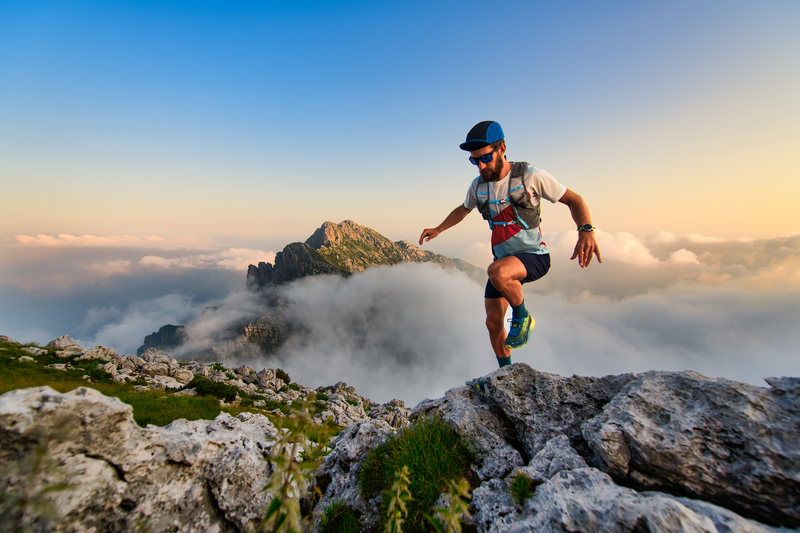
94% of researchers rate our articles as excellent or good
Learn more about the work of our research integrity team to safeguard the quality of each article we publish.
Find out more
ORIGINAL RESEARCH article
Front. Mar. Sci. , 13 January 2022
Sec. Marine Megafauna
Volume 8 - 2021 | https://doi.org/10.3389/fmars.2021.795942
This article is part of the Research Topic Assessment Approaches to Support Bycatch Management for Marine Mammals View all 13 articles
Marine megafauna plays an important functional role in marine ecosystems as top predators but are threatened by a wide range of anthropogenic activities. Bycatch, the incidental capture of non-targeted species in commercial and recreational fisheries, is of particular concern for small cetacean species, such as dolphins and porpoises. In the North-East Atlantic, common dolphin (Delphinus delphis, Linné 1758) bycatch has been increasing and associated with large numbers of animals stranding during winter on the French Atlantic seashore since at least 2017. However, uncertainties around the true magnitude of common dolphin bycatch and the fisheries involved have led to delays in the implementation of mitigation measures. Current data collection on dolphin bycatch in France is with non-dedicated observers deployed on vessels for the purpose of national fisheries sampling programmes. These data cannot be assumed representative of the whole fisheries' bycatch events. This feature makes it difficult to use classic ratio estimators since they require a truly randomised sample of the fishery by dedicated observers. We applied a newly developed approach, regularised multilevel regression with post-stratification, to estimate total bycatch from unrepresentative samples and total fishing effort. The latter is needed for post-stratification and the former is analysed in a Bayesian framework with multilevel regression to regularise and better predict bycatch risk. We estimated the number of bycaught dolphins for each week and 10 International Council for the Exploration of the Sea (ICES) divisions from 2004 to 2020 by estimating jointly bycatch risk, haul duration, and the number of hauls per days at sea (DaS). Bycatch risk in pair trawlers flying the French flag was the highest in winter 2017 and 2019 and was associated with the longest haul durations. ICES divisions 8.a and 8.b (shelf part of the Bay of Biscay) were estimated to have the highest common dolphin bycatch. Our results were consistent with independent estimates of common dolphin bycatch from strandings. Our method show cases how non-representative observer data can nevertheless be analysed to estimate fishing duration, bycatch risk and, ultimately, the number of bycaught dolphins. These weekly-estimates improve upon current knowledge of the nature of common dolphin bycatch and can be used to inform management and policy decisions at a finer spatio-temporal scale than has been possible to date. Our results suggest that limiting haul duration, especially in winter, could serve as an effective mitigation strategy.
Over the last 50 years, the conservation status of cetaceans has been deteriorating (Brownell et al., 2019). Over 80 species of cetaceans occur worldwide and bycatch, the non-intentional capture or killing of non-target species in commercial or recreational fisheries (Hall, 1996; Davies et al., 2009), remains a threat, especially to small-sized species (Scarff, 1977; Read et al., 2006; Avila et al., 2018; Anderson et al., 2020). Success stories in small cetacean conservation are the exception rather than the rule (e.g., Bessesen, 2018). Both Rogan et al. (2021) and Bearzi and Reeves (2021) opined of institutional failures to conserve cetaceans in European Waters in spite of current legislation (for example, the Habitats Directive, the Marine Strategy Framework Directive) or regional agreements such as the Agreement on the Conservation of Small Cetaceans of the Baltic and North Seas (ASCOBANS, see Table 1 for acronyms; ICES, 2020c). Over 20 species of small cetaceans have been registered in the North-East Atlantic, with roughly half of which occurring regularly (Course, 2021). Because of their slow life histories and their limited potential rates of increase, small cetaceans are particularly at risk of decline when anthropogenic activities induce additional mortality on populations (Read, 2008). Anthropogenic activities and their cumulative impacts can take a heavy toll on populations. Common species may disappear, such as short-beaked common dolphins (Delphinus delphis, hereafter called common dolphins) in the Adriatic Sea (Bearzi and Reeves, 2021), or are under many threats, e.g., in the Bay of Biscay (García-Baron et al., 2019; Murphy et al., 2021).
In 2013, the common dolphin's conservation status in the European Marine Atlantic, as assessed under Article 17 of the Habitats Directive, was “Unfavourable–Inadequate” because of fishery bycatch (Murphy et al., 2021). Common dolphin bycatch in the Bay of Biscay, in particular, has attracted a lot of media coverage since 2017 in international outlets1 and motivated (with bycatch of Harbour porpoise Phocoena phocoena in the Baltic Sea) a special request of Non-Governmental Organisations to the European Commission in 2019. The International Council for the Exploration of the Sea (ICES) advised in 2020, for the common dolphin in the Bay of Biscay, a combination of temporal closures of all métiers (i.e the combination of gear, target species, and fishing area) of concern and application of pingers on pair trawlers to mitigate bycatch outside of the period of closure (ICES, 2020b). Temporal closures, restricted to winter months in which strandings of common dolphins with evidence of bycatch have increased in recent years (ICES, 2020d), could have been implemented as emergency measures under the provisions of the Common Fisheries Policy. For 2021, France instead required the mandatory use of acoustic repulsive devices (pingers) on all pair trawlers flying the French Flag (code métier Pair trawlers and hereafter referred to as PTM) operating in the Bay of Biscay2, a technical mitigation measure whose efficiency was found wanting (Ulrich and Doerner, 2021). This decision against the advice of ICES was motivated by a lack of knowledge on common dolphins, including its abundance at the level of the whole North-East Atlantic (the currently recognised management unit: Murphy et al., 2013) and the extent of bycatch. The issue of managing uncomfortable knowledge through interpretation of scientific uncertainty can be raised (Schweder, 2000; Rayner, 2012); yet it should not eclipse that there are genuine difficulties in estimating accurately the true magnitude and the extent of bycatch of small cetaceans (Moore et al., 2021).
Several types of fishing gear are known to cause cetacean bycatch: drift nets, set gill, trammel nets, both pair and single midwater trawls, and some demersal trawls (Rogan and Mackey, 2007; Fernández-Contreras et al., 2010; Peltier et al., 2016). Accurate quantification of bycatch rates by fishing gears or métiers remains a challenging endeavour (Babcock et al., 2003; ICES, 2019). Traditionally, bycatch data are collected by onboard observers monitoring fishing operations and recording the unwanted catch of non-commercial species (Course, 2021). Ratio estimators, based on the number of observed hauls with bycatch over the total number of monitored hauls, are used (Alverson et al., 1994; page 18) but are plagued by large uncertainties due to low coverage and the usual small number of hauls with small cetacean bycatch (Babcock et al., 2003; Authier et al., 2021; Course, 2021). It may also happen that some bycatch events may not be reported by non-dedicated observers since they may drive observations for other purposes than report bycatch (e.g., commercial discards or stock assessments). A critical assumption behind the use of such ratio-estimators is that of a representative sample: this assumption is difficult to sustain unless monitoring is dedicated to marine mammals, and allocation of observers to fishing vessels is truly randomised (that is, not at the discretion of skippers). Even if we are willing to assume representative sampling, if coverage is low, the main challenge remains to extrapolate from sample to the whole fisheries. In France, monitoring of cetacean bycatch in fisheries is non-dedicated (Cornou et al., 2018), and the collected data are described as non-representative of the bycatch events, preventing the use of ratio-estimators (Anonymous, 2016; page 24).
This non-dedicated nature and the sparseness of the bycatch data complicates the use of state-of-the-art spatio-temporal models such as Vector-Autoregressive Spatio-Temporal (VAST) (Thorson, 2019). This framework accommodates density-dependence, spatial and temporal scales to estimate biomass or abundance or presence of a species (Thorson et al., 2015). Spatio-temporal models are also used to model the co-occurrence of commercial and bycaught species, allowing the estimate of bycatch risk with time-varying spatial effects (Ward et al., 2015). These types of model-based approaches methodologies allow modelling spatial and temporal auto-correlation through the use of Gaussian process priors. It is difficult to transfer a priori the same model-based structure to analyse small cetacean bycatch. Models such as VAST capitalise on the availability of catch data that are collected as part of fisheries monitoring. In contrast, bycatch monitoring is not as developed or efficiently enforced in many fisheries in Europe (ICES, 2019, 2020a; Sala et al., 2019), and bycatch data are typical of low quality and unrepresentative (Authier et al., 2021). In Europe, fisheries monitoring is carried out under the Data Collection Framework (DCF) but “remains not well-suited for the dedicated monitoring of rare and protected bycatch in high-risk fisheries since its main focus is the statistically-sound random sampling of all commercial fisheries” (Ulrich and Doerner, 2021). Because of these data quality issues, Authier et al. (2021) conducted a simulation study to gauge the potential of investigating recent methods for the analysis of non-representative samples (for a recent example of a model-based approach to estimate bycatch, refer to Luck et al., 2020) in the context of small cetacean bycatch: they concluded the potential of regularised multilevel regression with post-stratification to infer more accurately bycatch rates (although uncertainties remained large). The approach of Authier et al. (2021) also makes use of Gaussian process priors but does not necessarily assume that a large dataset has been collected.
We analysed historical bycatch monitoring data collected by onboard observers (from 2004 to 2020) on PTM, a métier historically associated with high levels of dolphin bycatch in the Bay of Biscay (ICES, 2019; Murphy et al., 2021). Leveraging recent modelling developments (see companion article; Authier et al., 2021), we jointly estimated bycatch risk, haul duration, and number of hauls per days at sea (DaS) from an updated and revised observer dataset on common dolphin bycatch. The modelling procedure accounts for the sparseness of the bycatch incident dataset and the low observer coverage through constraints. This type of constraint (which can be viewed as some sort of penalisation) is also called regularisation. We used structured priors, such as Gaussian processes, to achieve regularisation and leverage the within-year information at the weekly scale (inducing correlation between some weeks). Structured priors allow inducing some spatial- or temporal-dependency between so called random-effects whereas unstructured priors do not induce such dependency (but both assume exchangeability). Importantly, we used this model-based approach to disaggregate bycatch risk at the level of calendar weeks in order to document within-year variations. Estimates were summed over a whole year to investigate between-year variations in the number of bycaught dolphins. We compared these model-based estimates with strandings, both within- and between-years. Finally, we concluded with recommendations on conservation and mitigation.
The study area (Figure 1) encompasses 10 ICES divisions within area 27: it includes the Bay of Biscay, the English Channel, and part of the Celtic seas. These zones are associated with submesoscale and mesoscale oceanographic processes, such as eddies and upwelling, that enhance ecosystem productivity and result in high availability of fishes, including commercial species (e.g., European seabass Dicentrarchus labrax, Sardine Sardina pilchardus or Anchovy Engraulis encrasicolus). Each division can roughly be classified as oceanic or neritic: divisions 7.d, 7.e, 7.f, 7.g, 7.h, 8.a, 8.b, and 8.c are related to neritic ecosystems while divisions 7.j, and 8.d are related to oceanic ecosystems.
Two main sources of data were used. The first dataset, called ObsMer3 (”Observation des captures en Mer”), is collected as part of an onboard observer program set up within the Data Collection Framework of the Common Fisheries Policy. The ObsMer program is carried out by Ifremer (“Institut Français de Recherche pour l'Exploitation de la Mer”), under the supervision of the Directorate of Fisheries and Aquaculture (“Direction des pêches maritimes et de l'aquaculture,” DPMA). ObsMer observers' primary duty is to register the length and weight composition of catches. Still, they have to report any bycatch event if they witness such events. ObsMer data on PTM cover 4, 484 hauls between 2004 and 2021, of which 82 were associated with a bycatch event of at least 1 and up to 50 common dolphins. ObsMer provides, among other information, the geographic position, timing, and duration of hauls. Although ObsMer is aiming at a coverage of 10 and 5% of fishing effort for (level-3 métier) PTM for vessels of more than 15 m and less than 15 m, respectively, these figures are rarely, if ever, reached in practice: accepting onboard observers remains entirely at the discretion of skippers. The effort is quite low overall, ranging from 0 to 11% of Days at Sea (DaS) (Table 2). A DaS is any continuous period of 24 h (or part thereof) during which a vessel is present within an area and absent from the port (Anonymous, 2019). The number of observed hauls with at least one bycatch record is very small because the yearly percentage of observed hauls with a bycatch event never exceeded 4.5% and was 0 in nearly half of the surveyed years. ObsMer data on pair-trawlers are an unrepresentative sample of hauls, largely because allowing an observer remains largely at the discretion of skippers (Babcock et al., 2003; Benoît and Allard, 2009).
Table 2. Descriptive statistics for Observation des captures en Mer (ObsMer) and SACROIS data displayed for each year.
The second dataset provides monthly estimates of total fishing effort in each division. This dataset is generated from the algorithm SACROIS developed by Ifremer and integrates data from Vessel Monitoring System, log-books, and landing statistics (for boats longer than 18 m from January 1, 2004, and longer than 15 m from January 1, 2005; Système d'Information Halieutique, 2017). SACROIS aims at (1) correcting errors that could exists in the integrated dataset due to recording or collecting errors and (2) reconstitute métiers during the fishing trip as they are not recorded in logbooks or fish market data (Cornou et al., 2018). The SACROIS dataset provides the best available estimates of total effort, in DaS, between 2004 and 2020 (Table 2). There are also refusals from skippers due to administrative and security reasons. Skippers must file an application for authorisation to embark observers and even if they decide to file, the authorisation may be declined due to security reasons (e.g., not enough room or rails not high enough).
These two datasets are complementary for our purposes: ObsMer provides micro-level data on marine mammal bycatch at the resolution of hauls. From these data, bycatch risk may be estimated (Luck et al., 2020). Fishing trips effort data, on the other hand, are macro-level: they provide spatialised effort data at the scale of a whole fishing fleet. These population-level data on effort allows the post-stratification of bycatch risk estimated from observer data to obtain the number of bycaught dolphins (Authier et al., 2021). Descriptive statistics of both datasets are displayed in Table 2. Used in tandem, both datasets allow using regularised multilevel regression with post-stratification to estimate cetacean bycatch from non-representative samples (Authier et al., 2021).
Estimates were finally compared to strandings along the French Atlantic seaboard. The French Stranding Network, founded in the 1970s, is dedicated to the monitoring of marine mammal strandings along the shores of France (mainland and overseas). Around 400 trained volunteers are currently taking an active part in the network. These volunteers make the complete coverage of French coastlines possible. Standardised training of volunteers by permanent Observatoire Pelagis staff, which takes place two times a year, ensure the homogeneity, comparability, and standardisation of data collection procedures in the field. Observatoire Pelagis is mandated by the French Ministry of Ecology to train and deliver authorisation to handle carcasses of marine mammals (which are all protected species under national law). It also collates the data and analyse it to inform on the status of marine mammal populations. Stranding data for the period 2004–2020 were used. Only common dolphins found with lesions diagnostic of bycatch in fishing gear were considered (Kuiken, 1994) as well as those stranded during multiple stranding events, or “unusual mortality events” related to lesions diagnostic of bycatch. Multiple stranding events were defined as high numbers of strandings occurring in a restricted area with a common cause of death. The threshold was defined at 30 cetaceans over 10 consecutive days recorded along a maximal distance of 200 km in the Bay of Biscay, and 10 individuals per 10 days per 200 km of coastline along the coast of the western Channel (Peltier et al., 2014). Reverse drift modelling uses a deterministic drift model developed by Meteo France (Peltier et al., 2012) to reconstruct the trajectory of every stranded common dolphin from its stranding location to its likely area of death at sea. The number of dead stranded animals in each cell is then corrected by the cell-specific probability of being stranded (Peltier et al., 2016). These probabilities were estimated by numerical experiment in which the drift of carcasses in the study area was simulated in order to assess with which frequency they would reach a coastline (Peltier and Ridoux, 2015).
Observation des captures en Mer data allow both bycatch risk and haul duration to be modelled. The two may be correlated as a longer towing time may result in an increased likelihood of bycatch, all else being equal. Bycatch risk is defined at the level of a haul. Hauls can differ in duration as skippers may target different commercial species at different times of the year. However, the population-level data on effort is aggregated and available as DaS, the metric currently used in international fora (e.g., ICES Working Group on BYCatch, WGBYC). The number of hauls per DaS was also modelled from the ObsMer dataset in order to scale up bycatch risk per haul by the number of hauls per DaS. We modelled jointly bycatch risk, fishing duration of hauls, and the number of hauls per DaS of pair-trawlers flying the French flag at the week-level for each year between 2004 and 2020 (Table 2) and each ICES division (Figure 1). The goal of the approach is to model bycatch rates at the weekly scale for each year within each ICES division using a simple autoregressive model. To smooth the fluctuations of estimated bycatch rates in weekly estimates we constrained estimation using Gaussian Process structured priors. These priors allow (i) to estimate an average bycatch risk profile at the weekly scale and from this weekly average, (ii) to estimate year- and division-level deviations.
Let denote a normal distribution of location parameter d and scale parameter s. Let denote a gamma distribution of scale parameter a and rate parameter b. Let denote a log-normal distribution of location parameter d and scale parameter s. The gamma and the log-normal distribution are used and compared to model the likelihood of the haul duration since they assume a positive continuous distribution. These distribution laws are appropriate modelling choices for positively skewed data with a constant coefficient of variation. Let denote a Gaussian process of mean function m and covariance function c. A Gaussian Process is a prior distribution on a function f in which, for any vector x = (x1, …, xn), f(x) is drawn from a n−dimensional normal distribution with mean m(x) and covariance matrix depending only on the distances of the point x from each other (Gelman et al., 2021, page 465). In the following, we will drop the x and write in a shorthand manner to mean that the vector θ of n parameters has a Gaussian process prior and follows a multivariate normal distribution whose mean vector m is equal to m(x) and whose covariance matrix S is defined for any pairs (x, x′) as S(x, x′) = c(x, x′), where c is the covariance function of the Gaussian process prior.
Let i denotes the ith haul (fishing operation) happening in ICES statistical division j in week t of year k. Let yijkt, dijkt, and njkt denote, respectively, bycatch event (0 or 1), fishing duration (in hours, dijkt > 0), and the number of hauls per DaSjkt. Bycatch risk pjkt is estimated from
To account for strict positivity, fishing duration is modelled either with a Gamma or a log-normal likelihood:
The number of hauls per DaS is modelled assuming a zero-truncated Poisson likelihood:
Parameters and are rates. The linear predictors αjk are vectors of week-level parameters related to ICES division j and year k (dropping the superscript for convenience):
Parameter μ is the intercept. The vector ϵ aggregates the mean weekly effects (on the linear predictor scale) which are modelled with a first-order random walk to ensure some smoothness in between-week variations (Authier et al., 2021). The vector δk are year-specific deviations from the mean weekly pattern ϵ. The vector αjk are division-specific deviations from the mean yearly pattern δk. Smoothness in αjk and δk is controlled via the covariance matrices Σdivision = ΔdivisionΩΔdivision and Σyear = ΔyearΩΔyear.Matrices Σ. have dimensions nweek × nweek (53 × 53). These covariance matrices are decomposed into a product of a diagonal matrix Δ. (of dimension 53 × 53) with the common scale parameter on the diagonal, and a correlation matrix Ω (of dimension 53 × 53; Chen and Dunson, 2003):
Ω = Ω(t, t′) is a matrix with the correlation between week t and week t′ of dimensions nweek × nweek (53 × 53). A Matérn correlation function of order and range parameter fixed to was assumed: where d(t−t′) = |t − t′| is the temporal distance (in weeks) between weeks t and t′. The choice of the range parameter induces a temporal correlation of 0.05 after 4 weeks (that is, temporal independence after a month; Authier et al., 2021). The correlation matrix Ω is assumed known and is depicted in Figure 2. Equations 6 and 7 allow modelling an interaction between week, year, and division. The joint model defined in Equations (1), (2a), and (3) includes a time-varying component at the week-scale with interaction with year and division.
Simpler models without such interactions, and with only additive effects, were also fitted to the data. The simplest model included only additive random (unstructured) effects (dropping the superscript for convenience):
Models are multilevel, accommodating week-, year-, and division-level variations. They also use structured priors such as Gaussian processes or random walks to regularise estimation (Gao et al., 2019). More information on these models, and on applying (regularised) multilevel regression with post-stratification in the context of estimating bycatch, are detailed by Authier et al. (2021). Estimation was carried out in a Bayesian framework using programming language Stan (Carpenter et al., 2017) called from R v.4.0.1 (R Core Team, 2020) with library Rstan (Stan Development Team, 2020). Stan uses Hamiltonian dynamics in Markov chain Monte Carlo (MCMC) to sample values from the joint posterior distribution (Carpenter et al., 2017). Four chains were initialised from diffuse random starting points and run for a total of 2, 000 iterations, discarding the first 1, 000 as a warm-up. Default settings for the No-U-Turn Sampler (NUTS) were changed to 0.99 for adapt delta and 15 for max treedepth (Hoffman and Gelman, 2014). Priors are reported in Table 3. We fitted a total of 6 models of differing complexity (Table 4): we compared models assuming either gamma or a log-normal likelihood for haul duration, and models assuming additive effects vs. interactive effects of the week, year, and divisions. Model fitting was carried out on the supercomputer facilities of the “Mésocentre de calcul de Poitou Charentes (Université de Poitiers/ISAE-ENSMA/La Rochelle Université).” Codes are available at https://gitlab.univ-lr.fr/mauthier/cdptmbycatch. For confidentiality reasons, the actual dataset cannot be shared: a synthetic dataset, generated by predicting from the posterior distribution, is provided instead.
The number of unobserved hauls Njkt that happened in ICES statistical division j in week t of year k can be estimated from the number of observed DaS in ObsMer () and from total effort (and accounting for zero-truncation):
The total number of bycatch events in ICES statistical division j in week t of year k is estimated as the sum of events observed in ObsMer () and the number of unobserved hauls multiplied by bycatch risk ():
Similarly, for each year, the number of common dolphins bycaught in pair-trawlers can be estimated using the observed number of bycaught dolphins in ObsMer, the estimated number of unobserved hauls (Equation 7), bycatch risk, and either the median number of dolphins involved in a bycatch event (Table 2, or the grand median of m = 2 for years with no observed bycatch event). We used the median to attenuate the influence of some bycatch events involving up to 50 dolphins (Table 2). These estimates are thereafter referred to as model-based estimates.
The sample provided by ObsMer, a non-dedicated observer scheme of marine mammal bycatch, may not be representative of all bycatch. In addition, it provides very sparse data, with less than 100 observed events over 17 years (Table 4) when strandings have reached several hundred per week in recent years (ICES, 2020d) (for all causes of death). Despite this, the weekly pattern of bycatch risk provided by ObsMer roughly matches that of strandings, with an increase in winter (Figure 3). Despite this rough match, the ObsMer data also suggest a heightened risk in summer, especially in the 2000s, whereas strandings suggest such an increased risk in very recent years (Peltier et al., 2021).
Figure 3. Model-based estimates (posterior medians) of bycatch risk, haul duration, and number of hauls per Days at Sea (DaS) of pair-trawlers (PTM) flying the French flag operating in the study area. Each colour represents a different year (parameters αjkt in Equation 4) and the dotted black line the yearly average (parameters ϵt in Equation 4).
The number of stranded common dolphins with evidence of bycatch can be used to estimate the total bycatch mortality with reverse drift modelling (Peltier et al., 2016). These stranding-based estimates are now used in international working groups (ICES, 2020d). Reverse drift modelling corrects for at-sea drifting conditions, but cannot inform on which fishing gears were responsible for bycatch. Hence, strandings-based estimates are total estimates of bycatch and can be compared to model and observation based estimates of bycatch by French pair-trawlers. These model-based estimates use data independent from strandings, but they should not exceed stranding-based estimates. Second, whether model-estimates correlate with strandings-based ones is of interest to shed light on the increased mortality witnessed in the Bay of Biscay (Peltier et al., 2021). For each year, we checked the magnitude of model-based estimates against stranding-based ones and computed Pearson's correlation coefficient between the two time-series at the month level. To account for drift, these correlations were computed with and without a lag of 2 weeks when aggregating model-based estimates at the month level.
We built and compared six models (Table 4). Convergence was reached for all parameters with all . Model had the lowest WAIC and was selected as the best model for further inferences. Model included an interaction between week, year, and ICES division (Equations 6 and 7). All codes to fit models are available at https://gitlab.univ-lr.fr/mauthier/cdptmbycatch.
Haul duration, hauls per DaS, and bycatch risk per haul (Equations 7 and 8) were jointly estimated. Their temporal variations are displayed in Figure 3 for each week between 2004 and 2020. Haul duration was the highest in week 1 with a posterior median estimate of 5.8 h that decreased to 4.0 h in week 16, before dropping to 2 h in week 24. Haul duration increased up to 3 h in week 32 and plateaued until the end of the year. Remarkable years were 2017, 2019, and 2020 with the longest haul durations estimated from week 1 to 10. From week 10 onwards, years before 2012 displayed some variations in haul duration. In particular, duration was consistently smaller in 2004. In 2016, an increase in haul duration was estimated in week 48 (5 vs. 3 h on average across years).
Bycatch risk was maximum in week 1 (around 0.1) and decreased to almost 0 from week 8 onwards. 2017, 2019, and 2020 were the years with the highest estimated bycatch risk in the first 8 weeks. In particular, the risk was as high as 0.20 in 2017 for the first four consecutive years of the year. Two years prior to 2012 were associated with an increased risk between weeks 30 and 36. The year 2016 showed a rise in bycatch risk in week 48. Bycatch risk and haul duration were positively correlated with weeks in years associated with the highest risk and also having the longest haul duration. Numbers of hauls per DaS were negatively correlated with weeks with longer haul duration. There was little variation across years in numbers of hauls per DaS, but substantial within year variations.
Spatial variations in bycatch risk and haul duration are available as supplementary information. There were noteworthy differences between divisions regarding bycatch risk (see supplementary information). The overall signal was similar to the one observed in Figure 3 with the highest risk values estimated between weeks 1 and 8. Risk in 2017 and 2019 was higher by a factor of 5 in week 1 compared to other years. After week 8, this difference disappeared. With respect to divisions, division 8.a. was the one with the highest bycatch risk, with an estimate as high as 0.50 in winter 2017 and 2019.
The estimated total number of bycaught dolphins for each year is reported in Table 5. The study area was further divided into three strata: a neretic stratum in ICES subarea 7 (divisions 7.defgh) and another in subarea 8 (divisions 8.abc); and an oceanic stratum spanning subareas 7 and 8 (divisions 7.j and 8.d). Estimates were the lowest in the oceanic stratum of the study area and the largest in the neretic stratum spanning ICES subarea 8. The largest bycatch estimate was in 2017, with a posterior median of >600 common dolphins bycaught in PTM operating in the neretic stratum spanning ICES subarea 8. There were large between-year variations in estimates, ranging from less than a hundred (in 2018) to more than one thousand (in 2017). Uncertainties around model-based estimates were also large.
Strandings data were used to estimate common dolphins mortality due to fisheries following method described in Peltier et al. (2016) for each month from 1990 to 2020. Stranding-based estimates aggregate mortality due to all fisheries and do not distinguish between gears or métiers. Nevertheless, we correlated stranding-based estimates with our model-based estimates of mortality from PTM flying the French flag both between years (Figure 4) and within each year (Figure 4). For yearly estimates, correlations were computed on raw and standardised (mean centered and unit variance) values (Figure 4). Model-based estimates of bycatch by PTM were always below stranding-based estimates (which do not allow to disaggregate by métiers) save for 2010 (Figure 4). In 2010, model-based and stranding based estimates were 465 and 343, respectively, with a large overlap in credibility interval. At the year level, the Pearson correlation between stranding-based and model-based estimates was 0.25. Yearly variations between the two time series were more in phase from 2015 onwards (Figure 4). At the within year (between month) level, correlations between the two time-series were always positive. These within year correlations generally increased by 47% (median) when model-based estimates were aggregated by month with a lag of 2 weeks to account for drift (Figure 4). The temporal trend in within year correlation was negative over the study period.
Figure 4. Comparison of model-based (orange solid line) and stranding-based estimates (dark violet solid line) of common dolphin bycatch. Upper: Raw estimates with uncertainty intervals (80% for model-based estimates and 95% for stranding-based estimates); Middle: standardised (mean centred and unit variance) estimates. Bottom: Year-level and month-level (within each year) correlations were computed. At the within year (between month level), correlations were computed with and without 2-weeks lag. Change in the magnitude of the Pearson correlation coefficient is shown with an arrow.
From a non-representative sample of bycatch events of common dolphins collected over more than 15 years, we estimated bycatch risk and number of dolphins bycaught in PTM. Leveraging recent methodological developments in the analysis of non-representative samples (Gao et al., 2019; Authier et al., 2021), we built a joint model of bycatch risk, haul duration, and haul number per DaS to investigate changes within and between years in common dolphin bycatch. The years 2017 and 2019 were associated with the highest bycatch risk and the longest haul duration in winter.
We uncovered the within-year pattern in bycatch risk of common dolphins. Bycatch risk is the highest in winter, during the first weeks of a calendar year. This pattern is largely congruent with the pattern seen in strandings of common dolphins in the Bay of Biscay (Gilbert et al., 2021). Both stranding and observer data, which are independent, identified 2017 and 2019 as years with the highest risk of bycatch (Gilbert et al., 2021; Peltier et al., 2021). A limitation of stranding data is how the location of bycatch events must be inferred with reverse drift modelling (Peltier and Ridoux, 2015). The ObsMer data in contrast included geolocalised bycatch events, with a spatial resolution at the level of ICES divisions kept for analysis. Despite this coarse resolution, we could identify divisions 8.a and 8.b as the ones with the highest risk of bycatch by PTM.
The ICES Working Group on Bycatch (WGBYC) estimate bycatch of protected species, including common dolphins, in the North East Atlantic. Using data collected by onboard observers collected between 2005 and 2017, bycatch rates for ICES divisions on the continental shelf of the Bay of Biscay were estimated with ratio estimators (ICES, 2019). These estimates are not produced at the week level, but ICES (2019) also identified divisions 8.a and 8.b as the ones with the highest of bycatch in midwater trawls for common dolphins over the period 2005–2017 (p. 61). ICES (2019) estimated yearly rates ranging between 0.285 and 0.372 dolphins per DaS and warned against extrapolation given the low observer coverage (p. 61). Our model-based approach overcomes this limitation (Authier et al., 2021) and was able to identify, within each year, that weeks 3 to 5 were the ones with the highest bycatch numbers for both divisions 8.a and 8.b. These results were concomitant with the seasonal stranding pattern observed each year on the French seashore (that is, winter strandings; Gilbert et al., 2021): around 80% of all common dolphin strandings on the French Atlantic seashore is observed between the end of January and the beginning of April.
A key feature of our model-based approach is how it leverages correlations between bycatch risk, haul duration, and number of hauls per DaS (Figure 3). Some of the correlations are expected, such as the negative correlation between haul duration and the number of hauls per DaS. However, average haul duration is not constant within a year, with the variations reflecting the change in the commercial fish species targeted by PTM at different time of the year. These variations at the week-level were quite substantial and were taken into account when estimating bycatch in our model. There was a positive correlation between haul duration and a bycatch risk, with at least a two-fold increase in the later when haul duration exceeds 5 h (Figure 3). This was particularly evident in weeks 1 to 5 in 2017 and 2019 and week 48 in 2016. The latter was due to a single fishing trip with 5 hauls that lasted >10 h, each of which resulted in a bycatch event. We recommend, in light of the within-year pattern in haul duration (Figure 3), to investigate management actions and mitigation measures on limiting haul duration in winter to assess whether bycatch may also be reduced.
Another possible mitigation measure is to manage common dolphin interactions with PTM with spatio-temporal closures (and acoustic repulsive devices such as pingers) during the first week of a year, when bycatch is the highest. Such measures were explored by WKEMBYC (ICES, 2020d) to reduce bycatch mortality across several scenarios. The performance of each scenario was assessed with the Potential Biological Removal (Wade, 1998), bycatch reduction rate, and fishing effort reduction rate. WKEMBYC (ICES, 2020d) defined an efficiency score by the ratio between the latter two rates. This efficiency score is a trade-off between the expected bycatch reduction and the cost for the fishing industry (without direct economic consideration). WKEMBYC (ICES, 2020d) identified one scenario (scenario L) wherein 2 months closure from mid January to mid March for all fishing métier (and the use of pingers for “Bottom pair trawlers” (PTB) and PTM the rest of the year) was efficient. This scenario appears as a good compromise between bycatch reduction and a reduced cost for the industry. Another efficient scenario (scenario N) involves a 3-month closure from January to March and another 1 month from mid July to mid August for all métier (and the use of pingers for PTB and PTM the rest of year). This scenario can achieve the highest level of bycatch reduction but incurs a high cost to the industry. However, scenarios considered by WKEMBYC are emergency measures meant to reduce punctually common dolphin bycatch. Systematic spatio-temporal closures, which are usually not favoured by the fisheries, were not considered and remained to be explored. In contrast, mitigation measures relying on the large scale deployment of acoustic repulsive devices and the development of new such devices are underway (e.g., in the CetAMBICion project4).
There were large between-year variations in model-based estimates of common dolphin bycatch in the study area. To some extent, these variations were explained by other factors than bycatch risk. For example, the (posterior median) estimate is >600 dolphins in 2017 down to <100 in 2018. The total effort in DaS in the Bay of Biscay (divisions 8.a and 8.b) in the first 10 weeks of 2017, when bycatch risk was highest, is two times the value of total effort in 2018. The median number of dolphins involved in a bycatch event in 2017 was also two times the number in 2018 (2 and 1, respectively, Table 2). All else being equal, the estimate for 2017 is expected to be at least four times that of 2018. A further improvement of the model-based approach is to jointly model the number of dolphins involved in a bycatch event. This improvement will require accomodating a large overdispersion, but there were however less than 100 such events in the dataset and we chose to use the median. This is a cautionary choice since the median is less sensitive to the few events for more than 10 dolphins. The uncertainty in the median number of dolphins involved in a bycatch event is currently ignored: incorporating it in future development will further widen credibility intervals (which are already large; Authier et al., 2021). Thus, the model-based estimates are conservative estimates of bycatch by PTM.
Bycatch risk was also very variable between years: the large between-year variations may be due to ecological factors. Bycatch risk results from both fisheries activity within a particular division at a particular time and dolphin presence. The highest bycatch risk values were estimated for the 8 or 10 first weeks of each year within each division of the study area (Figure 3). Astarloa et al. (2021) found evidence of an increased abundance of common dolphins in the Bay of Biscay in recent years but weak correlations with biological and oceanographic variables, such as chlorophyll a concentration or sea surface temperature. ICES divisions 8.a and 8.b cover the continental shelf parts of the Bay of Biscay (Figure 1). These neritic divisions are witness to sub-mesoscale oceanographic processes and nutrient offloads from the Gironde estuary. Gilbert et al., 2021 correlated eddies and frontal structures with common dolphin mortality areas at sea in the Bay of Biscay (although these authors also concluded that oceanographic accounted for a small fraction of the overall variance in stranding numbers). In winter, the Bay of Biscay environment is characterised by a seasonal cross-shore (West to East) surface temperature gradient with the lowest temperature close to shore and intense frontal activity parallel to the coast (North to South) (Yelekçi et al., 2017). These frontal structures are freshwater fronts, correlated to the mixing of oceanic waters and cold freshwater inputs from river plumes (Yelekçi et al., 2017). These seasonal fronts may be targeted by both fisheries and common dolphins as areas where fish aggregate, thereby putting the latter at risk of bycatch by the former. In July and August, the mesoscale dynamic activity of the Bay of Biscay is rather different than in winter. In summer, there are mainly fronts due to tidal flow (Yelekçi et al., 2017). Summer tidal fronts are quite consistent from 1 year to the next because they are correlated to a repetitive process (i.e., tides) (Yelekçi et al., 2017). During summer, the main frontal activity is a seasonal tidal front, called the Ushant Front and located in front of the French Finistère county (Yelekçi et al., 2017). Its activity peaks in July and August (Yelekçi et al., 2017). We can speculate that the years associated with a high bycatch risk were also those when oceanographic processes favouring the spatial overlap (mediated by fish species; Spitz et al., 2013; Astarloa et al., 2021) between fisheries and common dolphins were particularly operant.
Stranding records are an independent source of data for estimating the number of bycaught dolphins (Peltier et al., 2016). Reverse drift modelling allows the death location of each stranded dolphin showing bycatch evidence for each month between 1990 and 2020 to be inferred. Observed stranding tallies for each month can be corrected for both stranding and buoyancy probabilities (Peltier and Ridoux, 2015). Reverse drift modelling cannot disaggregate estimates by métiers or fisheries but provides an independent estimates of total mortality due to bycatch in the study area: bycatch mortality due to PTM should be lower than the total estimated from strandings. This was verified for all years save for 2010, but uncertainties were large and credibility intervals had a large overlap. While the correlation between model-based and stranding-based estimates was modest at the year level, it was larger at the within-year level, especially after accounting for a lag due to drift (Figure 4). The magnitude of the within-year correlation decreased between 2005 and 2020. One interpretation is that of a change in the relative contribution of PTM in total dolphin mortality over time, with PTM having a lesser impact on common dolphins in recent years compared to the 2000s.
The model used to estimate the bycatch of common dolphins in PTM has been developed to address the issue of non-representative sampling (Authier et al., 2021). It relies on a post-stratification step that requires accurate effort data at the scale of the whole fleet. The effort measurement retained was that of DaS as in international working groups (e.g., ICES WGBYC; ICES, 2019). Leveraging this important piece of information required the joint modelling of risk at the haul level, haul duration, and that of the average number of hauls per DaS. This modelling choice proved successful for PTM but need not be so for other métiers, in particular for passive gears such as gillnets and setnets. In the later case, a better measure of effort at haul level is soak time, taking into account net length and height, and possible mesh size. These pieces of information may be difficult to collect and retrospectively obtain for post-stratification. Any method seeking to scale up a sample from onboard observer to the whole fleet must confront the difficult issue of accurate measurement and quantification of effort. The model developed for PTM may not necessarily transfer seamlessly to other gears or métiers.
Since 2021, PTM flying the French flag are required to use deterrent acoustic devices (pingers5). If these devices are efficient to reduce bycatch risk, this may be taken into account in the model, by adding a covariate in Equation (1). Doing so requires on the other hand to post-stratify on that covariate, which is likely to be a major hurdle. Ignoring the deployment of pingers need not be problematic as the model allows for between- and within-year variations in bycatch risk. Large-scale deployment of pingers in 2021, if effective in reducing risk, will manifest itself in an estimated risk lower compared to previous years. In other words, the model does not have to necessarily take into account all haul-level covariates as long as the aim is prediction rather than explanation (Authier et al., 2021). Taking explicitly into account the pinger effect is only required to make sense of the between- and within-year variations in risk, but not necessarily to estimate those variations.
While Authier et al. (2021) concluded on increased accuracy of using regularised multi-level regression with post-stratification to estimate bycatch with observer data, they also found that estimated precision was low. This was also the case in this study (Table 5). A simple way to increase precision is to include self-declared positive bycatch events from fishermen in Equation (7) and (8). Doing so provides a strong incentive for compliance on self-declaration and would result in increased precision as a greater number of hauls (and possibly DaS) would be monitored. Ultimately, full compliance would render modelling moot as bycatch would be perfectly known, if all events were properly recorded (e.g., with Electronic Remote Monitoring) or reported systematically and accurately in logbooks.
The common dolphin is one of the most abundant delphinid species within the North-East Atlantic (Hammond et al., 2021). This species may be described as a “keystone species” and an “umbrella species” considering its ecological importance (Murphy et al., 2021). The large additional mortality due to anthropogenic activities on this species triggered a dedicated working group on emergency measures in 2020: the workshop on fisheries emergencies measures to minimise bycatch of short-beaked common dolphins in the Bay of Biscay and harbour porpoises in the Baltic Sea (WKEMBYC) took place remotely in spring 2020 (ICES, 2020d) and informed an ICES advice that same year (ICES, 2020c). This advice led to an infringement procedure issued in July 2020 against France for failing its obligations under the Habitats Directive, which lists the common dolphin as a species requiring full protection on its Annex IV. The same day, the Paris Administrative Court of Justice condemned the French government for failing to transpose and apply in a timely manner the dispositions of the Habitats Directive and Technical Measures regulating fisheries6 (in French). Following the unprecedented number of strandings in 2017, a national working group with fishermen, their representatives, government officials, Non-Governmental Organizations, and academics was initiated to address the bycatch issue (Peltier et al., 2021). One recommended action was to improve estimates of bycatch due to high-risk métiers, and to develop adequate methodologies to analyse data from non-representative samples (Authier et al., 2021). The present work reports on a case study on PTM and operating for a large part in the Bay of Biscay, and to a lesser extent in the Celtic seas. The model-based estimates (i) can inform on pressures acting on common dolphins as required by the Marine Strategy Framework Directive (EU 2008/56) and (ii) heed ICES recommendation to develop estimation methods to make the best use of already collected data to inform management in a timely manner (ICES, 2020c).
Using a Potential Biological Removal (PBR) approach (Wade, 1998), ICES (2020d) estimated a removal limit of common dolphin for the whole North-East Atlantic of 4, 926 individuals. An annual bycatch no greater than PBR would allow the population of common dolphins to recover to or be maintained at or above 50% of carrying capacity with a probability of 0.95 (Wade et al., 2021). This conservation objective is, however, different from the ASCOBANS interim objective “to restore and/or maintain stocks/populations to 80% or more of the carrying capacity.” Genu et al. (this issue) tuned a modified PBR to a quantitative interpretation of the ASCOBANS interim objective: “a population should be able to recover to or be maintained at 80% of carrying capacity, with probability 0.8, within a 100-year period.” The removals limit computed using the modified PBR was down to 985 animals (that is, one fifth of PBR; Genu et al., this issue): in 2017, the estimated bycatch due to PTM and operating the Bay of Biscay amounted to more than 60% of this limit (Table 5). In recent years, the estimated contribution of this métier relative to the modified PBR remained large according to our results. Other fishing métiers could potentially impact the common dolphins in the Bay of Biscay resulting in mortality exceeding the threshold inferred by both modified and non-modified PBR. Regarding vessels flying the French flag, gill trammel netters (GTR), gillnetters (GNS), and pair trawlers were potentially associated with common dolphin mortality in ICES divisions 8.a and 8.b for different years (regarding the co-occurrence of mortality and fishing effort) (Peltier et al., 2021). Estimating the contribution of each métiers to overall mortality remains a difficult endeavor. Regarding the PBR removals limit used in WKEMBYC (ICES, 2020d), the overall mortality considering all the fishing métiers exceed PBR, notably from 2016 to 2019, years associated with the suspected highest contribution for the métiers listed above.
We have provided a case study on estimating bycatch of common dolphins by PTM and operating in the Bay of Biscay from a non-representative sample of bycatch events collected by non-dedicated onboard observers. Leveraging recent methodological developments in statistical modelling, we have illustrated how to use imperfect but currently available data to inform management. Our contribution thus heeds two recent recommendations: to use adequate estimation methods on existing data and to gauge the resulting estimates against threshold values for incidental bycatch, tuned to relevant conservation objectives. We evidenced a substantial contribution of PTM to common dolphin bycatch in the Bay of Biscay, especially in 2017. Considering the entire time series and the correlations with the estimates made from strandings, it is possible that other métiers than PTM were associated with bycatch, especially in recent years. Currently, the main mitigation measures recommended are spatio-temporal closures and the widespread use of acoustic deterrent devices on PTM/OTM and PTB to repel dolphins (ICES, 2020b). Spatio-temporal closures were not implemented in 2021 but systematic and mandatory deployment of pingers on trawls were7. Relevant to management in broadening the scope of potential measures is the evidenced correlation between bycatch risk and haul duration: further studies should investigate limiting haul duration (for example, below 5 h) as a complementary mitigation strategy, especially in winter.
The original contributions presented in the study are included in the article/supplementary material, further inquiries can be directed to the corresponding author/s.
Ethical review and approval was not required for the animal study because this study uses data that is collected as part of the Data Collection Framework under the Common Fishery Policy of the European Union.
ER and MA led the analyses, the conception, and writing of the paper. LD, TC, and SD curated and prepared the data. MG designed the shiny application. All authors support in analyses, paper conception, and writing, contributed to the article, and approved the submitted version.
This work was supported in the form of salaries from (ADERA to MA) and (HiDef to KM) but did not have any additional role in the study design, data collection, and analysis, decision to publish, or preparation of the manuscript.
MA and HP are employed by the commercial company ADERA which did not play any role in this study beyond that of employer.
The remaining authors declare that the research was conducted in the absence of any commercial or financial relationships that could be construed as a potential conflict of interest.
All claims expressed in this article are solely those of the authors and do not necessarily represent those of their affiliated organizations, or those of the publisher, the editors and the reviewers. Any product that may be evaluated in this article, or claim that may be made by its manufacturer, is not guaranteed or endorsed by the publisher.
We thank the reviewers, Emilie Leblond, Thomas Rimaud, and Julien Lamothe for their constructive comments. We are indebted to Mikaël Guichard and the dedicated staff at the Mésocentre de Calcul de Poitou Charentes.
1. ^https://www.nytimes.com/2019/05/02/world/europe/france-dolphins-fishing.html
2. ^https://www.mer.gouv.fr/protecting-cetaceans-annick-giradin-presents-7-commitments-made-french-state-fishermen-and
3. ^https://sih.Ifremer.fr/Ressources/ObsMer
4. ^https://www.cetambicion-project.eu/
5. ^https://www.mer.gouv.fr/protecting-cetaceans-annick-giradin-presents-7-commitments-made-french-state-fishermen-and
6. ^http://paris.tribunal-administratif.fr/content/download/172866/1715763/version/1/file/1901535.pdf
7. ^https://www.mer.gouv.fr/protecting-cetaceans-annick-giradin-presents-7-commitments-made-french-state-fishermen-and
Alverson, D. L., Freeberg, M. H., Murawski, S. A., and Pope, J. (1994). A Global Assessment of Fisheries Bycatch and Discards, Vol. 339. Rome: Food & Agriculture Organization.
Anderson, C. R., Herrera, M., Ilangakoon, A. D., Koya, K. M., Moazzam, M., Mustika, P. L., et al. (2020). Cetacean bycatch in Indian Ocean tuna gillnet fisheries. Endang. Species Res. 41, 39–53. doi: 10.3354/esr01008
Anonymous (2016). Rapport annuel sur la mise en øeuvre du règlement Européen (CE) 812/2004 établissant les mesures relatives aux captures accidentelles de cétacés dans les pêcheries. Rapport annuel réglementaire. Technical report, Direction des Pêches Maritimes et de L'aquaculture (DPMA).
Anonymous (2019). Commission Implementing Decision (EU) 2019/910 of 13 March 2019 Establishing the multiannual Union Programme for the Collection and Management of Biological, Environmental, Technical and Socioeconomic Data in the Fisheries and Aquaculture Sectors. Brussels: European Comission
Astarloa, A., Louzao, M., Andrade, J., Berrow, S., Boisseau, O., Brereton, T., et al. (2021). The role of climate, oceanography, and prey in driving decadal spatio-temporal patterns of a highly mobile top predator. Front. Mar. Sci. 8:665474. doi: 10.3389/fmars.2021.665474
Authier, M., Rouby, E., and Macleod, K. (2021). Estimating Bycatch from Non-Representative Samples with (Regularized) Multilevel Regression with Post-Stratification. Technical report, Observatoire Pelagis UMS3462 CNRS-LRUniv.
Avila, I. C., Kaschner, K., and Dormann, C. F. (2018). Current global risks to marine mammals: taking stock of the threats. Biol. Conserv. 221, 44–58. doi: 10.1016/j.biocon.2018.02.021
Babcock, E. A., Pikitch, E. K., and Hudson, C. G. (2003). How Much Observer Coverage Is Enough to Adequately Estimate Bycatch? Miami, FL: Pew Institute of Ocean Science.
Bearzi, G., and Reeves, R. R. (2021). Shifting baselines of cetacean conservation in Europe. ICES J. Mar. Sci. 78, 2337–2341. doi: 10.1093/icesjms/fsab137
Benoît, H., and Allard, J. (2009). Can the data from at-sea observer surveys be used to make general inference about catch composition and discards? Can. J. Fish. Aquat. Sci. 66, 2025–2039. doi: 10.1139/F09-116
Bessesen, B. (2018). Vaquita - Science, Politics, and Crime in the Sea of Cortez. Washington: Island Press.
Brownell, R. J., Reeves, R., Read, A., Smith, B., Thomas, P., Ralls, K., et al. (2019). Bycatch in gillnet fisheries threatens critically endangered small cetaceans and other aquatic megafauna. Endang. Species Res. 40, 285–296. doi: 10.3354/esr00994
Carpenter, B., Gelman, A., Hoffman, M. D., Lee, D., Goodrich, B., Betancourt, M., et al. (2017). Stan: a probabilistic programming language. J. Stat. Softw. 76, 1–32. doi: 10.18637/jss.v076.i01
Chen, Z., and Dunson, D. (2003). Random effects selection in linear mixed models. Biometrics 59, 762–769. doi: 10.1111/j.0006-341X.2003.00089.x
Cornou, A. S., Goascoz, N., Scavinner, M., Prioul, F., Sabbio, A., Dubroca, L., et al. (2018). Captures et rejets des métiers de pêche français. résultats des observations à bord des navires de pêche professionnelle en 2017. Technical report, Ifremer.
Course, G. P. (2021). Monitoring Cetacean Bycatch: An Analysis of Different Methods Aboard Commercial Fishing Vessels. ASCOBANS Secretariat, Bonn.
Davies, R., Cripps, S., Nickson, A., and Porter, G. (2009). Defining and estimating global marine fisheries bycatch. Mar. Policy 33, 661–672. doi: 10.1016/j.marpol.2009.01.003
Fernández-Contreras, M., Cardona, L., Lockyer, C., and Aguilar, A. (2010). Incidental bycatch of short-beaked common dolphins (Delphinus delphis) by pairtrawlers off Northwestern Spain. ICES J. Mar. Sci. 67, 1732–1738. doi: 10.1093/icesjms/fsq077
Gao, Y., Kennedy, L., Simpson, D., and Gelman, A. (2019). Improving Multilevel Regression and Poststratification with Structured Priors. Report, University of Toronto & Columbia University.
García-Baron, I., Santos, M. B., Uriarte, A., Inchausti, J. I., Escribano, J. M., Albisu, J., et al. (2019). which are the main threats affecting the marine megafauna in the Bay of Biscay? Continent. Shelf Res. 186, 1–12. doi: 10.1016/j.csr.2019.07.009
Gelman, A., Hill, J., and Vehtari, A. (2021). Regression and Other Stories, 1st Edn. Cambridge: Cambridge University Press. doi: 10.1017/9781139161879
Genu, M., Gilles, A., Hammond, P., Macleod, K., Paillé, J., and Paradinas, I. A. (this issue). Evaluating strategies for managing anthropogenic mortality on marine mammals: an R implementation with the package RLA. in preparation.
Gilbert, L., Rouby, E., Tew-Kaï, E., Spitz, J., Peltier, H., Quilfen, V., et al. (2021). Spatiotemporal models highlight influence of oceanographic conditions on common dolphin bycatch risk in the Bay of Biscay. Mar. Ecol. Prog. Ser. 679, 195–212. doi: 10.3354/meps13894
Griffin, J., and Brown, P. (2017). Hierarchical shrinkage priors for regression models. Bayesian Anal. 12, 135–159. doi: 10.1214/15-BA990
Hammond, P. S., Lacey, C., Gilles, A., Viquerat, S., Börjesson, P., Herr, H., et al. (2021). Estimates of Cetacean Abundance in European Atlantic Waters in Summer 2016 from the SCANS-III Aerial and Shipboard Surveys. Technical report, Sea Mammal Research Unit, University of Saint Andrews.
Hoffman, M. D., and Gelman, A. (2014). The no-U-turn sampler: adaptively setting path lengths in Hamiltonian Monte Carlo. J. Mach. Learn. Res. 15, 1593–1623.
ICES (2019). Working Group on Bycatch of Protected Species (WGBYC). Technical report, International Council for the Exploration of the Sea, Faro.
ICES (2020a). Bycatch of Protected and Potentially Vulnerable Marine Vertebrates - Review of National Reports under Council Regulation (EC) No. 812/2004 and Other Information. ICES Advice: Ecoregions in the Northeast Atlantic and Adjacent Seas. Technical report.
ICES (2020b). EU Request on Emergency Measures to Prevent Bycatch of Common Dolphin (Delphinus delphis) and Baltic Proper Harbour Porpoise (Phocoena phocoena) in the Northeast Atlantic. Report of the ICES Advisory Committee.
ICES (2020c). Road Map for ICES Bycatch Advice on Protected, Endangered, and Threatened Species. Report of the ICES Advisory Committee.
ICES (2020d). Workshop on Fisheries Emergency Measures to Minimize BYCatch of Short-Beaked Common Dolphins in the Bay of Biscay and Harbour Porpoise in the Baltic Sea (WKEMBYC). International Council for the Exploration of the Sea.
Kuiken, T. (1994). “Review of the criteria for the diagnosis of by-catch in cetaceans,” in Diagnosis of By-Catch in Cetaceans. Proc. 2nd. ECS Workshop on Cetacean Pathology (Montpellier), 38–43.
Louis, T., and Zeger, S. (2009). Effective communication of standard error and confidence interval. Biostatistics 10, 1–2. doi: 10.1093/biostatistics/kxn014
Luck, C., Jessopp, M., Tully, O., Cosgrove, R., Rogan, E., and Cronin, M. (2020). Estimating protected species bycatch from limited observer coverage: a case study of seal bycatch in static net fisheries. Glob. Ecol. Conserv. 24:e01213. doi: 10.1016/j.gecco.2020.e01213
Moore, J., Heinemann, D., Francis, T., Hammond, P., Long, K. J., Punt, A. E., et al. (2021). Estimating bycatch mortality for marine mammals: concepts and best practices. Front. Mar. Sci. 8, 752356. doi: 10.3389/fmars.2021.752356
Murphy, S., Evans, P. G. H., Pinn, E., and Pierce, G. J. (2021). Conservation management of common dolphins: lessons learned from the North-East Atlantic. Aquat. Conserv. 31, 137–166. doi: 10.1002/aqc.3212
Murphy, S., Pinn, E. H., and Jepson, P. D. (2013). “The short-beaked common dolphin (Delphinus delphis) in the North-East Atlantic: distribution, ecology, management and conservation status,” in Oceanography and Marine Biology: an Annual Review, ASCOBANS eds. (The Hague: CRC Press), 123–210.
Peltier, H., Authier, M., Caurant, F., Dabin, W., Daniel, P., Dars, C., et al. (2021). In the wrong place at the wrong time: identifying spatiotemporal co-occurrence of bycaught common dolphins and fisheries in the Bay of Biscay (NE Atlantic) from 2010 to 2019. Front. Mar. Sci. 8:617342. doi: 10.3389/fmars.2021.617342
Peltier, H., Authier, M., Deaville, R., Dabin, W., Jepson, P. D., van Canneyt, O., et al. (2016). Small cetacean bycatch as estimated from stranding schemes: the common dolphin case in the Northeast Atlantic. Environ. Sci. Policy 63, 7–18. doi: 10.1016/j.envsci.2016.05.004
Peltier, H., Dabin, W., Daniel, P., Van Canneyt, O., Dorémus, G., Huon, M., et al. (2012). The significance of stranding data as indicators of cetacean populations at sea: modelling the drift of cetacean carcasses. Ecol. Indicat. 18, 278–290. doi: 10.1016/j.ecolind.2011.11.014
Peltier, H., Jepson, P., Dabin, W., Deaville, R., Daniel, P., Van Canneyt, O., et al. (2014). The contribution of stranding data to monitoring and conservation strategies for cetaceans: developing spatially explicit mortality indicators for common dolphins (Delphinus delphis) in the eastern north-atlantic. Ecol. Indicat. 39, 203–214. doi: 10.1016/j.ecolind.2013.12.019
Peltier, H., and Ridoux, V. (2015). Marine megavertebrates adrift: a framework for the interpretation of stranding data in perspective of the European marine strategy framework directive and other regional agreements. Environ. Sci. Policy 54, 240–247. doi: 10.1016/j.envsci.2015.07.013
Pérez, M., Perrichi, L. R., and Ramírez, I. (2017). The scaled Beta2 distribution as a robust prior for scales. Bayesian Anal. 12, 615–637. doi: 10.1214/16-BA1015
R Core Team (2020). R: A Language and Environment for Statistical Computing. Vienna: R Foundation for Statistical Computing.
Rayner, S. (2012). Uncomfortable knowledge: the social construction of ignorance in science and environmental policy discourses. Econ. Soc. 41, 107–125. doi: 10.1080/03085147.2011.637335
Read, A. J. (2008). The looming crisis: interactions between marine mammals and fisheries. J. Mammal. 89, 541–548. doi: 10.1644/07-MAMM-S-315R1.1
Read, A. J., Drinker, P., and Northridge, S. (2006). Bycatch of marine mammals in us and global fisheries. Conserv. Biol. 20, 163–169. doi: 10.1111/j.1523-1739.2006.00338.x
Rogan, E., and Mackey, M. (2007). Megafauna bycatch in drift nets for albacore tuna (Thunnus alalunga) in the ne atlantic. Fish. Res. 86, 6–14. doi: 10.1016/j.fishres.2007.02.013
Rogan, E., Read, A. J., and Berggren, P. (2021). Empty promises: the European union is failing to protect dolphins and porpoises from fisheries by-catch. Fish Fish. 22, 1–5. doi: 10.1111/faf.12556
Sala, A., Konrad, C., and Doerner, H., (eds.). (2019). Review of the Implementation of the EU Regulation on the Incidental Catches of Cetaceans (STECF-19-07). Publications Office of the European Union, Luxembourg.
Scarff, J. E. (1977). The international management of whales, dolphins, and porpoises: an interdisciplinary assessment. Ecol. Law Quart. 6, 323–427.
Schweder, T. (2000). Distortion of uncertainty in science: Antarctic fin whales in the 1950s. J. Int. Wildlife Law Policy 3, 73–92. doi: 10.1080/13880290009353947
Spitz, J., Chouvelon, T., Cardinaud, M., Kostecki, C., and Lorance, P. (2013). Prey preferences of adult sea bass Dicentrarchus labrax in the Northeastern Atlantic: implications for bycatch of common dolphin Delphinus delphis. ICES J. Mar. Sci. 70, 452–461. doi: 10.1093/icesjms/fss200
Systéme d'Information Halieutique (2017). Données de production et d'effort de pêche (SACROIS) - 2003–2017.
Thorson, J. T. (2019). Guidance for decisions using the vector autoregressive spatio-temporal (VAST) package in stock, ecosystem, habitat and climate assessments. Fish. Res. 210, 143–161. doi: 10.1016/j.fishres.2018.10.013
Thorson, J. T., Skaug, H. J., Kristensen, K., Shelton, A. O., Ward, E. J., Harms, J. H., et al. (2015). The importance of spatial models for estimating the strength of density dependence. Ecology 96, 1202–1212. doi: 10.1890/14-0739.1
Ulrich, C., and Doerner, H., (eds.). (2021). Scientific, Technical and Economic Committee for Fisheries (STECF) - 66th Plenary Report (PLEN-21-01). Publications Office of the European Union, Luxembourg.
Wade, P. R. (1998). Calculating limits to the total allowable human-caused mortality of cetaceans and pinnipeds. Mar. Mammal Sci. 14, 1–37. doi: 10.1111/j.1748-7692.1998.tb00688.x
Wade, P. R., Long, K. J., Francis, T. B., Punt, A. E., Hammond, P. S., Heinemann, D., et al. (2021). Best practices for assessing and managing bycatch of marine mammals. Front. Mar. Sci. 8:757330. doi: 10.3389/fmars.2021.757330
Ward, E. J., Jannot, J. E., Lee, Y.-W., Ono, K., Shelton, A. O., and Thorson, J. T. (2015). Using spatiotemporal species distribution models to identify temporally evolving hotspots of species co-occurrence. Ecol. Appl. 25, 2198–2209. doi: 10.1890/15-0051.1
Keywords: additional mortality, anthropogenic activities, modelling, non-representative samples, conservation, small cetaceans, fisheries, post-stratification
Citation: Rouby E, Dubroca L, Cloâtre T, Demanèche S, Genu M, Macleod K, Peltier H, Ridoux V and Authier M (2022) Estimating Bycatch From Non-representative Samples (II): A Case Study of Pair Trawlers and Common Dolphins in the Bay of Biscay. Front. Mar. Sci. 8:795942. doi: 10.3389/fmars.2021.795942
Received: 15 October 2021; Accepted: 02 December 2021;
Published: 13 January 2022.
Edited by:
Jeff Moore, Southwest Fisheries Science Center (NOAA), United StatesReviewed by:
Stephen Stohs, Southwest Fisheries Science Center (NOAA), United StatesCopyright © 2022 Rouby, Dubroca, Cloâtre, Demanèche, Genu, Macleod, Peltier, Ridoux and Authier. This is an open-access article distributed under the terms of the Creative Commons Attribution License (CC BY). The use, distribution or reproduction in other forums is permitted, provided the original author(s) and the copyright owner(s) are credited and that the original publication in this journal is cited, in accordance with accepted academic practice. No use, distribution or reproduction is permitted which does not comply with these terms.
*Correspondence: Matthieu Authier, bWF0dGhpZXUuYXV0aGllckB1bml2LWxyLmZy
†Present address: Kelly Macleod, HiDef Aerial Surveying Limited, Lillyhall, United Kingdom
Disclaimer: All claims expressed in this article are solely those of the authors and do not necessarily represent those of their affiliated organizations, or those of the publisher, the editors and the reviewers. Any product that may be evaluated in this article or claim that may be made by its manufacturer is not guaranteed or endorsed by the publisher.
Research integrity at Frontiers
Learn more about the work of our research integrity team to safeguard the quality of each article we publish.