- 1South China Sea Fisheries Research Institute, Chinese Academy of Fishery Sciences, Guangzhou, China
- 2College of Marine Sciences, Shanghai Ocean University, Shanghai, China
- 3Scientific Observing and Experimental Station of South China Sea Fishery Resources & Environment, Ministry of Agriculture and Rural Affair, Guangzhou, China
- 4National Agricultural Experimental Station for Fishery Resources and Environment Dapeng, Shenzhen, China
- 5Guangxi Academy of Oceanology, Nanning, China
- 6Service Center for Agriculture and Agricultural Machinery of Fangchenggang, Fangchenggang, China
- 7Fangchenggang Fishery Radio, Fangchenggang, China
Artificial habitat deployment can restore natural habitats or supplement existing natural habitats. The effect of resource proliferation and protection is obvious. However, few reports have addressed the biological community association between artificial habitats and adjacent environments. Here, Illumina sequencing of 18S rDNA was performed, and the diversity, community structure, and co-occurrence networks of protists in different layers of artificial reefs (ARs) and adjacent seawater (WAR) were described to verify that constructing ARs in Bailong Pearl Bay improves local spatial heterogeneity and functional diversity. In terms of the degree of species interaction, the protist communities were ranked as follows: surface and bottom of WAR > ARs and WAR > different layers of ARs. The α-diversity of protists associated with ARs and WAR decreased with an increase in depth. Protist diversity was greater in WAR than in ARs. β-Diversity analysis revealed significant differences in protist community structure between WAR and ARs (P < 0.05), and the upper layers of ARs and the middle or bottom layers of ARs differed. The key topological features of protist networks showed more positive interspecific interactions in the AR-associated protist community, a higher degree of niche differentiation, and higher complexity and stability. The keystone protists in the bottom seawater layer displayed community functions that were biased toward initial fixation in the ocean carbon cycle. The AR-associated protist community tended to participate in carbon transfer in the food chain and decomposition and utilization of dissolved organic matter (DOM). This study revealed significant differences in protist community structure between ARs and the adjacent environment, and the ecological functions of the key phyla were found to be related. In conclusion, protist communities in WAR may provide food sources for AR-associated heterotrophic protists. A variety of key phyla associated with ARs have biological roles in the carbon pump via their ecological characteristics.
Introduction
Affected by factors such as environmental pollution, habitat degradation, overfishing and climate change, global marine fishery resources have declined significantly. To alleviate the degradation of marine habitats, artificial habitats have been deployed to restore lost natural habitats or to supplement existing natural habitats (Lemoine et al., 2019).
Artificial habitats are created via the manipulation of natural aquatic habitats through the addition of human-made or natural structures, principally to enhance fisheries but also to influence the life cycles of organisms or the functions of ecological systems for other purposes (William Seaman, 1993). The use and study of artificial habitats is a maturing area of fishery science. Many types of artificial habitats have been designed and employed in the protection and management of marine ecosystems. For example, fish-aggregating devices (FADs) are deployed at the surface or various levels in the water column and may be composed of automobile tires, natural wood or synthetic mesh that is suspended in the water column. Different from FADs, artificial reefs (ARs) are artificial habitats that have been scientifically selected and placed on the seabed. They simulate rock or natural coral reefs by adding complex topography to flat and featureless ocean bottoms. According to the selected materials, ARs can be divided into concrete reefs, stone reefs, tire reefs, steel reefs, plastic reefs, wooden bamboo reefs, scrap cars and ship reefs (Sheng, 2012).
To clarify the role of artificial habitats in global fisheries management, a large number of studies have been carried out, including studies on the influence of artificial habitats on marine environmental factors (Falcao et al., 2007; Yu et al., 2015), the trapping effect on fish and other marine organisms (Folpp et al., 2020; Paxton et al., 2020; Hall et al., 2021), the provision of spawning substrate for fish that produce sticky eggs (Sandström and Karås, 2002; Guo D. et al., 2020), the provision of refuges for juvenile fishes (Bolding et al., 2010; Höjesjö et al., 2015), the phenotypic responses of specific organisms adapted to artificial habitats (Dias et al., 2021), the impact of the introduction of alien species on local species (Rumbold et al., 2020; Janiak and Branson, 2021), and the protection effect of endangered species (Claassens and Harasti, 2020).
In addition to the above mentioned investigations on the effects of enhancement and resource protection, research on microbial diversity, community structure and temporal and spatial changes in artificial habitats has gradually proliferated. Soka et al. (2011) compared the population dynamics of coral-associated bacteria (CAB) in natural and artificial habitats. Sweet et al. (2011) investigated the development of bacterial biofilms on artificial corals and discovered that the dominant bacteria on artificial corals were also associated with natural coral tissue and reef systems. The bacterial communities formed on artificial corals were similar to those of natural corals. Onitsuka et al. (2013) examined the succession of bacterial communities on a newly established AR and neighboring natural rocky reefs in different seasons. Surveys of Daya Bay revealed seasonal and spatial differences in the structure of the protist communities in the AR, coral reef and open-water areas (Qin et al., 2019; Zhu et al., 2020, 2021).
A large number of survey results have confirmed that artificial habitats provide safe habitat conditions for marine aquatic organisms to gather, grow, multiply and avoid predators and to achieve the goals of optimizing the ecological environment, protecting and improving marine fishery resources, and increasing the yield of fish. Studies on microbial communities have also verified from a microscopic perspective that artificial habitats have a role similar to that of natural habitats. However, the survey subjects of the abovementioned studies were obtained from only a single medium (seawater or artificial habitats), and few reports address the biological community association between artificial habitats and adjacent natural environments. Marine protists exist in seawater, are associated with artificial habitats and are suitable for use in the study of the relationship between artificial habitats and adjacent seawater. Protists, as producers, consumers and decomposers, occupy an important ecological position in the microbial food web (Massana, 2011) and have an especially important pivotal role in the recycling, utilization and transmission of carbon sources. Changes in the structure of protist communities often affect the entire microbial food web and ecosystem (Finlay and Esteban, 1998). Based on the findings of previous studies and the ecological status of protists, this article discusses the differences and correlations between the structure and function of protist communities in artificial habitats (considering ARs as an example) and adjacent environments (seawater near ARs). The aim of this work is to deeply understand the ecological processes that exist between artificial habitats and adjacent natural environments and to deepen the understanding of the positive impacts of the construction of artificial habitats on the ecological environment.
Materials and Methods
Study Area and Sampling
Samples were collected in Bailong Pearl Bay, Fangchenggang, China. Bailong Pearl Bay is a closed bay with a southern subtropical climate, occupying an area from Dengtaling on the Bailong Peninsula to Huangwu Village in southern Wushan. Surface runoff in Bailong Pearl Bay is low, so the salinity is stable, and the average annual temperature is 23.0°C. Bailong Pearl Bay is China’s first AR test site and has formed a relatively stable ecosystem. The construction of ARs began in 1979, and a large-scale marine pasture demonstration area was planned in 2012. As of 2019, the total amount of sea designated for the project was 1,040 hm2. The types of ARs released during the construction process include rock reefs, old boat reefs, and reinforced concrete reefs with different specifications (Pei et al., 2020).
Samples were collected in March 2019. Figure 1 shows the locations of 3 sampling sites in ARs (AR1–AR3) in Bailong Pearl Bay. Divers utilized centrifuge tubes to scrape the attachments on the upper layer (ARS, 0.5 m from the top of the reef), middle layer (ARM, halfway point of the reef) and bottom layer (ARB, 0.5 m from the bottom of the reef) of the three ARs (reinforced concrete reefs, 3.6 m × 3.6 m × 5.5 m) (Supplementary Figures 1,2). Water samples were collected from the surface (WARS) and bottom (WARB) of the sea adjacent to the 3 ARs. A total of 9 seawater samples were immediately transferred to sterile cryogenic bottles at a low temperature. Within 24 h, 500 ml sea water samples were filtered through a membrane with a 0.2 μm pore diameter (Merck-Millipore, United States) using a suction device after passing through a 200 μm mesh-size prefilter to reduce the contribution of metazoans to the nucleic acid extracts. The centrifuge tubes and filtered samples were preserved at −80°C until further processing. The environmental data of each sample were measured by a YSI water quality instrument (YSI, United States) at the sampling sites and included seawater temperature (T), salinity (Sal), and dissolved oxygen (DO). The height of the AR is measured by the divers with a band tape, and the depths of different layers are calculated according to the water depth of the sampling sites (Supplementary Table 3).
18S rDNA Analysis of Protists
The sample filters were cut up with sterile scissors, and the total DNA was extracted according to the steps of the Power Soil DNA Kit (MO BIO, United States). A NanoDrop ND-1000 spectrophotometer (NanoDrop Technologies, Wilmington, DE, United States) was selected for quantification, and detection was performed on a 1% agarose gel. DNA extracts were kept at −80°C until processing.
The V4 region of the 18S rDNA gene was amplified using the universal primer set TAReuk454FWD1 (5′-CCA GCA SCY GCG GTA ATT CC-3′; Saccharomyces cerevisiae positions 565–584) and TAReukREV3 (5′-ACT TTC GTT CTT GAT YRA-3′; S. cerevisiae positions 964–981) (Stoeck et al., 2010), and then specific primers were synthesized with barcodes. Polymerase chain reaction (PCR) amplification was performed using Phusion® High-Fidelity PCR Master Mix (Biolabs, New England). PCR amplification of each sample was repeated 3 times. The PCR products of the same sample were mixed and detected by 2% agarose gel electrophoresis. Referring to the preliminary quantification results of electrophoresis, a QuantiFluor™-ST blue fluorescence quantification system (Promega, United States) was employed to detect and quantify the PCR products. The three replicate products of each sample were mixed, and then an Illumina PE250 library was constructed for sequencing. After the original sequence underwent quality control and filtering processes, the Uclust algorithm (version v.1.2.22q) was applied to divide the sequences with a similarity greater than 97% into the same operational taxonomic unit (OTU). The classification and annotation information of OTUs was obtained by comparison with the Silva database (Release1321), and sequences related to protists were selected for further analysis.
Data Analysis and Bioinformatics
According to the obtained OTU abundance matrix, a Venn diagram was created using the “VennDiagram” package to show the relationships among OTUs originating from different layers and locations. α-Diversity (Chao1 index, Shannon diversity index, and Simpson diversity index) was calculated using mothur (version v.1.30.12) (Supplementary Table 1). The Shannon index boxplot was created using the “reshape2” package and “ggplot2” package. One-way analysis of variance (ANOVA) was constructed to test whether the differences between two groups were significant. The analysis and mapping of β-diversity was performed using the “vegan” package and “ggplot2” package. Principal coordinate analysis (PCoA) based on the Bray–Curtis dissimilarity matrix was applied to determine the sizes of the community composition differences between two samples, and permutational multivariate analysis of variance (PERMANOVA) was performed to test whether the community differences between two groups were significant. A stacked area chart was created using the “ggplot2” package to analyze the difference in the relative abundance of the protists at different positions. The “igraph” package was employed to construct a co-occurrence network to explore the interrelationships among protists, and topological parameters such as the average degree, average path length, betweenness centralization, clustering coefficient, degree centralization, density, modularity, and the proportion of positive and negative correlations in each network graph were calculated. Redundancy analysis (RDA) based on the Bray–Curtis dissimilarity matrix was conducted to explore the relationships between environmental variables and the protist community, and Pearson correlation coefficients were calculated. The abovementioned data analyses and graphic visualization were completed in R software (v3.6.1).
Results
Shared Operational Taxonomic Unit Analysis
After screening and filtering the original sequencing data, the number of sequences required for subsequent analysis of each sample was obtained. As shown in Table 1, each sample included more than 30,000 effective labels.
The number of OTUs can represent the richness of species. According to Figure 2, a total of 695 OTUs were obtained in this study. The numbers of OTUs detected in the ARS, ARM, ARB, WARS, and WARB groups were 102, 103, 83, 201, and 206, respectively. The number of shared OTUs in the 5 groups was 19. The number of OTUs in WAR was 366, of which the number of unique OTUs was 221, accounting for 57.65%, indicating that 40% of the OTUs in the WAR could be detected on the ARs.
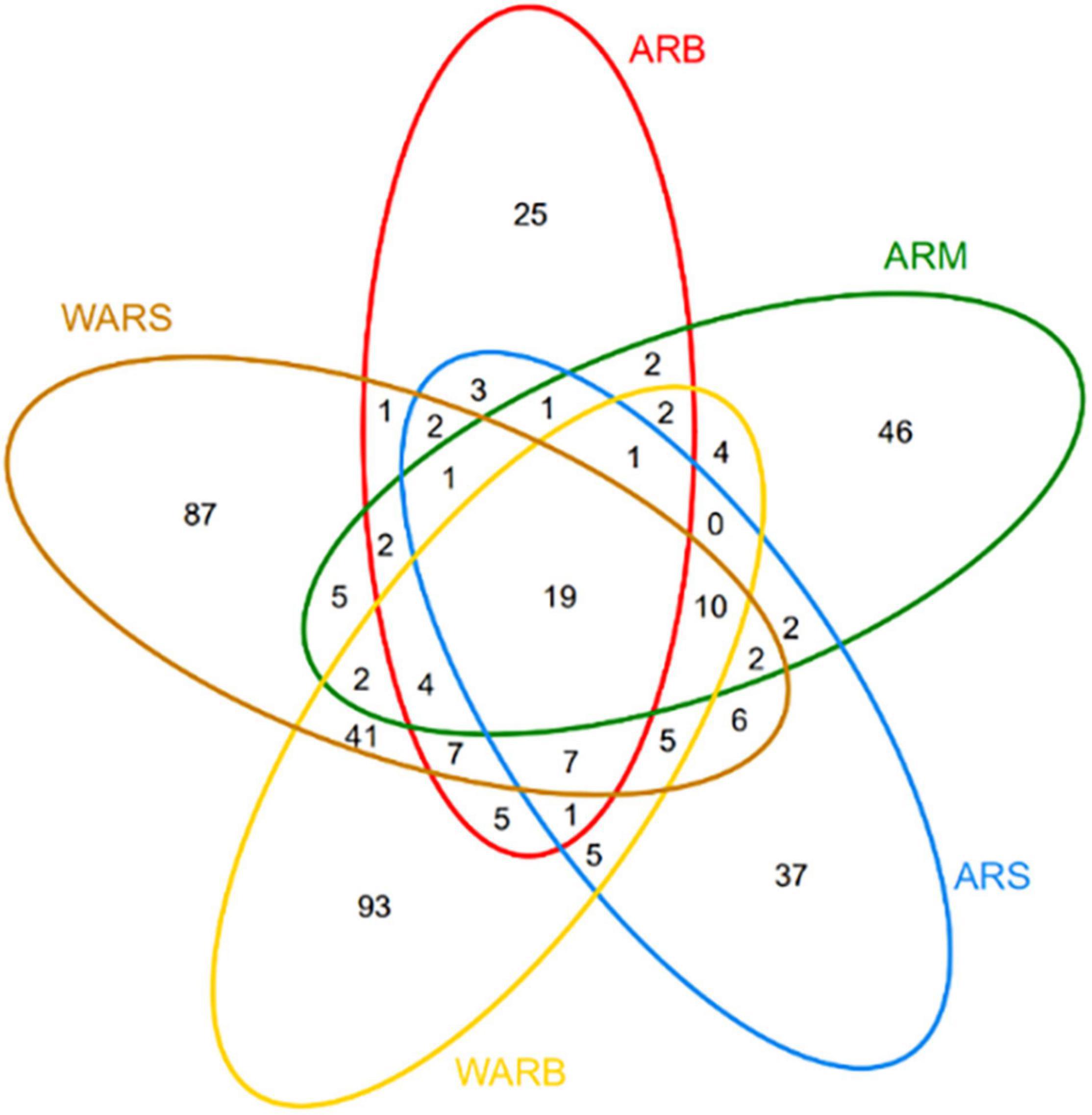
Figure 2. Venn diagram. The number in each region indicates the number of shared or unique OTUs for the group contained in the region.
To explore the degree of interaction between WAR protists and AR protists in different directions, the abovementioned five groups of two-by-two combinations were divided into 7 interaction groups. The shared number of OTUs in each interaction group is shown in Table 2. A comparison revealed that the degree of interaction is expressed as follows: between the surface and the bottom of the water > between the ARs and the WAR > between different layers of the ARs.
According to Figure 3, the Shannon index of protists in the ARs and WAR showed a decreasing trend in the vertical direction. The diversity of protist communities decreased with an increase in depth. In the horizontal direction, the Shannon index of the WAR was higher than that of the ARs. The WARS and ARS showed extremely significant differences (P < 0.01).
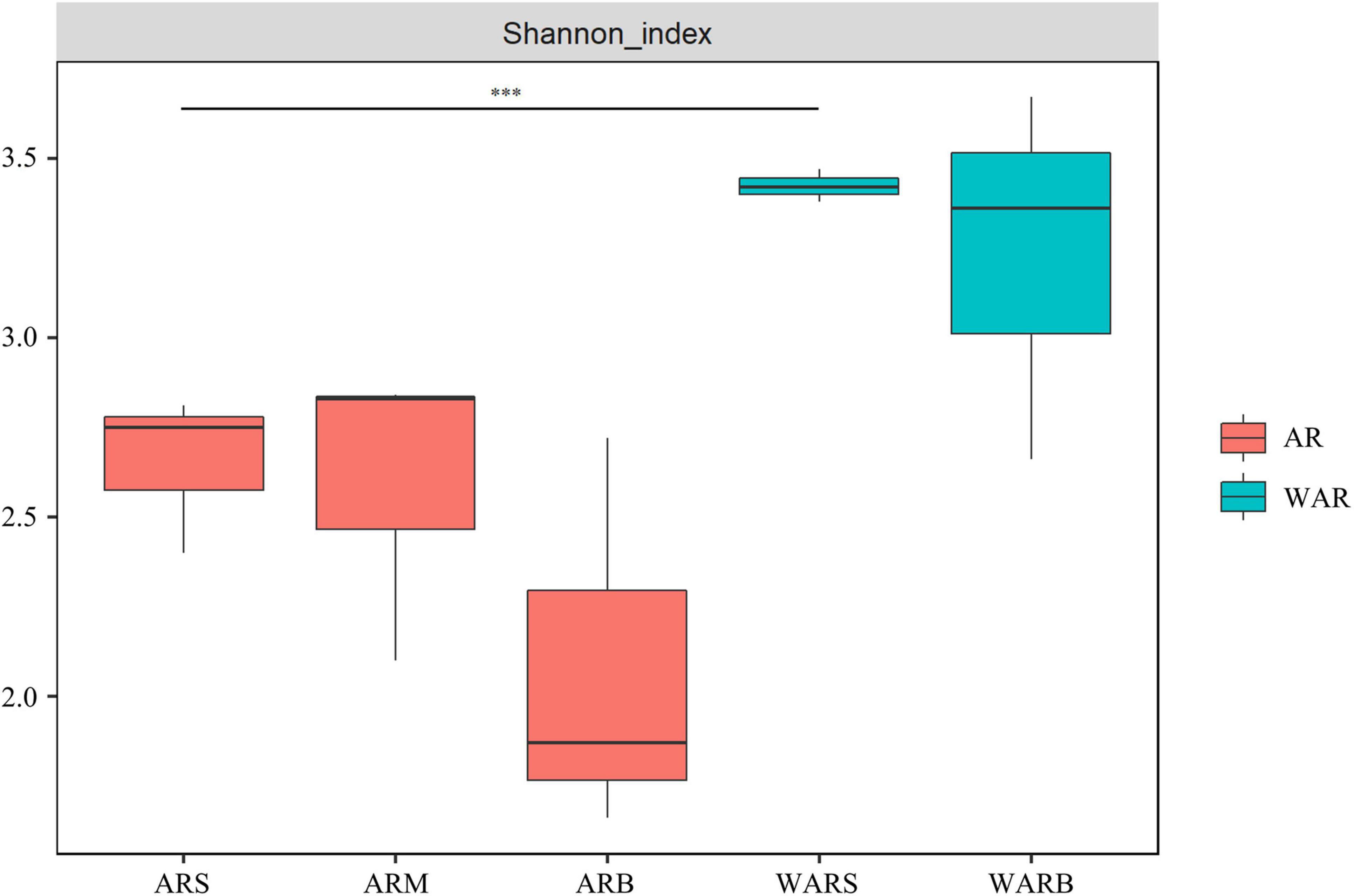
Figure 3. Shannon diversity index. Shannon diversity index of the upper, middle and bottom layers of the ARs (ARS, ARM, and ARB). Shannon diversity index of the surface WAR and bottom WAR (WARS and WARB, respectively). Comparing the samples at both ends of the line, *** indicates an extremely significant difference.
β-Diversity Analysis
The main purpose of β-diversity analysis was to determine the differences in the structure of the protist community between different sampling locations. Combined PCoA and permutational multivariate analysis of variance (PERMANOVA) (Figure 4) revealed that the distance between the ARs and the WAR was relatively far, indicating a significant difference in the structure of protist communities between the ARs and the WAR (P = 0.011). At different sampling locations on the ARs, the ARM and ARB were relatively close, and the centroid of the ARS was below the horizontal axis, which differs from the patterns observed for the other two groups.
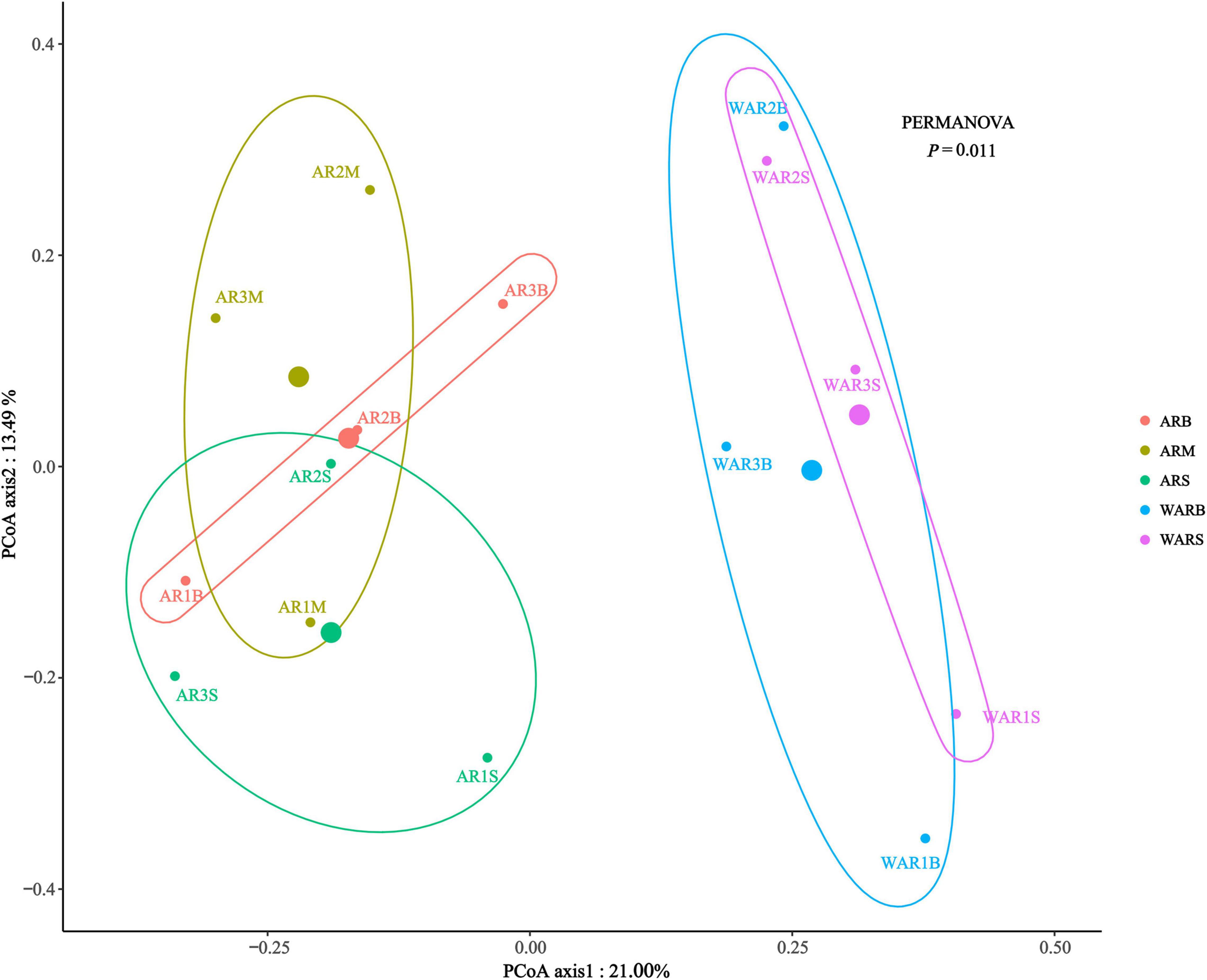
Figure 4. β-Diversity evaluated based on Bray–Curtis dissimilarities in all samples. PCoA plot of the Bray–Curtis distances. The largest point is the centroid of each group.
Community Composition
To analyze the spatial changes in the composition of the protist community, we investigated the relative abundance of AR-associated protists and protists in the WAR (Figure 5). At the phylum level, the protist community was dominated by Apicomplexa, Cercozoa, Ciliophora, Cryptomycota, Dinoflagellata, Gracilipodida, Incertae Sedis, MAST-9, Ochrophyta, Unclassified and other taxa. The results showed that the protist community changed with an increase in depth and revealed obvious differences between the AR community and WAR community in the same layer were noted. For example, the relative abundance of Dinoflagellata and Ochrophyta in the WAR was greater than that in the ARs, and these groups accounted for up to 61% of the community in the bottom layer of the seawater. Ciliophora showed a significant difference in the vertical direction in seawater (P < 0.01). In the horizontal direction, Cryptomycota showed a significant difference between WARB and ARS (P < 0.01). Dinoflagellata showed a difference between WARB and ARM (P < 0.05). The differences in different directions for other phyla were not significant (P > 0.05).
Co-occurrence Network Analysis
The co-occurrence network (Figure 6) captured 573 edges (ARS with 58 nodes), 599 edges (ARM with 69 nodes), 278 edges (ARB with 43 nodes), 593 edges (WARS with 72 nodes), and 606 edges (WARB with 78 nodes), including positive and negative relationships.
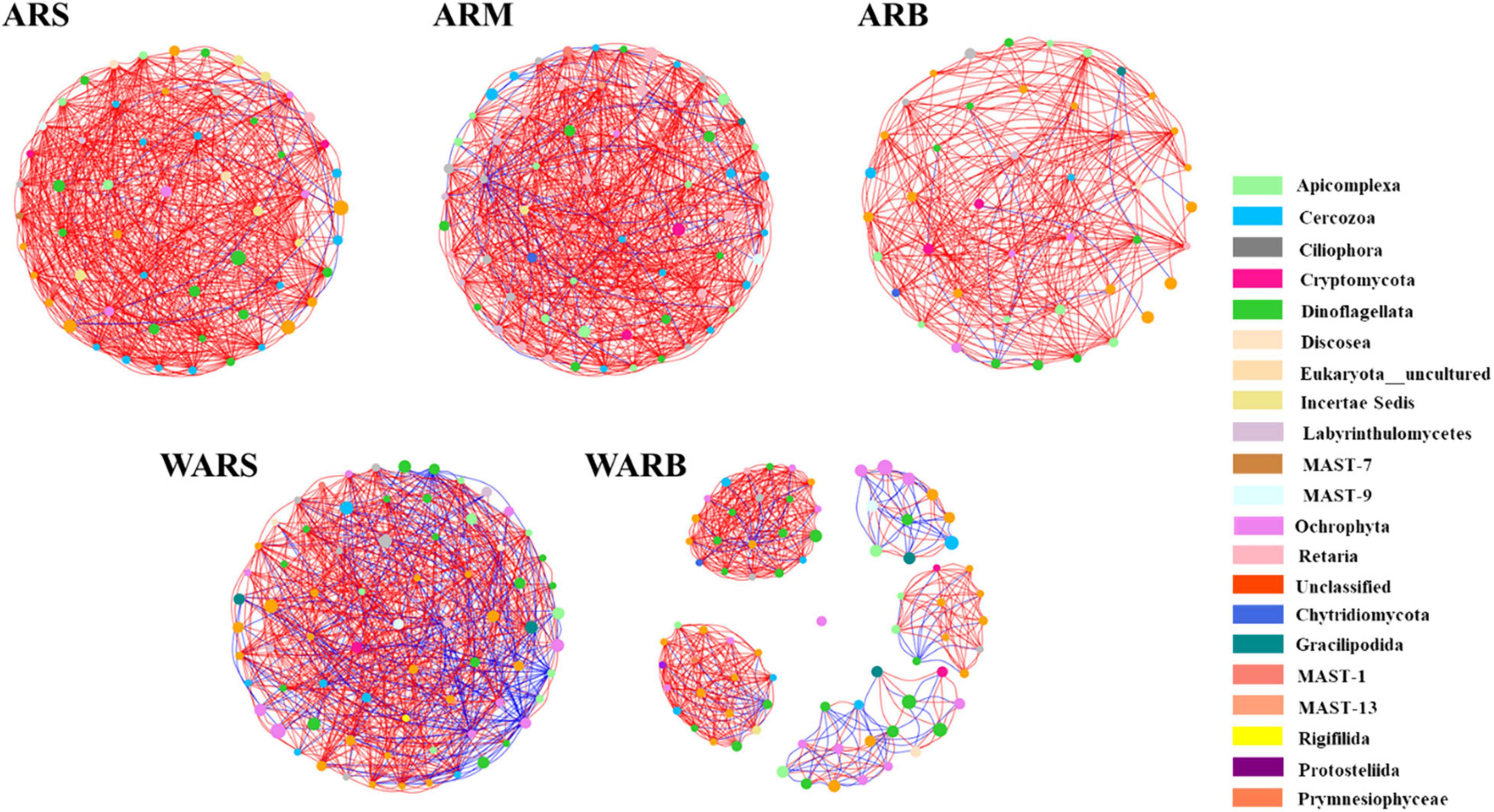
Figure 6. Co-occurrence networks of protist communities. Networks are colored by phylum. The size of the node indicates the abundance of the corresponding OTU. A red line indicates a positive interaction between two individual nodes, while a blue line indicates a negative interaction.
According to Table 3, the interaction network in WARB had the largest total number of nodes, and the ARS network had the highest degree of connection. In the vertical direction, as the depth increased, the total number of nodes in the WAR network increased, but the degree of connection decreased. From ARS to ARB, the total number of nodes in the network increased and then decreased. The degree of connection continued to decrease. In the horizontal direction, the number of nodes participating in network interactions from ARs to WARB increased, but the connectivity decreased. A shorter average path length indicates that most species in the network are connected through a few intermediate species. The clustering coefficient can measure the correlation in a network. Compared with that of WARB, the average path length of each group of ARS, ARM, ARB, and WARS was smaller, and the clustering coefficient was higher, indicating that these four groups of protist networks were more complex than the WARB group network and that their internal connections were closer. Density is employed to describe the complexity of the network structure. The complexity of the AR network was higher than that of the WAR network. As the depth increased, the complexity of the AR network decreased and then increased. The protist networks in the ARs and WAR were dominated by positive correlations. The relative numbers of positive correlations in each layer of the ARs occupied more than 90%. Among the different layers of the ARs, the module coefficient of the ARB network was larger than that of the ARS and ARM networks. Comparing the ARs and the WAR, the module coefficient of the AR networks was higher than that of the WAR networks.
In general, the WARS network was more complex than the WARB network. Compared with those of the surface and bottom layers of the seawater, the networks of the three sampling locations on the ARs were more complex, especially those for the upper and bottom layers of the ARs.
There was a certain degree of similarity in the composition of protist network nodes at the phylum level. The phyla that existed in each network were Dinoflagellata, Cercozoa, Apicomplexa, Ciliophora, Cryptomycota, Ochrophyta, and Unclassified. The shared phyla occupied more than 79% of the nodes in each network, and the proportion increased with an increase in depth. In the horizontal contrast, the proportion of Dinoflagellata and Ochrophyta for WARB network nodes was higher than that for AR networks, while the proportions of Cercozoa, Apicomplexa, Ciliophora and Cryptomycota were lower than those for AR networks.
Although the shared phyla occupied more network nodes and showed a greater abundance, their importance in the group was not significant. Average closeness centrality reflects the closeness between a node and other nodes in a network. The closer a node is to other nodes, the greater the proximity centrality and the more important it is. The top 6 phyla and their abundances in each network based on the average closeness centrality are listed in Supplementary Table 2. The data showed that the phyla with lower abundances in the ARS, ARM, and ARB networks had higher average closeness centrality, which meant that they were more critical in the protist community. This phenomenon was not observed in the WAR networks, especially WARS. Unclassified and Ochrophyta with higher abundances had higher average closeness centrality. In addition, the phyla with high average closeness centrality in the WAR networks did not correspond to the key phyla in the protist community of ARs. For example, the AR co-occurrence networks showed that Labyrinthulomycetes was a key phylum, but its average closeness centrality was small or it did not appear in the WAR networks. Ochrophyta had the highest average closeness centrality in the WARB network, but it was not critical in each layer of the ARs.
Environmental Drivers
The environmental factors differed significantly among the sampling locations (PERMANOVA, P < 0.01). To assess the role of environmental variables in shaping protist community composition, a redundancy analysis was conducted (Figure 7). DO and T had the strongest influence on the distribution and composition of protists (P < 0.01) (Table 4). The correlations between protist abundance and environmental parameters measured at the sampling locations were calculated (Spearman analysis). For example, DO was positively correlated with Cercozoa relative abundance (P < 0.05), DO was extremely positively correlated with MAST-9 relative abundance (P < 0.01), T was negatively correlated with Chytridiomycota relative abundance (P < 0.05), and DO was extremely negatively correlated with Chytridiomycota relative abundance (P < 0.01). The Spearman correlation coefficients showed that approximately 15% of the protists were significantly correlated with the environmental parameters (P < 0.05).
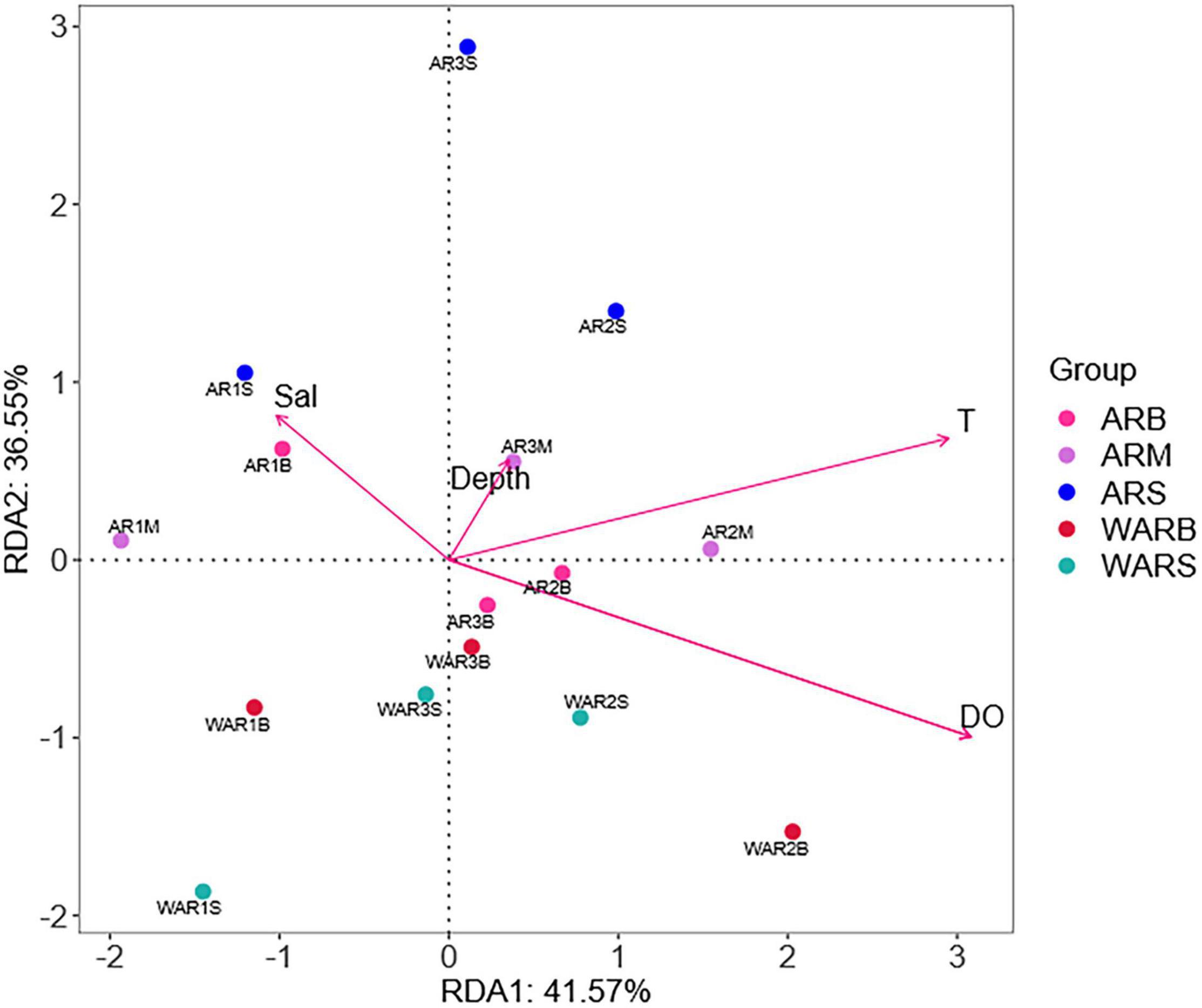
Figure 7. Redundancy analysis (RDA) based on the Bray–Curtis dissimilarity matrix. Numbers represent sample names; different colors represent sample groups in different sampling locations; arrows represent environmental factors; the angles between environmental factors represent positive and negative correlations between environmental factors (acute angle: positive correlation; obtuse angle: negative correlation; right angle: no correlation); and the closer the projection points and arrows are, the closer the relationship between the sample and the environmental factor is. The closer the projection points are, the more similar the attribute values of the environmental factors among samples are; that is, the environmental factors have the same influence on the samples.
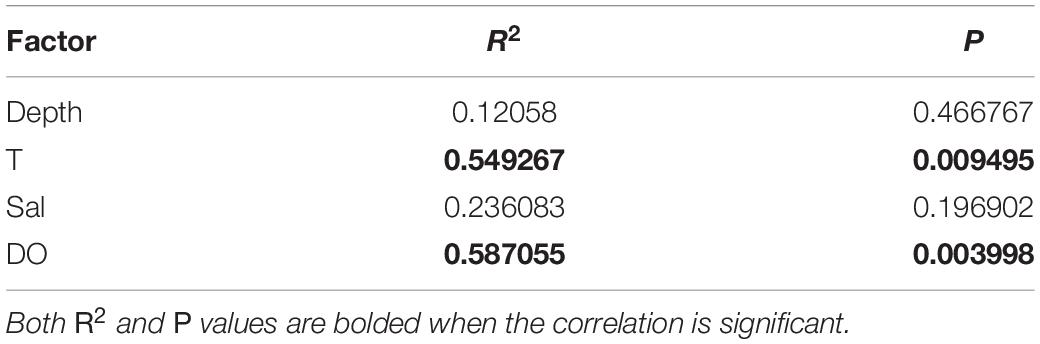
Table 4. Spearman analysis of community variability in terms of Sal, DO, T, and depth among sampling locations.
Discussion
Community Structure Reflects the Increase in the Spatial Heterogeneity of Artificial Reefs
High connectivity due to strong current transportation could weaken dispersal limitation and cause high dispersal ability (Xu et al., 2020). When ARs are placed on the bottom of the sea, the incoming flow is hindered by the ARs, resulting in flow separation. The seawater rises up to form an upwelling, which generally exists in the flow field effect of ARs under various construction modes and can promote and accelerate the water exchange between the seabed and the sea surface (Dongha et al., 2017; Guo Y. et al., 2020). There was also a strong bottom-up interaction of protists, so the interaction of protists between the surface and bottom layers of the seawater was the highest. Affected by the proportion of AR-associated protists, the degree of interaction between ARs and WAR was lower than that between the surface and bottom layers of the seawater. The distance between layers of the ARs was relatively shorter, but the degree of interaction was lower, indicating that compared with the artificial habitat itself, there was a higher degree of overlap between the artificial habitat and the adjacent seawater. Previous studies on single habitats have disregarded the strong species associations that may exist between artificial habitats and adjacent environments.
The flow field effect causes the nutrients and organic matter deposited on the sea floor to rise to the surface of the seawater, providing a rich material basis for the protists in the upper layer, but it also decreases the transparency of the seawater. This causes the growth of deep-layer protists to be limited by light (Jiang et al., 2018). Light restriction produced a trend in which the diversity of protist communities in the WAR and ARs decreased with an increase in sea depth. The protist biodiversity of surface water in Daya Bay was always higher than that of bottom water in different seasons (Zhu et al., 2020). The abovementioned two conclusions are consistent.
In addition, seawater and ARs belong to different habitat types. There were differences in the α-diversity and β-diversity of protists associated with ARs and WAR, which supported the uneven distribution of microbial diversity in different habitat types (Thompson et al., 2017). Habitat heterogeneity has been regarded as one of the main determinants of biodiversity (Horner-Devine et al., 2004). For example, in a comparison of protist communities in soil, freshwater and seawater ecosystems, soil α-diversity was the highest, and freshwater β-diversity was the highest (Singer et al., 2021). The spatial factors in the Yangtze River plume and its adjacent waters have an impact on the diversity of protist biodiversity (Guo X. et al., 2020). In this study, the difference in biodiversity between different layers of the ARs also proved that the construction of artificial habitats had increased the degree of spatial heterogeneity in the sea area and created a diversified local habitat in a limited space to support a variety of protists. Community structure and function provide the conditions. The ARs provided conditions for the formation and function of a variety of protist communities.
Most of the single-celled photosynthetic protists in aquatic systems are mixed trophic species. Dinoflagellata and Ochrophyta are mixed trophic protists. The ratio of their autotrophic and heterotrophic activities will be affected by many factors, such as light, nutrient elements and their feeding particles (Wang et al., 2018). The protist communities in the coral reef area of Daya Bay were dominated by Bacillariophyta, and the high species diversity and quantity of Bacillariophyta may be an important feature of the distribution of phytoplankton communities in coastal coral reef areas (Zhu et al., 2021). The AR area in Daya Bay was consistent with the coral reef area; both contained a higher abundance of single-celled phytoplankton (Qin et al., 2019). In this study, the abundance of Dinoflagellata and Ochrophyta in adjacent water bodies accounted for more than half of the total abundance, and the maximum abundance in bottom seawater was 61%. This finding shows that the biological effects produced by ARs are consistent in various sea areas and have the ecological function of promoting the growth of single-celled phytoplankton in sea areas. A large number of autotrophic protists in seawater convert dissolved inorganic carbon (DIC) into dissolved organic carbon (DOC) or actively use dissolved organic matter (DOM) (Wang et al., 2018). They serve as the starting point of the food chain or food web to provide rich food sources for the second trophic level. Compared with WAR, the proportion of heterotrophic protists in the composition of the AR-associated protist community is higher. The individual size and predation characteristics of different heterotrophic protists vary greatly. These protists can prey on various low-level organisms, such as algae, bacteria and other protists (Mohamed and Al-Shehri, 2013; Dayel and King, 2014). AR-associated protists consume carbon fixed by autotrophic organisms and then produce CO2 through respiration to return to the natural environment. They occupy multiple trophic levels in the marine food chain and food web and participate in the transfer and transformation of carbon. In addition, the process of heterotrophic protists preying on bacteria can also affect the community structure of bacteria, thereby indirectly affecting the degradation and conversion of DOC (Saleem et al., 2016).
Interspecific Interactions Reflect That Artificial Reefs Provide Better Long-Term Coexistence Conditions
There are multiple interactions among species in a biological community, such as symbiosis, parasitism, competition, and predation. These interactions determine the coexistence mode (Liu et al., 2019). Co-occurrence network analysis can reveal the direct or indirect interactions between microorganisms and biological and environmental factors (Zhou et al., 2011; Morris et al., 2019) and is widely utilized to study the interactions among microorganisms that coexist in the natural environment (Proulx et al., 2005). Therefore, in this paper, a co-occurrence network of five protist communities was constructed, focusing on the internal associations between the different layers of ARs and WAR and between ARs and WAR.
The connections in a symbiotic network are related to the interaction between nodes or niche overlap. The existence of positive interspecific associations is considered to be the cornerstone of microbial ecology in marine systems (Fuhrman, 2009). Positive correlations mean that genera co-occur more than expected by chance, which may be attributed to mutualism, parasitism, or predation, or alternatively, shared niche preferences. Negative correlations may suggest competitive exclusion, or alternatively, preferences for different niches (Mundra et al., 2021). The protist networks in the ARs and WAR were dominated by positive correlations. Compared with those in the WAR, the relative numbers of positive correlations in each layer of the ARs were higher. This finding indicated that there were beneficial interactions within the protist community in ARs. The surface of the ARs supported protist communities that were different from those in the WAR and that were more conducive to the survival of species.
The network module coefficient can indicate the degree of niche differentiation (Zhu et al., 2020). Among the different layers of the ARs, the module coefficient of the ARB network was larger than that of the ARS and ARM networks. Comparing the ARs and WAR, the module coefficient of the AR networks was higher than that of the WAR networks. The results showed that the degrees of niche differentiation of the protists in the lower and upper layers of the AR were higher than those in the middle and that the niche differentiation of the ARs was higher than that of the WAR. High modularity may cause increased ecosystem complexity and stability (Wang et al., 2016). Combining these results with the density results reveals that the AR-associated protist communities show stronger stability when resisting external environmental disturbances.
Ecological Function Reveals That Protists Associated With Artificial Reefs and WAR Contribute to the Microbial Carbon Pump
Closeness centrality reflects the closeness between a node and other nodes in the network and can be applied to identify key phyla. There were differences in key phyla of protists between the ARs and WAR, indicating that artificial habitats and adjacent seawater have different key species compositions. This phenomenon may be related to the dependent medium. For example, a study of bacterial communities in glacier-fed aquatic systems revealed that the main biomarkers in the water and sediment were inconsistent (Zhang et al., 2021).
Key taxa are drivers of microbial community structure and functioning (Banerjee et al., 2018). The first key phyla associated with the ARs and WAR and their abundances are listed in Table 5. Ciliophora was the first key phylum in WARS. This phylum serves not only as a primary consumer of bacteria, autotrophic and heterotrophic microplankton and nanoplankton (Weisse, 2017) but also as a food source for marine organisms, such as copepods (He et al., 2011). The coral-zooplankton symbiotic system in coral reefs can effectively facilitate the recycling of nutrients and energy. Zooplankton are the predators of primary producers; they also enter the coral reef food chain through trophic relationships as predators (mainly ciliates) (Rosenfeld et al., 1999; Tan et al., 2010). ARs are underwater anthropogenic structures deployed on the seabed near the coast to mimic natural reefs in terms of ecological functions (Lee et al., 2018). According to the results of relative abundance, surface seawater has sufficient light and nutrient conditions. The highest abundance of Dinoflagellata and Ochrophyta promoted the fixation of carbon, which also provided food security for the functional driving role of Ciliophora. Surface seawater effectively promoted the flow of carbon in the food chain and microbial food web. The first key group in the bottom seawater was Ochrophyta; it had a high abundance, indicating abundant primary producers that directly participate in the fixation of inorganic carbon through photosynthesis.
Picoeukaryotes are single-celled eukaryotes that represent the smallest size class of protists (0.2–2 μm), photosynthetic picoeukaryotes can have an important role in primary production (Orsi et al., 2018). Protist grazing is an important source of mortality for picoeukaryotes in general (Landry et al., 2011). Studies have shown that in the eastern North Pacific and in the Arctic Ocean, heterotrophic protists (such as Ciliophora) feed on microeukaryotic phytoplankton. The microbial loop starts from photosynthetic organisms (pico and microphytoplankton, Synechococcus, and Prochlorococcus) (He et al., 2011; Orsi et al., 2018). In this study, the AR-associated key phyla with large values of closeness centrality were heterotrophic protists, which may feed on photosynthetic protists in adjacent seawater. In contrast to the WAR, these key phyla did not have high abundance. Previous studies have shown that the impact of key taxa on community structure is attributed to strong ecological associations rather than high abundance (Shi et al., 2016; Banerjee et al., 2018), and the results shown by the AR network were consistent with this conclusion. A comparison of the top three key phyla in the upper, middle and lower protist communities of the ARs indicated that the key importance of Ciliophora and Cryptomycota gradually decreased from top to bottom. In aquatic and soil ecosystems, Cryptomycota and its hosts are very common. These organisms are secondary consumers in the food chain based on producers or debris and can effectively transfer carbon and energy from their hosts (primary consumers) to phytophagous zooplankton and other tertiary consumers. The process is likely to fine-tune the dynamics of the food chain and to increase the complexity of the food web (Bass et al., 2018). Both Reteria and Cercozoa belong to Rhizaria; their importance decreased first and then increased in the vertical direction. Labyrinthulomycetes was a key phylum that maintained high closeness centrality in all layers of the ARs. Previous studies have shown that the organisms in Labyrinthulomycetes are widely distributed, with high seasonal and regional abundance (Bongiorni and Dini, 2002). Researchers have discovered not only that Labyrinthulomycetes participate in the carbon transfer process of the marine food chain as predators of many fish and zooplankton but also their other functions and potentials in the marine carbon cycle. Labyrinthulomycetes protists have a unique ectoplasmic reticulum structure, which has multiple functions, such as adhesion, secretion, penetration and absorption, which can help them attach to granular microhabitats and consume granular and dissolved organic resources. Second, they can secrete a large amount of exopolysaccharides and promote the aggregation and sedimentation of organic particles, thereby contributing to the marine biological carbon pump. Labyrinthulomycetes can also secrete a variety of extracellular hydrolases to promote the decomposition and mineralization of marine organic matter to provide nutrients for themselves (Bai et al., 2021). The AR network of protists in this study showed that Labyrinthulomycetes was closely related to other species, so this phylum may have an important role in regulating the carbon storage efficiency of biological carbon pumps in AR areas.
In conclusion, the driving phyla of protist community functions were different in artificial habitats and adjacent seawater. The function of the adjacent seawater protist community was biased toward the initial fixation of carbon in the ocean carbon cycle. The high abundance of autotrophic protists may provide sufficient food sources for various AR-associated heterotrophic protists and ensure that key phyla can perform ecological functions. These protists will have more biological effects on biological carbon pumps through various processes, such as predation, excretion, degradation, and promotion of the aggregation and deaggregation of particulates.
Conclusion
In summary, this study chose the ARs of Bailong Pearl Bay as an example to analyze the differences and associations of the structure and function of the protist community in artificial habitats and adjacent environments. Protist diversity and community structure differed between the artificial habitat and the adjacent environment. Community structure also differed between layers of the same habitat. The key topological features of protist networks indicated a larger number of mutualistic relationships between the species of the protist community formed by the artificial habitat, which had higher complexity and stability. The construction of artificial habitats can create suitable habitats and coexistence conditions for more species. The key phyla differed between artificial habitats and adjacent seawater. These phyla may participate in the same food chain or microbial food web in a limited space and have roles in carbon fixation, transfer and transformation. In addition, a variety of key phyla have biological roles in biological carbon pumps related to their own ecological characteristics.
Data Availability Statement
The data presented in the study are deposited in the NCBI repository (https://www.ncbi.nlm.nih.gov/), accession number PRJNA788006.
Author Contributions
CQ conceived of the study and participated in its design. RL and YG drafted the manuscript and performed the field experiments. SZ provided scientific advice. DJ, HZ, JW, and NZ sorted the data. All authors have read and agreed to the published version of the manuscript.
Funding
This study was supported by the National Key Research and Development Program of China (No. 2018YFD0900905), Hainan Provincial Natural Science Foundation of China (No. 321CXTD446), the Government Commissioned Project of Fangchenggang Agriculture and Rural Bureau (No. FCZC2019-G3-10045-GXYL), the Key Research and Development Program of Fangchenggang (No. AB20014022), and South Sea Fisheries Research Institute, Chinese Academy of Fishery Sciences (No. 2020YJ04).
Conflict of Interest
The authors declare that the research was conducted in the absence of any commercial or financial relationships that could be construed as a potential conflict of interest.
Publisher’s Note
All claims expressed in this article are solely those of the authors and do not necessarily represent those of their affiliated organizations, or those of the publisher, the editors and the reviewers. Any product that may be evaluated in this article, or claim that may be made by its manufacturer, is not guaranteed or endorsed by the publisher.
Acknowledgments
We would like to thank Wentao Zhu and Wanni Pan for helping to complete the sample collection and field survey. We also thank the editor and reviewers for their conductive comments on our present work.
Supplementary Material
The Supplementary Material for this article can be found online at: https://www.frontiersin.org/articles/10.3389/fmars.2021.823409/full#supplementary-material
Footnotes
References
Bai, M., Xie, N., He, Y., Li, J., Collier, J. L., Hunt, D. E., et al. (2021). Vertical community patterns of Labyrinthulomycetes protists reveal their potential importance in the oceanic biological pump. Environ. Microbiol. [Epub ahead of print]. doi: 10.1111/1462-2920.15709
Banerjee, S., Schlaeppi, K., and Van Der Heijden, M. G. A. (2018). Keystone taxa as drivers of microbiome structure and functioning. Nat. Rev. Microbiol. 16, 567–576. doi: 10.1038/s41579-018-0024-1
Bass, D., Czech, L., Williams, B. A. P., Berney, C., Dunthorn, M., Mahe, F., et al. (2018). Clarifying the relationships between microsporidia and cryptomycota. J. Eukaryot. Microbiol. 65, 773–782. doi: 10.1111/jeu.12519
Bolding, B., Bonar, S., and Divens, M. (2010). Use of artificial structure to enhance angler benefits in lakes, ponds, and reservoirs: a literature review. Rev. Fish. Sci. 12, 75–96. doi: 10.1080/10641260490273050
Bongiorni, L., and Dini, F. (2002). Distribution and abundance of thraustochytrids in different Mediterranean coastal habitats. Aquat. Microb. Ecol. 30, 49–56. doi: 10.3354/ame030049
Claassens, L., and Harasti, D. (2020). Life history and population dynamics of an endangered seahorse (Hippocampus capensis) within an artificial habitat. J. Fish. Biol. 97, 974–986. doi: 10.1111/jfb.14452
Dayel, M. J., and King, N. (2014). Prey capture and phagocytosis in the choanoflagellate Salpingoeca rosetta. PLoS One 9:e95577. doi: 10.1371/journal.pone.0095577
Dias, G. M., Dutra, F. S., and Duarte, R. C. (2021). Artificial habitats induce plasticity in colonies of the marine bryozoan Schizoporella errata. J. Exp. Zool. B Mol. Dev. Evol. 336, 239–249. doi: 10.1002/jez.b.22942
Dongha, K., Jinho, W., and Bae, N. W. (2017). Intensively stacked placement models of artificial reef sets characterized by wake and upwelling regions. Mar. Technol. Soc. J. 51, 60–70.
Falcao, M., Santos, M. N., Vicente, M., and Monteiro, C. C. (2007). Biogeochemical processes and nutrient cycling within an artificial reef off Southern Portugal. Mar. Environ. Res. 63, 429–444. doi: 10.1016/j.marenvres.2006.12.001
Finlay, B. J., and Esteban, G. F. (1998). Freshwater protozoa: biodiversity and ecological function. Biodivers. Conserv. 7, 1163–1186.
Folpp, H. R., Schilling, H. T., Clark, G. F., Lowry, M. B., Maslen, B., Gregson, M., et al. (2020). Artificial reefs increase fish abundance in habitat-limited estuaries. J. Appl. Ecol. 57, 1752–1761. doi: 10.1111/1365-2664.13666
Fuhrman, J. A. (2009). Microbial community structure and its functional implications. Nature 459, 193–199. doi: 10.1038/nature08058
Guo, D., Zhou, L., Wang, G., Lai, H., Bi, S., Chen, X., et al. (2020). Use of artificial structures to enhance fish diversity in the Youjiang River, a dammed river of the Pearl River in China. Ecol. Evol. 10, 13439–13450. doi: 10.1002/ece3.6949
Guo, X., Wu, L., and Huang, L. (2020). Spatiotemporal patterns in diversity and assembly process of marine protist communities of the Changjiang (Yangtze River) plume and its adjacent waters. Front. Microbiol. 11:579290. doi: 10.3389/fmicb.2020.579290
Guo, Y., Zhang, S., and Lin, J. (2020). Study on unit reef construction mode based on upwelling effects. S. China Fish. Sci. 16, 71–79.
Hall, A. E., Herbert, R. J. H., and Stafford, R. (2021). Temporal and spatial variation in adult and juvenile mobile fauna associated with natural and artificial coastal habitats. Mar. Biol. 168:19. doi: 10.1007/s00227-021-03823-0
He, J., Cui, S., Zhang, F., He, P., and Lin, L. (2011). Progress in research on the marine microbial loop in the Arctic Ocean. Acta Ecol. Sin. 31, 294–301.
Höjesjö, J., Gunve, E., Bohlin, T., and Johnsson, J. I. (2015). Addition of structural complexity - contrasting effect on juvenile brown trout in a natural stream. Ecol. Freshw. Fish 24, 608–615. doi: 10.1111/eff.12174
Horner-Devine, M. C., Carney, K. M., and Bohannan, B. J. (2004). An ecological perspective on bacterial biodiversity. Proc. Biol. Sci. 271, 113–122. doi: 10.1098/rspb.2003.2549
Janiak, D. S., and Branson, D. (2021). Impacts of habitat and predation on epifaunal communities from seagrass beds and artificial structures. Mar. Environ. Res. 163:105225. doi: 10.1016/j.marenvres.2020.105225
Jiang, X., Ke, Z., Xiang, C., Li, J., Tan, Y., and Huang, L. (2018). Variations of picoplankton in Daya Bay in relation to environmental factors in summer and winter. Ecol. Sci. 37, 1–10.
Landry, M. R., Selph, K. E., Taylor, A. G., Décima, M., Balch, W. M., and Bidigare, R. R. (2011). Phytoplankton growth, grazing and production balances in the HNLC equatorial Pacific. Deep Sea Res. II Top. Stud. Oceanogr. 58, 524–535. doi: 10.1016/j.dsr2.2010.08.011
Lee, M. O., Otake, S., and Kim, J. K. (2018). Transition of artificial reefs (ARs) research and its prospects. Ocean Coast. Manag. 154, 55–65. doi: 10.1016/j.ocecoaman.2018.01.010
Lemoine, H. R., Paxton, A. B., Anisfeld, S. C., Rosemond, R. C., and Peterson, C. H. (2019). Selecting the optimal artificial reefs to achieve fish habitat enhancement goals. Biol. Conserv. 238:108200. doi: 10.1016/j.biocon.2019.108200
Liu, L., Chen, H., Liu, M., Yang, J. R., Xiao, P., Wilkinson, D. M., et al. (2019). Response of the eukaryotic plankton community to the cyanobacterial biomass cycle over 6 years in two subtropical reservoirs. ISME J. 13, 2196–2208. doi: 10.1038/s41396-019-0417-9
Massana, R. (2011). Eukaryotic picoplankton in surface oceans. Annu. Rev. Microbiol. 65, 91–110. doi: 10.1146/annurev-micro-090110-102903
Mohamed, Z. A., and Al-Shehri, A. M. (2013). Grazing on Microcystis aeruginosa and degradation of microcystins by the heterotrophic flagellate Diphylleia rotans. Ecotoxicol. Environ. Saf 96, 48–52. doi: 10.1016/j.ecoenv.2013.06.015
Morris, L. A., Voolstra, C. R., Quigley, K. M., Bourne, D. G., and Bay, L. K. (2019). Nutrient availability and metabolism affect the stability of coral-symbiodiniaceae symbioses. Trends Microbiol. 27, 678–689. doi: 10.1016/j.tim.2019.03.004
Mundra, S., Kjonaas, O. J., Morgado, L. N., Krabberod, A. K., Ransedokken, Y., and Kauserud, H. (2021). Soil depth matters: shift in composition and inter-kingdom co-occurrence patterns of microorganisms in forest soils. FEMS Microbiol. Ecol. 97:fiab022. doi: 10.1093/femsec/fiab022
Onitsuka, T., Kurogi, H., Niwa, K., Hayakawa, J., Terui, M., Asahi, T., et al. (2013). Succession of bacteria and benthic organisms on a newly-established artificial reef and neighboring natural rocky reefs at Nagai in Sagami Bay, Japan. Jpn. Soc. Aquac. Sci. 61, 277–292.
Orsi, W. D., Wilken, S., Del Campo, J., Heger, T., James, E., Richards, T. A., et al. (2018). Identifying protist consumers of photosynthetic picoeukaryotes in the surface ocean using stable isotope probing. Environ. Microbiol. 20, 815–827. doi: 10.1111/1462-2920.14018
Paxton, A. B., Newton, E. A., Adler, A. M., Van Hoeck, R. V., Iversen, E. S. Jr., Taylor, J. C., et al. (2020). Artificial habitats host elevated densities of large reef-associated predators. PLoS One 15:e0237374. doi: 10.1371/journal.pone.0237374
Pei, K., Wu, Y., and Yang, R. (2020). The earliest artificial reef test base in China——The summary of Fangchenggang Bailong Pearl Bay Marine Farm construction. Hebei Fish. 22, 27–63.
Proulx, S. R., Promislow, D. E., and Phillips, P. C. (2005). Network thinking in ecology and evolution. Trends Ecol. Evol. 20, 345–353. doi: 10.1016/j.tree.2005.04.004
Qin, C., Zhu, W., Ma, H., Duan, D., Zuo, T., Xi, S., et al. (2019). Are habitat changes driving protist community shifts? A case study in Daya Bay, China. Estuar. Coast. Shelf Sci. 227:106356. doi: 10.1016/j.ecss.2019.106356
Rosenfeld, Bresler, and Abelson (1999). Sediment as a possible source of food for corals. Ecol. Lett. 2, 345–348. doi: 10.1046/j.1461-0248.1999.00097.x
Rumbold, C., Battini, N., Giachetti, C. B., Castro, K. L., Obenat, S., and Schwindt, E. (2020). Biological invasions in artificial habitats: factors that determine the presence of native and exotic peracarid Crustacea species in Southwestern Atlantic. Mar. Biol. Res. 16, 340–355. doi: 10.1080/17451000.2020.1781187
Saleem, M., Fetzer, I., Harms, H., and Chatzinotas, A. (2016). Trophic complexity in aqueous systems: bacterial species richness and protistan predation regulate dissolved organic carbon and dissolved total nitrogen removal. Proc. Biol. Sci. 283:20152724. doi: 10.1098/rspb.2015.2724
Sandström, A., and Karås, P. (2002). Tests of artificial substrata as nursery habitat for young fish. J. Appl. Ichthyol. 18, 102–105.
Sheng, H. (2012). The Seasonal and Regional Differences of Benthic Macroalgae Communities in the Artificial Reefs of Laoshan Bay. Master’s thesis. Shandong: Ocean University of China.
Shi, S., Nuccio, E. E., Shi, Z. J., He, Z., Zhou, J., and Firestone, M. K. (2016). The interconnected rhizosphere: high network complexity dominates rhizosphere assemblages. Ecol. Lett. 19, 926–936. doi: 10.1111/ele.12630
Singer, D., Seppey, C. V. W., Lentendu, G., Dunthorn, M., Bass, D., Belbahri, L., et al. (2021). Protist taxonomic and functional diversity in soil, freshwater and marine ecosystems. Environ. Int. 146:106262. doi: 10.1016/j.envint.2020.106262
Soka, S., Hutagalung, R. A., and Yogiara</snm>, and Assisi, C. (2011). Population dynamic of Dendronephthya sp.-associated bacteria in natural and artificial habitats. HAYATI J. Biosci. 18, 57–60. doi: 10.4308/hjb.18.2.57
Stoeck, T., Bass, D., Nebel, M., Christen, R., Jones, M. D., Breiner, H. W., et al. (2010). Multiple marker parallel tag environmental DNA sequencing reveals a highly complex eukaryotic community in marine anoxic water. Mol. Ecol. 19, (Suppl. 1), 21–31. doi: 10.1111/j.1365-294X.2009.04480.x
Sweet, M. J., Croquer, A., and Bythell, J. C. (2011). Development of bacterial biofilms on artificial corals in comparison to surface-associated microbes of hard corals. PLoS One 6:e21195. doi: 10.1371/journal.pone.0021195
Tan, Y., Huang, L., Huang, X., Su, Q., Shi, X., and Huang, J. (2010). The relationships between ciliate composition, abundance, and environmental factors in Sanya Bay coral reef waters. Acta Ecol. Sin. 30, 6835–6844.
Thompson, L. R., Sanders, J. G., Mcdonald, D., Amir, A., Ladau, J., Locey, K. J., et al. (2017). A communal catalogue reveals Earth’s multiscale microbial diversity. Nature 551, 457–463. doi: 10.1038/nature24621
Wang, G., Bai, M., Xie, Y., Song, Z., Xie, N., and Zhang, S. (2018). Perspective and significance of unicellular protists in ocean carbon sink. Microbiol. China 45, 886–892.
Wang, Z., Liang, W., and Shao, J. (2016). Seasonal changes of phytoplamkton community in Daya Bay, South China Sea, between 2011 and 2012. Mar. Sci. 40, 53–58.
Weisse, T. (2017). Functional diversity of aquatic ciliates. Eur. J. Protistol. 61, 331–358. doi: 10.1016/j.ejop.2017.04.001
William Seaman, J. L. M. S. (1993). Artificial habitats for marine and freshwater fisheries (1991) - libgen.lc.pdf>. Rev. Fish Biol. Fish. 3:1.
Xu, Z., Li, Y., Lu, Y., Li, Y., Yuan, Z., Dai, M., et al. (2020). Impacts of the Zhe-min coastal Current on the biogeographic pattern of microbial eukaryotic communities. Prog. Oceanogr. 183:102309. doi: 10.1016/j.pocean.2020.102309
Yu, J., Chen, P., Tang, D., and Qin, C. (2015). Ecological effects of artificial reefs in Daya Bay of China observed from satellite and in situ measurements. Adv. Space Res. 55, 2315–2324. doi: 10.1016/j.asr.2015.02.001
Zhang, L., Delgado-Baquerizo, M., Shi, Y., Liu, X., Yang, Y., and Chu, H. (2021). Co-existing water and sediment bacteria are driven by contrasting environmental factors across glacier-fed aquatic systems. Water Res. 198:117139. doi: 10.1016/j.watres.2021.117139
Zhou, J., Deng, Y., Luo, F., He, Z., and Yang, Y. (2011). Phylogenetic molecular ecological network of soil microbial communities in response to elevated CO2. mBio 2:e00122-11. doi: 10.1128/mBio.00122-11
Zhu, W., Qin, C., Ma, H., Xi, S., Zuo, T., Pan, W., et al. (2020). Response of protist community dynamics and co-occurrence patterns to the construction of artificial reefs: a case study in Daya Bay, China. Sci. Total Environ. 742:140575. doi: 10.1016/j.scitotenv.2020.140575
Keywords: protists, artificial reefs, biodiversity, co-occurrence network, ecological functions
Citation: Li R, Guo Y, Qin C, Zhang S, Ji D, Zhang H, Wang J and Zhao N (2022) New Insight Into the Mechanism of Ecological Effects of Artificial Habitats: Elucidating the Relationship Between Protists Associated With Artificial Reefs and Adjacent Seawater. Front. Mar. Sci. 8:823409. doi: 10.3389/fmars.2021.823409
Received: 27 November 2021; Accepted: 20 December 2021;
Published: 12 January 2022.
Edited by:
Hui Zhang, Institute of Oceanology, Chinese Academy of Sciences (CAS), ChinaReviewed by:
Yaodong He, Tianjin University, ChinaZhi Huang, Temasek Polytechnic, Singapore
Zhongming Zheng, Ningbo University, China
Copyright © 2022 Li, Guo, Qin, Zhang, Ji, Zhang, Wang and Zhao. This is an open-access article distributed under the terms of the Creative Commons Attribution License (CC BY). The use, distribution or reproduction in other forums is permitted, provided the original author(s) and the copyright owner(s) are credited and that the original publication in this journal is cited, in accordance with accepted academic practice. No use, distribution or reproduction is permitted which does not comply with these terms.
*Correspondence: Chuanxin Qin, cWluY3hAc2NzZnJpLmFjLmNu
†These authors have contributed equally to this work and share first authorship