- 1College of Fisheries, Guangdong Ocean University, Zhanjiang, China
- 2Institute of Aquaculture, University of Stirling, Stirling, United Kingdom
- 3Chinese Academy of Inspection and Quarantine, Beijing, China
- 4Guangdong Provincial Key Laboratory of Pathogenic Biology and Epidemiology for Aquatic Economic Animal, Key Laboratory of Control for Disease of Aquatic Animals of Guangdong Higher Education Institutes, Zhanjiang, China
- 5Guangdong Provincial Laboratory of Southern Marine Science and Engineering, Zhanjiang, China
Biofloc technology (BFT) is one of the most promising technologies in global aquaculture for the purpose of improving water quality, waste treatment, and disease prevention in intensive aquaculture systems. However, characterization of the microbial species and antibiotic resistance potentially present in biofloc-based aquaculture environments is needed. In this study, we used high-throughput sequencing technology to comprehensively compare the bacterial communities in mariculture ponds of Penaeus monodon (P. monodon), by testing of water, biofloc, and intestine of P. monodon. Operational taxonomic units (OTUs) cluster analysis showed that the nine samples tested divided into 45 phyla and 457 genera. Proteobacteria was the dominant bacteria in water, biofloc and prawn intestine. In biofloc and intestine, the Ruegeria (2.23–6.31%) genus represented the largest proportion of bacteria, with Marivita (14.01–20.94%) the largest group in water. Microbial functional annotation revealed that in all the samples, genes encoding metabolism were predominant. The antibiotic resistance gene annotation showed the highest absolute abundance of patB, adeF, OXA-243, and Brucella_suis_mprF from Proteobacteria. PatB (11.33–15.01%), adeF (15.79–18.16%), OXA-243 (35.65%), and Brucella_suis_mprF (10.03%) showed the highest absolute abundance of antibiotic resistance genes in water, biofloc, and intestines, respectively. These findings may greatly increase our understanding of the characteristics of the microbiota of shrimp biofloc-based aquaculture systems and the complex interactions among shrimp, ambient microflora, and environmental variables. It provides a reference basis for policy on breeding, environmental safety, and maintaining food safety in the production of P. monodon.
Introduction
With the pollution of the marine aquatic environment and the decline of the wild fishing industry, aquaculture plays an increasingly important role in the sustainable supply of food. Penaeus monodon (P. monodon) (giant tiger shrimp) aquaculture accounts for a significant proportion of seafood consumption and is the second most farmed variety of shrimp worldwide (FAO, 2020).
However, bacterial diseases such as death by early mortality syndrome (EMS), acute hepatopancreatic necrosis disease (AHPND), and hepatopancreatic necrosis syndrome (HPNS) have reduced shrimp production by nearly 40%, resulting in global losses of over 1 billion dollars per year for shrimp aquaculture (Flegel, 2012; Lee et al., 2015; Huang et al., 2016). Furthermore, the misuse and unmonitored discharge of antibiotics has led to the proliferation and spread of antibiotic resistance genes (ARGs) and antibiotic-resistant bacteria in the aquatic environment, leading to poor outcomes for aquaculture and the surrounding environments (Pruden, 2014; Shlrene et al., 2020). The occurrence of ARGs, as environmental contaminants, in aquatic environments is an emerging public health concern, attracting global attention (Pruden et al., 2006; Sui et al., 2016; Dang et al., 2017).
As an environmentally friendly and economical aquaculture model, biofloc technology has continued to attract attention in aquaculture systems around the world (Wilson et al., 2006; Yoram, 2007; Mishra et al., 2008). A biofloc-based aquaculture system is a microbial treatment based on the composition of abundant microflora. In this system, chemoautotrophic and heterotrophic bacteria are involved in the formation of biofloc, which also include fungi, protozoa, zooplankton, and microalgae, which decompose food remnants and animal waste, remove excess ammonia and nitrogen from the water, and maintain a stable nutrient level (Yoram, 1999; Wei et al., 2020). Animal productivity is intimately linked to health and the gut microbiome is becoming increasingly recognized as an important driver of cultivation success (Corey et al., 2020). Besides, effective microorganisms can increase survival rates of shrimp and decrease the feed conversion ratio (Huang, 2009). In addition, biofloc can be used as a supplementary food source for animals, which can improve the growth performance and immunity of cultured organisms (Julie et al., 2014; Kim et al., 2015). Biofloc technology (BFT) cannot only enhance growth performance of an organism, elevate digestive enzyme activity, and strengthen antioxidant status, but also has been shown to improve immune response through the probiotic and immunostimulant effects of the microorganisms present in the biofloc (Yu et al., 2020).
In fact, most eukaryotes maintain close mutualistic relationships with microorganisms that are, in most cases, linked to their nutrient acquisition and thereby crucial for their performance and survival (Stéphane et al., 2015). There have been few studies of the bacterial communities and ARGs of biofloc (Surapun et al., 2020). In order to understand the optimal conditions for establishing and maintaining a biofloc, it is necessary to characterize the microorganisms present. Culture-dependent approaches present a limitation to characterizing the complete microbial population present in biofloc. It has been estimated that the cultivation approach commonly reveals only a small portion of the bacterial population present, of which only 0.1% has characterized (Zhou et al., 2019). Microbial ribosomal RNA (rRNA) genes can be detected and sequenced directly from water or biofloc without the need for culture. In this study, we used metagenomic sequencing to comprehensively compare the structure and function of bacterial communities in water, biofloc, and intestinal samples from a biofloc-based aquaculture system of P. monodon and analyzed the ARGs therein. This study provides data to support future improvements of the biofloc-based aquaculture system of P. monodon, which will inform the policies of breeding, environmental safety, and assist in maintaining food safety for this valuable species.
Materials and Methods
Pond Conditions
The P. monodon culture ponds used in this study were 3 m × 4 m, with a depth of 1.5 m and water depth of 1.2 m, the seedling size was 1.0 ± 0.1 cm, and the seedling density was 500 shrimp seeds/m3. The culture was according to the biofloc-based aquaculture environments. Glucose was used to adjust the C/N (carbon to nitrogen ratio) and sodium bicarbonate was used to adjust the total alkalinity and pH.
Sample Collection and Extraction of Total DNA of Microbial Flora
Samples were collected on June 15, 2020 from three shrimp biofloc ponds, located at Donghai Island Marine Biological Research Base, Guangdong Ocean University, Zhanjiang, Guangdong, China. At the time of sampling, the culture time of shrimp was 150 days and the average body length and body weight of puffers were 11.25 ± 0.79 cm and 19.67 ± 3.84 g, respectively. To fully reflect the pond condition, each intestinal sample randomly captured the intestines of 60 shrimps from a pond and pooled them into one sample. Water and biofloc samples were collected at five locations in the pond (the four diagonal and middle locations of the pond) and mixed into one sample. The suspended matter was settled by standing water samples for 1 to 2 h. The supernatant was filtered through a membrane (0.45 μm, Jinteng) with a diaphragm vacuum pump (give make and model of pump). Each filter membrane enriched with microorganisms was installed into a Biofil 50-ml centrifuge tube and labeled A1, A2, and A3, respectively. Biofloc samples of 25 ml were taken from the biofloc pool and labeled B1, B2, and B3, respectively. The intestinal samples were dissected by sterile scalpel and the entire intestine was removed under sterile conditions. The intestinal segments were immediately placed on ice in a RNA-free tube and labeled C1, C2, and C3, respectively. Each intestinal sample tube was placed in liquid nitrogen, transported to the laboratory, and stored at −80°C prior to DNA extraction. During 150 days of the study, no disease occurred in the shrimp and no antibiotics or drug treatments were applied to the ponds. The three ponds use water from the sea at the farming site. Hengxing shrimp compound feed was used throughout the whole process and the same management was carried out during the breeding period. The genomic DNA of water, biofloc, and intestinal samples was extracted by the cetyltrimethylammonium bromide (CTAB) method (Khalid et al., 2021) and the purity and concentration of DNA was measured by agar gel electrophoresis. DNA samples were diluted to 1 μg/μl with sterile water.
Physicochemical Parameters of Water Quality
Water quality indicators were tested at the same time as sampling. The pH and temperature were measured in situ with Shanghai Sanxin SX620 measuring pen. Concentrations of nitrite (NO2–N), nitrate (NO3–N), ammonia (NH4+-N), and total alkalinity (TA) were determined by the chemical method (Lei, 2006).
16S Ribosomal RNA Gene Amplification and Sequencing
To investigate the microbial communities of water, biofloc, and intestinal samples, the 16S rRNA gene was amplified using the diluted genomic DNA as the template and a set of universal primes, 16S V3-V4 primer 515F (5′- GTGCCAGCMGCCGCGGTAA-3′) and 806R (5′-GGACTACHVGGGTWTCTA AT-3′). All the PCR reactions were carried out with 15 μl of the Phusion® High-Fidelity PCR Master Mix (New England Biolabs, America), 2 μM of forward and reverse primers, and about 10 ng of template DNA. The PCR reaction procedures were as follows: thermal cycling consisted of initial denaturation at 98°C for 1 min, followed by 30 cycles of denaturation at 98°C for 10 s, annealing at 50°C for 30 s, and elongation at 72°C for 30 s and finally, 72°C for 5 min. A total of 5 μl of each PCR product was separated on a 2% agarose gel (Sangon Biotech, Shanghai, China) containing 0.1 μg ml–1 ethidium bromide in 0.5× Tris-acetate-EDTA (TAE) buffer (20 mM Tris and 10 mM acetic acid) (Servicebio, Wuhan, China). 100–2,000 bp DNA ladder (Sangon Biotech, Shanghai, China) was used as molecular size marker. PCR products were mixed in equidensity ratios. Then, this mixture of PCR products was purified with the Qiagen Gel Extraction Kit (Qiagen, Germany, United Kingdom). The purified PCR products were used for library preparation and high-throughput sequencing on a MiSeq sequencer (Novogene Illumina, Tianjin, China). The sequencing data were deposited into the Sequence Read Archive under accession number PRJNA758114.
Analysis and Processing of 16S Ribosomal RNA Gene Sequencing Data
Paired-end reads were assigned to samples based on their unique barcode and truncated by cutting off the barcode and primer sequence. Paired-end reads were merged using Flash (FLASH) (Magoč and Salzberg, 2011), a very fast and accurate analysis tool, which was designed to merge paired-end reads when at least some of the reads overlap the read generated from the opposite end of the same DNA fragment and the splicing sequences were called raw tags. Quality filtering on the raw tags was performed under specific filtering conditions to obtain the high-quality clean tags (Bokulich et al., 2013) according to the Quantitative Insights Into Microbial Ecology (QIIME) (Caporaso et al., 2010) quality-controlled process. The tags were compared with the reference database (Silva database), using UCHIME (a new program that detects chimeric sequences with two or more segments) algorithm (Robert et al., 2011) to detect the chimera sequences, remove the chimera sequences (Brian et al., 2011), and finally get effective tags.
Sequence analysis was performed by Uparse software (Edgar, 2013). Sequences with ≥97% similarity were assigned to the same operational taxonomic units (OTUs). Representative sequences for each OTU were screened for further annotation. For each representative sequence, the Silva database (Quast et al., 2013) was used based on the Mothur algorithm to annotate taxonomic information. Multiple sequence alignment with improved accuracy and speed (MUSCLE) (Edgar, 2004) (version 3.8.311) software was used to perform rapid multiple sequence alignment and obtain the phylogenetic relationship of all the OTUs representative sequences. The data of each sample were normalized and the sample with the least amount of data was used as the standard. The subsequent alpha diversity analysis and beta diversity analysis are based on the data after normalization. The differences among groups were analyzed by the one-way ANOVA with multiple comparisons of the Tukey’s honestly significant difference (HSD) test using the SPSS software version 17.0, SPSS 17.0. p < 0.05 was considered as statistically significant.
Metagenome Gene Amplification and Sequencing
The methods of quality control for DNA samples: degree of DNA degradation and potential contamination were monitored on 1% agarose gels. DNA concentration was measured using the Qubit® dsDNA Assay Kit in Qubit® 2.0 Fluorometer (Life Technologies, California, United States). The optical density (OD) value was between 1.8 and 2.0 and DNA contents above 1 μg were used to construct the library. A total amount of DNA per sample was used as input material for the DNA sample preparations. Sequencing libraries were generated using the NEBNext® Ultra DNA Library Prep Kit for Illumina (NEB, Ipswich. Massachusetts, United States) following the recommendations of the manufacturer and index codes were added to attribute sequences to each sample. The DNA sample was fragmented by sonication to a size of 350 bp and then DNA fragments were end-polished, A-tailed, and ligated with the full-length adaptor for Illumina sequencing with further PCR amplification. Finally, PCR products were purified (AMPure XP System, Hangzhou, China) and libraries were analyzed for size distribution by the Agilent 2100 Bioanalyzer System and quantified using real-time PCR.
Metagenome Sequencing, Data Preprocessing, and Assembly
Preprocessing of the raw data obtained from the Illumina HiSeq sequencing platform was conducted with Readfq (V82) to acquire the clean data for subsequent analysis. The specific processing steps were as follows: reads with low-quality bases were removed (default quality threshold value ≤38) above a certain portion (default length of 40 bp), reads with N base that reached a certain percentage were removed (default length of 10 bp), and reads with shared the overlap above a certain portion with adapter were removed (default length of 15 bp). Considering the possibility of host contamination might exist in samples, clean data needed to be blast to the host database, which default using Bowtie2.2.4 software (Bowtie2.2.43) to filter the reads that are of host origin. The Bowtie parameters (Karlsson et al., 2012, 2013) were: end-to-end, sensitive, I 200, and X 400.
Clean data were assembled and analyzed by SOAPdenovo software (version 2.04) (Luo et al., 2015), used MEGAHIT software (version 1.0.4-beta) to assemble clean data, and then disconnect the assembled scaftigs from the N connection and make scaftigs without N (Mende et al., 2012; Nielsen et al., 2014; Qin et al., 2014). The clean data of all the samples were compared with each scaffold through Bowtie 2.2.4 software to obtain unused paired end (PE) readings (Qin et al., 2014). Combined the unused reads of all the samples in the previous step and then used SOAPdenovo (version 2.04)/MEGAHIT (version 1.0.4-beta) software for mixed assembly, the parameters were the same as single assembly; disconnect the mixed assembly from the N connection scaffolds and got scaftigs. Filter all the fragments that were shorter than 500 bp in scaftigs for statistical analysis. The genes with reads ≤2 in each sample were filtered to obtain the final gene catalog (Unigenes) for subsequent analysis.
Metagenome Sequencing Data Analysis
According to the number of mapped reads and the length of genes, the abundance information of each gene in each sample was counted (Qin et al., 2010; Fredrik et al., 2012). Basic information statistics, core-pan gene analysis, sample correlation analysis, and gene number Venn diagram analysis are all based on the abundance of each gene in each sample in the gene catalog. Double index alignment of next-generation sequencing data (DIAMOND) (Buchfink et al., 2015) software (version 0.9.9) was used to blast Unigenes into bacteria, fungi, archaea, and viruses sequences extracted from the National Center for Biotechnology Information (NCBI) non-redundant (NR) database. For the final alignment results of each sequence, since each sequence may have multiple alignment results, the result of E-value ≤minimum E-value × 10 (Julia et al., 2014) was selected as the least common ancestor (LCA) algorithm applied to Metagenome Analyzer (MEGAN) (Daniel et al., 2011) system classification of software to ensure the species annotation information of the sequence. According to the LCA annotation results and the gene abundance table, a table containing the number and abundance information of each sample in each classification level (boundary, phylum, class, order, family, genus, and species) was obtained. Linear discriminant analysis Effect Size (LEfSe) analysis was performed by LEfSe software (the default linear discriminant analysis (LDA) score is 3) (Nicola et al., 2011). DIAMOND software (version 0.9.9) was used to blast Unigenes into the Kyoto Encyclopedia of Genes and Genomes (KEGG) (Kanehisa et al., 2006, 2014) functional database. According to the function annotation results and the gene abundance table, the predicted functional pathways of each sample microbiota were obtained.
Resistance Gene Annotation
The Resistance Gene Identifier (RGI) software was used to align Unigenes with the Comprehensive Antibiotic Resistance Database (CARD)4 (Mcarthur et al., 2013; Martínez et al., 2014; Jia et al., 2017) with the parameter set to Blastp (BLASTP), E-value ≤1e–30. According to the comparison result, the relative abundance of the antibiotic resistance ontology (ARO) in all the genes was calculated as parts per million (ppm). According to the abundance of the ARO, the abundance bar graph and the number difference between the resistant genomes are displayed. In the same way, the abundance distribution of resistance genes in each sample, the species attribution analysis of resistance genes, and the resistance mechanism of resistance genes were analyzed.
Results
Sequencing Results
The 16S rRNA sequencing results of 72,444–95,756 raw tags, 70,791–93,569 clean tags, and 47,842–62,966 effective tags were obtained from the nine samples (three water: AS, three biofloc: BS, and three intestinal samples: CS). As shown in Table 1, Good’s coverage revealed that 99.23–99.49% of species were obtained in all the nine samples. The Shannon and Simpson indexes, Chao1 together with abundance-based Coverage Estimator (ACE) values of the nine samples varied from 5.410 to 7.467, 0.900 to 0.977, 1096.049 to 1680.477, and 1165.578 to 1762.483, respectively. The Shannon index of BS and CS was significantly higher than that of AS (p < 0.05), indicating that the bacterial abundance of BS and CS was the highest of all the three samples and BS was higher than CS. Although the difference was not always statistically significant, biofloc had the highest species abundance, taking into account various indicators.
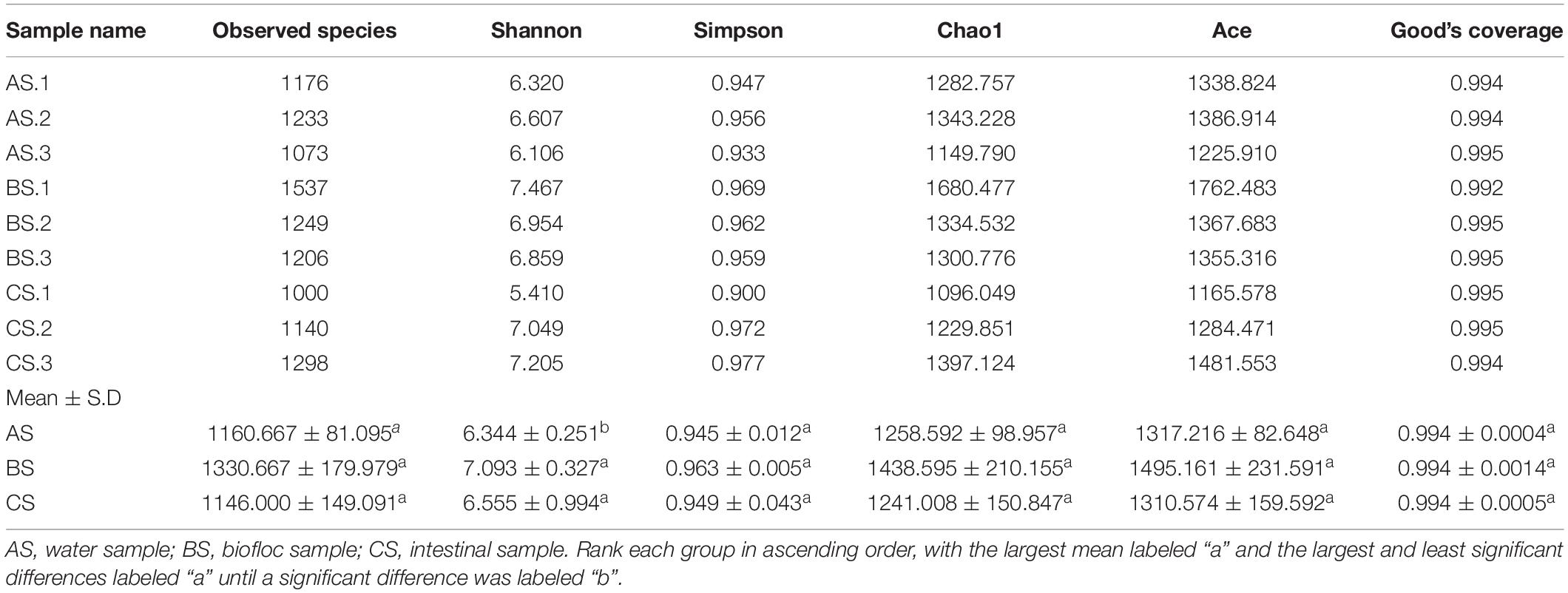
Table 1. Alpha diversity metrics of microbiotas in water, biofloc, and intestine of shrimp sampled from three biofloc ponds.
Taxonomic Profiles of Microbiota in Shrimp Biofloc Ponds
According to the OTUs clustering results, species information and species-based abundance distribution of the nine samples were obtained. Figures 1A–F depict species abundance detected at different taxonomic levels. In total, 45 identified phyla were observed and ten phyla were identified at an abundance >1%. As shown in Figure 1, in AS, Proteobacteria (55.67–61.04%), Bacteroidetes (8.89–10.26%), Chloroflexi (2.61–8.74%), Firmicutes (3.46–6.54%), and Actinobacteria (2.24–4.46%) were the most abundant phyla. Chloroflexi (14.26–17.30%) and Actinobacteria (4.81–9.25%) were the second and third dominant phylum after Proteobacteria (47.31–51.32%) in BS. In CS, the top three abundant phyla were Proteobacteria (55.41–62.15%), Firmicutes (10.01–14.28%), and Bacteroidetes (3.43–13.27%).
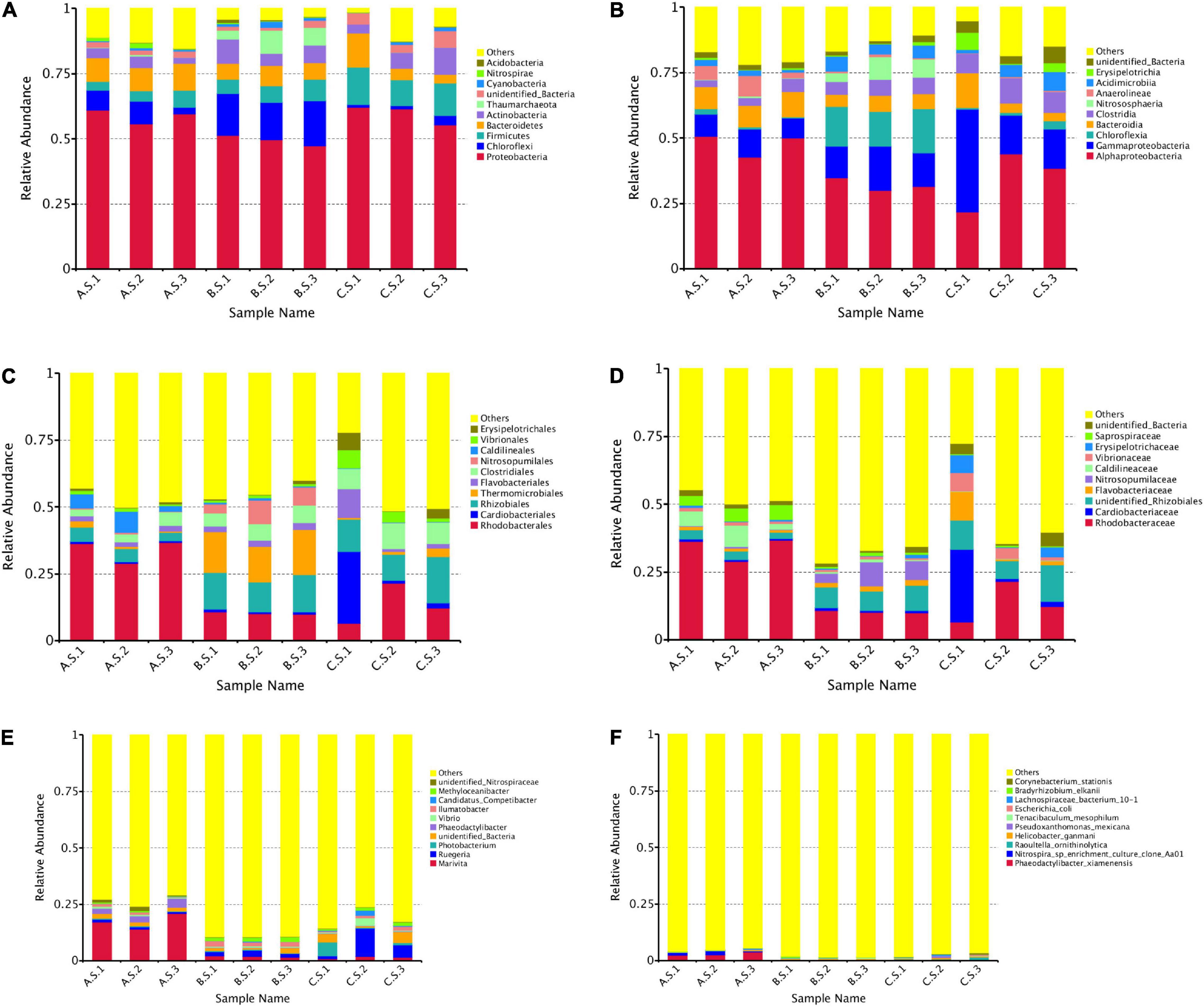
Figure 1. Relative abundances of the dominant bacteria in each sample obtained from 16S rRNA gene sequencing (A) (phylum), (B) (class), (C) (order), (D) (family), (E) (genus), and (F) (species). AS, BS, and CS were water, biofloc, and intestinal samples of shrimp sampled from biofloc ponds. AS, water sample; BS, biofloc sample; CS, intestinal sample; 16S rRNA, 16S ribosomal RNA.
At the class level (Figure 1B), Alphaproteobacteria (21.84–50.74%) was the most abundant class in AS, BS, and CS. Gammaproteobacteria (7.53–39.17%) was the second most abundant in AS and CS, but ranked third in BS. Chloroflexia (13.16–16.87%) was the second most common phylum in BS. In Figure 1C, Rhodobacterales (28.88–36.76%), Caldilineales (2.14–7.84%), and Rhizobiales (3.14–5.32%) were the top three most abundant order in AS. Thermomicrobiales (13.14–16.85%), Rhizobiales (11.16–13.91%), and Rhodobacterales (9.92–10.78%) were the most abundant order in BS. Rhodobacterales (6.49–21.55%), Rhizobiales (9.89–17.25%), and Cardiobacteriales (0.96–26.89%) were the most abundant orders in CS. Rhodobacteraceae (6.49–36.76%) was the most abundant family in AS, BS, and CS (Figure 1D). When the OTUs were considered at the genus level, a high diversity of microbes was identified. A total of 457 genera were detected in all the samples. In BS and CS, the genus level accounting for the largest proportion was Ruegeria (2.23–6.31%) and Marivita (14.01–20.94%) in AS (Figure 1E). The top 10 dominant genera were Marivita, Ruegeria, Photobacterium, unidentified_Bacteria, Phaeodactylibacter, Vibrio, Ilumatobacter, Candidatus_Competibacter, Methyloceanibacter, and unidentified_Nitrospiraceae.
LEfSe analysis was used to reveal specific taxa associated with each sample. As shown in Figure 2A, LEfSe analysis was conducted between AS, BS, and CS (Figure 2). AS relevant taxa were Bacteroidetes, Proteobacteria, Bacteroidia, Anaerolineae, Alphaproteobacteria, Phaeodactylibacter, and Marivita. BS relevant taxa were Chloroflexi, Thaumarchaeota, Actinobacteria, Chloroflexia, Nitrososphaeria, Gammaproteobacteria, and Acidimicrobiia. CS relevant taxa were Firmicutes, unidentified_Bacteria, Gammaproteobacteria, Clostridia, and Photobacterium. Orders and family were differentially abundant among samples as shown in Figures 2A–D.
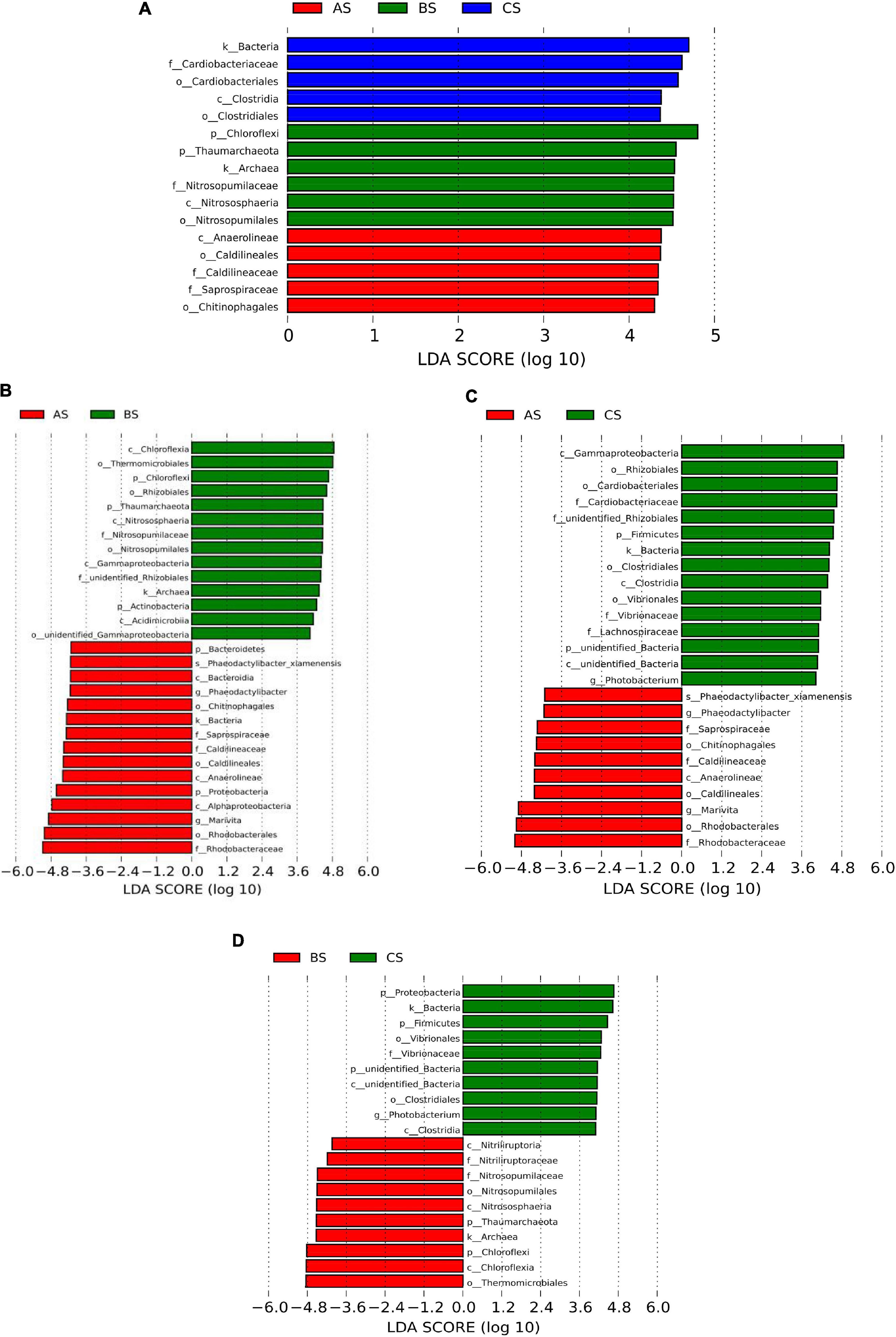
Figure 2. LEfSe analysis among AS vs. BS vs. CS (A), AS vs. BS (B), AS vs. CS (C), BS vs. CS (D). Only taxa with a LDA value higher than 4 are shown. AS, water sample; BS, biofloc sample; CS, intestinal sample.
The OTUs results were obtained according to the clustering. Figure 3A shows the common and unique OTUs among different groups. The percent of OTUs shared by AS and BS, AS and CS, and BS and CS were 57.99, 61.60, and 59.23%, respectively, implying AS and CS shared more OTUs. Simultaneously, the nonmetric multidimensional scale analysis (NMDS) analysis (Figure 3B) demonstrated that samples were classified into three large groups. In terms of potential pathogenic Vibrio, there were six unique to AS, five unique to BS, and six unique to CS. The total shared number of Vibrio species in the three groups was 37.
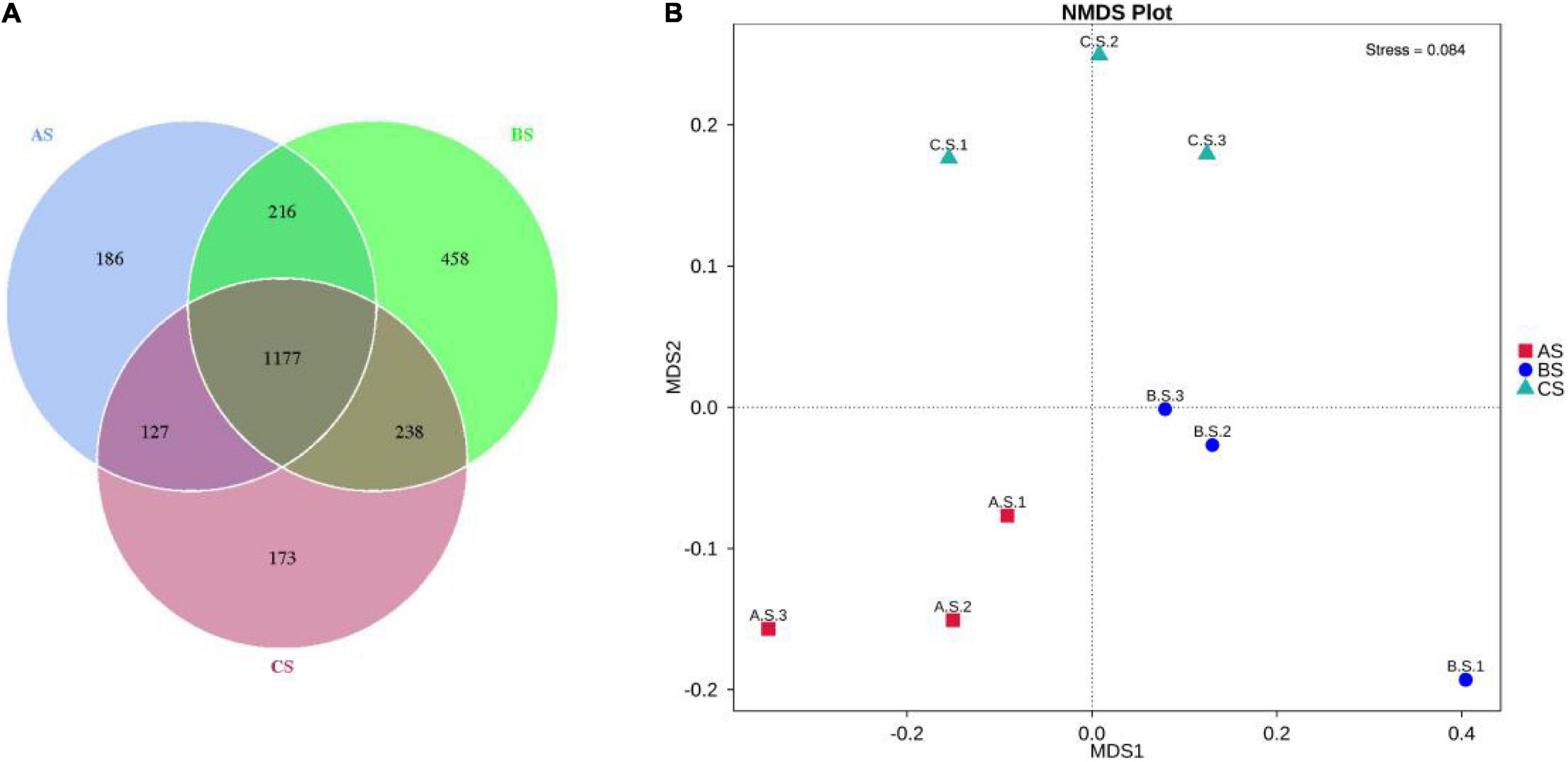
Figure 3. Venn diagram of microbial communities at OTUs level among AS, BS, and CS (A), NMDS analysis (B) among microbial communities (n = 9). AS, water sample; BS, biofloc sample; CS, intestinal sample, OTUs, operational taxanomic units.
Function Annotation Profiles of Microbiota in Shrimp Biofloc Ponds
DIAMOND software was used to compare the functional differences of the microbiota between shrimp intestines, water, and biofloc (Figures 4A–F). The relative abundance of functional pathways based on the KEGG annotation is shown in Figure 4. The functional pathways found to have higher relative abundance were mainly related to metabolic functions (especially amino acids and carbohydrates). Shrimp intestinal microbiota was found to have distinct functional pathways from the microbial communities in water and biofloc. The intestinal microbiota of shrimp was found to have different functional pathways from the microbial communities in water and biofloc. It is worth noting that the antimicrobial function in the predictive KEGG resistance pathway was found to be the most abundant and is lower in CS than AS and BS. The same was found to best true for infectious bacteria, which suggests that there is a link between these two pathways (Figure 4D).
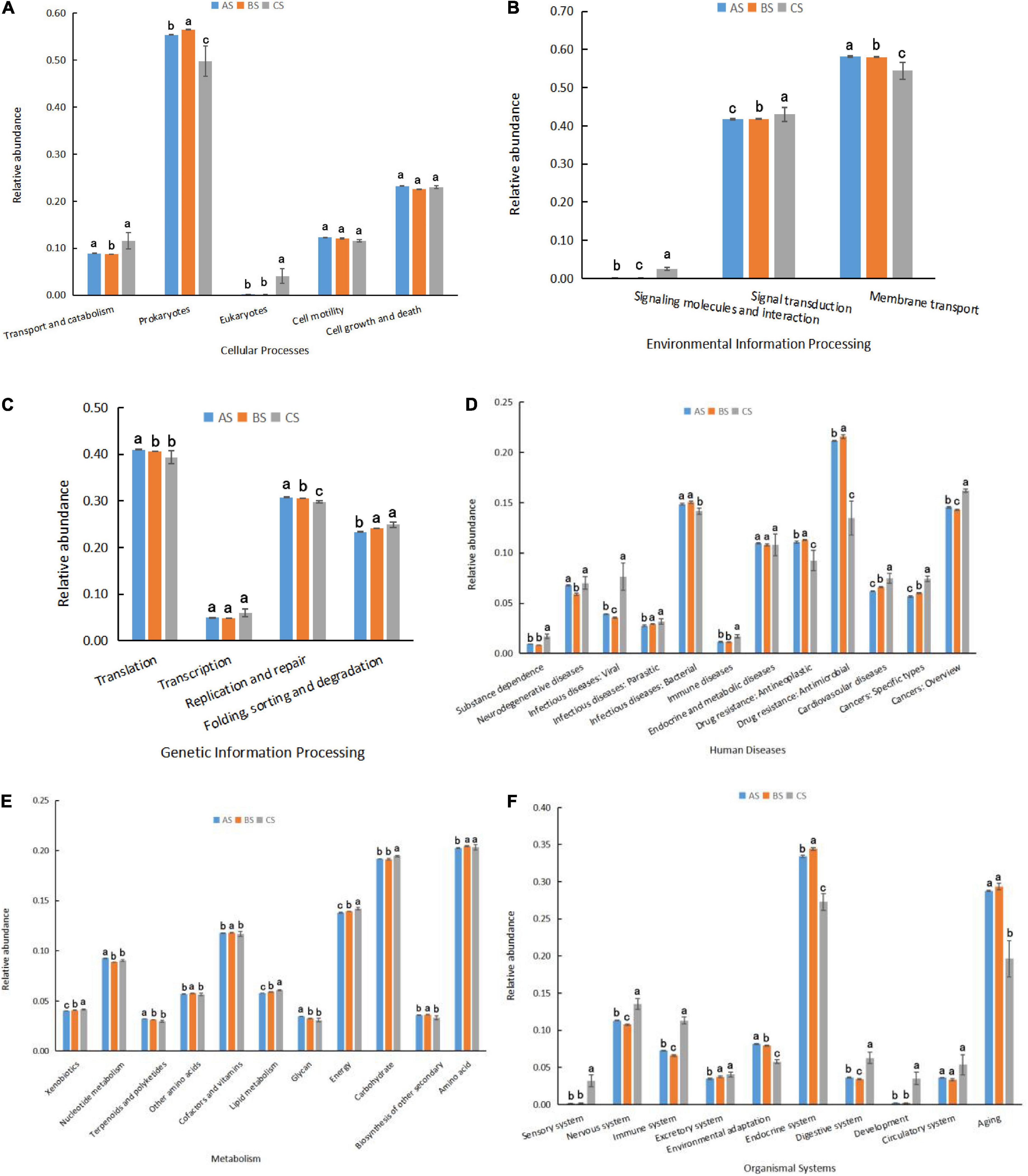
Figure 4. (A–F) Predicted functional pathways for microbiotas in shrimp rearing water (AS), biofloc (BS), and intestine (CS). Means with different superscripts are significantly different (p < 0.05) within each functional pathway. AS, water sample; BS, biofloc sample; CS, intestinal sample.
Resistance Gene Annotation of Microbiota in Shrimp Biofloc Ponds
Bacterial ARO annotation results showed that ARO species of AS, BS, and CS were significantly different (Figure 5A). In this study, the relative abundance of total ARGs of AS, BS, and CS was 3.76–3.92 × 10–4 ppm, 2.46–2.77 × 10–4 ppm, and 4.93–5.82 × 10–4 ppm, respectively. Among all the samples, the abundance of ARGs found in the intestine was the highest.
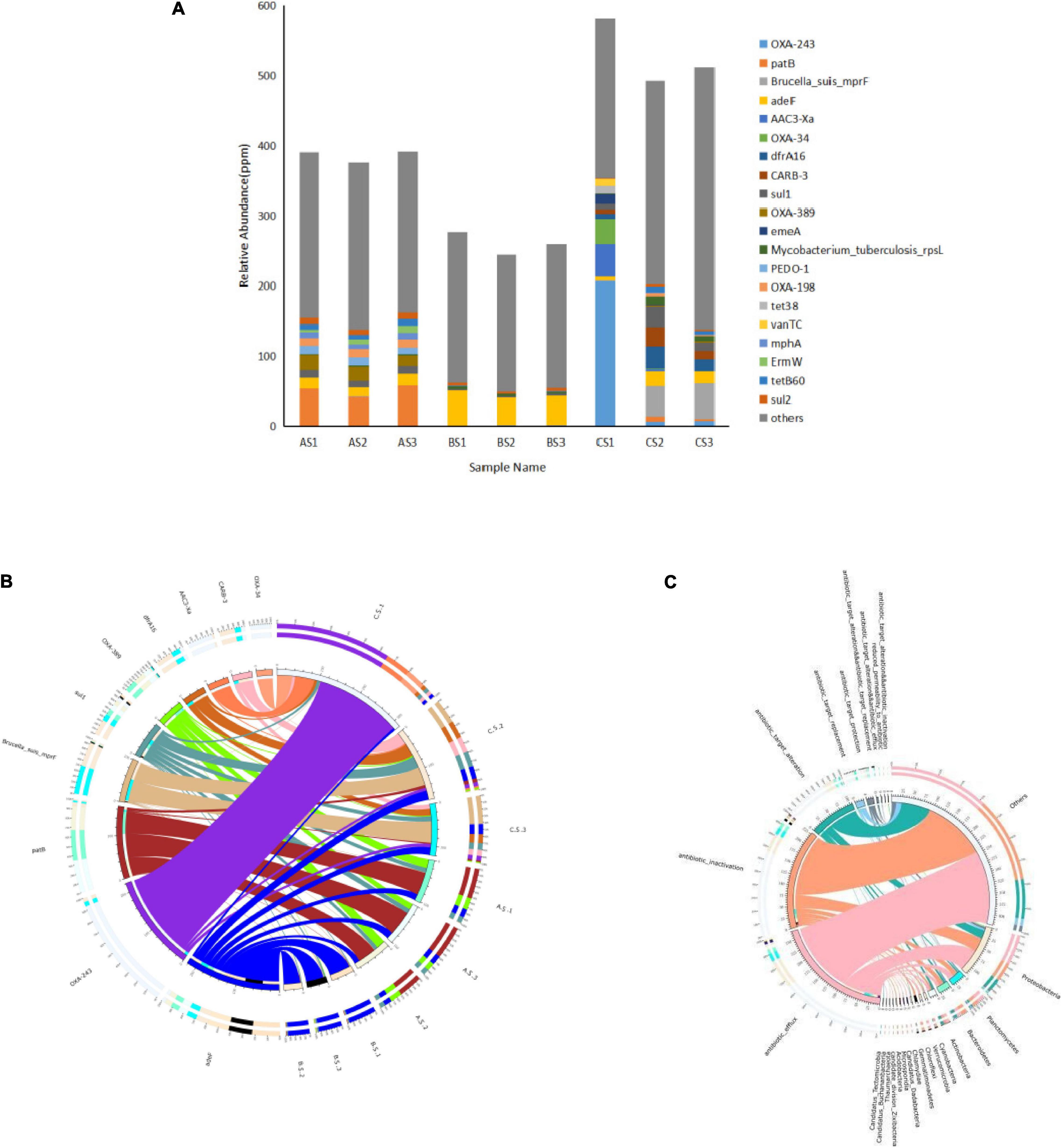
Figure 5. (A–C) Figure 5A represents the relative abundance of all the genes in each sample (unit ppm, the result of magnifying the original relative abundance data by 106 times). Figure 5B represents the ARO with the maximum abundance of top 10 (the circle diagram is divided into two parts, the sample information on the right and ARO information on the left). Different colors in the inner circle represent different samples and AROs, with the scale being relative abundance and the unit being PPM. Figure 5C represents an overview of resistance mechanisms and species (the circle diagram is divided into two parts). The right side shows the information of species at the level of phylum and the left side shows the information on resistance mechanism. The inner circle shows the resistance mechanism of different species and resistance with different colors and the scale shows the number of genes. On the left is the sum of the number of resistance genes in the species with this resistance mechanism and on the right is the sum of the number of resistance genes in the species with different resistance mechanisms. AS, water sample; BS, biofloc sample; CS, intestinal sample.
As shown in Figure 5B, in AS, the ARO with the highest absolute abundance is patB (11.33–15.01%). In BS, the ARO with the highest absolute abundance is adeF (15.79–18.16%), while in CS, the AROs with the highest absolute abundance are OXA-243 (35.65%) and Brucella_suis_mprF (10.03%). The most resistance mechanisms found were related to antibiotic efflux, with 270 resistance genes, followed by 229 resistance genes for the inactivation of antibiotics. Proteobacteria was found to have 118 resistance genes (Figure 5C).
The antibiotic resistance mechanisms of the ARGs detected in the testing samples included efflux proteins, β-lactamase, resistance nodulation division (RND) transporters, reductase, and defensin. The bacterial ARO in AS was mainly derived from Proteobacteria (31%), Bacteroidetes (14%), and Actinobacteria (6%). The bacterial ARO in BS was mainly derived from Proteobacteria (39%), Bacteroidetes (10%), and Planctomycetes (7%). The bacterial ARO in CS was mainly derived from Proteobacteria (39%), Planctomycetes (6%), and Actinobacteria (6%) (Figure 6).

Figure 6. Species identification analysis of AROs in AS, BS, and CS (the inner circle is the species distribution of ARO and the outer circle is the species distribution of all the genes in the group). AS, water sample; BS, biofloc sample; CS, intestinal sample.
Discussion
In this study, high-throughput sequencing and metagenomic analysis were used to investigate the diversity of bacterial communities and ARGs in three P. monodon biofloc ponds in Guangdong Province, China. Metagenomic analysis is a culture-independent molecular approach, which uses the generated metagenomic sequences to search, annotate, and predict targeted genes (Soucy et al., 2015). A total of 45 phyla and 457 genera were identified. Our results revealed that biofloc has the highest alpha diversity metric as shown by the Shannon index, consistent with the levels in Fenneropenaeus merguiensis (banana shrimp) cultured using biofloc technology (Wang et al., 2020). Proteobacteria was the predominant phylum in all the water, biofloc, and intestinal samples, consistent with the levels in environmental samples collected from Marsupenaeus japonicus (Japanese tiger prawn) ponds (Alexia et al., 2020), freshwater crab aquaculture environments (Fang et al., 2019), co-cultured oyster, and Penaeus vannamei (P. vannamei) (whiteleg shrimp) (Wang J. et al., 2019) and P. vannamei infected with white spot syndrome virus (Zeng et al., 2020). Besides Proteobacteria, other phyla such as Bacteroidetes, Chloroflexi, Firmicutes, and Actinobacteria were also abundant in the P. monodon biofloc aquaculture environment and the bacterial taxa showed more abundant species diversity compared to other aquaculture environments (Wobus et al., 2003; Gilbert et al., 2012; Tang et al., 2014). In human health, Na-Ri et al. (2015) proposed Proteobacteria as a potential diagnostic feature for ecological disorders and increased risk of disease. It is worth noting that Proteobacteria is the dominant phylum in crab and fish aquaculture farms and different aquaculture models (Colombo et al., 2016). These data reinforce the importance of carefully treating aquaculture wastewater before discharge into the environment to reduce the transfer of Proteobacteria to humans. In addition, Proteobacteria species richness was not the determinant of microbial community distribution in the pond (Liu et al., 2020; Shen et al., 2020). According to this study, microbial taxa might be the potential hosts of ARGs (Chen et al., 2017). Therefore, Proteobacteria may be a potential host for ARGs in biofloc-based aquaculture environments.
Resistance genes are ubiquitous in both the human gut microbes and other environmental microbes and infections caused by antibiotic-resistant bacteria are a major threat to public health (Ross et al., 2020). Aquaculture is known to be a reservoir of antibiotics and ARG (Syeda et al., 2020). As a new aquaculture model, it is necessary to evaluate the safety and environmental impact of biofloc-based aquaculture models by analyzing the ARGs within them. The successful application of metagenomics in environmental pollution tracking paves the way for the establishment of a new accurate source tracking framework, which greatly simplifies the ARGs variant-based source tracking process (Li et al., 2020). The relative abundance of total ARGs in aquaculture systems based on biofloc was about 2.46–5.82 × 10–4 ppm, which was similar to that of coastal industrial mariculture (0.27–4.55 × 10–4 ppm) (Wang et al., 2018). In this study, the relative abundance of total ARGs of water, biofloc, and intestinal was 3.76–3.92 × 10–4 ppm, 2.46–2.77 × 10–4 ppm, and 4.93–5.82 × 10–4 ppm, respectively. The relative abundances of ARGs were highest in the intestinal samples, which indicated that the shrimp intestines might be a more favorable habitat for aquaculture bacteria carrying ARGs (Su et al., 2018). The biofloc samples had the highest numbers in terms of species richness of bacteria; however, the bacteria in bioflocs carried fewer ARGs. Therefore, biofloc may be the key to controlling the concentration of ARGs in biofloc-based aquaculture. A total of 483 ARGs were identified in the biofloc-based aquaculture ponds in this study. This result was significantly lower than urban hospital and adjacent urban and suburban communities, which had 643 ARGs in airborne samples (He et al., 2020). However, the number of ARGs in biofloc-based aquaculture ponds was higher than that found in the river reservoir system (N24°210–25°060, E115°000–115°470) used for drinking water (242 ARGs) (Chen et al., 2019) and shrimp ponds in Maoming Guangdong Province (217 ARGs) (Zhou et al., 2019).
In this study, quinolone resistance gene patB was the most abundant ARGs in water, tetracycline resistance gene adeF was the most abundant ARGs in biofloc, and cephalosporin resistance gene OXA-243 and peptide resistance gene Brucella_suis_mprF were the most abundant ARGs in intestines, which contrast with the findings of some previous studies (Gao et al., 2012; Huang et al., 2017; Su et al., 2017). It has been shown that the ARGs carried by culturable bacteria are different during shrimp production in aquatic environments (Zhou et al., 2020). In natural water sediment (from the Pearl River), peptide resistance gene bacA, tetracycline resistance gene tet39, and sulfonamide resistance gene sul1 were the most abundant (Zhang et al., 2018).
Antibiotic resistance genes also have the characteristics of “propagation and persistence” (Mao et al., 2015). The transmission of the ARGs as a genetic element can be mediated by genetic mechanisms such as conjugation, transduction, and transformation (Soucy et al., 2015; Ma et al., 2017; Zhao, 2021). In particular, the ARGs in aquaculture environments can be transferred horizontally among microbes and ultimately to fish pathogens (Shah et al., 2012). In addition, the ARGs can also be discharged into the aquatic environment by livestock, agricultural, or aquaculture wastes via natural food chains (Liu et al., 2019). This poses a serious threat to human health, e.g., prolonged morbidity and increased mortality (Ashbolt, 2013; Chamosa et al., 2017). In most circumstances (even without the presence of excess inducers such as antibiotics or heavy metals), plasmids (Hall et al., 2017) and mobile genetic elements (MGEs) (e.g., class 1 integrons) commonly exist in aquatic environments (Gaze et al., 2011), which may cause dissemination of the ARGs in aquatic environment without the presence of antibiotics (Wang Y. et al., 2019). Under the condition of zero exchange water and no antibiotic addition in biofloc-based aquaculture ponds, the ARGs are likely to be transmitted through plasmids and MGEs. Resistant bacteria and genes when entering a healthy organism tend to proliferate, therefore forming a resistance module against the antibiotic within the organisms (Singer et al., 2016). The results of this study and previous studies on biofloc-based aquaculture systems indicate that the system can effectively reduce emissions of the ARGs to the coastal environment due to its low sewage discharge. But, once it is necessary to discharge aquaculture wastewater, more advanced wastewater treatment systems are needed.
Conclusion
In this study, the bacterial composition of water, biofloc, and shrimp intestine sampled from biofloc-based aquaculture ponds was found to be different. Species abundance of biofloc was the highest by combining various indexes. The common dominant phyla were Proteobacteria, Bacteroidetes, Chloroflexi, Firmicutes, and Actinobacteria. Overall, our results suggest that biofloc-based aquaculture is rich in opportunistic pathogen-associated taxonomic groups that may host ARGs associated with critically important antibiotics used in human medicine.
Data Availability Statement
The datasets presented in this study can be found in online repositories. The names of the repository/repositories and accession number(s) can be found below: NCBI (accession: PRJNA758114).
Author Contributions
XC, ZH, JZ, ML, YX, JZ, RH, SM, NW, HP, and CS researched data for the article, made substantial contributions to discussions of the content, wrote the article and reviewed and edited the manuscript before submission. All authors contributed to the article and approved the submitted version.
Funding
This study was supported by the key research and development projects in Guangdong Province (Grant No. 2020B0202010009), the Project of 2019 Annual Guangdong Provincial Special Financial Fund (Grant No. 2319412525), the Fangchenggang Science and Technology Plan Project (Grant No. AD19008017), the National Natural Science Foundation of China (Grant No. 32073015), and the Natural Science Foundation of Guangdong Province (Grant No. 2021A1515011078).
Conflict of Interest
The authors declare that the research was conducted in the absence of any commercial or financial relationships that could be construed as a potential conflict of interest.
Publisher’s Note
All claims expressed in this article are solely those of the authors and do not necessarily represent those of their affiliated organizations, or those of the publisher, the editors and the reviewers. Any product that may be evaluated in this article, or claim that may be made by its manufacturer, is not guaranteed or endorsed by the publisher.
Footnotes
- ^ http://www.drive5.com/muscle/
- ^ https://github.com/cjfields/readfq
- ^ http://bowtie-bio.sourceforge.net/bowtie2/index.shtml
- ^ https://card.mcmaster.ca/
References
Alexia, O., Regina, E. G., Eduardo, Q. G., Cristina, E. F., Roberto, H. H., and Alberto, P. R. (2020). Digestive microbiota of shrimp Penaeus vannamei and oyster Crassostrea gigas co-cultured in integrated multitrophic aquaculture system. Aquaculture 521:735059. doi: 10.1016/j.aquaculture.2020.735059
Ashbolt, N. J. (2013). Human health risk assessment (HHRA) for environmental development and transfer of antibiotic resistance. Environ. Health Perspect. 121, 993–1002. doi: 10.1289/ehp.1206316
Bokulich, N. A., Subramanian, S., Faith, J. J., Gevers, D., Gordon, J. I., Knight, R., et al. (2013). Quality-filtering vastly improves diversity estimates from Illumina amplicon sequencing. Nat. Methods. 10, 57–59. doi: 10.1038/nmeth.2276
Brian, J. H., Dirk, G., Ashlee, M. E., Mike, F., Doyle, V. W., Georgia, G., et al. (2011). Chimeric 16S rRNA sequence formation and detection in Sanger and 454-pyrosequenced PCR amplicons. Genome Res. 21, 494–504. doi: 10.1101/gr.112730.110
Buchfink, B., Xie, C., and Huson, D. H. (2015). Fast and sensitive protein alignment using DIAMOND. Nat. Methods 12, 59–60. doi: 10.1038/nmeth.3176
Caporaso, J. G., Kuczynski, J., Stombaugh, J., Bittinger, K., Bushman, F. D., Costello, E. K., et al. (2010). QIIME allows analysis of high-throughput community sequencing data. Nat. Methods 7, 335–336. doi: 10.1038/nmeth.f.303
Chamosa, L. S., Álvarez, V. E., Nardelli, M., Quiroga, M. P., Cassini, M. H., and Centrón, D. (2017). Lateral antimicrobial resistance genetic transfer is active in the open environment. Sci. Rep. 7:513. doi: 10.1038/s41598-017-00600-2
Chen, C. Q., Zheng, L., Zhou, J. L., and Zhao, H. (2017). Persistence and risk of antibiotic residues and antibiotic resistance genes in major mariculture sites in Southeast China. Sci. Total Environ. 580, 1175–1184. doi: 10.1016/j.scitotenv.2016.12.075
Chen, Y. H., Su, J. Q., Zhang, J. Y., Li, P., Chen, H. J., Zhang, B., et al. (2019). High-throughput profiling of antibiotic resistance gene dynamic in a drinking water river-reservoir system. Water Res. 149, 179–189. doi: 10.1016/j.watres.2018.11.007
Colombo, S., Arioli, S., Guglielmetti, S., Lunelli, F., and Mora, D. (2016). Virome associated antibiotic-resistance genes in an experimental aquaculture facility. FEMS Microbiol. Ecol. 92:fiw003. doi: 10.1093/femsec/fiw003
Corey, C. H., David, B., Grant, D. S., and Mark, V. G. (2020). Understanding the role of the shrimp gut microbiome in health and disease. J. Invertebr. Pathol. 186:107387. doi: 10.1016/j.jip.2020.107387
Dang, B., Mao, D., Xu, Y., and Luo, Y. (2017). Conjugative multiresistant plasmids in Haihe River and their impacts on the abundance and spatial distribution of antibiotic resistance genes. Water Res. 111, 81–91. doi: 10.1016/j.watres.2016.12.046
Daniel, H. H., Suparna, M., Hans-Joachim, R., Nico, W., and Stephan, C. S. (2011). Integrative analysis of environmental sequences using MEGAN4. Genome Res. 21, 1552–1560. doi: 10.1101/gr.120618.111
Edgar, R. C. (2004). MUSCLE: multiple sequence alignment with high accuracy and high throughput. Nucleic Acids Res. 32, 1792–1797. doi: 10.1093/nar/gkh340
Edgar, R. C. (2013). UPARSE: highly accurate OTU sequences from microbial amplicon reads. Nat. Methods 10, 996–998. doi: 10.1038/nmeth.2604
Fang, H., Huang, K. L., Yu, N. J., Ding, C. C., Wang, Z. F., and Zhao, C. (2019). Metagenomic analysis of bacterial communities and antibiotic resistance genes in the Eriocheir sinensis freshwater aquaculture environment. Chemosphere 224, 202–211. doi: 10.1016/j.chemosphere.2019.02.068
FAO (2020). The State of World Fisheries and Aquaculture: Sustainable Development in Action. Rome: FAO.
Flegel, T. W. (2012). Historic emergence, impact and current status of shrimp pathogens in Asia. J. Invertebr. Pathol. 110, 166–173. doi: 10.1016/j.jip.2012.03.004
Fredrik, H. K., Frida, F., Intawat, N., Valentina, T., Björn, F., Dina, P., et al. (2012). Symptomatic atherosclerosis is associated with an altered gut metagenome. Nat. Commun. 3:1245.
Gao, P., Mao, D., Luo, Y., Wang, L., Xu, B., and Xu, L. (2012). Occurrence of sulfonamide and tetracycline-resistant bacteria and resistance genes in aquaculture environment. Water Res. 46, 2355–2364. doi: 10.1016/j.watres.2012.02.004
Gaze, W. H., Zhang, L., Abdouslam, N. A., Hawkey, P. M., Calvo-Bado, L., Royle, J., et al. (2011). Impacts of anthropogenic activity on the ecology of class 1 integrons and integron-associated genes in the environment. ISME J. 5, 1253–1261. doi: 10.1038/ismej.2011.15
Gilbert, J. A., Steele, J. A., Caporaso, J. G., Steinbrück, L., Reeder, J., Temperton, B., et al. (2012). Defining seasonal marine microbial community dynamics. ISME J. 6, 98–308. doi: 10.1038/ismej.2011.107
Hall, J. P. J., Williams, D., Paterson, S., Harrison, E., and Brockhurst, M. A. (2017). Positive selection inhibits gene mobilization and transfer in soil bacterial communities. Nat. Ecol. Evol. 1, 1348–1353. doi: 10.1038/s41559-017-0250-3
He, P., Wu, Y., Huang, W. Z., Wu, X. W., Lv, J. Y., Liu, P. D., et al. (2020). Characteristics of and variation in airborne ARGs among urban hospitals and adjacent urban and suburban communities: a metagenomic approach. Environ. Int. 139:105625. doi: 10.1016/j.envint.2020.105625
Huang, L., Xu, Y. B., Xu, J. X., Ling, J. Y., Chen, J. L., Zhou, J. L., et al. (2017). Antibiotic resistance genes (ARGs) in duck and fish production ponds with integrated or non-integrated mode. Chemosphere 168, 1107–1114. doi: 10.1016/j.chemosphere.2016.10.096
Huang, Y. C. (2009). Effects of Effective Microorganisms (EM) on bacterial ecology and water quality for shrimp culture. J. Guangdong Ocean Univ. 29, 44–48.
Huang, Z. J., Chen, Y. G., Weng, S. P., Lu, X. F., Zhong, L. H., Fan, W. Z., et al. (2016). Multiple bacteria species were involved in hepatopancreas necrosis syndrome (HPNS) of Litopenaeus vannamei. Acta Sci. Nat. Univ. SunYatseni 55, 1–11.
Jia, B. F., Raphenya, A. R., Alcock, B., Waglechner, N., Guo, P. Y., Tsang, K. K., et al. (2017). CARD 2017: expansion and model-centric curation of the comprehensive antibiotic resistance database. Nucleic Acids Res. 45(D1):D566. doi: 10.1093/nar/gkw1004
Julia, O., Allyson, L. B., Clay, D., Sean, C., Betty, B., Robert, B., et al. (2014). Biogeography and individuality shape function in the human skin metagenome. Nature 514, 59–64. doi: 10.1038/nature13786
Julie, E., Muhammad, H. A., Enang, H. S., Sir, N., Peter, D. S., and Peter, B. (2014). Immune response and disease resistance of shrimp fed biofloc grown on different carbon sources. Fish Shellfish Immunol. 41, 332–339. doi: 10.1016/j.fsi.2014.09.004
Kanehisa, M., Goto, S., Hattori, M., Aoki-Kinoshita, K. F., Itoh, M., Kawashima, S., et al. (2006). From genomics to chemical genomics: new developments in KEGG. Nucleic Acids Res. 34(Database issue), 354–357. doi: 10.1093/nar/gkj102
Kanehisa, M., Goto, S., Sato, Y., Kawashima, M., Furumichi, M., and Tanabe, M. (2014). Data, information, knowledge and principle: back to metabolism in KEGG. Nucleic Acids Res. 42, 199–205. doi: 10.1093/nar/gkt1076
Karlsson, F. H., Fåk, F., Nookaew, I., Tremaroli, V., Fagerberg, B., Petranovic, D., et al. (2012). Symptomatic atherosclerosis is associated with an altered gut metagenome. Nat. Commun. 3:1245. doi: 10.1038/ncomms2266
Karlsson, F. H., Tremaroli, V., Nookaew, I., Bergström, G., Behre, C. J., Fagerberg, B., et al. (2013). Gut metagenome in European women with normal, impaired and diabetic glucose control. Nature 498, 99–103. doi: 10.1038/nature12198
Khalid, Z. M., Sameena, M. L., and Rovidha, S. R. (2021). “Chapter 7 – genomic DNA extraction from the plant leaves using the CTAB method,” in Advanced Methods in Molecular Biology and Biotechnology, eds K. Z. Masoodi, S. M. Lone, and R. S. Rasool (Cambridge, MA: Academic Press), 37–44. doi: 10.1016/B978-0-12-824449-4.00007-4
Kim, M. S., Min, E. Y., Kim, J. H., Koo, J. K., and Kang, J. C. (2015). Growth performance and immunological and antioxidant status of Chinese shrimp, Fennerpenaeus Chinensis reared in biofloc culture system using probiotics. Fish Shellfish Immunol. 47, 141–146. doi: 10.1016/j.fsi.2015.08.027
Lee, C. T., Chen, I. T., Yang, Y. T., Ko, T. P., Huang, Y. T., Huang, J. Y., et al. (2015). The opportunistic marine pathogen Vibrio parahaemolyticus becomes virulent by acquiring a plasmid that expresses a deadly toxin. Proc. Natl. Acad. Sci. U. S. A. 112, 10798–10803. doi: 10.1073/pnas.1503129112
Lei (2006). Environmental Chemistry Experiment in Aquaculture Water. Beijing: China Agriculture Press 5-6, 56–70.
Li, L. G., Huang, Q., Yin, X. L., and Zhang, T. (2020). Source tracking of antibiotic resistance genes in the environment: challenges, progress, and prospects. Water Res. 185, 116–127. doi: 10.1016/j.watres.2020.116127
Liu, X., Zhang, G., Liu, Y., Lu, S., Qin, P., Guo, X., et al. (2019). Occurrence and fate of antibiotics and antibiotic resistance genes in typical urban water of Beijing, China. Environ. Pollut. 246, 163–173. doi: 10.1016/j.envpol.2018.12.005
Liu, Z. G., Iqbal, M., Zeng, Z. B., Lian, Y. X., Zheng, A. F., Zhao, M. M., et al. (2020). Comparative analysis of microbial community structure in the ponds with different aquaculture model and fish by high-throughput sequencing. Microb. Pathog. 142:104101. doi: 10.1016/j.micpath.2020.104101
Luo, R. B., Liu, B. H., Xie, Y. L., Li, Z. Y., Huang, W. H., Yuan, J. Y., et al. (2015). Erratum: SOAPdenovo2: an empirically improved memory-efficient short-read de novo assembler. Gigascience 1:18. doi: 10.1186/s13742-015-0069-2
Ma, L. P., Li, A. D., Yin, X. L., and Zhang, T. (2017). The prevalence of integrons as the carrier of antibiotic resistance genes in natural and man-made environments. Environ. Sci. Technol. 51, 5721–5728. doi: 10.1021/acs.est.6b05887
Magoč, T., and Salzberg, S. L. (2011). FLASH: fast length adjustment of short reads to improve genome assemblies. Bioinformatics 27, 2957–2963. doi: 10.1093/bioinformatics/btr507
Mao, D., Yu, S., Rysz, M., Luo, Y., Yang, F., Li, F., et al. (2015). Prevalence and proliferation of antibiotic resistance genes in two municipal wastewater treatment plants. Water Res. 85, 458–466. doi: 10.1016/j.watres.2015.09.010
Martínez, J. L., Coque, T. M., and Baquero, F. (2014). What is a resistance gene? Ranking risk in resistomes. Nat. Rev. Microbiol. 13, 116–123. doi: 10.1038/nrmicro3399
Mcarthur, A. G., Waglechner, N., and Nizam, F. (2013). The comprehensive antibiotic resistance database. Antimicrob. Agents Chemother. 57:3348. doi: 10.1128/AAC.00419-13
Mende, D. R., Waller, A. S., Sunagawa, S., Järvelin, A. I., Chan, M. M., Arumugam, M., et al. (2012). Assessment of metagenomic assembly using simulated next generation sequencing data. PLoS One 7:31386. doi: 10.1371/journal.pone.0031386
Mishra, J. K., Samocha, T. M., Patnaik, S., Speed, M., Ryan, L. G., and Abdul, M. A. (2008). Performance of an intensive nursery system for the Pacific white shrimp, Litopenaeus vannamei, under limited discharge condition. Aquac. Eng. 38, 2–15. doi: 10.1016/j.aquaeng.2007.10.003
Na-Ri, S., Tae, W. W., and Jin-Woo, B. (2015). Proteobacteria: microbial signature of dysbiosis in gut microbiota. Trends Biotechnol. 33, 496–503. doi: 10.1016/j.tibtech.2015.06.011
Nicola, S., Jacques, I., Levi, W., Dirk, G., Larisa, M., Wendy, S. G., et al. (2011). Metagenomic biomarker discovery and explanation. Genome Biol. 12, 1–18. doi: 10.1186/gb-2011-12-6-r60
Nielsen, H. B., Almeida, M., Juncker, A. S., Rasmussen, S., Li, J., Sunagawa, S., et al. (2014). Identification and assembly of genomes and genetic elements in complex metagenomic samples without using reference genomes. Nat. Biotechnol. 32, 822–828. doi: 10.1038/nbt.2939
Pruden, A. (2014). Balancing water sustainability and public health goals in the face of growing concerns about antibiotic resistance. Environ. Sci. Technol. 48, 5–14. doi: 10.1021/es403883p
Pruden, A., Pei, R., Storteboom, H., and Carlson, K. H. (2006). Antibiotic resistance genes as emerging contaminants: studies in northern Colorado. Environ. Sci. Technol. 40, 7445–7450. doi: 10.1021/es060413l
Qin, J. J., Li, R. Q., Raes, J., Arumugam, M., Burgdorf, K. S., Manichanh, C., et al. (2010). A human gut microbial gene catalogue established by metagenomic sequencing. Nature 464, 59–65. doi: 10.1038/nature08821
Qin, N., Yang, F., Li, A., Prifti, E., Chen, Y., Shao, L., et al. (2014). Alterations of the human gut microbiome in liver cirrhosis. Nature 513, 59–64. doi: 10.1038/nature13568
Quast, C., Pruesse, E., Yilmaz, P., Gerken, J., Schweer, T., Yarza, P., et al. (2013). The SILVA ribosomal RNA gene database project: improved data processing and web-based tools. Nucl. Acids Res. 590–596. doi: 10.1093/nar/gks1219
Robert, C. E., Brian, J. H., Jose, C. C., Christopher, Q., and Rob, K. (2011). UCHIME improves sensitivity and speed of chimera detection. Bioinformatics 27, 2194–2200. doi: 10.1093/bioinformatics/btr381
Ross, S. M., Gregory, E. M., Lisa, E. L., and Willem, V. S. (2020). Horizontal transfer of antibiotic resistance genes in the human gut microbiome. Curr. Opin. Microbiol. 53, 35–43. doi: 10.1016/j.mib.2020.02.002
Shah, S. Q. A., Colquhoun, D. J., Nikuli, H. L., and Sørum, H. (2012). Prevalence of antibiotic resistance genes in the bacterial flora of integrated fish farming environments of Pakistan and Tanzania. Environ. Sci. Technol. 46, 8672–8679. doi: 10.1021/es3018607
Shen, X. X., Xu, M., Li, M., Zhao, Y. J., and Shao, X. H. (2020). Response of sediment bacterial communities to the drainage of wastewater from aquaculture ponds in different seasons. Sci. Total Environ. 717:137180.
Shlrene, Q., Asha, E., Ravindran, B., Kaizar, H., Naif, A. A., Mariadhas, V. A., et al. (2020). Veterinary antibiotics in animal manure and manure laden soil: scenario and challenges in Asian countries. J. King Saud Univ. Sci. 32, 1300–1305. doi: 10.1016/j.jksus.2019.11.015
Singer, A. C., Shaw, H., Rhodes, V., and Hart, A. (2016). Review of antimicrobial resistance in the environment and its relevance to environmental regulators. Front. Microbiol. 7:1728. doi: 10.3389/fmicb.2016.01728
Soucy, S. M., Huang, J., and Gogarten, J. P. (2015). Horizontal gene transfer: building the web of life. Nat. Rev. Genet. 16, 472–482. doi: 10.1038/nrg3962
Stéphane, H., Ruben, G. O., Antonio, G., Stijn, S., Gail, A., Sarah, L., et al. (2015). Microbiota and host nutrition across plant and animal kingdoms. Cell Host Microbe 603–616. doi: 10.1016/j.chom.2015.04.009
Su, H., Hu, X., Xu, Y., Xu, W., Huang, X., Wen, G., et al. (2018). Persistence and spatial variation of antibiotic resistance genes and bacterial populations change in reared shrimp in South China. Environ. Int. 119, 327–333. doi: 10.1016/j.envint.2018.07.007
Su, H. C., Liu, S., Hu, X. J., Xu, X. R., Xu, W. J., Xu, Y., et al. (2017). Occurrence and temporal variation of antibiotic resistance genes (ARGs) in shrimp aquaculture: ARGs dissemination from farming source to reared organisms. Sci. Total Environ. 607-608, 357–366. doi: 10.1016/j.scitotenv.2017.07.040
Sui, Q., Cao, X., Lu, S., Zhao, W., Qiu, Z., and Yu, G. (2016). Occurrence, sources and fate of pharmaceuticals and personal care products in the groundwater: a review. Emerg. Contam. 1, 14–24. doi: 10.1016/j.emcon.2015.07.001
Surapun, T., Intawat, N., Shawn, M. H., Pannita, S., Metavee, P., Kanittha, C., et al. (2020). Metagenomics in bioflocs and their effects on gut microbiome and immune responses in Pacific white shrimp. Fish Shellfish Immunol. 106, 733–741. doi: 10.1016/j.fsi.2020.08.042
Syeda, M. Z., Muhammad, J., Nan, X., and Riffat, N. M. (2020). Antibiotics and antibiotic resistant genes (ARGs) in groundwater: a global review on dissemination, sources, interactions, environmental and human health risks. Water Res. 187:116455. doi: 10.1016/j.watres.2020.116455
Tang, Y. Y., Tao, P. Y., Tan, J. G., Mu, H. Z., Peng, L., Yang, D. D., et al. (2014). Identification of bacterial community composition in freshwater aquaculture system farming of Litopenaeus vannamei reveals distinct temperature-driven patterns. Int. J. Mol. Sci. 15, 13663–13680. doi: 10.3390/ijms150813663
Wang, B., Qin, H. P., Liao, X. Z., Hu, S. K., Zhao, J. C., He, Z. H., et al. (2020). Effects of stocking density on bacterial community characterization of biofloc and intestine of Fenneropenaeus merguiensis. J. Hunan Agric. Univ. (Nat. Sci.) 46, 608–615.
Wang, J., Huang, Y. J., Xu, K. H., Zhang, X. Y., Sun, H. G., Fan, L. F., et al. (2019). White spot syndrome virus (WSSV) infection impacts intestinal microbiota composition and function in Litopenaeus vannamei. Fish Shellfish Immunol. 84, 130–137. doi: 10.1016/j.fsi.2018.09.076
Wang, J. H., Lu, J., Zhang, Y. X., Wu, J., Luo, Y. M., and Liu, H. (2018). Metagenomic analysis of antibiotic resistance genes in coastal industrial mariculture systems. Bioresour. Technol. 253, 235–243. doi: 10.1016/j.biortech.2018.01.035
Wang, Y., Lu, J., Mao, L., Li, J., Yuan, Z., Bond, P. L., et al. (2019). Antiepileptic drug carbamazepine promotes horizontal transfer of plasmid-borne multi-antibiotic resistance genes within and across bacterial genera. ISME J. 13, 509–522. doi: 10.1038/s41396-018-0275-x
Wei, G. S., Shan, D. P., Li, G. Z., Li, X., Tian, R. M., He, J. G., et al. (2020). Prokaryotic communities vary withfloc size in a biofloc technology-based aquaculture system. Aquaculture 529:735632. doi: 10.1016/j.aquaculture.2020.735632
Wilson, W. Jr., Heidi, A., Al, S., and Craig, L. B. (2006). Effect of natural production in a zero-exchange suspended microbial floc based super-intensive culture system for white shrimp Litopenaeus vannamei. Aquaculture 258, 396–403. doi: 10.1016/j.aquaculture.2006.04.030
Wobus, A., Bleul, C., Maassen, S., Scheerer, C., Schuppler, M., Jacobs, E., et al. (2003). Microbial diversity and functional characterization of sediments from reservoirs of different trophic state. FEMS Microbiol. Ecol. 46, 331–347. doi: 10.1016/S0168-6496(03)00249-6
Yoram, A. (1999). Carbon/nitrogen ratio as a control element in aquaculture systems. Aquaculture 176, 227–235. doi: 10.1016/S0044-8486(99)00085-X
Yoram, A. (2007). Feeding with microbial flocs by tilapia in minimal discharge biofloc technology ponds. Aquaculture 264, 140–147. doi: 10.1016/j.aquaculture.2006.11.025
Yu, Z., Li, L., Zhu, R., Li, M., Duan, J., Wang, J. Y., et al. (2020). Monitoring of growth, digestive enzyme activity, immune response and water quality parameters of Golden crucian carp (Carassius auratus) in zero water exchange tanks of biofloc systems. Aquac. Rep. 16:100283. doi: 10.1016/j.aqrep.2020.100283
Zeng, C. X., Lin, M., Li, Z. Q., Ma, Y., and Wang, S. H. (2020). The structural and functional characteristics of the gut microbiota of Marsupenaeus japonicus as revealed by 16S rRNA gene amplicon sequencing. Microbiol. China 47, 1857–1866.
Zhang, S. H., Yang, G. L., Hou, S. G., Zhang, T. J., Li, Z. G., and Liang, F. (2018). Distribution of ARGs and MGEs among glacial soil, permafrost, and sediment using metagenomic analysis. Environ. Pollut. 234, 339–346. doi: 10.1016/j.envpol.2017.11.031
Zhao, Z. (2021). Comparison of microbial communities and the antibiotic resistome between prawn mono and poly culture systems. Ecotoxicol. Environ. Saf. 207:111310. doi: 10.1016/j.ecoenv.2020.111310
Zhou, R. J., Zeng, S. Z., Hou, D. W., Liu, J., Weng, S. P., He, J. G., et al. (2019). Occurrence of human pathogenic bacteria carrying antibiotic resistance genes revealed by metagenomic approach: a case study from an aquatic environment. J. Environ. Sci. 8, 248–256. doi: 10.1016/j.jes.2019.01.001
Keywords: metagenomic analysis, antibiotic resistance genes, bacterial community, Penaeus monodon, biofloc
Citation: Chen XY, He ZH, Zhao JC, Liao MZ, Xue Y, Zhou JN, Hoare R, Monaghan SJ, Wang N, Pang HY and Sun CB (2022) Metagenomic Analysis of Bacterial Communities and Antibiotic Resistance Genes in Penaeus monodon Biofloc-Based Aquaculture Environments. Front. Mar. Sci. 8:762345. doi: 10.3389/fmars.2021.762345
Received: 21 August 2021; Accepted: 29 November 2021;
Published: 03 January 2022.
Edited by:
Siu Gin Cheung, City University of Hong Kong, Hong Kong SAR, ChinaReviewed by:
Teresa Maria Coelho Baptista, Polytechnic Institute of Leiria, PortugalAna Teresa Gonçalves, GreenCoLAB, Portugal
Copyright © 2022 Chen, He, Zhao, Liao, Xue, Zhou, Hoare, Monaghan, Wang, Pang and Sun. This is an open-access article distributed under the terms of the Creative Commons Attribution License (CC BY). The use, distribution or reproduction in other forums is permitted, provided the original author(s) and the copyright owner(s) are credited and that the original publication in this journal is cited, in accordance with accepted academic practice. No use, distribution or reproduction is permitted which does not comply with these terms.
*Correspondence: Huanying Pang, cGh5aW5nMTIxOEAxNjMuY29t; Chengbo Sun, c3VuY2JAZ2RvdS5lZHUuY24=