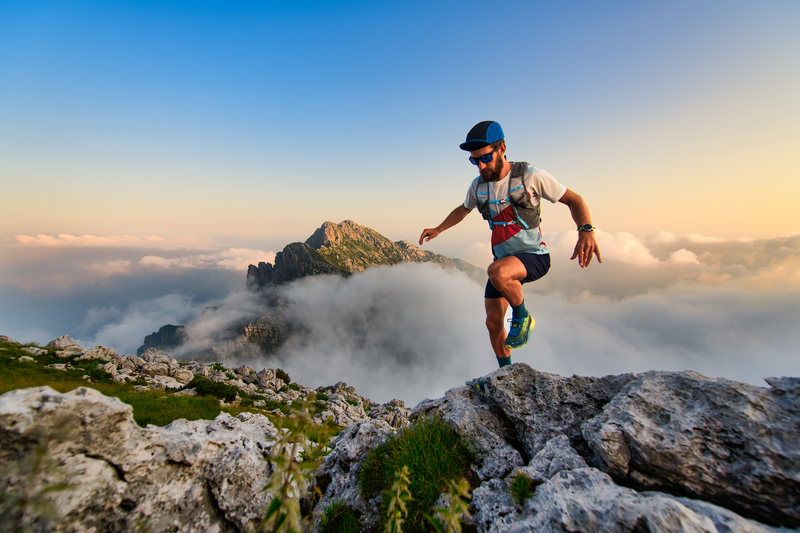
95% of researchers rate our articles as excellent or good
Learn more about the work of our research integrity team to safeguard the quality of each article we publish.
Find out more
REVIEW article
Front. Mar. Sci. , 05 November 2021
Sec. Ocean Observation
Volume 8 - 2021 | https://doi.org/10.3389/fmars.2021.751840
This article is part of the Research Topic Best Practices in Ocean Observing View all 85 articles
Marine animals equipped with biological and physical electronic sensors have produced long-term data streams on key marine environmental variables, hydrography, animal behavior and ecology. These data are an essential component of the Global Ocean Observing System (GOOS). The Animal Borne Ocean Sensors (AniBOS) network aims to coordinate the long-term collection and delivery of marine data streams, providing a complementary capability to other GOOS networks that monitor Essential Ocean Variables (EOVs), essential climate variables (ECVs) and essential biodiversity variables (EBVs). AniBOS augments observations of temperature and salinity within the upper ocean, in areas that are under-sampled, providing information that is urgently needed for an improved understanding of climate and ocean variability and for forecasting. Additionally, measurements of chlorophyll fluorescence and dissolved oxygen concentrations are emerging. The observations AniBOS provides are used widely across the research, modeling and operational oceanographic communities. High latitude, shallow coastal shelves and tropical seas have historically been sampled poorly with traditional observing platforms for many reasons including sea ice presence, limited satellite coverage and logistical costs. Animal-borne sensors are helping to fill that gap by collecting and transmitting in near real time an average of 500 temperature-salinity-depth profiles per animal annually and, when instruments are recovered (∼30% of instruments deployed annually, n = 103 ± 34), up to 1,000 profiles per month in these regions. Increased observations from under-sampled regions greatly improve the accuracy and confidence in estimates of ocean state and improve studies of climate variability by delivering data that refine climate prediction estimates at regional and global scales. The GOOS Observations Coordination Group (OCG) reviews, advises on and coordinates activities across the global ocean observing networks to strengthen the effective implementation of the system. AniBOS was formally recognized in 2020 as a GOOS network. This improves our ability to observe the ocean’s structure and animals that live in them more comprehensively, concomitantly improving our understanding of global ocean and climate processes for societal benefit consistent with the UN Sustainability Goals 13 and 14: Climate and Life below Water. Working within the GOOS OCG framework ensures that AniBOS is an essential component of an integrated Global Ocean Observing System.
The world’s oceans are an essential part of the global climate system Abraham et al. (2013). Central to understanding the earth’s climate is a thorough understanding of ocean physics and how the oceans are changing. Global efforts to coordinate ocean observations are overseen by the Global Ocean Observing System (GOOS), created by the Intergovernmental Oceanographic Commission (IOC) in March 1991 (Moltmann et al., 2019). This Framework for Ocean Observing (Lindstrom et al., 2012) applies a systems approach, with Essential Ocean Variables (EOVs) as a common focus. GOOS provides a formal assessment of feasibility, capacity and impact for each system component based on readiness levels, i.e., concept, pilot and mature. Enormous progress has been made in measuring the physical environment of the world’s oceans using several mature networks including those supported by GOOS; the Argo global profiling float array, GO-SHIP (repeat hydrography), SOOP (ships of opportunity), DBCP (drifters and buoys), OceanSITES (fixed moorings), and virtual constellations of satellites measuring sea surface temperature, ocean color, ocean surface topography, ocean surface vector winds, and ocean surface salinity (Moltmann et al., 2019).
Despite these major efforts, some important marine regions remain under-sampled, or challenging to observe, particularly the polar seas, coastal shelves, the world’s tropical oceans and the deep ocean (Abraham et al., 2013; von Schuckmann et al., 2016). Filling these gaps is crucial, especially in the Antarctic and Arctic high latitude oceans, that are currently changing rapidly. The Southern Ocean is considered the globally dominant region for heat and CO2 exchange (Frölicher et al., 2015). To date, the global research community has relied mainly on in situ measurements of ocean temperature from Argo profiling floats to quantify this imbalance (Roemmich et al., 2015; Riser et al., 2016). However, in the ice-covered high latitudes, in situ observations from ice-capable Argo (particularly in winter) whilst growing, are still relatively sparse (Roemmich et al., 2019; Smith et al., 2019). These observational gaps have arisen in large part due to the expense and limited seasonal access for ships to remote ice-covered regions, reducing opportunities for collecting observations (Goni et al., 2019; Roemmich et al., 2019). Moreover, although there is increasing societal need for data on ocean biogeochemistry and Biological and Ecosystem Essential Ocean Variables, e.g., genetic composition, ecosystem structure and function, species populations and individual traits, these also remain under-sampled globally.
Animal-borne oceanographic sensors are an effective way to help fill these data gaps and are rapidly becoming an essential component of an integrated GOOS (Fedak, 2013; Roquet et al., 2014, 2017; Treasure et al., 2017; Miloslavich et al., 2018; Harcourt et al., 2019; March et al., 2020). Electronic tags attached to marine animals with integrated miniaturized temperature and conductivity sensors (on average 103 per annum, Table 1) have augmented the capacity of ocean observing (Fedak, 2004, 2013) by sampling in extreme environments, e.g., under Antarctic sea-ice during the winter (Roquet et al., 2014; Treasure et al., 2017) and more recently in the world’s tropical oceans (Bousquet et al., 2020). Observations from animal-borne conductivity-temperature-depth (CTD) instruments (Roquet et al., 2013) are helping us to understand physical processes such as sea-ice formation (Charrassin et al., 2008; Tamura et al., 2016; Guo et al., 2019), Antarctic Bottom Water formation (Ohshima et al., 2013; Kitade et al., 2014; Williams et al., 2016; Mallet et al., 2018), ocean and ice shelf interactions (Silvano et al., 2016, 2018, 2019), and frontal system dynamics (Pauthenet et al., 2018; Dotto et al., 2021). They have also provided the most thorough assessment of Antarctic water mass characteristics conducted to date (Narayanan et al., 2019). Assimilation of NRT animal-borne profiles from tagged seals (Carse et al., 2015) and turtles (Miyazawa et al., 2018) into short-range ocean forecast models significantly decreased model error in some regions. This illustrates the maturity of animal telemetry as a discipline and the vital complementary role AniBOS can play in GOOS (Goto et al., 2017; Doi et al., 2019; Bousquet et al., 2020; Queste et al., 2020; Dotto et al., 2021). Marine animals in addition to the information they collect within the water column also provide essential observations from the world’s ocean surface. These animal-borne data are particularly valuable to the ocean operational and scientific communities because they complement satellite observations that can be obscured by clouds, provide comparative observations for calibrating those collected from space especially in the vicinity of tropical cyclones as shown from a study of turtles in the Indian Ocean (Bousquet et al., 2021). Animal-borne sensors also provide data on animal behavior in relation to in situ environmental conditions undertaking adaptive sampling by seeking areas of both physical and biological interest (Guinet et al., 2014; Labrousse et al., 2017a,b, 2018; Nachtsheim et al., 2019). Linking physical and biological data in this way allows us to quantify the differences in environmentally mediated behavior at the population, species and community levels (Chambault et al., 2016; Hindell et al., 2016, 2017, 2021; McMahon et al., 2017; Bestley et al., 2020; Citta et al., 2020).
Table 1. Summary of the number of Temperature-Conductivity (T-S), Temperature-Conductivity-Fluorescence (T-S-F), and Temperature (T) only tags deployed on animals between 2007 and 2021.
In addition to position, behavior, temperature and salinity, animal platforms can also collect essential biogeochemistry (chlorophyll-a and dissolved oxygen) and ecological information such as foraging efficiency (Bailleul et al., 2015; Sauzede et al., 2015; Vacquie-Garcia et al., 2017b; Harcourt et al., 2019; Baldry et al., 2020; Keates et al., 2020) that allows examination of scientific questions at the interface between physics and biology. These data streams align elegantly with other biological ocean observing network initiatives including the Biogeochemical Argo program (Claustre et al., 2019) thereby providing the basis for quantifying how physical oceanographic processes influence primary production and the distribution and abundance of mid trophic level prey fields (Goulet et al., 2019; Tournier et al., 2021), and providing information on the foraging success of predator species (Della Penna et al., 2015). Because predators integrate information across multiple trophic levels and spatio-temporal scales, they are sentinels of altered ecological dynamics arising from climate change (Hazen et al., 2019; Bestley et al., 2020).
Animal telemetry data are increasingly used for the conservation and management of marine systems (Hays et al., 2019). On their own, tracking data can elucidate spatial stock structure and transboundary movements (Maxwell et al., 2011, 2015; Hussey et al., 2015; Harrison et al., 2018; Sequeira et al., 2018), contributing to fisheries stock assessments (Block et al., 2005; Lédée et al., 2021). When combined with oceanographic information, movement data can be used to identify ecologically important areas and help guide the development of spatial management approaches such as dynamic ocean management (Hazen et al., 2018; Sequeira et al., 2019a) and provide information relevant to the creation of Marine Protected Areas (Hindell et al., 2020).
Animal Borne Ocean Sensors is the culmination of decades of scientific endeavor and technological development enabling a variety of biological and physical sensors to be incorporated into small data-logging or data-transmitting devices deployed on marine animals (Fedak et al., 2002; Fedak, 2013; Hussey et al., 2015). The number of species, studied and the amount of data being collected has proliferated in the last two decades (Fedak, 2013), requiring centralized coordination and management of metadata and data collections, and the creation and use of best practices, particularly in relation to data quality control and animal welfare. These activities will be the primary role of AniBOS. Here we present an overview of AniBOS1.
Since 2002, instrumented animals have generated and transmitted via the Global Telecommunication System (GTS) over 650,000 conductivity-temperature-depth (CTD) profiles. The animal-borne ocean observing community started as a series of independent programs to collect animal behavior and oceanographic data. However, the vast amount of generated data necessitated international coordination to provide standardized, quality-controlled data to the oceanographic community (Roquet et al., 2014). To facilitate and coordinate this effort, the Marine Mammals Exploring the Oceans Pole to Pole (MEOP2) was born as part of the International Polar Year (Roquet et al., 2017; Treasure et al., 2017). MEOP ensured that these animal-derived physical data were readily and publicly available for operational and scientific applications (Figure 1).
Figure 1. An overview schematic of the procedures followed to collect oceanographic data using instrumented marine animals (illustrated here with a southern elephant seal). After calibration, instruments are attached to the animal and records hydrographic profiles and behavioral observations during the animal’s at-sea foraging trips. The instruments transmit summarized pre-processed data via the Argos constellation of satellites. Hydrographic profiles are then delivered to the Global Telecommunication System (GTS) after near real time quality control. These near real time CTD data are made available publicly through Data Assembly Centers. See Data Management and Figure 5 below for a comprehensive description of data quality control, flow and distribution.
In November 2019, a workshop with key researchers and instrument manufacturers prepared, formalized, and outlined the structure, function, and data protocols (collection, quality control and sharing) for a global animal-borne ocean observing system. Following the workshop, an application to the GOOS Observation Coordination Group (OCG) for formal recognition as a contributing network was submitted in April 2020. AniBOS was officially recognized as an emerging GOOS network in June 2020.
The overarching goals of AniBOS are to enhance scientific understanding of our changing oceans via in situ data collection by marine animals, with the core mission of making oceanographic measurements and animal movement data from animal-borne instruments including from the most inaccessible and under sampled regions of the global ocean freely available.
The AniBOS network has four primary objectives.
1. Collect and disseminate quality controlled observations of physical and biogeochemical oceanographic data in a standardized manner that is comparable across sampling platforms.
2. Integrate AniBOS observations with existing and new/emerging GOOS networks and the CEOS (Committee on Earth Observation Satellites) satellite ocean virtual constellations.
3. Provide in situ habitat data at the scale and resolution at which animals operate in the ocean to understand how they respond to ocean variability and change.
4. Ensure the well-being of the animals carrying the sensors by following best practice(s) for animal care as outlined in the peer-reviewed ethical scientific literature.
The oceans play a central role in the earth’s climate system and are critical to life on Earth and to its expanding human population. The blue economy is worth up to USD 6 trillion a year. To ensure that the world’s oceans remain healthy and productive requires a comprehensive understanding of their physics and biology. Given the challenges to access many parts of the global ocean, there is a strong push through the Global Ocean Observing System’s Observation Coordination Group for a globally integrated complementary observing system to fill observational gaps.
The world’s oceans are vast and observing all regions, for all ocean processes of interest, is beyond any single observing network’s capacity, highlighting the need for a multi-pronged approach. March et al. (2020) assessed the gaps in oceanographic monitoring globally, highlighting those areas not covered by the global Argo observing network, currently the most comprehensive ocean observing network that provides vertical T-S profiles to 2000 m depth (Roemmich et al., 2019). However, despite Argo’s global fleet of around 4000 profiling floats, the high polar seas, shallow coastal shelves and high energy boundary currents remain under-sampled (Figure 2). This combined with the political challenges of sampling within Exclusive Economic Zones (Kraska et al., 2015; McLaughlin, 2015; Lennox et al., 2020), logistical difficulties and expense of accessing remote regions for deployments, and the high cost of some autonomous vehicles constrains where observations are made. Marine animals carrying oceanographic sensors offer realistic solutions to many of these issues because animals inhabit many of these poorly sampled regions and swim multiple times per day through the water column to depths of several hundred meters (Figures 1, 2) and are able to sample temperature and salinity at appropriate resolutions to complement observing networks such as Argo (Table 2).
Figure 2. The spatial and temporal distribution of the Argo network and animal-borne sensor observations. (A) Density distribution of Argo profiles, (B) density distribution of CTD casts from animal-borne sensors, (C) density distribution of animal telemetry records (i.e., only location observations), (D) annual number of observations generated by each instrument type. Note that animal-borne CTD data (sourced from the MEOP program) were mainly provided by the polar research community, whereas animal telemetry records from OBIS-SEAMAP (Halpin et al., 2009) highlight the potential of marine animals to cover unsampled regions from temperate and tropical regions. The OBIS-SEAMAP telemetry records were accessed and downloaded on 2nd May 2021.
Table 2. An overview comparing the attributes of AniBOS an emerging network and Argo a mature and globally comprehensive network.
OceanOPS (formerly known as JCOMMOPS) will provide key support to AniBOS for mapping coverage relative to other GOOS networks. OceanOPS does this through providing a dedicated focal point, developing specific performance monitoring tools, developing reporting capacity, promoting data and metadata harmonization and enabling operational tools (e.g., metadata integration, data distribution through the GTS and data visualization). OceanOPS also provides deployment summaries and enhances communication to GOOS through the annual OceanOPS report card.
Oceanographic data have been collected using sensors carried by a wide range of marine vertebrates, including marine mammals (phocid seals mainly), seabirds, turtles, sharks and other fish (Figure 3). The type of oceanographic data collected and the regions covered by marine animals vary depending on the taxon and the animal’s life history characteristics. While some species are deep divers (up to 2000 m) that migrate across ocean basins, others remain coastal and may only traverse the upper 100 m of the water column (Figure 3).
Figure 3. Schematic illustrating the Essential Ocean Variables (EOV) that can be measured across all latitudes by animal-borne sensors, using either archival, acoustic or satellite telemetry. These EOV provide key information about the state and dynamics of the ocean and the marine environment. The measurement of vertical temperature and salinity profiles are the most mature and constitute the core activity of AniBOS.
Phocid seals are large and can be readily captured and handled when hauled out on land or ice, facilitating attachment and recovery of telemetry devices. Tags can be easily glued to the animal’s fur or hair with epoxy (Field et al., 2012), causing minimal stress or attachment discomfort (Fedak, 2004). Elephant seals (Mirounga leonina and M. angustirostris) have been the most effective of all phocids in the collection of oceanographic data as they are deep divers, routinely diving to depths >600 m and even reaching depths as deep as 2000 m (Hindell et al., 2016), they traverse ocean basins, spend long periods at sea and have predictable cycles on land and at sea enhanced by fidelity to haul out sites (Boehlert et al., 2001; Costa, 2003; Fedak, 2004; Bailleul et al., 2010; Costa et al., 2012; Robinson et al., 2012; Hindell et al., 2016; Keates et al., 2020). Southern elephant seals also provide unprecedented sampling of coastal polynyas and regions of bottom water formation and therefore key observations on the dynamics of ocean ventilation (Ohshima et al., 2013, 2016; Malpress et al., 2017; Labrousse et al., 2018). The ice-obligated Weddell seal (Leptonychotes weddellii) is also a deep diver that often dives to the ocean floor along the Antarctic continental shelf (Piñones et al., 2019; Labrousse et al., 2021). However, individuals generally stay within a 100 km of the tagging location, providing detailed local sampling but limiting the breadth of observations compared to elephant seals (Heerah et al., 2016; Nachtsheim et al., 2019; Photopoulou et al., 2020). In contrast crabeater seals (Lobodon carcinophagus) are shallow divers (<100 m) but move extensively around the Antarctic continental shelf (Wall et al., 2007; Nachtsheim et al., 2017; Hückstädt et al., 2020), remaining almost exclusively in the pack-ice where they may sample environments not well covered by other species (Costa et al., 2008). Arctic seals; bearded (Erignathus barbatus), ringed (Pusa hispida), hooded (Cystophora cristata), and harp (Pagophilus groenlandicus) seals (Lydersen et al., 2004; Grist et al., 2014; Isachsen et al., 2014; Hamilton et al., 2018) exhibit a wide range of dive and movement patterns, with the hooded seal being the deepest diver (Vacquie-Garcia et al., 2017a). Gray (Halichoerus grypus) and harbor (Phoca vitulina) seals have collected oceanographic data in the temperate regions of the North Atlantic and North Pacific Oceans as well as in the High Arctic (Tverberg et al., 2014) and northern elephant seals have provided important oceanographic data from the North Pacific Ocean (Boehlert et al., 2001; Keates et al., 2020).
Sea lions and fur seals (Family Otariidae) are particularly appropriate to sample temperate coastal waters. They have delivered oceanographic profiles along both coasts of South America, the Galapagos Islands, Southwest Africa, the West Coast of North America across the Aleutian Islands to the northern reaches of Hokkaido Japan (Weise et al., 2010). They are also well suited for sampling the coastal zone of the South Island of New Zealand, the sub-Antarctic Islands and the southern coasts of Australia (Lowther et al., 2013; Foo et al., 2019; Wege et al., 2019) and the Sea of Okhotsk (Nakanowatari et al., 2017).
Cetaceans have proved less effective as ocean sensor platforms compared to pinnipeds because they are fully aquatic and hairless, making instrument deployments logistically more challenging. Nevertheless, valuable sampling in the Arctic has been accomplished using narwhals (Monodon monoceros), beluga (Delphinapterus leucas), and bowhead (Balaena mysticetus) whales (Laidre et al., 2002; Lydersen et al., 2002; Teilmann et al., 2020). However, cetaceans include highly migratory species that cover tropical to polar regions during their migrations having therefore a huge potential to assist increasing collection of Essential Ocean Variables. For animals such as these, efforts to devise improved and more efficient tagging methods could lead to significant benefits in the data that they can collect.
Seabirds can be highly effective platforms for ocean sensing. King (Aptenodytes patagonicus) and emperor (A. forsteri) penguins have provided temperature profiles from the Southern Ocean (Charrassin et al., 2002; Scheffer et al., 2012, 2016; Grist et al., 2014; Labrousse et al., 2019). Penguins and fur seals (Otariidae) from temperate regions of the Southern Hemisphere may also hold opportunities to provide temperature and other oceanographic data, albeit primarily in the upper 100m of the water column. Flying seabirds have provided surface measurements such as ocean currents and winds (Weimerskirch et al., 1995; Yoda et al., 2014; Yonehara et al., 2016; Goto et al., 2017) over great expanses of the tropical and temperate oceans. However, the kinds of instruments seabirds can carry are limited given their small size and concomitant weight restrictions on the tags associated with flight (Sokolov, 2011; Holton et al., 2021). Seabirds are currently an underutilized source of oceanographic data as seabird colonies can be found in most oceans and regions of the world and because of the increasing sophistication and miniaturization of instrumentation that can be attached to birds that record behavior and an increasing suite of environmental variables (Holton et al., 2021).
Sea turtles, sharks and large-bodied fish can be used to obtain oceanographic data in the temperate to tropical regions (McMahon et al., 2005; Thums et al., 2018; Andrzejaczek et al., 2019; Bousquet et al., 2020), integrating information from important EOVs or Essential Climate Variables (ECVs) from areas of interest to biodiversity (Miloslavich et al., 2018). Temperature-Depth profiles collected by loggerhead turtles (Caretta caretta) were integrated in ocean nowcast/forecasts that greatly improved the representation of mesoscale eddies and front variations in the Kuroshio-Oyashio Confluence region around Japan (Miyazawa et al., 2018). Moreover, Temperature-Depth profiles collected by olive Ridley turtles (Lepidochelys olivacea) were assimilated into an operational seasonal prediction system of regional sea surface temperatures in the Arafura Sea (Doi et al., 2019). There is a growing community of animal-borne ocean observers in the tropical oceans, e.g., the Sea Turtle for Ocean Research and Monitoring (STORM) project that studies the properties and variability of the tropical Indian Ocean to improve research on ocean dynamics and climate change (Bousquet et al., 2020). Many species of coastal and pelagic sharks, contrary to popular perceptions, frequently come to the surface and this behavior is ideal for determining at-sea locations and data transmission via satellite constellations such as Argos (Heithaus et al., 2007; Vandeperre et al., 2014; Meyer et al., 2018). Sharks have effectively collected data on dissolved oxygen (Coffey and Holland, 2015) and sub-surface warming (Koopman et al., 2014) and even temperature profiles in Arctic waters (Fisk et al., 2012).
Animal biotelemetry data are most commonly relayed through Service Argos and its constellation of polar-orbiting satellites (Argos, 2016). Because of limited available satellite bandwidth, continuously recorded measurements on-board the tag must be compressed and summarized prior to being transmitted either pseudo-randomly or following a set of user-defined rules to prioritize near real time CTD data transfer (Fedak et al., 2002; Boehme et al., 2009). Communication performance for marine animals typically ranges with between 70 to 97% of dives transmitted successfully for a year-long deployment (McMahon et al., 2007) and this is higher near the poles than at the equator due to improved satellite coverage, approximately 18 (range 14–28) vs. 8 (range 6–12) passes per day (Hays et al., 2007; Argos, 2016). On average approximately 450 bytes and 1700 bytes are transmitted daily at the equator and poles respectively. Animal location estimates are obtained as a by-product of this data relaying process by using the Doppler shift in frequencies between the tag and receiving satellites (Argos, 2016). Depending on the number of messages received along a satellite pass, derived positions are classified into one of seven location classes (LC3, LC2, LC1, LC0, LCA, LCB, and LCZ) and have a nominal error radius ranging from <250 m (LC 3) to 10 km (LC B) (Vincent et al., 2002; Costa et al., 2010; Hoenner et al., 2012; Argos, 2016; Irvine et al., 2020). Because marine mammals and reptiles commonly only surface to breathe briefly (e.g., ∼10% of the at-sea time for elephant seals), transmission opportunities are short and infrequent, typically resulting in large proportions of locations with high spatial errors (LC 0, A and B) (Lopez et al., 2015; Thomson et al., 2017). Positioning through the Argos system requires at least two received messages during a single satellite pass and was historically estimated using a non-linear least squares technique which was replaced in 2011 by a square root unscented Kalman filter algorithm (Lowther et al., 2015). State-space models further improve the accuracy of those location estimates and are now routinely employed for automated near real time quality control of animal movement data (Silva et al., 2014; Jonsen et al., 2020).
Further to these post hoc improvements, upgrades to the Argos constellation of satellites that now comprise eight operational satellites includes the launch of an additional constellation of 25 nanosatellites under Kinéis in 2023. These satellites will carry the new Argos 4 technology which improves data transmission rates as well as location accuracy due to its 8-fold increase in bandwidth and enhanced operational downlink transmission efficiency. This increase in satellite numbers will increase satellite revisit times from 100 to 15 min, improve the Doppler location estimation (under ideal conditions) from 250 to 150 m and importantly for animal-borne instruments, decrease the power requirements from 250 to 100 mW for transmitting data.
Alternative position acquisition systems exist and can been added to instruments, but adding these systems comes at a cost in terms of energy and bandwidth consumption and as a rule both systems are not typically run on the same instrument. The most popular compliment (to Argos) positioning system is Fastloc GPS (Wildtrack Telemetry Systems). Fastloc GPS offers lower power consumption and faster acquisition times (100 ms) than the traditional Global Positioning System (GPS), and computes animal location with high accuracy (95th percentile error for >6 satellites = 70 m) (Hazel, 2009; Witt et al., 2010; Dujon et al., 2014; Thomson et al., 2017). An animals’ dive and hydrographic profile positions are then estimated by linear interpolation between two neighboring Argos-derived locations (Roquet et al., 2014).
Temperature sensors have been used for decades to collect oceanographic data from many marine species including sharks, fish, turtles, seabirds and marine mammals. These temperature sensors are sufficiently accurate (0.1°C ± 0.05°C) at their typical sampling frequency of between 1 and 10 s to provide information over the typical lifespan (longevity = 12 months) of the instruments (McMahon et al., 2005) to observe important oceanographic features like thermoclines (Simmons et al., 2009), for improving regional seasonal climate predictions (Doi et al., 2019) and for improving nowcast/forecast systems to more accurately represent mesoscale eddies and front variations (Miyazawa et al., 2018). Temperature data is collected from multiple instrument types including pop-off, towed and surface-mount tags and are typically reported as Profiles of Depth and Temperature (PDT). PDTs are derived in different ways, depending on the instrument type and manufacturer programming. For example, ‘Fixed Depth’ PDTs report temperature at pre-determined depths corresponding to the World Ocean Database or World Ocean Atlas depth tables. Summary period PDTs, often created by pop-off tags deployed on non-air breathers, by contrast generate profiles based on user-defined bin ranges and the actual depths traversed by the tagged animal during the defined summary period. These PDTs are often created by pop-off tags deployed on non-air breathers. A ‘Dive’ PDT comes from an air-breathing animal and reports the depth-temperature pairs along the ascent of the dive (i.e., on the upcast). To maximize the amount of data that is transmitted each time the animal surfaces raw profiles are summarized using a broken stick algorithm that selects and sends only the important inflection points within each profile (Fedak et al., 2002). These broken stick PDTs are used in multiple tag types; instead of using pre-determined depth bins, or evenly binning depths between the minimum and maximum depths visited, depth-temperature pairs are chosen for a profile using a broken stick algorithm. The resolution and accuracy of temperature profiles will vary based on the manufacturer and model of the tag the details of which are recorded within the instrument metadata records.
Over the last 15 years, animals have been instrumented with CTD-SRDL (Conductivity-Temperature-Depth Satellite Relay Data Logger) tags to measure the vertical water profiles of temperature and salinity in the top 2,000 m. Two types of satellite-linked CTD tags are currently deployed on animals: CTD-SRDLs (Sea Mammal Research Unit (SMRU) Instrumentation, University of St Andrews, United Kingdom) (Fedak et al., 2002; Lydersen et al., 2002; Boehme et al., 2009; Roquet et al., 2014) and more recently SCOUT-CTDs (Wildlife Computers, Redmond, WA, United States) (Heide-Jorgensen et al., 2020). Typically, the CTD-SRDL consists of a pressure transducer, a platinum resistance thermometer and an inductive cell for measuring conductivity (Valeport Ltd., Devon, United Kingdom). The temperature and conductivity sensors have a precision (repeatability) of 0.005°C and 0.005 mS/cm, respectively. Before being taken into the field, conductivity sensors are calibrated in the laboratory by Valeport (Boehme et al., 2009; Roquet et al., 2011, 2014). The CTD-SRDLs are supplied with a three-point calibration that covers the sensor’s entire temperature range. Researchers can perform their own more tailored pre-deployment calibrations. When possible (∼40%) the instruments are also calibrated at sea against a ship-based CTD (Roquet et al., 2011, 2014). Carse et al. (2015) found that assimilation of NRT salinity profiles from CTD-SRDLs caused a high (salty) bias of up to 0.4 PSU in ocean forecast model background fields, which led to a decision not to assimilate NRT salinity profiles from mammals into the United Kingdom Meteorology Office’s global ocean model. This was thought to be due to the near-field effect on the inductive conductivity sensor causing it to measure high, and the inability to correct the salinity values in NRT. Collecting the highest quality data and making this available to the broader oceanographic operational and research communities remains a key focus for AniBOS. Ongoing research to reduce observational bias by applying appropriate corrections to the field observations also remains a priority. For the SMRU CTD-SRDLs, adjustments accounting for the thermal mass of the instrument have been investigated, which has led to improvements in the salinity measurements. To provide accurate temperature and salinity measurements to the community there are three adjustments that are made: (1) Salinity and temperature (Roquet et al., 2011), (2) Thermal mass error (Siegelman et al., 2019), and (3) Density-inversion removal (Barker and McDougall, 2017).
First the temperature-conductivity observations from the CTD-SRDLs are corrected for a pressure-dependent linear bias by comparing CTD-SRDL data to ship-based CTD measurements. To correct for salinity offsets a combination of methods is used (Roquet et al., 2011, 2014). The most accurate method uses the stable salinity maximum for seals foraging and collecting data south of the Southern Ocean. For other seals, a cross comparison method of the CTD-SRDL measurements is used. As a last resort, comparisons with historical data can be used to reduce the salinity offset. Note that the amplitude of the salinity offset has tended to decrease in the last decade as the quality of sensors and of the lab calibration procedure has increased. For seals that collected observations in frozen regions, a comparison of surface temperature with the freezing temperature can be used to adjust temperature measurements (Roquet et al., 2011, 2014).
A thermal mass error (TME) occurs whenever the temperature of waters surrounding the temperature and conductivity sensors is not yet equilibrated with the surrounding environment. To account for this well-known and commonly observed effect. Mensah et al. (2018) developed a method similar to the correction schemes used on ship borne CTD or glider measurements. Siegelman et al. (2019) developed further the correction scheme to simultaneously correct conductivity and temperature data.
Density inversions are common in oceanographic observations and occur due to instrumental noise and salinity spiking. Barker and McDougall (2017) have proposed a method to optimally remove density inversions which has been adapted for CTD-SRDL data. A detailed description of this procedure can be found in Siegelman et al. (2019). Sensor response time too may affect the measurements, but we are confident that neither sensor lags, approximately 0.7 s nor recording frequency 0.5 Hz affect the observations given they are within the general ascent speed of the seals which is between and 2 m/s.
The accuracy of the temperature and salinity measurements, after correction, is ±0.02°C and salinity to ±0.03 g/kg respectively, with large disparities depending, in particular, on the magnitude of the vertical gradient of temperature in the water column. Improvements in sensor technology, satellite bandwidth and more systematic testing of the sensors prior to their deployment could all contribute to achieving higher measurement accuracy.
Similar to the SMRU CTD-SRDLs, the new SCOUT CTD tag (Wildlife Computers, Redmond WA, United States) contains a pressure transducer, a fast-response high-precision temperature sensor and a platinized electrode-based cell custom-developed for Wildlife Computers by Soundnine Inc. (Kirkland WA, United States) for measuring conductivity. Typically, the resolution and accuracy of the temperature sensor is 0.001 and ±0.01°C and the conductivity sensor is ±0.05 mS/cm, which is approximately 0.05 PSU depending upon temperature over the range of 0–40 PSU. Soundnine Inc., performs laboratory calibration of the temperature and conductivity sensors on completely assembled SCOUT-CTDs prior to deployment. Calibration is also performed post-deployment if the tag is recovered. Long-term drift and the impact of biofouling on the conductivity readings have yet to be characterized. It is noteworthy that the electrode-based conductivity cells in the SCOUT-CTD, in contrast to inductive cells used in the SMRU CTD-SRDLs, are not subject to near-field effects that can cause instabilities in the salinity readings (Hooker and Boyd, 2003), which are corrected during delayed mode quality control processing of the SMRU CTD-SRDL observations (Mensah et al., 2018).
The SCOUT-CTD formats the depth, temperature and salinity readings into WOD/WOA (World Ocean Database/World Ocean Atlas) standard fixed depth profiles during the ascent phase of qualifying dives, then takes a Fastloc® GPS snapshot when the animal reaches the surface. Fastloc® GPS locations have a typical accuracy of 20–75 m. The CTD profiles and Fastloc® GPS snapshots are packaged into messages and transmitted through the Argos satellite system.
In addition to the aforementioned temperature and salinity measurements other parameters are currently measured or are likely to be available soon, including fluorescence to estimate chlorophyll-a concentration within the euphotic layer (Guinet et al., 2013; Biermann et al., 2015; Sauzede et al., 2015; Baldry et al., 2020; Keates et al., 2020). Dissolved oxygen sensors have been incorporated successfully into CTD tags deployed on southern elephant seals (Bailleul et al., 2015). These small low energy consumption oxygen sensors have been developed (Bailleul et al., 2015) could be easily incorporated into oceanographic tags deployed on marine animals (Bailleul et al., 2015). Dissolved oxygen measurements from deep (e.g., northern elephant seals can dive to depths of up to 2000 m Naito et al., 2017; Godard et al., 2020) diving animals might, for instance, be of particular importance in monitoring the extent of Oxygen Minimum Zones (Stramma et al., 2008). Acoustic, acceleration and magnetometer measurements have been used to estimate wind speed, sea state and wind direction (Cazau et al., 2017). The capability for onboard processing of acoustic signals on sensor tags is currently being developed and implemented to estimate wind speed in the open ocean. Indeed, ocean wind and surface current have been estimated successfully by deploying GPS tags on soaring seabirds (Yoda et al., 2014; Yonehara et al., 2016; Goto et al., 2017). Research is being conducted to implement a pH sensor in tags deployed on free-ranging animals, however, calibration issues associated with sensor drift remain to be resolved.
Many ecological parameters can be assessed from tags deployed on animals. They provide a direct way to investigate the link between physical oceanographic and biological processes at meso and sub-mesoscales and how physics structures biological fields (animal habitats) horizontally and vertically. Animal-borne observations provide information on EOVs/ECVs in areas and corridors of great importance to biodiversity (Harcourt et al., 2019; Hays et al., 2019; Hindell et al., 2020; Lennox et al., 2020). AniBOS will also contribute to marine management and policy decision making. For example, changes in ocean properties (temperature, acidity and sea-ice extent) will likely influence the properties of marine ecosystems which will require adaptive management of tools such as Marine Protected Areas as species and communities become re-distributed (Hindell et al., 2020). Data collected by AniBOS will therefore be important for integrating MPAs into broader climate action frameworks. Prey field abundance and vertical distribution can also be assessed along the track of marine animals equipped with acceleration sensors (Naito et al., 2017; Cox et al., 2018; Adachi et al., 2021), which detect prey capture attempts (Figure 4). Direct visual observations of what prey the animal is encountering can be obtained from animal-borne video systems (Davis et al., 1999; Hooker et al., 2002; Watanabe et al., 2006). This information is particularly valuable when related to simultaneous measurements of other habitat parameters such as temperature, salinity and phytoplankton concentrations (Naito et al., 2013; Guinet et al., 2014). Intermediate trophic levels are now being assessed by a broad range of techniques such as the monitoring of bioluminescence (Vacquie-Garcia et al., 2017b) and/or the deployment of very high frequency active acoustic devices (microsonar) to monitor the abundance, size and reflectance of biological particles within the water column (Goulet et al., 2019). While many of these technologies are novel, the promise of expanding the scope of observations is stimulating much research and technological development. It is anticipated that as these new technologies are adopted, the information they provide will become increasingly mainstream.
Figure 4. The vertical distribution (depth on the y-axis) of prey capture attempts detected by accelerometers (black dots) along the track of a female elephant seal from the Kerguelen Islands, overlaid with animal-borne high frequency (0.5 Hz) temperature records. This illustrates how physical and biological observations can be integrated to provide unique insights into oceanographic, behavioral and predator-prey processes – an ability unique to AniBOS. Source: C. Guinet, CEBC/CNRS, but also see Tournier et al. (2021).
Animal Borne Ocean Sensors activities, structure and vision are determined by three overarching committees: the Steering Committee, Data Committee and the Ethical Advisory Board each with specific tasks detailed below. Overall AniBOS activities are defined under 10 key terms of reference as follows:
• Develop an Implementation Plan for a global network of ocean profiles (e.g., temperature, salinity, chlorophyll-a) collected by animal-borne sensors, to contribute to GOOS.
• Promote and further develop the existing international consortium, to undertake the implementation and maintenance of the global network.
• Coordinate and exchange information with the Observations Coordination Group (OCG) of GOOS on scientific and technical issues to ensure sustained, high quality observing meeting the needs of the broader scientific community.
• Evaluate observing network coverage to guide the long-term development and evolution of the network.
• Provide advice and guidance on technical (sampling tools and sensors) innovations relevant to the network, including the development of best practices for sampling design and data quality control.
• Promote and maintain the highest levels of best practice in animal welfare.
• Facilitate and coordinate delivery of near real time data to the Global Telecommunication System (GTS) and quality-controlled data to a network of global data centers.
• Adhere to and promote FAIR (findable, accessible, interoperable and reusable) data principles.
• Liaise with other in situ ocean observing networks including; Argo, GO-SHIP, the ships-of-opportunity program, the tropical atmosphere-ocean array and ocean remote sensing programs to provide an integrated and comprehensive GOOS.
• Provide, as appropriate, reports on progress to the OCG and input to OCG forum meetings.
Ensuring the longevity of AniBOS as a contributor to GOOS and a vehicle for disseminating essential scientific knowledge is a core task of the AniBOS Steering Committee. Therefore, AniBOS under the guidance and leadership of the Steering Committee is dedicated to recruiting and retaining young researchers into research leadership roles providing Early Career Researchers (ECRs) with skills and experiences that will help foster this career progression. The current professional cohort (scientists and technical staff) has an important role to play in narrowing knowledge gaps, through the transfer of skills and expertise and to mentor and guide the next generation of researchers and scientists.
The specific tasks of the Steering Committee include:
• Provide leadership to implement the observing network.
• Coordinate network activities, including deployment regimes.
• Submit the scientific and operational plans of the network to GOOS OCG.
• Assist the co-chairpersons in the preparation of reports, reviewing action items of previous meetings and reporting these to the broader community.
• Promote and build stakeholder engagement.
• Promote broad international involvement, enhance coordination and collaboration to achieve integrated and sustained observations to end-users globally.
The Data Committee is responsible for the coordination and governance of the network’s data and metadata, which includes resolving issues that may hinder interoperability and reuse. This will be accomplished, in part, through the development and use of standardized and quality controlled netCDF templates that incorporate essential metadata attributes and data variables regardless of species, tag/sensor manufacturer, type, deployment location or facilitating nation (Sequeira et al., 2021). The Data Committee will also oversee data and metadata dissemination, ensuring adherence to FAIR (Wilkinson et al., 2016) data principles:
• Findable – Data and supplementary materials have sufficiently rich metadata and a unique and persistent identifier that is easily discoverable on the Web.
• Accessible – Data and metadata are archived in a trusted repository and publicly distributed in usable form.
• Interoperable – Data and metadata conform to accepted Earth Science geospatial data interoperability standards and leverage controlled vocabularies to enable both human and automated machine-to-machine usability.
• Reusable – Data and collections have an explicit usage license and provide accurate information on provenance, data acquisition and processing to enable consistent, reproducible science use.
• Open – Data is free and public, can be used, reused and redistributed by anyone, subject to appropriate attribution and sharing in the same manner they appear. The Creative Commons Attribution-ShareAlike 4.0 International license (CC-BY-SA 4.0) would apply.
The Data Committee will also work closely with the GOOS OCG to ensure that the AniBOS data policy and data management frameworks adhere to the overall OCG data strategy, which will ensure that AniBOS data and metadata are compatible with data efforts through World Meteorological Organization (WMO), the Intergovernmental Oceanographic Commission (IOC) and the International Oceanographic Data and Information Exchange (IODE) and follow existing data standards frameworks (Sequeira et al., 2021).
Animals collecting oceanographic data is truly a “win-win” situation as it provides data essential to animal conservation and management while also providing oceanographic data. The stability and future growth of AniBOS rests on our community promoting and adhering to best animal handling practices that minimize negative effects on animal welfare. The AniBOS Ethical Advisory Board provides this ethical oversight through its primary responsibilities, to:
• Ensure that the use of animals is justified, provide for the welfare of all animals involved in AniBOS science and incorporate Replacement, Reduction and Refinement principles (Dawkins, 2006).
• Ensure that network activities comply with and are conducted following the world’s best practice compiled in AniBOS referenced protocols (available on the website) and in compliance with local legal animal care regulations and requirements.
• When requested, review proposals to ensure that they meet the stringent compliance standards of the network on the use of animals for scientific and observational purposes.
• Provide ongoing feedback to the network membership on advances in best practice, training of staff and facilitate their adoption where appropriate.
• Collect feedback on the capture/handling of animals and the deployment of instruments to consistently improve protocols with the long-term perspective of improving animal welfare outcomes from instrument attachments.
To fill existing data gaps it is vital to determine priority regions and the number of sensors (or animals) that need to be deployed. An important consideration here is animal ethics and welfare of tagging marine animals, which have been discussed in detail elsewhere (McIntyre, 2014; Sequeira et al., 2019b) and is a high priority for AniBOS (see, The ethics of animal oceanographers below). The optimal sample size is a balance that: (1) allows for appropriate spatio-temporal coverage, (2) provides sufficient samples and (3) prioritizes animal welfare (i.e., reducing the number of animals used to the minimum needed and using tags appropriate to the species). The AniBOS Ethical Advisory Board will provide advice and guidance on protocols regarding these issues and, in so doing, assist members with ethics obligations within their individual jurisdictions.
Standardized data and metadata formats are required for interoperability with other ocean observation sources such as floats and gliders. Although the specific standardized specifications are currently under evaluation and may evolve, an initial standard to be adopted will be consistent with MEOP, using two data formats: time-referenced data and profiling (depth-referenced) data. The Argo data management group develops and maintains such data files3, in netCDF format following Climate and Forecast (CF) standards. The Sea-Mammals netCDF data format, currently used to store and distribute data in the MEOP databases4 has been adapted from the Argo data profile and trajectory format specifications.
The NASA/JPL Oceanographic In situ data Interoperability Project (OIIP) has progressed interoperability for marine animal tagging data through their development of the netCDF electronic tag data template specification (nc-eTAG; Tsontos et al., 2020). These templates are applicable to a broad spectrum of electronic tag data types and sensor measurements. The Data Committee, in collaboration with OIIP, the proposed GOOS - Animal Research and Tracking Initiative (GOOS-ARTI), the ongoing global MegaMove (marine megafauna movement) initiative, the International BioLogging Society and tag manufacturers, will explore the potential for continued refinement and adoption of the nc-eTAG data and metadata templates (after Sequeira et al., 2021), the International BioLogging Society and tag manufacturers, will explore the potential for refinement and adoption of the nc-eTAG data and metadata templates. All data products produced by AniBOS will be available in a format compatible with the other GOOS networks to enhance global ocean observing capacity. AniBOS will explore the utility of existing standards, such as the Sea-Mammals netCDF and nc-eTAG netCDF specifications, those currently in use across autonomous underwater vehicle programs and Argos floats.
To the extent possible, AniBOS will ensure relevant metadata flow directly from the tag manufacturers to OceanOPS and the DACs. However, it is anticipated that not all contributing tag manufacturers will be able to comply with this approach and therefore alternative, yet similarly robust methods will need to be explored for harvesting metadata in these cases.
The Data Committee and OceanOPS will collaborate to ensure that metadata identified across all components of AniBOS will be mapped, standardized and harmonized in order to fulfill OceanOPS and GOOS requirements on metadata5. In addition, AniBOS will work closely with the GOOS OCG to implement web-based machine-to-machine services for data and metadata that are to be publicly accessible. These services will increase interoperability with other ocean data networks coordinated under the GOOS OCG and with other science communities (e.g., OBIS-SEAMAP).
Essential to AniBOS is the need to provide quality-controlled, interoperable animal-borne ocean sensor data and metadata. These are publicly accessible and disseminated regularly in a coordinated manner to the global oceanographic community for near real time assimilation by operational models, for use by the broader research community and for education and public outreach. The network’s data and metadata management is determined by the Data Committee which includes representatives of the major tag manufacturers, data management specialists and end-users, in coordination with GOOS OCG and OceanOPS, and under the guidance of the AniBOS Steering Committee.
The data flow structure is guided by the Argo approach (Wong et al., 2021), but, adapted and simplified given the unique aspects of the network’s data and metadata (Figure 5). Oversight for data flow rests with the Data Committee. Data management will follow accepted community (oceanography and animal bio-logging) standards for the data flow from tags to a repository (Sequeira et al., 2021).
The network’s data and metadata originate with the tag manufacturers, who provide well-established infrastructures for decoding, archiving and serving data to their customers (Sequeira et al., 2021). AniBOS will harvest the decoded data directly from the manufacturers’ web portals. Researchers who purchase and deploy tags on animals have the option of sharing location and sensor data with the network. Researchers will provide metadata about tag deployments (e.g., location, date, species, tag programming). Additional tag metadata (e.g., sensor sensitivities and calibrations, factory programming defaults, firmware versions) are as a matter of course provided by the tag manufacturers (black lines Figure 5). Assignment of WMO identification numbers to tag deployments, essential for registration of tags as observing platforms in the WMO system, is typically completed by the regional Data Assembly Centers (DACs) and supported/overseen by OceanOPS.
Near real time data are relayed to the DACs where automated processes are used to: (1) standardize the data and metadata into agreed-upon formats (see below); (2) conduct preliminary quality control of the data (consistent with Argo floats and gliders); (3) generate Level 1 products - initially ocean CTD profiles; (4) convert Level 1 products to BUFR (Binary Universal Form for the Representation of meteorological data) messages and transmit those via the GTS to operational centers for NRT assimilation (within 24 h; red line, Figure 5). Metadata will be monitored by the OceanOPS Information Center where, for example, missing WMO identification numbers will be assigned to tags prior to their deployment. Level 1 products and metadata will be sent, within 24 h, to a Global Data Assembly Center (GDAC), where additional scrutiny can be applied prior to public access by end users, national research facilities and aggregated databases. The GDAC is operated using a federated strategy and united by a high level of data format standardization that will make complete metadata (e.g., compliant with the ISO 19115 standard for geospatial metadata) publicly discoverable, downloadable and queryable via interoperable web services and technologies using standard protocols.
Delayed-mode data products (Level 2) are produced (yearly release is targeted), benefiting from the input of regional data experts, researchers, manufacturers and technical experts collaborating on the AniBOS network. The AniBOS Data Committee will supervise this work in association with the DAC’s. These products are subjected to stringent processing that include the quality control of location and ocean profile data. The delayed-mode quality control (DMQC) processes will be standardized globally, using the Level 1 product built by the GDAC as the reference dataset onto which editing, re-calibration and data adjustment will be applied. The DMQC builds upon the set of similar procedures that were developed by MEOP, or by different regional teams (Roquet et al., 2011, 2014; Siegelman et al., 2019; Jonsen et al., 2020). New methods to accommodate new tags and/or sensors become available will be developed as necessary. The GDAC provides all Level 2 data publicly available and also archives the data at appropriate national/regional facilities.
Currently, the United States Integrated Ocean Observing System (IOOS) Animal Telemetry Network (ATN) and Australia’s Integrated Marine Observing System (IMOS) Australian Ocean Data Network serve as regional DACs. The Ocean Tracking Network (OTN, Canada) is prepared to act as an additional DAC at a global scale, serving Canada as well as regions without a national or regional DAC. Additional organizations with the technical facilities and expertise to serve as regional DACs will also be considered. The ATN could provide the required infrastructure to run the GDAC. This potential structure will need to be explored further and will depend on available resources. AniBOS will archive data within facilities such as the National Centers for Environmental Information (NCEI) at the National Oceanic and Atmospheric Administration (NOAA) in the United States, which would ensure the long-term, secure preservation of data, metadata and provenance information. Additional storage facilities are available through IMOS and OTN. DOIs for data citation will be issued upon data archiving, typically by the regional DACs.
All sensor accuracy/precision and calibration attributes will be reported in the metadata products to ensure appropriate handling during QC and creation of derived products. The minimum precision associated with the data provided by a tag is critical and will vary depending on manufacturer and tag type. Similarly, measurement accuracy will need to be specified for all datasets (e.g., location, temperature, salinity, and chlorophyll-a). Rather than imposing strict minimum thresholds on sensor accuracy and precision, this approach will allow greater assimilation of observation data sets across diverse tag types. To ensure consistent data quality across platforms AniBOS will adopt the Argo flagging system (Argo data management, doi6).
Animals collecting oceanographic data have enhanced greatly our understanding of animal behavior and how the environmental affects this behavior. Central to the ongoing success of AniBOS is ensuring that contributors use the best animal handling practice that prioritizes animal welfare (Table 3). The Ethical Advisory Board is a central component of AniBOS (see above). The board’s primary focus is to provide ethical oversight of the network activities involving the handling of animals and the attachment of sensors. The AniBOS community has been proactive and already much has been done to ensure animal welfare is at the core of our activities. This has been done through publishing standard operating procedures that describe in detail the capture, handling, instrument attachment and care of animals (Table 3). However, given the likely expansion to a broader range of species and the development of new sensor packages, ongoing research into quantifying the effects of capture, handling and attachment methods will be necessary. An important part of this will be quantifying the effects that carrying instruments has on animal behavior and vital rates.
Table 3. A summary of the AniBOS community’s documented contributions to best practice for ensuring the highest data and animal welfare standards.
Globally there is a need to increase ocean observing to all parts of the ocean and how this ocean monitoring is translated from observations to applications. AniBOS is committed to helping retain a healthy and resilient ocean, with marine ecosystems that are better understood, protected, restored and managed to promote conservation and food security. AniBOS provides open and equitable access to technology, data and information to narrow knowledge and observational gaps while helping society understand and respond to changing conditions.
Ongoing long-term funding is an essential part of maintaining AniBOS and indeed GOOS. Currently AniBOS relies on a mixture of investment derived from several national research projects and in Australia longer term investment from the Integrated Marine Observing System and this represents a vulnerability to the network. However, to build greater funding security AniBOS has secured endorsement of the network through the UN Decade of the Ocean initiative. International endorsements like this capture the attention of national programs that may facilitate government investment. Indeed, two of the countries currently participating in AniBOS have already made formal budget requests for additional resources to enhance and sustain their national program contributions to AniBOS illustrating national recognition of the network’s value and importance.
While data sharing within the marine ecology community has previously occurred intermittently a number of studies illustrate and demonstrate the increased productivity by data sharers within the animal tracking community (Nguyen et al., 2017; Lennox et al., 2020); several independent initiatives where multiple datasets have already been shared for large synthetic studies, e.g., the Retrospective Analysis of Antarctic Tracking Data (Hindell et al., 2020; Ropert-Coudert et al., 2020) the Global Shark Movement project (Queiroz et al., 2019); MegaMove (Sequeira et al., 2018) as well as mandated data sharing by data providers (Hill et al., 2010). In addition, some data centers are now including the minting of DOI’s as part of their data archiving service (e.g., the U.S. ATN Data Assembly Center).
Animal Borne Ocean Sensors is a global inclusive network that has welcomed contributions of data collected from the global ocean from the onset and emphasized the need for community engagement and consideration of currently under-sampled ocean regions as a means to narrow technological, scientific and capacity development gaps (Fedak, 2004; Hussey et al., 2015; Yonehara et al., 2016; Treasure et al., 2017; Hamilton et al., 2018; Newman et al., 2019; Tanhua et al., 2019; Bousquet et al., 2020; Hindell et al., 2020). AniBOS aims to engage with relevant groups to identify priority regions and accommodate the role that human impact variables might have in the ocean observing system. This represents a long-term objective of GOOS and requires connecting to new communities of experts and institutions.
In addition to the vital contribution AniBOS makes to GOOS, animal-borne sensors are also ideal platforms for education and outreach activities and for more general communication of science to society, from children in classrooms to interested citizens. The “Follow the Glider” initiative7 is a nice example of how to reach the public with ocean science. Like ARGONIMAUX8 in which AniBOS is an active participant, “Follow the Glider” is a web-based educational tool aimed at students and teachers. AniBOS is currently working with the COVERAGE project and collaborators at the NASA Jet Propulsion Laboratory to develop an interactive web platform to visualize and interact with animal borne CTD data sets. These are valuable forums to raise awareness in the broader community about the marine environment, climate change, the interaction between animal performance and climate and the conservation of biodiversity using these data collected by marine animals equipped with CTD-SRDLs.
Animal Borne Ocean Sensors delivers information, knowledge and solutions for the next decade and beyond across three essential GOOS themes of global importance: ocean health, climate, and near real time services. The large number of possible animal platforms and the relatively low cost of the instrumentation, can play a leading integrative and capacity building role in enhancing global ocean observing.
Among the many Essential Ocean Variables that can be monitored using animal-borne ocean sensors, the measurement of vertical temperature and salinity profiles are the most mature and are the core focus of AniBOS. In future, the range of EOVs provided by AniBOS will expand. Chlorophyll (fluorescence) sensors are now widely deployed on electronic tags, while dissolved oxygen sensors are being developed. The measurement of other variables such as wind, surface waves or surface currents is currently in the pilot phase, but have great potential in the future. The information AniBOS provides freely to the global community directly benefits:
• Ocean science
• Operational ocean and weather forecasting
• Marine conservation and living resource management
• The blue economy
• Indigenous peoples
• Climate change research (including projections, impact assessments, socioeconomic modeling)
• Policy makers
• Educational institutions
The oceans are the next great economic frontier (Rayner et al., 2019; Jouffray et al., 2020). For this “Blue Economy” to be sustainable and to ensure that rapidly expanding marine developments do not compromise the socioeconomic benefits and essential ecosystem services humanity derives from the ocean, managers and policy makers need to be informed by comprehensive monitoring of the ocean (Rayner et al., 2019; Brodie Rudolph et al., 2020). The oceanographic observations AniBOS collects contributes along with the other GOOS (Moltmann et al., 2019) networks to the information needed by the global community to benefit from and sustainably manage the ocean.
CM and FR lead the writing of the manuscript to which all authors contributed equally.
Funding and support for the November 2019 Network Development Workshop was provided by the Integrated Marine Observing System (IMOS) and the Australia Research Council’s Special Research Initiative for Antarctic Gateway Partnership (SR140300001) through the University of Tasmania’s Institute of Marine and Antarctic Studies. IMOS is a national collaborative research infrastructure, supported by the Australian Government and operated by a consortium of institutions as an unincorporated joint venture, with the University of Tasmania as Lead Agent. This research contributes to the Australian Research Council Discovery Project DP180101667 and DP210103091. SBe was supported under the Australian Research Council DECRA DE180100828. IJ was supported by Macquarie University’s co-Funded Fellowship Program with external partners: Office of Naval Research (N00014-18-1-2405); the Integrated Marine Observing System – Animal Tracking Facility; the Ocean Tracking Network; Taronga Conservation Society; Birds Canada; and Innovasea/Vemco. AS was supported by a 2020 Pew Fellowship in Marine Conservation. DM was supported by the European Union’s Horizon 2020 Research and Innovation Program under the Marie Skłodowska-Curie grant agreement (No. 794938).
The authors declare that the research was conducted in the absence of any commercial or financial relationships that could be construed as a potential conflict of interest.
All claims expressed in this article are solely those of the authors and do not necessarily represent those of their affiliated organizations, or those of the publisher, the editors and the reviewers. Any product that may be evaluated in this article, or claim that may be made by its manufacturer, is not guaranteed or endorsed by the publisher.
We are extremely grateful to all of the AniBOS community for their support and commitment to the network.
Abraham, J. P., Baringer, M., Bindoff, N. L., Boyer, T., Cheng, L. J., Church, J. A., et al. (2013). A review of global ocean temperature observations: implications for ocean heat content estimates and climate change. Rev. Geophys. 51, 450–483. doi: 10.1002/rog.20022
Adachi, T., Takahashi, A., Costa, D. P., Robinson, P. W., Hückstädt, L. A., Peterson, S. H., et al. (2021). Forced into an ecological corner: round-the-clock deep foraging on small prey by elephant seals. Sci. Adv. 7:eabg3628. doi: 10.1126/sciadv.abg3628
Andrzejaczek, S., Gleiss, A. C., Pattiaratchi, C. B., and Meekan, M. G. (2019). Patterns and drivers of vertical movements of the large fishes of the epipelagic. Rev. Fish Biol. Fish. 29, 335–354. doi: 10.1007/s11160-019-09555-1
Apostle, R., Gazit, T., and Haward, M. (2016). Ocean tracking and marine species protection in Australia and Canada: science, technology, and knowledge brokering. Ocean Dev. Int. Law 47, 368–377. doi: 10.1080/00908320.2016.1229944
Argos (2016). Argos User’s Manual: Worldwide Tracking and Environmental Monitoring by Satellite. Toulouse: Argos.
Bailleul, F., Authier, M., Ducatez, S., Roquet, F., Charrassin, J. B., Cherel, Y., et al. (2010). Looking at the unseen: combining animal bio-logging and stable isotopes to reveal a shift in the ecological niche of a deep diving predator. Ecography 33, 709–719. doi: 10.1111/j.1600-0587.2009.06034.x
Bailleul, F., Vacquie-Garcia, J., and Guinet, C. (2015). Dissolved oxygen sensor in animal-borne instruments: an innovation for monitoring the health of oceans and investigating the functioning of marine ecosystems. PLoS One 10:e0132681. doi: 10.1371/journal.pone.0132681
Baldry, K., Strutton, P. G., Hill, N. A., and Boyd, P. W. (2020). Subsurface chlorophyll-a maxima in the Southern Ocean. Front. Mar. Sci. 7:671. doi: 10.3389/fmars.2020.00671
Barker, P. M., and McDougall, T. J. (2017). Stabilizing hydrographic profiles with minimal change to the water masses. J. Atmos. Ocean. Technol. 34, 1935–1945. doi: 10.1175/JTECH-D-16-0111.1
Bestley, S., Ropert-Coudert, Y., Bengtson Nash, S., Brooks, C. M., Cotté, C., Dewar, M., et al. (2020). Marine ecosystem assessment for the Southern Ocean: birds and marine mammals in a changing climate. Front. Ecol. Evol. 8:566936. doi: 10.3389/fevo.2020.566936
Biermann, L., Guinet, C., Bester, M., Brierley, A., and Boehme, L. (2015). An alternative method for correcting fluorescence quenching. Ocean Sci. 11, 83–91. doi: 10.5194/os-11-83-2015
Block, B. A., Teo, S. L., Walli, A., Boustany, A., Stokesbury, M. J., Farwell, C. J., et al. (2005). Electronic tagging and population structure of Atlantic bluefin tuna. Nature 434, 1121–1127. doi: 10.1038/nature03463
Boehlert, G. W., Costa, D. P., Crocker, D. E., Green, P., O’Brien, T., Levitus, S., et al. (2001). Autonomous pinniped environmental samplers: using instrumented animals as oceanographic data collectors. J. Atmos. Ocean. Technol. 18, 1882–1893. doi: 10.1175/1520-0426(2001)018<1882:APESUI>2.0.CO;2
Boehme, L., Lovell, P., Biuw, M., Roquet, F., Nicholson, J., Thorpe, S. E., et al. (2009). Animal-borne CTD-satellite relay data loggers for real-time oceanographic data collection. Ocean Sci. 5, 685–695. doi: 10.5194/os-5-685-2009
Bousquet, O., Barruol, G., Cordier, E., Barthe, C., Bielli, S., Calmer, R., et al. (2021). Impact of tropical cyclones on inhabited areas of the SWIO Basin at present and future horizons. part 1: overview and observing component of the research project RENOVRISK-CYCLONE. Atmosphere 12:544. doi: 10.3390/atmos12050544
Bousquet, O., Dalleau, M., Bocquet, M., Gaspar, P., Bielli, S., Ciccione, S., et al. (2020). Sea Turtles for ocean research and monitoring: overview and initial results of the STORM project in the Southwest Indian Ocean. Front. Mar. Sci. 7:594080. doi: 10.3389/fmars.2020.594080
Brodie Rudolph, T., Ruckelshaus, M., Swilling, M., Allison, E. H., Osterblom, H., Gelcich, S., et al. (2020). A transition to sustainable ocean governance. Nat. Commun. 11:3600. doi: 10.1038/s41467-020-17410-2
Carey, M. J. (2009). The effects of investigator disturbance on procellariiform seabirds: a review. N. Z. J. Zool. 36, 367–377. doi: 10.1080/03014220909510161
Carse, F., Martin, M. J., Sellar, A., and Blockley, E. W. (2015). Impact of assimilating temperature and salinity measurements by animal-borne sensors on FOAM ocean model fields. Q. J. R. Meteorol. Soc. 141, 2934–2943. doi: 10.1002/qj.2613
Cazau, D., Pradalier, C., Bonnel, J., and Guinet, C. (2017). Do southern elephant seals behave like weather buoys? Oceanography 30, 140–149. doi: 10.5670/oceanog.2017.236
Chambault, P., de Thoisy, B., Heerah, K., Conchon, A., Barrioz, S., Dos Reis, V., et al. (2016). The influence of oceanographic features on the foraging behavior of the olive ridley sea turtle Lepidochelys olivacea along the Guiana coast. Progr. Oceanogr. 142, 58–71. doi: 10.1016/j.pocean.2016.01.006
Charrassin, J.-B., Hindell, M. A., Rintoul, S. R., Roquet, F., Sokolov, S., Biuw, M., et al. (2008). Southern Ocean frontal structure and sea ice formation rates revealed by elephant seals. Proc. Natl. Acad. Sci. U.S.A. 105, 11634–11639. doi: 10.1073/pnas.0800790105
Charrassin, J.-B., Park, Y.-H., Le Maho, Y., and Bost, C.-A. (2002). Penguins as oceanographers unravel hidden mechanisms of marine productivity. Ecol. Lett. 5, 317–319. doi: 10.1046/j.1461-0248.2002.00341.x
Citta, J. J., Okkonen, S. R., Suydam, R. S., Quakenbush, L., Bryan, A. L., and Olnes, J. (2020). Beluga dive behavior relative to fronts and stratified layers near Barrow Canyon, Alaska. Deep Sea Res. I Oceanogr. Res. Pap. 165:103392. doi: 10.1016/j.dsr.2020.103392
Claustre, H., Johnson, K. S., and Takeshita, Y. (2019). Observing the global ocean with biogeochemical-argo. Annu. Rev. Mar. Sci. 12, 23–48. doi: 10.1146/annurev-marine-010419-010956
Coffey, D. M., and Holland, K. N. (2015). First autonomous recording of in situ dissolved oxygen from free-ranging fish. Anim. Biotelem. 3:47. doi: 10.1186/s40317-015-0088-x
Costa, D. (2003). The secret life of marine mammals. Oceanography 6, 120–128. doi: 10.5670/oceanog.1993.07
Costa, D. P., Breed, G. A., and Robinson, P. W. (2012). “New insights into pelagic migrations: implications for ecology and conservation,” in Annual Review of Ecology, Evolution, and Systematics, ed. D. J. Futuyma 73–96. doi: 10.1146/annurev-ecolsys-102710-145045
Costa, D. P., Klinck, J. M., Hofmann, E. E., Dinniman, M. S., and Burns, J. M. (2008). Upper ocean variability in west Antarctic Peninsula continental shelf waters as measured using instrumented seals. Deep Sea Res. II Top. Stud. Oceanogr. 55, 323–337. doi: 10.1016/j.dsr2.2007.11.003
Costa, D. P., Robinson, P. W., Arnould, J. P., Harrison, A. L., Simmons, S. E., Hassrick, J. L., et al. (2010). Accuracy of ARGOS locations of Pinnipeds at-sea estimated using Fastloc GPS. PLoS One 5:e8677. doi: 10.1371/journal.pone.0008677
Cox, S. L., Orgeret, F., Gesta, M., Rodde, C., Heizer, I., Weimerskirch, H., et al. (2018). Processing of acceleration and dive data on-board satellite relay tags to investigate diving and foraging behaviour in free-ranging marine predators. Methods Ecol. Evol. 9, 64–77. doi: 10.1111/2041-210X.12845
Davis, R. W., Fuiman, L. A., Williams, T. M., Collier, S. O., Hagey, W. P., Kanatous, S. B., et al. (1999). Hunting behaviour of a marine mammal beneath the Antarctic fast ice. Science 283, 993–996. doi: 10.1126/science.283.5404.993
Dawkins, M. S. (2006). A user’s guide to animal welfare science. Trends Ecol. Evol. 21, 77–82. doi: 10.1016/j.tree.2005.10.017
Della Penna, A., De Monte, S., Kestenare, E., Guinet, C., and d’Ovidio, F. (2015). Quasi-planktonic behavior of foraging top marine predators. Sci. Rep. 5:18063. doi: 10.1038/srep18063
Doi, T., Storto, A., Fukuoka, T., Suganuma, H., and Sato, K. (2019). Impacts of temperature measurements from sea turtles on seasonal prediction around the Arafura Sea. Front. Mar. Sci. 6:719. doi: 10.3389/fmars.2019.00719
Dotto, T. S., Mata, M. M., Kerr, R., and Garcia, C. A. E. (2021). A novel hydrographic gridded data set for the northern Antarctic Peninsula. Earth Syst. Sci. Data 13, 671–696. doi: 10.5194/essd-13-671-2021
Dujon, A. M., Lindstrom, R. T., Hays, G. C., and Backwell, P. (2014). The accuracy of Fastloc-GPS locations and implications for animal tracking. Methods Ecol. Evol. 5, 1162–1169. doi: 10.1111/2041-210X.12286
Fedak, M. A. (2004). Marine animals as platforms for oceanographic sampling: a “win/win” situation for biology and operational oceanography. Mem. Natl. Inst. Polar Res. 58, 133–147.
Fedak, M. A. (2013). The impact of animal platforms on polar ocean observation. Deep Sea Res. II Top. Stud. Oceanogr. 88-89, 7–13. doi: 10.1016/j.dsr2.2012.07.007
Fedak, M., Lovell, P., McConnell, B., and Hunter, C. (2002). Overcoming the constraints of long range radio telemetry from animals: getting more useful data from smaller packages. Integr. Comp. Biol. 42, 3–10. doi: 10.1093/icb/42.1.3
Field, I. C., Bradshaw, C. J. A., McMahon, C. R., Harrington, J., and Burton, H. R. (2002). Effects of age, size and condition of elephant seals (Mirounga leonina) on their intravenous anaesthesia with tiletamine and zolazepam. Vet. Rec. 151, 235–240. doi: 10.1136/vr.151.8.235
Field, I. C., Harcourt, R. G., Boehme, L., de Bruyn, P. J. N., Charrassin, J. B., McMahon, C. R., et al. (2012). Refining instrument attachment on phocid seals. Mar. Mamm. Sci. 28, E325–E332. doi: 10.1111/j.1748-7692.2011.00519.x
Fisk, A. T., Lydersen, C., and Kovacs, K. M. (2012). Archival pop-off tag tracking of Greenland sharks Somniosus microcephalus in the high arctic waters of Svalbard, Norway. Mar. Ecol. Progr. Ser. 468, 255–265. doi: 10.3354/meps09962
Foo, D., McMahon, C., Hindell, M., Goldsworthy, S., and Bailleul, F. (2019). Influence of shelf oceanographic variability on alternate foraging strategies in long-nosed fur seals. Mar. Ecol. Progr. Ser. 615, 189–204. doi: 10.3354/meps12922
Frölicher, T. L., Sarmiento, J. L., Paynter, D. J., Dunne, J. P., Krasting, J. P., and Winton, M. (2015). Dominance of the Southern Ocean in anthropogenic carbon and heat uptake in CMIP5 models. J. Clim. 28, 862–886. doi: 10.1175/JCLI-D-14-00117.1
Godard, M., Manté, C., Guinet, C., Picard, B., and Nerini, D. (2020). Diving behavior of Mirounga leonina: a functional data analysis approach. Front. Mar. Sci. 7:595. doi: 10.3389/fmars.2020.00595
Godley, B. J., Richardson, S., Broderick, A. C., Coyne, M. S., Glen, F., and Hays, G. C. (2002). Long-term satellite telemetry of the movements and habitat utilisation by green turtles in the Mediterranean. Ecography 25, 352–362. doi: 10.1034/j.1600-0587.2002.250312.x
Goni, G. J., Sprintall, J., Bringas, F., Cheng, L., Cirano, M., Dong, S., et al. (2019). More than 50 Years of successful continuous temperature section measurements by the global expendable bathythermograph network, its integrability, societal benefits, and future. Front. Mar. Sci. 6:452. doi: 10.3389/fmars.2019.00452
Goto, Y., Yoda, K., and Sato, K. (2017). Asymmetry hidden in birds’ tracks reveals wind, heading, and orientation ability over the ocean. Sci. Adv. 3:e1700097. doi: 10.1126/sciadv.1700097
Goulet, P., Guinet, C., Swift, R., Madsen, P. T., and Johnson, M. (2019). A miniature biomimetic sonar and movement tag to study the biotic environment and predator-prey interactions in aquatic animals. Deep Sea Res. I Oceanogr. Res. Pap. 148, 1–11. doi: 10.1016/j.dsr.2019.04.007
Grist, J. P., Josey, S. A., Boehme, L., Meredith, M. P., Laidre, K. L., Heide-Jørgensen, M. P., et al. (2014). Seasonal variability of the warm Atlantic water layer in the vicinity of the Greenland shelf break. Geophys. Res. Lett. 41, 8530–8537. doi: 10.1002/2014GL062051
Guinet, C., VacquiÈ-Garcia, J., Picard, B., Bessigneul, G., Lebras, Y., Dragon, A. C., et al. (2014). Southern elephant seal foraging success in relation to temperature and light conditions: insight into prey distribution. Mar. Ecol. Progr. Ser. 499, 285–301. doi: 10.3354/meps10660
Guinet, C., Xing, X., Walker, E., Monestiez, P., Marchand, S., Picard, B., et al. (2013). Calibration procedures and first dataset of Southern Ocean chlorophyll a profiles collected by elephant seals equipped with a newly developed CTD-fluorescence tags. Earth Syst. Sci. Data 5, 15–29. doi: 10.5194/essd-5-15-2013
Guo, G., Shi, J., Gao, L., Tamura, T., and Williams, G. D. (2019). Reduced sea ice production due to upwelled oceanic heat flux in Prydz Bay, East Antarctica. Geophys. Res. Lett. 46, 4782–4789. doi: 10.1029/2018GL081463
Halpin, P., Read, A., Fujioka, E., Best, B., Donnelly, B., Hazen, L., et al. (2009). OBIS-SEAMAP: the world data center for marine mammal, sea bird, and sea turtle distributions. Oceanography 22, 104–115. doi: 10.5670/oceanog.2009.42
Hamilton, C. D., Kovacs, K. M., and Lydersen, C. (2018). Individual variability in diving, movement and activity patterns of adult bearded seals in Svalbard, Norway. Sci. Rep. 8:16988. doi: 10.1038/s41598-018-35306-6
Harcourt, R., Sequeira, A. M. M., Zhang, X., Roquet, F., Komatsu, K., Heupel, M., et al. (2019). Animal-borne telemetry: an integral component of the ocean observing toolkit. Front. Mar. Sci. 6:326. doi: 10.3389/fmars.2019.00326
Harrison, A. L., Costa, D. P., Winship, A. J., Benson, S. R., Bograd, S. J., Antolos, M., et al. (2018). The political biogeography of migratory marine predators. Nat. Ecol. Evol. 2, 1571–1578. doi: 10.1038/s41559-018-0646-8
Hawkins, P. (2004). Bio-logging and animal welfare: practical refinements. Mem. Natl. Inst. Polar Res. 58, 58–68.
Hays, G. C., Bailey, H., Bograd, S. J., Bowen, W. D., Campagna, C., Carmichael, R. H., et al. (2019). Translating marine animal tracking data into conservation policy and management. Trends Ecol. Evol. 34, 459–473. doi: 10.1016/j.tree.2019.01.009
Hays, G. C., Bradshaw, C. J. A., James, M. C., Lovell, P., and Sims, D. W. (2007). Why do Argos satellite tags deployed on marine animals stop transmitting? J. Exp. Mar. Biol. Ecol. 349, 52–60. doi: 10.1016/j.jembe.2007.04.016
Hazel, J. (2009). Evaluation of fast-acquisition GPS in stationary tests and fine-scale tracking of green turtles. J. Exp. Mar. Biol. Ecol. 374, 58–68. doi: 10.1016/j.jembe.2009.04.009
Hazen, E. L., Abrahms, B., Brodie, S., Carroll, G., Jacox, M. G., Savoca, M. S., et al. (2019). Marine top predators as climate and ecosystem sentinels. Front. Ecol. Environ. 17:565–574. doi: 10.1002/fee.2125
Hazen, E. L., Scales, K. L., Maxwell, S. M., Briscoe, D. K., Welch, H., Bograd, S. J., et al. (2018). A dynamic ocean management tool to reduce bycatch and support sustainable fisheries. Sci. Adv. 4:eaar3001. doi: 10.1126/sciadv.aar3001
Heerah, K., Hindell, M. A., Andrews-Goff, V., Field, I. C., McMahon, C. R., and Charrassin, J. B. (2016). Contrasting behaviour between two populations of an ice-obligate predator in East Antarctica. Ecol. Evol. 7, 606–618. doi: 10.1002/ece3.2652
Heide-Jorgensen, M. P., Blackwell, S. B., Williams, T. M., Sinding, M. H. S., Skovrind, M., Tervo, O. M., et al. (2020). Some like it cold: temperature-dependent habitat selection by narwhals. Ecol. Evol. 10, 8073–8090. doi: 10.1002/ece3.6464
Heithaus, M. R., Wirsing, A. J., Dill, L. M., and Heithaus, L. I. (2007). Long-term movements of tiger sharks satellite-tagged in Shark Bay, Western Australia. Mar. Biol. 151, 1455–1461. doi: 10.1007/s00227-006-0583-y
Hill, K., Moltmann, T., Proctor, R., and Allen, S. (2010). The Australian integrated marine observing system: delivering data streams to address national and international research priorities. Mar. Technol. Soc. J. 44, 65–72. doi: 10.4031/MTSJ.44.6.13
Hindell, M. A., McMahon, C. R., Bester, M. N., Boehme, L., Costa, D., Fedak, M. A., et al. (2016). Circumpolar habitat use in the southern elephant seal: implications for foraging success and population trajectories. Ecosphere 7:e01213. doi: 10.1002/ecs2.1213
Hindell, M. A., McMahon, C. R., Harcourt, R., Arce, F., Guinet, C., and Jonsen, I. (2021). Inter- and intra-sex habitat partitioning in the highly dimorphic southern elephant seal. Ecol. Evol. 11, 1620–1633. doi: 10.1002/ece3.7147
Hindell, M. A., Reisinger, R. R., Ropert-Coudert, Y., Hückstädt, L. A., Trathan, P. N., Bornemann, H., et al. (2020). Tracking of marine predators to protect Southern Ocean ecosystems. Nature 580, 87–92. doi: 10.1038/s41586-020-2126-y
Hindell, M. A., Sumner, M., Bestley, S., Wotherspoon, S., Harcourt, R., Lea, M.-A., et al. (2017). Decadal changes in habitat characteristics influence population trajectories of southern elephant seals. Glob. Change Biol. 23, 5136–5150. doi: 10.1111/gcb.13776
Hoenner, X., Whiting, S. D., Hindell, M. A., and McMahon, C. R. (2012). Enhancing the use of Argos satellite data for home range and long distance migration studies of marine animals. PLoS One 7:e40713. doi: 10.1371/journal.pone.0040713
Holton, M. D., Wilson, R. P., Teilmann, J., and Siebert, U. (2021). Animal tag technology keeps coming of age: an engineering perspective. Philos. Trans. R. Soc. B Biol. Sci. 376:20200229. doi: 10.1098/rstb.2020.0229
Hooker, S. K., and Boyd, I. L. (2003). Salinity sensors on seals: use of marine predators to carry CTD data loggers. Deep Sea Res. I Oceanogr. Res. Pap. 50, 927–939. doi: 10.1016/S0967-0637(03)00055-4
Hooker, S. K., Boyd, I. L., Jessopp, M., Cox, O., Blackwell, J., Boveng, P. L., et al. (2002). Monitoring the prey-field of marine predators: combining digital imaging with datalogging tags. Mar. Mamm. Sci. 18, 680–697. doi: 10.1111/j.1748-7692.2002.tb01066.x
Horning, M., Andrews, R. D., Bishop, A. M., Boveng, P. L., Costa, D. P., Crocker, D. E., et al. (2019). Best practice recommendations for the use of external telemetry devices on pinnipeds. Anim. Biotelem. 7:20. doi: 10.1186/s40317-019-0182-6
Hückstädt, L. A., Piñones, A., Palacios, D. M., McDonald, B. I., Dinniman, M. S., Hofmann, E. E., et al. (2020). Projected shifts in the foraging habitat of crabeater seals along the Antarctic Peninsula. Nat. Clim. Change 10, 472–477. doi: 10.1038/s41558-020-0745-9
Hussey, N. E., Kessel, S. T., Aarestrup, K., Cooke, S. J., Cowley, P. D., Fisk, A. T., et al. (2015). Aquatic animal telemetry: a panoramic window into the underwater world. Science 348:1221. doi: 10.1126/science.1255642
Irvine, L. M., Winsor, M. H., Follett, T. M., Mate, B. R., and Palacios, D. M. (2020). An at-sea assessment of Argos location accuracy for three species of large whales, and the effect of deep-diving behavior on location error. Anim. Biotelem. 8:20. doi: 10.1186/s40317-020-00207-x
Isachsen, P. E., Sørlie, S. R., Mauritzen, C., Lydersen, C., Dodd, P., and Kovacs, K. M. (2014). Upper-ocean hydrography of the Nordic Seas during the International Polar Year (2007–2008) as observed by instrumented seals and Argo floats. Deep Sea Res. I Oceanogr. Res. Pap. 93, 41–59. doi: 10.1016/j.dsr.2014.06.012
Jabour-Green, J., and Bradshaw, C. J. A. (2004). The “capacity to reason” in conservation biology and policy: the southern elephant seal branding controversy. J. Nat. Conserv. 12, 25–39. doi: 10.1016/j.jnc.2004.04.001
Jeffers, V. F., and Godley, B. J. (2016). Satellite tracking in sea turtles: how do we find our way to the conservation dividends? Biol. Conserv. 199, 172–184. doi: 10.1016/j.biocon.2016.04.032
Jonsen, I. D., Basson, M., Bestley, S., Bravington, M. V., Patterson, T. A., Pedersen, M. W., et al. (2013). State-space models for bio-loggers: a methodological road map. Deep Sea Res. II Top. Stud. Oceanogr. 88-89, 34–46. doi: 10.1016/j.dsr2.2012.07.008
Jonsen, I., McMahon, C. R., Patterson, T., Auger-Methe, M., Harcourt, R., Hindell, M. A., et al. (2018). Movement behaviour responses to environment: fast inference of individual variation with a mixed effects model. Ecology 10:e02566. doi: 10.1101/314690
Jonsen, I., Patterson, T. A., Doherty, P. D., Godley, B. J., Grecian, W. J., Guinet, C., et al. (2020). A continuous-time state-space model for rapid quality-control of Argos locations from animal-borne tags. Mov. Ecol. 8:31. doi: 10.1186/s40462-020-00217-7
Jouffray, J.-B., Blasiak, R., Norström, A. V., Österblom, H., and Nyström, M. (2020). The blue acceleration: the trajectory of human expansion into the ocean. One Earth 2, 43–54. doi: 10.1016/j.oneear.2019.12.016
Keates, T. R., Kudela, R. M., Holser, R. R., Hückstädt, L. A., Simmons, S. E., and Costa, D. P. (2020). Chlorophyll fluorescence as measured in situ by animal-borne instruments in the northeastern Pacific Ocean. J. Mar. Syst. 203:103265. doi: 10.1016/j.jmarsys.2019.103265
Kitade, Y., Shimada, K., Tamura, T., Williams, G. D., Aoki, S., Fukamachi, Y., et al. (2014). Antarctic bottom water production from the vincennes Bay Polynya, East Antarctica. Geophys. Res. Lett. 41, 3528–3534. doi: 10.1002/2014GL059971
Koopman, H., Westgate, A., Siders, Z., and Cahoon, L. (2014). Rapid subsurface ocean warming in the bay of fundy as measured by free-swimming basking sharks. Oceanography 27, 14–16. doi: 10.5670/oceanog.2014.32
Kraska, J., Crespo, G. O., and Johnston, D. W. (2015). Bio-logging of marine migratory species in the law of the sea. Mar. Policy 51, 394–400. doi: 10.1016/j.marpol.2014.08.016
Labrousse, S., Orgeret, F., Solow, A. R., Barbraud, C., Bost, C. A., Sallée, J. B., et al. (2019). First odyssey beneath the sea ice of juvenile emperor penguins in East Antarctica. Mar. Ecol. Progr. Ser. 609, 1–16. doi: 10.3354/meps12831
Labrousse, S., Ryan, S., Roquet, F., Picard, B., McMahon, C. R., Harcourt, R., et al. (2021). Weddell seal behaviour during an exceptional oceanographic event in the Filchner-Ronne Ice Shelf in 2017. Antarct. Sci. 33, 252–264. doi: 10.1017/S0954102021000092
Labrousse, S., Sallee, J. B., Fraser, A., Massom, R., Reid, P., Hobbs, W., et al. (2017a). Variability in sea ice cover and climate elicit sex specific responses in an Antarctic predator. Sci. Rep. 7:43236. doi: 10.1038/srep43236
Labrousse, S., Sallée, J. B., Fraser, A. D., Massom, R., Reid, P., Sumner, M., et al. (2017b). Under the sea ice: exploring the relationship between sea ice and the foraging behaviour of southern elephant seals in East Antarctica. Progr. Oceanogr. 156, 17–40. doi: 10.1016/j.pocean.2017.05.014
Labrousse, S., Williams, G., Tamura, T., Bestley, S., Sallee, J. B., Fraser, A. D., et al. (2018). Coastal polynyas: winter oases for subadult southern elephant seals in East Antarctica. Sci. Rep. 8:3183. doi: 10.1038/s41598-018-21388-9
Laidre, K. L., Heide-Jorgensen, M. P., and Dietz, R. (2002). Diving behaviour of narwhals (Monodon monoceros) at two coastal localities in the Canadian High Arctic. Can. J. Zool. 80, 624–635. doi: 10.1139/z02-041
Lédée, E. J. I., Heupel, M. R., Taylor, M. D., Harcourt, R. G., Jaine, F. R. A., Huveneers, C., et al. (2021). Continental-scale acoustic telemetry and network analysis reveal new insights into stock structure. Fish Fish. 22, 987–1005. doi: 10.1111/faf.12565
Lennox, R. J., Harcourt, R., Bennett, J. R., Davies, A., Ford, A. T., Frey, R. M., et al. (2020). A novel framework to protect animal data in a world of eco-surveillance. BioScience 70, 468–476. doi: 10.1093/biosci/biaa035
Lindstrom, E., Gunn, J., Fischer, A., McCurdy, A., Glover, L. K., and Members, T. T. (2012). Framework for Ocean Observing: Prepared for the Task Team for an Integrated Framework for Sustained Ocean Observing (IFSOO). IOC Information Documents, 1284. Paris: UNESCO. doi: 10.5270/OceanObs09-FOO
Lopez, R., Malardé, J.-P., Danès, P., and Gaspar, P. (2015). Improving Argos doppler location using multiple-model smoothing. Anim. Biotelem. 3:32. doi: 10.1186/s40317-015-0073-4
Lowther, A. D., Harcourt, R. G., Page, B., and Goldsworthy, S. D. (2013). Steady as he goes: at-sea movement of adult male Australian sea lions in a dynamic marine environment. PLoS One 8:e74348. doi: 10.1371/journal.pone.0074348
Lowther, A. D., Lydersen, C., Fedak, M. A., Lovell, P., and Kovacs, K. M. (2015). The Argos-CLS kalman filter: error structures and state-space modelling relative to fastloc GPS Data. PLoS One 10:e0124754. doi: 10.1371/journal.pone.0124754
Lydersen, C., Anders Nost, O., Kovacs, K. M., and Fedak, M. A. (2004). Temperature data from Norwegian and Russian waters of the northern Barents Sea collected by free-living ringed seals. J. Mar. Syst. 46, 99–108. doi: 10.1016/j.jmarsys.2003.11.019
Lydersen, C., Anders Nost, O., Lovell, P., McConnell, B. J., Gammelsrod, T., Hunter, C., et al. (2002). Salinity and temperature structure of a freezing Arctic fjord—monitored by white whales (Delphinapterus leucas). Geophys. Res. Lett. 29:2119. doi: 10.1029/2002GL015462
Mallet, H. K. W., Boehme, L., Fedak, M., Heywood, K. J., Stevens, D. P., and Roquet, F. (2018). Variation in the distribution and properties of circumpolar deep water in the Eastern Amundsen Sea, on seasonal timescales, using seal-borne tags. Geophys. Res. Lett. 45, 4982–4990. doi: 10.1029/2018GL077430
Malpress, V., Bestley, S., Corney, S., Welsford, D., Labrousse, S., Sumner, M., et al. (2017). Bio-physical characterisation of polynyas as a key foraging habitat for juvenile male southern elephant seals (Mirounga leonina) in Prydz Bay, East Antarctica. PLoS One 12: e0184536. doi: 10.1371/journal.pone.0184536
March, D., Boehme, L., Tintore, J., Velez-Belchi, P. J., and Godley, B. J. (2020). Towards the integration of animal-borne instruments into global ocean observing systems. Glob. Change Biol. 26, 586–596. doi: 10.1111/gcb.14902
Maxwell, S. M., Breed, G. A., Nickel, B. A., Makanga-Bahouna, J., Pemo-Makaya, E., Parnell, R. J., et al. (2011). Using satellite tracking to optimize protection of long-lived marine species: olive ridley sea turtle conservation in Central Africa. PLoS One 6:e19905. doi: 10.1371/journal.pone.0019905
Maxwell, S. M., Hazen, E. L., Lewison, R. L., Dunn, D. C., Bailey, H., Bograd, S. J., et al. (2015). Dynamic ocean management: defining and conceptualizing real-time management of the ocean. Mar. Policy 58, 42–50. doi: 10.1016/j.marpol.2015.03.014
McGowan, J., Beger, M., Lewison, R. L., Harcourt, R., Campbell, H., Priest, M., et al. (2017). Integrating research using animal-borne telemetry with the needs of conservation management. J. Appl. Ecol. 54, 423–429. doi: 10.1111/1365-2664.12755
McIntyre, T. (2014). Trends in tagging of marine mammals: a review of marine mammal biologging studies. Afr. J. Mar. Sci. 36, 409–422. doi: 10.2989/1814232X.2014.976655
McLaughlin, R. J. (2015). Bio-logging as marine scientific research under the law of the sea: a commentary responding to James Kraska, Guillermo Ortuño Crespo, David W. Johnston, bio-logging of marine migratory species in the law of the sea, marine policy 51 (2015) 394–400. Mar. Policy 60, 178–181. doi: 10.1016/j.marpol.2015.06.010
McMahon, C. R., Autret, E., Houghton, J. D. R., Lovell, P., Myers, A. E., and Hays, G. C. (2005). Animal-borne sensors successfully capture the real-time thermal properties of ocean basins. Limnol. Oceanogr. Methods 3, 392–398. doi: 10.4319/lom.2005.3.392
McMahon, C. R., Bradshaw, C. J. A., and Hays, G. C. (2007). Satellite tracking reveals unusual diving characteristics for a marine reptile, the olive ridley turtle (Lepidochelys olivacea). Mar. Ecol. Progr. Ser. 329, 239–252. doi: 10.3354/meps329239
McMahon, C. R., Burton, H. R., McLean, S., Slip, D., and Bester, M. N. (2000). Field immobilisation of southern elephant seals with intravenous tiletamine and zolazepam. Vet. Rec. 146, 251–254. doi: 10.1136/vr.146.9.251
McMahon, C. R., Field, I. C., Bradshaw, C. J. A., White, G. C., and Hindell, M. A. (2008). Tracking and data-logging devices attached to elephant seals do not affect individual mass gain or survival. J. Exp. Mar. Biol. Ecol. 360, 71–77. doi: 10.1016/j.jembe.2008.03.012
McMahon, C. R., Harcourt, R. G., Burton, H. R., Daniel, O., and Hindell, M. A. (2017). Seal mothers expend more on offspring under favourable conditions and less when resources are limited. J. Anim. Ecol. 86, 359–370. doi: 10.1111/1365-2656.12611
McMahon, C. R., Harcourt, R., Bateson, P. P., and Hindell, M. A. (2012). Animal welfare and decision making in wildlife research. Biol. Conserv. 153, 254–256. doi: 10.1016/j.biocon.2012.05.004
Mensah, V., Roquet, F., Siegelman-Charbit, L., Picard, B., Pauthenet, E., and Guinet, C. (2018). A correction for the thermal mass–induced errors of CTD tags mounted on marine mammals. J. Atmos. Ocean. Technol. 35, 1237–1252. doi: 10.1175/JTECH-D-17-0141.1
Meyer, C. G., Anderson, J. M., Coffey, D. M., Hutchinson, M. R., Royer, M. A., and Holland, K. N. (2018). Habitat geography around Hawaii’s oceanic islands influences tiger shark (Galeocerdo cuvier) spatial behaviour and shark bite risk at ocean recreation sites. Sci. Rep. 8:4945. doi: 10.1038/s41598-018-23006-0
Miloslavich, P., Seeyave, S., Muller-Karger, F., Bax, N., Ali, E., Delgado, C., et al. (2018). Challenges for global ocean observation: the need for increased human capacity. J. Operat. Oceanogr. 12, S137–S156. doi: 10.1080/1755876X.2018.1526463
Miyazawa, Y., Kuwano-Yoshida, A., Doi, T., Nishikawa, H., Narazaki, T., Fukuoka, T., et al. (2018). Temperature profiling measurements by sea turtles improve ocean state estimation in the kuroshio-oyashio confluence region. Ocean Dyn. 69, 267–282. doi: 10.1007/s10236-018-1238-5
Moltmann, T., Turton, J., Zhang, H.-M., Nolan, G., Gouldman, C., Griesbauer, L., et al. (2019). A Global Ocean Observing System (GOOS), delivered through enhanced collaboration across regions, communities, and new technologies. Front. Mar. Sci. 6:291. doi: 10.3389/fmars.2019.00291
Nachtsheim, D. A., Jerosch, K., Hagen, W., Plotz, J., and Bornemann, H. (2017). Habitat modelling of crabeater seals (Lobodon carcinophaga) in the Weddell Sea using the multivariate approach Maxent. Polar Biol. 40, 961–976. doi: 10.1007/s00300-016-2020-0
Nachtsheim, D. A., Ryan, S., Schröder, M., Jensen, L., Oosthuizen, W. C., Bester, M. N., et al. (2019). Foraging behaviour of Weddell seals (Leptonychotes weddellii) in connection to oceanographic conditions in the southern Weddell Sea. Progr. Oceanogr. 173, 165–179. doi: 10.1016/j.pocean.2019.02.013
Naito, Y., Costa, D. P., Adachi, T., Robinson, P. W., Fowler, M., Takahashi, A., et al. (2013). Unravelling the mysteries of a mesopelagic diet: a large apex predator specializes on small prey. Funct. Ecol. 27, 710–717. doi: 10.1111/1365-2435.12083
Naito, Y., Costa, D. P., Adachi, T., Robinson, P. W., Peterson, S. H., Mitani, Y., et al. (2017). Oxygen minimum zone: an important oceanographic habitat for deep-diving northern elephant seals, Mirounga angustirostris. Ecol. Evol. 7, 6259–6270. doi: 10.1002/ece3.3202
Nakanowatari, T., Ohshima, K. I., Mensah, V., Mitani, Y., Hattori, K., Kobayashi, M., et al. (2017). Hydrographic observations by instrumented marine mammals in the Sea of Okhotsk. Polar Sci. 13, 56–65. doi: 10.1016/j.polar.2017.06.001
Nankey, P., Filippi, N., Kuhn, C. E., Dickerson, B., and Liwanag, H. E. M. (2021). Under pressure: effects of instrumentation methods on fur seal pelt function. Mar. Mamm. Sci. 37, 1363–1374. doi: 10.1111/mms.12817
Narayanan, A., Gille, S. T., Mazloff, M. R., and Murali, K. (2019). Water mass characteristics of the Antarctic margins and the production and seasonality of dense shelf water. J. Geophys. Res. Oceans 124, 9277–9294. doi: 10.1029/2018JC014907
Newman, L., Heil, P., Trebilco, R., Katsumata, K., Constable, A., van Wijk, E., et al. (2019). Delivering sustained, coordinated, and integrated observations of the Southern Ocean for global impact. Front. Mar. Sci. 6:433. doi: 10.3389/fmars.2019.00433
Nguyen, V. M., Brooks, J. L., Young, N., Lennox, R. J., Haddaway, N., Whoriskey, F. G., et al. (2017). To share or not to share in the emerging era of big data: perspectives from fish telemetry researchers on data sharing. Can. J. Fish. Aquat. Sci. 74, 1260–1274. doi: 10.1139/cjfas-2016-0261
Ohshima, K. I., Fukamachi, Y., Williams, G. D., Nihashi, S., Roquet, F., Kitade, Y., et al. (2013). Antarctic bottomwater production by intense sea-ice formation in the cape darnley polynya. Nat. Geosci. 6, 235–240. doi: 10.1038/ngeo1738
Ohshima, K. I., Nihashi, S., and Iwamoto, K. (2016). Global view of sea-ice production in polynyas and its linkage to dense bottom water formation. Geosci. Lett. 3:13. doi: 10.1186/s40562-016-0045-4
Pauthenet, E., Roquet, F., Madec, G., Guinet, C., Hindell, M., McMahon, C. R., et al. (2018). Seasonal meandering of the polar front upstream of the kerguelen plateau. Geophys. Res. Lett. 45, 9767–9774. doi: 10.1029/2018GL079614
Photopoulou, T., Heerah, K., Pohle, J., and Boehme, L. (2020). Sex-specific variation in the use of vertical habitat by a resident Antarctic top predator. Proc. R. Soc. B Biol. Sci. 287:20201447. doi: 10.1098/rspb.2020.1447
Piñones, A., Hofmann, E. E., Costa, D. P., Goetz, K., Burns, J. M., Roquet, F., et al. (2019). Hydrographic variability along the inner and mid-shelf region of the western Ross Sea obtained using instrumented seals. Progr. Oceanogr. 174, 131–142. doi: 10.1016/j.pocean.2019.01.003
Queiroz, N., Humphries, N. E., Couto, A., Vedor, M., da Costa, I., Sequeira, A. M. M., et al. (2019). Global spatial risk assessment of sharks under the footprint of fisheries. Nature 572, 461–466. doi: 10.1038/s41586-019-1444-4
Queste, B. Y., Abrahamsen, E. P., du Plessis, M. D., Gille, S. T., Gregor, L., Mazloff, M. R., et al. (2020). Southern Ocean. Bull. Am. Meteorol. Soc. 101, S307–S309.
Rayner, R., Jolly, C., and Gouldman, C. (2019). Ocean observing and the blue economy. Front. Mar. Sci. 6:330. doi: 10.3389/fmars.2019.00330
Riser, S. C., Freeland, H. J., Roemmich, D., Wijffels, S., Troisi, A., Belbéoch, M., et al. (2016). Fifteen years of ocean observations with the global Argo array. Nat. Clim. Change 6, 145–153. doi: 10.1038/nclimate2872
Robinson, P. W., Costa, D. P., Crocker, D. E., Gallo-Reynoso, J. P., Champagne, C. D., Fowler, M. A., et al. (2012). Foraging behavior and success of a mesopelagic predator in the northeast Pacific Ocean: insights from a data-rich species, the northern elephant seal. PLoS One 7:e36728. doi: 10.1371/journal.pone.0036728
Roemmich, D., Alford, M. H., Claustre, H., Johnson, K., King, B., Moum, J., et al. (2019). On the future of Argo: a global, full-depth, multi-disciplinary array. Front. Mar. Sci. 6:439. doi: 10.3389/fmars.2019.00439
Roemmich, D., Church, J., Gilson, J., Monselesan, D., Sutton, P., and Wijffels, S. (2015). Unabated planetary warming and its ocean structure since 2006. Nat. Clim. Change 5, 240–245. doi: 10.1038/nclimate2513
Ropert-Coudert, Y., Van de Putte, A. P., Reisinger, R. R., Bornemann, H., Charrassin, J.-B., Costa, D. P., et al. (2020). The retrospective analysis of Antarctic tracking data project. Sci. Data 7:94. doi: 10.1038/s41597-020-0406-x
Roquet, F., Boehme, L., Block, B. A., Charrassin, J. B., Costa, D., Guinet, C., et al. (2017). Ocean observations using tagged animals. Oceanography 30:139. doi: 10.5670/oceanog.2017.235
Roquet, F., Charrassin, J. B., Marchand, S., Boehme, L., Fedak, M., Reverdin, G., et al. (2011). Delayed-mode calibration of hydrographic data obtained from animal-borne satellite relay data loggers. J. Atmos. Ocean. Technol. 28, 787–801. doi: 10.1175/2010JTECHO801.1
Roquet, F., Williams, G. D., Hindell, M., Harcourt, R., McMahon, C. R., Charrassin, J. B., et al. (2014). A Southern Indian ocean database of hydrographic profiles obtained with instrumented elephant seals. Sci. Data 1:140028. doi: 10.1038/sdata.2014.28
Roquet, F., Wunsch, C., Forget, G., Heimbach, P., Guinet, C., Reverdin, G., et al. (2013). Estimates of the Southern Ocean general circulation improved by animal-borne instruments. Geophys. Res. Lett. 40, 6176–6180. doi: 10.1002/2013GL058304
Sauzede, R., Lavigne, H., Claustre, H., Uitz, J., Schmechtig, C., D’Ortenzio, F., et al. (2015). Vertical distribution of chlorophyll a concentration and phytoplankton community composition from in situ fluorescence profiles: a first database for the global ocean. Earth Syst. Sci. Data 7, 261–273. doi: 10.5194/essd-7-261-2015
Scheffer, A., Bost, C. A., and Trathan, P. N. (2012). Frontal zones, temperature gradient and depth characterize the foraging habitat of king penguins at South Georgia. Mar. Ecol. Progr. Ser. 465, 281–297. doi: 10.3354/meps09884
Scheffer, A., Trathan, P. N., Edmonston, J. G., and Bost, C.-A. (2016). Combined influence of meso-scale circulation and bathymetry on the foraging behaviour of a diving predator, the king penguin (Aptenodytes patagonicus). Progr. Oceanogr. 141, 1–16. doi: 10.1016/j.pocean.2015.10.005
Sequeira, A. M. M., Hays, G. C., Sims, D. W., Eguíluz, V. M., Rodríguez, J. P., Heupel, M. R., et al. (2019a). Overhauling ocean spatial planning to improve marine megafauna conservation. Front. Mar. Sci. 6:639. doi: 10.3389/fmars.2019.00639
Sequeira, A. M. M., Heupel, M. R., Lea, M. A., Eguíluz, V. M., Duarte, C. M., Meekan, M. G., et al. (2019b). The importance of sample size in marine megafauna tagging studies. Ecol. Appl. 29:e01947. doi: 10.1002/eap.1947
Sequeira, A. M. M., O’Toole, M., Keats, T. R., McDonnell, L. H., Braun, C. D., Hoenner, X., et al. (2021). A standardisation framework for bio-logging data to advance ecological research and conservation. Methods Ecol. Evol. 12, 959–961. doi: 10.1111/2041-210X.13593
Sequeira, A. M. M., Rodriguez, J. C., Eguiìluza, V. M., Harcourt, R., Hindell, M., Sims, D. W., et al. (2018). Convergence of marine megafauna movement patterns in coastal and open oceans. Proc. Natl. Acad. Sci. U.S.A. 115, 3072–3077. doi: 10.1073/pnas.1716137115
Siegelman, L., Roquet, F., Mensah, V., Riviere, P., Pauthenet, E., Picard, B., et al. (2019). Correction and accuracy of high- and low-resolution CTD data from animal-borne instruments. J. Atmos. Ocean. Technol. 36, 745–760. doi: 10.1175/JTECH-D-18-0170.1
Silva, M. A., Jonsen, I., Russell, D. J. F., Prieto, R., Thompson, D., and Baumgartner, M. F. (2014). Assessing performance of bayesian state-space models fit to argos satellite telemetry locations processed with kalman filtering. PLoS One 9, e92277. doi: 10.1371/journal.pone.0092277
Silvano, A., Rintoul, S. R., Kusahara, K., Peña-Molino, B., Wijk, E., Gwyther, D. E., et al. (2019). Seasonality of warm water intrusions onto the continental shelf near the Totten Glacier. J. Geophys. Res. Oceans 124, 4272-4289. doi: 10.1029/2018JC014634
Silvano, A., Rintoul, S. R., Pena-Molino, B., Hobbs, W. R., van Wijk, E., Aoki, S., et al. (2018). Freshening by glacial meltwater enhances melting of ice shelves and reduces formation of Antarctic Bottom Water. Sci. Adv. 4:eaa9467. doi: 10.1126/sciadv.aap9467
Silvano, A., Rintoul, S., and Herraiz-Borreguero, L. (2016). Ocean-ice shelf interaction in East Antarctica. Oceanography 29, 130–143. doi: 10.5670/oceanog.2016.105
Simmons, S. E., Tremblay, Y., and Costa, D. P. (2009). Pinnipeds as ocean-temperature samplers: calibrations, validations, and data quality. Limnol. Oceanogr. Methods 7, 648–656. doi: 10.4319/lom.2009.7.648
Smith, G. C., Allard, R., Babin, M., Bertino, L., Chevallier, M., Corlett, G., et al. (2019). Polar ocean observations: a critical gap in the observing system and its effect on environmental predictions from hours to a season. Front. Mar. Sci. 6, 1–28. doi: 10.3389/fmars.2019.00429
Sokolov, L. V. (2011). Modern telemetry: new possibilities in ornithology. Biol. Bull. 38, 885–904. doi: 10.1134/S1062359011090081
Stramma, L., Johnson, G. C., Sprintall, J., and Mohrholz, V. (2008). Expanding oxygen-minimum zones in the tropical oceans. Science 320, 655–658. doi: 10.1126/science.1153847
Tamura, T., Ohshima, K. I., Fraser, A. D., and Williams, G. D. (2016). Sea ice production variability in Antarctic coastal polynyas. J. Geophys. Res. Oceans 121, 2967–2979. doi: 10.1002/2015JC011537
Tanhua, T., McCurdy, A., Fischer, A., Appeltans, W., Bax, N., Currie, K., et al. (2019). What we have learned from the framework for ocean observing: evolution of the global ocean observing system. Front. Mar. Sci. 6:471. doi: 10.3389/fmars.2019.00471
Teilmann, J., Agersted, M. D., and Heide-Jørgensen, M. P. (2020). A comparison of CTD satellite-linked tags for large cetaceans - Bowhead whales as real-time autonomous sampling platforms. Deep Sea Res. I Oceanogr. Res. Pap. 157:103213. doi: 10.1016/j.dsr.2020.103213
Thomson, J. A., Börger, L., Christianen, M. J. A., Esteban, N., Laloë, J. O., and Hays, G. C. (2017). Implications of location accuracy and data volume for home range estimation and fine-scale movement analysis: comparing Argos and Fastloc-GPS tracking data. Mar. Biol. 164:204. doi: 10.1007/s00227-017-3225-7
Thums, M., Rossendell, J., Guinea, M., and Ferreira, L. C. (2018). Horizontal and vertical movement behaviour of flatback turtles and spatial overlap with industrial development. Mar. Ecol. Progr. Ser. 602, 237–253. doi: 10.3354/meps12650
Tournier, M., Goulet, P., Johnson, M., Nerini, D., Fonvieille, N., and Guinet, C. (2021). A novel animal-borne miniature echosounder to observe the distribution and migration patterns of intermediate trophic levels in the Southern Ocean. J. Mar. Syst. 223:103608. doi: 10.1016/j.jmarsys.2021.103608
Treasure, A. M., Roquet, F., Ansorge, I. J., Bester, M. N., Boehme, L., Bornemann, H., et al. (2017). Marine mammals exploring the oceans pole to pole: a review of the MEOP consortium. Oceanography 30, 62–68. doi: 10.5670/oceanog.2017.234
Tsontos, V. M., Lam, C., and Arms, S. C. (2020). netCDF Templates for Electronic Tagging Data. JPL URS CL, 19-6563. doi: 10.6084/m6569.figshare.10159820
Tverberg, V., Nøst, O. A., Lydersen, C., and Kovacs, K. M. (2014). Winter sea ice melting in the Atlantic water subduction area, Svalbard Norway. J. Geophys. Res. Oceans 119, 5945–5967. doi: 10.1002/2014JC010013
Vacquie-Garcia, J., Mallefet, J., Bailleul, F., Picard, B., and Guinet, C. (2017b). Marine bioluminescence: measurement by a classical light sensor and related foraging behavior of a deep diving predator. Photochem. Photobiol. 93, 1312–1319. doi: 10.1111/php.12776
Vacquie-Garcia, J., Lydersen, C., Biuw, M., Haug, T., Fedak, M. A., and Kovacs, K. M. (2017a). Hooded seal Cystophora cristata foraging areas in the Northeast Atlantic ocean-investigated using three complementary methods. PLoS One 12:e0187889. doi: 10.1371/journal.pone.0187889
Vandeperre, F., Aires-da-Silva, A., Fontes, J., Santos, M., Serrao Santos, R., and Afonso, P. (2014). Movements of blue sharks (Prionace glauca) across their life history. PLoS One 9:e103538. doi: 10.1371/journal.pone.0103538
Vincent, C., McConnell, B. J., Ridoux, V., and Fedak, M. A. (2002). Assessment of Argos location accuracy from satellite tags deployed on captive gray seals. Mar. Mamm. Sci. 18, 156–166. doi: 10.1111/j.1748-7692.2002.tb01025.x
von Schuckmann, K., Palmer, M. D., Trenberth, K. E., Cazenave, A., Chambers, D., Champollion, N., et al. (2016). An imperative to monitor Earth’s energy imbalance. Nat. Clim. Change 6, 138–144. doi: 10.1038/nclimate2876
Wall, S. M., Bradshaw, C. J. A., Southwell, C. J., Gales, N. J., and Hindell, M. A. (2007). Crabeater seal diving behaviour in eastern Antarctica. Mar. Ecol. Progr. Ser. 337, 265–277. doi: 10.3354/meps337265
Watanabe, Y., Bornemann, H., Liebsch, N., Plotz, J., Sato, K., Naito, Y., et al. (2006). Seal-mounted cameras detect invertebrate fauna on the underside of an Antarctic ice shelf. Mar. Ecol. Progr. Ser. 309, 297–300. doi: 10.3354/meps309297
Wege, M., de Bruyn, P. J. N., Hindell, M. A., Lea, M. A., and Bester, M. N. (2019). Preferred, small-scale foraging areas of two Southern ocean fur seal species are not determined by habitat characteristics. BMC Ecol. 19:36. doi: 10.1186/s12898-019-0252-x
Weimerskirch, H., Wilson, R. P., Guinet, C., and Koudil, M. (1995). Use of seabirds to monitor sea-surface temperatures and to validate satellite remote-sensing measurements in the Southern Ocean. Mar. Ecol. Progr. Ser. 126, 299–303. doi: 10.3354/meps126299
Weise, M. J., Harvey, J. T., and Costa, D. P. (2010). The role of body size in individual-based foraging strategies of a top marine predator. Ecology 91, 1004–1015. doi: 10.1890/08-1554.1
Wilkinson, M. D., Dumontier, M., Aalbersberg, I. J., Appleton, G., Axton, M., Baak, A., et al. (2016). The FAIR guiding principles for scientific data management and stewardship. Sci. Data 3:60018. doi: 10.1038/sdata.2016.18
Williams, G. D., Herraiz-Borreguero, L., Roquet, F., Tamura, K., Ohshima, K. I., Fukamachi, Y., et al. (2016). The suppression of Antarctic bottom water formation by melting ice shelves in Prydz Bay. Nat. Commun. 7:12577. doi: 10.1038/ncomms12577
Wilson, R. P., and McMahon, C. R. (2006). Measuring devices on wild animals: what constitutes acceptable practice? Front. Ecol. Environ. 4:147–154. doi: 10.1890/1540-9295(2006)004[0147:MDOWAW]2.0.CO;2
Witt, M. J., Åkesson, S., Broderick, A. C., Coyne, M. S., Ellick, J., Formia, A., et al. (2010). Assessing accuracy and utility of satellite-tracking data using Argos-linked Fastloc-GPS. Anim. Behav. 80, 571–581. doi: 10.1016/j.anbehav.2010.05.022
Wong, A., Keeley, R., Carval, T., and Argo Data Management Team (2021). Argo Quality Control Manual for CTD and Trajectory Data. Brest: Ifremer.
Yoda, K., Shiomi, K., and Sato, K. (2014). Foraging spots of streaked shearwaters in relation to ocean surface currents as identified using their drift movements. Progr. Oceanogr. 122, 54–64. doi: 10.1016/j.pocean.2013.12.002
Keywords: animal behavior, climate change, Essential Ocean Variables (EOVs), marine animals, physical oceanography
Citation: McMahon CR, Roquet F, Baudel S, Belbeoch M, Bestley S, Blight C, Boehme L, Carse F, Costa DP, Fedak MA, Guinet C, Harcourt R, Heslop E, Hindell MA, Hoenner X, Holland K, Holland M, Jaine FRA, Jeanniard du Dot T, Jonsen I, Keates TR, Kovacs KM, Labrousse S, Lovell P, Lydersen C, March D, Mazloff M, McKinzie MK, Muelbert MMC, O’Brien K, Phillips L, Portela E, Pye J, Rintoul S, Sato K, Sequeira AMM, Simmons SE, Tsontos VM, Turpin V, van Wijk E, Vo D, Wege M, Whoriskey FG, Wilson K and Woodward B (2021) Animal Borne Ocean Sensors – AniBOS – An Essential Component of the Global Ocean Observing System. Front. Mar. Sci. 8:751840. doi: 10.3389/fmars.2021.751840
Received: 02 August 2021; Accepted: 12 October 2021;
Published: 05 November 2021.
Edited by:
Johannes Karstensen, GEOMAR Helmholtz Center for Ocean Research Kiel, GermanyReviewed by:
Taavi Liblik, Tallinn University of Technology, EstoniaCopyright © 2021 McMahon, Roquet, Baudel, Belbeoch, Bestley, Blight, Boehme, Carse, Costa, Fedak, Guinet, Harcourt, Heslop, Hindell, Hoenner, Holland, Holland, Jaine, Jeanniard du Dot, Jonsen, Keates, Kovacs, Labrousse, Lovell, Lydersen, March, Mazloff, McKinzie, Muelbert, O’Brien, Phillips, Portela, Pye, Rintoul, Sato, Sequeira, Simmons, Tsontos, Turpin, van Wijk, Vo, Wege, Whoriskey, Wilson and Woodward. This is an open-access article distributed under the terms of the Creative Commons Attribution License (CC BY). The use, distribution or reproduction in other forums is permitted, provided the original author(s) and the copyright owner(s) are credited and that the original publication in this journal is cited, in accordance with accepted academic practice. No use, distribution or reproduction is permitted which does not comply with these terms.
*Correspondence: Clive R. McMahon, Y2xpdmUubWNtYWhvbkB1dGFzLmVkdS5hdQ==
Disclaimer: All claims expressed in this article are solely those of the authors and do not necessarily represent those of their affiliated organizations, or those of the publisher, the editors and the reviewers. Any product that may be evaluated in this article or claim that may be made by its manufacturer is not guaranteed or endorsed by the publisher.
Research integrity at Frontiers
Learn more about the work of our research integrity team to safeguard the quality of each article we publish.