- 1Department of Applied Science, R.O.C. Naval Academy, Kaohsiung, Taiwan
- 2Department of Oceanography, National Sun Yat-sen University, Kaohsiung, Taiwan
- 3Research Center for Environmental Changes, Academia Sinica, Taipei, Taiwan
- 4Institute of Marine Environment and Ecology, National Taiwan Ocean University, Keelung, Taiwan
- 5Center of Excellence for the Oceans, National Taiwan Ocean University, Keelung, Taiwan
- 6Institute of Fisheries Science, National Taiwan University, Taipei, Taiwan
Satellite-based observations of primary production (PP) are broadly used to assess carbon fixation rate of phytoplankton in the global ocean with small spatiotemporal limitations. However, the remote sensing can only reach the ocean surface, the assumption of a PP vertically exponential decrease with increasing depth from the surface to the bottom of euphotic zone may cause a substantial and potential discrepancy between in situ measurements and satellite-based observations of PP. This study compared euphotic zone integrated PP derived from measurements based on ship-based in situ incubation (i.e., PPin situ) and those derived from the satellite-based vertically generalized production model (VGPM; PPVGPM) for the period 2003∼2016 at the South East Asian Time-series Study (SEATS) station. PP values obtained during the NE-monsoon (NEM: Nov∼Mar; PPin situ = 323 ± 134; PPVGPM = 443 ± 142 mg-C m–2 d–1) were ∼2-fold higher than those recorded during the SW-monsoon (SWM: Apr∼Oct; PPin situ = 159 ± 58; PPVGPM = 250 ± 36 mg-C m–2 d–1), regardless of the method used for derivation. The main reason for the higher PP values during the NEM appears to have been a greater abundance of inorganic nutrients were made available by vertical advection. Note that on average, PPin situ estimates were ∼50% lower than PPVGPM estimates, regardless of the monsoon. These discrepancies can be mainly attributed to differences from the euphotic zone depth between satellite-based and in situ measurements. The significantly negative relationship between PP measurements obtained in situ and sea surface temperatures observed throughout this study demonstrates that both methods are effective indicators in estimating PP. Overall, our PPin situ analysis indicates that a warming climate is unfavorable for primary production in low-latitude open ocean ecosystems.
Introduction
Primary production at the bottom of the marine food web plays a key role in the ocean ecosystem (Pauly and Christensen, 1995) and represents a major pathway for sequestration and/or cycling of atmospheric CO2 by the oceans. However, this process is highly susceptible to environmental and climatic changes (Buitenhuis et al., 2013; Hung et al., 2013, 2016; Liu et al., 2021; Zhong et al., 2021). It is important for oceanographers to gain a comprehensive understanding of the spatiotemporal characteristics of primary production (Field et al., 1998; Campbell et al., 2002; Tang et al., 2008; Tilstone et al., 2015).
The quantification of oceanic primary production is generally based on in situ measurements pertaining to incubation wherein the euphotic zone integrated primary production (PP) is calculated via trapezoidal integration (i.e., the PP inventory is evaluated by integrating PP divided into small trapezoids from the surface to the bottom of the euphotic zone). Measurements of PP by the radiolabeled carbon (C-14) uptake method has been extensively used in marine environments since the introduction of this method to determine the carbon uptake rate of phytoplankton (Nielsen, 1952; Hama et al., 1983; Gong, 1993; Shiah et al., 2000). Despite considerable research in the western North Pacific (WNP), i.e., East China Sea, South China Sea (SCS), and Taiwan Strait, to establish phytoplankton carbon fixation rates, researchers continue to debate whether phytoplankton growth conditions at the surface are indicative of conditions in deeper waters. Obtaining measurements of temperature at depth is straightforward; however, the in situ observation of PP (PPin situ) requires considerable effort in terms of manpower and time. Specifically, seawater samples collected at discrete depths must be incubated on deck within an incubator using running surface water for cooling (Shiah et al., 2000, 2003, 2005; Chen, 2005; Lai et al., 2014; Chen et al., 2016). Note also that gaps in PPin situ coverage inevitably lead to discrepancies in corresponding estimates. The first empirical algorithm for PP predictions based on remote sensing was proposed by Balch et al. (1989). Behrenfeld and Falkowski (1997) developed an attractive alternative approach to estimating global PP using a small number of inputs. Their vertically generalized production model (VGPM; PPVGPM) is widely regarded as the most highly optimized yet usable methods for PP estimation (Kameda and Ishizaka, 2005; Yamada et al., 2005; Ishizaka et al., 2007; Hill and Zimmerman, 2010).
The semi-analytical VGPM uses satellite-based data as an input to calculate PP. Thus, it is conceivable that data measured in situ could be used as an alternative to satellite-based input data, and vice versa (Behrenfeld and Falkowski, 1997; Hill and Zimmerman, 2010). The VGPM can be used to derive PP using satellite-based observations, such as remote passive ocean color, sea surface temperature (SST), surface optimal carbon fixation rate () per unit chlorophyll a (Chl) of the euphotic zone (Zeu), surface Chl concentration (Chls), surface light intensity (E0), and the surface light diffuse attenuation coefficient (Kd) (Yamada et al., 2005; Ishizaka et al., 2007). Nonetheless, it is still problematic whether the assumption based on peak values of PP and Chls occur at the surface and decrease exponentially with depth until reaching the bottom of the Zeu is true (Hill and Zimmerman, 2010; Buitenhuis et al., 2013). Furthermore, the reliability of VGPM in evaluating PP diminishes when geographic features, vertical hydrographic distributions, regional characteristics, extreme weather events, and climate change are taken into consideration (Dierssen, 2010; Friedland et al., 2012; Hung et al., 2013, 2016; Chen et al., 2015; Shih et al., 2015, 2020b). In the absence of a robust estimation method, the results derived from PPVGPM cannot be relied upon to reflect the actual situation throughout the oceans. Empirical models developed by Dunne et al. (2005); Laws et al. (2011), and Henson et al. (2011) are widely used by oceanographers to estimate particulate organic carbon (POC) export flux; however, reliance on PPVGPM also calls into question all corresponding estimates pertaining to global carbon export flux and oceanic carbon sequestration.
The South East Asian Time-series Study (SEATS) conducted in the South China Sea (SCS) was the lowest latitude time-series program implemented during the Joint Global Ocean Flux Study era (Karl et al., 2003; Wong et al., 2007). Numerous studies have characterized the upper ocean of the SCS as stratified and oligotrophic (Chen et al., 2004; Chen, 2005; Wong et al., 2007). Thus, biological activity and regulation of biogeochemical responses depend heavily on dynamic perturbations, including the yearly monsoon, typhoons, storms, internal waves, Kuroshio intrusion, and atmospheric deposition as well as nutrient supply in the form of phytoplankton nitrogen fixation (Liu et al., 2002; Chou et al., 2006; Chen et al., 2008, 2020; Du et al., 2013; Yang et al., 2014; Li et al., 2018; Shih et al., 2020a).
Throughout the SCS basin, the annual modeled PP ranges from 280 to 343 and PPVGPM ranges from 308 to 354 mg-C m –2 d–1 (Table 1; Liu et al., 2002; Tan and Shi, 2009; Ma et al., 2014). High PP values are generally associated with the strong NE-monsoon (NEM) system during the cold season, whereas low PP values are associated with a relative weak SW-monsoon (SWM) during the warm season (Table 1). Based on long-term satellite-based SST records, Chen et al. (2020) reported that declining PP can be attributed at least in part to rising SST (0.012°C y–1). Their and several selected researches in our study went a long way toward establishing a connection between monsoons and PP; however, all of the suppositions are based on indirect measurements; i.e., remote sensing (Liu et al., 2002; Hao et al., 2007; Zhao et al., 2008; Tan and Shi, 2009; Pan et al., 2012; Ma et al., 2014; Chen et al., 2020), rather than direct in situ incubation, such as the C13 and C14 methods (Liu et al., 2002; Chen et al., 2004, 2007; Ning et al., 2004). Note also that even PP research based on in situ oceanographic analysis is limited to short-term observations rather than long-term time-series (Chen, 2005; Chen et al., 1998, 2006; Liu et al., 2002; Tseng et al., 2005).
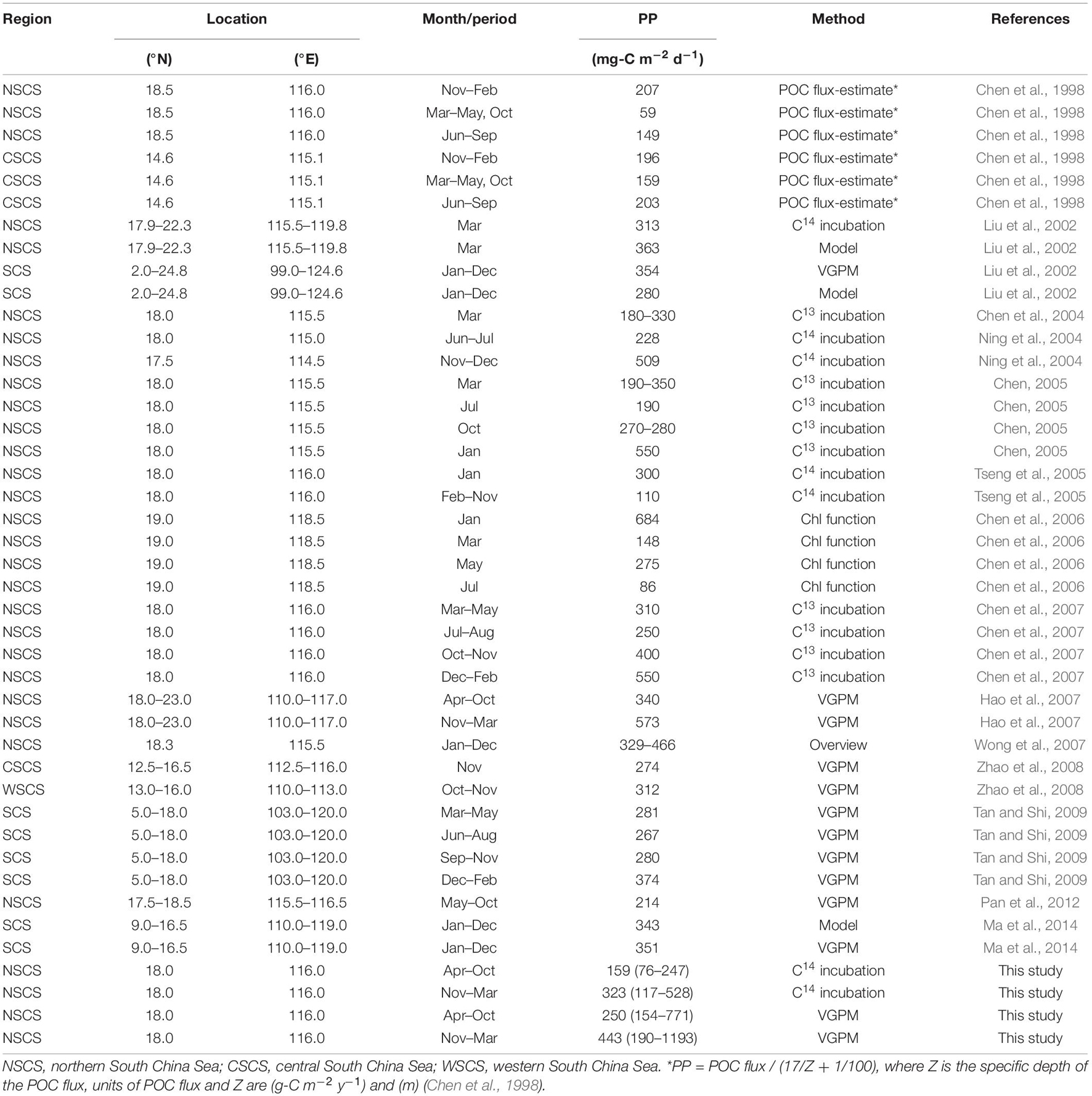
Table 1. Integrated primary production in the euphotic zone (PP) based on selected studies conducted in the South China Sea.
Remote sensing has made it possible to conduct large-scale PP monitoring over extended durations; however, most existing sensing technology is limited to the upper ocean and there is little evidence supporting the assumption of an exponential decrease in PP as a function of depth (Buitenhuis et al., 2013; Shih et al., 2020b). In the current study, we employed data obtained in the SEATS time-series study to elucidate long-term variations in PPin situ under the prevailing monsoon system. We also looked for discrepancies between satellite-based and in situ observations, which could potentially influence PP estimates derived using the VGPM algorithm.
Materials and Methods
In situ Observation
A total of 44 in situ observations were conducted at the SEATS site (18 °N, 116 °E) at regular intervals between 1998 and 2016. These expeditions involved hydrographic seawater sampling and biogeochemical field experiments using research vessels (RV; Ocean Researcher I, Ocean Researcher III, and Fishery Researcher I) (Karl et al., 2003; Wong et al., 2007). On-deck incubation of PP in accordance with 14C protocols was also conducted during 20 of 25 expeditions between 2003 and 2016 (Figure 1). Conductivity–temperature–depth (CTD) (SBE9/11, SeaBird) and quantum scalar irradiance (QSP-200L, Biospherical) were, respectively, used to obtain vertical profiles of temperature and underwater photosynthetically available radiation (PAR). A Biospherical instrument (QSR-240) was used to obtain daily PAR measurements (i.e., daily surface light intensity). In that study, Zeu was defined as the depth at which underwater PAR reached 1%. The mixed layer depth (MLD) was defined as the deepest depth at which the temperature was 0.8°C lower than at the surface (Kara et al., 2000; Chou et al., 2006).
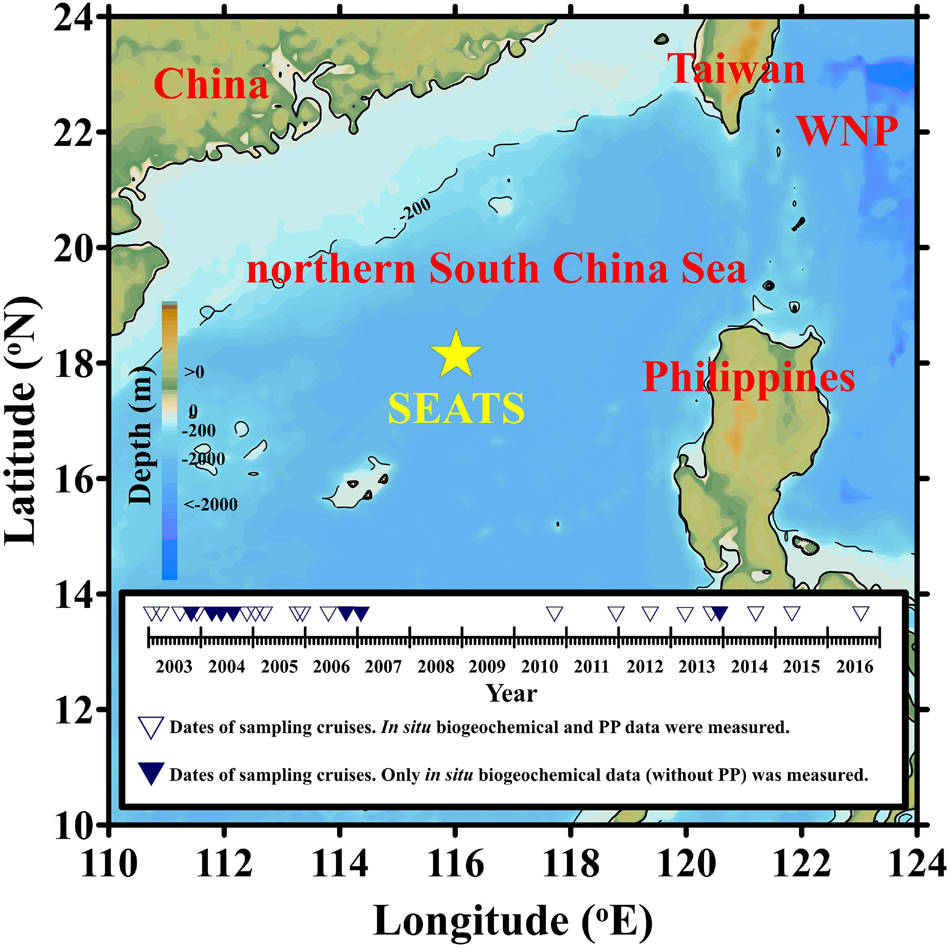
Figure 1. Location of the South East Asian Time-series Study (SEATS) site. Inner box represents the date of cruises conducted at the SEATS site. Major and minor ticks, respectively, exhibit intervals of years and months. WNP refers to the western North Pacific.
Seawater samples were collected at discrete depths to determine Chl concentrations and PP values. Briefly, 2L seawater samples were filtered using 47-mm GF/F filters before obtaining Chl concentrations (via acidification) using a Turner 10-AU-005 fluorometer (Gong et al., 2003; Shiah et al., 2003). PP was measured using the 14C assimilation method (Parsons et al., 1984) following incubation under an artificial light source (2,000 μE m–2 s–1 with full spectrum from 350 to 2450 nm, similar to sunlight) for ∼3 h in a proprietary isolation container using flowing surface seawater for cooling. Following incubation and acidification (0.5 N HCl), radioactive substances collected in 25-mm GF/F filters were transported to a lab for quantification using a scintillation counter (Packard 2200) (Gong et al., 2003; Shiah et al., 2003; Lai et al., 2014). Finally, PP was calculated via trapezoidal integration (i.e. integrated from surface to 1% PAR). Note that SST, Chl and PP measurements from the Ocean Data Bank (Ministry of Science and Technology, Taiwan1) were used to compensate for deficiencies in in situ observations. Some of the hydrographic records (i.e., SST) and biogeochemical parameters (i.e., PP, Chls) of May–October from 2003 to 2006 have been present by Pan et al. (2012).
Remote Sensing Observation
Between 2003 and 2016, the daily data of level-3 products were obtained using a passive ocean color MODerate resolution Imaging Spectroradiometer (Aqua sensor; MODIS-Aqua) with spatial resolution of 4 km for the region covering 17.5–18.5°N and 115.5–116.5°E. We also collected PPVGPM, SST, E0, Kd, and Chls values from the Environmental Research Division’s Data Access Program (ERDDAP), National Oceanic and Atmospheric Administration, Department of Commerce, U. S.2. Note that 8-day composite data were applied in situations where daily observations were missing. Note also that PPVGPM values obtained from ERDDAP were estimated using VGPM developed by Behrenfeld and Falkowski (1997).
Plausible deviations between PPVGPM and PPin situ were examined by comparing data obtained from satellites vs. data obtained in situ, including i.e., SSTsate, ()sate, E0–sate, [E0/(E0+4.1)]sate, Kd–sate, Zeu–sate, Chls–sate; SSTin situ, ()in situ, E0–in situ, [E0/(E0+4.1)]in situ, Kd–in situ, Zeu–in situ, Chls–in situ. Note that this analysis was conducted using the VGPM algorithm proposed by Behrenfeld and Falkowski (1997) (with far fewer input variables), as Equation 2–1:
where the Dirr refers to the photoperiod. Zeu was estimated from the average Kd of the water column (Behrenfeld and Falkowski, 1997 and references therein).
was computed using the empirical equation proposed by Behrenfeld and Falkowski (1997), as Equation 2–2, where T refers to the incubation temperature measured in the deck incubator as SSTin situ or SSTsate. In cases where SST exceeded 28.5°C, a constant value of 4.0 mg-C mg-Chl–1 h–1 was taken as (Behrenfeld and Falkowski, 1997; Hu et al., 2014).
To evaluate the impact of monsoonal force on time-series variations, all data sets were divided into two groups: SW-monsoon (SWM, Apr. to Oct., including inter-monsoons of Apr and Oct) and NE-monsoon (NEM, Nov. to Mar). Variations between satellite-based observations and in situ measurements were examined by averaging all relevant values and reporting them as mean ± standard deviation (SD). Linear regression was used to assess relationships between any two of the variables. The t-tests were used to compare sets of observations with the significance level set at p = 0.10.
Results
Time-Series Distributions: PP, SST, E0, Kd, and Chls
As shown in Figure 2A, PPin situ was ∼2.0 times higher during the NEM (117–528, average = 323 ± 134 mg-C m–2 d–) than during the SWM (76–247, average = 159 ± 58 mg-C m–2 d–1) (Table 2). PPVGPM values between 2003 and 2016 were as follows: SWM was 250 ± 36; 154–771 mg-C m–2 d–1 and NEM was 443 ± 142; 190–1,153 mg-C m–2 d–1 (Figure 2A). Note that PPVGPM during the NEM was ∼1.8 times higher than during the SWM (p < 0.01) (Table 2). The differences in PPin situ and PPVGPM between the NEM and SWM are in line with those reported by Ning et al. (2004); Chen (2005), and Hao et al. (2007), all of which were obtained from the same area of the SCS using different methods (Table 1). PPVGPM exhibited monsoonal variations resembling the curve derived from PPin situ; however, the magnitudes were 37% higher during the NEM and 57% higher during the SWM. On average, PPin situ estimates were ∼50% lower than PPVGPM estimates, regardless of monsoon.
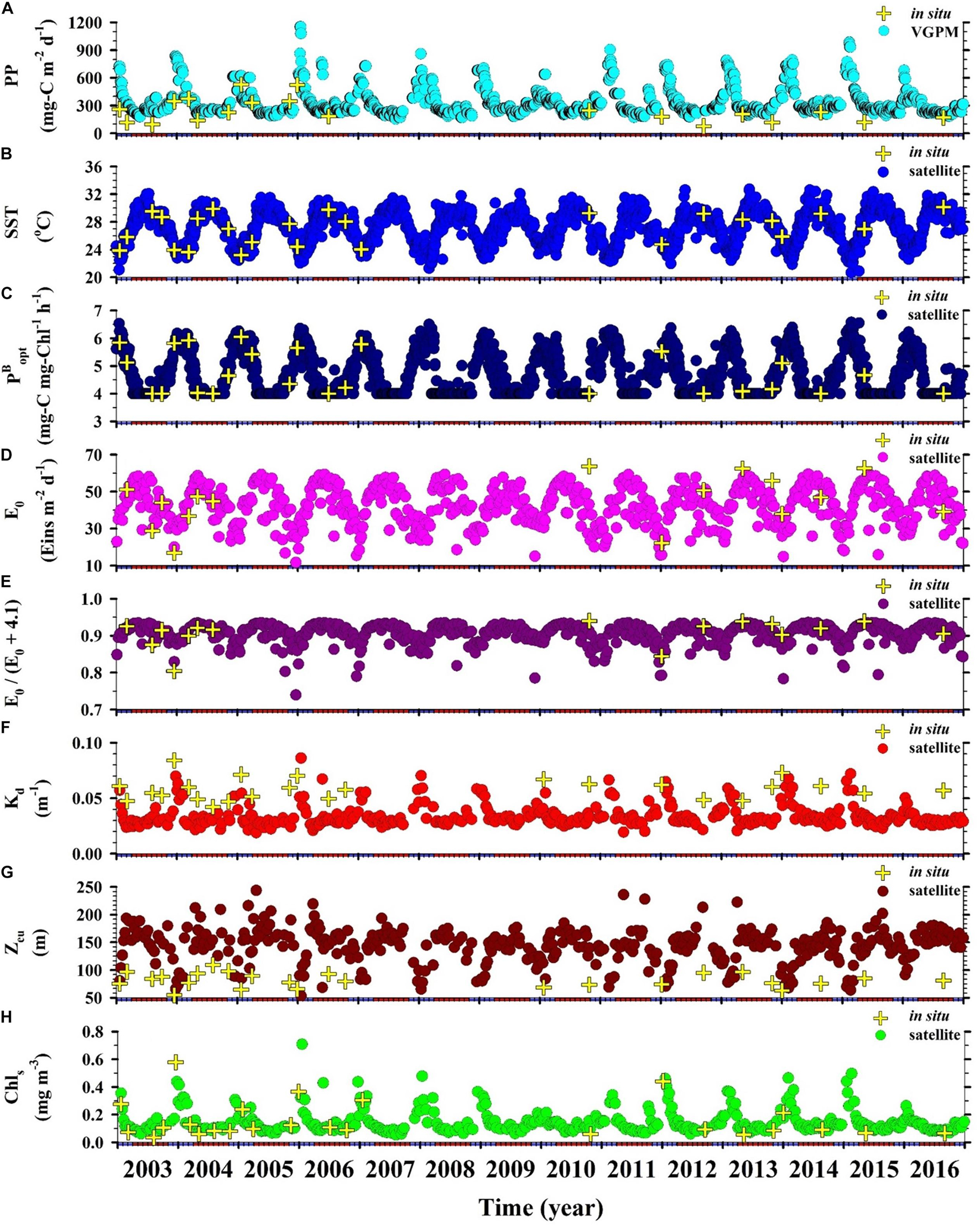
Figure 2. Time-series distributions of satellite-based (circles) and in situ measurements obtained at the SEATS site (crosses): (A) Euphotic zone integrated primary production, PP; (B) sea surface temperature, SST; (C) optimal carbon fixation rate, ; (D) sea surface daily light intensity, E0; (E) E0/(E0+4.1) ratio; (F) light diffuse attenuation coefficient, Kd; (G) depth of euphotic zone, Zeu; (H) sea surface Chl, Chls. Red and blue colors on the horizontal axis, respectively, indicate SWM (Apr–Oct) and NEM (Nov–Mar).
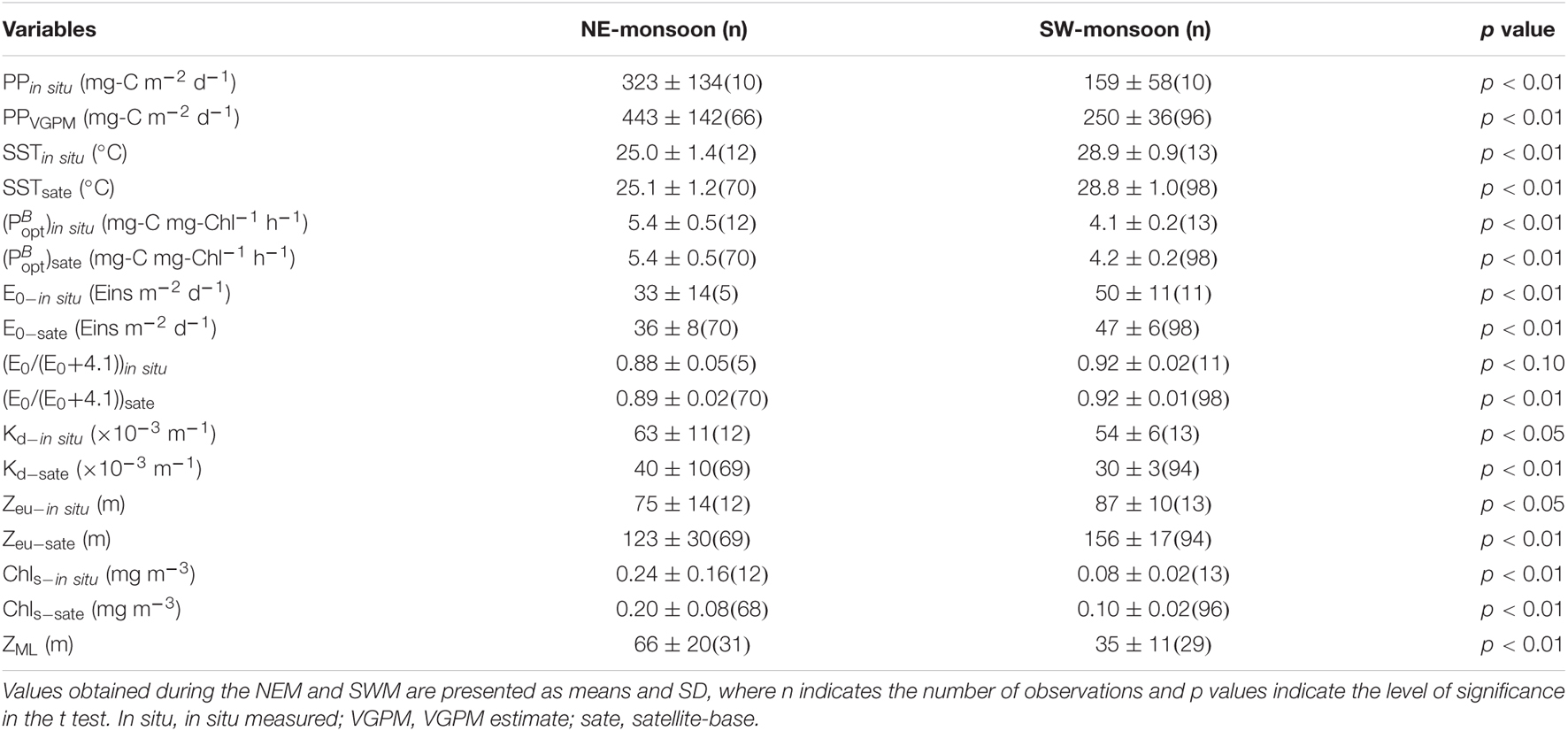
Table 2. Summary of euphotic zone PP (PP) and its relevant variables in the current study conducted at the SEATS: SST, , E0, E0/(E0+4.1), Kd, Zeu, Chls and ZML.
The lowest SSTin situ (25.0 ± 1.4°C) and SSTsate (25.1 ± 1.2°C) values were obtained under the prevailing NEM during the cold season. The highest SSTin situ (28.9 ± 0.9°C) and SSTsate (28.8 ± 1.0°C) values were obtained under the prevailing SWM during the warm season (Table 2). The low SST values recorded during the NEM can be attributed to the combined dynamics of NEM-driven vertical mixing of seawater and reduced solar radiation (Tseng et al., 2005; Zhou et al., 2020). By contrast, the showed a synchronous but opposite distribution (Figures 2B,C). The ()in situ and ()sate values computed from SST for the NEM, respectively, ranged from 4.4 to 6.1 and 4.0 to 6.6 mg-C mg-Chl–1 h–1, and the average value was the same (5.4 ± 0.5 mg-C mg-Chl–1 h–1). The ()in situ and ()sate values for the SWM, respectively, ranged from 4.0 to 4.4 and 4.0 to 6.0 mg-C mg-Chl–1 h–1, with mean values of 4.1 ± 0.2 and 4.2 ± 0.2 mg-C mg-Chl–1 h–1 (Table 2).
E0–in situ and E0–sate values in the SWM (50 ± 11 and 47 ± 6 Eins m–2 d–1) were higher than those in the NEM (33 ± 14 and 36 ± 8 Eins m–2 d–1) (see Figures 2D,E and Table 2). From the perspective of variables in the VGPM algorithm, E0/(E0+4.1) ratios varied little between the in situ measurement and satellite-based observation. The variable of [E0/(E0+4.1)] thereby seemed not an important parameter to influence the PP level. Zeu was estimated using the expression of –ln(0.01)/Kd (Kirk, 1994; Behrenfeld and Falkowski, 1997), which led to a reversal of monsoonal Kd and Zeu distributions (see Figures 2F,G). The mean Zeu–in situ was deeper during the SWM than during the NEM (87 ± 10 and 75 ± 14 m, respectively). The Zeu–sate as well as the Zeu–in situ presented similar trends between SWM and NEM (156 ± 17 and 120 ± 30 m, respectively) (Table 2). Zeu values were shallower in the NEM than in the SWM, due perhaps to reduced light penetration resulting from more abundant biomass in the water column (Chen, 2005; Tseng et al., 2005; Chen et al., 2008).
Figure 2H presents long-term temporal variations in Chls. In situ measurements and satellite-based observations revealed a distinct NEM maximum (0.24 ± 0.16 and 0.20 ± 0.08 mg m–3, respectively) and a SWM minimum (0.08 ± 0.02 and 0.10 ± 0.02 mg m–3, respectively). Chls–in situ and Chls–sate concentrations were, respectively, 0.07 to 0.58 and 0.09 to 0.71 mg m–3 during the NEM, and were, respectively, 0.04 to 0.11 and 0.05 to 0.43 mg m–3 during the SWM. For the same location, our observations were close to the data reported by Chen (2005) and Tseng et al. (2005). The SWM minimum Chls values in the current study were nearly the same as those reported in the oligotrophic ocean time-series studies at HOT (Hawaii Ocean Time-Series) and BATS (Bermuda Atlantic Time-Series Study) during the summer (0.05 mg m–3); however, NEM values at SEATS exceeded the winter maximum at HOT and BATS (0.1 and 0.3 mg m–3, respectively) (Karl et al., 2003). The high NEM maximum at SEATS may perhaps be explained by an increase in phytoplankton biomass (particularly larger sizes of >3 μm), which was stimulated by the deepening ZML (Table 2). The deeper nutrient-rich water was then efficiently transported to the upper surface water under the influence of NEM given that the nutrient-cline depth was shallower in SCS than in other oceans (Chen, 2005; Tseng et al., 2005).
Monthly Variations in PP, SST, E0, Kd and Chls
The PPVGPM values in this study are in good agreement with PPin situ in terms of amplitude as well as phase. Overall, we observed a maximum PPin situ of 394 ± 190 in January (NEM) and a minimum of 143 ± 70 mg-C m–2 d–1 in August (SWM) (Figure 3A). The magnitude of PPVGPM was higher than the values obtained using in situ measurements; however, the trend over a span of 12 months was similar, with a pronounced NEM peak 619 ± 113 mg-C m–2 d–1 in January and the lowest SWM value of 230 ± 28 mg-C m–2 d–1 in September. These results are similar to those reported by other researchers for same area using different methods, such as the particulate organic carbon flux re-calculation (NEM: 207, SWM: 149 mg-C m–2 d–1) (Chen et al., 1998); on-deck C14 incubation (NEM: 300–509, SWM: 110–228 mg-C m–2 d–1) (Ning et al., 2004; Tseng et al., 2005); on-deck C13 incubation (NEM: 190–550, SWM: 190–280 mg-C m–2 d–1) (Chen, 2005; Chen et al., 2007), and Chl empirical function (NEM: 148–684, SWM: 86–275 mg-C m–2 d–1) (Chen et al., 2006; Table 1).
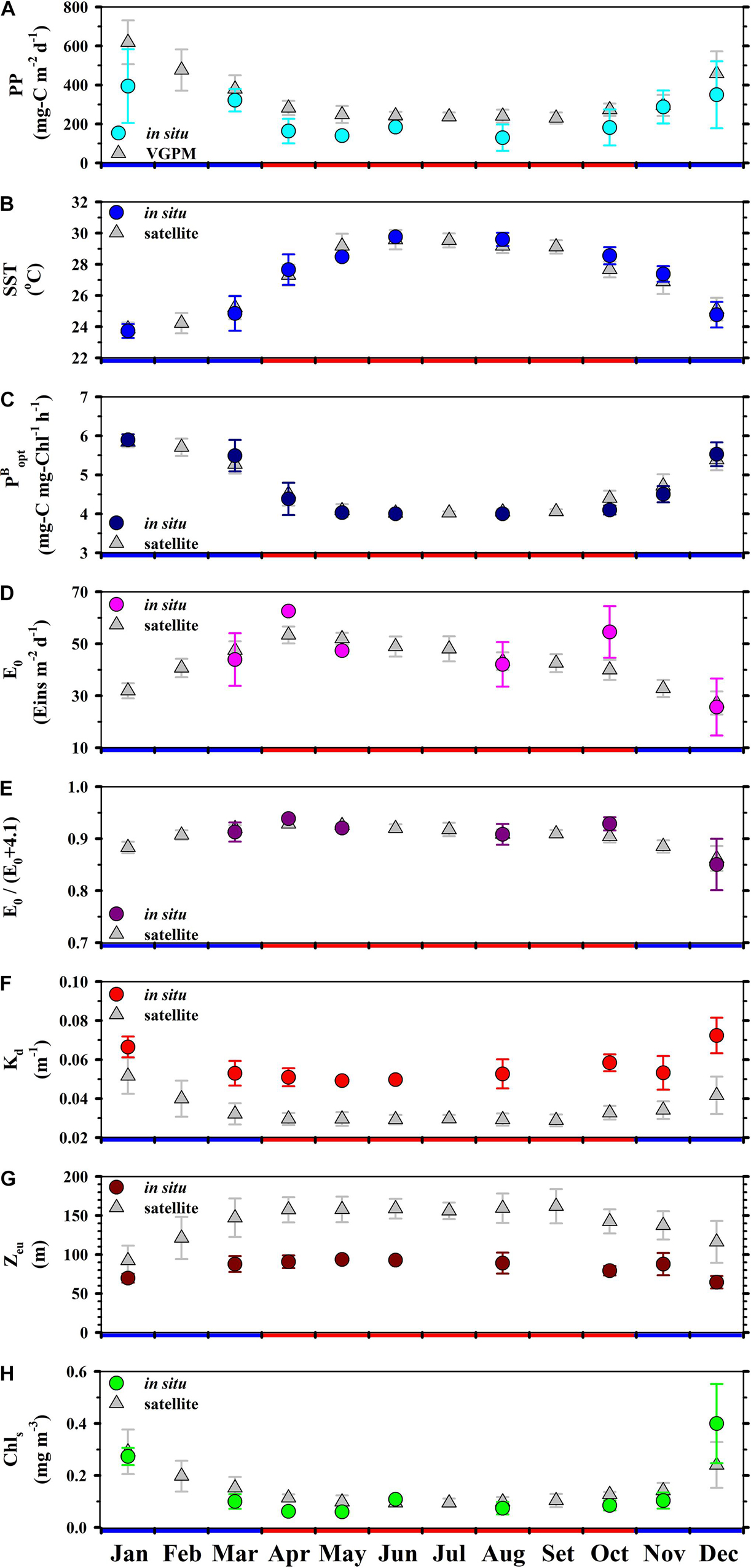
Figure 3. Monthly averages (±1 SD) of (A) PP, (B) SST, (C) (D) E0, (E) E0/(E0+4.1), (F) Kd, (G) Zeu, (H) Chls at the SEATS site. Circles and triangles, respectively, represent in situ measurements and satellite-based observations. Red and blue colors on the horizontal axis, respectively, indicate SWM (Apr–Oct) and NEM (Nov–Mar).
We observed opposing trends between monthly SST and the corresponding . The highest ()in situ values (5.9 mg-C mg-Chl–1 h–1) were observed in January and the lowest (4.0 mg-C mg-Chl–1 h–1) were observed in August. The ()sate values converted from SSTsate (5.8 and 4.0 mg-C mg-Chl–1 h–1 in January and June, respectively) were nearly identical to those for the same time slots obtained via in situ measurements (Figures 3B,C). The highest mean monthly E0–in situ in April (63 ± 0 Eins m–2 d–1) was higher than the highest mean monthly E0–sate (53 ± 3 Eins m–2 d–1). Overall, E0–in situ and E0–sate levels in December were very similar (∼26 and ∼27 Eins m–2 d–1, respectively). Based on E0–in situ, the highest E0/(E0+4.1)in situ ratio (0.94) occurred in April and the lowest E0/(E0+4.1)in situ ratio (0.85) occurred in December. E0/(E0+4.1)sate values of 0.93 in April and 0.86 in December were similar with the E0/(E0+4.1)in situ values (Figures 3D,E). As for parameterization, it appears that and E0/(E0+4.1) values had little influence on VGPM results (PP), regardless of whether the values were obtained from in situ measurements or satellite-based observation.
We did not observe large monsoonal variations in Kd–in situ and Kd–sate; however, the highest Kd–in situ (0.072 ± 0.009 m–1) was obtained in December and the highest Kd–sate (0.052 ± 0.009 m–1) was obtained in January. These high Kd values resulted in a shallower Zeu–in situ (64 ± 8 m) and Zeu–sate (92 ± 19 m), compared to the values converted from the Kd–in situ (0.053 ± 0.007 m–1) for August (Zeu–in situ = 89 ± 13 m) and the Kd–sate (0.029 ± 0.003 m–1) for September (Zeu–sate = 162 ± 22 m) (Figures 3F,G). The shallow Zeu observed in December and January can be attributed mainly to an increase in phytoplankton biomass that reduces the light penetration in that region during the NEM (Chen, 2005; Tseng et al., 2005; Chen et al., 2008).
Figure 3H presents temporal variations in Chls–in situ and Chls–sate. Conspicuously high Chls–in situ values were observed in December (0.40 ± 0.15 mg m–3) and high Chls–sate values were observed in January (0.29 ± 0.09 mg m–3). The high Chls values observed throughout the NEM were triggered by the monsoonal force, which stimulated phytoplankton photosynthesis, thereby increasing the phytoplankton abundance or enriching the area with Chls from subsurface waters (Liu et al., 2002; Tseng et al., 2005). The drop in Chls–in situ and Chls–sate values to nearly < 0.10 mg m–3 during the SWM has previously been reported in studies focusing on the same region of the SCS (Liu et al., 2002; Chen, 2005; Tseng et al., 2005; Shih et al., 2020a). Similar findings were also recorded in oligotrophic time-series studies, such as HOT and BATS (Karl et al., 2003).
Relationships Among of PP, SST, E0, Kd and Chls in in situ Measurements and Satellite-Based Observations
PPin situ was generally lower than PPVGPM; however, we observed a significantly positive correlation between these two parameters; i.e., slope = 0.70, r2 = 0.42, p < 0.01 (Figure 4A). This clearly indicates the feasibility of the VGPM for predictions; however, tuning would be required for the study area. As shown in Figure 4, we identified significantly positive correlations between PPin situ and PPVGPM as well as their respective variables SST, E0, Kd and Chls. As indicated by high r2 and low p values with slopes close to 1, the most significant correlations were found in SST and E0 (Figures 4C–F) : SST (slope = 0.92, r2 = 0.92, p < 0.01) and E0 (slope = 0.62, r2 = 0.58, p < 0.01). Taken together, these results indicate that SSTsate and E0–sate were the variables most strongly correlated with SSTin situ and E0–in situ, providing the most accurate estimates of PP when using the VGPM. As indicated by the 1:1 lines in Figures 4B,G,H, the parameters with the greatest variability in terms of slope were Chls (slope = 0.41, r2 = 0.74, p < 0.01) and Kd (slope = 0.59, r2 = 0.50, p < 0.01). This suggests Chls–sate and Kd–sate could potentially bias PP estimates obtained using the VGPM.
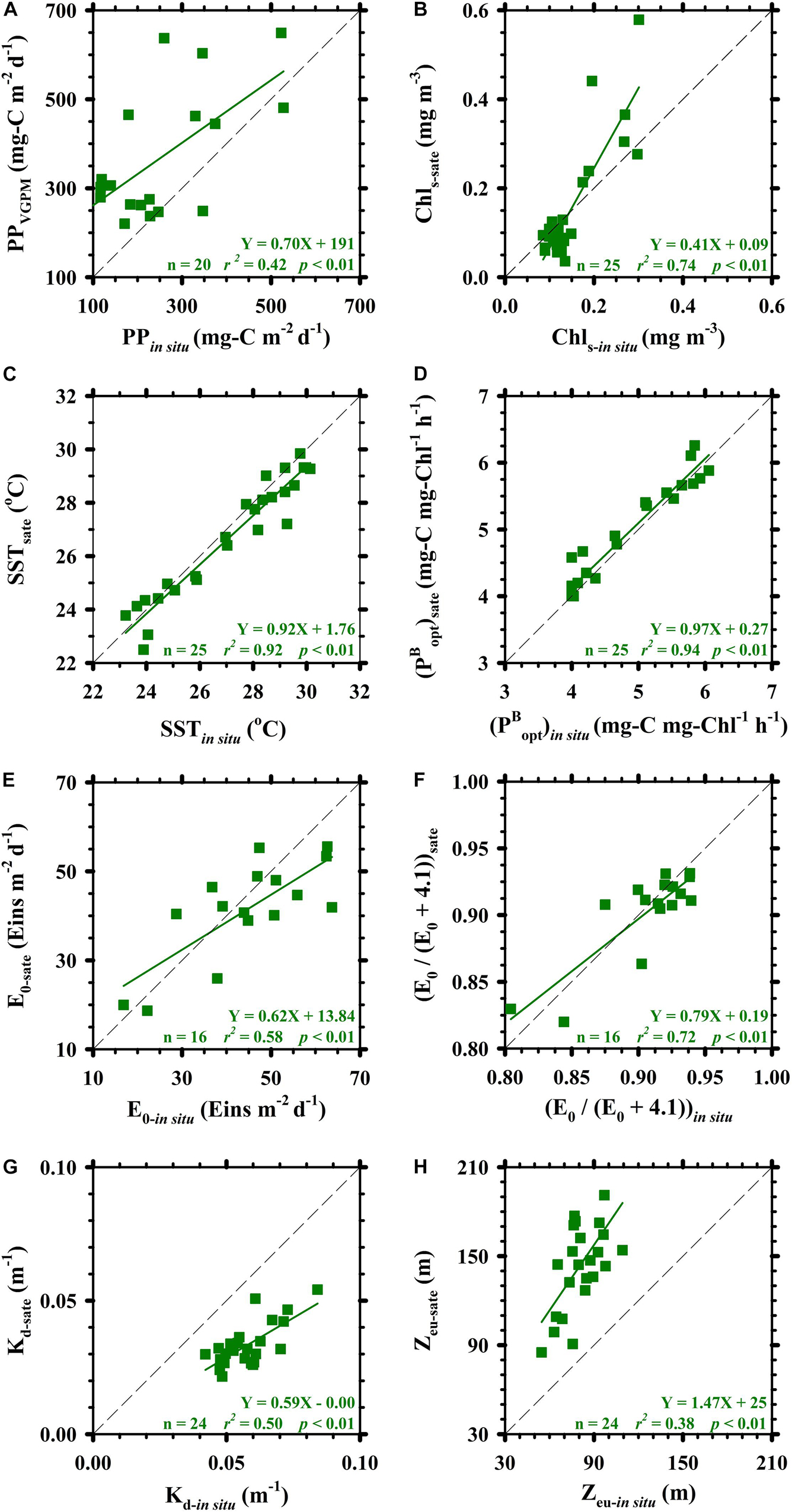
Figure 4. Linear relationships among satellite-based observations and in situ measurements. Panels (A–H) are PP, Chls, SST, (estimated from SST), E0, E0/(E0+4.1) ratio, Kd, and Zeu (estimated from Kd), respectively. Diagonals in panels (A–H) are 1:1 lines.
The fact that the regression line between PPVGPM and PPin situ lies above the 1:1 line indicates that PPVGPM estimates exceeded PPin situ. When using the VGPM to estimate PP, the two main variables are and E0/(E0+4.1), derived from in situ measurements and satellite-based observations of SST (SSTin situ and SSTsate) and E0 (E0–in situ and E0–sate). Between SSTin situ and SSTsate as well as between E0–in situ and E0–sate, we observed nearly linear relationships (i.e., close to the 1:1 diagonal). This suggests that and E0/(E0+4.1) were not scaling variables governing the magnitude of PP in this study. Influences derived from these two variables [, E0/(E0+4.1)] of the both methods on calculated results were less than 1%. By contrast, the correlations between Chls–in situ and Chls–sate, and Zeu–in situ and Zeu–sate (converted from Kd–in situ and Kd–sate, respectively) had a pronounced impact on VGPM PP estimates. If we considered only the difference between Chls–in situ and Chls–sate, then PP values estimated using VGPM would be slightly higher (∼ 5%, depended on the given case) than those obtained using in situ measurements. If we considered only the difference between Zeu–in situ and Zeu–sate, then PP values estimated using VGPM would be apparently 1.72-fold higher than those obtained using in situ measurements.
Discussion
Uncertainty in Estimating PP Due to Differences Between Chls–in situ and Chls–sate as Well as Zeu–in situ and Zeu–sate: Implications
Our analysis of revealed a number of potential uncertainties pertaining to PP estimation using the VGPM algorithm. Most of the discrepancies were due primarily to differences between Zeu–in situ and Zeu–sate as well as between Chls–in situ and Chls–sate. Overall, the product of Zeu and Chls (i.e., the phytoplankton inventory in the euphotic zone), suggests that the base assumption of vertically distributed standing stock biomass in low latitude waters (SCS) may perhaps be erroneous. If so, then it will be necessary to reformulate methods for the prediction of biomass standing stocks when implementing the VGPM algorithm (Ning et al., 2004; Hill and Zimmerman, 2010).
The fundamental structure of the VGPM is based on a relationship between integrated phytoplankton biomass in the euphotic zone and Chls (Behrenfeld and Falkowski, 1997; Hill and Zimmerman, 2010). Thus, obtaining accurate estimates of PP by comparing PPin situ and PPVGPM results depends on reliable estimates of the integrated phytoplankton biomass in the euphotic zone. However, passive satellites recording the color of the ocean surface are unable to elucidate the situation at arbitrary depths below the surface (Hill and Zimmerman, 2010; Shih et al., 2020b). Contrary to the assumption that Chl decreases exponentially with depth, most observations in the SCS revealed that the subsurface Chl maximum (SCM) was often found at great depths (Liu et al., 2002; Chen, 2005; Shih et al., 2020a, b). This makes it very difficult or even impossible to estimate the integrated biomass in the euphotic zone simply as a product of Zeu and Chls. Enhancing the reliability of the VGPM requires that we increase the number of PPin situ observations and the corresponding variables in order to improve the correlation between our assumptions pertaining to phytoplankton integrated biomass and actual measurements obtained in the field. This is particularly important in phytoplankton populations, dynamics and assemblages in specific locations under specific conditions (Hill and Zimmerman, 2010; Shih et al., 2015, 2020b). Only by increasing the number of observations and enhancing our analysis of water composition will it be possible to reduce the uncertainty associated with Zeu and Chls in estimating PP using the VGPM.
The mean PP in the euphotic zone (PP/Zeu, mg-C m–3 d–1) presented a positive linear relationship between (PPVGPM/Zeu–sate) and (PPin situ/Zeu–in situ); i.e., slope: 0.51, r2 = 0.39, p < 0.01 (Figure 5A). Higher values were observed during the NEM (4.7 ± 2.4 and 3.8 ± 2.2 mg-C m–3 d–1 of PPin situ/Zeu–in situ and PPVGPM/Zeu–sate, respectively) and lower values were observed during the SWM (1.9 ± 0.8 and 1.7 ± 0.4 mg-C m–3 d–1 of PPin situ/Zeu–in situ and PPVGPM/Zeu–sate, respectively). The slope of 0.51 for PPVGPM/Zeu–sate and PPin situ/Zeu–in situ was lower than that of PPVGPM and PPin situ (slope = 0.70), such that most data fell on the right side of the 1:1 line. Ratios of PPVGPM/Zeu–sate were nearly 20% lower than those of PPin situ/Zeu–in situ, indicating that Zeu–sate was deeper than Zeu–in situ, particularly during the SWM. This also indicates that the VGPM parameter Zeu–sate indeed substantially affected PP estimates in this study. It has been proposed that satellite-based Kd values have to be calibrated against the zenith solar angle during the data processing in accordance with the methods outlined by Lee et al. (2005) and Li et al. (2015). However, users may not to confirm the processing from the downloaded or retrieved satellite-based products of PP and its relevant variables.
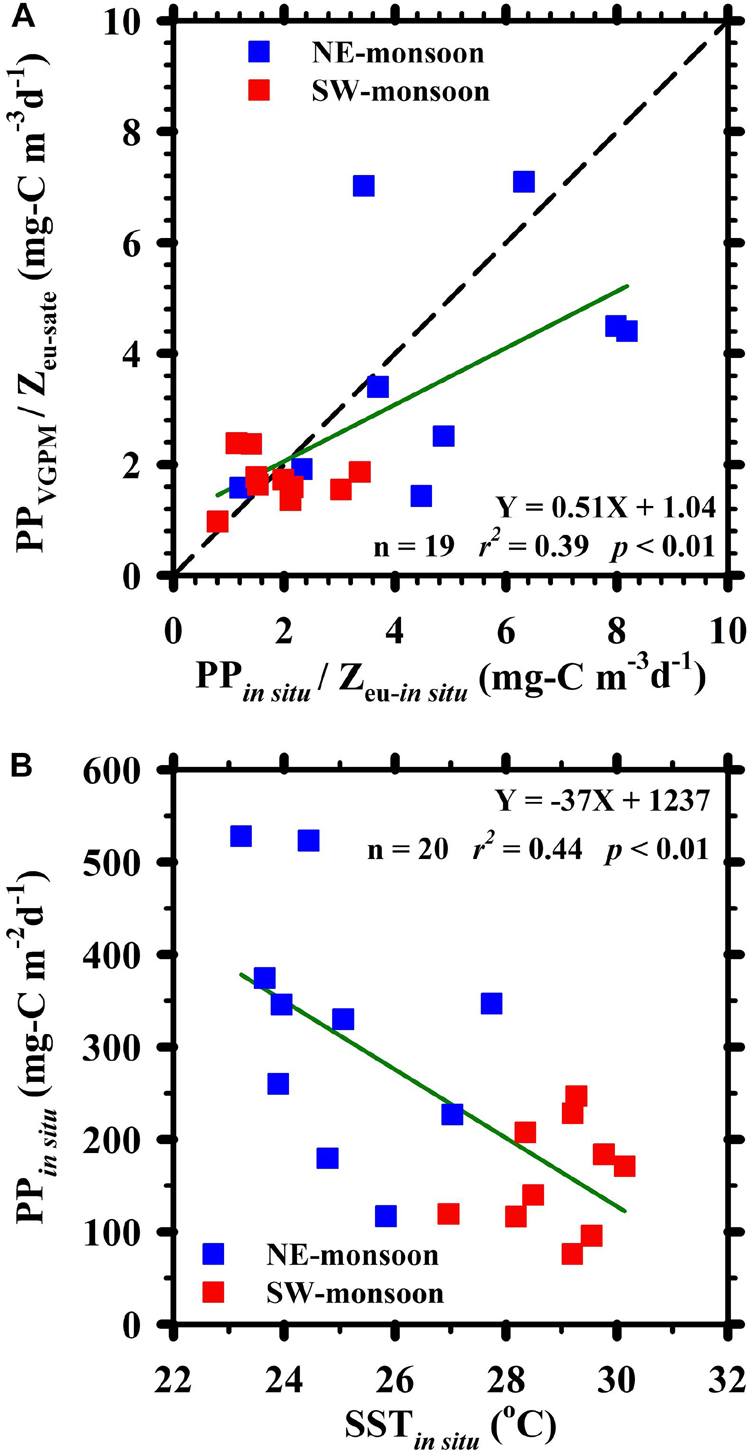
Figure 5. (A) Relationship between satellite-based observations of (PPVGPM / Zeu–sate) and in situ measurements of (PPin situ / Zeu–in situ) ratios; (B) relationship between PPin situ and SSTin situ. Red and blue symbols, respectively, indicate observations made during the SW-monsoon and NE-monsoon. The diagonal in panel (A) is 1:1 line.
The significant linear correlation between ()sate and ()in situ (slope = 0.97, r2 = 0.94, p < 0.01; Figure 4D) is simply a reflection of the relationship between the two SST records, resulting from the fact that is computed as a function of SST (i.e., a seventh-order polynomial, Equation 2–2) (Behrenfeld and Falkowski, 1997). Thus, any potential deviations between SST and had only a negligible influence on PP estimates obtained using VGPM. Many studies have nevertheless concluded that the accuracy of VGPM-based estimates of PP are poor, when using the 7th order polynomial of SST (Equation 2–2) to calculate the input of (Mizobata and Saitoh, 2004; Kameda and Ishizaka, 2005; Yamada et al., 2005; Siswanto et al., 2006; Ishizaka et al., 2007; Tang et al., 2008; Tang and Chen, 2016).
Researchers have reported that much of the uncertainty in estimating PPVGPM is related to the computation of under the effects of phytoplankton physiology, growth conditions, abundance, size, and productivity (Gong and Liu, 2003; Kameda and Ishizaka, 2005; Yamada et al., 2005). It has been suggested that is influenced by SST as well as E0 and various biological parameters, such as Chl concentration. represents an optimal daily carbon fixation rate in the water column previously described as a 7th order polynomial of SST, however, when SST exceeds 28.5°C, remains fixed at a constant 4 mg-C mg-Chl–1 h–1 (Behrenfeld and Falkowski, 1997; Mizobata and Saitoh, 2004), indicating that tends to be underestimated when SST exceeds 28.5°C. In low-latitude regions of the SCS, the constant mentioned above usually occurred in late spring, summer, and early fall, during which SSTin situ and SSTsate both exceeded 28.5°C. This increased the margin between actual PP values and the estimates obtained by inputting derived using SSTin situ or SSTsate. The reliability of estimates obtained using the VGPM seventh-order polynomial SST algorithm is not universally applicable (temporally or spatially). This has prompted oceanographers to tune existing methods or devise new methods for the precise estimation of , especially for ocean water at low latitudes.
Our results reveal that the satellite-derived primary production (e.g., PPVGPM) may significantly affect global carbon export flux to deep waters, but what is the overall significance and impact of these PP values on POC fluxes? For example, Dunne et al. (2005) used the empirical model expression (Equation 4–1) to estimate carbon flux (or sequestration) in oceans. PP (mg-C m–2 d–1), Zeu (m) and SST (°C) are three major factors affecting the estimated values of POC flux which is almost linearly proportional to PP.
According to the expression 4–1, the POC fluxes estimated from in situ measurements (PPin situ, SSTin situ and Zeu–in situ) of Hung et al. (2000); Hung and Gong (2007), and Shih et al. (2015) were from 12 to 319 mg-C m–2 d–1, an average of 20% less than the trap POC fluxes (25–274 mg-C m–2 d–1) (Figure 6). As described above, the inputs of SSTin situ and Zeu–in situ to the expression were fixed, the PPin situ was replaced by the PPVGPM, an average difference between estimated and trap POC fluxes (estimated POC flux: 16–781 mg-C m–2 d–1) was a factor of 2. It has been suggested that the uncertainty of these POC fluxes is quite large if satellite-based PP is used to estimate carbon sequestrations in oceans. If the discrepancy between in situ measurements and satellite-based observations of PP can be diminished and the reliance on them (e.g., PPVGPM) can be increased, it is to dedicate the potential importance and goal of the present study.
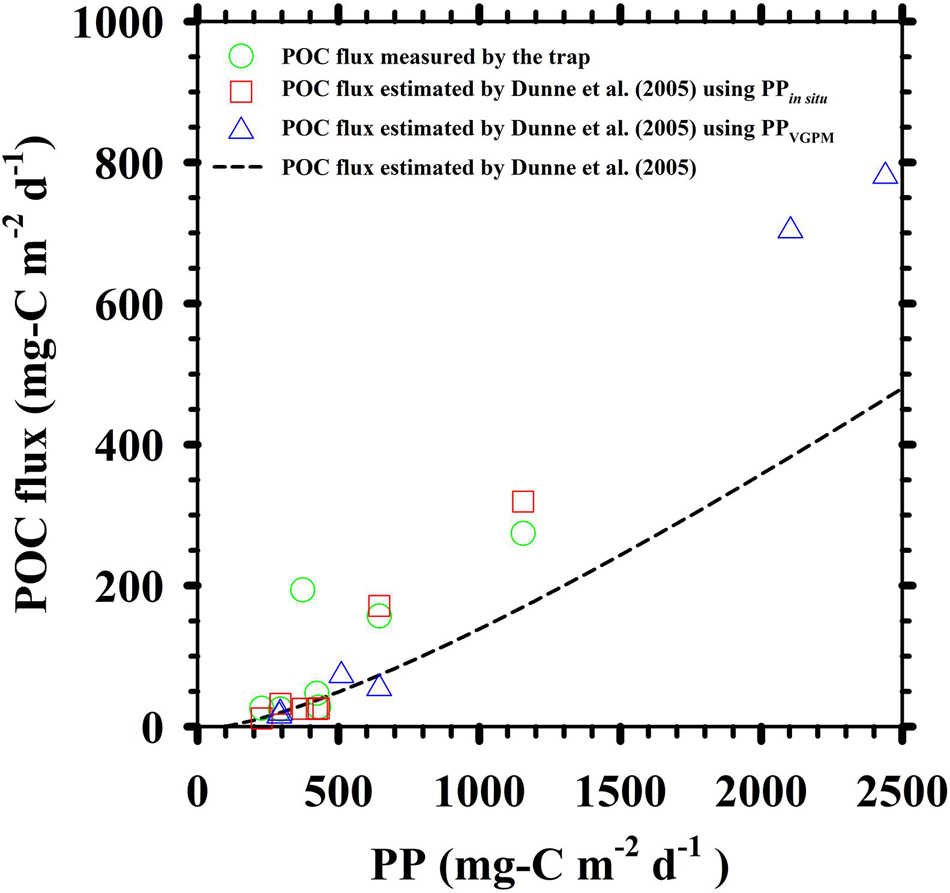
Figure 6. A comparison of the difference between in situ trap measured and estimated POC fluxes. The estimated POC fluxes were computed according to the empirical model expression revealed by Dunne et al. (2005). The dashed line represented the proportional relationship of estimated POC flux and PP. The PPin situ and PPVGPM were also exerted on the expression to estimate POC fluxes (red square and blue triangle, respectively). In situ measurements of PPin situ and trap measured POC fluxes (green circle) were based on Hung et al. (2000); Hung and Gong (2007), and Shih et al. (2015).
Impact of Global Warming on in situ Observations
In both the Atlantic and Pacific oceans, increased phytoplankton biomass is observed at low latitudes during the boreal warm season. Other than equatorial upwelling, there are no other conspicuous seasonal trends in biogeochemical activities (Dandonneau et al., 2004). In the Arabian Sea, enhanced biogeochemical responses are also observed at low latitudes during the SWM (Banse and English, 2000). In this study, long-term and monthly variations in PPin situ and PPVGPM demonstrated influential monsoonal system at the SEATS (Figures 2, 3 and Table 2). The concurrence of low SST, deep ZML, high Chls, shallow Zeu, and increased PP suggest that increased phytoplankton biomass or specific phytoplankton communities dominating were triggered during the NEM. It has been reported that the average nitrate+nitrite (N+N) concentration, one of the key nutrients for phytoplankton growth in the SCS, in the MLD in the seasons of NEM (0.1–0.3 μM) is ∼10 times higher than of SWM (∼0.03 μM) (Tseng et al., 2005). Moreover, the inventory of N+N and the depth of nitracline in the NEM (30 ± 19 mmol m–2 and 28–62 m, respectively) have been observed a ∼ 4.5 fold higher and a ∼ 25–50% shallower than those reported in the SWM (7 ± 4 mmol m–2 and 52–82 m, respectively) (Chen, 2005; Shih et al., 2020a). Evidences of abundant nutrient (e.g., N+N) and shallow nitracline depth favoring biological activities, imply that the growth of phytoplankton communities and associated photosynthesis were affected mainly by vertical advection providing inorganic nutrients from deeper waters, under the NEM system prevailing in the SCS (Liu et al., 2002; Bai et al., 2018; Chen et al., 2020; Zhou et al., 2020).
The global decrease in PP is particularly pronounced in high-latitude waters, which lose roughly 2,000 Mt-C y–1 (Mt = 1012 g), accounting for a 70% decline in carbon fixation via photosynthesis (Gregg et al., 2003). To compare annually reductions in PPin situ (−11 mg-C m–2 d–1 y–1) vs. the annual PPin situ (241 mg-C m–2 d–1; mean PPin situ of NEM and SWM; Table 1), it indicated that the annual reduction in carbon fixation via photosynthesis was ∼ -5% y–1. Based on the 200 m isobath boundary of oligotrophic waters in the SCS (2.76 × 1012 m2; Lin et al., 2003), the e-ratios were 5–16% in the SCS (Hung and Gong, 2010; Shih et al., 2019) and the decreased in carbon fixation was roughly 11 Mt-C, thereby accounting for 30–90% of the export production. This suggests a gradual decrease in the efficiency of photosynthetic carbon fixation by phytoplankton. Nevertheless, satellite-based observations do not show the signs of global warming on carbon fixation and sequestration.
Figure 5B illustrates the significantly negative relationship between PPin situ and SSTin situ at the SEATS site. The slope of −37 mg-C m–2 d–1°C –1 was exceptionally close to the −36 mg-C m–2 d–1°C –1 reported in previous studies (Chen and Chen, 2006; Chen et al., 2007, 2008). Scaling factors related to the increase in SSTin situ caused by global changes are extremely complex (Sarmiento et al., 1998; Hung et al., 2010; Bai et al., 2018). Notwithstanding the complexity of factors governing SSTin situ, they can still be used to estimate PP values. The differences between daytime and nighttime SSTin situ values were statistically insignificant, during the NEM as well as the SWM (one-tail t test: p = 0.15 and 0.24, respectively (Figure 6). We therefore surmise that SST sampling schedules had no effect on the overall results. Furthermore, we observed a strong statistically significant correlation between SSTsate and SSTin situ (Figure 4C), indicating the efficacy of SST in estimating PP distributions over a broad horizontal area, regardless of the method used for derivation.
The straightforward relationship between PPin situ and SSTin situ is important when seeking to predict PP values and estimate new or export production in euphotic zones. Under the environmental conditions described above, the reduction in carbon fixation due to photosynthesis would be ∼−15% °C–1, and the decrease in carbon fixation would be roughly 37 Mt-C, thereby accounting for a 1- to 3-fold quantity of export production. The negative correlation between PPin situ and SSTin situ in the current study matched the findings observed in mid-latitude tropical/subtropical regions of the Pacific and Atlantic oceans (Chen, 2000; Tilstone et al., 2009), but differed drastically from those reported in high-latitude regions (Kudryavtseva et al., 2018).
Generally speaking, the sampling resolution of in situ time-series is too low to eliminate temporal uncertainty over all timespans. Fortunately, we can use SSTsate to compensate for deficiencies in SSTin situ coverage (Bai et al., 2018; Chen et al., 2020). Only one daily SSTsate reading can be obtained at any given location; however, it would be perfectly reasonable to substitute that value with one obtained SSTin situ. We observed a statistically significant linear relationship between SSTsate with SSTin situ (Figure 4C); however, differences between daytime and nighttime SSTin situ measurements did not reach the level of significance (Figure 7). This suggests that SST estimates obtained using either method could be used to assess the influence of temperature on biogeochemical phenomena, such as PP.
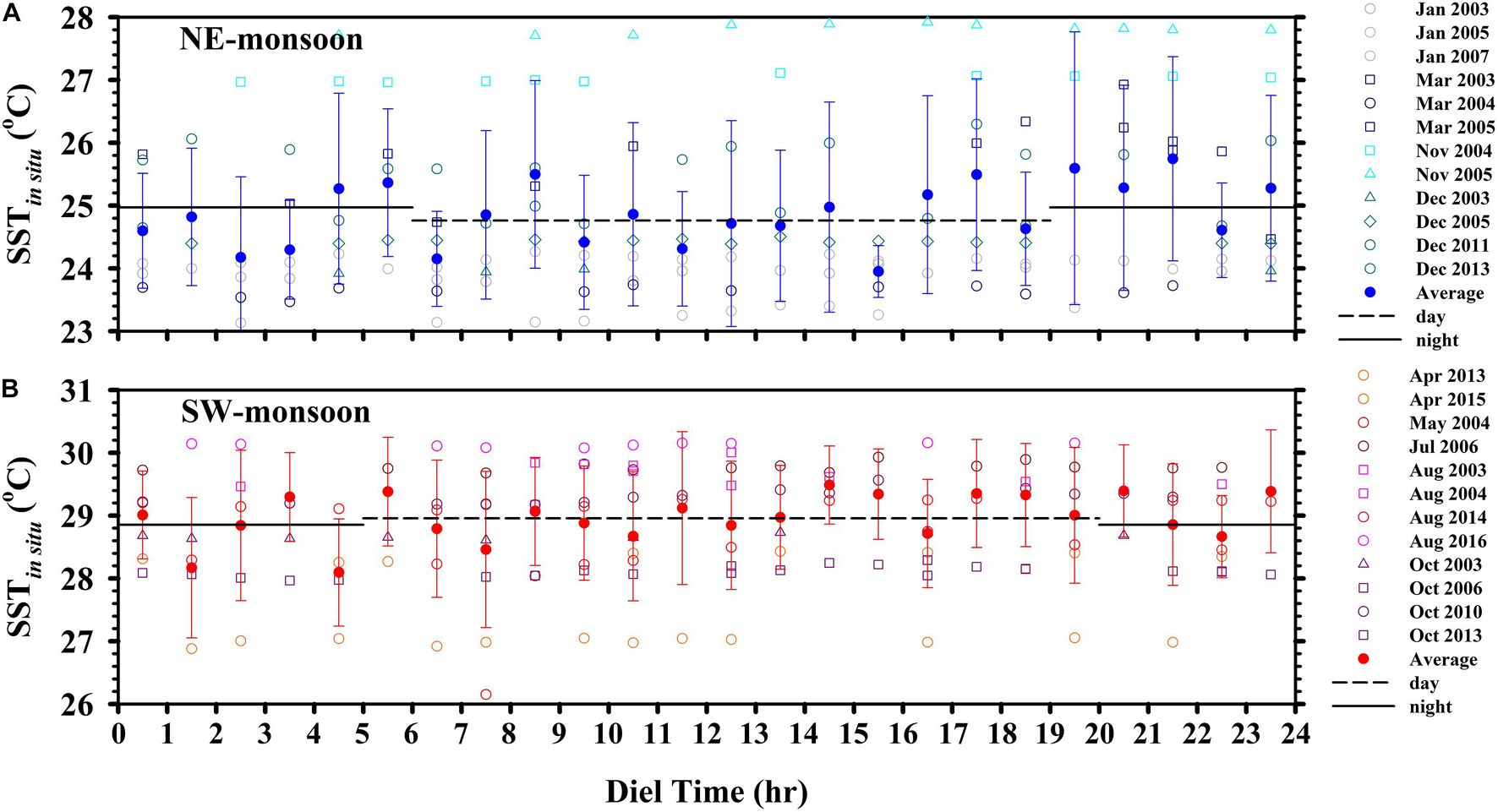
Figure 7. Averaged diel records (±1 SD) of SST during the (A) NE-monsoon and (B) SW-monsoon. Horizontal dashed and solid lines indicate the mean daytime measurements (GMT+8: 06–18 and 05–19 h, NE- and SW-monsoons, respectively) and nighttime measurements (GMT+8: 19–05 and 20–04 h, NE- and SW-monsoons, respectively).
Asynchronous variations between PPin situ and PPVGPM and their related variables in the VGPM algorithm indicate the following: (1) Satellite-based evaluations depend primarily on the assumption of an exponential decrease in the vertical distribution of PP from the surface to the euphotic zone base. Nonetheless, remote sensing cannot penetrate beyond the surface, and therefore cannot reflect the true vertical distribution of PP at depth (Hung et al., 2010; Buitenhuis et al., 2013; Shih et al., 2013, 2020b). This assumption is the primary cause for uncertainty between PP values obtained from satellite-based observations (i.e., PPVGPM) and those derived from in situ measurements (i.e., PPin situ). (2) When using satellite-based observations to estimate horizontal PP distributions, mathematical extrapolation/interpolation is commonly used to compensate for gaps in data coverage resulting from cloud coverage, heavy rains, rough seas, extreme weather events, natural episodes, suspended particles, and/or chromophoric dissolved organic matter. Thus, this approach cannot reflect “true” or “in situ” biogeochemistry responses to PP in the oceans. (Boyd and Trull, 2007; Shang et al., 2008; Tang et al., 2008; Hung et al., 2009, 2010; Shih et al., 2020a). For decades, SEATS has been used as a natural laboratory for studies of prolonged environmental changes and reciprocal biogeochemical responses. It is time to tune existing models or develop more reliable models if we are to gain meaningful estimates of biogeochemical phenomena in the oceans. The proposed calibration method aimed at improving PP estimates for the VGPM is expected to enhance our understanding of changes in the SCS.
Summary
Our time-series study (2003 ∼ 2016) at SEATS compared PP estimates based on in situ measurements and those based on the VGPM in the SCS during the NEM and SWM. PP values obtained during the NEM exceeded those obtained during the SWM, which appears to indicate that weather conditions during the cold season are conducive to high PP values. PPin situ values were roughly 50% lower than PPVGPM values, regardless of the season (NEM or SWM). These discrepancies can be attributed to the satellite-based integrated phytoplankton biomass in the euphotic zone. The discrepancies can be derived as the product of Zeu and Chls, which are two main variables in the VGPM algorithm, especially the impact of difference between in situ and satellite-based Zeu on the magnitude of PP.
The observed overall decrease in PPin situ can be partially explained by an increase in SSTin situ. Our results also showed that SSTsate could be used to predict horizontal PP distributions over extended time scales, based on our observation of a statistically significant relationship between SSTsate with SSTin situ. A significantly negative relationship between PPin situ and SSTin situ appears to indicate that global changes, such as oceanic warming, could have a negative impact on ocean biogeochemistry in low-latitude regions of the SCS. Nonetheless, further research will be required to assess the influence of global changes on biogeochemical phenomena, particularly in low-latitude waters. The SEATS has been used for decades to assess the sensitivity and resilience of low-latitude oceans to environmental fluctuations. Our analysis of discrepancies between in situ measurements and satellite-based observations could help to guide revisions aimed at enhancing the robustness and reliability of the VGPM in estimating biogeochemical responses. Satellite-based data could be used to expand the spatiotemporal scale of observations and thereby shed light on the actual biogeochemical effects of global environmental changes in low-latitude regions of the SCS.
Data Availability Statement
The original contributions presented in the study are included in the article/supplementary material, further inquiries can be directed to the corresponding author.
Author Contributions
Y-YS, F-KS, and C-CH wrote the manuscript with contributions from W-CC. C-YL, J-HT, Y-SW, and C-CL performed the experiments and created the tables and figures. Y-YS, F-KS, C-CH, W-CC, C-YL, J-HT, Y-SW, C-CL and C-YK reviewed and revised the manuscript. All authors listed have made substantial, direct, and intellectual contribution to the work and approved it for publication.
Funding
This research was supported by the MOST (Ministry of Sciences and Technology, Taiwan) under grant numbers 108-2611-M-012-001, 108-2611-M-110-019-MY3, 109-2611-M-012-001, 109-2740-M-110-001, 110-2611-M-012-002, 110-2740-M-110-001, and 110-2611-M-019-005.
Conflict of Interest
The authors declare that the research was conducted in the absence of any commercial or financial relationships that could be construed as a potential conflict of interest.
Publisher’s Note
All claims expressed in this article are solely those of the authors and do not necessarily represent those of their affiliated organizations, or those of the publisher, the editors and the reviewers. Any product that may be evaluated in this article, or claim that may be made by its manufacturer, is not guaranteed or endorsed by the publisher.
Acknowledgments
We would like to thank the assistance given us by the crews of R/V Ocean Researcher I and R/V Fishery Researcher I. We would also like to thank the unsung heroes and contributors who have contributed to the SEATS program.
Footnotes
References
Bai, Y., He, X. Q., Yu, S. J., and Chen, C. T. A. (2018). Changes in the ecological environment of the marginal seas along the Eurasian Continent from 2003 to 2014. Sustainability 10:635. doi: 10.3390/su10030635
Balch, W. M., Abbott, M. R., and Eppley, R. W. (1989). Remote sensing of primary production—I. A comparison of empirical and semi-analytical algorithms. Deep Sea Res. A 36, 281–295. doi: 10.1016/0198-0149(89)90139-8
Banse, K., and English, D. C. (2000). Geographical differences in seasonality of CZCS-derived phytoplankton pigment in the Arabian Sea for 1978–1986. Deep Sea Res. II 47, 1623–1677. doi: 10.1016/S0967-0645(99)00157-5
Behrenfeld, M. J., and Falkowski, P. G. (1997). Photosynthetic rates derived from satellite-based chlorophyll concentration. Limnol. Oceanogr. 42, 1–20. doi: 10.4319/lo.1997.42.1.0001
Boyd, P. W., and Trull, T. W. (2007). Understanding the export of biogenic particles in oceanic waters: is there consensus? Prog. Oceanogr. 72, 276–312. doi: 10.1016/j.pocean.2006.10.007
Buitenhuis, E. T., Hashioka, T., and Le Quéré, C. (2013). Combined constraints on global ocean primary production using observations and models. Glob. Biogeochem. Cycles 27, 847–858. doi: 10.1002/gbc.20074
Campbell, J., Antoine, D., Armstrong, R., Arrigo, K., Balch, W., Barber, R., et al. (2002). Comparison of algorithms for estimating ocean primary production from surface chlorophyll, temperature, and irradiance. Glob. Biogeochem. Cycles 16:1035. doi: 10.1029/2001GB001444
Chen, C. C., Shiah, F. K., Chung, S. W., and Liu, K. K. (2006). Winter phytoplankton blooms in the shallow mixed layer of the South China Sea enhanced by upwelling. J. Mar. Syst. 59, 97–110. doi: 10.1016/j.jmarsys.2005.09.002
Chen, X., Pan, D., Bai, Y., He X., Chen, C. T. A., Kang, Y., et al. (2015). Estimation of typhoon-enhanced primary production in the South China Sea: a comparison with the Western North Pacific. Cont. Shelf Res. 111, 286–293. doi: 10.1016/j.csr.2015.10.003
Chen, C. T. A., Yu, S., Huang, T. H., Lui, H. K., Bai, Y., and He, X. (2020). “Changing biogeochemistry in the South China Sea,” in Changing Asia-Pacific Marginal Seas. Atmosphere, Earth, Ocean & Space, eds C. T. A. Chen and X. Guo (Singapore: Springer), doi: 10.1007/978-981-15-4886-4_12
Chen, J., Zheng, L., Wiesner, M. G., Chen, R., Zheng, Y., and Wong, H. K. (1998). Estimations of primary production and export production in the South China Sea based on sediment trap experiments. Chin. Sci. Bull. 43, 583–586. doi: 10.1007/BF02883645
Chen, T. Y., Tai, J. H., Ko, C. Y., Hsieh, C., Chen, C. C., Jiao, N., et al. (2016). Nutrient pulses driven by internal solitary waves enhance heterotrophic bacterial growth in the South China Sea. Environ. Microbiol. 18, 4312–4323. doi: 10.1111/1462-2920.13273
Chen, Y. L. L. (2000). Comparisons of primary productivity and phytoplankton size structure in the marginal regions of southern East China Sea. Cont. Shelf Res. 20, 437–458. doi: 10.1016/S0278-4343(99)00080-1
Chen, Y. L. L. (2005). Spatial and seasonal variations of nitrate-based new production and primary production in the South China Sea. Deep Sea Res. I 52, 319–340. doi: 10.1016/j.dsr.2004.11.001
Chen, Y. L. L., and Chen, H. Y. (2006). Seasonal dynamics of primary and new production in the northern South China Sea: the significance of river discharge and nutrient advection. Deep Sea Res. I 53, 971–986. doi: 10.1016/j.dsr.2006.02.005
Chen, Y. L. L., Chen, H. Y., Karl, D. M., and Takahashi, M. (2004). Nitrogen modulates phytoplankton growth in spring in the South China Sea. Cont. Shelf Res. 24, 527–541. doi: 10.1016/j.csr.2003.12.006
Chen, Y. L. L., Chen, H. Y., Lin, I. I., Lee, M. A., and Chang, J. (2007). Effects of cold eddy on phytoplankton production and assemblages in Luzon strait bordering the South China Sea. J. Oceanogr. 63, 671–683. doi: 10.1007/s10872-007-0059-9
Chen, Y. L. L., Chen, H. Y., Tuo, S. H., and Ohki, K. (2008). Seasonal dynamics of new production from Trichodesmium N2 fixation and nitrate uptake in the upstream Kuroshio and South China Sea basin. Limnol. Oceanogr. 53, 1705–1721. doi: 10.4319/LO.2008.53.5.1705
Chou, W. C., Chen, Y. L. L., Sheu, D. D., Shih, Y. Y., Han, C. A., Cho, C. L., et al. (2006). Estimated net community production during the summertime at the SEATS time-series study site, northern South China Sea: implications for nitrogen fixation. Geophys. Res. Lett. 33:L22610. doi: 10.1029/2005gl025365
Dandonneau, Y., Deschamps, P. Y., Nicolas, J. M., Loisel, H., Blanchot, J., Montel, Y., et al. (2004). Seasonal and interannual variability of ocean color and composition of phytoplankton communities in the North Atlantic, equatorial Pacific and South Pacific. Deep Sea Res. II 51, 303–318. doi: 10.1016/j.dsr2.2003.07.018
Dierssen, H. M. (2010). Perspectives on empirical approaches for ocean color remote sensing of chlorophyll in a changing climate. Proc. Natl. Acad. Sci. U.S.A. 107, 17073–17078. doi: 10.1073/pnas.0913800107
Du, C., Liu, Z., Dai, M., Kao, S. J., Cao, Z., Zhang, Y., et al. (2013). Impact of the Kuroshio intrusion on the nutrient inventory in the upper northern South China Sea: insights from an isopycnal mixing model. Biogeosciences 10, 6419–6432. doi: 10.5194/bg-10-6419-2013
Dunne, J. P., Armstrong, R. A., Gnanadesikan, A., and Sarmiento, J. L. (2005). Empirical and mechanistic models for the particle export ratio. Glob. Biogeochem. Cycles 19:GB4026. doi: 10.1029/2004GB002390
Field, C. B., Behrenfeld, M. J., Randerson, J. T., and Falkowski, P. (1998). Primary production of the biosphere: integrating terrestrial and oceanic components. Science 281, 237–240. doi: 10.1126/science.281.5374.237
Friedland, K. D., Stock, C., Drinkwater, K. F., Link, J. S., Leaf, R. T., Shank, B. V., et al. (2012). Pathways between primary production and fisheries yields of large marine ecosystems. PLoS One 7:e28945. doi: 10.1371/journal.pone.0028945
Gong, G. C. (1993). Correlation of chlorophyll a concentration and sea tech fluorometer fluorescence in seawater. Acta Oceanogr. Taiwan. 31, 117–126.
Gong, G. C., and Liu, G. J. (2003). An empirical primary production model for the East China Sea. Cont. Shelf Res. 23, 213–224. doi: 10.1016/S0278-4343(02)00166-8
Gong, G. C., Wen, Y. H., Wang, B. W., and Liu, G. J. (2003). Seasonal variation of chlorophyll a concentration, primary production and environmental conditions in the subtropical East China Sea. Deep Sea Res. II 50, 1219–1236. doi: 10.1016/s0967-0645(03)00019-5
Gregg, W. W., Conkright, M. E., Ginoux, P., O’Reilly, J. E., and Casey, N. W. (2003). Ocean primary production and climate: global decadal changes. Geophys. Res. Lett. 30:1809. doi: 10.1029/2003GL016889
Hama, T., Miyazaki, T., Ogawa, Y., Iwakuma, T., Takahashi, M., Otsuki, A., et al. (1983). Measurement of photosynthetic production of a marine phytoplankton population using a stable 13C isotope. Mar. Biol. 73, 31–36. doi: 10.1007/BF00396282
Hao, Q., Ning, X., Liu, Y., Cai, Y., and Le, F. (2007). Satellite and in situ observations of primary production in the northern South China Sea. Acta Oceanol. Sin. 29, 58–68. (in Chinese with English abstract)
Henson, S. A., Sanders, R., Madsen, E., Morris, P. J., Le Moigne, F., and Quartly, G. D. (2011). A reduced estimate of the strength of the ocean’s biological carbon pump. Geophys. Res. Lett. 38:L04606. doi: 10.1029/2011GL046735
Hill, V. J., and Zimmerman, R. C. (2010). Estimates of primary production by remote sensing in the Arctic Ocean: assessment of accuracy with passive and active sensors. Deep Sea Res. I 57, 1243–1254. doi: 10.1016/j.dsr.2010.06.011
Hu, Z., Tan, Y., Song, X., Zhou, L., Lian, X., Huang, L., et al. (2014). Influence of mesoscale eddies on primary production in the South China Sea during spring inter-monsoon period. Acta Oceanol. Sin. 33, 118–128. doi: 10.1007/s13131-014-0431-8
Hung, C. C., Chen, Y. F., Hsu, S. C., Wang, K., Chen, J. F., and Burdige, D. J. (2016). Using rare earth elements to constrain particulate organic carbon flux in the East China Sea. Sci. Rep. 6:33880. doi: 10.1038/srep33880
Hung, C. C., and Gong, G. C. (2007). Export flux of POC in the main stream of the Kuroshio. Geophys. Res. Lett. 34:L18606. doi: 10.1029/2007GL030236
Hung, C. C., and Gong, G. C. (2010). POC/234Th ratios in particles collected in sediment traps in the northern South China Sea. Estuar. Coast Shelf Sci. 88, 303–310. doi: 10.1016/j.ecss.2010.04.008
Hung, C. C., Gong, G. C., Chou, W. C., Chung, C. C., Lee, M. A., Chang, Y., et al. (2010). The effect of typhoon on particulate organic carbon flux in the southern East China Sea. Biogeosciences 7, 3007–3018. doi: 10.5194/bg-7-3007-2010
Hung, C. C., Gong, G. C., Chung, W. C., Kuo, W. T., and Lin, F. C. (2009). Enhancement of particulate organic carbon export flux induced by atmospheric forcing in the subtropical oligotrophic northwest Pacific Ocean. Mar. Chem. 113, 19–24. doi: 10.1016/j.marchem.2008.11.004
Hung, C. C., Tseng, C. W., Gong, G. C., Chen, K. S., Chen, M. H., and Hsu, S. C. (2013). Fluxes of particulate organic carbon in the East China Sea in summer. Biogeosciences 10, 6469–6484. doi: 10.5194/bg-10-6469-2013
Hung, C. C., Wong, G. T. F., Liu, K. K., Shiah, F. K., and Gong, G. C. (2000). The effects of light and nitrate levels on the relationship between nitrate reductase activity and 15NO3- uptake: field observations in the East China Sea. Limnol. Oceanogr. 45, 836–848. doi: 10.4319/lo.2000.45.4.0836
Ishizaka, J., Siswanto, E., Itoh, T., Murakami, H., Yamaguchi, Y., Horimoto, N., et al. (2007). Verification of vertically generalized production model and estimation of primary production in Sagami Bay, Japan. J. Oceanogr. 63, 517–524. doi: 10.1007/s10872-007-0046-1
Kameda, T., and Ishizaka, J. (2005). Size-fractionated primary production estimated by a two-phytoplankton community model applicable to ocean color remote sensing. J. Oceanogr. 61, 663–672. doi: 10.1007/s10872-005-0074-7
Kara, A. B., Rochford, P. A., and Hurlburt, H. E. (2000). An optimal definition for ocean mixed layer depth. J. Geophys. Res. 105, 16803–16821. doi: 10.1029/2000jc900072
Karl, D. M., Bates, N. R., Emerson, S., Harrison, P. J., Jeandel, C., Llinâs, O., et al. (2003). “temporal studies of biogeochemical processes determined from ocean time-series observations during the JGOFS era,” in Ocean Biogeochemistry: The Role of the Ocean Carbon Cycle in Global Change, ed. M. J. R. Fasham (New York, NY: Springer), 239–267. doi: 10.1007/978-3-642-55844-3_11
Kirk, J. T. O. (1994). Light and Photosynthesis in Aquatic Ecosystems. Cambridge: Cambridge University Press, 509.
Kudryavtseva, E., Bukanova, T., and Bubnova, E. (2018). “Primary productivity estimates based on the remote sea surface temperature data in the Baltic Sea,” in Proceedings of the 2018 IEEE/OES Baltic International Symposium (BALTIC), Klaipeda, 1–4. doi: 10.1109/BALTIC.2018.8634855
Lai, C. C., Fu, Y. W., Liu, H. B., Kuo, H. Y., Wang, K. W., Lin, C. H., et al. (2014). Distinct bacterial-production–DOC–primary-production relationships and implications for biogenic C cycling in the South China Sea shelf. Biogeosciences 11, 147–156. doi: 10.5194/bg-11-147-2014
Laws, E. A., D’Sa, E., and Naik, P. (2011). Simple equations to estimate ratios of new or export production to total production from satellite-derived estimates of sea surface temperature and primary production. Limnol. Oceanogr. 9, 593–601. doi: 10.4319/lom.2011.9.593
Lee, Z. P., Darecki, M., Carder, K. L., Davis, C. O., Stramski, D., and Rhea, W. J. (2005). Diffuse attenuation coefficient of downwelling irradiance: an evaluation of remote sensing methods. J. Geophys. Res. 110:C02017. doi: 10.1029/2004JC002573
Li, D., Chou, W. C., Shih, Y. Y., Chen, G. Y., Chang, Y., Chow, C. H., et al. (2018). Elevated particulate organic carbon export flux induced by internal waves in the oligotrophic northern South China Sea. Sci. Rep. 8:2042. doi: 10.1038/s41598-018-20184-9
Li, T., Bai, Y., Li, G., He, X., Chen, C.-T. A., Gao, K., et al. (2015). Effects of ultraviolet radiation on marine primary production with reference to satellite remote sensing. Front. Earth Sci. 9:237–247. doi: 10.1007/s11707-014-0477-0
Lin, I., Liu, W. T., Wu, C. C., Wong, G. T. F., Hu, C. M., Chen, Z. Q., et al. (2003). New evidence for enhanced ocean primary production triggered by tropical cyclone. Geophys. Res. Lett. 30:1718. doi: 10.1029/2003gl017141
Liu, K. K., Chao, S. Y., Shaw, P. T., Gong, G. C., Chen, C. C., and Tang, T. Y. (2002). Monsoon-forced chlorophyll distribution and primary production in the South China Sea: observations and a numerical study. Deep Sea Res. I 49, 1387–1412. doi: 10.1016/S0967-0637(02)00035-3
Liu, L., Bai, Y., Sun, R., and Niu, Z. (2021). Stereo observation and inversion of the key parameters of global carbon cycle: project overview and mid-term progressess. Remote Sens. Technol. Appl. 36, 11–24. doi: 10.11873/j.issn.1004-0323.2021.1.0011 (in Chinese with English abstract)
Ma, W., Chai, F., Xiu, P., Xue, H., and Tian, J. (2014). Simulation of export production and biological pump structure in the South China Sea. Geo-Mar. Lett. 34, 541–554. doi: 10.1007/s00367-014-0384-0
Mizobata, K., and Saitoh, S. (2004). Variability of Bering Sea eddies and primary productivity along the shelf edge during 1998–2000 using satellite multisensor remote sensing. J. Mar. Syst. 50, 101–111. doi: 10.1016/j.jmarsys.2003.09.014
Nielsen, E. S. (1952). The use of radio-active carbon (C14) for measuring organic production in the sea. ICES J. Mar. Sci. 18, 117–140. doi: 10.1093/icesjms/18.2.117
Ning, X., Chai, F., Xue, H., Cai, Y., Liu, C., and Shi, J. (2004). Physical-biological oceanographic coupling influencing phytoplankton and primary production in the South China Sea. J. Geophys. Res. 109:C10005. doi: 10.1029/2004JC002365
Pan, X., Wong, G. T. F., Shiah, F. K., and Ho, T. Y. (2012). Enhancement of biological productivity by internal waves: observations in the summertime in the northern South China Sea. J. Oceanogr. 68, 427–437. doi: 10.1007/s10872-012-0107-y
Parsons, T. R., Maita, Y., and Lalli, C. M. (1984). A Manual of Chemical and Biological Methods for Seawater Analysis. New York, NY: Pergamon, 173.
Pauly, D., and Christensen, V. (1995). Primary production required to sustain global fisheries. Nature 374, 255–257. doi: 10.1038/374255a0
Sarmiento, J. L., Hughes, T. M. C., Stouffer, R. J., and Manabe, S. (1998). Simulated response of the ocean carbon cycle to anthropogenic climate warming. Nature 393, 245–249. doi: 10.1038/30455
Shang, S., Li, L., Sun, F., Wu, J., Hu, C., Chen, D., et al. (2008). Changes of temperature and bio-optical properties in the South China Sea in response to Typhoon Lingling, 2001. Geophys. Res. Lett. 35:L10602. doi: 10.1029/2008GL033502
Shiah, F. K., Chung, S. W., Kao, S. J., Gong, G. C., and Liu, K. K. (2000). Biological and hydrographical responses to tropical cyclones (typhoons) in the continental shelf of the Taiwan Strait. Cont. Shelf Res. 20, 2029–2044. doi: 10.1016/s0278-4343(00)00055-8
Shiah, F. K., Gong, G. C., and Chen, C. C. (2003). Seasonal and spatial variation of bacterial production in the continental shelf of the East China Sea: possible controlling mechanisms and potential roles in carbon cycling. Deep Sea Res. II 50, 1295–1309. doi: 10.1016/S0967-0645(03)00024-9
Shiah, F. K., Tu, Y. Y., Tsai, H. S., Kao, S. J., and Jan, S. (2005). A case study of system and planktonic responses in a subtropical warm plume receiving thermal effluents from a power plant. Terr. Atmos. Ocean. Sci. 16, 513–528. doi: 10.3319/TAO.2005.16.2.513(O)
Shih, Y. Y., Hsieh, J. S., Gong, G. C., Chou, W. C., Lee, M. A., Hung, C. C., et al. (2013). Field observations of changes in SST, chlorophyll and POC flux in the southern East China Sea before and after the passage of typhoon Jangmi. Terr. Atmos. Ocean. Sci. 24, 899–910. doi: 10.3319/TAO.2013.05.23.01(Oc)
Shih, Y. Y., Hung, C. C., Gong, G. C., Chung, W. C., Wang, Y. H., Lee, I. H., et al. (2015). Enhanced particulate organic carbon export at eddy edges in the oligotrophic western North Pacific ocean. PLoS One 10:e0131538. doi: 10.1371/journal.pone.0131538
Shih, Y. Y., Hung, C. C., Huang, S. Y., Muller, F. L. L., and Chen, Y. H. (2020a). Biogeochemical variability of the upper ocean response to typhoons and storms in the northern South China Sea. Front. Mar. Sci. 7:151. doi: 10.3389/fmars.2020.00151
Shih, Y. Y., Hung, C. C., Tuo, S., Shao, H. J., Chow, C. H., Muller, F. L. L., et al. (2020b). The impact of eddies on nutrient supply, diatom biomass and carbon export in the northern South China Sea. Front. Earth Sci. 8:537332. doi: 10.3389/feart.2020.537332
Shih, Y. Y., Lin, H. H., Li, D., Hsieh, H. H., Hung, C. C., and Chen, C. T. A. (2019). Elevated carbon flux in deep waters of the South China Sea. Sci. Rep. 9:1496. doi: 10.1038/s41598-018-37726-w
Siswanto, E., Ishizaka, J., and Yokouchi, K. (2006). Optimal primary production model and parameterization in the eastern East China Sea. J. Oceanogr. 62, 361–372. doi: 10.1007/s10872-006-0061-7
Tan, S. C., and Shi, G. Y. (2009). Spatiotemporal variability of satellite-derived primary production in the South China Sea, 1998–2006. J. Geophys. Res. 114:G03015. doi: 10.1029/2008JG000854
Tang, S., and Chen, C. (2016). Novel maximum carbon fixation rate algorithms for remote sensing of oceanic primary productivity. IEEE J. Sel. Top. Appl. Earth Obs. Remote Sens. 9, 5202–5208. doi: 10.1109/JSTARS.2016.2574898
Tang, S., Chen, C., Zhan, H., Zhang, J., and Yang, J. (2008). An appraisal of surface chlorophyll estimation by satellite remote sensing in the South China Sea. Int. J. Remote Sens. 29, 6217–6226. doi: 10.1080/01431160802175579
Tilstone, G., Smyth, T., Poulton, A., and Hutson, R. (2009). Measured and remotely sensed estimates of primary production in the Atlantic Ocean from 1998 to 2005. Deep Sea Res. II 56, 918–930. doi: 10.1016/j.dsr2.2008.10.034
Tilstone, G. H., Taylor, B. H., Blondeau-Patissier, D., Powell, T., Groom, S. B., and Rees, A. P. (2015). Comparison of new and primary production models using SeaWiFS data in contrasting hydrographic zones of the northern North Atlantic. Remote Sens. Environ. 156, 473–489. doi: 10.1016/j.rse.2014.10.013
Tseng, C. M., Wong, G. T. F., Lin, I. I., Wu, C. R., and Liu, K. K. (2005). A unique seasonal pattern in phytoplankton biomass in low-latitude waters in the South China Sea. Geophys. Res. Lett. 32:L086080. doi: 10.1029/2004gl022111
Wong, G. T. F., Ku, T. L., Mulholland, M., Tseng, C. M., and Wang, D. P. (2007). The SouthEast Asian time-series study (SEATS) and the biogeochemistry of the South China Sea-an overview. Deep Sea Res. II 54, 1434–1447. doi: 10.1016/J.DSR2.2007.05.012
Yamada, K., Ishizaka, J., and Nagata, H. (2005). Spatial and temporal variability of satellite primary production in the Japan Sea from 1998 to 2002. J. Oceanogr. 61, 857–869. doi: 10.1007/s10872-006-0005-2
Yang, J. Y. T., Hsu, S. C., Dai, M., Hsiao, S. S. Y., and Kao, S. J. (2014). Isotopic composition of water-soluble nitrate in bulk atmospheric deposition at Dongsha Island: sources and implications of external N supply to the northern South China Sea. Biogeosciences 11, 1833–1846. doi: 10.5194/bg-11-1833-2014
Zhao, H., Tang, D., and Wang, Y. (2008). Comparison of phytoplankton blooms triggered by two typhoons with different intensities and translation speeds in the South China Sea. Mar. Ecol. Prog. Ser. 365, 57–65. doi: 10.3354/meps0741838
Zhong, J., Wallin, M. B., Wang, W., Li, S.-L., Guo, L., Dong, K., et al. (2021). Synchronous evaporation and aquatic primary production in tropical river networks. Water Res. 200:117272. doi: 10.1016/j.watres.2021.117272
Keywords: carbon fixation rate, remote sensing, time-series study, global warming, low-latitude ocean, VGPM
Citation: Shih Y-Y, Shiah F-K, Lai C-C, Chou W-C, Tai J-H, Wu Y-S, Lai C-Y, Ko C-Y and Hung C-C (2021) Comparison of Primary Production Using in situ and Satellite-Derived Values at the SEATS Station in the South China Sea. Front. Mar. Sci. 8:747763. doi: 10.3389/fmars.2021.747763
Received: 26 July 2021; Accepted: 02 September 2021;
Published: 04 October 2021.
Edited by:
Bernardo Antonio Perez Da Gama, Fluminense Federal University, BrazilReviewed by:
Hartmut Schulz, University of Tuebingen, GermanyDavid Michael Karl, University of Hawaii, United States
Copyright © 2021 Shih, Shiah, Lai, Chou, Tai, Wu, Lai, Ko and Hung. This is an open-access article distributed under the terms of the Creative Commons Attribution License (CC BY). The use, distribution or reproduction in other forums is permitted, provided the original author(s) and the copyright owner(s) are credited and that the original publication in this journal is cited, in accordance with accepted academic practice. No use, distribution or reproduction is permitted which does not comply with these terms.
*Correspondence: Chin-Chang Hung, cchung@mail.nsysu.edu.tw