension of current knowledge from species level to an integrated system level since many species may co-exist and interact among each other in a natural coastal system. In addition, variation in ecological traits may lead to large differences in bio-morphodynamics mediated by different species in different circumstances (Viles, 2020). Focus on only a few selected species but omission of other interacting species and feedback mechanisms among themselves and the environment may lead to incomplete or even biased knowledge (Reinhardt et al., 2010; Arlinghaus et al., 2021; Brückner et al., 2021). Despite of an increasing consensus that benthic fauna plays an important role in mediating morphological evolution at spatical scales much larger than its habitat (Nasermoaddeli et al., 2017; Brückner et al., 2021), development of numerical models quantifying associated bio-morphodynamics is still at an explorative stage. The hindering factors include not only limited understanding of fundamental biological/bio-physical processes affecting morphological development and dynamic feedback loops among them but also a shortage of data for model calibration and confirmation of simulation results especially at a large spatial scale (Arlinghaus et al., 2021).
Tidal embayments represent one of the most dynamic coastal systems which are persistently shaped by hydro-morphodynamics and bio-morphodynamics. Disentangling respective impact of individual abiotic and biotic drivers as well as their dynamic interactions and combined effects on morphological development of tidal embayments is difficult. For example, an investigation by Benninghoff and Winter (2019) of the German Wadden Sea, which consists of a series of tidal embayments and associated ebb deltas, barrier islands, salt marshes and estuaries, found that the region has undergone net sediment deposition in most tidal flats whilst net erosion in subtidal channels based on comparison of bathymetric data between 1998 and 2016. On the other hand, several abiotic (e.g. mean sea level rise, tides, waves and boundary sediment supply) and biotic (e.g. flora and fauna) factors can individually or jointly cause erosion in tidal channels and/or deposition in tidal flats (Marciano et al., 2005; Van Maanen et al., 2013a; Van Maanen et al., 2013b; Van Maanen et al., 2015; Zhang and Arlinghaus, 2022) and the exact mechanisms explaining the observed changes of the Wadden Sea have yet to be explored (Benninghof and Winter, 2019).
In this study, we intend to bridge part of the knowledge gap by quantifying the importance of benthic fauna in guiding long-term (decadal to centennial) coastal morphological evolution at a large and regional scale and disentangling its impact from abiotic drivers. To achieve this, we applied a novel 3-Dimensional numerical model, which resolves dynamic interactions between environmental drivers (hydrodynamics, morphodynamics, temperature), food availability and benthic fauna to an idealized domain as analogous to typical tidal embayments. Benthic fauna is represented by three functional groups according to its major impacts (bioturbation, bio-deposition and bio-stabilization) on sediment dynamics. Specifically, we aim to address the following questions:
I. How and to what extent can benthic fauna modify embayment-scale coastal morphology?
II. How and to what extent can interactions between different functional groups of benthic fauna influence long-term morphological development of tidal embayments?
Material and methods
In order to derive a general understanding of the importance of benthic fauna in guiding long-term and large-scale morphological development of tidal embayments, we have adopted an idealized initial coastal morphology which is composed of an offshore area, a tidal inlet and a tidal basin to mimic typical tidal embayments such as the Wadden Sea tidal basins (Figure 1). The similar initial morphology has been used in various studies to understand the sensitivity of morphological evolution to bottom slope, tidal current strength and sediment properties (Marciano et al., 2005), sea level rise (Van Maanen et al., 2013b) and coastal vegetation (mangroves, Van Maanen et al., 2015).
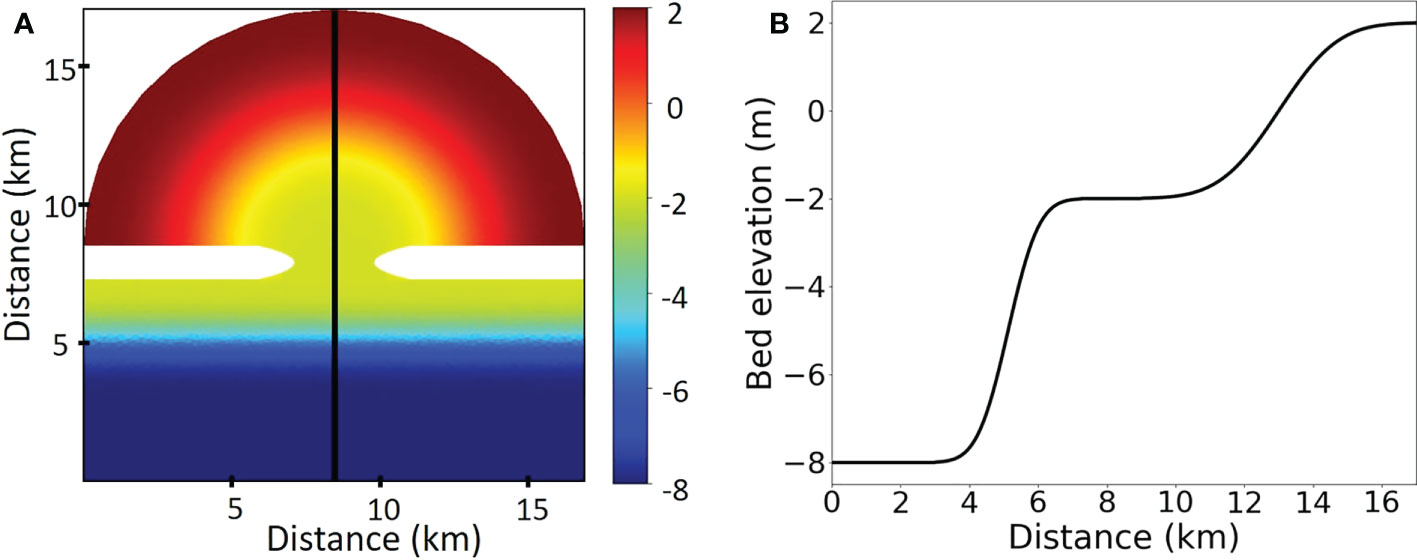
Figure 1 The initial bathymetry (unit: m) used in this study shown in aerial perspective (A) and cross-shore along the center line (B). The blank areas in (A) indicate unerodible lands above the water limit.
Hydrodynamic module
The 3-dimensional semi-implicit modeling system SCHISM was used to simulate hydrodynamics (Zhang et al., 2016). It solves the Reynolds-averaged Navier-Stokes equation on an unstructured grid employing a Garlekin finite element method (FEM) for horizontal and a finite volume method (FVM) approach for vertical velocities. Turbulence closure is implemented according to the k-kl closure scheme described in Umlauf and Burchard (2003).
Sediment module
The sediment model SED3D (Pinto et al., 2012) is part of SCHISM. Sediment is divided into multiple classes, each with a characteristic grain size. Cohesive and non-cohesive sediments are distinguished. Non-cohesive sediments (sands) can be transported in both suspension and bed load depending on the shear stress and settling velocity, while cohesive sediment (clay, silt and organic detritus) is transported in suspension. Transport of each pre-defined sediment class is computed independently.
Due to the cohesion arising from electrochemical surface charges and extracellular polymeric substances (EPS) secreted by benthic organisms including diatoms and bacteria, fine-grained particles can aggregate into flocs with settling velocity increased by orders of magnitude (Mikkelsen and Pejrup, 2000). The processes of flocculation depend on a variety of parameters including salinity, suspended sediment concentration (SSC), turbulence shear , organic matter component and temperature (Manning et al., 2011; Klassen, 2017; Zhang et al., 2020). In this study, the impact of flocculation on sediment settling velocity is considered. Settling velocity of cohesive sediment is calculated by the following Equation 1, which has been proven robust in capturing sediment dynamics in the Ems and Weser estuaries (Malcharek, 1995; Weilbeer, 2005):
where w0 is a reference settling velocity in still water depending on the particle grain size, m=1 and n=100 are empirical constants, and G is the turbulence shear.
To account for morphodynamics, the seabed is represented by a dynamic layered system adopted from the model ROMS (Warner et al., 2008). Sediment layers (thickness, age, fraction of each sediment class) at each grid cell are modified at each computational time step according to deposition or erosion fluxes.
In mixed seabed with both sandy and muddy particles, sediment stability changes dramatically when a certain critical mud content pcrit is exceeded which marks the transition from non-cohesive to cohesive seabed (Van Ledden, 2001). The value for the critical mud content is highly dependent on the lithology of clay and ranges between 5% to 30% of mud (Van Ledden, 2002). The formulation by Van Ledden (2003) was adopted to calculate the in situ critical shear stress Tc:
where pm is the mud content, Tc,s and Tc,m are the critical shear stress for resuspension of sands and mud, respectively, and γ=1 is an empirical constant.
Benthic fauna module
The benthic fauna module represents a novel component in the 3D model system (Figure 2). It incorporates (1) growth/decline functions of benthic fauna in response to environmental drivers food availability, (2) bioturbation and related effect in vertical transport of particulate organic carbon in sediments as well as effects in sediment stability (bio-destabilization), (3) bio-deposition, and (4) bio-stabilization. Major model functions relevant to this study are introduced in the following sub-sections, with technical details (numerical implementation schemes) provided in the Supplement Information.
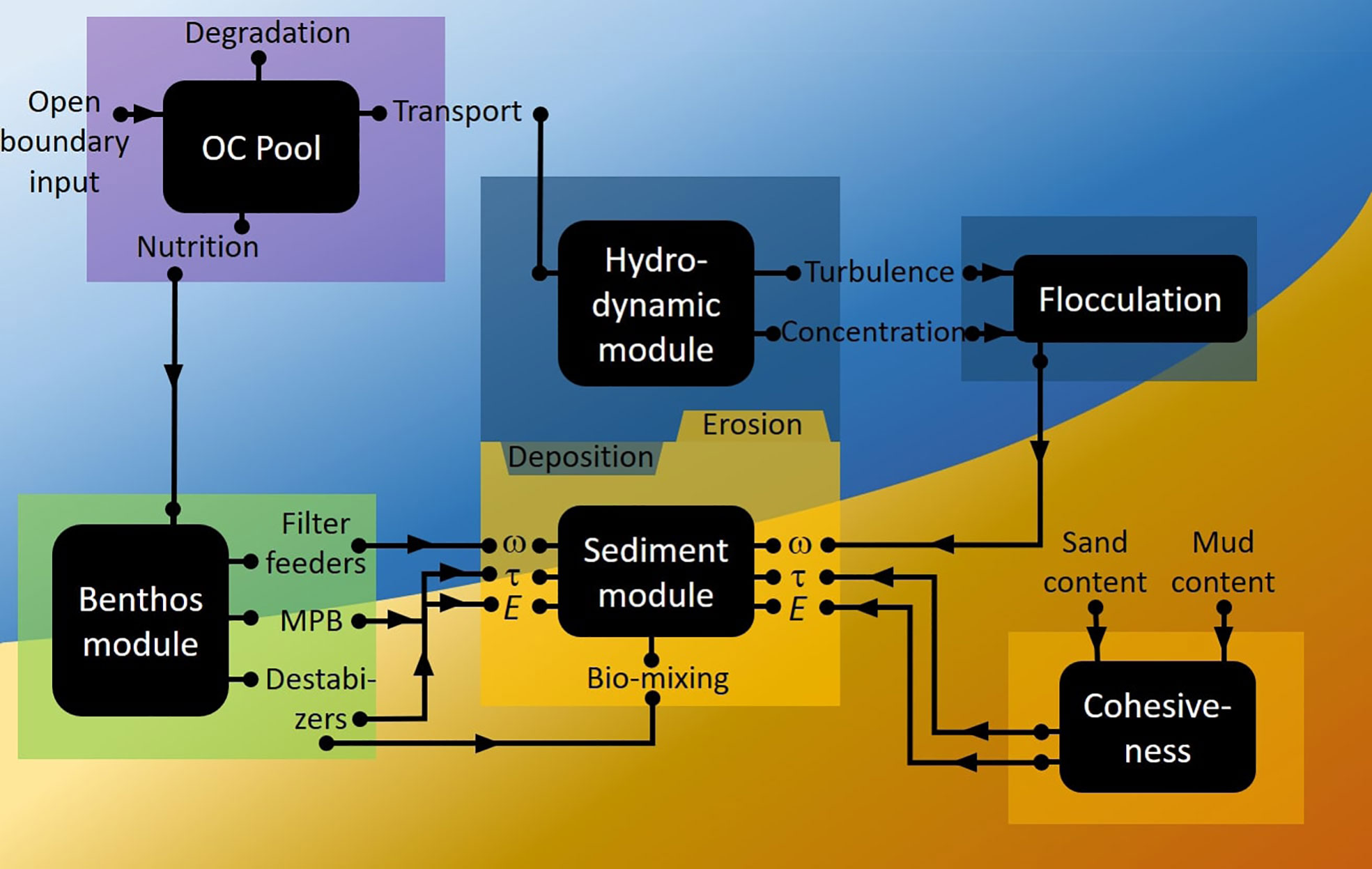
Figure 2 Schematic overview of numerical model components and their coupling. The hydrodynamic and sediment modules are from the SCHISM model, while other components were integrated to the SCHISM model as module (benthic fauna module) or subroutines (flocculation of cohesive sediments and organic detritus). Exchange of parameters between the model components is indicated by the arrows.
Benthic infauna and bioturbation
Algorithms for calculating the growth/decline of benthic infauna and associated bioturbation intensity are adopted from the Total Organic Carbon-Macrobenthos Interaction Model (TOCMAIM) developed by Zhang and Wirtz (2017). The model mechanistically links benthic infaunal biomass and bioturbation intensity to food quantity and quality as well as constraint by stressors (mortality caused by predators and oxygen deficiency). Food quantity and quality are represented by the content and lability of particulate organic carbon (POC), respectively. The theoretical basis of the model is built on the hypothesis that (a) the community structure of benthic infauna is mainly dependent on the quality of POC settled on the seafloor, which further controls the intensity of bioturbation; (b) bioturbation in turn affects the vertical transport of POC in sediments; and (c) the vertical positioning of benthic infaunal biomass reflects a trade-off between benefits (i.e., quantity and quality of food) and costs (i.e., respiration and mortality). Details of model principles and mathematical descriptions, sensitivity analysis of model parameters and application to station data are elucidated in Zhang and Wirtz (2017). Model applications to case study areas (North Sea) were introduced in Zhang et al. (2019) and Zhang et al. (2021).
In the model, POC is divided into three pools depending on the degradability, namely, labile (i.e., of high quality nutrient), semi-labile (i.e., of intermediate quality nutrient), and refractory (i.e., of low-quality nutrient). These three POC pools are modeled as cohesive sediment classes, with each characterized by a first-order degradation rate (representing a sink term). Degraded POC provides a source for inorganic cohesive sediment class. The mass balance equation of each sediment class in seabed taking into account the impact of bioturbation, deposition/erosion caused by bottom currents and degradation of POC is calculated by:
where Cs,i is the mass concentration of class i in sediment depth z, p is the sediment porosity, w is the deposition/erosion rate, and Db is the bioturbation diffusivity. The source term on the right hand side of the equation refers to the transformation of organic sediment to inorganic sediment (i.e., Source = 0 for all organic cohesive sediment and sand classes, and > 0 for inorganic cohesive sediment class), and the sink term refers to loss of organic cohesive sediment due to degradation and uptake of benthic infauna. Temporal change of benthic infaunal biomass B is calculated by:
where G and L represent the rate of gain and loss, respectively. The former is dependent on available food resources and temperature, and the latter is controlled by respiration and mortality (Zhang and Wirtz, 2017).
Bioturbation diffusivity Db scales with the local benthic infaunal biomass through a power law and inversely proportional to local food resource (i.e., the three POC pools):
where β, b are empirical parameters linking body-size and abundance to biomass (Zhang and Wirtz, 2017). ai (i=1, 2,3) are coefficients representing the efficiency of the POC pool in terms of gaining benthic infaunal biomass, with higher quality POC gaining biomass more efficiently as expressed by a1>a2>a3. The above formulation explicitly links the bioturbation diffusivity with (1) the body-size and abundance of benthic infauna (through scaling with biomass), and (2) the local food resource which determines the intensity of vertical movements of benthic infauna to derive enough nutrition for metabolism and growth.
TOCMAIM is coupled to the sediment module adopting the same dynamic seabed layer scheme (section 2.2) so that a two-way exchange between hydro-morphodynamics and benthic faunal functioning is ensured (Figure 2). In this study, all empirical parameters and coefficients of the benthic infauna module including those in Equation 3-5 are adopted from the field application in Zhang et al. (2021) listed in Table 2. As shown in Equation 3, bioturbation is considered as a diffusive process in the seabed sediment module. Not only the mass of each sediment class but also associated properties (e.g. median grain size and mud content) are mixed by bioturbation, which consequently modify the critical shear stress for sediment resuspension. Such mixing effect in sediment properties is termed biomixing. Biomixing has a destabilization effect in sediment because it loosens the upper-most sediment layers (normally up to 20-30 cm deep in sediments) where bioturbation exists (Sanford, 2008). The numerical scheme to implement the destabilization effect of biomixing in sediments and the empirical constants is explained in Supporting Information.
Bio-deposition
The benthic infauna model (section 2.3.1) provides estimates on the growth and decline of biomass in response to deposition and erosion of POC caused by bottom currents but does not account for deposition induced by benthic fauna themselves (so-called bio-deposition). The presence of suspension and filter feeders such as mussels effectively increases the settling velocity of sediment particles in the bottom most water layer. The magnitude of resulting bio-deposition of sediments depends on the filtration rate, ingestion rate and biomass of suspension/filter feeders. To account for bio-deposition, the suspension feeder model from the (US Army Corps of Engineers, 2000) was added as part of the benthic fauna module (Figure 2). Temporal change of the biomass S of suspension/filter feeders is given by:
where g is the growth rate, r and φ are the loss rates cause by respiration and predation, respectively. The growth rate depends on the ingestion rate I, the concentration of suspended POC in bottom water, and respective nutritional value ai for the POC class i as defined in Equation 5. Parameterizations of g, r and β are provided in Supporting Information. The rate of bio-deposition is then calculated by:
Bio-stabilization
A major contribution to sediment stabilization is made by extracellular polymeric substances (EPS) secreted by benthic organisms including microphytobenthos (e.g. diatoms) and bacteria that are correlated with Chlorophyll-a content in the sediment (Arlinghaus et al., 2021). The approach from Paarlberg et al. (2005) was adopted to include bio-stabilization by relating the critical shear stress for erosion and erosion rate Er to the stabilization functions fT and fE associated with EPS:
where Tc and Er are the reference values of the critical shear stress for erosion (see Equation 2) and the erosion rate without biological impact, respectively. Chl- normally degrades with a decay constant between 7/yr and 30/yr (Stephens et al., 1997), which is close to that of the labile POC class (20/yr) (Zhang and Wirtz, 2017). Therefore, it is assumed that Chl- is contained only in the fresh, labile POC class in our model. A constant ratio of labile carbon (unit: g) to Chl- (unit: μg) as 40 found by (Jakobsen and Markager, 2016) is used to estimate the content of Chl-a in sediments, which is then used to calculate fT and fE (Paarlberg et al., 2005) given by:
Model configuration
The total domain size is 17 by 17 km² (Figure 1). The horizontal resolution of each grid cell is about 150 m in the tidal inlet and the basin, and gradually decreases offshore to 300 m at the seaward boundary. Eleven equidistant vertical layers using the Generalized sigma coordinates (Song and Haidvogel, 1994) were used. Configuration of key model parameters is given in Table 1.
The initial seabed material consists of uniform sands with D50 = 120 μm. Cohesive sediments (inorganic and organic classes) are imported from the seaward boundary (30 mg L-1 for inorganic mud and 10 mg L-1 for labile POC). This concentration of suspended particulate matter (SPM) represents a typical value in coastal waters (Pleskachevsky et al., 2005). In total five sediment classes are considered in the study and their corresponding reference values of settling velocity, critical shear stress for erosion and erosion rate are listed in Table 2.
The model is forced by a semidiurnal tide at its seaward boundary with an amplitude of 1.5 m. Higher-order components are produced by bottom friction and current-topography interaction during the propagation of the tidal wave through the model domain. The chosen value for the tidal amplitude at the open boundary is comparable to the average tidal amplitude of 1.42 m at the mouth of a tidal embayment (Jade Bay) in the German Wadden Sea measured by Bundesanstalt für Gewässerkunde (BfG). Morphological acceleration in updating the bed level change is used to save the computational time so that a stable state of coastal morphology can be reached more rapidly. Sensitivity runs using an acceleration factor of 10 indicate similar results to that of 40 but requires much longer computational time. Larger values produced spurious features such as random pockmarks and mounds. Therefore an acceleration factor of 40 was used in all simulations presented in this study. Benthic fauna induced bed level change is added up to the hydrodynamics induced change in the same grid cell at every time step. Wind-waves, Coriolis force, effect of temperature and seasonality on growth and decline of benthic fauna were excluded in order to reduce complexity of the model.
Channel detection and extraction
Morphological change of the embayment driven by tides is characterized by development of complicated channel network, as is the case in nature. In order to quantify the morphological changes, a channel detection method was developed based on image analysis inspired by Passalacqua et al. (2010). The stepwise procedure is illustrated in Figure 3 and utilizes the python library OpenCV (Gracia et al., 2015; Guillen, 2019). As a first step the raw image was converted into a greyscale image with pixel values between 0 and 255. In order to remove background noise while preserving the channel structures, anisotropic diffusion (Perona and Malik, 1990; Borroto-Fernandez et al., 2013) was applied to the greyscale image. Next, an adaptive thresholding method was used to create a binary image depending on the averaged local pixel values. Some channels might get disconnected after the adaptive thresholding. To solve this problem, a correction algorithm was then performed to reconnect those channels and remove irrelevant features (e.g. islands at both sides of the tidal inlet). The new image was then turned into a one-pixel-width representation. Intersections and end points of all channels were subsequently identified by counting the non-zero neighbors of each pixel. All intersecting pixels were then changed to zero to identify different branches. The total number of channels as well as the length of each channel branch were finally quantified as the last step in the procedure (Figure 3). The derived values serve as a database for our analysis of simulation results.
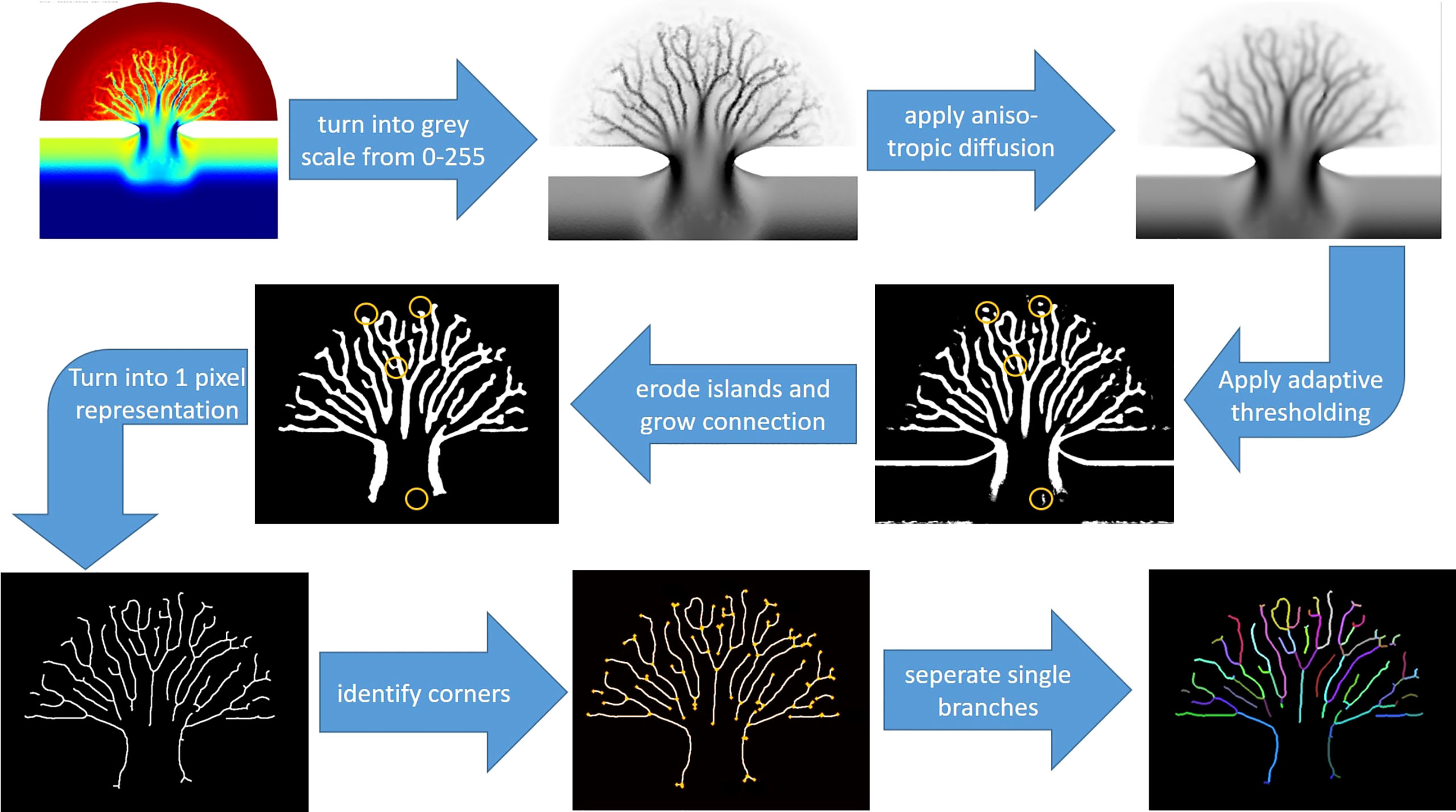
Figure 3 A step-by-step procedure of the channel extraction method is shown starting from a color image leading to the identification and extraction of all channel branches. The python library OpenCV is utilized.
Scenarios for modeling
Previous studies have revealed a significant control by bottom slope, tidal current strength and sediment properties (Marciano et al., 2005), sea level rise (Van Maanen et al., 2013b), coastal vegetation (Van Maanen et al., 2015), initial bathymetry and tidal forcing (Van Maanen et al., 2013a) on morphological development of tidal embayments. In this study, we focus on the impact of benthic fauna which has not been investigated yet in tidal embayment systems. Benthos is represented by three major functional groups (as described in section 2.3) instead of specific species. To this end, simulation results of an abiotic scenario by switching off the benthic fauna module in the 3D model system are used as the reference results. Four other simulations which include the benthic faunal impacts individually or jointly, named as Bio_destabilization, Bio_stabilization, Bio_deposition and Bio_all, are then compared with the reference results (named as Reference) for a quantitative analysis.
Results
Morphological development
Starting from the initial bathymetry (Figure 1), all simulations show a quick morphological development in the first 100 years especially in the first few decades (Figure 4A). Sediment scouring in the tidal inlet due to accelerated flood currents leads to a massive redistribution of sediment over the entire tidal basin within the first few years. During ebb-tides large amounts of sediment are transported offshore and exported out of the embayment through the inlet, subsequently deposited and forming an ebb tidal delta (Figure 5). The development of channel network in the embayment starts with the formation of two main channels at the inlet, which become increasingly deeper and gradually extend into the basin. During the extension of the main channels, secondary channels in the form of bifurcations appear, and further develop into more bifurcations when they extend to shallower area, ultimately forming a complex of channel network in all simulations (Figure 5). Following a rapid morphological development in the first few decades, a general pattern of the channel network is established and the speed of development slows down. In the scenarios Bio_stabilization, Bio_deposition and Bio_all, a stable state in which sediment import and export through the inlet are balanced is reached after simulation of 500 years (Figure 4A). In the other two scenarios (Reference and Bio_destabilization) a stable state is not yet reached after simulation of 500 years and the embayment is still governed by a net sediment export (i.e. erosion). However, statistical results indicate that a clear spatial distribution pattern of channels is established at this time and shows only minor changes afterwards in both scenarios (Figure 6). Based on the relatively stable morphology established after year 500 in all scenarios, and for the sake of affordable computational effort, the simulation results till year 500 were analyzed to understand the role of benthic fauna in mediating long-term coastal morphological evolution at an embayment-scale.
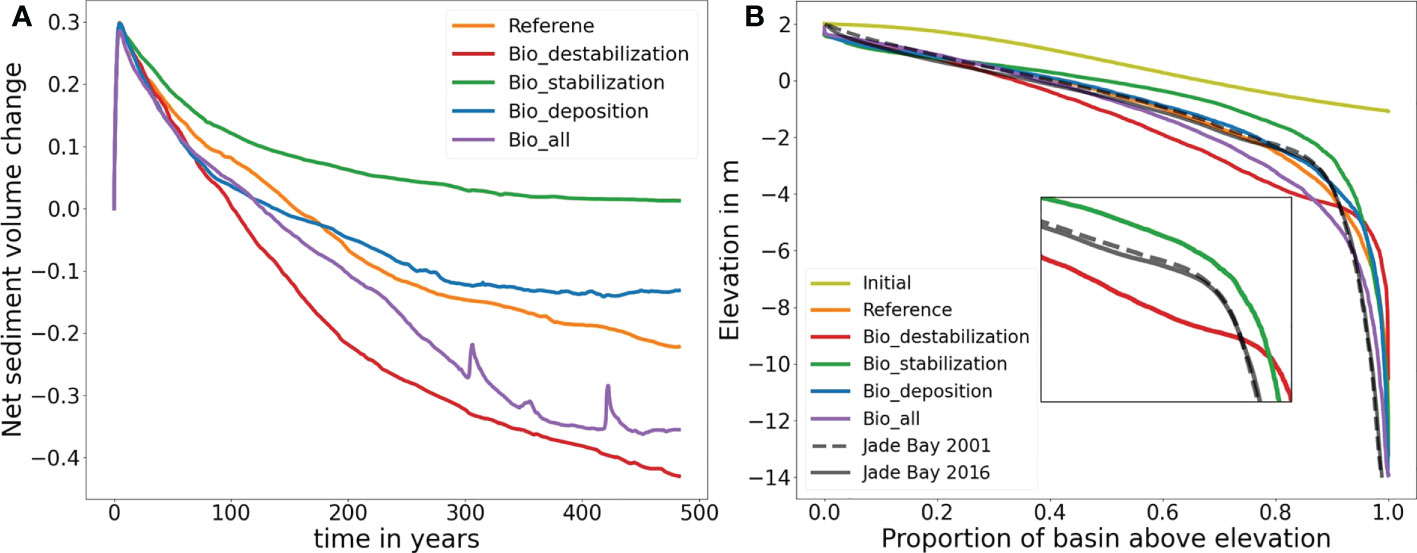
Figure 4 (A) Temporal evolution of the tidal embayment in all simulations with regard to the net sediment volume change at the basin (normalized by the initial total water volume in the basin; negative value implies export). (B) Hypsometric curves of all simulation results after 500 years. Note that the hypsometry curve of a real tidal embayment (Jade Bay in the Wadden Sea) for two different years 2001 and 2016 (data source: Sievers et al., 2020) are also plotted in (B) for comparison.
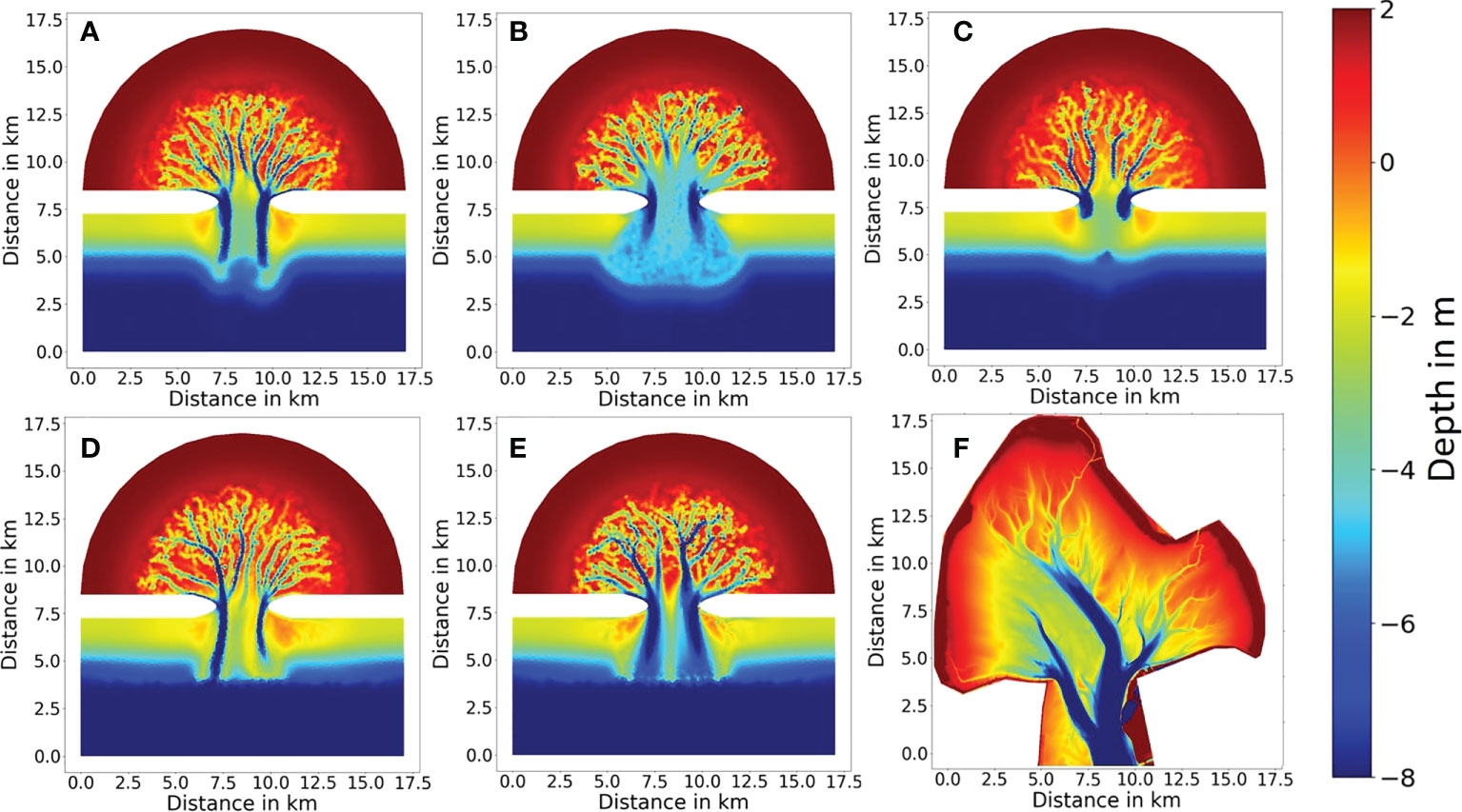
Figure 5 Simulated morphology at year 500 in (A) Reference run, (B) Bio_destabilization, (C) Bio_stabilization, (D) Bio_deposition, and (E) Bio-all. Bathymetry of a real tidal embayment (Jade Bay) is shown in (F) (data source: Sievers et al., 2020). Unit is m. Note that the belt-like deposition on the offshore side of the unerodible islands in all simulations is due to an exclusion of alongshore transport in the model.
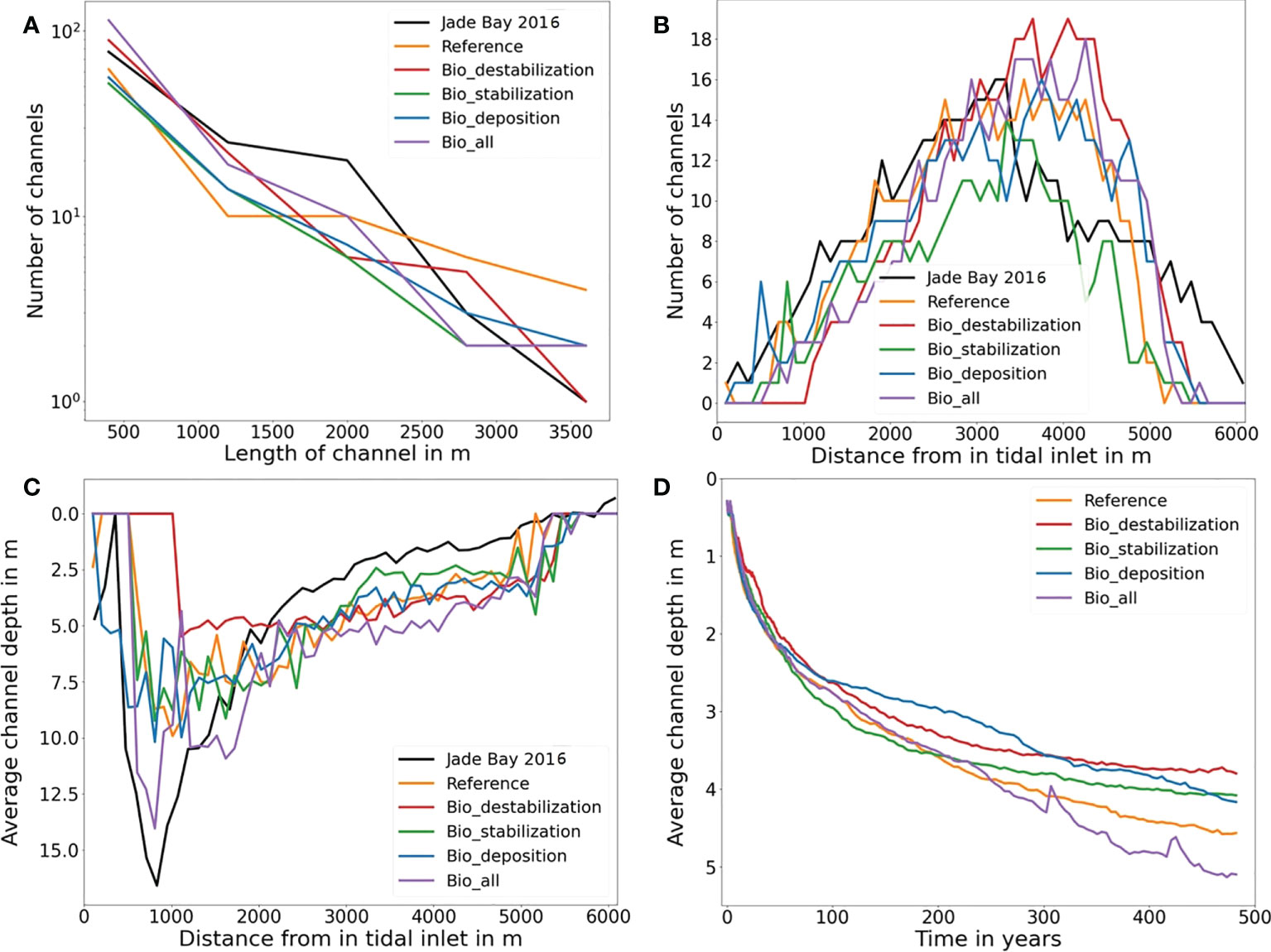
Figure 6 Statistics of channels in all simulations at year 500. (A) Number of channels in relation to the channel length. Note that the y-axis is in logarithmic scale. (B) Number of channels in relation to the distance from the inlet. (C) Average channel depth in relation to the distance from the inlet. (D) Change of average channel depth with simulation time. Note that statistics of channel in a natural tidal embayment (Jade Bay) are also included in (A–C).
Channel network
Statistics of channels in all simulations shown in Figure 6A indicate that the relationship between channel length and number of channels in each of the scenario follows an exponential function, which is consistent with the concept proposed by Horton (Horton, 1945; Strahler, 1953). Along with a consistent decrease in channel length with distance from the tidal inlet, the number of channels increases until a maximum is reached, and then start to decrease with further distance from the inlet (Figure 6B). The average channel depth shows an increasing trend when moving away from the inlet until a maximum is reached at a distance between 0.8-1.1 km from the inlet, and then decreases gradually with further distance from the inlet (Figure 6C). Temporal change of the mean channel depth shows that the value rises rapidly in the first 100 years in all simulations, and gradually slows down afterwards. In three scenarios (Reference, Bio_deposition and Bio_all) the mean channel depth still continue to increase at year 500 with a small rate, while in the other two scenarios (Bio_destabilization and Bio_stabilization) the values approach a stable level (Figure 6D).
Reference result - abiotic scenario
Both the simulated morphology and statistics of channel networks (Figures 4–6) indicate significant differences among the simulations. In the abiotic scenario (Reference run), two similarly long and deep channels develop in the inlet (Figure 5A). Tides passing through the narrow inlet creates strong currents reinforcing the deepening of the main channels. They extend into the tidal basin and bifurcate into various channels which become smaller and shallower along a landward extension. The average channel depth in the entire basin is 4.5 m at year 500 (Figure 6D). The maximum number of channels is reached at approximately 4 km from the tidal inlet which is similar to the result in Van Maanen et al. (2013b). The highest order in the channel network is four, which is also in line with the study by Marciano et al. (2005).
The scenario is ebb-dominated with a net export of sediment over the whole course of the simulation period (500 yrs, see Figure 4A). Due to a lack of biomixing between sediment layers, all deposited mud accumulates on the seabed surface until being resuspended or buried beneath new deposits. As consequence, mud content is very high in the surface sediment layer at the tidal flat (outside the channels). At year 500, mud content takes up more than ~10% in most tidal flats and at some places even close to 100% (Figure 7A). In contrast, mud content is normally within a few percentage in the channels due to energetic tidal currents which impede deposition. As mud content increases, the seabed sediment becomes more resilient to hydrodynamic erosion (see Equation 2), which further facilitates accumulation of mud and stabilizes the morphology of the embayment.
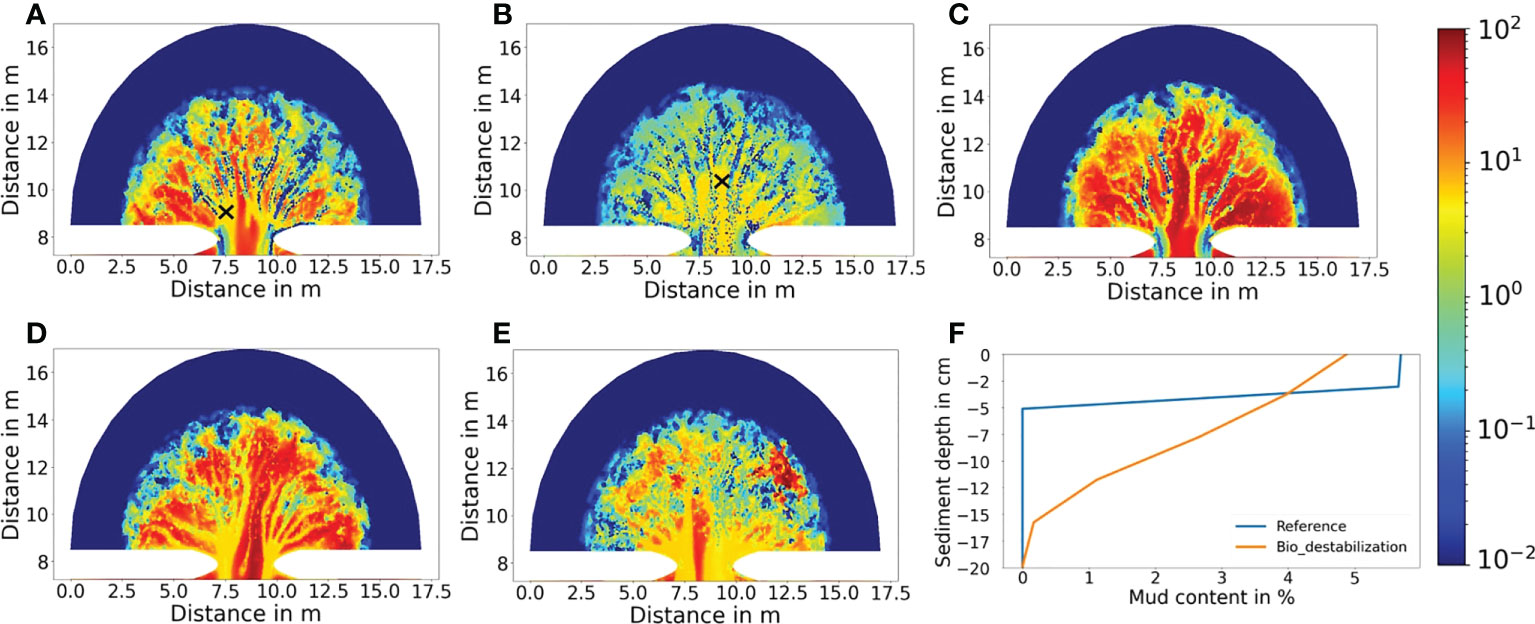
Figure 7 Simulated mud content (in percentage) at year 500 for the (A) Reference result, (B) the Bio_destabilization scenario, (C) the Bio_stabilization scenario, (D) the Bio_deposition scenario and (E) the Bio_all scenario. (F) Comparison of typical vertical profile of mud content in seafloor sediment at tidal flats between the reference result (exclusion of benthic faunal impact) and the Bio_destabilization scenario (inclusion of bio-mixing). Locations of the sites are indicted by X mark in (A) and (B), respectively.
Impacts of benthic fauna
Bioturbation and biomixing
Simulated morphological development of the tidal embayment with a destabilization effect caused by benthic fauna through bioturbation (i.e. the Bio_destabilization scenario) shows a remarkable difference with the reference result (Figures 5A, B). Compared to the reference scenario in which the two main channels at the inlet are deep, with a large depth gradient at their banks and long extension offshore, the two main channels in the Bio_destabilization scenario appears less distinctive with a relatively small depth gradient at the banks and much shorter in their length. The average channel depth in the entire basin is 3.7 m, being the smallest among all scenarios. The spatial gradient of depth in the channel network is also lowest among all scenarios. On the other hand, the channels are wider than the reference result. The embayment area within 2.5 km from the inlet is featured by erosion which makes the channels less distinctive and the first channel bifurcations occur further onshore than the reference results (Figure 5B). Compared to the reference results, less long channels (length > 1.5 km) whilst shorter channels (length < 1.5 km) develop in the Bio_destabilization scenario (Figure 6A). The hypsometric curve of Bio_destabilization in Figure 4B shows that the basin as a whole is deeper although the main channels are shallower than the reference result. This is accompanied by a decrease in area of intertidal flats. A unique feature in the hypsometric curve of the Bio_destabilization scenario is a plateau indicating a considerable portion (~15%) of the embayment area within water depth between 4 and 5 m (Figure 4B), which corresponds to the erosional semicircle area within 2.5 km from the inlet (Figure 5B).
The above-mentioned differences are attributed to a reworking of sediments by benthic fauna. Input of organic carbon driven by tides through the inlet and subsequent deposition in the embayment (Figure 8B) fosters a growth of benthic fauna with biomass between a few tens and a few hundred g C m-2 in the tidal flat (Figure 9A). In contrast, benthic faunal biomass is persistently low (<10 g C m-2) in the channels due to lack of deposition. Mud and POC are vertically mixed into deeper layers of sediment by benthic fauna through bioturbation (Figures 7B and 8B). As consequence, mud content in the upper-most surface sediment layer is generally below 10% over a major part of the tidal flat, much smaller than the reference result (Figure 7B). Reduction of mud content together with a loosening effect on the sediment caused by biomixing leads to a decrease of critical shear stress for resuspension, which makes surface sediment more susceptible to erosion and transport. To adapt to the impact of bio-destabilization, the basin morphology evolves in a way that less mud and POC are imported to the basin (so that benthic fauna becomes less leading to less bio- destabilization) and more sediment is exported out of the basin compared to the reference result (Figure 4A). The channel widening in the Bio_destabilization scenario is also caused by a dynamic feedback between deposition, benthic facilitated erosion and hydrodynamics. In the tidal flat area that is in a direct vicinity of the channels, a reduction of current velocities allows deposition of mud and POC, which feeds a growth of benthic fauna there. However, subsequent bio-destabilization caused by benthic fauna facilitates sediment resuspension, causing an erosion at the channel banks. As consequence, the channels become wider instead of deeper compared to the reference result. Deposition of mud and POC as well as resultant benthic faunal biomass are spatially re-organized until a balance between deposition and erosion is established. This feedback mechanism is responsible for creation of the erosional area within 2.5 km from the inlet (Figure 5B) and accounts for wider but shallower channel morphology than the reference result. Bio-destabilization also leads to generation of more short and lower order channels (Figure 6A).
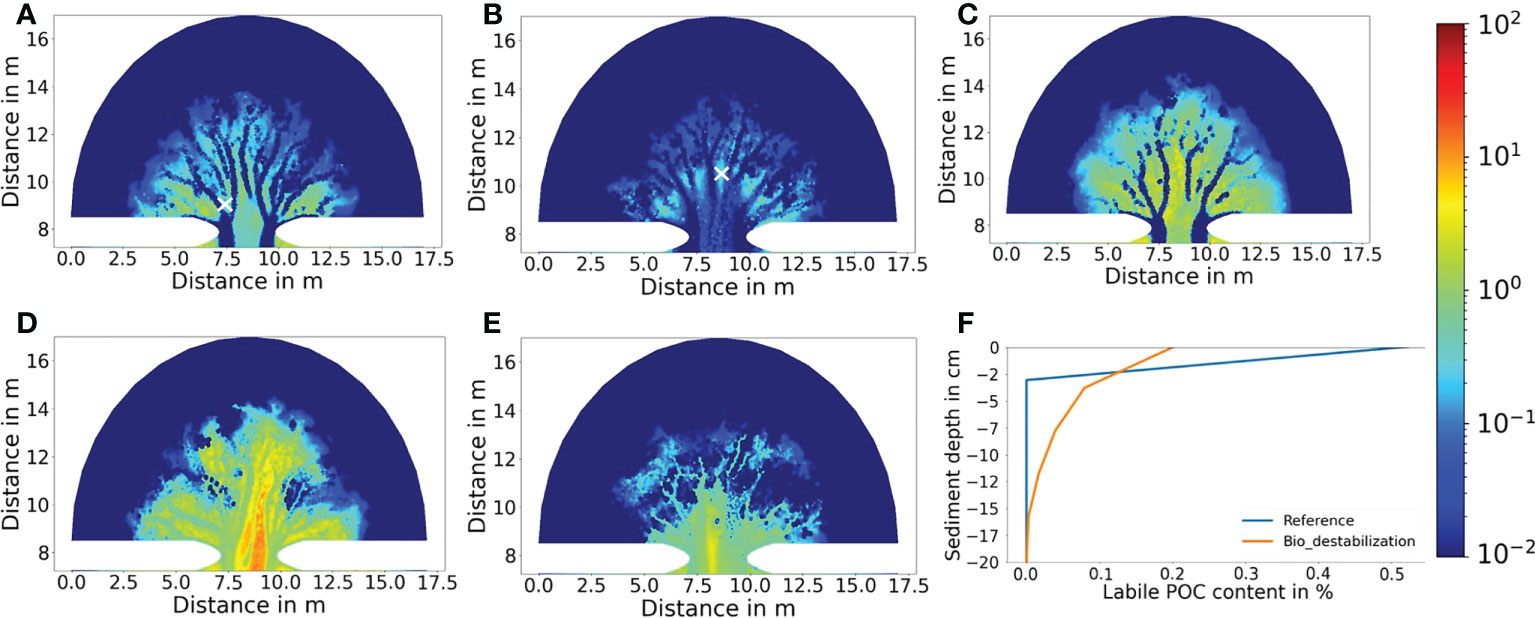
Figure 8 Simulated labile POC content in percentage at year 500 for the (A) reference result, (B) the Bio_destabilization scenario, (C) the Bio_stabilization scenario, (D) the Bio_deposition scenario and (E) the Bio_all scenario. (F) Comparison of typical vertical profile of labile POC content in seafloor sediment at tidal flats between the reference result (exclusion of benthic faunal impact) and the Bio_destabilization scenario (inclusion of bio-mixing). Locations of the sites are indicted by X mark in (A, B), respectively.
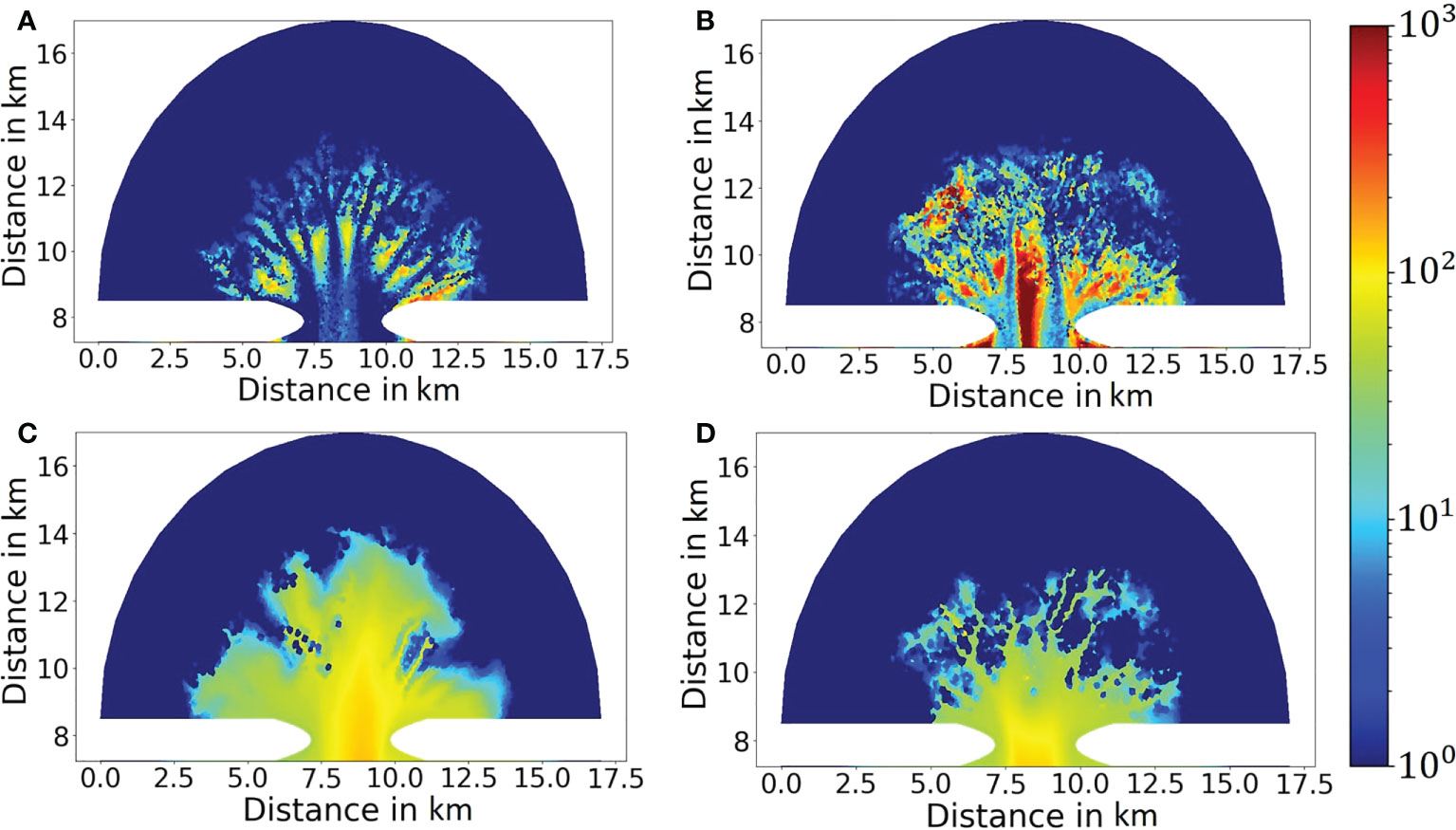
Figure 9 Biomass (unit: g C m-2) of destabilizing benthos in the Bio_destabilization scenario (A) and the Bio_all scenario (B). Biomass of filter/suspension feeders in the Bio_deposition scenario (C) and the Bio_all scenario (D).
Bio-stabilization
Bio-stabilization caused by benthos and POC on sediment also has a profound impact on long-term morphological development of the tidal embayment (Figure 5C). The effect is opposite to the Bio-destabilization scenario. Time series of calculated net sediment volume change suggest that in this scenario a stable state is met before year 500 (Figure 4A). The hypsometric curve of this scenario (Figure 4B) indicates that the tidal basin exports much less sediment than other scenarios, resulting in a higher overall elevation of the tidal embayment compared to other scenarios and a larger intertidal flat area. Import of mud from offshore area and subsequent deposition increases both the mud content and bio-stabilization effect associated with POC content. These jointly lead to a higher threshold for sediment resuspension and seabed becomes more resilient to erosion. Therefore, the two main channels at the inlet are much shorter compared to the reference result (Figure 5C). The entire embayment becomes increasingly stable along time and impedes the development of low-order bifurcations. This explains why the total number of channels in this scenario is smallest among all scenarios (Figures 5C and 6B). Since tidal currents are confined in fewer major channels, enhanced current velocity during peak tidal flows leads to a scouring in the channels. The average channel depth is 4.1 m at year 500 (Figure 6D). However, this value is smaller than the reference result (4.7 m). This is mainly due to the stabilization effect which is also present in lower order channels, where tidal currents are less energetic and allow more deposition of mud and POC than in higher order main channels (Figure 7C).
Benthic stabilization exerted by deposited mud and POC gradually changes the embayment geometry. Due to the stabilization effect less sediment is eroded in the basin and meanwhile deposition of imported mud and POC in the tidal flats is increasingly facilitated. Furthermore, altered hydrodynamics increase the overall concentration and import of mud and POC to the basin compared to the reference scenario (see Supplement Information). These jointly lead to a net sediment import to the embayment, and an increased basin elevation. Both mud content and POC content are strongly increased in the seabed surface sediment compared to the reference scenario (Figures 7C and 8C). In a large portion of the tidal flats mud content even approaches 100% in the surface sediment layer at year 500.
Bio-deposition
The impact of bio-deposition caused by suspension and filter feeders on morphological development of the embayment (Bio_deposition scenario) shows both similarities as well as significant differences to the Bio_stabilization scenario. The average channel depth is 4.15 m at year 500 in this scenario (Figure 6D), being very close to the result (4.1 m) in the Bio_ stabilization scenario. High mud and POC content are also seen in a large portion of the tidal flats (Figures 7D and 8D). The hypsometric curve of this scenario lies in between the curves of the reference result and the Bio_stabilization scenario (Figure 4B), indicating that bio-deposition is able to elevate the overall bed level of the embayment, increase the intertidal area and reduce net sediment export compared to the reference result. The statistics of number of channels with regard to the distance to the inlet (Figure 6B) shows a similar channel development pattern to the reference results within a distance of ~3.5 km from the inlet, but with more channels developed further away from the inlet. This indicates that bio-deposition may facilitate bifurcations in low-order channels.
A clear feature in the sedimentation of mud and POC in the Bio_deposition scenario, which marks its major difference with previously mentioned scenarios, is that mud and POC also accumulate inside the channels (Figures 7D and 8D). Mud content ranges from a few up to 10% in the main high-order channels and slightly decreases in secondary low-order channels. POC content shows a similar pattern with maximum value of ~2% in the main channels. Such abnormally high mud and POC content in the channels, in comparison to other scenarios, is attributed to bio-deposition. The simulated biomass of filter/suspension feeders ranges between 20 and 100 g C m-2 in the main channels as well as over the tidal flats between the channels (Figure 9C). The high biomass ensures a high ingestion rate of suspended particles in the bottom water layer and results in a high rate of bio-deposition (as implemented in Equation 7). The spatial distribution of filter/suspension feeder biomass shows a clear difference with that of bioturbating infauna (Figure 9A). This is because that the latter depends on abiotic (only related to bottom hydrodynamics) deposition of POC, which is favored in tidal flats but impeded in channels due to energetic tidal currents, while the former depends on the amount of suspended POC in the bottom water, which shows small difference between tidal flats and channels.
Combined effects of benthic fauna
In a natural embayment environment, all biological impacts are combined and interact with physical drivers. The hypsometric curve of the scenario which combines all biological impacts (Bio_all) lies in between the curves of the reference result and Bio_destabilization, indicating that in this scenario more sediment is exported out of the embayment than the reference result but the amount is less than that in Bio_destabilization (Figure 4B). Unlike the hypometric curve of Bio_destabilization which is characterized by a plateau corresponding to a similar depth (4-5 m) in the erosional semicircle area within 2.5 km from the inlet (Figure 5B), such pattern is not seen in the Bio_all scenario. Another clear difference is also seen in the channel morphology (Figure 5E). The two main channels at the inlet are deep and wide in this scenario, with relatively sharp boundary (large gradient in bathymetry) with adjacent tidal flats. The number of channels developed within 3.5 km from the inlet is similar to that of the Bio_destabilization scenario and smaller than the reference result. However, more channels develop further away from the inlet (> 3.5 km) in this scenario than the reference result, although being less than the Bio_destabilization scenario (Figure 6B).
The average channel depth is 5.1 m at year 500, being largest among all scenarios (Figure 6D). This is attributed to interactions between different benthic functional groups (bioturbators and filter/suspension feeders, Figure 11). The coexistence of bioturbators (which destabilize sediments) and filter/suspension feeders (which stabilize sediments) has a profound impact on the embayment morphology. Bioturbators are mainly distributed in the tidal flats while filter/suspension feeders are located in both tidal flats and channels (Figures 9B, D). However, biomass of bioturbators in this scenario is significantly higher than that in the Bio_destabilization scenario (Figure 9A), whilst biomass of filter/suspension feeders is remarkably lower than that in the Bio_deposition scenario (Figure 9C). This result indicates that filter/suspension feeders promote the growth of bioturbators (positive feedback), whilst bioturbators in turn would lead to a decline of filter/suspension feeders (negative feedback). Such feedback is caused by a change of food (POC) availability. Mud and POC content in surface sediments in the Bio_all scenario is higher than that in the Bio_destabilization scenario but lower than the Bio_deposition scenario (Figures 7, 8). This indicates that more food (POC) is transferred into sediment through bio-deposition compared to the Bio_destabilization scenario which excludes this process. The increased POC facilitates an increase of bioturbators in terms of biomass in both tidal flat and channel area. At some parts of the tidal flats (e.g. area between the main channels) the biomass of bioturbators is even increased by an order of magnitude (Figures 9A, B). As the biomass increases, bioturbators exert an increasing destabilization impact on sediment at both the channel edges and within the channel, leading to a widening and deepening of the channels (Figure 5E) compared to the Bio_deposition scenario. Cozzoli (2016) found that in strong hydrodynamic conditions, benthic bioturbation impact may increase at lower density of abundance because of a mutual feedback between hydrodynamics and benthic fauna. This is confirmed in the simulation results showing that the average channel depth is largest in the Bio_all scenario among all scenarios. A widening and deepening of the channels caused by bioturbators lead to a decrease of current velocity and sediment transport rate in the channels. As a consequence, POC availability to filter/suspension feeders is reduced, leading to a decline of their biomass compared to the Bio_deposition scenario (Figures 9C, D).
Discussion
Comparison with existing literature and real environments
Since our simulation results are based on idealized model domain and simplified forcing, a direct point-to-point validation of our simulation results against a real coastal system is not feasible. To assess the reliability of the model results, we divide the model domain into two zones, namely sub-tidal and inter-tidal zones, and compare the simulation results in these two zones with existing literature and data from the Jade Bay in the German Wadden Sea that shares similar environmental configuration (hydrodynamic forcing, domain size, sediment composition) with the idealized model setup in a qualitative and statistical manner. In particular, we focus on comparison of parameters that are relevant to benthic faunal impact, including the spatial distribution of biomass, functional groups, bioturbation intensity, bio-depositional rate and the magnitude of erosion/deposition thickness caused by benthic fauna.
Statistics of the Jade Bay exhibits similar distribution in channel network (Figure 6) and hypsometric curve (Figure 4B) with the simulation results. Biomass of benthic fauna in tidal embayments of the Wadden Sea is found to range between 1 and 100 g C m-2 (Beukema, 1974; Dekker, 1989; Essink et al., 1998). In the Jade Bay, measurements conducted in 1930s, 1970s and 2009 show that the spatially averaged biomass of benthic fauna varied between 12 and 20 g C m-2 (Schückel et al., 2015a). In 2009, the spatially averaged biomass in the Jade Bay was 19.2 g C m-2, with ~7 g C m-2 from filter/suspension feeders and ~12 g C m-2 from bioturbators (Schückel et al., 2015a). Compared to the measurement data in the Jade Bay, our simulation result (Bio_all) shows a larger spatially averaged biomass over the entire embayment area (~40 g C m-2), with local peak values up to a few hundred g C m-2 (Figure 9). However, although being larger than the measured value in the Jade bay, our simulation result is comparable to data from other tidal embayments. Measurements in the western and northern parts of the Wadden Sea show seasonal fluctuation of spatial average biomass between 10 and 90 g C m-2 (Reise et al., 1994; Beukema and Dekker, 2020). In local sites with high abundance of filter/suspension feeders such as mussel beds of M. edulis, biomass can reach to 2000 g C m-2 (Büttger et al., 2008). Therefore, the predicted biomass of benthic fauna in our simulation results lies in a reasonable range when compared to real tidal embayments. With regard to spatial distribution pattern of functional groups, existing literature suggested its correlation with environmental variables. In the Jade Bay, abiotic variables most strongly correlated to benthic faunal communities are tidal current velocity, water depth, mud and TOC contents, which together account for 35% of the total variation (Schückel et al., 2015b). In addition, Chlorophyll- content, which is correlated with fresh POC, appears to be another important variable significantly correlated with some bioturbating species (e.g. Peringia ulvae, Retusa obtusa) (Singer et al., 2016). These major influencing variables are taken into account in our model (section 2). The species distribution maps in the Jade Bay compiled by Singer et al. (2016) show that the inter-tidal flats are mainly dominated by bioturbating species (Tubificoides benedii, Peringia ulvae, Retusa obtusa), whilst sub-tidal channels are inhabited by both bioturbators (Tubificoides benedii, Scoloplos armiger) and filter/suspension feeders (Macoma balthica). Such distribution pattern is also reproduced in our simulation results (Figure 9).
Bioturbation diffusivity derived by fitting observed vertical distributions of tracers varies over 3 orders of magnitudes (10-2–10 cm2 day-1) among different seafloor settings in the North Sea including the Wadden Sea (Boon et al., 1998; Teal et al., 2008; Jørgensen and Parkes, 2010). In addition, bioturbation may vary by more than one order of magnitude even at the same site because of seasonal changes in food supply and in the metabolism of benthic organisms (Brown et al., 2004; Zhang et al., 2019). Compilation of existing measurements by Teal et al. (2008) indicates that the mean value of bioturbation diffusivity in the North Sea is 0.16 cm2 day-1. Given that the average biomass of benthic fauna in the southern North Sea is ~8 g C m-2 according to the ICES survey in 2000 (Rees et al., 2007), the higher abundance of benthic fauna in the Jade Bay (19 g C m-2) should result in higher bioturbation intensity than in the southern North Sea. Therefore a mean value of bioturbation diffusivity larger than 0.16 cm2 day-1 is expected in the Jade Bay, despite that actual field measurements in the Jade Bay are absent. Our simulation results indicate that the bioturbation diffusivity ranges between 0.01 and a 10 cm2 day-1 in the tidal embayment with a spatial mean value of 0.41 cm2 day-1 (Figure 10A), which may serve as a reference value for the Jade Bay and other embayments in the Wadden Sea to assess a regional impact of bioturbators in mediating particle fluxes. Modeled bio-deposition rates range from 0.1 to 100 g m-2 day-1 (Figure 10B). This value range is in line with studies of Mytilus edulis with bio-deposition rates between 0.2 – 1.2 g m-2 day-1 for sparsely populated small patches (Kautsky and Evans, 1987). Oyster beds of Cassostrea gigas are reported with rates of 40-180 g m-2 day-1 (Mitchell, 2006). Mussel beds in the North and Wadden Sea can reach several 100 g m-2 day-1 (Dittmann, 1987; Flemming and Delafontaine, 1994).
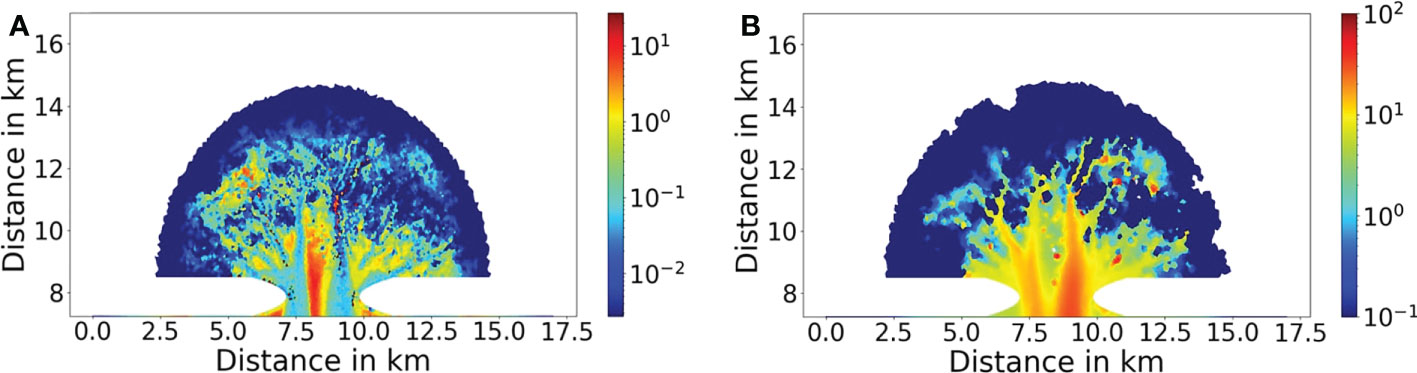
Figure 10 Bioturbation diffusivity (A, unit: cm2 day-1) by bioturbators and bio-deposition rate (B, unit: g m-2 day-1) by filter/suspension feeders in the Bio_all run.
A comparison of hypsometric curves of the Jade Bay between 2001 and 2016 (Figure 4B) suggests that the area underwent slight erosion during 2001-2016. The curve of 2016 shows a plateau at water depth of ~2 m which is the transition depth between intertidal (tidal flats) and subtidal (channel) areas. Such plateau is a characteristic of the scenario Bio_destabilization as described previously. On the other hand, this feature is not evident in the curve of 2001. A remarkable shift in proportion of functional groups between 1970s and 2009 was observed in the Jade Bay, with bioturbating deposit feeders (destabilizers) increased from ~ 20% to almost 70% (Schückel and Kröncke, 2013). Such increase of bioturbators might explain the plateau feature and decrease in the hypsometric curve of 2016, which is supported by our simulation results (Bio_destabilization).
Former bio-morphological modeling studies indicate that changes in deposition and erosion caused by benthic fauna can be in the same order of magnitude as changes caused by hydrodynamic drivers (Wood and Widdows, 2002). This is confirmed in our results. Existing investigations on short-term (monthly to decadal scale) impact indicate that the average thickness of deposition and erosion caused by benthic fauna ranged from a few cm (Wood and Widdows, 2002; Paarlberg et al., 2005; Lumborg et al., 2006) to a few tens of cm on a monthly scale (Paarlberg et al., 2005), from a few mm (Borsje et al., 2008) to a few tens of cm on an annual scale (Orvain et al., 2012) and several m on a decadal scale (Brückner et al., 2021). Such order of magnitude in morphological change and its time scale dependence are also seen in our simulation results. Further, our results indicate that the difference in average thickness of deposition or erosion between bioturbated and non-bioturbated scenarios is around 1 m at both the channel and tidal flats at year 500 (Figure 6). Such results imply that on a long-term (centennial) scale, the most significant impact of benthic fauna on large-scale coastal morphological development is a re-organization of the channel network and hypsometric distribution, rather than an absolute change in elevation.
Future research needs
Dynamic interactions between biota and landform have been recognized as a critical mechanism in controlling morphological evolution of complex coastal systems (Murray et al., 2008; Reinhardt et al., 2010; Marani et al., 2010). On the other hand, a recent review by Arlinghaus et al. (2021) pointed out that the development of bio-morphodynamic numerical models remains still at an initial stage and these models are of limited use for explanation and prediction of natural systems. The authors attributed the reasons to (1) lack of understanding of fundamental biological/bio-physical processes affecting morphological development and especially dynamic feedback loops among them, (2) scarcity in data for model calibration of biological/bio-physical processes, and (3) scarcity in data for confirming model results. An appropriate model complexity needs to be found in order to reconcile the limitations of process understanding on one hand and the ability to calibrate and validate the model on the other hand (French et al., 2016; Larsen et al., 2016). For this reason, most existing modeling studies only regard one-way control of benthic fauna on morphodynamics, and are focused on species level with a few species and small spatial scale (Arlinghaus et al., 2021). Some recent studies underline the necessity of differentiating between species of the same functional group and focusing on their mutual interactions (such as competition and grazing) and interaction with the environment (Brückner et al., 2020; Brückner et al., 2021). In an estuarine environment, Brückner et al. (2021) found that morphological change can be mainly driven by efficient bioturbators albeit with a small abundance, whilst highly abundant but less effective species only have minor impact. Direct interactions between these species determine each other’s abundance and indirect feedback over eco-engineering of the habitat creates suitable area for co-existing species. These results clearly highlight the importance of feedback control and implementation of species interaction in numerical modeling.
In this study, we propose a simplified implementation of multi-functional groups instead of species. Although no direct species interaction was implemented in the model, indirect feedback was mediated via the availability of food (POC). A negative feedback from erosion to bioturbators is a shortage of food that limits the increase of biomass and therefore would lead to a decrease of bioturbation and bio-destabilization. Sedimentation of mud and POC promoted by EPS and filter/suspension feeders is a positive feedback increasing the biomass of bioturbators. In addition, a geomorphological feedback arises between the benthos and the altered hydrodynamic conditions of the system towards a higher or lower sediment and POC import. This geomorphological feedback is guided by the relative expansion (Bio_stabilization, Bio_deposition) and reduction (Bio_destabilization) of tidal flats seen in the hypsometry (Figure 4B) which favors flood dominance and subsequent sediment import in the first case and ebb dominance and sediment export in the latter case (Zhou et al., 2017). Such indirect feedbacks between functional groups and between functional groups and morphology are illustrated in Figure 11. The positive geomorphological feedback of species promoting bio-deposition and stabilization may thus lead to a continuous growth of tidal flats which become increasing shallower over time, while at the same time the benthic faunal biomass continuously increase. Adding the negative feedback control of bioturbators may counteract this trend by preventing tidal flat expansion and reducing the food supply to deposition- and stabilization-promoting species. Therefore, the hypsometric curve of the Bio_all scenario lies in between the purely destabilized scenario on the lower limit and the purely stabilized scenario on the upper limit (Figure 4B). Furthermore, bio-stabilization and deposition may facilitate growth of bioturbators in hydrodynamically active regions, resulting in a larger channel depth (Figure 6D) and width (Figure 5E) when comparing Bio_all with the other scenarios. Clearly, more research efforts are needed to incorporate dynamic interactions between biota and landform as well as interactions between different functional groups of biota in quantitative analysis and modeling of coastal systems.
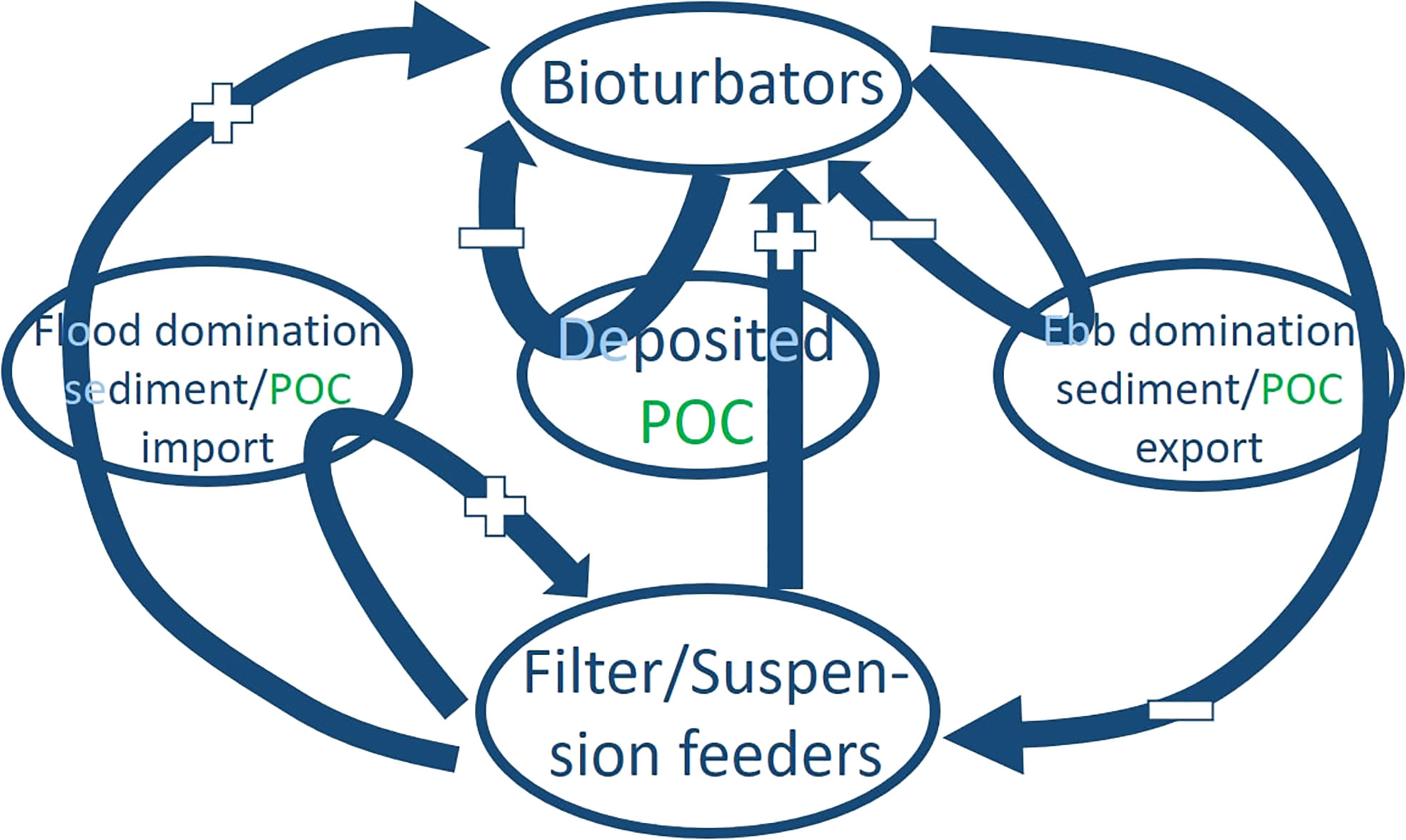
Figure 11 Illustration of feedback between two major functional groups in terms of growth (“+”) or decline (“-”) in biomass. Internal feedback within each of the group is also indicated.
Conclusions
By application of a novel 3-dimensional numerical model resolving dynamic interactions between environmental drivers and benthic fauna to a simplified tidal embayment configuration, this study aims to understand (1) the quantitative importance of benthic fauna in guiding long-term (decadal to centennial) coastal morphological evolution at a large and regional scale, (2) the individual and combined impact of multiple benthic functional groups in shaping coastal morphology and (3) interaction between benthic fauna and hydro-morphodynamics. Comparison with a real tidal embayment (Jade bay in the Wadden Sea) and existing literature justified the general validity of the model results, leading to the following conclusions.
Each of the investigated benthic faunal impacts, namely bio-destabilization caused by bioturbators, bio-deposition by filter/suspension feeders, and bio-stabilization associated with EPS secreted by benthic organisms including microphytobenthos and bacteria, may lead to a profound change of the embayment morphology in terms of development of channel network (channel depth, width, length and bifurcations) and overall elevation of adjacent tidal flats. Bioturbation-induced sediment mixing and bio-destabilization may result in a net sediment export out of the embayment, whilst bio-deposition and bio-stabilization tend to alter the embayment toward a net sediment import environment. A combined effect of these biophysical processes leads to a unique response of the embayment morphology due to interactions between different benthic functional groups. While bioturbators promote erosion which has a negative feedback (reduction of food) to their growth, a positive feedback exerted by suspension/filter-feeders increased the biomass and spatial abundance of bioturbators. In addition, a negative geomorphological feedback by bioturbators through enhanced erosion decreases the biomass of suspension/filter-feeders compared to the case in which bioturbators are absent.
From a modeling perspective, development of bio-morphodynamic models should consider not only dynamic interactions between biota and landform but also interactions between different benthic lifeforms, which is equally important in guiding morphological development of complex coastal systems.
Data availability statement
Publicly available datasets were analyzed in this study. This data can be found here: https://datenrepository.baw.de/startseite.
Author contributions
All authors conceived and designed the study. Numerical simulations were supervised by WZ and carried out by PA. PA and WZ analyzed results and wrote the paper. All authors contributed to manuscript revision. All authors approved the final version of the manuscript and agree to be held accountable for the content therein.
Funding
This study is a contribution to the I2B project “Unravelling the linkages between benthic biological functioning, biogeochemistry and coastal morphodynamics – from big data to mechanistic modelling “ funded by Helmholtz-Zentrum Hereon. It is also supported by the Helmholtz PoF programme “The Changing Earth – Sustaining our Future” on its Topic 4: Coastal zones at a time of global change.
Conflict of interest
The authors declare that the research was conducted in the absence of any commercial or financial relationships that could be construed as a potential conflict of interest.
Publisher’s note
All claims expressed in this article are solely those of the authors and do not necessarily represent those of their affiliated organizations, or those of the publisher, the editors and the reviewers. Any product that may be evaluated in this article, or claim that may be made by its manufacturer, is not guaranteed or endorsed by the publisher.
Supplementary material
The Supplementary Material for this article can be found online at: https://www.frontiersin.org/articles/10.3389/fmars.2022.1011760/full#supplementary-material
References
Alves R., Van Colen C., Vincx M., Vanaverbeke J., De Smet B., Guarini J. M., et al. (2017). A case study on the growth of lanice conchilega (Pallas 1766) aggregations and their ecosystem engineering impact on sedimentary processes. J. Exp. Mar. Biol. Ecol. 489, 15–23. doi: 10.1016/j.jembe.2017.01.005
Andersen T., Pejrup M. (2011). “Biological influences on sediment behavior and transport,” in Treatise on estuarine and coastal science, vol. 2 . Eds. Wolanski E., McLusky D. S. (Waltham: Academic Press), 289–309.
Angamuthu B., Darby S. E., Nicholls R. J. (2018). Impacts of natural and human drivers on the multi-decadal morphological evolution of tidally-influenced deltas. Proc. Mathematical Physical Eng. Sci. 474. doi: 10.1098/rspa.2018.0396
Arlinghaus P., Zhang W., Wrede A., Schrum C., Neumann A. (2021). Impact of benthos on morphodynamics from a modeling perspective. Earth-Science Rev. 221. doi: 10.1016/j.earscirev.2021.103803
Benninghoff M., Winter C. (2019). Recent morphologic evolution of the German wadden Sea. Sci. Rep. 9, 9293. doi: 10.1038/s41598-019-45683-1
Beukema J. (1974). Seasonal changes in the biomass of the macro-benthos of a tidal flat area in the Dutch wadden Sea. Netherlands J. Sea Res. 8, 94–107. doi: 10.1016/0077-7579(74)90028-3
Beukema J. J., Dekker R. (2020). Half a century of monitoring macrobenthic animals on tidal flats in the Dutch wadden Sea. Mar. Ecol. Prog. Ser. 8, 1–18. doi: 10.3354/meps13555
Boon A. R., Duineveld G. C. A., Berghuis E. M., van der Weele J. A. (1998). Relationships between benthic activity and the annual phytopigment cycle in near-bottom water and sediments in the southern north Sea. Estuarine Coast. Shelf Sci. 46 (1), 1–13. doi: 10.1006/ecss.1997.0264
Borroto-Fernández M., Mecias A., Hidalgo M. (2013). Image smoothing using the perona-malik equation with a new estimation method of the contrast parameter. Comput. Vision Med. Image Process. IV VIPIMAGE 2013. 23, 238–252. doi: 10.1201/b15810-13
Borsje B. W., de Vries M., Hulscher S., de Boer G. (2008). Modeling large-scale cohesive sediment transport affected by small-scale biological activity. estuarine. Coast. Shelf Sci. 78, 468–480. doi: 10.1016/j.ecss.2008.01.009
Brown J. H., Gillooly J. F., Allen A. P., Savage V. M., West G. B. (2004). Toward a metabolic theory of ecology. Ecology 85 (7), 1771–1789. doi: 10.1890/03-9000
Brückner M., Braat L., Schwarz C., Kleinhans M. (2020). What came first, mud or biostabilizers? Elucidating interacting effects in a coupled model of mud, saltmarsh, microphytobenthos, and estuarine morphology. Water Resour. Res. 56, e2019WR026945. doi: 10.1029/2019WR026945
Brückner M., Schwarz C., Coco G., Baar A., Boechat A. M., Kleinhans M. (2021). Benthic species as mud patrol - modelled effects of bioturbators and biofilms on large-scale estuarine mud and morphology. Earth Surface Processes Landforms 46, 1128–1144. doi: 10.1002/esp.5080
Büttger H., Asmus H., Asmus R., Buschbaum C., Dittmann S., Nehls G. (2008). Community dynamics of intertidal soft-bottom mussel beds over two decades. Helgol Mar. Res. 62, 23–36. doi: 10.1007/s10152-007-0099-y
Chen X., Zhang C., Townend I. H., Paterson D. M., Gong Z., Jiang Q., et al. (2021). Biological cohesion as the architect of bed movement under wave action. Geophysical Res. Lett. 48, e2020GL092137. doi: 10.1029/2020GL092137
Cozzoli F. (2016). Modelling biota-sediment interactions in estuarine environments. Dissertation. 111–136. doi: 10.13140/RG.2.1.3642.2163
Craft C. (2000). Co-Development of wetland soils and benthic invertebrate communities following salt marsh creation. Wetlands Ecol. Manage. 8, 197–207. doi: 10.1023/A:1008448620605
Dairain A., Legeay A., Montaudouin X. (2019). Influence of parasitism on bioturbation: from host to ecosystem functioning. Mar. Ecol. Prog. Ser. 619, 201–214. doi: 10.3354/meps12967
Dekker R. (1989). The macrozoobenthos of the subtidal western dutch wadden sea. i. biomass and species richness. Netherlands J. Sea Res. 23, 57–68. doi: 10.1016/0077-7579(89)90043-4
Dittmann S. (1987). Die bedeutung der biodeposite für die benthosgemeinschaft der wattsedimente, unter besonderer berücksichtigung der miesmuschel mytilus edulis l (Germany: Universität Göttingen). PhD Thesis.
Essink K., Beukema J., Madsen P., Michaelis H., Vedel G. (1998). Long-term development of biomass of intertidal macrozoobenthos in different parts of the wadden sea. governed by nutrient loads? Senckenbergiana Maritima 29, 25–35. doi: 10.1007/BF03043940
Flemming B., Delafontaine M. (1994). Biodeposition in a juvenile mussel bed of the East Frisian wadden Sea (Southern north Sea). Aquat. Ecol. 28, 289–297. doi: 10.1007/BF02334197
French J., Payo A., Murray A. B., Orford J., Eliot M., Cowell P. (2016). Appropriate complexity for the prediction of coastal and estuarine geomorphic behaviour at decadal to centennial scales. Geomorphology 256, 3–16. doi: 10.1016/j.geomorph.2015.10.005
Gracia G., Aranda J., Gracia I., Suarez O., Tercero J., Enano N. (2015). Learning image processing with OpenCV. (Birmingham (UK): Packt Publishing).
Guillen G. (2019). Digital image processing with Python and OpenCV (Berlin (Germany): Springer). doi: 10.1007/978-1-4842-5299-4_5
Hastings A., Byers J., Crooks J., Cuddington K., Jones C., Lambrinos J., et al. (2007). Ecosystem engineering in space and time. Ecol. Lett. 10, 153–164. doi: 10.1111/j.1461-0248.2006.00997.x
Holzhauer H., Borsje B. W., van Dalfsen J., Wijnberg K., Hulscher S., Herman P. (2019). Benthic species distribution linked to morphological features of a barred coast. J. Mar. Sci. Eng. 8, 16. doi: 10.3390/jmse8010016
Horton R. (1945). Erosional development of streams and their drainage basins: hydro-physical approach to quantitative morphology. Geological Soc. America Bull. 56, 275–370. doi: 10.1130/0016-7606(1945)56[275:EDOSAT]2.0.CO;2
Jakobsen H., Markager S (2016). Carbon-to-chlorophyll ratio for phytoplankton in temperate coastal waters: Seasonal patterns and relationship to nutrients. Limnology and Oceanography. doi: 10.1002/lno.10338
Jørgensen B. B., Parkes R. J. (2010). Role of sulfate reduction and methane production by organic carbon degradation in eutrophic fjord sediments (Limfjorden, Denmark). Limnol. Oceanogr. 55 (3), 1338–1352. doi: 10.4319/lo.2010.55.3.1338
Jones S. C., Lawton J. H., Shachak M. (1994). Organisms as ecosystem engineers. Ecosyst. Manage. 69, 373–386 doi: 10.1007/978-1-4612-4018-1_14
Kautsky N., Evans S. (1987). Role of biodeposition by mytilus edulis in the circulation of matter and nutrients in a Baltic coastal system. Mar. Ecol. Prog. Ser. 38, 201–212. doi: 10.3354/meps038201
Klassen I. (2017). Three-dimensional numerical modeling of cohesive sediment flocculation processes in turbulent flows (Karlsruhe (Germany): Karlsruher Institut für Technologie). PhD Thesis.
Kristensen E., Penha-Lopes G., Delefosse M., Valdemarsen T., Organo Quintana C., Banta G. (2012). What is bioturbation? need for a precise definition for fauna in aquatic science. Mar. Ecol. Prog. Ser. 446, 285–302. doi: 10.3354/meps09506
Larsen L., Eppinga M., Passalacqua P., Getz W., Rose K., Liang M. (2016). Appropriate complexity landscape modeling. Earth Sci. Rev. 160, 111–130. doi: 10.1016/j.earscirev.2016.06.016
Leonardi N., Carnacina I., Donatelli C., Ganju N., Plater A., Schuerch M., et al. (2018). Dynamic interactions between coastal storms and salt marshes: A review. Geomorphol. Volume 301, 92–107. doi: 10.1016/j.geomorph.2017.11.001
Li J., Chen X., Townend I., Shi B., Du J., Gao J., et al. (2021). A comparison study on the sediment flocculation process between a bare tidal flat and a clam aquaculture mudflat: The important role of sediment concentration and biological processes. Mar. Geol. 434, 106443. doi: 10.1016/j.margeo.2021.106443
Lindqvist S., Engelbrektsson J., Eriksson S., Hulth S. (2016). Functional classification of bioturbating macrofauna in marine sediments using time-resolved imaging of particle displacement and multivariate analysis. Biogeosci. Discuss 1–30. doi: 10.5194/bg-2016-411
Lumborg U., Andersen T., Pejrup M. (2006). The effect of hydrobia ulvae and microphytobenthos on cohesive sediment dynamics on an intertidal mudflat described by means of numerical modelling. Estuarine Coast. Shelf Sci. 68, 208–220. doi: 10.1016/j.ecss.2005.11.039
Malcherek A. (1995). Mathematische Modellierung von Strömungen und Stofftransportprozessen in Ästuaren. Hannover. : Institut für Strömungsmechanik und Elektronisches Rechnen im Bauwesen der Universität Hannover. Institut für Strömungsmechanik und Elektronisches Rechnen im Bauwesen der Universität Hannover, Bericht. 44. Available at: https://www.unibw.de/wasserwesen/personen-hydro/malcherek
Manning A., Baugh J., Soulsby R., Spearman J., Whitehouse R. (2011). Cohesive sediment flocculation and the application to settling flux modelling. InTech. 1, 91–109 doi: 10.5772/16055
Marani M., D'Alpaos A., Lanzoni S., Carniello L., Rinaldo A. (2010). The importance of being coupled: stable states and catastrophic shifts in tidal biomorphodynamics. J. Geophys. Res. Earth Surf 115, F04004. doi: 10.1029/2009JF001600
Marciano R., Wang Z. B., Hibma A., de Vriend H., Defina A. (2005). Modeling of channel patterns in short tidal basins. J. Geophysical Res. 110, F01001 doi: 10.1029/2003JF000092
Mikkelsen O., Pejrup M. (2000). In situ particle size spectra and density of particle aggregates in a dredging plume. Mar. Geol. 170, 443–459. doi: 10.1016/S0025-3227(00)00105-5
Mitchell M. (2006). In situ biodeposition rates of pacific oysters (Crassostrea gigas) on a marine farm in southern Tasmania (Australia). Aquaculture 257, 194–203. doi: 10.1016/j.aquaculture.2005.02.061
Möller I., Kudella M., Rupprecht F., Spencer T., Paul M., Van Wesenbeeck B. K., et al. (2014). Wave attenuation over coastal salt marshes under storm surge conditions. Nat. Geosci. 7 (10), 727–731. doi: 10.1038/ngeo2251
Mudd S.M., Fagherazzi S., Morris J.T., Furbish D.J. (2004). Flow, sedimentation, and biomass production on a vegetated salt marsh in south Carolina: Toward a predictive model of marsh morphologic and ecologic evolution. in The Ecogeomorphology of Tidal Marshes. Fagherazzi S., Marani M., Blum L.K.. doi: 10.1029/CE059p0165
Murray F., Douglas A., Solan M. (2014). Species that share traits do not necessarily form distinct and universally applicable functional effect groups. Mar. Ecol. Prog. Ser. 516, 23–34. doi: 10.3354/meps11020
Murray A. B., Knaapen M., Tal M., Kirwan M. (2008). Biomorphodynamics: physical-biological feedbacks that shape landscapes. Water Resour. Res. 44. doi: 10.1029/2007WR006410
Murray F., Widdicombe S., Mcneill C., Douglas A. (2017). Assessing the consequences of environmental impacts: variation in species responses has unpredictable functional effects. Mar. Ecol. Prog. Ser. 583, 35–47. doi: 10.3354/meps12358
Nasermoaddeli M. H., Lemmen C., Koesters F., Stigge G., Kerimoglu O., Burchard H., et al. (2017). A model study on the large-scale effect of macrofauna on the suspended sediment concentration in a shallow shelf sea. Estuar. Coast. Shelf Sci. 211, 62–76. doi: 10.1016/j.ecss.2017.11.002
Orvain F., Le Hir P., Sauriau P.-G., Lefebvre S. (2012). Modelling the effects of macrofauna on sediment transport and bed elevation: Application over a cross-shore mudflat profile and model validation. Estuar. Coast. Shelf Sci. 108, 64–75. doi: 10.1016/j.ecss.2011.12.036
Paarlberg A. J., Knaapen M., de Vries M., Hulscher S., Wang Z. B. (2005). Biological influences on morphology and bed composition of an intertidal flat. Estuar. Coast. Shelf Sci. 64, 577–590. doi: 10.1016/j.ecss.2005.04.008. 577-590.
Passalacqua P., Do Trung T., Foufoula-Georgiou E., Sapiro G., Dietrich W. E. (2010). A geometric framework for channel network extraction from lidar: nonlinear diffusion and geodesic paths. J. Geophys Res. 115, F01002. doi: 10.1029/2009JF001254
Perona P., Malik J. (1990). Scale-space and edge detection using anisotropic diffusion. IEEE Trans. Pattern Anal. Mach. Intell. 23, 629–639. doi: 10.1109/34.56205
Pinto L., Fortunato A., Zhang Y., Oliveira A., Sancho F. (2012). Development and validation of a three-dimensional morphodynamic modelling system for non-cohesive sediments. Ocean Model. 57–58, 1–14. doi: 10.1016/j.ocemod.2012.08.005
Pleskachevsky A., Gayer G., Horstmann J., Rosenthal W. (2005). Synergy of satellite remote sensing and numerical modelling for monitoring of suspended particulate matter. Ocean Dynamics 55 (1), 2–9. doi: 10.1016/S0422-9894(03)80021-1
Rees H. L., Eggleton J. D., Rachor E., Berghe E. (2007). Structure and dynamics of the north Sea benthos. ICES Cooperative Res. Rep. 288, 258. Available at: https://www.vliz.be/en/imis?module=ref&refid=114857.
Reinhardt L., Jerolmack D., Cardinale B., Vanacker V., Wright J. (2010). Dynamic interactions of life and its landscape: feedbacks at the interface of geomorphology and ecology. Earth Surf. Process. Landf. 35, 78–101. doi: 10.1002/esp.1912
Reise K., Herre E., Sturm M. (1994). Biomass and abundance of macrofauna in intertidal sediments of königshafen in the northern wadden Sea. Helgolander Meeresunters 48, 201–215. doi: 10.1007/BF02367036
Sanford L. (2008). Modeling a dynamically varying mixed sediment bed with erosion, deposition, bioturbation, consolidation, and armoring. Comput. Geosci. 34, 1263–1283. doi: 10.1016/j.cageo.2008.02.011
Schückel U., Beck M., Krönke I. (2015b). Macrofauna communities of tidal channels in jade bay (German wadden sea): spatial patterns, relationships with environmentalcharacteristics, and comparative aspects. Mar. Biodiversity 45 (4), 841–855. doi: 10.1007/s12526-014-0308-2
Schückel U., Kröncke I. (2013). Temporal changes in intertidal macrofauna communities over eight decades: A result of eutrophication and climate change. Estuarine Coast. Shelf Sci. 117, 210–218. doi: 10.1016/j.ecss.2012.11.008
Schückel U., Krönke I., Baird D. (2015a). Linking long-term changes in trophic structure and function of an intertidal macrobenthic system to eutrophication and climate change using ecological network analysis. Mar. Ecol. Prog. Ser. 536, 25–38. doi: 10.3354/meps11391
Shi B., Pratolongo P. D., Du Y., Li J., Yang S. L., Wu J., et al. (2020). Influence of macrobenthos (Meretrix meretrix Linnaeus) on erosion - accretion processes in intertidal flats: a case study from a cultivation zone. J. Geophys. Res. Biogeosci. 125, e2019JG005345. doi: 10.1029/2019JG005345
Shi B., Yang S. L., Temmerman S., Bouma T., Ysebaert T., Wang S., et al. (2021). Effect of typhoon-induced intertidal-flat erosion on dominant macrobenthic species (Meretrix meretrix). Limnol Oceanogr. 66, 4197–4209. doi: 10.1002/lno.11953
Sievers J., Rubel M., Milbradt P. (2020). EasyGSH-DB: Bathymetrie, 1996-2016) (Karlsruhe (Germany): Bundesanstalt für Wasserbau). doi: 10.48437/02.2020.K2.7000.0002
Singer A., Schückel U., Beck M., Bleich O., Brumsack H., Freund H., et al. (2016). Small-scale benthos distribution modelling in a north Sea tidal basin in response to climatic and environmental changes 1970s-2009). Mar. Ecol. Prog. Ser. 551, 13–30. doi: 10.3354/meps11756
Song Y. T., Haidvogel D. B. (1994). A semi-implicit ocean circulation model using a generalized topography following coordinate system. J. Comput. Phys. 115, 228–248. doi: 10.1006/jcph.1994.1189
Stal L. (2010). Microphytobenthos as a biogeomorphological force in intertidal sediment stabilization. Ecol. Eng. 36, 236–245. doi: 10.1016/j.ecoleng.2008.12.032
Stephens M., Kadko D., Smith C., Latasa M. (1997). Chlorophyll-a and pheopigments as tracers of labile organic carbon at the central equatorial Pacific seafloor. Geochimica et Cosmochimica Acta 61. 10.1016/S0016-7037(97)00358-X
Strahler M. (1953). Hypsometric (area-altitude) analysis of erosional topology. Geological Soc. Am. Bull. 63, 1117–1142. doi: 10.1130/0016-7606(1952)63[1117:HAAOET]2.0.CO;2
Teal L. R., Bulling M. T., Parker E. R., Solan M. (2008). Global patterns of bioturbation intensity and mixed depth of marine soft sediments. Aquat. Biol. 2 (3), 207–218. doi: 10.3354/ab00052
Umlauf L., Burchard H. (2003). A generic length-scale equation for geophysical turbulence models. J. Mar. Res. Chesapeake Bay Program, Annapolis, Maryland, USA 61 (2), 235–265(31). doi: 10.1357/002224003322005087
US Army Corps of Engineers (2000). Development of a suspension feeding and deposit feeding benthos model for Chesapeake bay. (Annapolis, Maryland, USA:Chesapeake Bay Program).
Valdemarsen T., Quintana C. O., Thorsen S. W., Kristensen E. (2018). Benthic macrofauna bioturbation and early colonization in newly flooded coastal habitats. PloS One 13 (4), e0196097. doi: 10.1371/journal.pone.0196097
van Ledden M. (2001). Modelling of sand-mud mixtures. part II: A process-based sand-mud model (Delft (NL): Delft Cluster publicatienummer Z2840.00). doi: 10.1016/S1568-2692(02)80041-9
van Ledden M. (2002). A process-based sand-mud model. Proc. Mar. Sci. 5, 577–594. doi: 10.1016/S1568-2692(02)80041-9
van Ledden M. (2003). Sand-mud segregation in estuaries and tidal basins (Delft (NL): Delft University of Technology). PhD thesis.
van Maanen B., Coco G., Bryan K. R. (2013a). Modelling the effects of tidal range and initial bathymetry on the morphological evolution of tidal embayments. Geomorphology 191, 23–34. doi: 10.1016/j.geomorph.2013.02.023
van Maanen B., Coco G., Bryan K. (2015). On the ecogeomorphological feedbacks that control tidal channel network evolution in a sandy mangrove setting. Proc. R. Soc. A. 471, 20150115. doi: 10.1098/rspa.2015.0115
van Maanen B., Coco G., Bryan K., Friedrichs C. (2013b). Modeling the morphodynamic response of tidal embayments to sea-level rise. Ocean Dynamics 63, 1249–1262. doi: 10.1007/s10236-013-0649-6
Viles H. (2020). Biogeomorphology: past, present and future. Geomorphology 366, 106809. doi: 10.1016/j.geomorph.2019.06.022
Wang C., Temmerman S. (2013). Does biogeomorphic feedback lead to abrupt shifts between alternative landscape states?: an empirical study on intertidal flats and marshes. J. Geophysical Res. (Earth Surface). 118, 229–240. doi: 10.1029/2012JF002474
Warner J., Signell R., Harris C., Arango H. (2008). Development of a three-dimensional, regional, coupled wave, current, and sediment-transport model. Comput. Geosci. 34, 1284–1306. doi: 10.1016/j.cageo.2008.02.012
Weilbeer H. (2005). Modeling the transport of cohesive sediments (in (Germany))Darmstädter wasserbauliches kolloquium / DWA-seminar feststofftransportmodelle. Ed. Darmstadt T. U. (Darmstadt (Germany): Instiut für Wasserbau und Wasserwirtschaft). Heft. 139.
Wood R., Widdows J. (2002). A model of sediment transport over an intertidal transect, comparing the influences of biological and physical factors. Limnol. Oceanogr. - LIMNOL OCEANOGR 47, 848–855. doi: 10.4319/lo.2002.47.3.0848
Wood R., Widdows J. (2003). Modelling intertidal sediment transport for nutrient change and climate change scenarios. Sci. Total Environ. 314-316, 637–649. doi: 10.1016/S0048-9697(03)00088-3
Zhang W., Arlinghaus P. (2022). Climate, coast and morphology. Ox. Res. Encyclopedia Climate Sci. doi: 10.1093/acrefore/9780190228620.013.814
Zhang W., Neumann A., Daewel U., Wirtz K., van Beusekom J. E. E., Eisele A., et al. (2021). Quantifying importance of macrobenthos for benthic-pelagic coupling in a temperate coastal shelf sea. J. Geophysical Res.: Oceans 126, e2020JC016995. doi: 10.1029/2020JC016995
Zhang Y., Ren J., Zhang W. (2020). Flocculation under the control of shear, concentration and stratification during tidal cycles. J. Hydrol. 586, 124908. doi: 10.1016/j.jhydrol.2020.12490
Zhang W., Schneider R., Kolb J., Teichmann T., Dudzinska-Nowak J., Harff J., et al. (2015). Land-sea interaction and morphogenesis of coastal foredunes - a modelling case study from the southern Baltic Sea coast. Coast. Eng. 99, 148–166. doi: 10.1016/j.coastaleng.2015.03.005
Zhang W., Wirtz K. (2017). Mutual dependence between sedimentary organic carbon and infaunal macrobenthos resolved by mechanistic modeling. J. Geophysical Res.: Biogeosci. 122, 2509–2526. doi: 10.1002/2017JG003909
Zhang W., Wirtz K., Daewel U., Wrede A., Kröncke I., Kuhn G., et al. (2019). The budget of macrobenthic reworked organic carbon: A modeling case study of the north Sea. J. Geophysical Res.: Biogeosci. 124, 1446-1471. doi: 10.1029/2019JG005109
Zhang Y., Ye F., Stanev E., Grashorn S. (2016). Seamless cross-scale modelling with SCHISM. Ocean Model. 102, 64–81. doi: 10.1016/j.ocemod.2016.05.002
Keywords: mophological evolution, benthos, bioturbation, tidal basin, modeling, functional groups
Citation: Arlinghaus P, Zhang W and Schrum C (2022) Small-scale benthic faunal activities may lead to large-scale morphological change- A model based assessment. Front. Mar. Sci. 9:1011760. doi: 10.3389/fmars.2022.1011760
Received: 04 August 2022; Accepted: 29 September 2022;
Published: 17 October 2022.
Edited by:
Chunyan Li, Louisiana State University, United StatesReviewed by:
Regan Nicholaus, Mbeya University of Science and Technology, TanzaniaBenwei Shi, East China Normal University, China
Copyright © 2022 Arlinghaus, Zhang and Schrum. This is an open-access article distributed under the terms of the Creative Commons Attribution License (CC BY). The use, distribution or reproduction in other forums is permitted, provided the original author(s) and the copyright owner(s) are credited and that the original publication in this journal is cited, in accordance with accepted academic practice. No use, distribution or reproduction is permitted which does not comply with these terms.
*Correspondence: Peter Arlinghaus, cGV0ZXIuYXJsaW5naGF1c0BoZXJlb24uZGU=; Wenyan Zhang, d2VueWFuLnpoYW5nQGhlcmVvbi5kZQ==