- 1School of Chemistry, University of Auckland, Auckland, New Zealand
- 2Veterinary Faculty, Institute of Preclinical Sciences, University of Ljubljana, Ljubljana, Slovenia
- 3Laboratoire des Sciences de l’Environnement Marin (LEMAR) CNRS, IRD, Ifremer, Université de Bretagne Occidentale, Plouzané, France
- 4Department of Biology, Biotechnical Faculty, University of Ljubljana, Ljubljana, Slovenia
- 5Cawthron Institute, Nelson, New Zealand
The search for effective yet environmentally friendly strategies to prevent marine biofouling is hampered by the large taxonomic diversity amongst fouling organisms and a lack of well-defined conserved molecular targets. The acetylcholinesterase enzyme catalyses the breakdown of the neurotransmitter acetylcholine, and several natural antifouling allelochemicals have been reported to display acetylcholinesterase inhibitory activity. Our study is focussed on establishing if acetylcholinesterase can be used as a well-defined molecular target to accelerate discovery and development of novel antifoulants via sequential high-throughput in silico screening, in vitro enzymatic studies of identified compound libraries, and in vivo assessment of the most promising lead compounds. Using this approach, we identified potent cholinesterase inhibitors with inhibitory concentrations down to 3 μM from a 10,000 compound library. The most potent inhibitors were screened against five microfouling marine bacteria and marine microalgae and the macrofouling tunicate Ciona savignyi. No activity was seen against the microfoulers but a potent novel inhibitor of tunicate settlement and metamorphosis was discovered. Although only one of the identified active cholinesterase inhibitors displayed antifouling activity suggesting the link between cholinesterase inhibition and antifouling is limited to certain compound classes, the study highlights how in silico screening employed regularly for drug discovery can also facilitate discovery of antifouling leads.
Introduction
Marine biofouling organisms rapidly settle and colonise any surface submerged in the sea to form complex mixed biofouling communities that can impair both intended mechanical function and material durability (Vinagre et al., 2020). Effective biofouling management is essential for maintaining function of vessels and fixed infrastructure, with poor antifouling countermeasures having major economic and ecological repercussions (Yebra et al., 2004; Schultz et al., 2011). Historically, surfaces have been protected using antifouling coatings that operate via broad-spectrum biocidal properties. While effective, collateral damage to non-target marine organisms and extended environmental half-lives and sediment accumulation has led to historic and emerging bans on many broad-spectrum antifouling biocides (Yebra et al., 2004; Dafforn et al., 2011). While innovative counter measurements such as iodine bubbles (Dickenson et al., 2017), UV-light (Richard et al., 2021) grooming strategies (Hearin et al., 2015) and functional coatings (Tian et al., 2020; Jin et al., 2021) are being researched, it is clear that more targeted and less toxic alternate antifouling technologies are urgently needed (Vinagre et al., 2020).
A key challenge to developing new antifouling technologies is the large taxonomic diversity implicated in the problem, including both micro- (e.g., bacteria, diatoms) and macro-organisms (e.g., tunicates, barnacles, mussels, seaweeds) (Vinagre et al., 2020). The realisation that a combination of several mechanisms of action may be needed to target the diverse range of organisms involved in biofouling communities has spurred research into innovative coating technologies that exploit strategies evolved by marine and terrestrial organisms to prevent competition, overgrowth, and colonisation by others (Lejars et al., 2012; Flemming, 2020; Maan et al., 2020). Physicochemical and mechanical material properties such as surface energy, hardness, charge, and hydrophobicity can be straightforwardly designed and probed (Lejars et al., 2012; Selim et al., 2020). The development and design of novel environmentally friendly antifouling chemicals is, however, less straightforward to address (Flemming, 2020; Maan et al., 2020).
Settlement repelling compounds, in general, interfere with biochemical signal transduction pathways used by biofouling organisms to select a site to settle and initiate attachment and metamorphosis (Herzberg et al., 2021). The approach is intuitively attractive and there are many examples of natural allelochemicals active against one or a few biofouling taxa (Qian et al., 2009; Moodie et al., 2017a,b; Liu et al., 2020). However, broadly effective settlement repelling compounds have been elusive to date and only a few common structural features stand out as clearly associated with antifouling activity. Brominated modified dipeptic derivatives represent an exception with numerous highly active natural and synthetic antifouling compounds being reported (Sjögren et al., 2004; Hanssen et al., 2014; Trepos et al., 2014; Labriere et al., 2020). One of the underlying reasons to this generally poorly established structure activity relationship is the wide range of fouling organisms involved in biofouling and thus a high number of possible molecular and mechanistic targets which limits the possibility for rational design and screening of compound libraries (Qian et al., 2013; Vinagre et al., 2020). It further highlights how challenging it is to develop a single “silver-bullet” type repelling antifouling compound that is not also a general biocide (Flemming, 2020).
The ability to target well defined receptors and biosynthetic pathways relies on understanding the mode of action, and to rationalise this challenge, initial in silico screening and docking studies of plausible leads is an important part of modern drug discovery and development (Terstappen and Reggiani, 2001; de Souza Neto et al., 2020). While the in silico approach is rarely employed outside of medicinal chemistry, chemical library screening can facilitate effective design of new bioactive compounds in diverse range of end-use scenarios including antifouling. The potential of this strategy is exemplified by the targeted screening of octopamine receptor activators which ultimately led to the development of α2-adrenoceptor agonist medetomidine into the commercial antifouling product Selektope®, (Lind et al., 2010). Selektope®, prevents the settlement of barnacle larvae by stimulating active swimming away from surfaces, illustrating how a targeted screen is applicable for the development novel repelling antifouling technologies (Lind et al., 2010). One bottleneck to applying this approach is a lack of well-defined molecular targets to prevent organism settlement.
Of the known plausible molecular targets for marine antifouling (Qian et al., 2013), several natural antifouling allelochemicals inhibit acetylcholinesterase (AChE) (Moodie et al., 2019), for example polymeric alkylpyridinium salts from the sponge Reniera sarai (Faimali et al., 2003b), barrettin and analogs form the sponge Geodia barrette (Olsen et al., 2016) and secondary metabolites from the marine bacterium Salinispora arenicola (Melo, 2016). Acetlycholine has likewise been demonstrated to play a role in how macrofoulers choose surfaces to settle on and subsequently attach. Inhibiting AChE has been shown in laboratory bioassays to prevent settlement and attachment of the barnacle Balanus Amphitrite (Faimali et al., 2003a; Blihoghe et al., 2011), the blue mussel Mytilus edulis (Dobretsov and Qian, 2003), the bryozoan Bugula neritina (Yu et al., 2007), the ascidian Ciona intestinalis (Mansueto et al., 2012), and the goose barnacle Pollicepes pollicepes (Almeida et al., 2015). These observations suggest that the cholinergic system may modulate certain settling mechanisms, or it could indicate that the cholinesterase enzyme ligands display overlapping structural features with other molecular targets involved in the settlement (Moodie et al., 2019).
To probe the potential link between AChE inhibition and antifouling activity we have undertaken a rational in silico design, screening, and assessment approach for AChE inhibition of marine larvae using 10,000 compounds from the Chembridge screening library. The compounds were virtually screened for binding in an AChE homology model and several selection criteria were employed to produce theoretical binders which were evaluated in vitro as AChE inhibitors. The lead compounds were used to identify structural analogs, and these were rescreened experimentally to determine the binding affinity to AChE. Selected compounds, both potent AChE inhibitors as well as inactive controls, were finally screened in vivo against a set of microfouling bacteria and microalgae as well as against macrofouling tunicate Ciona savignyi. Our study provides a model of how modern computational approaches can be combined with high-throughput screening approaches to accelerate the generation of antifouling leads and how a well-defined molecular target can optimise outcomes.
Materials and Methods
Homology Model
The 3D structure of the target protein was developed using homology modelling. The query sequence was obtained from Crassostrea gigas AChE (XP_011454985.2). A template for this sequence was identified using Basic Local Alignment Search Tool (BLAST) (Altschul et al., 1990). The crystal structure for AChE (PDB ID: 6G1U, 1.79Å, Tetronarce californica) (Galdeano et al., 2018) was selected as the template and had an overall sequence similarity of 45% and an E-value of 7 × 10–166. A sequence alignment was performed using the ClustalW server tool (Larkin et al., 2007). Homology models were generated using MODELLER 9.17 (Fiser and Šali, 2003), the model with the lowest MODELLER objective function was chosen for refinement and further validation. The SciGRESS FJ 2.9 program (Stewart, 2009) was used to prepare the homology model for docking by adding hydrogens, resolving any clashes, correcting missing valences and hydrogen energy minimisation using the MM2 protocol (Allinger, 1977). Validation of the model was conducted using the Ramachandran plot and evaluation from the ProSA web server (Wiederstein and Sippl, 2007).
Virtual Screening
For the first screening run, a library of compounds was prepared using the SciGRESS FJ program using the MM2 force field (Allinger, 1977) to optimise the structures and remove any salts. The library selection was from the Chembridge EXPRESS screening library, which comprises small compounds with a mean molecular weight of 355 Da, mean cLogP of 1.6 and mean total polar surface area of 66.8 Å (Schultz et al., 2011). A majority of the compounds within this library are defined by drug-like and lead-like descriptors, making them suitable targets for further development. The centre of the modelled binding pocket was defined by the positioning of the catalytic triad of AChE, (x = –58.4790, y = 58.3950, z = 19.8190) with 10 Å radius. The compounds were initially screened at 50% search efficiency, and 10 runs per compound. The basic amino acids lysine and arginine were defined as protonated and aspartic and glutamic acids were assumed to be deprotonated. The GoldScore (GS) (Jones et al., 1997), ChemScore (CS) (Eldridge et al., 1997; Verdonk et al., 2003), ChemPLP (PLP) (Korb et al., 2009) and ASP (Mooij and Verdonk, 2005) scoring functions were implemented to validate the predicted binding modes and relative energies of the ligands using the GOLD v5.4 software suite. The output for the scoring functions is dimensionless, a greater score predicts better likelihood of binding success. A higher screening efficiency of 100% and 100 runs per compound were used for the second round of screening. A consensus model was used to evaluate the pose of each compound, compounds that had similar poses determined by at least three of the four scoring functions were considered as possible candidates (Charifson et al., 1999). The final step of the virtual screen was the visual inspection in which the position of the compound within the pocket, and all protein ligand interactions, were evaluated for binding potential. Compounds with unfavourable poses or interactions were deemed unsuitable for selection.
In vitro Cholinesterase Inhibition Assay
Cholinesterase activities were measured by the Ellman method (Ellman et al., 1961) adapted for microtiter plates, as described by Ristovski et al. (2018) using electric eel acetylcholinesterase (eeAChE), human recombinant AChE (hrAChE) or horse serum butyrylcholinesterase (BChE) (all Sigma-Aldrich, St. Louis, Missouri, United States), all dissolved in 100 mM potassium phosphate buffer (pH 7.4) to 0.0075 U/mL. Stock solutions of the tested compounds (1 mg/mL) were prepared in 100% ethanol and progressively diluted in 100 mM potassium phosphate buffer (pH 7.4) to a final volume of 50 μL. Acetylthiocholine chloride and 5,5′-dithiobis-2-nitrobenzoic acid were dissolved in the same buffer in the respective final concentrations of 1 mM and 0.5 mM, and added in 100 μL volumes to the wells of the microtiter plates. Aliquots of 50 μL of each of the cholinesterases were added to start the reactions, which were followed spectrophotometrically at 405 nm and 25°C over 5 min using a kinetic microplate reader (Dynex Technologies Inc, Chantilly, Virginia, United States). Blank reactions without the inhibitors were run in the presence of the appropriate dilution of ethanol in 100 mM potassium phosphate buffer (pH 7.4). The concentrations of the compounds inducing 50% inhibition of enzyme activity (IC50) were determined as mean values ± SEM of three independent measurements. The data were analysed using the OriginPro software (OriginPro, 2020, OriginLab Corporation, Northampton, Massachusetts, United States). Active compounds were classified as either potent (IC50 < 10 μM), moderate (IC50 10–100 μM) or weak inhibitors (IC50 > 100 μM) based on their IC50 values (Moodie et al., 2019).
In vitro Microfouling Screening
The active compounds from the first screen and their analogs were evaluated against five microfouling organisms, including marine bacteria, Vibro harveyi (DSM 19623, Halomonas aquamarina (DSM 30161) and Pseudoalteromonas citrea (DSM 8771), and the two microalgae Porphyridium purpureum (AC122) and Exanthemachrysis gayraliae (AC15). The adhesion and growth inhibiting potential against the microfoulers was investigated in a 96-well format at 10 and 100 μg/mL employing the methodology described by Hellio et al. (2015) Briefly, stock solution of the selected compounds (2 mg/mL) were prepared in 20% ethanol and diluted to 10 and 100 μg/mL. Aliquots (100 μL) of diluted compound were distributed in microplates and evaporated at 25°C under vacuum. For the marine bacteria, 100 μL of stock culture at 2 × 108 cells/mL in peptone marine broth were added to each well. Bacterial growth was monitored at 620 nm after incubation for 48 h at 25°C. The bacterial adhesion was quantified at 545 nm after crystal violet staining of residual biofilm after careful washing of the wells. For microalgae, 100 μL of algal culture (0.1 mg chlorophyll a/L) in F/2 medium were added to the wells as described for the bacterial growth experiments above. The culture was maintained for 5 days at 20°C and the microalgal growth was quantified via fluorescent analysis (excitation at 485 nm, emission at 645 nm) of chlorophyll a released upon addition of 100 μL of methanol to the wells. Chlorophyll extraction was also performed on the adhered cells, after using a multichannel pipette to eliminate all the non-attached cells, and quantified by fluorescence measurement to determine the algal adhesion.
In vitro Macrofouling Screening Against Ciona savignyi
The ability of the rescreened leads to inhibit the settlement and metamorphosis of the Pacific transparent sea squirt Ciona savignyi larvae was investigated. Compounds were screened for effects following methods described in Cahill et al. (2016). Adult brood stock were harvested from Nelson Marina, Nelson, New Zealand and held in a recirculating seawater system (18 ± 1°C, 33 ± 1 PSU) and fed bulk-cultured Isochorysis glabana until ready to spawn (as indicated by full egg and sperm ducts). Three individuals were surgically spawned, cross-fertilised in artificial seawater, and left for 24 h to hatch. Freshly hatched larvae were diluted in additional artificial seawater to yield final larval concentration of 3 ± 1 larvae/mL. Aliquots (7.1 mL) of this larval suspension were added to wells of 12-well tissue culture plates (Corning Costar) which contained serial dilutions of the test compound in small amounts (70 μL) of 20% ethanol. The range of concentrations of each compound assessed was 0.25, 0.5, 2.5, 5, 12.5, 25, 50, and 100 μg/mL. Blank and solvent controls were also included, and three replicates were performed in all cases (N = 3). Plates were held at 18 ± 1°C for 5 days, after which the number of successfully settled and metamorphosed individuals were counted. Dose-responses were modelled using Weibull curve fitting (as dictated by model fit) and nominal concentration estimates that reduced settlement and metamorphosis relative to the controls by 50% (IC50) using R statistical software (R Core Team, 2020).
Results and Discussion
Homology Modelling
Fifty models were built using the MODELLER software package (Fiser and Šali, 2003). The model with the lowest objective function was chosen for further refinement and validation. Stereochemistry of the model was evaluated using a Ramachandran plot. The plot identified that 97.9% of the amino acids were in their highly preferred regions while the remaining 2.1% were preferred observations. No amino acids were positioned in questionable locations. The ProSA server result generated a Z-score value of –9.49 indicating that the overall model quality is more comparable to an NMR structure rather than an X-ray structure. Given the low sequence similarity a lower Z-score was expected. However, given the limited options the homology model was used for the virtual screen.
In silico Screening – Cholinesterase Docking
Molecular docking has been employed in several studies to develop an understanding of novel cholinesterase inhibitors for neurological applications (Correa-Basurto et al., 2007; Farrokhnia and Nabipour, 2014; Moodie et al., 2019). To probe a virtual screening approach for generating antifouling leads, compounds with theoretical binding to the active site of an AChE homology model of a marine fouling organism (C. gigas) were searched for from 10,000-compound library. The compounds from the screening library were selected, prepared, and docked to the AChE homology model to identify potentially active compounds for further analysis.
Selection of compounds to proceed to the next round of screening was based on the scoring threshold generated for each scoring function. The first-round thresholds of 31 (GS), 41(CS), 43(PLP), and 21(ASP) resulted in a total of 1,058 compounds continuing for the second round of screening. The selected compounds were then redocked to the active site of AChE with more stringent scoring thresholds set at 51 (GS), 25(CS), 60(PLP), 35(ASP) resulting in 201 compounds with sufficient binding scores. These compounds were visually inspected and further evaluated based on their pose and bonding interactions within the binding pocket. Finally, 39 compounds displaying good theoretical fit were purchased from the commercial vendor for initial testing in the biological assays (Figure 1).
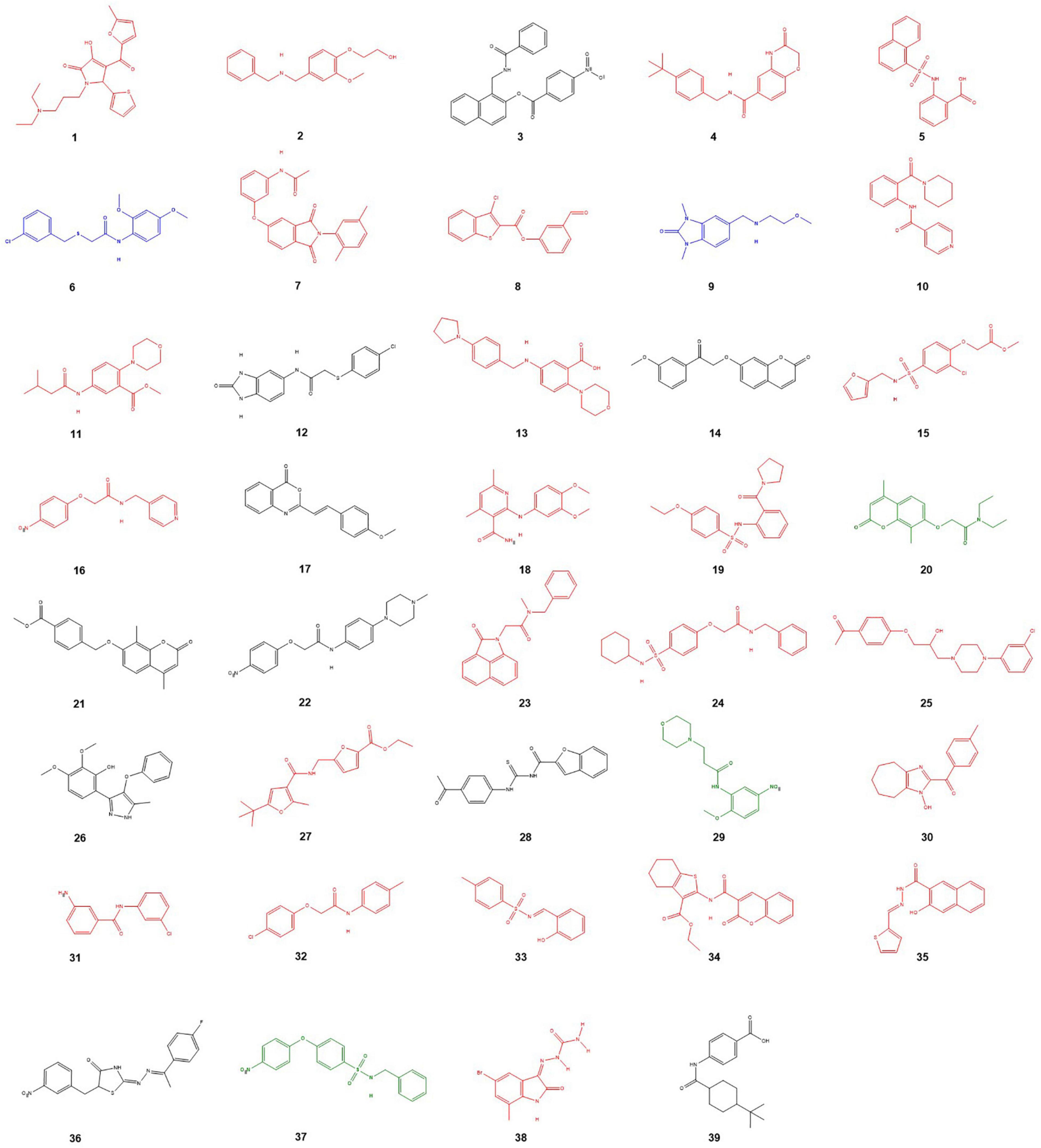
Figure 1. Structures of the compounds selected for the first in vitro screen. Compounds in black were too insoluble for in vitro testing. Red compounds were inactive. Weakly active compounds (6,9) are blue and moderately active compounds (20,29,37) are displayed in green.
In vitro Screening – Cholinesterase Enzyme Inhibition
The initial leads were tested for their ability to inhibit electric eel AChE. Compounds 3, 12, 14, 17, 21, 22, 26, 28, 35, 36, and 39 were shown experimentally to be too hydrophobic to allow dissolution in either water, ethanol or DMSO which excluded them from the in vitro evaluation. Of the remaining 28 compounds, five displayed either weak (IC50 > 400 uM, compounds 6 and 9) or moderate inhibitory activity (IC50 10–100 μM, compounds 20, 29, and 37). The remainder of compounds were inactive. The five active compounds (6, 9, 20, 29, and 37) were used as the basis for further activity refinement via analog searches of the active scaffolds. A search for all commercially available analogs was conducted and 19 additional compounds were subsequently purchased for testing after taking compound hydrophobicity into account (ClogP < 4.0) as a key selection criterion (Figure 2).
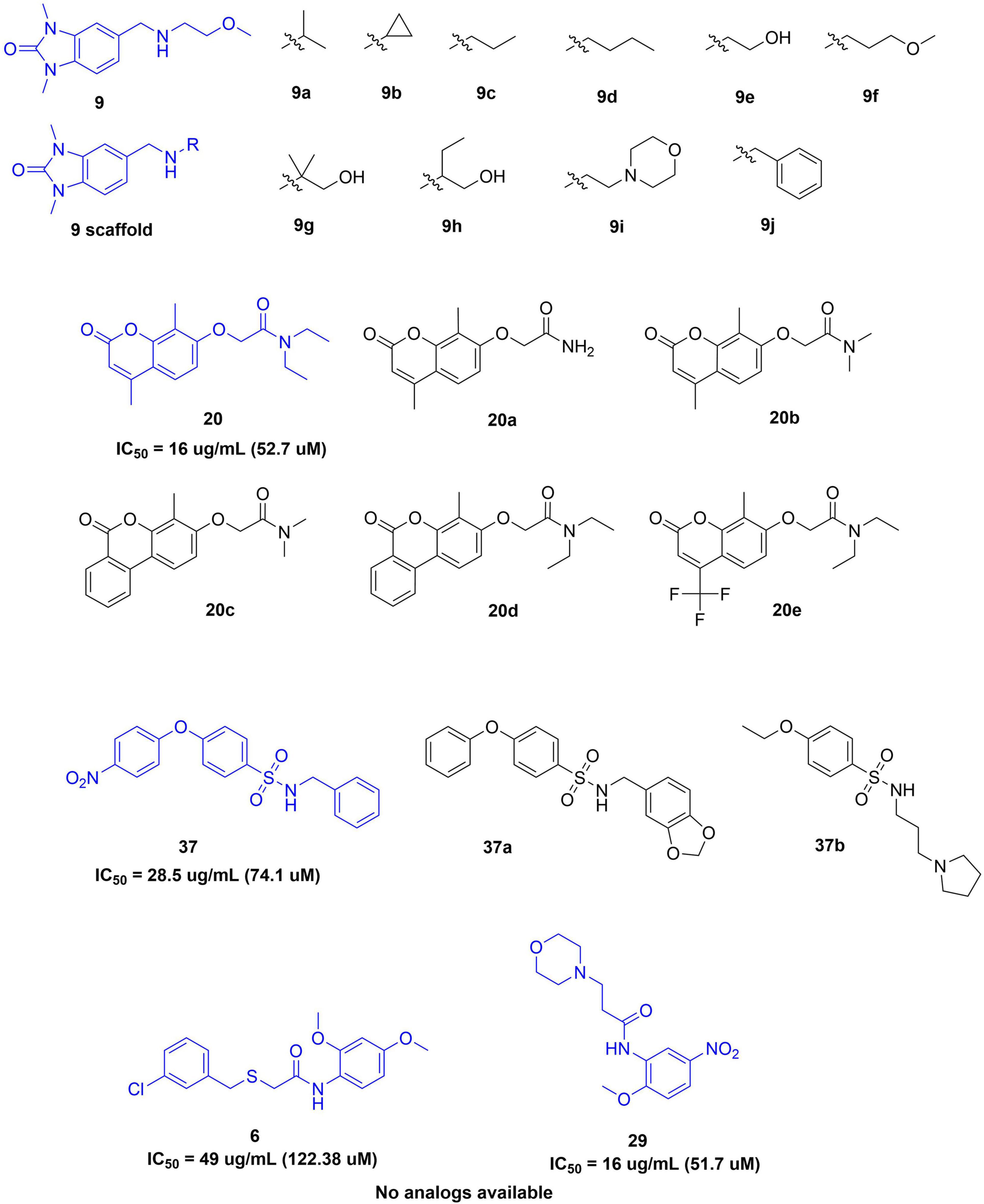
Figure 2. Structures of analogs designed and selected for the second in vitro screen and for micro- and macrofouling evaluation.
When the 19 compounds were rescreened for eeAChE, hrAChE, and BCh inhibitory activities, seven analogs displayed moderate or high inhibitory activity in a dose-response dependent manner (Figure 3 and Table 1). IC50 values against eeAChE ranged from 3.36 ± 0.39 μM for 20e to 169.54 ± 0.91 μM for 37a. Most compounds were active against eeAChE only and only two compounds (20c and 37) were active inhibitors of all three enzymes.
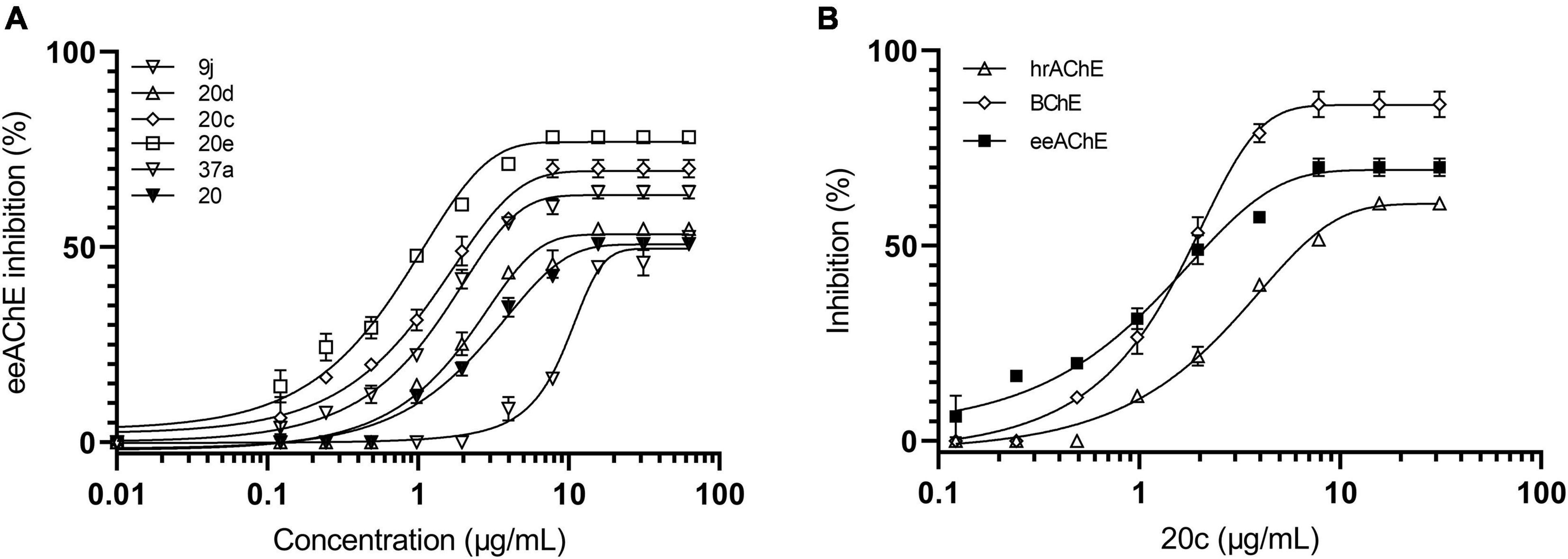
Figure 3. Inhibition of cholinesterase enzymes. (A) Inhibition of eeAChE by compounds 9j, 20, 20c, 20d, 20e, and 37a. The IC50 values toward eeAChE were determined as 9.44 μM for 9j, 52.7 μM for 20, 7.23 μM for 20c, 35.35 μM for 20d, 3.36 μM for 20e and 169.54 μM for 37a. (B) Inhibition of eeAChE, hrAChE and BChE by compound 20c. The IC50 values toward eeAChE, hrAChE and BChE were determined to be 7.23 μM, 22.81 μM and 5.94 μM, respectively.
The activity seen for the most active compounds is high (Moodie et al., 2019) and shows how the virtual screening is able to guide the discovery of novel structures. For this study, the analog design/selection was based on commercially available compounds and no chemical modifications or synthetic procedures were employed to modify the leads. Given the inhibitory activities observed, these lead structures identified could form the foundation for future rational design and structure-activity relationship studies.
No suitable analogs of 6 and 29 were commercially available but several analogs of 9 with structural diversity in the N-alkyl chain were included in the second screen. Of these analogs (9 – 9j), only 9j displayed pronounced cholinesterase inhibition with a potent inhibitory activity against eeAChE of 9.44 ± 0.85 μM. This experimental observation was supported by subsequent docking studies. Docking predictions indicated consistent poses for the non-active compounds based on the four scoring functions used to generate results. Compound 9j had a different predicted pose (Figure 4), showing the opposite pattern, predicting a hydrogen bonding interaction with SER124 and Pi Stacking interactions with TRP83, and TRP 331.
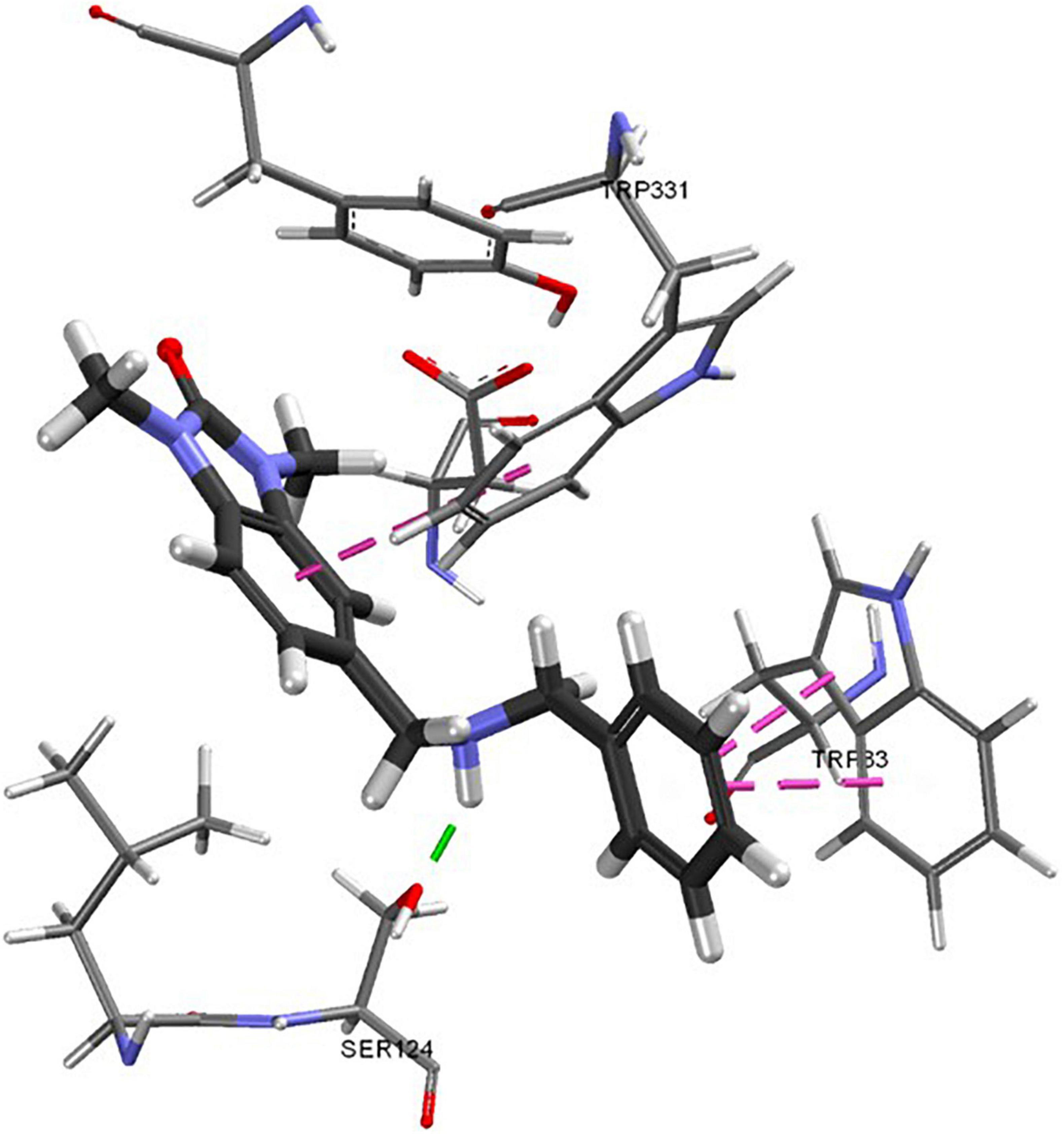
Figure 4. Predicted binding of 9j to the homology model. Green dashes indicate hydrogen bonding interactions, pink dashes indicate π-π interactions.
Four of the five chromenone analogs of compound 20 displayed positive results in the cholinesterase bioassays with only terminal amide 20a being inactive. Interestingly compound 20b (49 μM) and 20e (3 μM) appears to be selective for eeAChE while 20 and 20d and showed activity against both eeAChE and hsBChE indicating they may be a less selective inhibitors. Finally, 20c showed a high activity against all enzymes illustrating a high general cholinesterase inhibitory profile.
Selected analogs were redocked to investigate the difference in activity seen between evaluated enzymes. Compound 20b showed a consistent predicted binding pose by all four scoring functions. The chromenone moiety was positioned deep in the pocket with the tail facing the surface (Figure 5). The chromenone carbonyl moiety has a predicted hydrogen bonding interaction with GLY119. The ester also has a predicted interaction with SER124 positioned in the middle of the pocket. The chromenone moiety also features multiple hydrophobic interactions with TRP83.
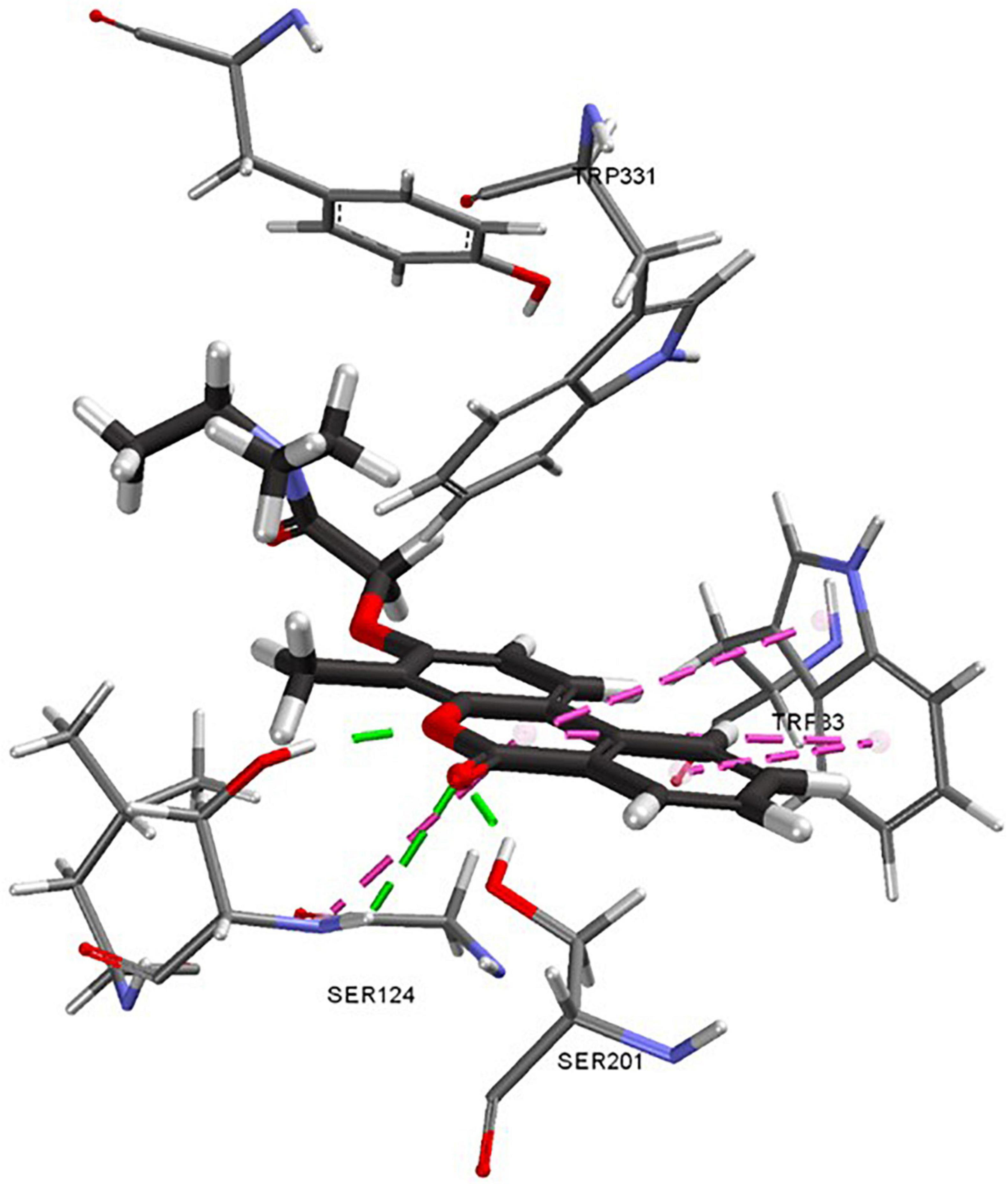
Figure 5. Predicted docking pose of compound 20d. Green dashes indicate hydrogen bonding interactions and pink dashes indicate π-π interactions.
Docking results of compound 20d showed a predicted shift in position, aligning the chromenone moiety closer to allow for hydrogen bonding interactions with both SER198 and SER121 (Figure 6). The shift in positioning now indicates that the hydrophobic interactions of this moiety are taking place with TRP331. The interaction with SER198 may be the reason for increased activity in the eeAChE assay and new activity in the hsBChE assay, as the amino acid is known to play a part in catalytic activity.
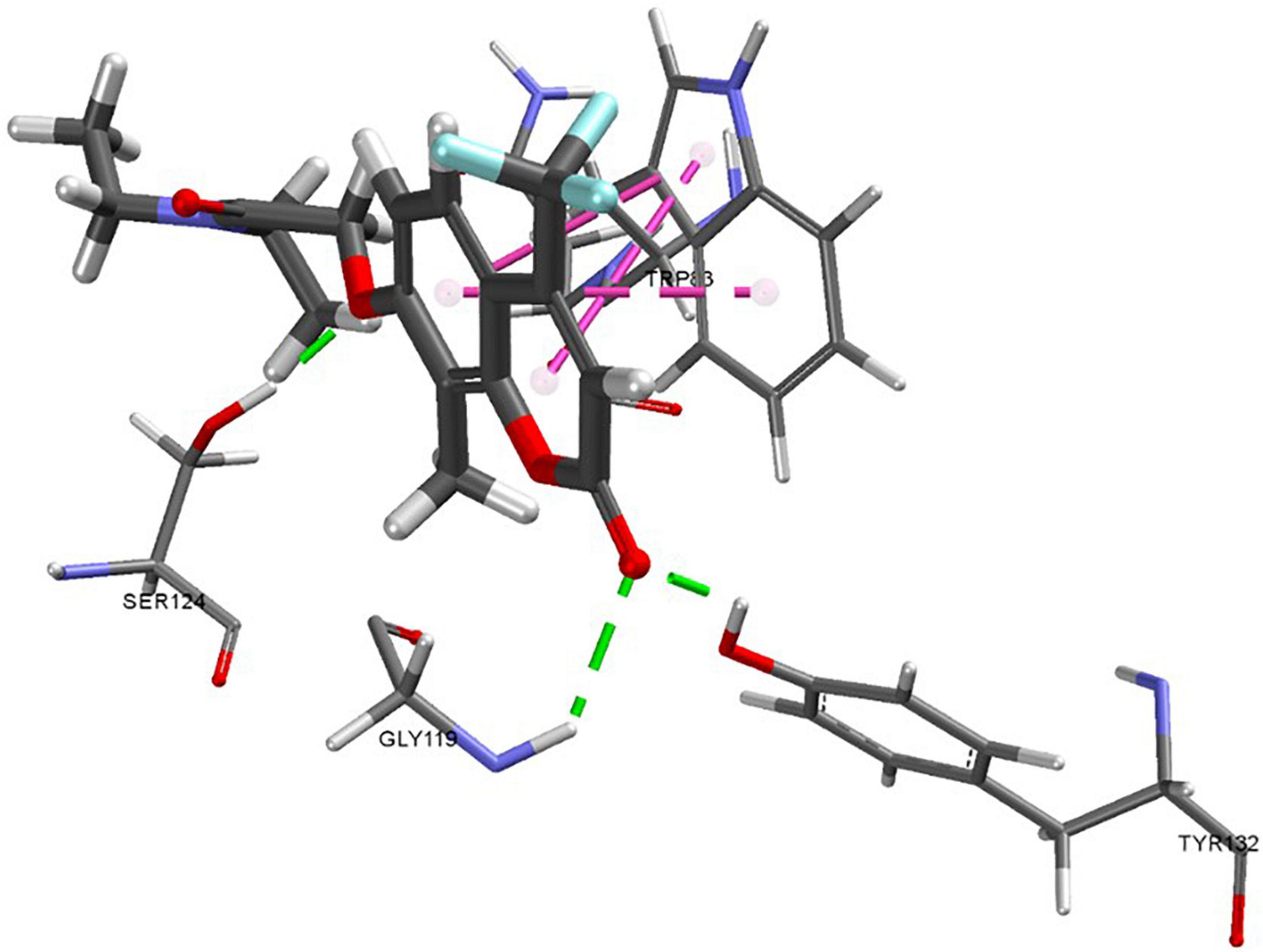
Figure 6. Predicted docking pose of compound 20e. Green dashes indicate hydrogen bonding interactions and pink dashes indicate π-π interactions.
Compound 20e has structural similarities to 20d, featuring a trifluromethyl group on the chromenone in place of the phenyl ring. This alternation reduced activity against hsBChE but increased activity for eeAChE. The pose prediction for 20e compound varies from 20d with the chromenone moiety rotated and predicted to have hydrogen bonding interactions with GLY119 and TYR132. The pi-pi interactions appear to stack more compared to 20d. The impact of the trifluoromethyl can be observed when comparing 20 to 20e, as the only difference is the substitution of the methyl with trifluoromethyl, resulting in a large increase of activity against eeAChE. This observation implies that the change has either altered the polarity of the compound, resulting in a less stable energy conformation within the pocket, or the halogen interactions and size had been more favourable. Chromenone class compounds are known for their activity in human neurotransmission (Piazzi et al., 2003; Najafi et al., 2016) so it is interesting that the virtual screen has generated compounds that are not active against the hrAChE which implies that these compounds may be worth further exploring.
Of the two analogs of compound 37 evaluated, only 37a displayed any activity, representing a reduction in comparison to the generally active 37. Both compounds share a very similar pose, indicating that the difference in activity could be due to the loss of the nitro group.
In vivo Screening – Biofouling Settlement and Metamorphosis
Having established a wide range of inhibitory activities against three cholinesterase enzymes, the compounds were subsequently experimentally assessed in vivo against five microfoulers and one macrofouler. Microfouling can cause microbially induced corrosion and it also leads to loss of hydrodynamic properties despite being caused by microscopic organisms. The microfouling screen assessed settlement/adhesion and growth of selected marine bacteria and microalgae. Both the active compounds from the second cholinesterase screen as well as the inactive ones were included. No reduction in either the settlement or growth of the five microfouling organisms was observed over the course of the experiments (data not shown) suggesting that the compounds are inactive at the two concentrations evaluated (10 and 100 μg/mL). While no specific antifouling activity was observed, the lack of effect on the growth of the microorganisms also indicate that these compounds are not generally toxic.
The 11 active compounds (Table 1) were also evaluated as inhibitors for the metamorphosis of larvae of the seasquirt C. savignyi, an invasive macrofouler that is problematic in scenarios including ship hull fouling and aquaculture. Out of the 11 compounds, 9j was shown to be a potent inhibitor with an IC50 of 4.60 ± 0.39 μg/mL as determined from the dose-response curve (Figure 7). None of the other compounds displayed any activity against C. savignyi at the employed concentrations.
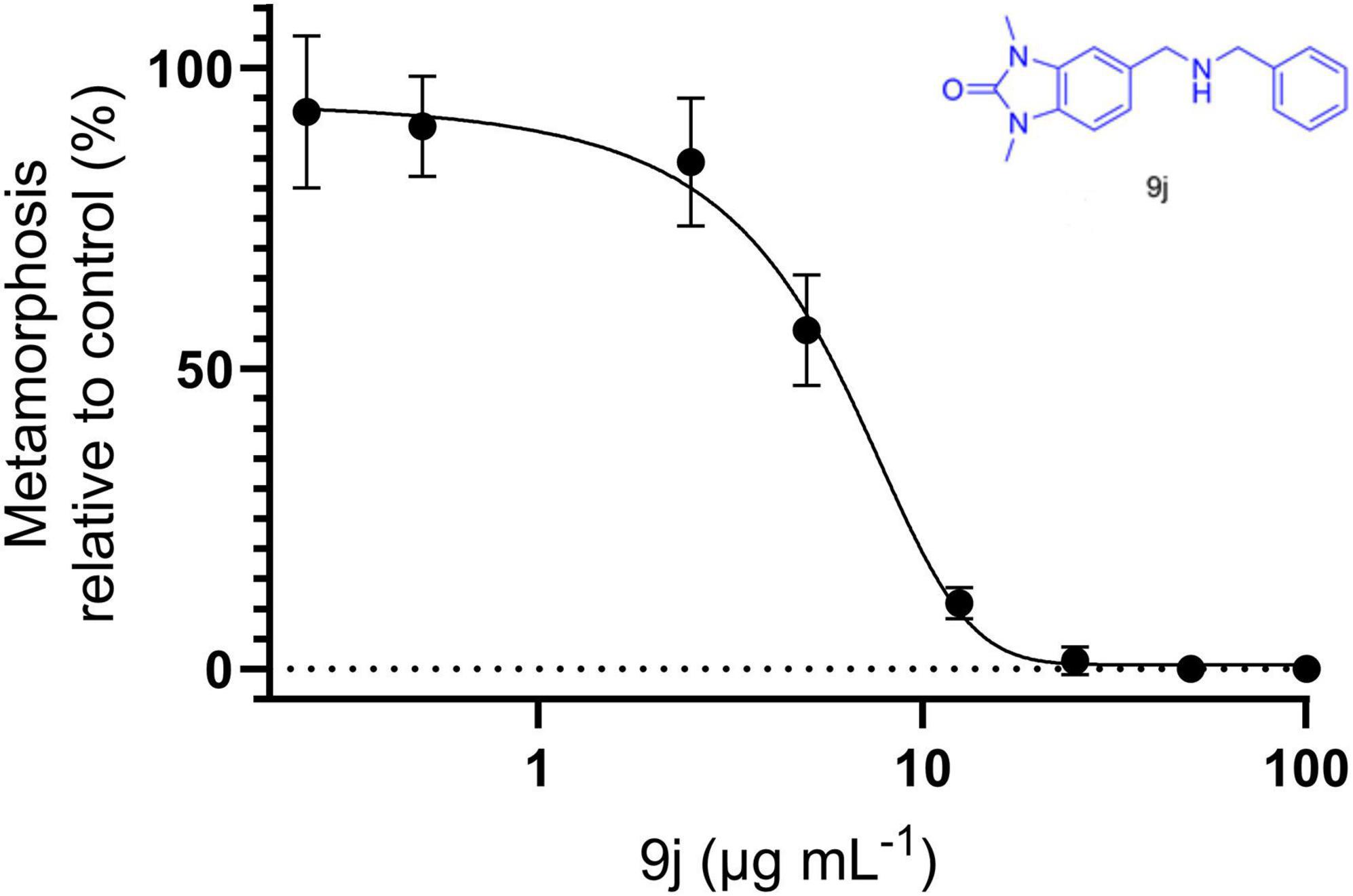
Figure 7. Dose-response curve illustrating the effect of 9j on the metamorphosis of C. savignyi larvae.
While 9j is clearly a potent novel inhibitor of both C. savignyi larval metamorphosis and eeAChE, none of the other active cholinesterase inhibitor analogs were active in the antifouling screens. The initial lead structure 9 was only weakly active and it is likely that 9j would not have been discovered without inclusion of analogs after the initial in vitro screen. Several of the other inactive antifouling compounds display similar or stronger cholinesterase inhibitory potential in vitro (20c, 20d, and 20e) and cholinesterase inhibition at low micromolar concentrations and our study shows that this does not automatically equal a strong antifouling effect even though several natural products have been reported with these dual bioactivities. Key structural requirements are at play and these warrants further research. In addition, our study illustrates the importance of performing in vivo experiments to verify effects predicted from in silico and/or in vitro screening experiments.
The fact that none of the compounds were active against the microfoulers was more expected as these primitive microorganisms lack the nervous system of the eukaryotes. Prokaryotic cholinesterases exist, but despite catalysing the same chemical transformation, they share little structural homology with the eukaryotic counterparts and overlapping binding preferential of compounds from the virtual hrAChE screen is not expected (To et al., 2020). Nevertheless, this study highlights that the cholinesterase enzyme family represent a valid target for several types of antifouling organisms.
Conclusion
The current study probes the correlation between cholinesterase inhibition and antifouling and describes a computational approach to search for novel antifoulants via virtual homology screening. A selection of novel cholinesterase inhibitors was discovered through the in silico and in vitro screening process. From these leads a single novel antifouling compound was identified with strong inhibitory effects on C. savignyi larval metamorphosis. No compounds with activity against marine bacteria or microalgae were discovered. Our study illustrates a rational and rapid screening strategy to identify novel structural leads for antifouling lead development and it also highlights that a strong in vitro cholinesterase activity is not directly transferable to a high in vivo antifouling activity.
Data Availability Statement
The raw data supporting the conclusions of this article will be made available by the authors, without undue reservation.
Author Contributions
HA, PC, and JS planned and conceived the study, interpreted the data, and selected compounds. HA performed the virtual screening and the computational experiments. TT, RF, and KS performed the cholinesterase studies and compiled the results. VF and CH performed the microalgal antifouling experiments. PC performed the macrofouling screen. JS compiled all data and drafted the initial manuscript. All authors contributed to generate the final manuscript.
Funding
This study was funded by the New Zealand Ministry for Business Innovation and Employment (Contract number CAWX1805) and by the Slovenian Research Agency programmes P4-0053 and P1-0207.
Conflict of Interest
The authors declare that the research was conducted in the absence of any commercial or financial relationships that could be construed as a potential conflict of interest.
Publisher’s Note
All claims expressed in this article are solely those of the authors and do not necessarily represent those of their affiliated organizations, or those of the publisher, the editors and the reviewers. Any product that may be evaluated in this article, or claim that may be made by its manufacturer, is not guaranteed or endorsed by the publisher.
References
Allinger, N. L. (1977). Conformational analysis. 130. MM2. A hydrocarbon force field utilizing V1 and V2 torsional terms. J. Am. Chem. Soc. 99, 8127–8134. doi: 10.1021/ja00467a001
Almeida, J. R., Freitas, M., Cruz, S., Leão, P. N., Vasconcelos, V., and Cunha, I. (2015). Acetylcholinesterase in biofouling species: characterization and mode of action of cyanobacteria-derived antifouling agents. Toxins 7, 2739–2756. doi: 10.3390/toxins7082739
Altschul, S. F., Gish, W., Miller, W., Myers, E. W., and Lipman, D. J. (1990). Basic local alignment search tool. J. Mol. Biol. 215, 403–410.
Blihoghe, D., Manzo, E., Villela, A., Cutignano, A., Picariello, G., Faimali, M., et al. (2011). Evaluation of the antifouling properties of 3-alyklpyridine compounds. Biofouling 27, 99–109. doi: 10.1080/08927014.2010.542587
Cahill, P. L., Atalah, J., Selwood, A. I., and Kuhajek, J. M. (2016). Metamorphosis of the invasive ascidian Ciona savignyi: environmental variables and chemical exposure. PeerJ 4:e1739. doi: 10.7717/peerj.1739
Charifson, P. S., Corkery, J. J., Murcko, M. A., and Walters, W. P. (1999). Consensus scoring: a method for obtaining improved hit rates from docking databases of three-dimensional structures into proteins. J. Med. Chem. 42, 5100–5109. doi: 10.1021/jm990352k
Correa-Basurto, J., Flores-Sandoval, C., Marín-Cruz, J., Rojo-Domínguez, A., Espinoza-Fonseca, L. M., and Trujillo-Ferrara, J. G. (2007). Docking and quantum mechanic studies on cholinesterases and their inhibitors. Eur. J. Med. Chem. 42, 10–19. doi: 10.1016/j.ejmech.2006.08.015
Dafforn, K. A., Lewis, J. A., and Johnston, E. L. (2011). Antifouling strategies: history and regulation, ecological impacts and mitigation. Mar. Pollut. Bull. 62, 453–465. doi: 10.1016/j.marpolbul.2011.01.012
de Souza Neto, L. R., Moreira-Filho, J. T., Neves, B. J., Maidana, R. L. B. R., Guimarães, A. C. R., Furnham, N., et al. (2020). In silico strategies to support fragment-to-lead optimization in drug discovery. Front. Chem. 8:93. doi: 10.3389/fchem.2020.00093
Dickenson, N. C., Krumholz, J. S., Hunsucker, K. Z., and Radicone, M. (2017). Iodine-infused aeration for hull fouling prevention: a vessel-scale study. Biofouling 33, 955–969. doi: 10.1080/08927014.2017.1393803
Dobretsov, S. V., and Qian, P.-Y. (2003). Pharmacological induction of larval settlement and metamorphosis in the blue mussel Mytilus edulis L. Biofouling 19, 57–63. doi: 10.1080/0892701021000060860
Eldridge, M. D., Murray, C. W., Auton, T. R., Paolini, G. V., and Mee, R. P. (1997). Empirical scoring functions: I. The development of a fast empirical scoring function to estimate the binding affinity of ligands in receptor complexes. J. Comput. Aided Mol. Design 11, 425–445. doi: 10.1023/a:1007996124545
Ellman, G. L., Courtney, K. D., Andres, V. Jr., and Featherstone, R. M. (1961). A new and rapid colorimetric determination of acetylcholinesterase activity. Biochem. Pharmacol. 7, 88–95. doi: 10.1016/0006-2952(61)90145-9
Faimali, M., Sepčić, K., Turk, T., and Geraci, S. (2003b). Non-toxic antifouling activity of polymeric 3-alkylpyridinium salts from the Mediterranean sponge Reniera sarai (Pulitzer-Finali). Biofouling 19, 47–56. doi: 10.1080/0892701021000036966
Faimali, M., Falugi, C., Gallus, L., Piazza, V., and Tagliafierro, G. (2003a). Involvement of acetyl choline in settlement of Balanus amphitrite. Biofouling 19, 213–220. doi: 10.1080/0892701021000044228
Farrokhnia, M., and Nabipour, I. (2014). Marine natural products as acetylcholinesterase inhibitor: comparative quantum mechanics and molecular docking study. Curr. Comput. Aided Drug Design 10, 83–95. doi: 10.2174/1573409910666140408155615
Fiser, A., and Šali, A. (2003). Modeller: generation and refinement of homology-based protein structure models. Methods Enzymol. 374, 461–491.
Flemming, H.-C. (2020). Biofouling and me: my Stockholm syndrome with biofilms. Water Res. 173:115576. doi: 10.1016/j.watres.2020.115576
Galdeano, C., Coquelle, N., Cieslikiewicz-Bouet, M., Bartolini, M., Pérez, B., Clos, M., et al. (2018). Increasing polarity in tacrine and huprine derivatives: potent anticholinesterase agents for the treatment of myasthenia gravis. Molecules 23:634. doi: 10.3390/molecules23030634
Hanssen, K. Ø, Cervin, G., Trepos, R., Petitbois, J., Haug, T., Hansen, E., et al. (2014). The bromotyrosine derivative ianthelline isolated from the arctic marine sponge Stryphnus fortis inhibits marine micro-and macrobiofouling. Mar. Biotechnol. 16, 684–694. doi: 10.1007/s10126-014-9583-y
Hearin, J., Hunsucker, K. Z., Swain, G., Stephens, A., Gardner, H., Lieberman, K., et al. (2015). Analysis of long-term mechanical grooming on large-scale test panels coated with an antifouling and a fouling-release coating. Biofouling 31, 625–638. doi: 10.1080/08927014.2015.1081687
Hellio, C., Trepos, R., Aguila-Ramírez, R. N., and Hernández-Guerrero, C. J. (2015). “Protocol for assessing antifouling activities of macroalgal extracts,” in Natural Products From Marine Algae, eds D. B. Stengel and S. Connan (New York, NY: Springer), 421–435. doi: 10.1007/978-1-4939-2684-8_27
Herzberg, M., Berglin, M., Eliahu, S., Bodin, L., Agrenius, K., Zlotkin, A., et al. (2021). Efficient prevention of marine biofilm formation employing a surface-grafted repellent marine peptide. ACS Appl. Bio Mater. 4, 3360–3373. doi: 10.1021/acsabm.0c01672
Jin, H., Tian, L., Bing, W., Zhao, J., and Ren, L. (2021). Toward the application of graphene for combating marine biofouling. Adv. Sustain. Syst. 5:2000076. doi: 10.1002/adsu.202000076
Jones, G., Willett, P., Glen, R. C., Leach, A. R., and Taylor, R. (1997). Development and validation of a genetic algorithm for flexible docking. J. Mol. Biol. 267, 727–748. doi: 10.1006/jmbi.1996.0897
Korb, O., Stutzle, T., and Exner, T. E. (2009). Empirical scoring functions for advanced protein- ligand docking with plants. J. Chem. Inf. Modeling 49, 84–96. doi: 10.1021/ci800298z
Labriere, C., Elumalai, V., Staffansson, J., Cervin, G., Le Norcy, T., Denardou, H., et al. (2020). Phidianidine A and synthetic analogues as naturally inspired marine antifoulants. J. Nat. Products 83, 3413–3423. doi: 10.1021/acs.jnatprod.0c00881
Larkin, M. A., Blackshields, G., Brown, N. P., Chenna, R., McGettigan, P. A., McWilliam, H., et al. (2007). Clustal W and clustal X version 2.0. Bioinformatics 23, 2947–2948. doi: 10.1093/bioinformatics/btm404
Lejars, M. N., Margaillan, A., and Bressy, C. (2012). Fouling release coatings: a nontoxic alternative to biocidal antifouling coatings. Chem. Rev. 112, 4347–4390.
Lind, U., Rosenblad, M. A., Frank, L. H., Falkbring, S., Brive, L., Laurila, J. M., et al. (2010). Octopamine receptors from the barnacle Balanus improvisus are activated by the α2-adrenoceptor agonist medetomidine. Mol. Pharmacol. 78, 237–248. doi: 10.1124/mol.110.063594
Liu, L.-L., Wu, C.-H., and Qian, P.-Y. (2020). Marine natural products as antifouling molecules–a mini-review (2014–2020). Biofouling 36, 1210–1226. doi: 10.1080/08927014.2020.1864343
Maan, A. M., Hofman, A. H., de Vos, W. M., and Kamperman, M. (2020). Recent developments and practical feasibility of polymer-based antifouling coatings. Adv. Funct. Mater. 30:2000936. doi: 10.1002/adfm.202000936
Mansueto, V., Cangialosi, M. V., and Arukwe, A. (2012). Acetylcholinesterase activity in juvenile Ciona intestinalis (Ascidiacea, Urochordata) after exposure to tributyltin. Caryologia 65, 18–26.
Melo, F. D. V. (2016). Isolation And Identification Of Bioactive Secondary Metabolites From Salinispora Arenicola Obtained From Ocean Sediments From The Madeira Archipelago. Ph. D. Dissertation. Lisboa: Faculdade de ciencias tecnologia universidae nova De lisboa.
Moodie, L. W. K., Trepos, R., Cervin, G., Bråthen, K. A., Lindgård, B., Reiersen, R., et al. (2017a). Prevention of marine biofouling using the natural allelopathic compound batatasin-III and synthetic analogues. J. Nat. Prod. 80, 2001–2011. doi: 10.1021/acs.jnatprod.7b00129
Moodie, L. W. K., Trepos, R., Cervin, G., Larsen, L., Larsen, D. S., Pavia, H., et al. (2017b). Probing the structure–activity relationship of the natural antifouling agent polygodial against both micro-and macrofoulers by semisynthetic modification. J. Nat. Prod. 80, 515–525. doi: 10.1021/acs.jnatprod.6b01056
Moodie, L. W., Sepčić, K., Turk, T., Frangež, R., and Svenson, J. (2019). Natural cholinesterase inhibitors from marine organisms. Nat. Prod. Rep. 36, 1053–1092. doi: 10.1039/c9np00010k
Mooij, W. T., and Verdonk, M. L. (2005). General and targeted statistical potentials for protein–ligand interactions. Proteins 61, 272–287.
Najafi, Z., Saeedi, M., Mahdavi, M., Sabourian, R., Khanavi, M., Tehrani, M. B., et al. (2016). Design and synthesis of novel anti-Alzheimer’s agents: acridine-chromenone and quinoline-chromenone hybrids. Bioorg. Chem. 67, 84–94. doi: 10.1016/j.bioorg.2016.06.001
Olsen, E. K., Hansen, E., Moodie, L. W., Isaksson, J., Sepčić, K., Cergolj, M., et al. (2016). Marine AChE inhibitors isolated from Geodia barretti: natural compounds and their synthetic analogs. Org. Biomol. Chem. 14, 1629–1640. doi: 10.1039/c5ob02416a
Piazzi, L., Rampa, A., Bisi, A., Gobbi, S., Belluti, F., Cavalli, A., et al. (2003). 3-(4-{[Benzyl (methyl) amino] methyl} phenyl)-6, 7-dimethoxy-2 H-2-chromenone (AP2238) Inhibits Both Acetylcholinesterase and acetylcholinesterase-induced β-amyloid aggregation: a dual function lead for alzheimer’s disease therapy. J. Med. Chem. 46, 2279–2282. doi: 10.1021/jm0340602
Qian, P.-Y., Chen, L., and Xu, Y. (2013). Mini-review: molecular mechanisms of antifouling compounds. Biofouling 29, 381–400. doi: 10.1080/08927014.2013.776546
Qian, P.-Y., Xu, Y., and Fusetani, N. (2009). Natural products as antifouling compounds: recent progress and future perspectives. Biofouling 26, 223–234. doi: 10.1080/08927010903470815
R Core Team (2020). R: A Language And Environment For Statistical Computing. Vienna: R Foundation for Statistical Computing.
Richard, K. N., Hunsucker, K. Z., Gardner, H., Hickman, K., and Swain, G. (2021). The application of UVC used in synergy with surface material to prevent marine biofouling. J. Mar. Sci. Eng. 9:662.
Ristovski, S., Uzelac, M., Kljun, J., Lipec, T., Uršič, M., Zemljič Jokhadar, Š, et al. (2018). Organoruthenium prodrugs as a new class of cholinesterase and glutathione-S-transferase inhibitors. Chem. Med. Chem. 13, 2166–2176. doi: 10.1002/cmdc.201800432
Schultz, M. P., Bendick, J. A., Holm, E. R., and Hertel, W. M. (2011). Economic impact of biofouling on a naval surface ship. Biofouling 27, 87–98. doi: 10.1080/08927014.2010.542809
Selim, M. S., El-Safty, S. A., Shenashen, M. A., Higazy, S. A., and Elmarakbi, A. (2020). Progress in biomimetic leverages for marine antifouling using nanocomposite coatings. J. Mater. Chem. B 8, 3701–3732. doi: 10.1039/c9tb02119a
Sjögren, M., Göransson, U., Johnson, A.-L., Dahlström, M., Andersson, R., Bergman, J., et al. (2004). Antifouling activity of brominated cyclopeptides from the marine sponge Geodia barretti. J. Nat. Prod. 67, 368–372. doi: 10.1021/np0302403
Terstappen, G. C., and Reggiani, A. (2001). In silico research in drug discovery. Trends Pharmacol. Sci. 22, 23–26.
Tian, L., Yin, Y., Jin, H., Bing, W., Jin, E., Zhao, J., et al. (2020). Novel marine antifouling coatings inspired by corals. Mater. Today Chem. 17:100294. doi: 10.1016/j.mtchem.2020.100294
To, T. A., Gagné-Thivierge, C., Couture, M., Lagüe, P., Yao, D., Picard, M. -È, et al. (2020). Structural insights into the putative bacterial acetylcholinesterase ChoE and its substrate inhibition mechanism. J. Biol. Chem. 295, 8708–8724. doi: 10.1074/jbc.RA119.011809
Trepos, R., Cervin, G., Hellio, C., Pavia, H., Stensen, W., Stensvåg, K., et al. (2014). Antifouling compounds from the sub-arctic ascidian Synoicum pulmonaria: synoxazolidinones A and C, pulmonarins A and B, and synthetic analogues. J. Nat. Prod. 77, 2105–2113. doi: 10.1021/np5005032
Verdonk, M. L., Cole, J. C., Hartshorn, M. J., Murray, C. W., and Taylor, R. D. (2003). Improved protein–ligand docking using gold. Proteins 52, 609–623.
Vinagre, P. A., Simas, T., Cruz, E., Pinori, E., and Svenson, J. (2020). Marine biofouling: a european database for the marine renewable energy sector. J. Mar. Sci. Eng. 8:495. doi: 10.3390/jmse8070495
Wiederstein, M., and Sippl, M. J. (2007). ProSA-web: interactive web service for the recognition of errors in three-dimensional structures of proteins. Nucleic Acids Res. 35 (suppl_2), W407–W410. doi: 10.1093/nar/gkm290
Yebra, D. M., Kiil, S., and Dam-Johansen, K. (2004). Antifouling technology—past, present and future steps towards efficient and environmentally friendly antifouling coatings. Prog. Org. Coat. 50, 75–104. doi: 10.1016/j.porgcoat.2003.06.001
Keywords: homology screening, in silico screening, in vitro enzymatic studies, cholinesterase, AChE inhibitor, antifouling
Citation: Arabshahi HJ, Trobec T, Foulon V, Hellio C, Frangež R, Sepčić K, Cahill P and Svenson J (2021) Using Virtual AChE Homology Screening to Identify Small Molecules With the Ability to Inhibit Marine Biofouling. Front. Mar. Sci. 8:762287. doi: 10.3389/fmars.2021.762287
Received: 21 August 2021; Accepted: 24 November 2021;
Published: 13 December 2021.
Edited by:
Kelli Zargiel Hunsucker, Florida Institute of Technology, United StatesReviewed by:
Sriyutha Murthy, Bhabha Atomic Research Centre, IndiaHuichao Jin, Jilin University, China
Copyright © 2021 Arabshahi, Trobec, Foulon, Hellio, Frangež, Sepčić, Cahill and Svenson. This is an open-access article distributed under the terms of the Creative Commons Attribution License (CC BY). The use, distribution or reproduction in other forums is permitted, provided the original author(s) and the copyright owner(s) are credited and that the original publication in this journal is cited, in accordance with accepted academic practice. No use, distribution or reproduction is permitted which does not comply with these terms.
*Correspondence: Johan Svenson, johan.svenson@cawthron.org.nz; orcid.org/0000-0002-4729-9359