- 1National Oceanography Centre, University of Southampton, Southampton, United Kingdom
- 2British Antarctic Survey, National Environmental Research Council, Cambridge, United Kingdom
- 3School of Ocean Science, Bangor University, Bangor, United Kingdom
Climate-driven changes in disturbance are a major threat to ecosystem Functional diversity. The selective mechanisms underlying ecosystem response to disturbance are far from universal and remain the subject of scientific debate. Ice scouring of the shallow Antarctic benthos is one of the largest disturbance gradients in the natural environment and thus provides an opportunity to investigate how disturbance gradients influence functional structure of a biological assemblage. The Western Antarctic Peninsula, in particular, is a hotspot of climate-driven environmental change. Addressing how this system might respond to species loss is critical. Previous surveys across the shallowest 100 m of the seabed, detected unimodal changes in diversity and a shift in assemblage composition in response to disturbance gradients. This study investigated how functional traits and associated functional diversity change across the depth gradient. Our results revealed that selective mechanisms, such as disturbance filtering and inter-species competition, reduce functional redundancy at the extremes of the disturbance gradient. Our study highlights areas of potential vulnerability to future environmental change due to low functional redundancy. Threatening the important negative (mitigating) feedbacks on climate change, through blue carbon, currently provided by Antarctic continental shelf benthic assemblages.
Introduction
Disturbance in an assemblage, community or ecosystem is an abrupt change in conditions of at least one key resource, or controlling factor, for life (e.g., temperature, light, salinity, turbidity, availability of space, etc). Disturbance events occur in all natural ecosystems on varying time scales and magnitudes, and are key factors in controlling assemblage structure and function (Dell et al., 2019). Disturbance-diversity patterns and relationships are complex and often poorly understood, especially in the sea. These patterns are far from universal and some are under strong debate. However, some studies have found unimodal relationships, which some researchers attribute to the Intermediate Disturbance Hypothesis (IDH) (Connell, 1978; Hughes et al., 2007; Huston, 2014). The mechanism underlying this pattern is suggested as a combination of two factors, high levels of disturbance restricting species to all but the most disturbance tolerant, and low levels of disturbance allowing increased competitive exclusion (Mouillot et al., 2013). However, many exceptions to the IDH have been reported through both empirical (Bongers et al., 2009; Svensson et al., 2012) and theoretical studies (dos Santos et al., 2011).
Functional traits are defined as a morphological, physiological or behavioral trait, which affects fitness via growth, survival or reproduction (Violle et al., 2007). The loss of species in a system through disturbance may not necessarily reflect a decline in functional diversity, as high diversity assemblages may contain high functional redundancy, with multiple taxa (e.g., species) exhibiting strong overlap in traits (de Bello et al., 2007). This concept is fundamental to ecology (Cardinale et al., 2012) and crucial to understanding the response of species-rich environments to changing disturbance patterns (Mouillot et al., 2014). We follow the definitions and calculations for functional redundancy as defined by Ricotta et al. (2016) that species occupying the same functional space (i.e., similar trait values) are considered redundant or partially redundant as their loss would not reduce the functional volume occupied by the wider assemblage (indicated by functional richness). The ability of higher-diversity environments to compensate for species loss through replacement with other functionally similar species is considered to be an “insurance effect” (Wright et al., 2021). Such an effect is why higher levels of biodiversity may offer a buffer to ecosystems against loss of ecosystem functions, making them more resilient to species loss (Tilman et al., 2012; Isbell et al., 2015). However, findings from field studies suggest more complex responses, with resistance to initial disturbance (Pfisterer and Schmid, 2002) and the complementarity of species (Craven et al., 2016) as additional critical factors influencing the insurance effect.
The assemblages of the Antarctic shallows, particularly macro/megabenthos are ideal to test how functional redundancy varies across a disturbance gradient. High-latitude continental shelf Antarctic benthos are highly diversified (Teixidó et al., 2007) with the Southern Ocean macrobenthos estimated to exceed 5000 species (Aronson et al., 2007) and high regional biodiversity in the Western Antarctic Peninsula (WAP) polar benthos (Clarke and Johnston, 2003). In particular sessile suspension feeders tend to be a species-rich group and can dominate below 60 m depth, coinciding with the peak in diversity (Robinson et al., 2020). Although the assumption that these species are complementary (overlapping traits) is likely a simplification, as species utilise different components of sinking food particles (Obermüller et al., 2010) or seafloor habitats (Bowden et al., 2006). Further, the Antarctic shallows are a useful system to study disturbance gradients due to the ice scour gradient across depth, as macro/megabenthos tend to have no innate resistance against direct impact, resulting in a universally high mortality rate regardless of taxa or functional trait (Peck et al., 1999; Lee et al., 2001).
The continental shelf around Antarctica is anomalously deep, with the shelf break more than 800 m deep in places (Clarke et al., 2007). Whilst the seabed at 100 m depth typifies the depth of global continental shelves, around the Antarctic this depth represents the “shallows.” This depth zone contains one of the largest natural disturbance gradients on earth, due to the frequent scouring of icebergs (Barnes, 2017). Ice scour rates are predicted to shift dramatically in the Western Antarctic Peninsula (WAP) over the next century (Rogers et al., 2020) owing to calving from ice shelf collapse and glacier retreat (Morley et al., 2020), and reductions in both the duration and extent of sea ice (Parkinson, 2014). Throughout this study depth is used as a proxy for disturbance, however, depth is reported as many other environmental factors vary across 10–100 m depth range and were considered, for a part of this work [for a detailed description of environmental factor and their relative influence see Robinson et al. (2021)].
Recent work along the WAP narrowed the bathymetry of the peak in biodiversity to between 50 and 60 m depth, coincident with the depth of intermediate levels of ice scour disturbance, confirming expectations that ice scour may maintain high biodiversity across these depths (Robinson et al., 2021). Assuming that IDH does indeed explain the diversity-disturbance pattern seen across this depth gradient, the mechanisms behind this observation should be reflected in assemblage functional structure (Houseman and Gross, 2011; Villéger et al., 2011). Hence, at high disturbance levels, species with disturbance-sensitive traits will be filtered out (species filtering) (Venn et al., 2011) whereas at low disturbance levels those species with poor-competitive traits will be mainly excluded (Edwards et al., 2011).
Antarctic benthos are globally important as many species are endemic, only occurring within the Southern Ocean (SCAR-MarBIN) and provide important ecosystem services, such as “blue carbon” pathways from biologically fixed carbon to sequestration (Barnes and Sands, 2017; Bax et al., 2020; Cavanagh et al., 2021). The geography of accumulation of ‘blue carbon’ has been linked to the number of functional benthic groups (Barnes and Sands, 2017). As the climate drives rapid changes in the WAP including marine warming, longer phytoplankton blooms and changes in disturbance regime (Morley et al., 2020), the identification of areas of low functional redundancy could allow their use to predict potential disruption of these “blue carbon” pathways.
In this study, we aim to use the shallow Antarctic benthos as a case study to investigate changes to functional structure across one of the world’s largest disturbance gradients (Smale et al., 2008), as well as to provide important ecological information for an environment facing imminent change. To describe the change in functional structure from 10 to 100 m depth, we use Community-Weighted Means (CWM) of functional traits with multi-dimensional scaling. We then test how functional richness, evenness, divergence and redundancy change across depth when compared with theoretical null models. Our hypothesis is that functional redundancy will be significantly lower at the extremes of the depth range than null models predict. This outcome would suggest which selective mechanisms, such as species filtering by disturbance and competitive exclusion may be responsible and presents a robust model system for understanding the effects of a disturbance gradient on functional diversity.
Materials and Methods
Study Area
The study areas consisted of three sites spaced along a steeply sloping rocky shore located in Ryder Bay, Adelaide Island, Western Antarctic Peninsula (Figure 1). All sites had similar topography, orientation within the bay and proximity (all sites within 4 km) suggesting similar exposure to tidal current and iceberg scour, within the bay. The Rothera Time Series (RaTS) (Clarke et al., 2008) provides year-round, weekly oceanographic data across our study depths from 1997 onward. These sites are characterised by low temperatures (−1.9 to 2°C), highly variable seasonal sea ice cover and a strong seasonal summer phytoplankton bloom (Legge et al., 2017).
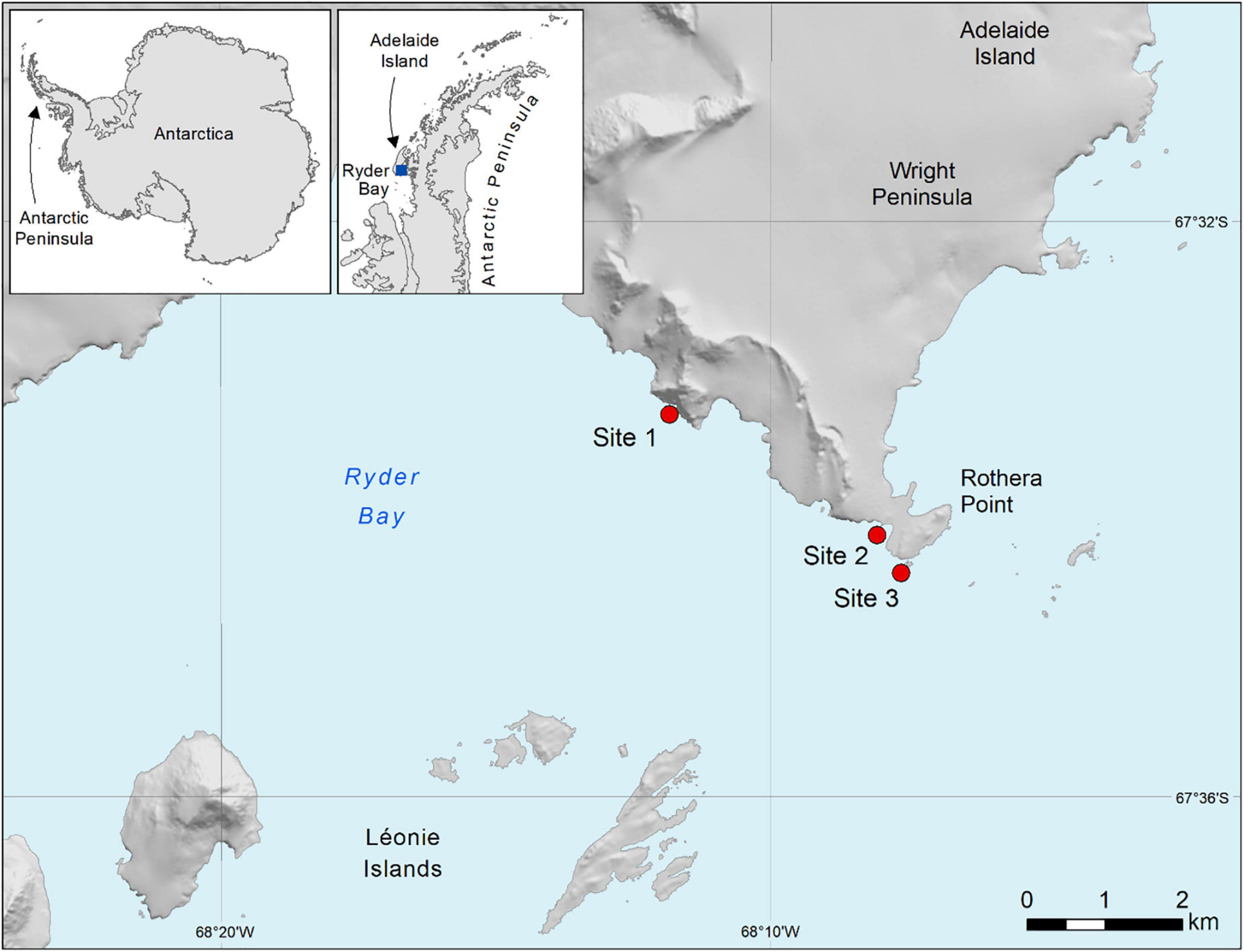
Figure 1. Map of the study area (sample sites are in red) in the Western Antarctic Peninsula, each site is a steep rock slope on Adelaide Island (67°35′S, 068°07′W). Map Modified from Robinson et al. (2021).
Macrofauna Sampling
At each of the three sites, 50 random areas of seabed were recorded at every 10 m depth interval from 10 to 100 m depth (total 1,500 images), collected between February and June 2016, using a modified mini-ROV, DeepTrekkerG2. Macro/megafauna (> 0.5 mm) were originally described across this depth gradient by Robinson et al. (2021) linking peaks in biodiversity to intermediate ice scour rates, with each specimen with the sample image being counted and identified to into morphotypes. Here, morphotypes are defined as a species or group of species that a have a distinct or conspicuous morphology that allows identification and catergorisation from other species across all sample images. All taxa were recorded as Operational Taxonomic Units (OTUs), as not all specimens could be identified to species level. Many taxa were identified to species level and confirmed through comparisons with reference collections, however, as most specimens were identified from images, broader morphotype categories were implemented. Functional traits were assigned to species names where possible, where taxonomic resolutions were coarser, functional information from a similar or same taxonomic group was used. Additional information on movement and trophic group was gathered from observations from the ROV video and images.
Functional Traits Calculations
From the 171 OTUs we quantified 4 functional traits: trophic group, adult mobility, body form and larvae type (Table 1). The framework for the functional trait analysis and functional diversity calculations follows the approach developed by de Bello et al. (2021). The food webs of polar shallow-water benthic assemblages are to date poorly explored; but see Norkko et al. (2007), Gillies et al. (2012, 2013), Jansen et al. (2018) and Marina et al. (2018). However, studies have shown a high degree of omnivory and a high abundance of predator-scavengers (Puccinelli et al., 2020). We collated trophic data from the literature (Supplementary Table 1) and combined the results of multiple studies to establish the breadth of traits occupied by single species (Table 1). Both categorical traits (trophic group and body form) were quantified using dummy variables with fuzzy coding to account for multiple feeding modes or similarities between body types (de Bello et al., 2021). Fuzzy coding assigns a single taxon or species a proportionate score across multiple categorical dummy variables to indicate the extent that the taxon exhibits each trait de Bello et al. (2021). Within categorical traits, even proportions were ascribed to different trophic groups and body forms (e.g., predator-scavengers were always 0.5 scavenger and 0.5 predator). This approach was utilised as species have the capacity to alter their diet due to local environmental variability and food availability (Peck et al., 2005; Rossi et al., 2019; Sporta Caputi et al., 2020), and body forms do not have intermediate values.

Table 1. List of functional traits of the macro and megabenthic fauna found between 10 and 100 m depth at 3 sites in Ryder Bay, Western Antarctic Peninsula (Figure 1) including the functional trait scale, descriptions and evidence source.
Body form functional traits categories were derived directly from previous functional groups ascribed to benthic macrofauna by Barnes and Sands (2017) and Jansen et al. (2018). Body forms such as “erect” and “flat” were employed to allow a degree of similarity between body forms such as “erect 3D-branching” and “erect 2D-laminar” to account for their similar raised position on the seafloor. “Hard” and “soft” body forms were also included as further modifiers between species that were covered by hard protective structures (external skeletons) and those that were not.
Adult mobility and larvae type functional traits were characterised into ordinal variables, based on distinct groups, with higher numbers indicating a greater degree of mobility or dispersal (Table 1). These mobility and larval types were restricted to broad classifications, as detailed information does not exist for all taxa [see Stanwell-Smith et al. (1999)]. Furthermore, environmental conditions, such as currents and season, combined with sparseness of taxon-specific larval information made it unlikely that an accurate estimation could be attempted, so a coarse resolution of data was adopted (Ershova et al., 2019).
Functional Structure Calculations
All statistical analysis was preformed using R v3.5.2. Community-Weighted Means were calculated for every trait as , for each sample, j, where pi is relative abundance of species i in the sample and Ti is the trait value of the same species and N is total species number in the sample (Díaz et al., 2007). All samples were grouped across both months, February and July, as multivariate analysis revealed no significant difference in assemblage structure (Supplementary Figure 1). We performed one-way ANOVA, to detect any significant change in functional trait expression across the 10–100 m depth gradient, with post-hoc turkey test implemented to identify which depths differed significantly. Body form traits present in < 5% of individuals were excluded from analysis, as we considered that too few representatives available to draw reliable conclusions. Pairwise functional distances between functional traits were computed using Gower distances, to allow the mixing of different types of variables, whilst giving them equal weighting (Legendre and Legendre, 2012). To visualise functional structure between samples across the depth gradient, CWM traits computed using Gower distances, were plotted in multidimensional space using Principle Coordinate Analysis (PCoA).
Functional diversity indices were calculated from Gower dissimilarity matrix using OTU functional traits following the framework developed by Villéger et al. (2008), Laliberté and Legendre (2010), and Mouillot et al. (2013). This approach produced the following four multidimensional facets of functional diversity: functional richness (FRic), functional evenness (FEve), functional divergence (FDiv) and functional redundancy (FRed), as proposed by Ricotta et al. (2016). Linear and polynomial regression analyses were used to identify the functional change across depth, with AIC values used to distinguish between models of best fit.
Null Model Construction
To test whether OTUs functional traits changed due to depth and/or the underlying environmental conditions, or if the changes were a result of OTUs richness and abundance change, patterns in OTUs functional traits were tested against randomised null models. We performed 10,000 randomisations of each null model in order to calculate the Standardized Effect Size (SES) for each functional diversity index as described by Gotelli and McCabe (2002), . Null models were created by randomizing the trait matrix, assigning each OTU as a random functional identity; this had the benefit of preserving OTU richness, abundance and any functional trait correlations across all models (de Bello et al., 2021). As SES values are expressed in units of standard deviation from the null mean, a non-directional one-sample Z test was implemented to test if there was a significant difference between the observed mean or the null mean (μ = 0), null standard deviation set at 3 (99.73% of the population).
Results
Community-Weighted Means Functional Traits
Community-Weighted Means (CWM) trophic groups indicated that the shallows were dominated by an abundance of grazers (Figure 2B) and autotrophs (Figure 2A), peaking between 10 and 20 m depth (0.63 and 0.30 CWM abundance, respectively), reaching a near 0 CWM abundance at 50 m and 80 m, respectively (Figure 2M). In contrast, suspension feeders were characterised by a high CWM abundance at 80–90 m depth with a unimodal distribution (Figure 2F). The predators (Figure 2C), scavengers (Figure 2D) and deposit feeders (Figure 2E) increase in proportional with depth all asymptote of 0.12 CWM abundance was reach at 30 m for predators and the rest at 60 m. We found that 3D-branching forms (Figure 2G) increased with depth across the entire 10–100 m gradient to 0.06 CWM abundance, whilst erect forms generally increased with depth but approached an asymptote at 50 m depth (0.10 CWM abundance). Hard body forms (Figure 2H) peaked in the shallows (0.6 CWM abundance; 10 m depth), until reaching near zero abundance at 50 m depth. The flat body form (Figure 2I), covering both encrusting fauna and taxa that have a low profile, such as asteroids and echinoids, were highly prevalent encompassing nearly half of individuals (0.50 CWM abundance) but lowest at 10–20m depth (between 0.30 and 0.40 CWM abundance). The tubeworm body form (Figure 2J) had a unimodal distribution across depth, with CWM abundance peaking around 70 m depth (0.10 CWM abundance). Community-Weighted Means adult mobility (Figure 2K) and larval type (Figure 2L) showed a similar distribution with decreasing abundance from 1.54 CWM mobility and 2.77 CWM larvae dispersal at 10 m before reaching an asymptote at 50 m depth, with 0.75 CWM mobility and 2.30 CWM mobility. All levels or categories were significantly different across depth regardless of the trait considered (see Supplementary Table 5).
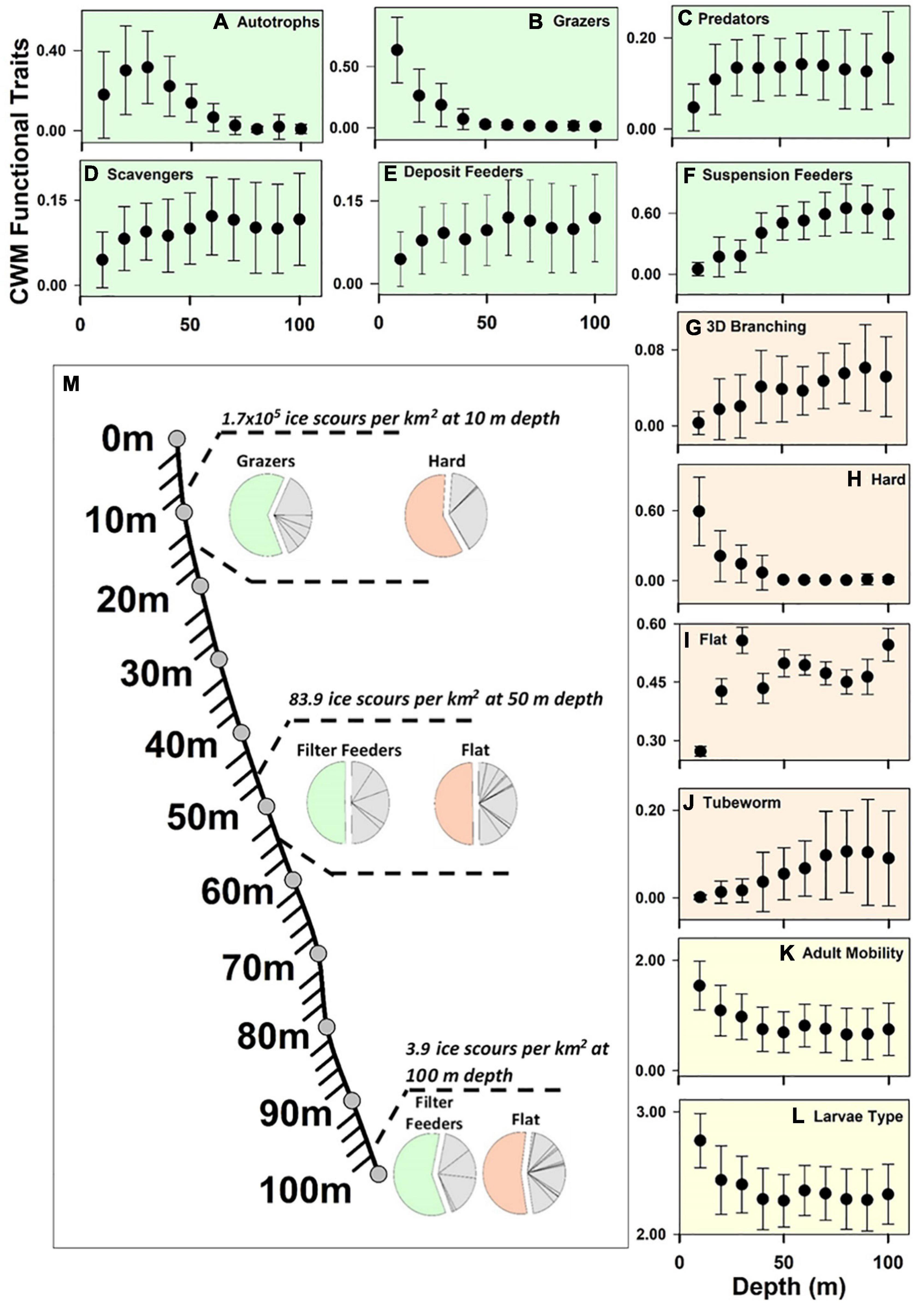
Figure 2. Scatterplots of average Community Weighted Mean functional traits across 10 to 100 m depth. Green scatter plots contain feeding modes, orange body forms and yellow the two univariate functional traits adult mobility and larval types. Error bars show standard deviation around the mean. The Bathymetry chart is measured from Site 3 Cheshire Island (Figure 1). Pie charts of feeding mode and body form show the major functional trait at 10, 50 and 100 m depth, with equivalent ice scour per square kilometers provided at each corresponding depth from Ryder bay (Bowler et al., 2019).
Principle Coordinate Analysis of Community-Weighted Means
Our PCoA analysis (Figure 3) showed significant correlation between the Euclidian distances of the first two principle axes and the CWM Gower distances (Mantel test, r = 0.65, p = 0.004, permutations = 200) observation demonstrates that most of the variability (78.0%) can be shown in two-dimensional functional space. Between 10 and 100 m depth there was no clear zonation in functional structure of individuals. There was a gradual shift between depths with a broad level of overlap, however, the degree of variability diminished at depths deeper than 50 m and there was an increased functional similarity across individuals (Figure 3). A greater degree of variation was seen in the functional structure at 50 – 100 m (Figure 3) which could be attributed the increase in the relative abundance of various erect body forms including 3D-branching and tubeworms (Figures 2F,G,J).
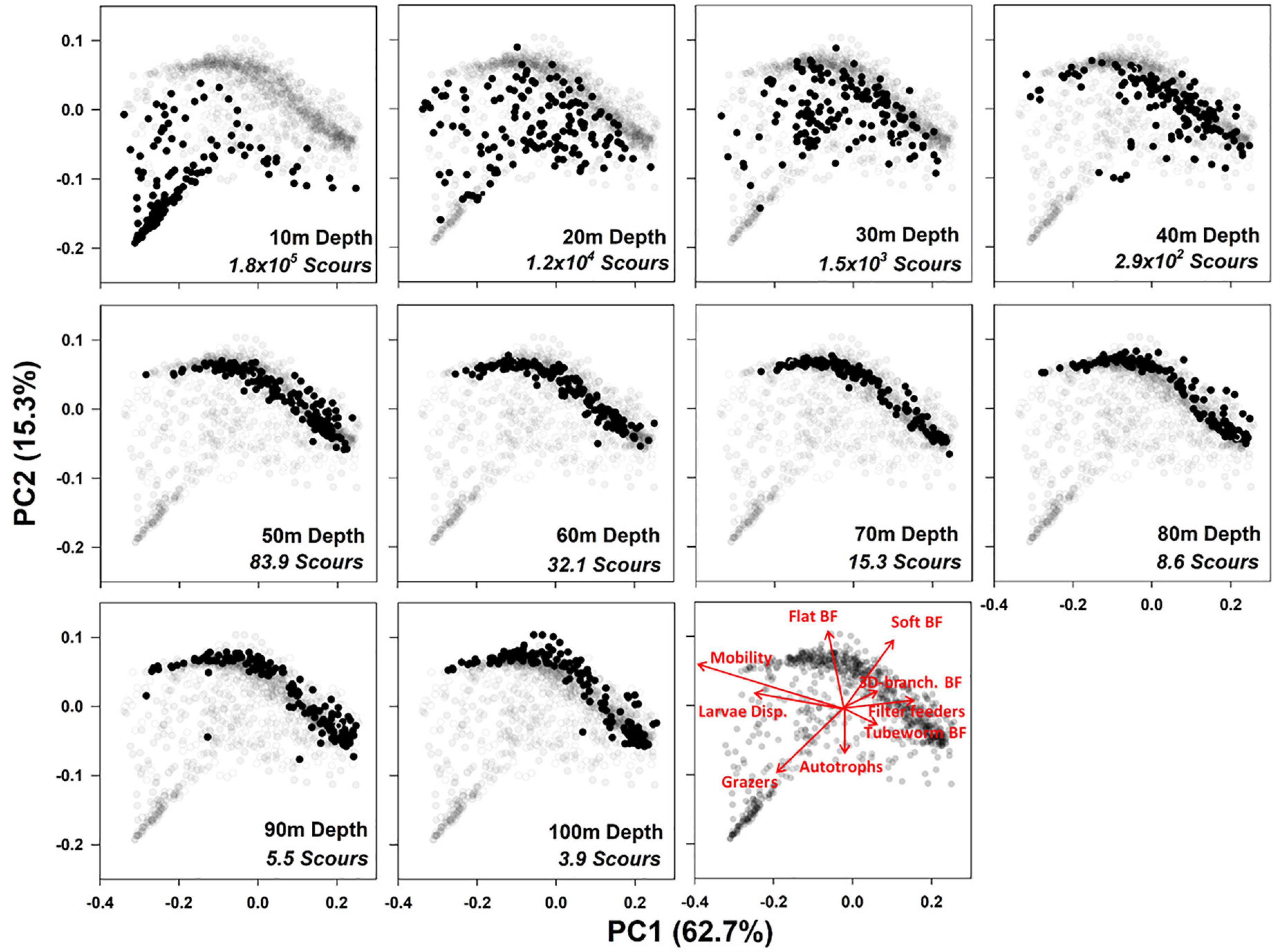
Figure 3. Principal Coordinate Analysis of Community Weighted Means of all Functional Traits with selected set of vectors displayed. Each black circle is a sample at the designated depth. Greyed out samples are from all depths. Principle coordinate 1 and 2 axis explain variation 62.7% and 15.3%, respectively. Vectors are in red, BF: Body Form. Scour: Ice scour per square kilometers depth from Ryder bay (Bowler et al., 2019). ANOSIM test results after 999 permutations, R = 0.216, p = 0.01.
Functional Diversity Along Ice Scour Disturbance Gradient
In FRic, the volume of multidimensional functional space occupied by all OTUs in the assemblage changed significantly with depth [correlation: r2 = 40.1, F(1479) = 330.39, p < 0.001, Figure 4B]. OTU richness also varied across depth with 5.77 OTU richness at 10 m depth, peaking at 60 m depth with an OTU richness of 22.04 at decreasing to 14.77 OTU richness at 100 m depth [for full details see Robinson et al. (2021)]. Changes in FRic comprised a sharp increase with depth, peaking at 50 m depth, which was followed by a slight decrease. This pattern strongly correlated with species richness [correlation: r2 = 70.64, F(1479) = 96.93, p < 0.001, Figure 4A]. Although SES FRic was slightly lower than the null model [1-sample Z test: Z(1479) = −9.90, p < 0.001, Supplementary Figure 5A] this is likely to be an artefact of the model assigning OTUs randomly with no bias toward rarer, likely more functionally unique, OTUs (de Bello et al., 2021). FEve is a measure of the regularity in abundance of OTUs across functional space for a given assemblage. Across 10-80 m depths the FEve analysis showed a significant decrease in Functional Evenness, followed by an increase between 80 and 100 m [correlation: r2 = 48.72, F(1479) = 468.39, p < 0.001, Figure 4D]. With SES FEve we found that traits had a greater degree of regularity across functional space at depths shallower than 50 m depth than would have been expected given the OTUs compositions [1-sample Z test: Z(1483) = 11.83, p < 0.001, Supplementary Figure 5C]. FDiv, the proportion of total abundance supported by species with the most extreme values within an assemblage, showed a significant increase with depth [correlation: r2 = 14.48, F(1479) = 26.62, p < 0.001, Figure 4C]. However, SES FDiv demonstrated values within what would be expected for the measured OTU richness and abundance [1-sample Z test: Z(1483) = −0.26, p = 0.791, Supplementary Figure 5B]. With FRed, a measure of the saturation in functionally similar OTUs in an assemblage (de Bello et al., 2007), we found a unimodal pattern across depth peaking at 60–80 m [correlation: r2 = 15.23, F(1479) = 23.85, p < 0.001, Figure 4E]. Standardized Effect Size FRed recorded a significant under-redundancy with values significantly lower than expected for the given the OTUs composition, except between 50 and 80 m depth [1-sample Z test: Z(1494) = −5.20, p < 0.001, Supplementary Figure 5D].
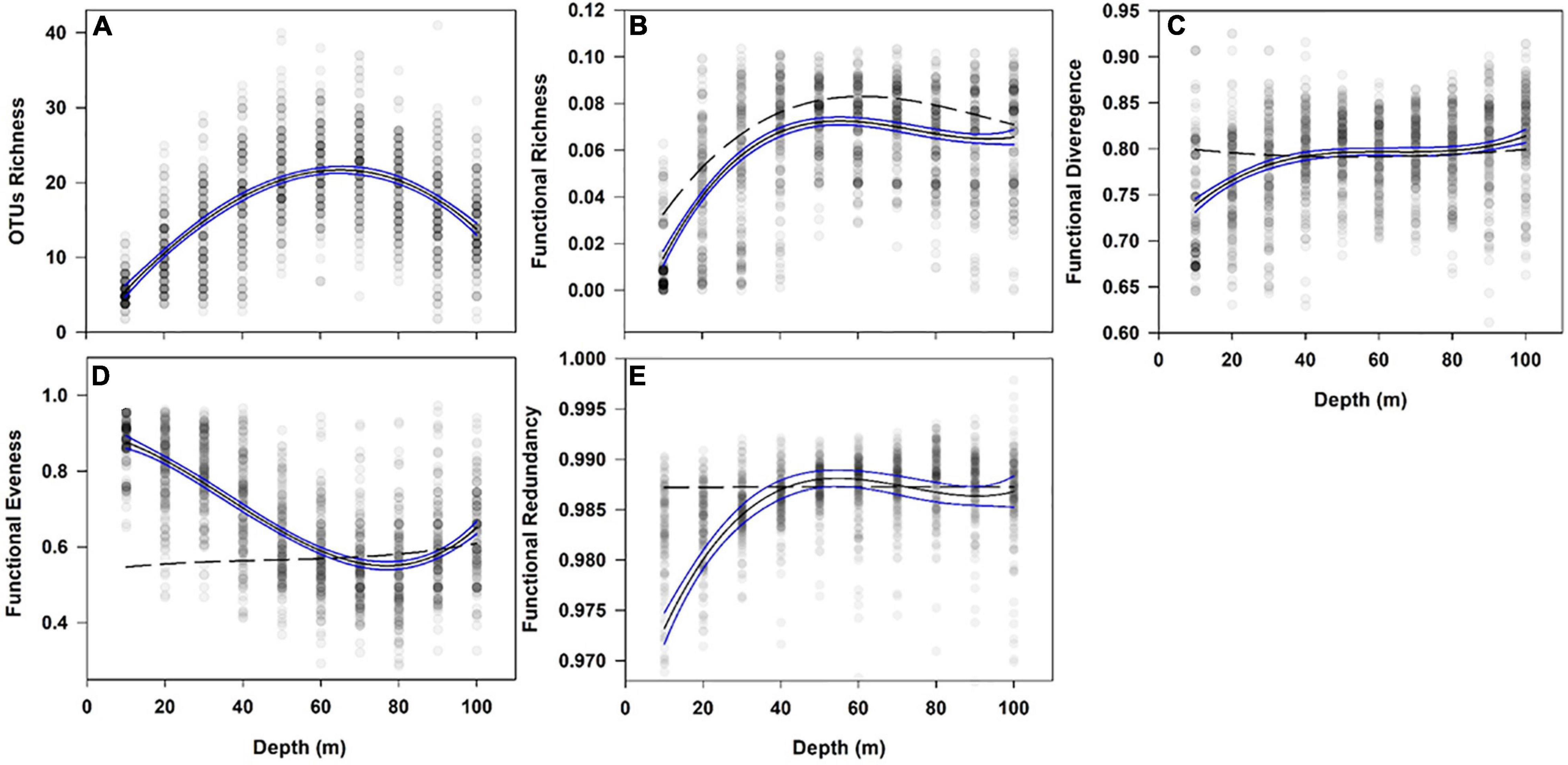
Figure 4. Relationship of Operational Taxonomic Units (OTUs) diversity and Functional Diversity across 10–100°m depth. Line (model) of best first is non-linear regression (cubic model, black line). Points are samples with increasing shades of grey representing a greater number of samples. Blue lines are 95% confidence intervals. Black dotted line are null functional diversity estimates. Total sample number minimum 1484 per panel. (A) – OTUs Richness, (B) – Functional Richness, (C) – Functional Divergence, (D) – Functional Evenness and (E) – Functional Redundancy.
Discussion
The shallow marine benthos around Antarctica has high levels of biodiversity and endemism (Chown et al., 2015), however, the selective mechanisms driving distribution are still poorly understood. To address this, data-sparse species (De Broyer et al., 2014) can be placed into broader taxonomic or functional groups (Jansen et al., 2018). The current study used functional traits to define an OTUs ecological role, morphology and life history, which are all believed to be related to performance and therefore likely related to abundance and presence within the environment (Webb et al., 2010). Adult mobility and larval type traits in particular have been directly linked to improved resilience of species subject to ice scour disturbance and providing advantage in high disturbance environments (Robinson et al., 2020). This approach potentially provides a greater mechanistic understanding of assemblage response to change, particularly in distinguishing between selective and neutral processes (Mouillot et al., 2013). There is growing evidence that competitive interaction and species filtering by disturbance (both selective processes), are at least partially driven, or at least influenced by functional traits (Venn et al., 2011). These selective processes resulted in a significant reduction in functional redundancy at the extremes of the disturbance gradient, identifying areas of vulnerability to future environmental change and highlighted the potential loss of important negative feedback loops on climate change in this environment.
We found these depths (50–80 m) were coincident with a peak in functional diversity, which could be mainly explained by change in OTUs richness and composition alone, in line with the hypothesis of this study. The Intermediate Disturbance Hypothesis maintains a peak in species diversity at intermediate levels of disturbance (Connell, 1978), in this instance because of two selective forces, ice scour disturbance and inter-species competition negating each other. As there is an under-redundancy (redundancy significantly below that expected for a given assemblage structure) at either end of the depth gradient, we consider this strong evidence that the distribution of OTUs are not random and given the large degree of eurybathy seen amongst the Antarctic benthic macrofauna (Brey et al., 1996; Lowe, 2002), specific functional traits or groups are likely being reduced in relative abundance at either end of the depth gradient. Previous work on polar functional structure has found a high degree of taxonomic surrogacy in functional groups (Barnes and Sands, 2017). This was reflected in our null models with the majority of OTUs having some degree of functional overlap.
Above 50 m depth, the benthos is heavily disturbed by frequent ice scouring causing a high rate of mortality amongst all macro and megafauna (Convey et al., 2014), which has resulted in a change in functional structure. Due to the strongly stochastic nature of iceberg scour (Convey et al., 2014; Fraser et al., 2020), abundance of the assemblage can be highly variable (Peck et al., 1999; Barnes, 2017), this study focuses on relative abundance on compositional change for this reasons to consider the longer-term change caused by a disturbance regime. We attribute this observation to ice scour disturbance filtering out selected traits, which are not advantageous for persisting in a high disturbance environment, such as sessile life stages and low dispersal capabilities (Robinson et al., 2020). Extremely low functional redundancy was found above 50 m, suggesting that high disturbance, increases environment’s vulnerability to future change but not necessarily to the disturbance itself, as resistance or in this case, resilient species, persist within the environment (Hughes et al., 2007).
Between 80 and 100 m depth, suspension feeders such as bryozoans and sponges with a wide variety of body forms were dominant. The significant decrease in functional redundancy could be attributed to inter-species competition removing species that occupy similar niches (Cadotte, 2007). Across these depths there is also a drop in OTU richness, with shift to fewer OTU present, but even accounting for this, the decrease in functional redundancy was still significant. However, if correct, such a trend needs to be interpreted with some caution. A limitation of our results is that this reduction was only detected between 80 and 100 m depth, a 20 m depth zone. Extrapolating deeper is without data support, and is likely a complex system, as competitive species exclusion will likely not result in the same trends seen for ice scour disturbance. Loss in functional redundancy can be somewhat attributed to the gaining of new body forms in sessile suspension feeders, with fewer representatives (Robinson et al., 2021). These taxa could act as ecosystem engineers facilitating utilisation (Gili and Coma, 1998; Gili et al., 2001) or colonisation of space by other species (McClintock et al., 2005). The loss of such groups could lead to a cascade effect reducing all major taxa (Mitchell et al., 2020).
The increase in inter-species competition at the extreme low disturbance within the gradient could reduce the number of functionally similar species or those that occupy a similar ecological niche, reducing functional redundancy even across relatively high diversity (Mouillot et al., 2013). This trade-off between disturbance resilience or resistance at high disturbance and inter-species competition at low disturbance, are mechanisms that have long been used to explain community structure (Cadotte, 2007). This study highlights the potential vulnerability of environments on either extreme end of a depth gradient through functional redundancy and loss of the insurance effect, which is coincident with a disturbance gradient. At intermediate levels of disturbance (found around 50–60 m depth), functional redundancy was within the range expected due to assemblage structure change, suggesting disturbance is just frequent enough to reduce interspecies competition without becoming a strong selective pressure itself, but is likely acting as a neutral (random) mechanism. Therefore, disturbance, at any level, does not provide additional insurance or redundancy against species loss, nor against environmental change [other than the disturbance itself (Hughes et al., 2007)] and at the extremes this may leave the environment vulnerable. However, this explanation does not consider intra-species trait variability, in particular sponges with flexible morphologies (Danis, 2013), in response to changes in current (velocity and direction) and space (as a limiting resource). A constraint of the current study is the use of broad functional traits, which is an oversimplification of the food and substrate utilised by suspension feeding organisms (Morley et al., 2016). As a result, functional redundancy may be lower at depth than previously estimated in this study, due to the use of broader functional traits, potentially leading to an overestimation of functional redundancy.
The WAP is predicted to undergo further large environmental changes in temperature (Meredith et al., 2017), ice cover (Parkinson, 2014), and phytoplankton blooms (Rogers et al., 2020), mainly due to climate change (Meredith et al., 2017). Because benthic growth increases with marine ice loss (Fillinger et al., 2013; Barnes, 2015) and possibly moderate temperature increase (Ashton et al., 2017), increasing carbon fixation and sequestration acts as a negative feedback on climate change (Peck et al., 2010; Barnes, 2018). Increasingly frequent iceberg scouring between (0 and 50 m depth) may open space for further benthic growth, which may be important in driving annual carbon accumulation (Barnes and Sands, 2017).Thus, the vulnerability of biota in the shallows is likely to become a critical issue [see, e.g., Morley et al. (2020)]. And, although Antarctic benthos can show high functional group surrogacy (Barnes and Sands, 2017) and food webs that are relatively stable against perturbations (Rodriguez et al., 2021), this study has highlighted that extremes disturbance gradient causes regions of vulnerability. In the future environmental change will likely lead to extirpation of species from certain depth boundary, as multiple environmental factors change, however, the functional structure will likely remain relatively stable particularly between 40 and 80 m depth. However, it is likely that the extreme shallows (above 40 m depth), will see the earliest shifts in functional structure as they face the largest environmental changes and lowest functional redundancy. This study would recommend functional evenness as a useful sentinel metric, to monitor this environment as it is independent of the immediate impacts of ice scour disturbance but will respond to changes in disturbance regime. Current prediction suggest environmental changes will likely lead to increased benthic growth in the future, blue carbon pathways across the continental shelf could potentially become the world’s largest negative (mitigating) feedback on climate change (Barnes et al., 2021). However, areas of low functional redundancy may be vulnerable to future environmental change and ultimately lead to disruption of these blue carbon pathways.
Data Availability Statement
The original contributions presented in the study are included in the article/Supplementary Material, further inquiries can be directed to the corresponding author.
Author Contributions
BR collected data, designed the methodology, and led the writing of the manuscript. DB provided ideas and critically insight into the methodology. LG assisted with conceptualisation and visualisation. SM also assisted with conceptualisation, visualization, and administration. All authors contributed critically to the drafts and final manuscript, and approving the final publication.
Funding
This study was funded by the Natural Environmental Research Council (NERC) core funding to the Biodiversity and Adaptations Team of the British Antarctic Survey, and BR was also funded by the British Antarctic Survey Wharf Modernisation Project via NERC Doctoral Training Program SPITFIRE (NE/L002531/1), through the University of Southampton.
Conflict of Interest
The authors declare that the research was conducted in the absence of any commercial or financial relationships that could be construed as a potential conflict of interest.
Publisher’s Note
All claims expressed in this article are solely those of the authors and do not necessarily represent those of their affiliated organizations, or those of the publisher, the editors and the reviewers. Any product that may be evaluated in this article, or claim that may be made by its manufacturer, is not guaranteed or endorsed by the publisher.
Acknowledgments
We thank summer and wintering teams at Rothera Research Station (2009–2016) for their support. As well as the BAS MAGIC team, Francesco de Bello for his advice on functional diversity analysis and Jan Jansen for providing guidance on functional trait body forms.
Supplementary Material
The Supplementary Material for this article can be found online at: https://www.frontiersin.org/articles/10.3389/fmars.2021.797112/full#supplementary-material
Nomenclature
Resource Identification Initiative
To take part in the Resource Identification Initiative, please use the corresponding catalog number and RRID in your current manuscript. For more information about the project and for steps on how to search for an RRID, please click here.
Life Science Identifiers
Life Science Identifiers (LSIDs) for ZOOBANK registered names or nomenclatural acts should be listed in the manuscript before the keywords with the following format:
urn:lsid: < Authority > : < Namespace > : < ObjectID > [: < Version > ]
For more information on LSIDs please see Inclusion of Zoological Nomenclature section of the guidelines.
References
Aronson, R. B., Thatje, S., Clarke, A., Peck, L. S., Blake, D. B., Wilga, C. D., et al. (2007). Climate change and invasibility of the Antarctic Benthos. Annu. Rev. Ecol. Evol. Syst. 38, 129–154.
Ashton, G. V., Morley, S. A., Barnes, D. K., Clark, M. S., and Peck, L. S. (2017). Warming by 1 C drives species and assemblage level responses in Antarctica’s marine shallows. Curr. Biol. 27, 2698.–2705. 2698-2705. e3, doi: 10.1016/j.cub.2017.07.048
Barnes, D., Sands, C., Paulsen, M., Moreno, B., Moreau, C., Held, C., et al. (2021). Societal importance of Antarctic negative feedbacks on climate change: blue carbon gains from sea ice, ice shelf and glacier losses. Sci. Nat. 108, 1–14.
Barnes, D. K. A. (2015). Antarctic sea ice losses drive gains in benthic carbon drawdown. Curr. Biology 25, R789–R790. doi: 10.1016/j.cub.2015.07.042
Barnes, D. K. A. (2017). Iceberg killing fields limit huge potential for benthic blue carbon in Antarctic shallows. Glob. Chang. Biol. 23, 2649–2659. doi: 10.1111/gcb.13523
Barnes, D. K. A. (2018). Blue Carbon on Polar and Subpolar Seabeds. Carbon Capture, Utilization and Sequestration. London, UK: IntechOpen.
Barnes, D. K. A., and Sands, C. J. (2017). Functional group diversity is key to Southern Ocean benthic carbon pathways. PLoS One 12:e0179735. doi: 10.1371/journal.pone.0179735
Bax, N., Sands, C. J., Gogarty, B., Downey, R. V., Moreau, C. V., Moreno, B., et al. (2020). Perspective: increasing blue Carbon around Antarctica is an ecosystem service of considerable societal and economic value worth protecting. Glob. Change Biol. 27, 5–12. doi: 10.1111/gcb.15392
Bongers, F., Poorter, L., Hawthorne, W. D., and Sheil, D. (2009). The intermediate disturbance hypothesis applies to tropical forests, but disturbance contributes little to tree diversity. Ecol. Lett. 12, 798–805. doi: 10.1111/j.1461-0248.2009.01329.x
Bowden, D. A., Clarke, A., Peck, L. S., and Barnes, D. K. A. (2006). Antarctic sessile marine benthos: colonisation and growth on artificial substrata over three years. Mar. Ecol. Prog. Ser. 316, 1–16.
Bowler, D., Bjorkmann, A., Dornelas, M., Myers-Smith, I., Navarro, L., Niamir, A., et al. (2019). The geography of the Anthropocene differs between the land and the sea. bioRxiv [Preprint]. bioRxiv:432880,
Brey, T., Dahm, C., Gorny, M., Klages, M., Stiller, M., and Arntz, W. (1996). Do Antarctic benthic invertebrates show an extended level of eurybathy? Antarctic Sci. 8, 3–6.
Cadotte, M. W. (2007). Competition–colonization trade-offs and disturbance effects at multiple scales. Ecology 88, 823–829. doi: 10.1890/06-1117
Cardinale, B. J., Duffy, J. E., Gonzalez, A., Hooper, D. U., Perrings, C., Venail, P., et al. (2012). Biodiversity loss and its impact on humanity. Nature 486, 59–67. doi: 10.1038/nature11148
Cavanagh, R. D., Melbourne-Thomas, J., Grant, S. M., Barnes, D. K., Hughes, K. A., Halfter, S., et al. (2021). Future risk for southern ocean ecosystem services under climate change. Front. Mar. Sci. 7: 615214. doi: 10.3389/fmars.2020.615214
Chown, S. L., Clarke, A., Fraser, C. I., Cary, S. C., Moon, K. L., and Mcgeoch, M. A. (2015). The changing form of Antarctic biodiversity. Nature 522, 431–438. doi: 10.1038/nature14505
Clarke, A., Griffiths, H. J., Linse, K., Barnes, D. K. A., and Crame, J. A. (2007). How well do we know the Antarctic marine fauna? A preliminary study of macroecological and biogeographical patterns in Southern Ocean gastropod and bivalve molluscs. Divers. Distribut. 13, 620–632.
Clarke, A., and Johnston, N. M. (2003). “Antarctic marine benthic diversity,” in Oceanography and Marine Biology, An Annual Review, Vol. 41, eds R. N. Gibson and R. J. A. Atkinson (London: Taylor and Francis), 47–114.
Clarke, A., Meredith, M. P., Wallace, M. I., Brandon, M. A., and Thomas, D. N. (2008). Seasonal and interannual variability in temperature, chlorophyll and macronutrients in northern Marguerite Bay, Antarctica. Deep Sea Res. Part II Top. Stud. Oceanogr. 55, 1988–2006. doi: 10.1016/j.dsr2.2008.04.035
Connell, J. H. (1978). Diversity in tropical rain forests and coral reefs. Science 199, 1302–1310. doi: 10.1126/science.199.4335.1302
Convey, P., Chown, S. L., Clarke, A., Barnes, D. K., Bokhorst, S., Cummings, V., et al. (2014). The spatial structure of Antarctic biodiversity. Ecol. Monogr. 84, 203–244.
Craven, D., Isbell, F., Manning, P., Connolly, J., Bruelheide, H., Ebeling, A., et al. (2016). Plant diversity effects on grassland productivity are robust to both nutrient enrichment and drought. Philos. Trans. R. Soc. B Biol. Sci. 371:20150277. doi: 10.1098/rstb.2015.0277
Danis, B. (2013). A Field Guide to Antarctic Sponges. Project Implemented by SCAR-marBIN and ANTABIF. Available online at: http://afg.scarmarbin.be/guides/144164-a-field-guide-to-antarctic-sponges (accessed October 27, 2014)
de Bello, F., Carmona, C. P., Dias, A. T., Götzenberger, L., Moretti, M., and Berg, M. P. (2021). Handbook of Trait-Based Ecology: From Theory to R Tools. Cambridge, MA: Cambridge University Press.
de Bello, F. D., Lepš, J., Lavorel, S., and Moretti, M. (2007). Importance of species abundance for assessment of trait composition: an example based on pollinator communities. Commun. Ecol. 8, 163–170. doi: 10.1556/comec.8.2007.2.3
De Broyer, C., Koubbi, P., Griffiths, H., and Grant, S. A. (2014). Biogeographic Atlas of the Southern Ocean. Cambridge: Scientific Committee on Antarctic Research.
Dell, J., Salcido, D., Lumpkin, W., Richards, L. A., Pokswinski, S., Loudermilk, E. L., et al. (2019). Interaction diversity maintains resiliency in a frequently disturbed ecosystem. Front. Ecol. Evol. 7:145. doi: 10.3389/fevo.2019.00145
Díaz, S., Lavorel, S., De Bello, F., Quétier, F., Grigulis, K., and Robson, T. M. (2007). Incorporating plant functional diversity effects in ecosystem service assessments. Proc. Natl. Acad. Sci. U.S.A. 104, 20684–20689. doi: 10.1073/pnas.0704716104
dos Santos, F. A. S., Johst, K., and Grimm, V. (2011). Neutral communities may lead to decreasing diversity-disturbance relationships: insights from a generic simulation model. Ecol. Lett. 14, 653–660. doi: 10.1111/j.1461-0248.2011.01626.x
Edwards, K. F., Klausmeier, C. A., and Litchman, E. (2011). Evidence for a three-way trade-off between nitrogen and phosphorus competitive abilities and cell size in phytoplankton. Ecology 92, 2085–2095. doi: 10.1890/11-0395.1
Ershova, E., Descoteaux, R., Wangensteen, O., Iken, K., Hopcroft, R., Smoot, C., et al. (2019). Diversity and distribution of meroplanktonic larvae in the Pacific Arctic and connectivity with adult benthic invertebrate communities. Front. Mar. Sci. 6:490. doi: 10.3389/fmars.2019.00490
Fillinger, L., Janussen, D., Lundälv, T., and Richter, C. (2013). Rapid glass sponge expansion after climate-induced Antarctic ice shelf collapse. Curr. Biol. 23, 1330–1334. doi: 10.1016/j.cub.2013.05.051
Fraser, A. D., Massom, R. A., Ohshima, K. I., Willmes, S., Kappes, P. J., Cartwright, J., et al. (2020). High-resolution mapping of circum-Antarctic landfast sea ice distribution, 2000–2018. Earth Syst. Sci. Data 12, 2987–2999. doi: 10.5194/essd-12-2987-2020
Gili, J.-M., and Coma, R. (1998). Benthic suspension feeders: their paramount role in littoral marine food webs. Trends Ecol. Evol. 13, 316–321. doi: 10.1016/s0169-5347(98)01365-2
Gili, J.-M., Coma, R., Orejas, C., López-González, P. J., and Zabala, M. (2001). Are Antarctic suspension-feeding communities different from those elsewhere in the world? Polar Biol. 24, 473–485. doi: 10.1007/s003000100257
Gillies, C., Stark, J., Johnstone, G., and Smith, S. D. (2013). Establishing a food web model for coastal Antarctic benthic communities: a case study from the Vestfold Hills. Mar. Ecol. Prog. Ser. 478, 27–41.
Gillies, C. L., Stark, J. S., Johnstone, G. J., and Smith, S. D. (2012). Carbon flow and trophic structure of an Antarctic coastal benthic community as determined by δ13C and δ15N. Estuar. Coast. Shelf Sci. 97, 44–57. doi: 10.1016/j.ecss.2011.11.003
Gotelli, N. J., and McCabe, D. J. (2002). Species co-occurrence: a meta-analysis of JM Diamond’s assembly rules model. Ecology 83, 2091–2096.
Houseman, G. R., and Gross, K. L. (2011). Linking grassland plant diversity to species pools, sorting and plant traits. J. Ecol. 99, 464–472.
Hughes, A. R., Byrnes, J. E., Kimbro, D. L., and Stachowicz, J. J. (2007). Reciprocal relationships and potential feedbacks between biodiversity and disturbance. Ecol. Lett. 10, 849–864. doi: 10.1111/j.1461-0248.2007.01075.x
Huston, M. A. (2014). Disturbance, productivity, and species diversity: empiricism vs. logic in ecological theory. Ecology 95, 2382–2396.
Isbell, F., Craven, D., Connolly, J., Loreau, M., Schmid, B., Beierkuhnlein, C., et al. (2015). Biodiversity increases the resistance of ecosystem productivity to climate extremes. Nature 526, 574–577. doi: 10.1038/nature15374
Jansen, J., Hill, N. A., Dunstan, P. K., Eléaume, M. P., and Johnson, C. R. (2018). Taxonomic resolution, functional traits, and the influence of species groupings on mapping Antarctic seafloor biodiversity. Front. Ecol. Evol. 6:81. doi: 10.3389/fevo.2018.00081
Laliberté, E., and Legendre, P. (2010). A distance-based framework for measuring functional diversity from multiple traits. Ecology 91, 299–305. doi: 10.1890/08-2244.1
Lee, H., Vanhove, S., Peck, L., and Vincx, M. (2001). Recolonisation of meiofauna after catastrophic iceberg scouring in shallow Antarctic sediments. Polar Biol. 24, 918–925.
Legge, O. J., Bakker, D. C. E., Meredith, M. P., Venables, H. J., Brown, P. J., Jones, E. M., et al. (2017). The seasonal cycle of carbonate system processes in Ryder Bay, West Antarctic Peninsula. Deep Sea Res. Part II Top. Stud. Oceanogr. 139, 167–180.
Lowe, S. (2002). What Relationship Exists Between Antarctic Coastal and Abyssal Benthic Marine Fauna? Ph.D. thesis. Canterbury: University of Canterbury.
Marina, T. I., Salinas, V., Cordone, G., Campana, G., Moreira, E., Deregibus, D., et al. (2018). The food web of Potter Cove (Antarctica): complexity, structure and function. Estuar. Coast. Shelf Sci. 200, 141–151.
McClintock, J. B., Amsler, C. D., Baker, B. J., and Van Soest, R. W. (2005). Ecology of Antarctic marine sponges: an overview. Integr. Comp. Biol. 45, 359–368. doi: 10.1093/icb/45.2.359
Meredith, M. P., Stammerjohn, S. E., Venables, H. J., Ducklow, H. W., Martinson, D. G., Iannuzzi, R. A., et al. (2017). Changing distributions of sea ice melt and meteoric water west of the Antarctic Peninsula. Deep Sea Res. Part II Top. Stud. Oceanogr. 139, 40–57. doi: 10.1016/j.dsr2.2016.04.019
Mitchell, E. G., Whittle, R. J., and Griffiths, H. J. (2020). Benthic ecosystem cascade effects in Antarctica using Bayesian network inference. Commun. Biol. 3, 1–7. doi: 10.1038/s42003-020-01310-8
Morley, S. A., Abele, D., Barnes, D. K., Cárdenas, C. A., Cotté, C., Gutt, J., et al. (2020). Global drivers on Southern Ocean ecosystems: changing physical environments and anthropogenic pressures in an Earth system. Front. Mar. Sci. 7:547188. doi: 10.3389/fmars.2020.547188
Morley, S. A., Berman, J., Barnes, D. K. A., De Juan Carbonell, C., Downey, R. V., and Peck, L. S. (2016). Extreme phenotypic plasticity in metabolic physiology of Antarctic demosponges. Front. Ecol. Evol. 3:157. doi: 10.3389/fevo.2015.00157
Mouillot, D., Graham, N. A., Villeger, S., Mason, N. W., and Bellwood, D. R. (2013). A functional approach reveals community responses to disturbances. Trends Ecol. Evol. 28, 167–177. doi: 10.1016/j.tree.2012.10.004
Mouillot, D., Villeger, S., Parravicini, V., Kulbicki, M., Arias-Gonzalez, J. E., Bender, M., et al. (2014). Functional over-redundancy and high functional vulnerability in global fish faunas on tropical reefs. Proc. Natl. Acad. Sci. U.S.A. 111, 13757–13762. doi: 10.1073/pnas.1317625111
Norkko, A., Thrush, S., Cummings, V., Gibbs, M., Andrew, N., Norkko, J., et al. (2007). Trophic structure of coastal Antarctic food webs associated with changes in sea ice and food supply. Ecology 88, 2810–2820. doi: 10.1890/06-1396.1
Obermüller, B. E., Morley, S. A., Barnes, D. K. A., and Peck, L. S. (2010). Seasonal physiology and ecology of Antarctic marine benthic predators and scavengers. Mar. Ecol. Prog. Ser. 415, 109–126.
Parkinson, C. L. (2014). Global sea ice coverage from satellite data: annual cycle and 35-Yr trends. J. Clim. 27, 9377–9382. doi: 10.1175/jcli-d-14-00605.1
Peck, L. S., Barnes, D. K., Cook, A. J., Fleming, A. H., and Clarke, A. (2010). Negative feedback in the cold: ice retreat produces new carbon sinks in Antarctica. Glob. Change Biol. 16, 2614–2623. doi: 10.1111/j.1365-2486.2009.02071.x
Peck, L. S., Barnes, D. K., and Willmott, J. (2005). Responses to extreme seasonality in food supply: diet plasticity in Antarctic brachiopods. Mar. Biol. 147, 453–463. doi: 10.1007/s00227-005-1591-z
Peck, L. S., Brockington, S., Vanhove, S., and Beghyn, M. (1999). Community recovery following catastrophic iceberg impacts in a soft-sediment shallow-water site at Signy Island, Antarctica. Mar. Ecol. Prog. Ser. 186, 1–8. doi: 10.3354/meps186001
Pfisterer, A. B., and Schmid, B. (2002). Diversity-dependent production can decrease the stability of ecosystem functioning. Nature 416, 84–86. doi: 10.1038/416084a
Puccinelli, E., Smart, S. M., and Fawcett, S. E. (2020). Temporal variability in the trophic composition of benthic invertebrates in the Indian Sub-Antarctic Ocean. Deep Sea Res. Part I Oceanogr. Res. Papers 163:103340. doi: 10.1016/j.dsr.2020.103340
Ricotta, C., De Bello, F., Moretti, M., Caccianiga, M., Cerabolini, B. E., and Pavoine, S. (2016). Measuring the functional redundancy of biological communities: a quantitative guide. Methods Ecol. Evol. 7, 1386–1395. doi: 10.1111/2041-210x.12604
Robinson, B. J., Barnes, D., Grange, L., and Morley, S. (2021). Intermediate ice scour disturbance is key to maintaining a peak in biodiversity within the shallows of the Western Antarctic Penisula. Sci. Rep. 11:16712. doi: 10.1038/s41598-021-96269-9
Robinson, B. J., Barnes, D. K., and Morley, S. A. (2020). Disturbance, dispersal and marine assemblage structure: a case study from the nearshore Southern Ocean. Mar. Environ. Res. 160:105025. doi: 10.1016/j.marenvres.2020.105025
Rodriguez, I. D., Marina, T. I., Schloss, I., and Saravia, L. A. (2021). Marine food webs are more complex but less stable in sub-Antarctic than in Antarctic regions. bioRxiv [Preprint]. doi: 10.1101/2021.04.12.439560
Rogers, A., Frinault, B., Barnes, D., Bindoff, N., Downie, R., Ducklow, H., et al. (2020). Antarctic futures: an assessment of climate-driven changes in ecosystem structure, function, and service provisioning in the Southern Ocean. Annu. Rev. Mar. Sci. 12, 87–120. doi: 10.1146/annurev-marine-010419-011028
Rossi, L., Caputi, S. S., Calizza, E., Careddu, G., Oliverio, M., Schiaparelli, S., et al. (2019). Antarctic food web architecture under varying dynamics of sea ice cover. Sci. Rep. 9, 1–13. doi: 10.1038/s41598-019-48245-7
Smale, D. A., Brown, K. M., Barnes, D. K., Fraser, K. P., and Clarke, A. (2008). Ice scour disturbance in Antarctic waters. Science 321:371. doi: 10.1126/science.1158647
Sporta Caputi, S., Careddu, G., Calizza, E., Fiorentino, F., Maccapan, D., Rossi, L., et al. (2020). Seasonal food web dynamics in the Antarctic benthos of Tethys Bay (Ross Sea): implications for biodiversity persistence under different seasonal sea-ice coverage. Front. Mar. Sci. 7: 594454. doi: 10.3389/fmars.2020.594454
Stanwell-Smith, D., Peck, L. S., Clarke, A., Murray, A. W., and Todd, C. D. (1999). The distribution, abundance and seasonality of pelagic marine invertebrate larvae in the maritime Antarctic. Philos. Trans. R. Soc. Lond. Ser. B Biol. Sci. 354, 471–484. doi: 10.1098/rstb.1999.0398
Svensson, J. R., Lindegarth, M., Jonsson, P. R., and Pavia, H. (2012). Disturbance–diversity models: what do they really predict and how are they tested? Proc. R.Soc. B Biol. Sci. 279, 2163–2170. doi: 10.1098/rspb.2011.2620
Teixidó, N., Garrabou, J., Gutt, J., and Arntz, W. (2007). Iceberg disturbance and successional spatial patterns: the case of the shelf Antarctic benthic communities. Ecosystems 10, 143–158. doi: 10.1007/s10021-006-9012-9
Tilman, D., Reich, P. B., and Isbell, F. (2012). Biodiversity impacts ecosystem productivity as much as resources, disturbance, or herbivory. Proc. Natl. Acad. Sci. U.S.A. 109, 10394–10397. doi: 10.1073/pnas.1208240109
Venn, S. E., Green, K., Pickering, C. M., and Morgan, J. W. (2011). Using plant functional traits to explain community composition across a strong environmental filter in Australian alpine snowpatches. Plant Ecol. 212, 1491–1499. doi: 10.1007/s11258-011-9923-1
Villéger, S., Mason, N., and Mouillot, D. (2008). New multidimensional functional diversity indices for a multifaced framework in functional ecology. Ecology 89, 2290–2301. doi: 10.1890/07-1206.1
Villéger, S., Novack-Gottshall, P. M., and Mouillot, D. (2011). The multidimensionality of the niche reveals functional diversity changes in benthic marine biotas across geological time. Ecol. Lett. 14, 561–568. doi: 10.1111/j.1461-0248.2011.01618.x
Violle, C., Navas, M.-L., Vile, D., Kazakou, E., Fortunel, C., Hummel, I., et al. (2007). Let the concept of trait be functional! Oikos 116, 882–892. doi: 10.1111/j.0030-1299.2007.15559.x
Webb, C. T., Hoeting, J. A., Ames, G. M., Pyne, M. I., and Leroy Poff, N. (2010). A structured and dynamic framework to advance traits-based theory and prediction in ecology. Ecol. Lett. 13, 267–283. doi: 10.1111/j.1461-0248.2010.01444.x
Keywords: ecosystem, functional diversity (FD), insurance effect, Antarctica – coastal ecology, iceberg scour, blue carbon and ecosystem services, standard effect size, selective mechanism
Citation: Robinson BJO, Barnes DKA, Grange LJ and Morley SA (2022) The Extremes of Disturbance Reduce Functional Redundancy: Functional Trait Assessment of the Shallow Antarctic Benthos. Front. Mar. Sci. 8:797112. doi: 10.3389/fmars.2021.797112
Received: 22 October 2021; Accepted: 31 December 2021;
Published: 17 February 2022.
Edited by:
Susana Carvalho, King Abdullah University of Science and Technology, Saudi ArabiaReviewed by:
Santiago Pineda Metz, Alfred Wegener Institute Helmholtz Centre for Polar and Marine Research (AWI), GermanyDaniel Pech, El Colegio de la Frontera Sur, Mexico
Copyright © 2022 Robinson, Barnes, Grange and Morley. This is an open-access article distributed under the terms of the Creative Commons Attribution License (CC BY). The use, distribution or reproduction in other forums is permitted, provided the original author(s) and the copyright owner(s) are credited and that the original publication in this journal is cited, in accordance with accepted academic practice. No use, distribution or reproduction is permitted which does not comply with these terms.
*Correspondence: Ben J. O. Robinson, benson@bas.ac.uk