- 1School of Oceanography, University of Washington, Seattle, WA, United States
- 2Darling Marine Center, University of Maine, Walpole, ME, United States
- 3Maine Department of Marine Resources, West Boothbay Harbor, ME, United States
Peptides and proteins were identified during a controlled laboratory degradation of the marine diatom Thalassiosira weissflogii by a surface seawater microbiome. Samples from each time point were processed both with and without the protease trypsin, allowing a partial differentiation between peptides produced naturally by microbial enzymatic degradation and peptides produced from the laboratory digestion of intact protein. Over the 12-day degradation experiment, 31% of the particulate organic carbon was depleted, and there was no preferential degradation of the overall protein pool. However, there was distinct differentiation in the cellular location, secondary structure and modifications between peptides produced by microbial vs. laboratory breakdown. During the initial period of rapid algal decay and bacterial growth, intracellular components from the cytoplasm were consumed first, resulting in the accumulation of membrane-associated proteins and peptides in the detrital pool. Accompanying the enrichment of membrane protein material was an increase in the importance of ɑ-helix motifs. Methylated arginine, a post-translational modification common in cell senescence, was found in high amounts within the microbially produced detrital peptide pool, suggesting a link between in-cell modification and resistance to immediate degradation. Another modification—asparagine deamidation—accumulated within the detrital peptides. Protein taxonomies showed the bacterial community decomposing the algal material was rich in Proteobacteria, and protein annotations showed abundant transportation of solubilized carbohydrates and small peptides across membranes. At this early stage of diagenesis, no changes in bulk amino acids (THAA) were observed, yet a proteomic approach allowed us to observe selective changes in diatom protein preservation by using amino acid sequences to infer subcellular location, secondary structures, and post-translational modifications (PTMs).
Introduction
The application of “omics” to marine systems has accelerated in recent decades and contributed to a deeper understanding of the roles microorganisms play in global biogeochemical cycles (Kleiner, 2019; Saito et al., 2019). Meanwhile, geochemical techniques have shown that some of the largest pools of carbon in the ocean are the detrital remains of these organisms, both in dissolved (Aluwihare et al., 1997; Benner and Kaiser, 2003; Jiao et al., 2010) and particulate organic matter (Wakeham et al., 1997; Hedges et al., 2001; Lee et al., 2004). Though largely unexplored, analytical advances are increasing the potential for “omic” approaches to illuminate carbon cycling in marine detritus (Nunn et al., 2010; Moore et al., 2012, 2014).
Much of the identifiable detrital organic matter in the ocean is proteinaceous (Mayer et al., 1988; Wakeham et al., 1997; Lee et al., 2004). This composition may be unsurprising given the abundance of proteins in living organisms: diatoms, one of the major primary producers in the ocean, are approximately 25% protein by dry weight (Olofsson et al., 2019). Photoautotrophic bacteria, such as Prochlorococcus, can be as much as 70% protein (Finkel et al., 2016). Determining the origins and fates of protein in marine systems is therefore critical to a predictive understanding of carbon transport and storage in a changing ocean.
Proteins are often assumed to be labile and subject to degradation (Nunn et al., 2003; Pantoja et al., 2009). Yet, some proteins have been shown to survive long exposure in seawater (Keil and Kirchman, 1994; Dong et al., 2010) and have been identified in the detrital dissolved organic matter pool (Tanoue et al., 1995; Suzuki et al., 1997; Yamada and Tanoue, 2003), in coastal and open ocean marine sediments (Mayer et al., 1986, 1988; Estes et al., 2019) and in sediment pore water (Schmidt et al., 2011; Abdulla et al., 2018; Fejjar et al., 2021). Algal proteinaceous material has been shown to survive microbial degradation in 4,000 year old sediments (Knicker et al., 1996). Thus, the geochemical evidence implies that while many proteins or constituents of proteins may indeed be labile, some component of proteinaceous material has the potential to be preserved into the geologic record. However, the underlying reasons that certain proteins or their constituents are preserved while others are rapidly degraded remain largely elusive.
The aquatic geochemical literature is rich in regards to protein and amino acid degradation. Heterotrophic bacteria first secrete free or tethered endoproteases to break larger proteins to smaller peptides (Hollibaugh and Azam, 1983; Hoppe et al., 2002; Obayashi and Suzuki, 2008). Exopeptidases then cleave individual amino acids off the termini, as shown via mass spectral detection of marine detrital protein (Nunn et al., 2003; Roth and Harvey, 2006), and peptides under around 600 Da can be taken up by heterotrophic bacteria and some phytoplankton (Weiss et al., 1991; Mulholland and Lee, 2009). Encapsulation and/or sorption interactions with organic matter and inorganic mineral surfaces may limit bacterial enzyme access to (and degradation of) protein and proteinaceous compounds (Mayer, 1994; Nagata and Kirchman, 1996; Hedges et al., 2001; Knicker et al., 2001).
With the application of metaproteomics tools to marine detirial systems, peptides sourced from algal organelles and membranes have emerged as more resistant to rapid degradation in environmental and laboratory studies (Yamada and Tanoue, 2003; Nunn et al., 2010; Moore et al., 2014). Certain secondary structures such as ɑ-helices and random coils appear to be retained within detritus while other motifs are degraded (Nunn et al., 2010), and peptides containing modified amino acids have been shown to accumulate in detrital pools of marine organic matter (Yamada and Tanoue, 2003; Liu et al., 2010; Abdulla et al., 2018; Duffy et al., in press).
Previous studies that apply metaproteomic techniques to marine detritus have generally added trypsin or other proteolytic enzymes to break proteins into smaller constituent peptides (Nunn et al., 2010; Moore et al., 2012, 2014). This proteolytic approach is typical in liquid chromatography-mass spectrometry (LC-MS) “bottom-up” proteomics experiments which analytically require small, ionizable peptides for detection rather than bulky and complex intact proteins (Laskay et al., 2013; Saito et al., 2019). However, this analytical requirement may obscure intriguing dynamics of natural protein breakdown and digestion in the environment. In the present study we complement existing protein degradation studies by additionally looking for small peptides produced solely by microbial degradation during the experiment. This naturally produced peptide pool represents a combination of endogenous small peptides and those that have been liberated from larger proteins but are not yet fully degraded. We leverage the amino acid sequences gained from metaproteomic techniques to interrogate the subcellular origins, secondary structures, modifications, and amino acid compositions of the degrading algal proteinaceous material over time.
Materials and Methods
The experimental workflow can be divided into distinct phases: the algal degradation experiment itself; amino acid and peptide extraction; peptide identification via a combination of a database searching and de novo peptide sequencing approaches; and data analytics.
Algal Degradation
A culture of the centric diatom Thalassiosira weissflogii was grown to approximately 1 × 106 cells/mL, concentrated by centrifugation, and frozen at −80°C to render the algal culture non-viable. Aliquots were thawed and resuspended to 2 g/L by dry weight using 1 μm filtered and UV sterilized seawater from the Damariscotta Estuary, Gulf of Maine. Unsterilized seawater filtered to 1 μm was used as an inoculum to induce bacterial decomposition of the algal detritus, with 1 mL added to 15 L of algal suspension. At each time point (1, 2, 5, and 12-days), 100 mL of the degradation slurry was vacuum filtered onto 25 mm diameter 0.3 um pore size glass fiber membranes (Sterlitech GF75) at 4°C and stored frozen prior to proteomic extraction and analysis. Further experimental details about the degradation can be found in Adams et al. (2019).
Total Hydrolyzable Amino Acids
Approximately 30 mg of material scraped from a filter was hydrolyzed in 6 N HCl as described in Cowie and Hedges (1992). Amino acids were derivatized as in Gray et al. (2017) using the AccQ Tag Ultra derivatization kit from Waters (Milford, Massachusetts). Amino acids were separated and quantified via LC-MS using a full scan method in positive ion mode, with a scan range of 100–600 m/z and a resolution of 60,000, on a Thermo Scientific Q Exactive HF Orbitrap.
Proteomic Sample Extraction
Peptides were extracted with and without the use of the serine protease trypsin, which specifically cleaves sequences on the carboxyl side (or the “C-terminal side”) of lysine and arginine, except when either is bound to a C-terminal proline. Peptides created via trypsin digestion are usually between 700 and 1,500 daltons (6–14 amino acids), an ideal range for mass spectrometry detection (Laskay et al., 2013). Approximately 100 mg of material was scraped from a filter into detergent-free 50 mM ammonium bicarbonate lysis buffer with 5 mM ethylenediaminetetraacetic acid (EDTA). The chilled suspension was lysed via three cycles each of mechanical disruption with silica beads (50% 100 μm diameter and 50% 400 μm), freeze-thawing in a methanol-dry ice bath, and 30 s in a high-power water bath sonicator. The supernatant was removed after centrifugation at 4,800 rpm and the protein concentration was determined using a modified Lowry assay using reagents from Bio-Rad (Hercules, California). Extracted protein was subjected to reduction of disulfides with dithiothreitol and carbamidomethylation with iodoacetamide. One half (300 μL) of the protein extraction was subjected to in-solution digestion with trypsin (Promega Gold) overnight at room temperature by a ratio of 1 μg trypsin: 25 μg total protein in 50 mM ammonium bicarbonate buffer with 5 mM EDTA. The remaining 300 μL of protein extraction was left untreated with trypsin. Both trypsin and no-trypsin treatments were desalted using NestGroup macro-spin C18 columns (Southborough, Massachusetts) and resuspended in 5% acetonitrile with 0.1% formic acid and Waters Hi3 E. coli peptide standard mixture (100 fmol/L).
Reverse-phase liquid chromatography-high resolution mass spectrometry (LC-HRMS) analysis was performed in duplicate with a Thermo Science (Waltham, Massachusetts) EASY-nanoLC system coupled to a Thermo Orbitrap Fusion Tribrid HRMS. Peptides were separated on a home-packed analytical column consisting of a 37 cm long, 75-μm i.d. fused-silica capillary column packed with C18 particles (Magic C18AQ, 100°A, 5 mm; Michrom) coupled to a 4 cm long, 100 m i.d. precolumn (Magic C18AQ, 200°A, 5 mm; Michrom). Solvents of 100% LC/MS grade water with 0.1% formic acid (A) and 100% LC/MS grade acetonitrile with 0.1% formic acid (B) were used to elute peptides over a 120-min gradient from 5 to 35% solvent B. All analyses were carried out in positive mode at an NSI spray voltage of 2 kV. Data-dependent acquisition (DDA) on the top 10 ions was carried out using first higher energy collision dissociation (HCD) and then electron transfer dissociation (ETD) fragmentation methods for duplicate injections. The MS1 (parent peptide ion) scan range was 400–2,000 m/z.
Proteomic Data Analysis
Peptides were identified from the raw mass spectra using a combination database-driven and database-independent de novo sequencing approach. De novo peptide sequencing, where the amino acid sequence of peptides is determined solely from the mass spectra without comparison to a reference database (Allmer, 2011), was advantageous in this study because we lacked a paired metagenome to thoroughly characterize the microbial community from the seawater inoculum (Muth et al., 2015, 2018; O’Bryon et al., 2020). The combining of database and de novo is termed de novo-directed proteomics, and was performed using PeaksDB within Peaks Studio (v8.5; Bioinformatics Solutions, Waterloo, Canada; Zhang et al., 2012). The de novo-directed approach has been shown to significantly improve sensitivity and accuracy in comparison to existing database search techniques (Zhang et al., 2012).
For database searches we used a reference protein database composed of 84,000 sequence entries predicted from transcriptomes of 8 T. weissflogii strains contained in the Marine Microbial Metatranscriptome Sequencing Project (NCBI BioProject PRJNA248394, Keeling et al., 2014). We added to the reference database two Gulf of Maine surface seawater metagenomes (Yooseph et al., 2007) from the Global Ocean Survey (GOS) as an aid in identifying the seawater microbes degrading the algal detritus. Additionally, we searched against a database of common mass spectral contaminants (Mellacheruvu et al., 2013). Search parameters for both database searching and de novo sequencing included 8 maximum modifications per peptide, 15 ppm peptide mass tolerance, and 0.5 Da fragment mass tolerance. For the trypsin-digested fractions, we performed both tryptic (maximum 2 missed cleavages) and non-enzymatic constraint searches, which means that all possible peptides up to 60 residues were considered. For the fractions not treated with trypsin, only non-enzymatic constraint searches were performed. Results from technical replicates and fragmentation strategies were combined.
Peptide identification confidences are calculated differently between the database search-identified and de novo sequenced peptides. For the de novo identifications, an amino acid-level confidence score is calculated based on mass deviation from spectral features and expressed as a percentage value. We accepted de novo peptides>80% average residue local confidence (ALC) with no single amino acid score<50%. For database searches, a false discovery rate (FDR) was set<1.0% using a reversed database target-decoy strategy (i.e., searching against reversed reference protein sequences) (Elias and Gygi, 2010). De novo sequencing was also incorporated into the database searches, as PeaksDB first compares de novo sequences to the reference database to find approximate matches and decrease the search space. Agreement between de novo sequences and database search hits are also used, in part, to generate peptide-level confidence scores derived from the p-value indicating the statistical significance of the peptide-spectrum match (the −10 lgP score). The threshold was a −10 lgP score>20. Such a score is equivalent to a p-value of 1%, signifying the probability that the identification is to a false peptide sequence (Zhang et al., 2012).
Protein identifications are notoriously difficult in samples containing many different organisms because some peptides are shared in proteins from multiple taxa. We required a minimum of 1 unique peptide per protein identification. Matching of proteins from peptides found only by de novo sequencing is detailed below.
Searching for Amino Acid Modifications
Since amino acids are frequently modified after translation, either for cell-specific purposes or during degradation, a computationally efficient method is needed to search for the myriad possible post-translational modifications (PTMs). We used the open modification search tool PeaksPTM (Han et al., 2011), with parameters set to tryptic or non-enzymatic constraint, 2 or fewer missed cleavages, 15 ppm peptide mass tolerance, 0.2 Da fragment mass tolerance, minimum A-score > 200 (a measure of modification location confidence), fixed carbamidomethylation of cysteine, and variable oxidation of methionine. Based on PeaksPTM results, the most frequently occurring modifications were used to evaluate whether adding additional PTMs to the overall searches altered the rate of false discovery. That is, we sought the optimal balance between searching for PTMs while avoiding a vast search space that leads to decreased sensitivity (Noble, 2015; Timmins-Schiffman et al., 2017). A series of PeaksDB searches and Peaks sequencing runs of the combined data set (using same search parameters as above) with increasing numbers of variable modifications was performed to find the optimal set of PTMs to include in searches (most peptide identifications<1% FDR). Using these PTM ramping results, 10 optimal variable PTMs were selected for the final searches. They included, in addition to methionine oxidation: deamidation of asparagine, methylation of arginine, oxidation of tyrosine, methylation of lysine, oxidation of lysine, oxidation of arginine, oxidation of proline, acetylation of lysine, and glutamine cyclization (the conversion of glutamine to pyro-glutamine).
Mapping de novo Peptides to Proteins
The de novo-directed PeaksDB workflow used here outputs peptides matched to the database and de novo peptides (sequences only, no additional information). To identify peptides that came from the diatom detritus or bacterial proteins not found by database searching, the de novo peptides were aligned to the reference database (T. weissflogii transcriptomes with GOS Gulf of Maine metagenomes) using PepExplorer (Leprevost et al., 2014). PepExplorer considers common de novo sequencing errors and limitations (such as leucine and isoleucine equivalence or other combinations of amino acids having the same mass) and identifies regions of local similarity between sequences. We also performed an alignment of the sequences against a reversed version of the database to estimate a false discovery rate, which was kept<1%. Alignments were accepted at a 95% identify agreement cutoff and protein identification required at least one unique peptide alignment.
Many de novo peptides did not match back to the reference database, which was not unexpected given the low number of sequences in the database for heterotrophic bacteria and the GOS sampling locations being more open ocean (Yooseph et al., 2007) compared to the estuarine seawater inoculum. To overcome this limitation, I additionally mapped the de novo peptides to proteins contained in the entire UniProt KnowledgeBase database (The UniProt Consortium, 2018), which contains over 200 million sequences from thousands of taxa. The mapping was performed using the UniPept lowest common ancestor tool (Mesuere et al., 2016), which is built specifically for metaproteomic data and determines the taxonomic origins of peptides to the lowest possible phylogenetic rank (since some peptides are highly conserved, they may match as to only “Bacteria,” or even “Organism,” rather than to a species or genus level). The UniPept output provides the best view of the bacteria present in the experiment given the lack of genes or transcripts from which to build an ideal reference database.
Gene Ontology Terms and Secondary Structures
To identify gene ontology (GO) term annotations, the peptide sequences were aligned to the UniProt protein database using UniPept’s metaproteomic functional analysis tool (Gurdeep Singh et al., 2019), which is built upon a lowest common ancestor peptide search described above. The GO categories were condensed from the broader set in order to eliminate redundancy using the REViGO tool1 as well as manually.
Protein and protein secondary structures for all samples were estimated using the Proteus2 algorithm (Montgomerie et al., 2008) for the combination of proteins identified by PeaksDB database searching and de novo sequencing with database mapping with PepExplorer. Output is the highest likelihood of individual amino acids being part of the following structure classes: random coil, ɑ-helices, β-strands, membrane ɑ-helices, and membrane β-strands.
Spectral files, databases, and peptide identification (pepXML) files have been deposited to the ProteomeXchange Consortium via the PRIDE partner repository with the data set identifier PXD027843.
Results and Discussion
General Characteristics of the Degradation
As previously described (Adams et al., 2019), algal biomass decreased by a factor of 2.4 during the 12-day incubation. Meanwhile, bacterial abundance increased by a factor of 5. Total particulate carbon decreased by 31% over the 12 days (Figure 1). In total, the algal detritus was partly remineralized via respiration and partly converted to bacterial biomass, but mostly remained as necromass (Adams et al., 2019). The degradation experiment started with a small inoculum of bacteria added to a detrital slurry that contained some algal proteases present during diatom cell harvesting. The algal detritus also likely contained small amounts of bacteria (or bacterial necromass) from the diatom culture, which was not confirmed to be axenic. The living bacteria then responded to the detritus and began to grow exponentially (Figure 1), during which time they released enzymes in order to break down and take up the diatom organic matter. The ratio of enzymatically hydrolyzable amino acids (a biomimetic measurement of protein bioavailability, see Mayer et al., 1995) to the organic carbon content remained constant throughout the experiment (Adams et al., 2019) indicating that there was no selective remineralization or preservation of proteins relative to other organic material at this early stage of degradation. However, by looking closely at peptides, we show that numerous compositional changes occurred in the protein pool during the 12 day period.
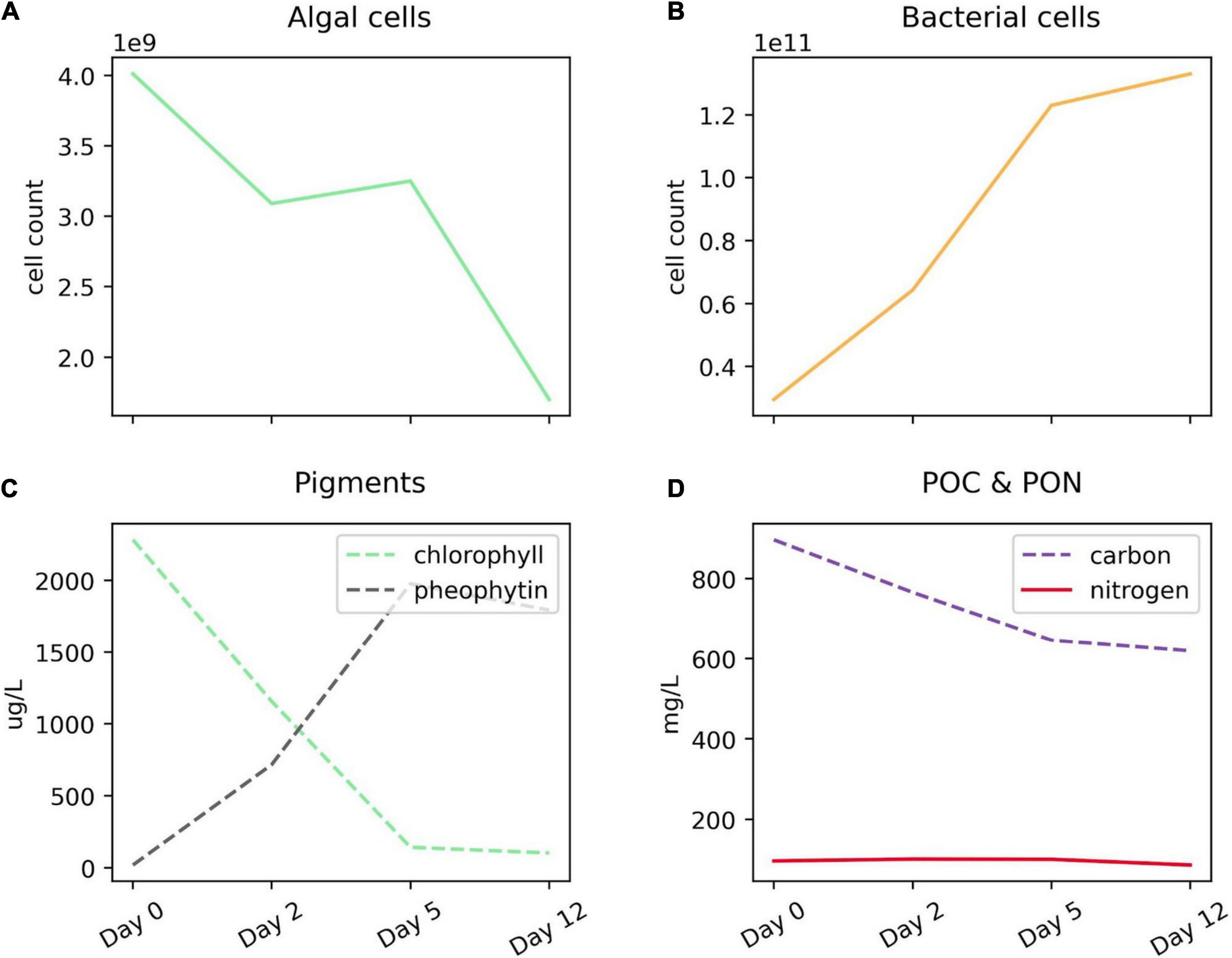
Figure 1. Progression of Thalassiosira weissflogii degradation experiment. (A) Algal and (B) bacterial cell counts over the 12-day incubation period; (C) chlorophyll and its degradation product, pheophytin; and (D) particulate carbon and nitrogen (filtered on GF/F membranes). While algal cellular material is degrading between days 2 and 5, the silica-based cell walls remain and are identifiable via microscopy, leading to the static or slightly increasing diatom cell counts (A). For more information about algal degradation see Adams et al. (2019).
Our interpretation of protein dynamics during the degradation experiment relies on the distinction between two different pool of peptides: those produced in situ during the experiment (not treated with trypsin, which we will refer to as “naturally digested peptides”) and those produced artificially by the laboratory digestion (referred to as “trypsin-digested peptides”). We hypothesize that the naturally digested peptides represent a mixture of proteinaceous material that is being accessed by the heterotrophic bacteria and peptides that have been released from larger proteins. The trypsin-digested peptides should represent the total of all digestible proteinaceous material present at each time point, including that which is not being actively degraded (e.g., the material that has resisted degradation thus far). Differences between these two diatom protein-derived pools provides information about relative labilities of the substrate proteins, in addition to information about the bacterial strategies of degrading the diatom necromass.
Both the trypsin and naturally digested peptide pools should also contain peptides sourced from the bacteria growing in the experiment. Given cell counts and estimated carbon per diatom and bacterial cells, there is about 150 times more algal protein than bacterial protein in the day 0 time point, and this decreases to 15 times more after 12-days (Adams et al., 2019). These peptides can be used to determine the types of bacteria present during the experiment, and the cellular functions that these bacteria are performing (e.g., Bergauer et al., 2017; Mikan et al., 2020). In the following section we examine the peptide results with the goal of trying to better understand the degradative processes occurring over the course of experiment and their implications for detrital protein cycling in the marine systems.
Algal Peptide Identification and Characteristics
The naturally digested and trypsin-digested peptides are methodologically distinct yet analytically overlapping pools. The naturally digested peptides are present in the background of the trypsin-digested fraction, since the incubation preceded proteomic sample extraction and laboratory digestion. The mass window of MS1 detection is 400–2,000 m/z. Typically, peptides produced via trypsin digestion are between 6 and 14 amino acids in length; in this data, the average trypsin-digested peptide length was 10.3 amino acids and the average peptide (MS1) mass was 1,114 Da. By contrast, the average length of peptides in the naturally digested fractions was 10.1 and the average mass, 1,082 Da. Larger polypeptides created by microbial degradation processes, if large enough, would likely have sites available to cleavage by trypsin. Thus there is additional overlap between these two pools because some larger naturally digested peptides are further subjected to trypsin digestion in the trypsin-digested fraction.
The number of identified diatom peptides and proteins peaked at day 2 during the initial phase of exponential bacterial growth, and then decreased to lowest values at day 12 (Table 1). We sorted the algal peptides by their gene ontology cellular compartment terms (GO Terms; Figure 2; see also Riffle et al., 2017; Mikan et al., 2020). The data were aggregated into eight major cellular compartments, and the three numerically dominant groups were chloroplast, cell membrane, and cytoplasmic proteins (Figure 2). In the case of membrane-bound subcellular compartments, proteins associated with organellar membranes were counted as from that organelles. For example, thylakoid membrane GO terms were classified under “Chloroplast,” for simplicity. The trypsin-digested day 0 fraction had a cellular component GO term distribution consistent with that of living diatom cells: alongside the degradation experiment’s algal GO terms we plot those from peptides obtained from two Thalassiosira pseudonana proteomes published by Dyhrman et al. (2012) (Figure 2).
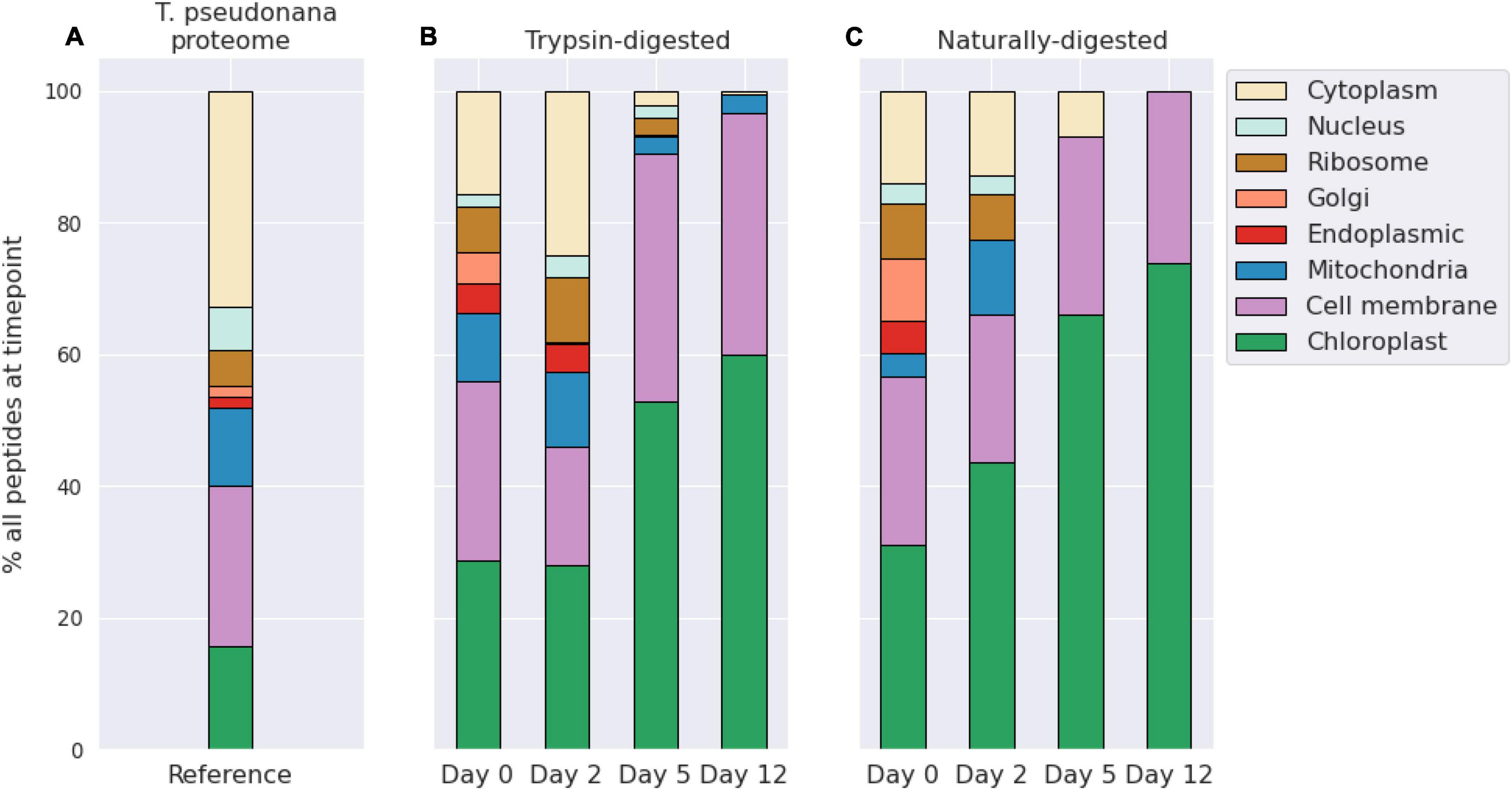
Figure 2. Bar plots showing the distribution of diatom peptides from different cellular compartments over a 12-day degradation experiment, as determined by peptide gene ontology (GO) terms annotations. Peptide distributions are shown as overall percentages of peptides for each timepoint and for the reference proteome. Shown as context are peptides identified in a proteome of Thalassiosira pseudonana cultures from Dyhrman et al. (2012) (A). Trypsin-digested (B) and naturally digested fractions (digestion only by natural microbial community, (C) are shown separately. Numbers of peptides at each timepoint and treatment are shown in Table 1. GO terms shown were condensed from a broader set to eliminate redundancy for ease of visualization using REViGO (http://revigo.irb.hr/) and further manually organized into broad categories.
The naturally digested peptides contained far fewer GO term identifications after day 2, and by day 12 the only identifications in that fraction were from chloroplast and cell membrane peptides. This indicates that peptides and proteins from other cellular components were effectively degraded during the initial phase of bacterial exponential growth (Figure 1) and were not being actively degraded at day 12 because they were no longer present. Consistent with this membrane recalcitrance hypothesis is that the number of identifications of trypsin-digested peptides sourced from larger proteins also decreased for most cellular compartments other than membranes and the chloroplast (Figure 2). However, there were still some trypsin-digested peptides at day 12 for components from the mitochondria and cytoplasm, suggesting that perhaps cellular location alone does not necessarily determine whether a protein or peptide will survive the initial stages of environmental degradation.
The number of trypsin-digested peptides of cytoplasmic location also dropped precipitously after day 2, while chloroplast and membrane peptides remained abundantly detectable throughout the 12-day experiment (Figure 2). This pattern indicates a shift in the detrital protein pool from one that mimics an intact living cell to one that is dominated by proteins that are associated with membranes. These findings are consistent with Laursen et al. (1996), who showed membrane proteins to be the more refractory fraction of phytoplankton protein by physical separation of subcellular fractions of phytoplankton cells. Their study reported higher proteolysis rate constants for the cytoplasmic fraction (>1.2 h–1) than for the membrane fraction (0.2–1 h–1), which correlated negatively with the ratio of chlorophyll to pheophytin (Laursen et al., 1996).
Studies of algal nutritional value to zooplankton and other animals also suggest preferential soluble protein consumption. In an evaluation of elemental uptake from diatom detritus, Reinfelder and Fisher (1991) showed that metal assimilation efficiencies of marine copepods were directly related to the cytoplasmic content of diatoms. This relationship indicates that the copepods sourced nearly all of their nutrition from the diatom cytoplasm rather than from other cellular constituents (Reinfelder and Fisher, 1991). Our experiment didn’t involve zooplankton grazing, so these results cannot easily be extended to those settings since larger organisms’ gut enzymes and mechanical maceration strategies were not part of our experimental system. However, the selective consumption of cytoplasmic protein components does indicate that membrane protein solubility or structure are factors in differential consumption by bacteria.
Preserved Protein Motifs
To further interrogate the proteinaceous material that survived the degradation, five classes of peptide secondary structure were evaluated: random coil, ɑ-helices, β-strands, transmembrane ɑ-helices, and transmembrane β-strands. Closely set ɑ-helices contain strong hydrogen bonding between weakly polar (Ser, Thr, Cys) and strongly polar (Asn, Gln, Glu, Asp, His, Arg, Lys) amino acid residues of neighboring ɑ-helices (Liu et al., 2004). Helices are commonly found in transmembrane proteins (Sakai and Tsukihara, 1998; Stevens and Arkin, 2000), and their ability to bend can account for the hydrophobic mismatch of the lipid bilayer (Yeagle et al., 2007). In contrast, β-strands lay relatively flat and have been hypothesized in a marine context to adhere to mineral surfaces—ultimately aiding to their protection and resulting in enhanced preservation (Shamblin et al., 1998; Oleschuk et al., 2000; Ovesen et al., 2011). “Random coil” is not a true secondary structure, but rather is an aggregate term for short sequences where there is an absence of a helix or sheet character (Smith et al., 1996). Benchmarking of the Proteus2 machine-learning algorithm we use here showed per-residue prediction accuracy to be 87–91% for transmembrane ɑ-helices, 86% for transmembrane β-strands, and 88% for non-membrane secondary structures (Montgomerie et al., 2008).
We note a progressive change in secondary structure distribution as the degradation proceeded, with membrane ɑ-helices becoming more important (Figure 3). At the same time, β-strands became a less common motif. This trend in secondary structure distributions is consistent with the GO term evidence indicating that membrane proteins are preferentially retained in the system and suggests that their tightly wrapped, difficult to denature, secondary structure could be a factor aiding in preservation. Random coils are the most common motif, and they do not change in relative importance over time, indicating that they are not particularly prone to resistance or degradation in this experiment, or that the coil category is too broad to capture any selective processes. These results are generally consistent with those of Nunn et al. (2010) in a similar degradation of a marine diatom. They identified 23 and 4 diatom peptides after 10 and 23 days of incubation with a seawater microbiome, respectively. Of the surviving 4 diatom peptides at the end of the experiment, 2 had transmembrane domains (Nunn et al., 2010).
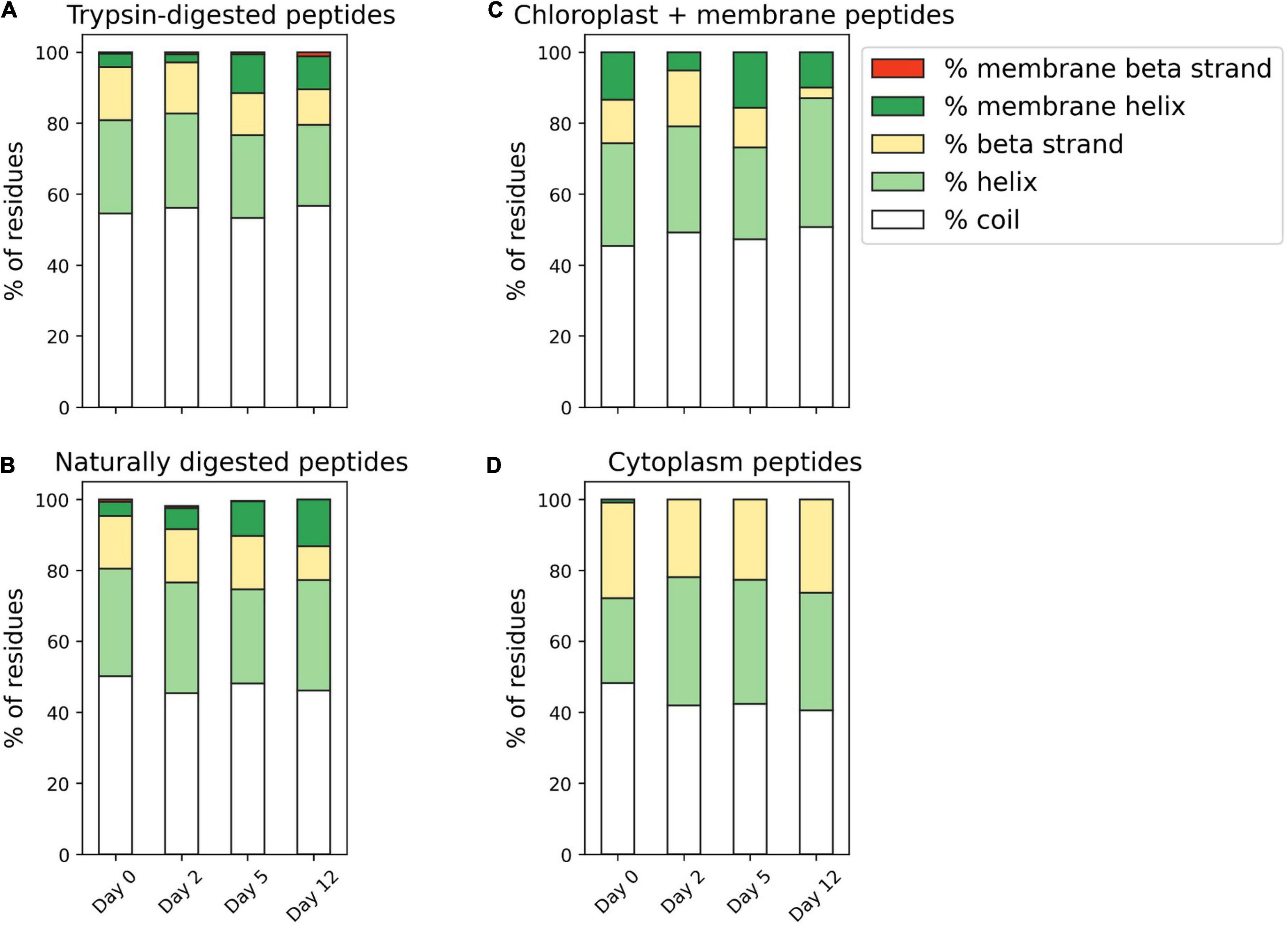
Figure 3. (A,B) Secondary structure predictions of algal peptides identified in trypsin-digested and naturally digested fractions at four points during the 12-day degradation experiment. Secondary structure motifs (coil, ɑ-helix, β-strand, membrane ɑ-helix, membrane β-strand) were predicted from full protein sequences using Proteus2 (Montgomerie et al., 2008) and the relative contribution of motifs determined by the identifying peptide’s relative peptide area abundance. (C,D) Secondary structure predictions of just algal chloroplast and membrane peptides and algal cytoplasmic peptides that are identified at each time point of the degradation. For this comparison, proteins identified in the trypsin-digested and naturally digested fractions were combined and adjusted by relative peptide area abundance.
To further investigate the theory that secondary structure is related to enhanced preservation of membrane protein components, we also compared the predicted secondary structure of just algal chloroplast/integral component of membrane peptides and algal cytoplasmic peptides that are identified at each time point of the degradation (Figure 3). Chloroplast and membrane peptides, the bulk of diatom peptide identifications on day 12 (from 167 individual proteins across both trypsin and naturally digested fractions), were 50.7% coil, 36.3% ɑ-helix, 3.02% β-strand, 9.93% transmembrane ɑ-helix, and 0% membrane β-strand. In comparison, the cytoplasmic peptides (from 98 proteins across both trypsin and naturally digested fractions) identified in the initial day 0 proteome were comparatively more enriched in β-strands and depleted in ɑ-helices and transmembrane ɑ-helices, with 48.3% coil, 23.8% and. In compariβ-strand, 0% transmembrane ɑ-helix, and 0% transmembrane β-strand. Supporting the theory that ɑ-helices are linked to preservation is that the few surviving cytoplasmic peptides on day 12 (3 proteins total, all in the trypsin-digested fraction) had 10% more ɑ-helix character than the initial day 0 cytoplasmic peptides, at 40.6% coil, 33.1% ɑ-helix, 26.3% β-strand, 0% transmembrane ɑ-helix, and 0% transmembrane β-strand (Figure 3). These findings are consistent with the notion that hydrophobic interactions appear to be important in preserving membrane-associated proteins and peptides during early diagenesis (Nguyen and Harvey, 2001; Nunn et al., 2010). Together, peptide subcellular localization annotations and secondary structure analyses connect previous observations of membrane-associated protein selective preservation for bacteria (Nagata et al., 1998; Kaiser and Benner, 2008; Jiao et al., 2010) and phytoplankton (Laursen et al., 1996; Nguyen and Harvey, 2001; Wolfe et al., 2006) to molecular characteristics like 3-dimensional shape and hydrophobicity.
While subcellular location and secondary structure may be significant components that allow certain proteins to resist the early stages of degradation, two other factors have been observed in the literature; an abundance of post-translationally modified amino acids in degradation-resistant material and the enrichment of certain amino acids, like glycine, in the recalcitrant proteinaceous pool (by measurement of total hydrolyzable amino acids).
Post-translational Modifications of Amino Acids
Protein PTMs such as oxidation, phosphorylation, and methylation, play critical roles in a diverse range of biological processes like signaling, protein activity and transport, and regulation of gene expression (Shen et al., 2008; Cain et al., 2014). PTMs are also associated with in vivo protein recycling and cell senescence (Cain et al., 2014; Dhillon and Denu, 2017). For these purposes, the “PTM” umbrella includes modifications to amino acids that could occur in detritus, including those due to protein consumption or abiotic transformations.
In the marine environment, PTMs have been linked to degradation and early diagenesis. For example, the amino acid beta alanine accumulates in the hydrolyzable phase of marine sedimentary organic matter via modification of aspartic acid (Cowie and Hedges, 1994). Modification of the nitrogen-containing side chains of glutamine, asparagine and arginine can lead to the accumulation of peptides containing deaminated side chains within anoxic marine sediment pore waters (Abdulla et al., 2018). We recently observed deamidated peptides in the sinking POM from the eastern tropical North Pacific oxygen deficient zone (Duffy et al., in press), where Van Mooy et al. (2002) hypothesized that amino acids could be selectively deaminated in order to provide reduced nitrogen to fuel chemoautotrophic processes (Van Mooy et al., 2002). In laboratory experiments, Keil and Kirchman showed that methylated peptides were accessed less efficiently by bacteria than non-methylated peptides (Keil and Kirchman, 1992). Glycan modifications of proteins contribute to stability, a mechanism that’s been used to increase the shelf life of protein and peptide-based pharmaceuticals (Zhou and Qiu, 2019). Indeed, Keil and Kirchman showed that glycosylated ribulose-1,5-bisphosphate (RuBisCo) was degraded 100 times more slowly than its unmodified counterpart (Keil and Kirchman, 1993). All together, the marine literature suggests that PTMs are highly relevant to protein degradation, as the by-products of protein degradation processes and/or as potential factors in degradation resistance.
In the present study, we first performed a non-discriminatory “open” search of thousands of possible PTMs in the UniMod database using PeaksPTM (see above in “Materials and Methods”). We then selected only the 10 most frequently occurring mass modifications in the open search results for the suite of PTMs in the ultimate database and de novo peptide searches. Our goal was to identify patterns in PTM distribution to learn more about their roles in degradation and preservation. To that end, we wanted to distinguish between PTMs present in algal protein prior to degradation and PTMs associated with the degradative process. We compared the PTMs observed in the naturally digested peptide pool, which should represent proteins being actively degraded by the bacteria, against the trypsin-digested peptides. This comparison was done for only the algal protein; no bacterial proteins were included in this differential analysis of PTMs (Figure 4).
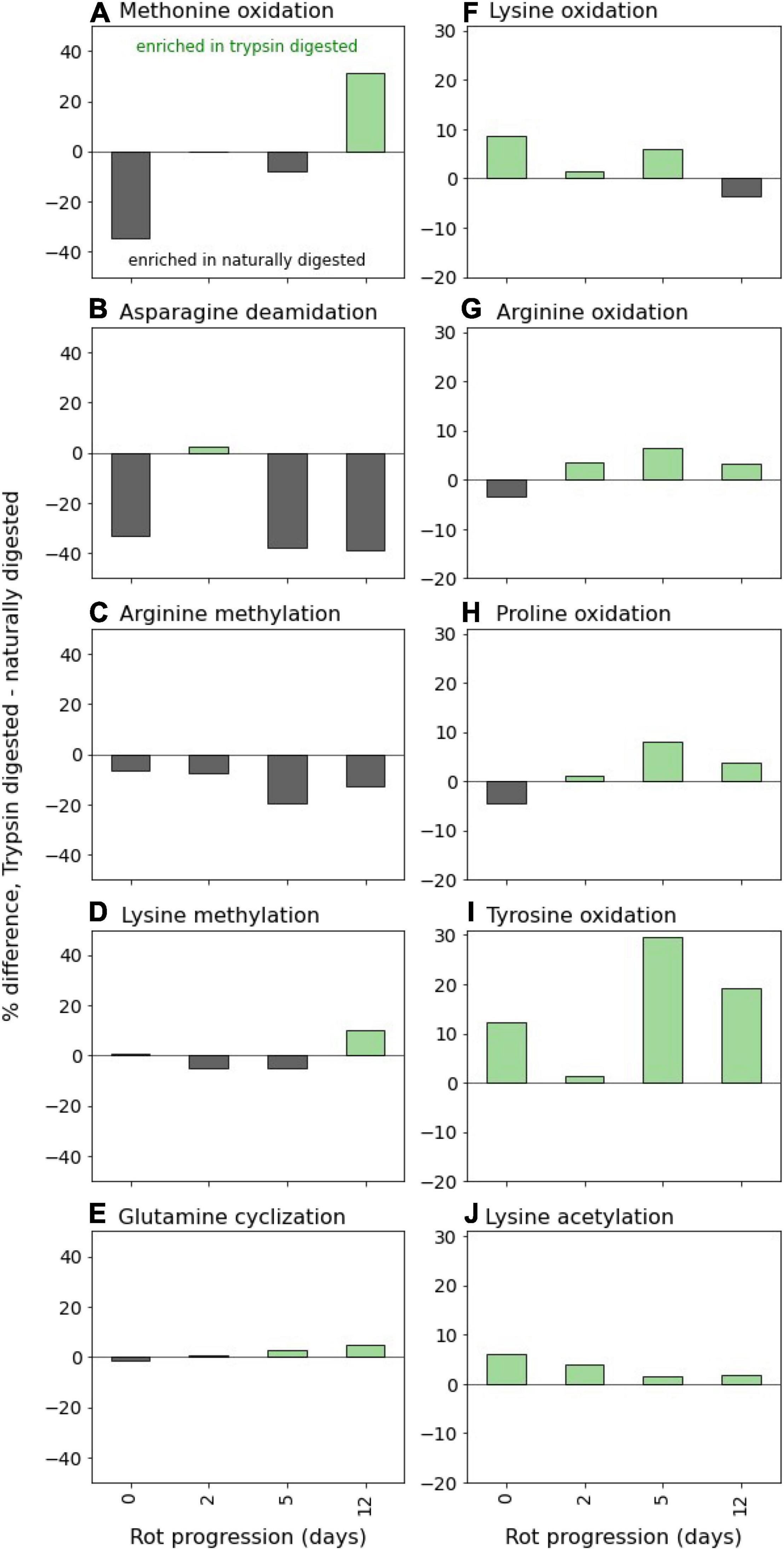
Figure 4. Post-translational modifications (PTMs) of algal peptides at four time points along the 12-day incubation. Shown are the difference between trypsin-digested naturally digested peptides for the 10 variable modifications included in the database searches and de novo sequencing parameters, which were selected from preliminary open modification searches of the mass spectral data (see section “Materials and Methods”): (A) methionine oxidation, (B) asparagine deamidation, (C) arginine methylation, (D) lysine methylation, (E) glutamine cyclization (pyro-glutamation), (F) lysine oxidation, (G) arginine oxidation, (H) proline oxidation, (I) tyrosine oxidation, and (J) lysine acetylation. PTM distributions are expressed as the differences in percent of residue modification in the entire peptide pool (database and de novo sequences) as corrected by relative peptide area abundance. Thus, positive values indicate the PTM is relatively enriched in the trypsin-digested peptides, and negative values mean the PTM is relatively enriched in the naturally digested peptides. Bacterial peptides were not included in the PTM analysis. Y-axis scales are the same between columns (A–J).
Oxidation PTMs were generally enriched in the trypsin-digested component, implying that these modifications occurred within the cell prior to heterotrophic attack. Generally, they did not increase in relative abundance over time in the trypsin-digested peptide pool, suggesting that oxidations don’t meaningfully impact the lability of proteinaceous material (Figure 4). It’s notable that during the experiment, chloroplast protein-derived peptides increasingly became dominant among identifications (Figure 2). The algal culture was exposed to light before the degradation experiment in the dark. Photosynthesis as an oxygenic process produces active oxygen species and radicals which can cause damage to cells. Oxidations of amino acids are frequent PTMs in photosynthesis-associated proteins (Aro et al., 2005; Galetskiy et al., 2011). Similarly, lysine acetylation of chloroplast proteins has been demonstrated in plants (Lehtimäki et al., 2015) and in diatoms (Chen X.-H. et al., 2018), though the PTM frequently occurs elsewhere in the cell (Chen Z. et al., 2018). The abundance of thylakoid membrane and chloroplast-associated proteins that accumulate due to preferential preservation (Figure 2) is likely why oxidations and lysine acetylations are dominant PTMs of the trypsin-digested peptides (Figure 4).
In contrast, two PTMs were strongly associated with the presumably more detrital naturally digested peptides: deamidation of asparagine and arginine methylation (Figure 4). This observation that asparagines and arginines are more modified in detrital proteins has several possible explanations. These modifications could have occurred within the living cell, resulting in a pool of protein that was easily accessible, which is why they were found more in the naturally degraded peptide pool. Alternatively, these PTMs might have been created during the degradation process and could accumulate because once created they are further degraded slower than their unmodified counterparts. This latter explanation would account for the effective accumulation of deamidated peptides in both sediment pore waters (Abdulla et al., 2018) and in sinking particulate matter (Duffy et al., in press). Thus, we hypothesize that deamination occurs during degradation, though continued research is warranted.
Unlike deamidation, methylation most likely occurs within the living cell, where it is a common PTM that is used as a control of numerous cellular functions (Ghesquière et al., 2011). Methylated peptides have been shown to be inefficiently assimilated and degraded by heterotrophic bacteria (Keil and Kirchman, 1992), suggesting that PTMs produced within living cells may also play a role in determining the rate of protein degradation.
Our open PTM searches did not yield high levels of protein glycosylation, a very broad classification of modification that entails a sugar-amino acid bond. Though glycosylation is an extremely common enzymatic PTM in both eukaryotes and prokaryotes, the non-enzymatic form of the reaction (sometimes called “glycation,” or the Maillard reaction) has been posited as a mechanism of environmental protein preservation via geopolymer formation (Collins et al., 1992; Burdige, 2007). Glycosylation has been shown to decrease microbial protein degradation rates in incubations (Keil and Kirchman, 1993) and has been found in high abundance in seawater (Yamada and Tanoue, 2003). Peptides with glycosylations are difficult to ionize and detect under the mass spectral conditions we used here (Alley et al., 2013), and to evaluate them more accurately would require different proteomics preparation techniques (e.g., as performed by Moore et al., 2014). Given the findings here and in the literature, more targeted work is needed to better understand the effect that PTMs have during the early stages of protein degradation.
We examined the PTM distribution across peptides from different subcellular compartments (Supplementary Figure 1), and found that (1) most modified peptides were from cell membrane and cytoplasm proteins, and (2) the relative distribution of PTMs across the subcellular compartments did not change significantly over the 12-day degradation, even as the overall distribution of peptides from the subcellular locations did change to become more membrane- and chloroplast-dominated (Figure 2). This result suggests that mechanisms other than modification status may be more important to overall preferential degradation pattern in this system, though the enrichment of certain PTMs in the trypsin-digested peptides or naturally digested peptides (Figure 4) points to subtler preferential degradation patterns amongst modified peptides.
Amino Acid Compositions
One of the deepest sets of literature related to protein degradation in marine systems is that of the “total hydrolyzable amino acid” pool (Wakeham et al., 1997; Dauwe and Middelburg, 1998; Dauwe et al., 1999; Lee et al., 2004). THAA analyses show clear trends during long-term carbon degradation and preservation including an accumulation of the amino acids glycine, serine and threonine (Dauwe and Middelburg, 1998), and the creation of the non-protein amino acids beta-alanine and gamma-aminobutyric acid from aspartic and glutamic acids (Cowie and Hedges, 1994). Despite the widespread use of degradation indices derived from THAA analyses, it has been difficult to reconcile changes in bulk amino acid compositions to known protein amino acid compositions, especially during the very early stages of degradation (Keil et al., 2000). We compared the amino acid composition of the peptides identified during the degradation experiment to the THAA pool, which I measured independently. To facilitate this comparison, we plotted mole fractions of amino acids in the THAA against those in the identified algal peptides, combining the naturally and trypsin-digested amino acid compositions adjusted for relative peptide abundance (Figure 5). The near 1:1 agreement between the two approaches indicates two things: (a) the protein amino acid compositions measured using the newer “omic” approach can be effectively integrated into the large body of literature based on THAA analysis, which will become useful as peptidomic approaches are applied to samples further along the degradation pathway (e.g., sediment samples); and (b) while the omic approach identifies specific ways in which protein undergoes degradation in the ocean, the early stages remain remarkably “non-selective” at the bulk molecular level (Hedges et al., 2001).
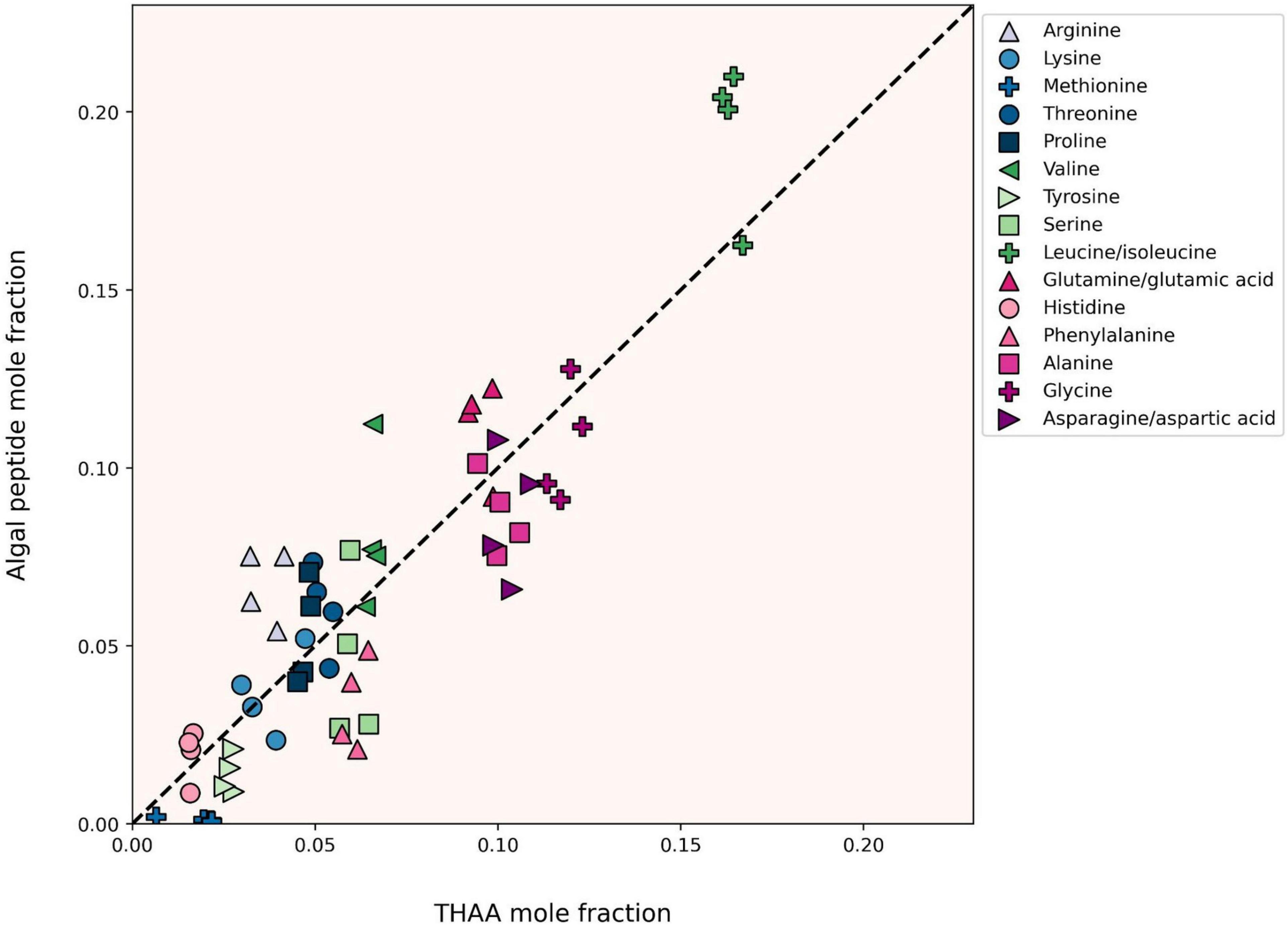
Figure 5. Mole fractions of individual amino acids from all four degradation time points as derived from total acid hydrolyzable amino acid analysis (THAA, x-axis) and tandem MS/MS-based proteomics (Peptide, y-axis). The dashed 1:1 line represents perfect agreement between approaches. The peptide amino acid compositions plotted here are derived from both trypsin-digested and naturally digested proteomics fractions. Label-free peptide quantitation was determined by the relative peptide area abundance.
Bacterial Community
The microbial inoculum for the degradation experiment was sourced from the Damariscotta River Estuary, whose marine waters come from the Gulf of Maine. Heterotrophic bacteria grew exponentially through the middle stages of the experiment (Figure 1). A peptide-based lowest common ancestor analysis was performed using UniPept (Gurdeep Singh et al., 2019) to assign bacterial taxa to the lowest phylogenetic level possible. The taxonomic hits were then adjusted to account for peptide spectral abundance and aggregated at the class level (Figure 6A). There is precedence for using label-free metaproteomic data for microbial biomass determinations (Kleiner et al., 2017), and here we use the approach to learn about the community of microbes degrading the algal detritus.
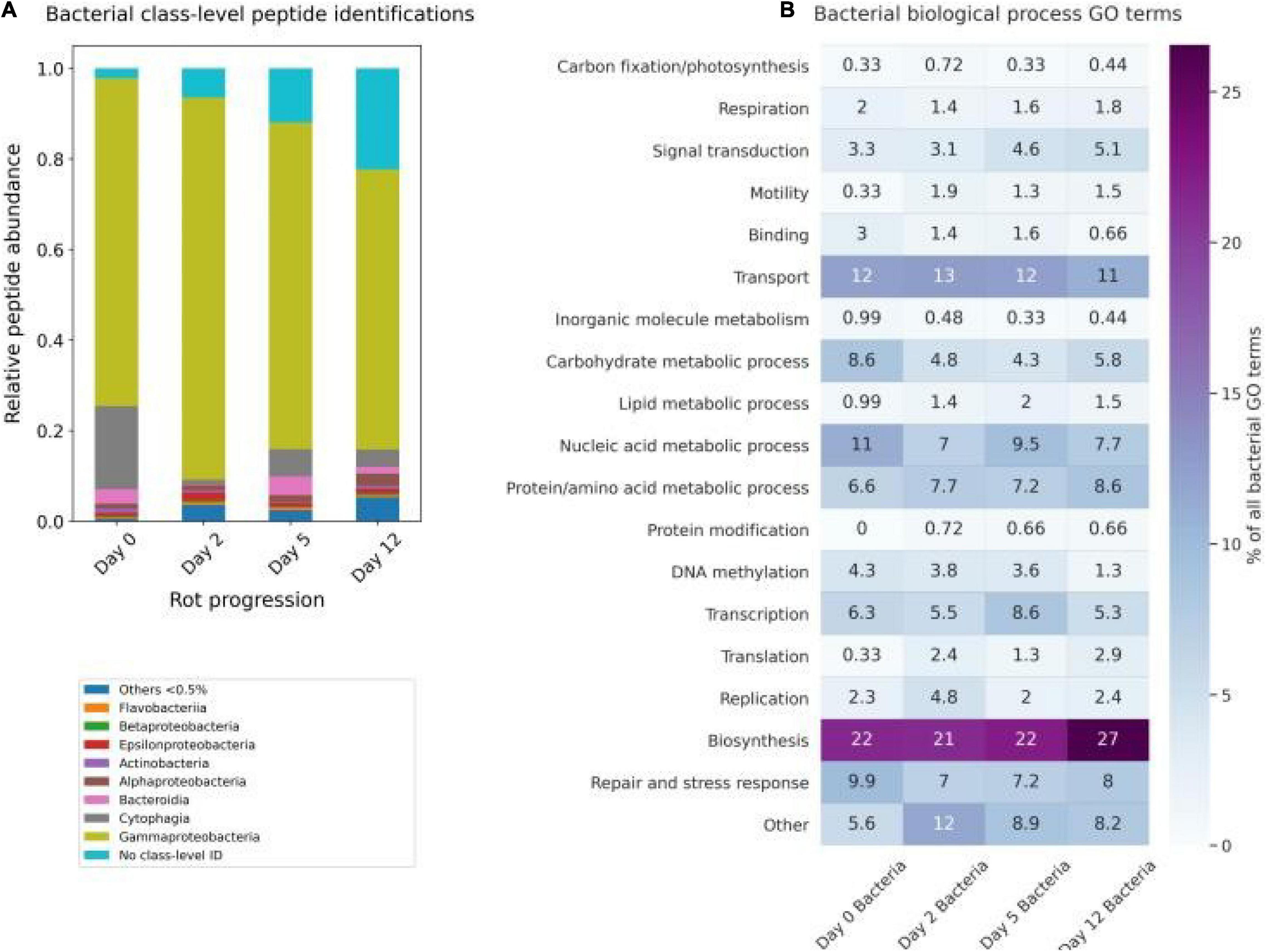
Figure 6. Bacterial progression of the 12-day algal degradation. (A) Relative abundance contribution of the major bacterial taxonomic classes (>0.5%) from the time point proteomes adjusted by relative peptide area abundance. (B) The percentage of the total number of bacterial peptide biological process gene ontology (GO) terms. GO terms shown were condensed from a broader set to eliminate redundancy for ease of visualization using the REViGO (available http://revigo.irb.hr/) and further manually organized into broader categories.
There is taxonomic overlap of the initial composition in this study to that of a pyrosequencing survey of planktonic microbes at three stations in the Gulf of Maine (Li et al., 2011), though it is worth noting that no estuarine sites were included in that work. We found the microbial community at the initial time point was dominated by Gammaproteobacteria (∼60% of the peptides), with notable contributions of Cytophaga (∼5%), Bacteroidia (∼2%), and Alphaproteobacteria (∼2%) (Figure 6A). While Li et al. (2011) survey found clades of SAR11 to dominate surface seawater in the open waters of the Gulf of Maine, some Gammaproteobacteria are symbionts of diatoms (Amin et al., 2012). Since we didn’t sample the seawater itself for peptidomics, just the filtered diatom rot slurry, the dominance of a diatom symbiont makes sense. During the 12-day experiment, the microbial community composition changed minimally, with two exceptions: Firstly, there was a 10-fold increase of peptides that were bacterial but could not be uniquely identified at the class level or below, from 2.2% of peptides on day 0 to 22% of peptides by day 12 (Figure 6A). At the same time, the contribution of low abundance (<0.5% of all peptides) classes increased from 0.53% on day 0 to 5.4% on day 12 (Figure 6A). Both increases, in non-specific bacterial peptides and in low-abundance class peptides, are potential indications that the diversity of the bacterial community was increasing during the experiment.
The increase of non-specific bacterial peptides can be explained in two ways. Most likely, a true increase in diversity and richness resulted from a broader array of shared, conserved proteins being produced in the system by related bacteria. An alternative hypothesis is that there was an ingrowth of bacterial necromass generating degraded bacterial peptides that are no longer very taxonomically specific. Bacterial necromass in this case could be from organisms in the seawater inoculum or bacteria present during the diatom culture growth. For instance, Gammaproteobacteria are known to thrive within the phycosphere of diatoms (Amin et al., 2012), and their peptide abundance peaked at day 2 when the active bacteria were growing exponentially. It is difficult to discern which of these hypotheses is correct and further work will need to be done to evaluate the processing of bacterial detritus by other bacteria. Nonetheless, the overall taxonomic changes observed in the bacterial peptidome during the 12 days were minimal.
Unlike the somewhat ambiguous taxonomic information within the bacterial peptidome, biological process GO terms associated with the bacterial peptides provide a clearer view of how the bacterial community responded to the algal detritus (Figure 6B). The most commonly detected GO terms are associated with transmembrane transport, carbohydrate metabolism, and DNA replication and transcription (Figure 6B). These are the terms most strongly associated with bacterial growth (Mikan et al., 2020) and suggest that most of the bacterial peptides detected are from living bacteria. This strengthens support for the hypothesis that the Gammaproteobacteria in the samples are for the most part living and not detrital.
Our bacterial functional data are generally consistent with recent work by Mikan et al. (2020), who used metaproteomic tools and a GO-term based functional analysis to evaluate the heterotrophic bacterial response to a pulse of detrital organic matter in two Arctic microbiomes during 10-day shipboard incubations. In that study, the bacterial community increased protein synthesis, carbohydrate degradation, and cellular redox processes while simultaneously decreasing C1 metabolism (Mikan et al., 2020). Throughout this experiment we observe steady levels of transmembrane transport and protein metabolism terms. Carbohydrate metabolic process GO terms maximize in the first 2 days of the degradation, with increasing biosynthesis GO terms by day 12 (Figure 6B). Mikan et al. (2020) suggested that the bacterial community shifted their carbon acquisition strategies intracellularly before there were large shifts in the taxonomic structure of the community. Without a paired metagenome or metatranscriptome with which to perform proteomic database searches, these bacterial peptide data and paired GO term data are not as complete, but show the same general trends, lending further support to the notion of Mikan et al. (2020) that functional composition and redundancy, not taxonomy, may be the most relevant factor when evaluating how effectively organic matter is or will be processed by bacteria in the ocean.
Conclusion and Future Directions
In this study we evaluated proteinaceous material in a marine system both with and without the use of trypsin as an extraction and identification tool. To our knowledge, this is the first such attempt to disentangle proteins that are being degraded by microbes from those that are resistant to degradation. We show that proteins from specific cellular locations are preferentially preserved during the initial stages of degradation. As has been hypothesized and demonstrated for bacterial membrane proteins (Nagata et al., 1998; Kaiser and Benner, 2008; Jiao et al., 2010) and algal membrane proteins (Laursen et al., 1996; Nguyen and Harvey, 2001; Wolfe et al., 2006), we conclusively illustrate that proteins associated with diatom chloroplasts and membranes resist initial degradation better than those without such association. The many membrane-associated and few cytoplasm peptides that resist degradation also are relatively enriched in ɑ-helices and depleted in β-strands, consistent with the cellular location data since ɑ-helices are enriched in membrane proteins. However, the extent to which ɑ-helices themselves lead to degradation resistance remains to be more thoroughly evaluated, as (1) ɑ-helices are not exclusive to membrane proteins and (2) there could be other reasons for membrane protein survival over time that causes membrane proteins’ ɑ-helix rich motifs to become enriched in detrital material.
The novel application of proteomics without the use of trypsin also allowed for the evaluation of how PTMs relate to protein degradation. We found that the oxidation and acetylation PTMs observed likely originated within the living cell, with only asparagine deamidation and arginine methylation being predominantly associated with the degraded peptide pool. We hypothesize that PTMs have an impact on the bioavailability of peptides during early diagenesis, but again more work is needed to evaluate the extent to which PTMs provide protection. In all, we add to earlier evidence of selective protein degradation mechanisms enabled by proteomic techniques (Dong et al., 2010; Nunn et al., 2010; Moore et al., 2012, 2014; Bridoux et al., 2015). Continued advancements in metaproteomic instrumentation and computational capabilities have great potential to better our understanding of protein degradation and preservation dynamics in the ocean.
Data Availability Statement
The datasets presented in this study can be found in online repositories. The names of the repository/repositories and accession number(s) can be found below: https://www.ebi.ac.uk/pride/archive/PXD027843.
Author Contributions
CA conducted algal degradation experiments. LM designed and directed algal degradation experiments. KH and JN contributed to proteomics sample preparation. MD conducted all proteomics data analyses. RK directed proteomics experiments. MD wrote the manuscript with input from all co-authors. All authors contributed to the article and approved the submitted version.
Funding
Funding for this research came from the National Science Foundation (OCE 1542240 to LM and RK) and the NSF Graduate Research Fellowship Program (DGE-1762114 to MD).
Conflict of Interest
The authors declare that the research was conducted in the absence of any commercial or financial relationships that could be construed as a potential conflict of interest.
Publisher’s Note
All claims expressed in this article are solely those of the authors and do not necessarily represent those of their affiliated organizations, or those of the publisher, the editors and the reviewers. Any product that may be evaluated in this article, or claim that may be made by its manufacturer, is not guaranteed or endorsed by the publisher.
Acknowledgments
We are very grateful for the assistance of Priska von Haller at the University of Washington Proteomics Resource Center to Laura Carlson and Anitra Ingalls for THAA measurements. We also thank Eli Moore for his useful points in conversation.
Supplementary Material
The Supplementary Material for this article can be found online at: https://www.frontiersin.org/articles/10.3389/fmars.2021.757245/full#supplementary-material
Footnotes
References
Abdulla, H. A., Burdige, D. J., and Komada, T. (2018). Accumulation of deaminated peptides in anoxic sediments of Santa Barbara Basin. Geochim. Cosmochim. Acta 223, 245–258. doi: 10.1016/j.gca.2017.11.021
Adams, C., Mayer, L., Rawson, P., Brady, D., and Newell, C. (2019). Detrital protein contributes to oyster nutrition and growth in the Damariscotta estuary, Maine, USA. Aquac. Environ. Interact. 11, 521–536. doi: 10.3354/aei00330
Alley, W. R., Mann, B. F., and Novotny, M. V. (2013). High-sensitivity analytical approaches for the structural characterization of glycoproteins. Chem. Rev. 113, 2668–2732. doi: 10.1021/cr3003714
Allmer, J. (2011). Algorithms for the de novo sequencing of peptides from tandem mass spectra. Expert Rev. Proteomics 8, 645–657. doi: 10.1586/epr.11.54
Aluwihare, L. I., Repeta, D. J., and Chen, R. F. (1997). A major biopolymeric component to dissolved organic carbon in surface sea water. Nature 387, 166–169. doi: 10.1038/387166a0
Amin, S. A., Parker, M. S., and Armbrust, E. V. (2012). Interactions between diatoms and bacteria. Microbiol. Mol. Biol. Rev. 76, 667–684. doi: 10.1128/MMBR.00007-12
Aro, E.-M., Suorsa, M., Rokka, A., Allahverdiyeva, Y., Paakkarinen, V., Saleem, A., et al. (2005). Dynamics of photosystem II: a proteomic approach to thylakoid protein complexes. J. Exp. Bot. 56, 347–356. doi: 10.1093/jxb/eri041
Benner, R., and Kaiser, K. (2003). Abundance of amino sugars and peptidoglycan in marine particulate and dissolved organic matter. Limnol. Oceanogr. 48, 118–128. doi: 10.4319/lo.2003.48.1.0118
Bergauer, K., Fernandez-Guerra, A., Garcia, J. A. L., Sprenger, R. R., Stepanauskas, R., Pachiadaki, M. G., et al. (2017). Organic matter processing by microbial communities throughout the Atlantic water column as revealed by metaproteomics. Proc. Natl. Acad. Sci. U.S.A. 115, E400–E408. doi: 10.1073/pnas.1708779115
Bridoux, M., Neibauer, J., Ingalls, A., Nunn, B., and Keil, R. (2015). Suspended marine particulate proteins in coastal and oligotrophic waters. J. Mar. Syst. 143, 39–48. doi: 10.1016/j.jmarsys.2014.10.014
Burdige, D. J. (2007). Preservation of organic matter in marine sediments: controls, mechanisms, and an imbalance in sediment organic carbon budgets? Chem. Rev. 107, 467–485. doi: 10.1021/cr050347q
Cain, J. A., Solis, N., and Cordwell, S. J. (2014). Beyond gene expression: the impact of protein post-translational modifications in bacteria. J. Proteomics 97, 265–286. doi: 10.1016/j.jprot.2013.08.012
Chen, X.-H., Li, Y.-Y., Zhang, H., Liu, J.-L., Xie, Z.-X., Lin, L., et al. (2018). Quantitative proteomics reveals common and specific responses of a marine diatom Thalassiosira pseudonana to different macronutrient deficiencies. Front. Microbiol. 9:2761. doi: 10.3389/fmicb.2018.02761
Chen, Z., Luo, L., Chen, R., Hu, H., Pan, Y., Jiang, H., et al. (2018). Acetylome profiling reveals extensive lysine acetylation of the fatty acid metabolism pathway in the diatom Phaeodactylum tricornutum. Mol. Cell. Proteomics 17, 399–412. doi: 10.1074/mcp.RA117.000339
Collins, M. J., Westbroek, P., Muyzer, G., and de Leeuw, J. W. (1992). Experimental evidence for condensation reactions between sugars and proteins in carbonate skeletons. Geochim. Cosmochim. Acta 56, 1539–1544. doi: 10.1016/0016-7037(92)90223-6
Cowie, G. L., and Hedges, J. I. (1992). Improved amino acid quantification in environmental samples: charge-matched recovery standards and reduced analysis time. Mar. Chem. 37, 223–238. doi: 10.1016/0304-4203(92)90079-P
Cowie, G. L., and Hedges, J. I. (1994). Biochemical indicators of diagenetic alteration in natural organic matter mixtures. Nature 369, 304–307. doi: 10.1038/369304a0
Dauwe, B., and Middelburg, J. J. (1998). Amino acids and hexosamines as indicators of organic matter degradation state in North Sea sediments. Limnol. Oceanogr. 43, 782–798. doi: 10.4319/lo.1998.43.5.0782
Dauwe, B., Middelburg, J. J., Herman, P. M. J., and Heip, C. H. R. (1999). Linking diagenetic alteration of amino acids and bulk organic matter reactivity. Limnol. Oceanogr. 44, 1809–1814. doi: 10.4319/lo.1999.44.7.1809
Dhillon, R. S., and Denu, J. M. (2017). Using comparative biology to understand how aging affects mitochondrial metabolism. Mol. Cell. Endocrinol. 455, 54–61. doi: 10.1016/j.mce.2016.12.020
Dong, H.-P., Wang, D.-Z., Dai, M., and Hong, H.-S. (2010). Characterization of particulate organic matter in the water column of the South China Sea using a shotgun proteomic approach. Limnol. Oceanogr. 55, 1565–1578. doi: 10.4319/lo.2010.55.4.1565
Duffy, M. E., Neibauer, J. A., Adams, J., Lundeen, R. A., Rocap, G., Ingalls, A. E., et al. (in press). Protein cycling in the eastern tropical North Pacific oxygen deficient zone: ade novo-discovery peptidomic approach. Limnol. Oceanogr.
Dyhrman, S. T., Jenkins, B. D., Rynearson, T. A., Saito, M. A., Mercier, M. I., Alexander, H., et al. (2012). The transcriptome and proteome of the diatom Thalassiosira pseudonana reveal a diverse phosphorus stress response. PLoS One 7:e33768. doi: 10.1371/journal.pone.0033768
Elias, J. E., and Gygi, S. P. (2010). Target-decoy search strategy for mass spectrometry-based proteomics. Methods Mol. Biol. 604, 55–71. doi: 10.1007/978-1-60761-444-9_5
Estes, E. R., Pockalny, R., D’Hondt, S., Inagaki, F., Morono, Y., Murray, R. W., et al. (2019). Persistent organic matter in oxic subseafloor sediment. Nat. Geosci. 12, 126–131. doi: 10.1038/s41561-018-0291-5
Fejjar, S., Melanson, A., and Tremblay, L. (2021). Pore waters as a contributor to deep-water amino acids and to deep-water dissolved organic matter concentration and composition in estuarine and marine waters. Mar. Chem. 233:103985. doi: 10.1016/j.marchem.2021.103985
Finkel, Z. V., Follows, M. J., Liefer, J. D., Brown, C. M., Benner, I., and Irwin, A. J. (2016). Phylogenetic diversity in the macromolecular composition of microalgae. PLoS One 11:e0155977. doi: 10.1371/journal.pone.0155977
Galetskiy, D., Lohscheider, J. N., Kononikhin, A. S., Popov, I. A., Nikolaev, E. N., and Adamska, I. (2011). Mass spectrometric characterization of photooxidative protein modifications in Arabidopsis thaliana thylakoid membranes. Rapid Commun. Mass Spectrom. 25, 184–190. doi: 10.1002/rcm.4855
Ghesquière, B., Jonckheere, V., Colaert, N., Van Durme, J., Timmerman, E., Goethals, M., et al. (2011). Redox proteomics of protein-bound methionine oxidation. Mol. Cell. Proteomics 10:M110.006866. doi: 10.1074/mcp.M110.006866
Gray, N., Zia, R., King, A., Patel, V. C., Wendon, J., McPhail, M. J. W., et al. (2017). High-speed quantitative UPLC-MS analysis of multiple amines in human plasma and serum via precolumn derivatization with 6-aminoquinolyl-N-hydroxysuccinimidyl carbamate: application to acetaminophen-induced liver failure. Anal. Chem. 89, 2478–2487. doi: 10.1021/acs.analchem.6b04623
Gurdeep Singh, R., Tanca, A., Palomba, A., Van der Jeugt, F., Verschaffelt, P., Uzzau, S., et al. (2019). Unipept 4.0: functional analysis of metaproteome data. J. Proteome Res. 18, 606–615. doi: 10.1021/acs.jproteome.8b00716
Han, X., He, L., Xin, L., Shan, B., and Ma, B. (2011). PeaksPTM: mass spectrometry-based identification of peptides with unspecified modifications. J. Proteome Res. 10, 2930–2936. doi: 10.1021/pr200153k
Hedges, J. I., Baldock, J. A., Gélinas, Y., Lee, C., Peterson, M., and Wakeham, S. G. (2001). Evidence for non-selective preservation of organic matter in sinking marine particles. Nature 409, 801–804. doi: 10.1038/35057247
Hollibaugh, J. T., and Azam, F. (1983). Microbial degradation of dissolved proteins in seawater1. Limnol. Oceanogr. 28, 1104–1116. doi: 10.4319/lo.1983.28.6.1104
Hoppe, H.-G., Arnosti, C., and Herndl, G. (2002). “Ecological significance of bacterial enzymes in the marine environment,” in Enzymes in the Environment, eds R. G. Burns and R. P. Dick (New York, NY: Marcel Dekker). doi: 10.1201/9780203904039.ch3
Jiao, N., Herndl, G. J., Hansell, D. A., Benner, R., Kattner, G., Wilhelm, S. W., et al. (2010). Microbial production of recalcitrant dissolved organic matter: long-term carbon storage in the global ocean. Nat. Rev. Microbiol. 8, 593–599. doi: 10.1038/nrmicro2386
Kaiser, K., and Benner, R. (2008). Major bacterial contribution to the ocean reservoir of detrital organic carbon and nitrogen. Limnol. Oceanogr. 53, 99–112. doi: 10.4319/lo.2008.53.1.0099
Keeling, P. J., Burki, F., Wilcox, H. M., Allam, B., Allen, E. E., Amaral-Zettler, L. A., et al. (2014). The Marine Microbial Eukaryote Transcriptome Sequencing Project (MMETSP): illuminating the functional diversity of eukaryotic life in the oceans through transcriptome sequencing. PLoS Biol. 12:e1001889. doi: 10.1371/journal.pbio.1001889
Keil, R. G., and Kirchman, D. L. (1992). Bacterial hydrolysis of protein and methylated protein and its implications for studies of protein degradation in aquatic systems. Appl. Environ. Microbiol. 58, 1374–1375.
Keil, R. G., and Kirchman, D. L. (1993). Dissolved combined amino acids: chemical form and utilization by marine bacteria. Limnol. Oceanogr. 38, 1256–1270. doi: 10.4319/lo.1993.38.6.1256
Keil, R. G., and Kirchman, D. L. (1994). Abiotic transformation of labile protein to refractory protein in sea water. Mar. Chem. 45, 187–196. doi: 10.1016/0304-4203(94)90002-7
Keil, R. G., Tsamakis, E., and Hedges, J. (2000). “Early diagenesis of particulate amino acids in marine systems,” in Perspectives in Amino Acid and Protein Geochemistry, eds G. A. Goodfriend, M. J. Collins, M. L. Fogel, S. A. Macko, and J. F. Wehmiller (Oxford: Oxford University Press), 69–82.
Kleiner, M. (2019). Metaproteomics: much more than measuring gene expression in microbial communities. MSystems 4:e00115-19. doi: 10.1128/mSystems.00115-19
Kleiner, M., Thorson, E., Sharp, C. E., Dong, X., Liu, D., Li, C., et al. (2017). Assessing species biomass contributions in microbial communities via metaproteomics. Nat. Commun. 8:1558. doi: 10.1038/s41467-017-01544-x
Knicker, H., del Río, J. C., Hatcher, P. G., and Minard, R. D. (2001). Identification of protein remnants in insoluble geopolymers using TMAH thermochemolysis/GC–MS. Org. Geochem. 32, 397–409. doi: 10.1016/S0146-6380(00)00186-8
Knicker, H., Scaroni, A. W., and Hatcher, P. G. (1996). 13C and 15N NMR spectroscopic investigation on the formation of fossil algal residues. Org. Geochem. 24, 661–669. doi: 10.1016/0146-6380(96)00057-5
Laskay, ÜA., Lobas, A. A., Srzentić, K., Gorshkov, M. V., and Tsybin, Y. O. (2013). Proteome digestion specificity analysis for rational design of extended bottom-up and middle-down proteomics experiments. J. Proteome Res. 12, 5558–5569. doi: 10.1021/pr400522h
Laursen, A. K., Mayer, L. M., and Townsend, D. W. (1996). Lability of proteinaceous material in estuarine seston and subcellular fractions of phytoplankton. Mar. Ecol. Prog. Ser. 136, 227–234. doi: 10.3354/meps136227
Lee, C., Wakeham, S., and Arnosti, C. (2004). Particulate organic matter in the sea: the composition conundrum. AMBIO 33, 565–575. doi: 10.1579/0044-7447-33.8.565
Lehtimäki, N., Koskela, M. M., and Mulo, P. (2015). Posttranslational modifications of chloroplast proteins: an emerging field. Plant Physiol. 168, 768–775. doi: 10.1104/pp.15.00117
Leprevost, F. V., Valente, R. H., Lima, D. B., Perales, J., Melani, R., Yates, J. R., et al. (2014). PepExplorer: a similarity-driven tool for analyzing de novo sequencing results. Mol. Cell. Proteomics 13, 2480–2489. doi: 10.1074/mcp.M113.037002
Li, W. K. W., Andersen, R. A., Gifford, D. J., Incze, L. S., Martin, J. L., Pilskaln, C. H., et al. (2011). Planktonic microbes in the Gulf of Maine area. PLoS One 6:e20981. doi: 10.1371/journal.pone.0020981
Liu, W., Eilers, M., Patel, A. B., and Smith, S. O. (2004). Helix packing moments reveal diversity and conservation in membrane protein structure. J. Mol. Biol. 337, 713–729. doi: 10.1016/j.jmb.2004.02.001
Liu, Z., Kobiela, M. E., McKee, G. A., Tang, T., Lee, C., Mulholland, M. R., et al. (2010). The effect of chemical structure on the hydrolysis of tetrapeptides along a river-to-ocean transect: AVFA and SWGA. Mar. Chem. 119, 108–120. doi: 10.1016/j.marchem.2010.01.005
Mayer, L. M. (1994). Relationships between mineral surfaces and organic carbon concentrations in soils and sediments. Chem. Geol. 114, 347–363. doi: 10.1016/0009-2541(94)90063-9
Mayer, L. M., Linda L, S., Sawyer, T., Plante, C. J., Jumars, P. A., and Sel, R. L. (1995). Bioavailable amino acids in sediments: a biomimetic, kinetics based approach. Limnol. Oceanogr. 40, 511–520. doi: 10.4319/lo.1995.40.3.0511
Mayer, L. M., Macko, S. A., and Cammen, L. (1988). Provenance, concentrations and nature of sedimentary organic nitrogen in the Gulf of Maine. Mar. Chem. 25, 291–304. doi: 10.1016/0304-4203(88)90056-4
Mayer, L. M., Schick, L. L., and Setchell, F. W. (1986). Measurement of protein in nearshore marine sediments. Mar. Ecol. Prog. Ser. 30, 159–165. doi: 10.3354/meps030159
Mellacheruvu, D., Wright, Z., Couzens, A. L., Lambert, J.-P., St-Denis, N., Li, T., et al. (2013). The CRAPome: a contaminant repository for affinity purification mass spectrometry data. Nat. Methods 10, 730–736. doi: 10.1038/nmeth.2557
Mesuere, B., Willems, T., der Jeugt, F. V., Devreese, B., Vandamme, P., and Dawyndt, P. (2016). Unipept web services for metaproteomics analysis. Bioinformatics 32, 1746–1748. doi: 10.1093/bioinformatics/btw039
Mikan, M. P., Harvey, H. R., Timmins-Schiffman, E., Riffle, M., May, D. H., Salter, I., et al. (2020). Metaproteomics reveal that rapid perturbations in organic matter prioritize functional restructuring over taxonomy in western Arctic Ocean microbiomes. ISME J. 14, 39–52. doi: 10.1038/s41396-019-0503-z
Montgomerie, S., Cruz, J. A., Shrivastava, S., Arndt, D., Berjanskii, M., and Wishart, D. S. (2008). PROTEUS2: A web server for comprehensive protein structure prediction and structure-based annotation. Nucleic Acids Res. 36(Suppl_2), W202–W209. doi: 10.1093/nar/gkn255
Moore, E. K., Harvey, H. R., Faux, J. F., Goodlett, D. R., and Nunn, B. L. (2014). Protein recycling in Bering Sea algal incubations. Mar. Ecol. Prog. Ser. 515, 45–59. doi: 10.3354/meps10936
Moore, E. K., Nunn, B. L., Goodlett, D. R., and Harvey, H. R. (2012). Identifying and tracking proteins through the marine water column: Insights into the inputs and preservation mechanisms of protein in sediments. Geochim. Cosmochim. Acta 83, 324–359. doi: 10.1016/j.gca.2012.01.002
Mulholland, M., and Lee, C. (2009). Peptide hydrolysis and the uptake of dipeptides by phytoplankton. Limnol. Oceanogr. 54, 856–868. doi: 10.4319/lo.2009.54.3.0856
Muth, T., Hartkopf, F., Vaudel, M., and Renard, B. Y. (2018). A potential golden age to come-current tools, recent use cases, and future avenues for de novo sequencing in proteomics. Proteomics 18:e1700150. doi: 10.1002/pmic.201700150
Muth, T., Kolmeder, C. A., Salojärvi, J., Keskitalo, S., Varjosalo, M., Verdam, F. J., et al. (2015). Navigating through metaproteomics data: a logbook of database searching. Proteomics 15, 3439–3453. doi: 10.1002/pmic.201400560
Nagata, T., and Kirchman, D. (1996). Bacterial degradation of protein adsorbed to model submicron particles in seawater. Mar. Ecol. Prog. Ser. 132, 241–248. doi: 10.3354/meps132241
Nagata, T., Fukuda, R., Koike, I., Kogure, K., and Kirchman, D. (1998). Degradation by bacteria of membrane and soluble protein in seawater. Aquat. Microb. Ecol. 14, 29–37. doi: 10.3354/ame014029
Nguyen, R. T., and Harvey, H. R. (2001). Preservation of protein in marine systems: hydrophobic and other noncovalent associations as major stabilizing forces. Geochim. Cosmochim. Acta 65, 1467–1480. doi: 10.1016/S0016-7037(00)00621-9
Noble, W. S. (2015). Mass spectrometrists should only search for peptides they care about. Nat. Methods 12, 605–608. doi: 10.1038/nmeth.3450
Nunn, B. L., Norbeck, A., and Keil, R. G. (2003). Hydrolysis patterns and the production of peptide intermediates during protein degradation in marine systems. Mar. Chem. 83, 59–73. doi: 10.1016/S0304-4203(03)00096-3
Nunn, B. L., Ting, Y. S., Malmström, L., Tsai, Y. S., Squier, A., Goodlett, D. R., et al. (2010). The path to preservation: using proteomics to decipher the fate of diatom proteins during microbial degradation. Limnol. Oceanogr. 55, 1790–1804. doi: 10.4319/lo.2010.55.4.1790
Obayashi, Y., and Suzuki, S. (2008). Occurrence of exo- and endopeptidases in dissolved and particulate fractions of coastal seawater. Aquat. Microb. Ecol. 50, 231–237. doi: 10.3354/ame01169
O’Bryon, I., Jenson, S. C., and Merkley, E. D. (2020). Flying blind, or just flying under the radar? The underappreciated power of de novo methods of mass spectrometric peptide identification. Protein Sci. 29, 1864–1878. doi: 10.1002/pro.3919
Oleschuk, R. D., McComb, M. E., Chow, A., Ens, W., Standing, K. G., Perreault, H., et al. (2000). Characterization of plasma proteins adsorbed onto biomaterials by MALDI-TOFMS. Biomaterials 21, 1701–1710. doi: 10.1016/S0142-9612(00)00054-5
Olofsson, M., Lindehoff, E., and Legrand, C. (2019). Production stability and biomass quality in microalgal cultivation – contribution of community dynamics. Eng. Life Sci. 19, 330–340. doi: 10.1002/elsc.201900015
Ovesen, R. G., Nielsen, J., and Bruun Hansen, H. C. (2011). Biomedicine in the environment: sorption of the cyclotide kalata B2 to montmorillonite, goethite, and humic acid. Environ. Toxicol. Chem. 30, 1785–1792. doi: 10.1002/etc.579
Pantoja, S., Rossel, P., Castro, R., Cuevas, L. A., Daneri, G., and Córdova, C. (2009). Microbial degradation rates of small peptides and amino acids in the oxygen minimum zone of Chilean coastal waters. Deep Sea Res. II 56, 1055–1062. doi: 10.1016/j.dsr2.2008.09.007
Reinfelder, J. R., and Fisher, N. S. (1991). The assimilation of elements ingested by marine copepods. Science 251, 794–796. doi: 10.1126/science.251.4995.794
Riffle, M., May, D. H., Timmins-Schiffman, E., Mikan, M. P., Jaschob, D., Noble, W. S., et al. (2017). MetaGOmics: a web-based tool for peptide-centric functional and taxonomic analysis of metaproteomics data. Proteomes 6:2. doi: 10.3390/proteomes6010002
Roth, L., and Harvey, H. (2006). Intact protein modification and degradation in estuarine environments. Mar. Chem. 106, 33–45. doi: 10.1016/J.MARCHEM.2005.10.025
Saito, M. A., Bertrand, E. M., Duffy, M. E., Gaylord, D. A., Held, N. A., Hervey, W. J., et al. (2019). Progress and challenges in ocean metaproteomics and proposed best practices for data sharing. J. Proteome Res. 18, 1461–1476. doi: 10.1021/acs.jproteome.8b00761
Sakai, H., and Tsukihara, T. (1998). Structures of membrane proteins determined at atomic resolution. J. Biochem. 124, 1051–1059.
Schmidt, F., Koch, B. P., Elvert, M., Schmidt, G., Witt, M., and Hinrichs, K.-U. (2011). Diagenetic transformation of dissolved organic nitrogen compounds under contrasting sedimentary redox conditions in the Black Sea. Environ. Sci. Technol. 45, 5223–5229. doi: 10.1021/es2003414
Shamblin, S. L., Hancock, B. C., and Zografi, G. (1998). Water vapor sorption by peptides, proteins and their formulations. Eur. J. Pharm. Biopharm. 45, 239–247. doi: 10.1016/S0939-6411(98)00006-X
Shen, Y., Hixson, K., Tolić, N., Camp, D., Purvine, S., Moore, R. J., et al. (2008). Mass spectrometry analysis of proteome-wide proteolytic post-translational degradation of proteins. Anal. Chem. 80, 5819–5828. doi: 10.1021/ac800077w
Smith, L. J., Fiebig, K. M., Schwalbe, H., and Dobson, C. M. (1996). The concept of a random coil: residual structure in peptides and denatured proteins. Fold. Des. 1, R95–R106. doi: 10.1016/S1359-0278(96)00046-6
Stevens, T. J., and Arkin, I. T. (2000). Do more complex organisms have a greater proportion of membrane proteins in their genomes? Proteins 39, 417–420. doi: 10.1002/(SICI)1097-0134(20000601)39:4<417::AID-PROT140<3.0.CO;2-Y
Suzuki, S., Kogure, K., and Tanoue, E. (1997). Immunochemical detection of dissolved proteins and their source bacteria in marine environments. Mar. Ecol. Prog. Ser. 158, 1–9. doi: 10.3354/meps158001
Tanoue, E., Nishiyama, S., Kamo, M., and Tsugita, A. (1995). Bacterial membranes: possible source of a major dissolved protein in seawater. Geochim. Cosmochim. Acta 59, 2643–2648. doi: 10.1016/0016-7037(95)00134-4
The UniProt Consortium (2018). UniProt: the universal protein knowledgebase. Nucleic Acids Res. 46, 2699–2699. doi: 10.1093/nar/gky092
Timmins-Schiffman, E., May, D. H., Mikan, M., Riffle, M., Frazar, C., Harvey, H. R., et al. (2017). Critical decisions in metaproteomics: achieving high confidence protein annotations in a sea of unknowns. ISME J. 11, 309–314. doi: 10.1038/ismej.2016.132
Van Mooy, B. A. S., Keil, R. G., and Devol, A. H. (2002). Impact of suboxia on sinking particulate organic carbon: enhanced carbon flux and preferential degradation of amino acids via denitrification. Geochim. Cosmochim. Acta 66, 457–465. doi: 10.1016/S0016-7037(01)00787-6
Wakeham, S. G., Lee, C., Hedges, J. I., Hernes, P. J., and Peterson, M. J. (1997). Molecular indicators of diagenetic status in marine organic matter. Geochim. Cosmochim. Acta 61, 5363–5369. doi: 10.1016/S0016-7037(97)00312-8
Weiss, M. S., Abele, U., Weckesser, J., Welte, W., Schiltz, E., and Schulz, G. E. (1991). Molecular architecture and electrostatic properties of a bacterial porin. Science 254, 1627–1630. doi: 10.1126/science.1721242
Wolfe, A., Edlund, M., Sweet, A., and Creighton, S. (2006). A first account of organelle preservation in Eocene nonmarine diatoms: observations and paleobiological implications. Palaios 21, 298–304. doi: 10.2110/palo.2005.p05-14e
Yamada, N., and Tanoue, E. (2003). Detection and partial characterization of dissolved glycoproteins in oceanic waters. Limnol. Oceanogr. 48, 1037–1048. doi: 10.4319/lo.2003.48.3.1037
Yeagle, P. L., Bennett, M., Lemaître, V., and Watts, A. (2007). Transmembrane helices of membrane proteins may flex to satisfy hydrophobic mismatch. Biochim. Biophys. Acta 1768, 530–537. doi: 10.1016/j.bbamem.2006.11.018
Yooseph, S., Sutton, G., Rusch, D. B., Halpern, A. L., Williamson, S. J., Remington, K., et al. (2007). The Sorcerer II global ocean sampling expedition: expanding the universe of protein families. PLoS Biol. 5:e16. doi: 10.1371/journal.pbio.0050016
Zhang, J., Xin, L., Shan, B., Chen, W., Xie, M., Yuen, D., et al. (2012). PEAKS DB: De novo sequencing assisted database search for sensitive and accurate peptide identification. Mol. Cell. Proteomics 11:M111.010587. doi: 10.1074/mcp.M111.010587
Keywords: marine organic matter, amino acids (AA), protein recycling, protein degradation, marine metaproteomics, post-translational modifications (PTM), early diagenesis, phytoplankton bloom degradation
Citation: Duffy ME, Adams CM, Homolka KK, Neibauer JA, Mayer LM and Keil RG (2022) Degradation of Diatom Protein in Seawater: A Peptide-Level View. Front. Mar. Sci. 8:757245. doi: 10.3389/fmars.2021.757245
Received: 12 August 2021; Accepted: 07 December 2021;
Published: 21 January 2022.
Edited by:
Jin Liu, Peking University, ChinaReviewed by:
Weichao Huang, Carnegie Institution for Science (CIS), United StatesXin Lin, Xiamen University, China
Copyright © 2022 Duffy, Adams, Homolka, Neibauer, Mayer and Keil. This is an open-access article distributed under the terms of the Creative Commons Attribution License (CC BY). The use, distribution or reproduction in other forums is permitted, provided the original author(s) and the copyright owner(s) are credited and that the original publication in this journal is cited, in accordance with accepted academic practice. No use, distribution or reproduction is permitted which does not comply with these terms.
*Correspondence: Megan E. Duffy, duffyme@uw.edu
†ORCID: Megan E. Duffy, orcid.org/0000-0002-3212-4927; Khadijah K. Homolka, orcid.org/0000-0002-5208-8740; Jacquelyn A. Neibauer, orcid.org/0000-0001-9920-2558; Richard G. Keil, orcid.org/0000-0001-7483-1606