- 1Nansen Environmental and Remote Sensing Center, Bergen, Norway
- 2Nansen Environmental and Remote Sensing Center, and Bjerknes Centre for Climate Research, Bergen, Norway
- 3Geophysical Institute, University of Bergen, Bergen, Norway
Phytoplankton blooms provide biomass to the marine trophic web, contribute to the carbon removal from the atmosphere and can be deadly when associated with harmful species. This points to the need to understand the phenology of the blooms in the Barents, Norwegian, and North seas. We use satellite chlorophyll-a from 2000 to 2020 to assess robust climatological and the interannual trends of spring and summer blooms onset, peak day, duration and intensity. Further, we also correlate the interannual variability of the blooms with mixed layer depth (MLD), sea surface temperature (SST), wind speed and suspended particulate matter (SPM) retrieved from models and remote sensing. The climatological spring blooms start on March 10th and end on June 19th. The climatological summer blooms begin on July 13th and end on September 17th. In the Barents Sea, years of shallower mixed layer (ML) driven by both calm waters and higher freshwaters input keeps the phytoplankton in the euphotic zone, causing the spring bloom to start earlier and reach higher biomass but end sooner due to the lack of nutrients upwelling from the deep. In the Norwegian Sea, a correlation between SST and the spring blooms is found. Here, warmer waters are correlated to earlier and stronger blooms in most regions but with later and weaker blooms in the eastern Norwegian Sea. In the North Sea, years of shallower ML reduces the phytoplankton sinking below the euphotic zone and limits the SPM increase from the bed shear stress, creating an ideal environment of stratified and clear waters to develop stronger spring blooms. Last, the summer blooms onset, peak day and duration have been rapidly delaying at a rate of 1.25-day year–1, but with inconclusive causes based on the parameters assessed in this study.
Introduction
Phytoplankton blooms play a crucial role in the marine trophic web and global climate. By assimilating the sunlight, carbon dioxide and nutrients, the algae produce high biomass concentration blooms that feed zooplankton and the higher trophic levels or sink below the euphotic zone. Thus, the algae blooms can support the development of fish larvae (Townsend and Cammen, 1987; Platt et al., 2003; Vikebø et al., 2012; Asch et al., 2019), provide biomass to the benthic fauna (Zhang et al., 2015) and also contribute to the carbon removal from the atmosphere (Legendre, 1990; Leblanc et al., 2018). Conversely, harmful algae blooms (HABs) can be devastating and lethal (Pettersson and Pozdnyakov, 2013; Gobler, 2020). The high organic matter concentration generated during the bloom can damage or clog fish gills (Chang et al., 1990; Kent et al., 1995) and increase bacteria activity, depleting the dissolved oxygen and causing hypoxia in fishes (Harrison et al., 2017; Mohd-Din et al., 2020). Besides, some algal species can produce toxins, leading to the mortality of fish or even humans when contaminated mussels are consumed (Tangen, 1977; Kaartvedt et al., 1991; Landsberg et al., 2020). The influence of algae blooms on natural living resources and global climate points to the need of assessing the phenology of algae blooms, such as the date of onset, duration, date of the bloom reaching its maximum biomass and the maximum biomass (intensity).
In the Barents, Norwegian, and North seas, two well-known seasonal blooms are the spring and later summer/autumn blooms. During the spring, the sunlight increases and allows the phytoplankton to consume the nutrients upwelled to the upper layers during the winter storms and through terrestrial river discharges (in the coastal waters). At the same time, the mixed layer (ML) shallows above the Sverdrup critical depth and makes the phytoplankton biomass production exceed respiration losses, triggering the spring bloom (Sverdrup, 1953). During summer or autumn, the surface waters are depleted of nutrients due to the algae consumption during the spring bloom. Remineralization, upwelling and river runoff refresh the surface waters with nutrients again, leading to a secondary bloom called summer or autumn bloom (Sverdrup, 1953; Glen Harrison et al., 2013; Sundby et al., 2016).
Although the main processes of spring and summer blooms are well understood in the Barents, Norwegian, and North seas, there is a need to understand mechanisms that can influence the phytoplankton bloom phenology on interannual variability as well as its response to climate change. During a survey campaign in 2013 in the North Atlantic, Naustvoll et al. (2020) observed that regions with earlier shallowing of ML are related to earlier spring blooms. Using satellite data from 2003 to 2017 along the Norwegian coast, Vikebø et al. (2019) found that years with strong winds delay the spring bloom onset. Using a water column model, Opdal et al. (2019) suggested that reducing the water transparency could delay the spring bloom onset in the North Sea. While these studies provided valuable insight on the variability of spring blooms onset and its potential driving mechanisms, still little is known about the duration, date of the peak and intensity of the spring bloom. Besides, the phenology of summer blooms has not been addressed to our knowledge in this region. Thanks to the available extended period of optical remote sensing data (from 1998 to present), one can now more robustly assess the spatial distribution, climatology, trend and potential drivers of interannual variability of both spring and summer blooms.
Here we take advantage of ocean color remote sensing data to provide a comprehensive assessment of both spring and summer blooms phenology in the Barents, Norwegian, and North seas. We use pattern recognition tools to cluster the bloom phenology in regions of comparable statistical behavior, which reduces small-scale noise and allows for a coherent visualization of the properties of the blooms phenology–onset, duration, peak day and intensity. Furthermore, the extended data set used (2000–2020) allows for providing a primary analysis of the trend of the property of the bloom phenology and of potential drivers that can influence the interannual variability.
Materials and Methods
Study Region
This study focuses on the Norwegian shelf seas, including the North Sea, the Norwegian Sea, and the Barents Sea entrance (Figure 1). The North Sea is shallow, with depth varying from 20 m in the southern region to 700 m in the south of Norway (Eisma et al., 1987). In the Norwegian Sea, the shallowest waters are on the Norwegian Continental Shelf, varying from 100 to 400 m, and the deepest waters are in the Norway Basin, ranging from 3600 to 3800 m. The Barents Sea has a wide continental shelf varying from 100 to 300 m deep (Perry, 1986).
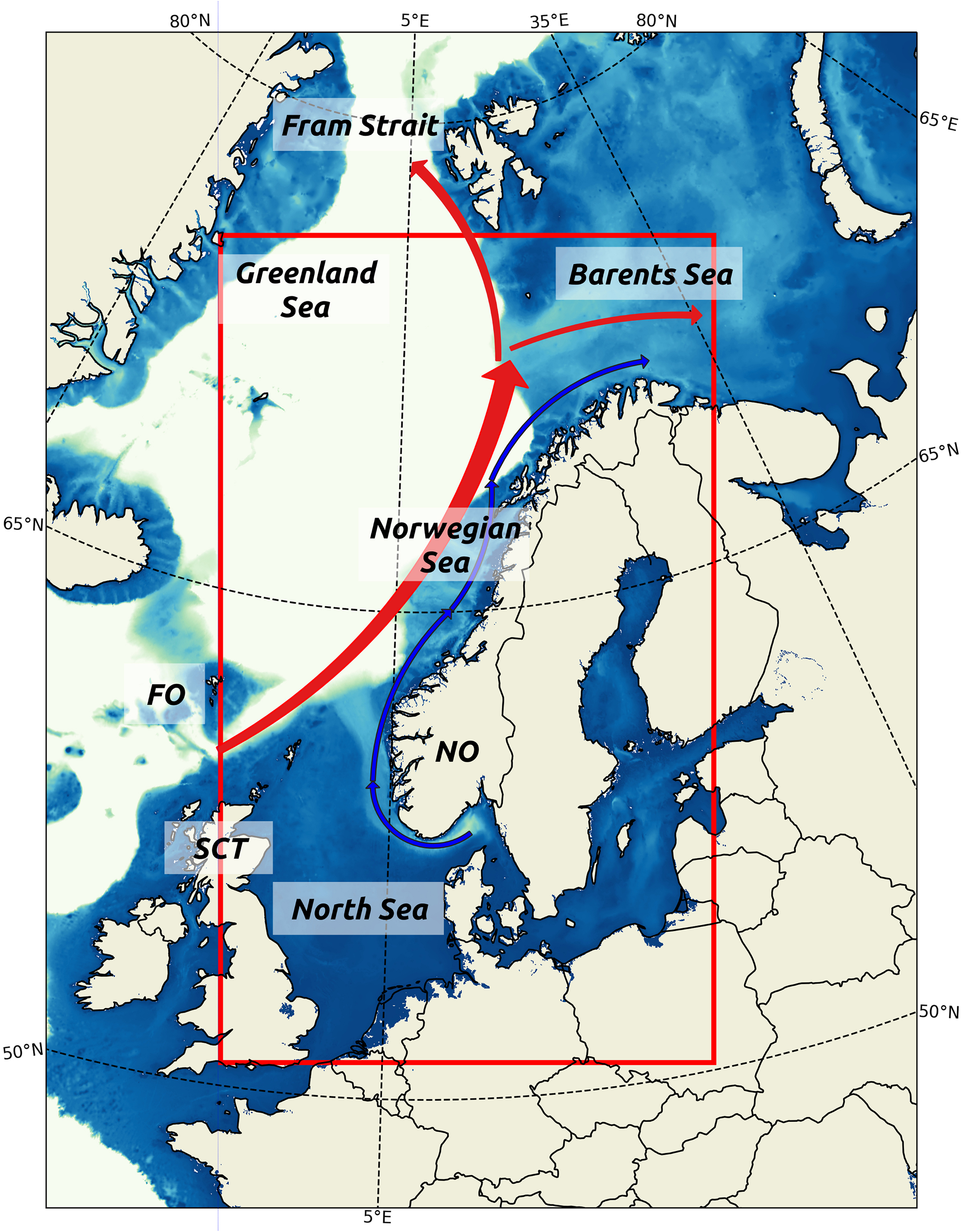
Figure 1. The study region. The red box delimits the area where the blooms phenology is assessed. Red arrow is a coarse representation of the NwAC and blue arrow is the NCC. Abbreviations are Faroe Islands (FO), Norway (NO), and Scotland (SCT).
Two significant currents dominate the circulation in the study region, the Norwegian Atlantic Current (NwAC) and the Norwegian Coastal Current (NCC). The NwAC originates from the North Atlantic Current as it flows between the Faroe Islands and Scotland and continues northward along with the Norwegian Continental Shelf break. The NwAC splits into two branches close to the Barents Sea. One branch flows eastward into the Barents Sea, while the other flows northward into the Fram Strait (Furevik et al., 2002; Eldevik et al., 2009). The NCC flows from the south of Norway and along the Norwegian coast up the Barents Sea. It is substantially fresher than the Atlantic Water as it transports fresh waters from the land inflow, the Baltic Sea and the North Sea (Mork, 1981).
Satellite Chlorophyll-a Measurements
Chlorophyll-a concentration (mg m–3) is used as a proxy to phytoplankton biomass and has been retrieved regularly by satellite remote sensing since 1998. We accessed satellite data from the European Space Agency (ESA) Ocean Colour Climate Change Initiative (OC-CCI) project (Sathyendranath et al., 2019), product version 5, which has a spatial resolution of 4 km at a fixed geographical grid and binned in an 8-day average between 1998 and 2020 (Table 1). The OC-CCI data is intended for climate studies and merges Chl-a concentration estimated from MODIS, MERIS, OLCI, SeaWiFS, and VIIRS sensors. We have used the data set in the 8-day average bin so that cloud contaminated grid cells are reduced and allow a continuous estimation of the Chl-a time series. From 1998 to 1999, there is only SeaWiFS data available and many grid cells with gaps in the high latitudes of the Norwegian Sea. Thus, we have excluded these 2 years from our analysis.
Global validation of the OC-CCI satellite data with in situ Chl-a measurements (n = 17901) showed good agreements between the two data sets–with a correlation coefficient (R) of 0.78, a root mean square difference of 0.3 mg m–3 and a bias of 0.003 mg m–3 (Calton, 2020). Note that the OC-CCI product uses an algorithm tuned to perform best in open ocean case-1 waters, where the phytoplankton abundance controls both direct and indirect the optical properties of water (Morel and Prieur, 1977). The OC-CCI Chl-a product has limited validity for coastal case-2 waters where the land input or resuspension of suspended particulate matter (SPM) and yellow substance contributes significantly to the optical properties. Since case-2 waters occur mainly close to the coastline and standard case-1 water algorithms perform poorly in such regions (Blondeau-Patissier et al., 2004; Folkestad et al., 2007), we have discarded grid cells within 30 km off the coastline from the analysis.
This study relies entirely on exploiting information on the phytoplankton dynamics derived from satellite remote sensing sensors. Since passive sensors depend on the solar light scattering, there is no optical satellite data in the winter darkness. Reliable data are only available from February to November in the southern region of the study region and from March to October in the northern region. Although the low sun angle and light availability during the winter may not be sufficient to trigger algae blooms, the bloom onset may occur just before the satellite measurements have sufficient quality when the light starts increasing. This issue was observed in the North Sea (see section “Sub-regions and Chlorophyll-a Validation”).
Auxiliary Data
Auxiliary data is used to correlate the interannual variability of bloom phenology (onset, peak day, duration, and intensity) with potential key drivers. The parameters assessed are the mixed layer depth (MLD), sea surface temperature (SST), wind speed, SPM concentration, and river runoff (Table 1).
We accessed SST (K) from the ESA SST CCI and C3S global SST Reprocessed product level 4, available on the Copernicus Marine Environment Monitoring Service (CMEMS)1. The product is created by running the Operational Sea Surface Temperature and Sea Ice Analysis (OSTIA) system (Good et al., 2020), which combines satellite (AATSR, ATSR, SLSTR, and AVHRR) and in situ observations to produce gap-free maps of daily average SST at 0.05° of spatial resolution (Merchant et al., 2019).
Suspended particulate matter (g m––3) data was obtained by satellite observations and accessed from the GlobColour project2. SPM is estimated using Gohin (2011) algorithm on MODIS, MERIS, OLCI, SeaWiFS, and VIIRS sensors, and binned at an 8-day interval at a 4 km of spatial resolution. Note that SPM is estimated by radiometric measurements from the same optical sensors used for estimating Chl-a concentration, and they may share a common bias.
The MLD (m) is provided by the CMEMS Arctic MFC TOPAZ system (Sakov et al., 2012; Xie et al., 2017). The TOPAZ system is a coupled ocean–sea ice data assimilation system for the North Atlantic and the Arctic Ocean. The model couples a Hybrid Coordinate Ocean Model (Bleck, 2002) with an elasto-viscous-plastic sea ice model (Hunke and Dukowicz, 1997). TOPAZ assimilates available ocean and sea ice data with the Ensemble Kalman filter (Evensen, 2003) every week. The MLD is calculated using a density criterion with a threshold of 0.01 kg m–1, as in Petrenko et al. (2013) and Ferreira et al. (2015).
Surface wind speed (m s–1) was obtained from the IFREMER CERSAT Global Blended Mean Wind Fields reprocessed product accessed from the CMEMS. Wind speed is derived from scatterometers (ASCAT-A and ASCAT-B satellites), the SSMIS radiometers (F16, F17, F18, and F19 satellites) and the WindSat radiometer onboard the Coriolis satellite. All satellite observations are binned into a single product with a 6-hourly wind field at a 0.25° of spatial resolution.
We use the daily flow data from the Tana (70.070°N, 28.016°E) and Målselva (69.035°N, 18.658°E) rivers accessed from The Norwegian Water Resources and Energy Directorate3. The river flow was used as a proxy of coastal waters freshening to discuss the MLD variability in sections “Barents Sea: Stronger, Earlier and Shorter Spring Blooms Driven by Shallower Mixed Layer” and “Mixed Layer Depth and Sea Surface Temperature Influence on the Spring Bloom Phenology in the Norwegian Sea.”
Clustering Sub-Regions
We have used cluster analysis to objectively identify 20 sub-regions of similar bloom phenology using the 21 years of Chl-a time series. In the pre-processing, we subset the time series between the Julian days 60 and 300 to exclude the winter when there is a lack of data. Although we have accessed Chl-a data binned in 8-day average, 30.8% of the data cube (latitude × longitude × time) is still missing–mainly in the Greenland Sea–due to the cloud contamination. We interpolate those missing values as we need them for clustering the sub-regions. For each year, we use linear interpolation (limit = 10-time intervals) for filling the missing values over time, reducing the missing data to 5.2%. The remaining missing values are in the beginning and end of the time series in the higher latitudes. Since we cannot interpolate values in the time series borders, we filled them with 0.01, assuming that the Chl-a concentration is virtually null but still present during the beginning and end of the winter. We emphasize that the filling value with 0.01 was only used for clustering and not further used to assess the blooms phenology.
We use principal component analysis to reduce the dimension of 630-time intervals to 300 components, accounting for 95% of the Chl-a time variability. Then, we use the k-means algorithm (MacQueen, 1967) fed with the components as features and the grid cells as observations. We chose the optimal number of sub-regions (k) as the maximum value where coherent (spatially continuous) sub-regions were still obtained. With more than 20 clusters, we got noisy sub-regions composed of a few grid cells. We use the k-means++ algorithm (Arthur and Vassilvitskii, 2007) to define the initial seeds and avoid changing the sub-regions areas each time the k-means is reproduced.
In the post-processing, we observed that four sub-regions in the Greenland Sea were heavily contaminated by clouds and probably sea ice. We set a high interpolation limit of 10-time intervals in the pre-processing to address this issue during the clustering. However, this wide range of interpolation made the Chl-a time series in the Greenland Sea too linear and unrealistic to assess the blooms phenology. For this reason, we have excluded those four sub-regions, resulting in 16 sub-regions. Furthermore, a few grid cells along the sub-regions boundaries were overlapping each other sub-region. We have smoothed the boundaries by removing those grid cells and interpolating with the nearest sub-region.
For assessing the clustering performance, we computed the Silhouette score (Rousseeuw, 1987) using the 300 components of every grid cell:
where S is the Silhouette score for a given grid cell i, b is the mean nearest-cluster Euclidean distance, and a is the mean intra-cluster Euclidean distance. A Silhouette score higher than 0 means that the Chl-a time series of one individual grid cell is more similar–in the Euclidean space–to the cells of the same sub-region than the remaining regions.
Satellite Chlorophyll-a Validation
We assess the performance of the OC-CCI Chl-a product in the 16 sub-regions by comparing the daily OC-CCI Chl-a with in situ Chl-a collected on the same day (Table 1). The in situ Chl-a data was provided by the Norwegian Institute of Marine Research (IMR) from two different sources. One was retrieved from the Norwegian Marine Data Centre4 with the registered id imr_11. The other was provided by the Plankton Research Group (PRG) (courtesy of Dr. Kjell Gundersen) and was analyzed by the Plankton Chemistry Laboratory at IMR. For measuring in situ Chl-a, a standard volume (265 mL) is collected and filtered onto a 25 mm GFF filter and stored frozen (−20°C) until analysis in the land-based laboratory. Samples are transported in specially designed coolers, with an internal temperature recorder rated for −20°C for a minimum of 3 days. The samples are thawed in 90% acetone in the laboratory and stored at +4°C overnight before analysis on a Turner Design 10AU fluorometer. The fluorometer is regularly calibrated using a solid standard with known fluorescence following Holm-Hansen and Riemann (1978). Measurements of the top 10 m have been averaged to compare well with the remote sensing data. Note that the satellite data is calibrated with Chl-a estimated by high-performance liquid chromatography (HPLC), which slightly differs from Chl-a estimated by fluorometers available in this study (Neveux et al., 1990; Reynolds et al., 2001; Giannini et al., 2021).
To match the in situ and satellite Chl-a data, we followed the protocols described by Bailey and Werdell (2006) with some slight adjustments. As the satellite product is a merged output of different sensors and has more than one measurement time, we matched the satellite data with in situ data collected on the same day and between 09:00 and 15:00 UTC+2. For each match-up, we extracted the average of a 3 × 3 window from the satellite product and compared it with the in situ measurements. Besides, we computed the coefficient of variation in the same window for assessing spatial homogeneity in the satellite product. Windows with a coefficient of variation higher than 30% were considered spatial heterogeneous and unsuitable for assessing the satellite data accuracy, so we removed them. Last, we removed a few in situ samples with values lower than 0.1 mg m–3, which were substantially overestimating the mean absolute percentage error (MAPE).
The satellite Chl-a validation includes linear correlation (R), MAPE, and the root mean squared error (RMSE):
where X and Y are the independent and dependent variables, respectively; cov is the covariance function; σ is the standard deviation; n is the number of samples; t is the sample. For the satellite and in situ match-ups, X is the in situ Chl-a, and Y is the satellite Chl-a. Furthermore, we also fit a linear regression between satellite and in situ match-ups.
Bloom Phenology Estimates
This study only focuses on the seasonal spring and summer blooms that last a couple of weeks. As mentioned in section “Satellite Chlorophyll-a Measurements,” the Chl-a ocean color data is averaged into 8-day bins with the frequency of Chl-a variations in each bin reaching as high as 1/8 day–1. We use functional data analysis (Ramsay and Silverman, 2005) to smooth the seasonal Chl-a time series and remove high frequencies from Chl-a variability. We smooth each year time series of each grid cell using a Fourier series with five basis coefficients. The number of basis coefficients can significantly impact the smoothness of the time series. On one hand, having too many basis coefficients will fail to smooth the time series enough and contains the high frequencies of Chl-a variability. On the other hand, too few basis coefficients can exaggeratedly smooth the time series and miss the phytoplankton blooms. We tested the number of basis coefficients varying from 3 to 10 on 1 year of data, and five basis coefficients were found to be the most suitable for representing the seasonality of spring and summer blooms (not shown). With less than four basis coefficients, some summer blooms in the Barents Sea were not detected, and with more than six basis coefficients, the weekly variability of Chl-a was included for spring bloom in the North Sea.
We estimate the bloom onset, peak day, duration and intensity using the smoothed Chl-a time series. The peak day corresponds to the local Chl-a maxima, and the bloom intensity is the Chl-a concentration at the peak. Since there is high uncertainty in the ocean color derived Chl-a concentration estimate below 0.5 mg m–3 (see section “Sub-regions and Chlorophyll-a Validation”), the local Chl-a maximum is only computed when values reach more than 0.5 mg m–3.
Several methods were considered for estimating the bloom onset from the time series of Chl-a observations, such as the threshold method, the rate of change method and the cumulative sum method (Brody et al., 2013). The threshold method computes the climatological median of a Chl-a time series, defines a threshold value above the median, and the onset is estimated when the Chl-a concentration reaches this threshold. The rate of change method estimates the onset as the date with the highest increase of Chl-a before the peak. The cumulative sum method computes the cumulative sum from the beginning of the time series to the peak, and the onset is estimated when the sum reaches a percentual threshold of the cumulative sum (e.g., 15%). The threshold method cannot be applied in our case because there is no data available during the winter, and the climatological median of the Chl-a time series cannot be estimated. We found the rate of change method to delay the onset by a few weeks for blooms of longer duration (not shown). The cumulative sum method estimates the onset when the Chl-a starts to increase and can be applied despite the lack of winter data, and it is retained for the following analysis. We use the 15% threshold to estimate the bloom onset as recommended in Brody et al. (2013) for subpolar regions.
Since the annual Chl-a time series usually has two peaks of bloom intensity, in spring and summer, and the ocean color data is not available at the beginning of the year during the winter, we had to define the beginning of the time series for computing each bloom onset. For the spring bloom onset, the beginning of the time series was defined as the first local minima of the annual Chl-a time series. For estimating the summer bloom onset, the beginning of the time series was defined as the first local minima between the first and second peaks. Last, the bloom duration is estimated as the time between the onset and peak day.
Climatology, Trends and Detrended Correlation Assessment
We estimate the spring and summer blooms phenology for each grid cell of each year. The climatological spring and summer blooms onset, peak day, duration and intensity are estimated by averaging the grid cells belonging to each of the 16 sub-regions over the entire period. We also estimate the average value of the auxiliary parameters during the climatological bloom period (from onset to peak day) of each sub-region and year. For computing the trends of all parameters assessed, we use the sub-regions averaged values of each year and fit a linear least-squares regression. In order to relate the interannual variability of blooms phenology characteristics with key potential drivers, we subtract the trends from the interannual variability. Then, we use the Pearson correlation between the blooms phenology and the auxiliary parameters.
The correlation significance level is estimated using a probability density function (Student, 1908). For a given two random datasets with zero correlation, a probability density function of R is drawn for a given n (number of years). Then, we use a two-sided Wald test between the estimated R and the probability density function, and the null hypothesis is that the R is zero for an α threshold of 0.05. In practical terms, for a given n = 21 the −0.42 < R < 0.42 is insignificant. Note that it was ensured that there is no autocorrelation in the interannual variability. To estimate the trend significance, we test the slope of the linear least-squares regression using a two-sided Wald test with an α threshold of 0.05.
Results
Sub-Regions and Chlorophyll-a Validation
The Barents, Norwegian, and North seas have been clustered into 16 sub-regions using the Chl-a time series (Figure 2). Four sub-regions (1–4) are in the Barents Sea, six sub-regions are in the Norwegian Sea (5–10), and the other six regions are in the North Sea (11–16). All 16 sub-regions are well localized in specific geographical regions except for sub-region 11, which starts in the central North Sea, extends throughout Skagerrak and covers the southern part of the NCC. Based on earlier investigations (Pozdnyakov et al., 2017), we initially intended to split the sub-region 11. However, all further clustering analyses gave nearly identical results for splitting the sub-region further, so we decided to keep it as one sub-region.
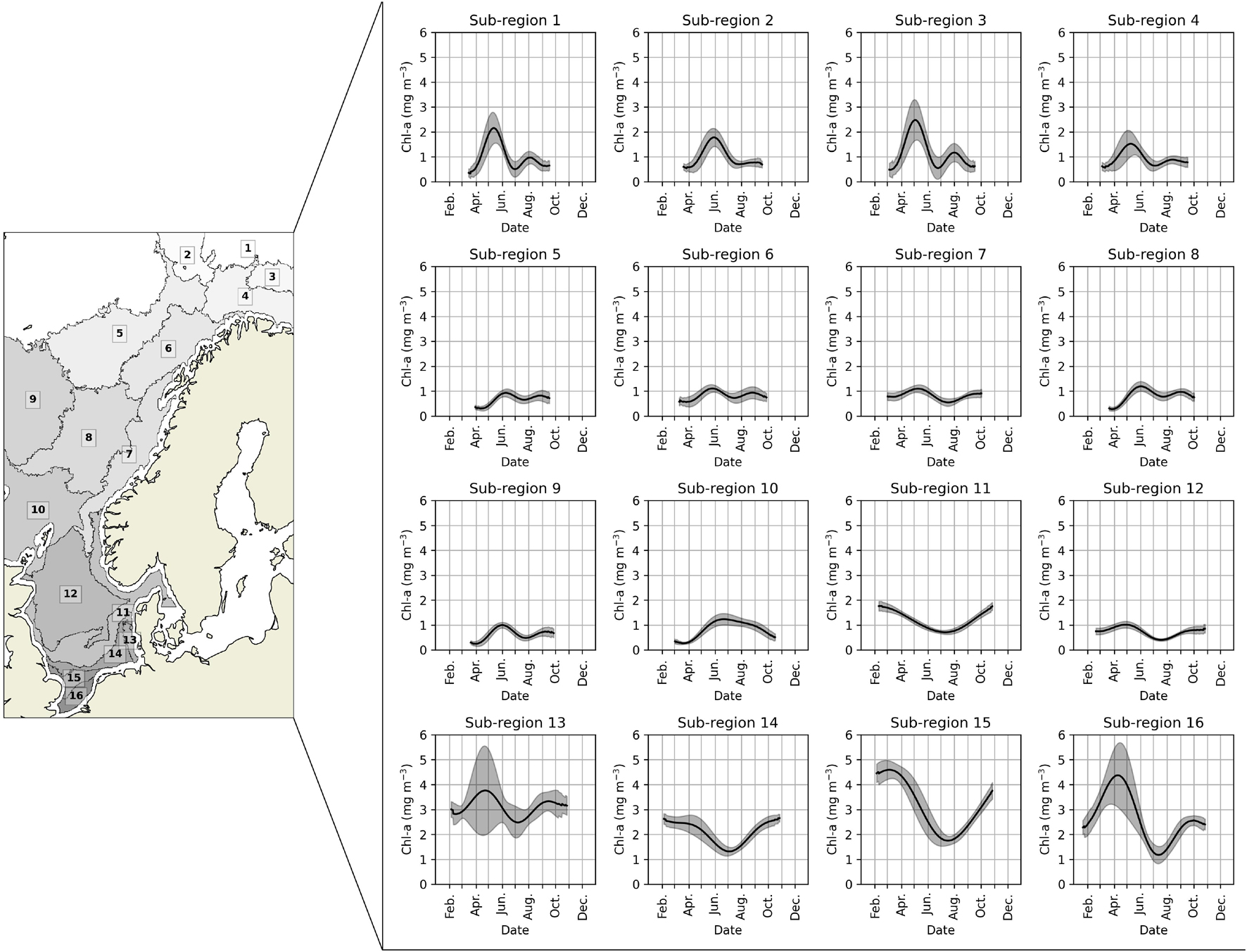
Figure 2. The clustered sub-regions (left) and their annual Chl-a time series (right). The solid black line is the average over the period 2000–2020 and the shaded area is one standard deviation of the interannual variability.
The 16 sub-regions showed heterogeneous annual Chl-a time series patterns and, consequently, different spring and summer blooms phenology (Figure 2). The sub-regions heterogeneity is also supported by the Silhouette score, where 84% of the grid cells showed values higher than 0 (Supplementary Figure 1). Most grid cells with a Silhouette score lower than 0 are concentrated on the sub-regions boundaries. The Chl-a time-series patterns are expected to vary among the sub-regions smoothly rather abruptly. Thus, Silhouette scores lower than 0 in the boundaries represent transitional areas among the Chl-a time-series patterns shown in Figure 2.
As explained in section “Satellite Chlorophyll-a Measurements,” satellite data is only of sufficient quality after the beginning of February to the end of October, and there is no data available during the winter. This restriction limited the assessment of the spring bloom phenology in the southernmost sub-regions 11 and 14. There, Chl-a concentration only decreases after the middle of February, and there is no spring peak. Besides, the secondary bloom seems to reach its peak after October. Without detecting the bloom peaks, it is not possible to assess the bloom phenology in those regions. Another limitation occurs in sub-region 2 and 10, where the summer bloom developed only in a few grid cells and in some years. Since summer blooms seem rare in those sub-regions, it is unfeasible to compute a robust summer bloom climatology based on the 21 years dataset.
The OC-CCI product shows a fair agreement with in situ Chl-a concentration in sub-regions from 1 to 12 (Figure 3). The R varies from 0.37 to 0.77, MAPE varies from 33 to 63% and RMSE varies from 0.3 to 0.8 mg m–3. Most regions show results comparable to the OC-CCI overall validation for case-1 waters, which showed an R of 0.78 and RMSE of 0.23 for Chl-a logged data (Calton, 2020). Besides, the match-up also shows that the main source of the misfits relates to a satellite overestimation of Chl-a concentrations for values lower than 0.5 mg m–3. This overestimation for the lower-level concentrations is not of great concern for assessing the seasonal bloom phenology because the bloom is only computed when the peak reaches values above 0.5 mg m–3. Furthermore, we do not have access to enough in situ samples (n < 8) matching satellite data in sub-regions from 13 to 16. Since other studies show a need to use case-2 water algorithms for retrieving Chl-a concentration in the south of the North Sea (De Cauwer et al., 2004; Tilstone et al., 2012), we decided to exclude those sub-regions in the assessment of bloom phenology.
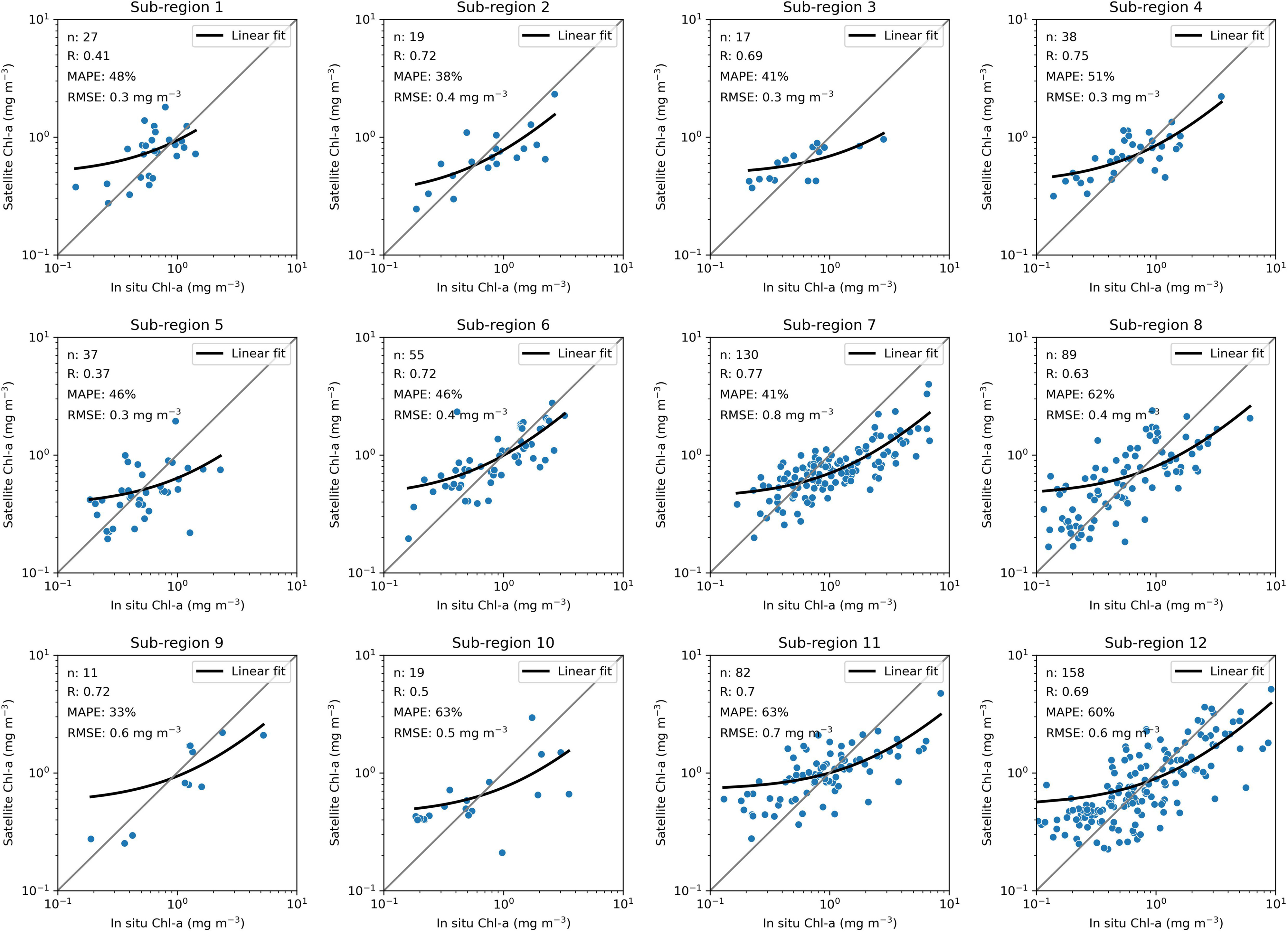
Figure 3. Comparison between in situ and satellite Chl-a concentration for each sub-region. The black line is a linear model fitted using least squared regression, and it is curved due to the axes log scale.
Bloom Climatology
The average spring bloom in the North Sea (sub-region 12) starts on March 10th and lasts until April 26th for 46 days (Figure 4), reaching 1.2 mg m–3 at the peak during the studied period. In the Norwegian Sea (sub-regions 6–10), the timing (onset, peak day, and duration) of the spring blooms has high longitudinal variability. The average bloom starts on March 30th in the eastern side and on May 3rd in the western sub-regions, reaching its peak between May 10th and June 19th, respectively. The spring bloom lasts between 39 and 45 days in most sub-regions. However, the bloom lasts for 58 days in the sub-region 10 in the southern Norwegian Sea, which is the longest spring bloom in the study region. The spring bloom intensity varies from 1.1 to 1.4 mg m–3 in the Norwegian Sea. In the Barents Sea (sub-regions 1–4), the spring bloom starts between April 11th and 27th and ends between May 7th and 28th, lasting for up to 33 days, which is the shortest spring bloom of the study region. Nevertheless, the Barents Sea shows the strongest intensity of the spring blooms that vary on average from 1.8 to 2.7 mg m–3.
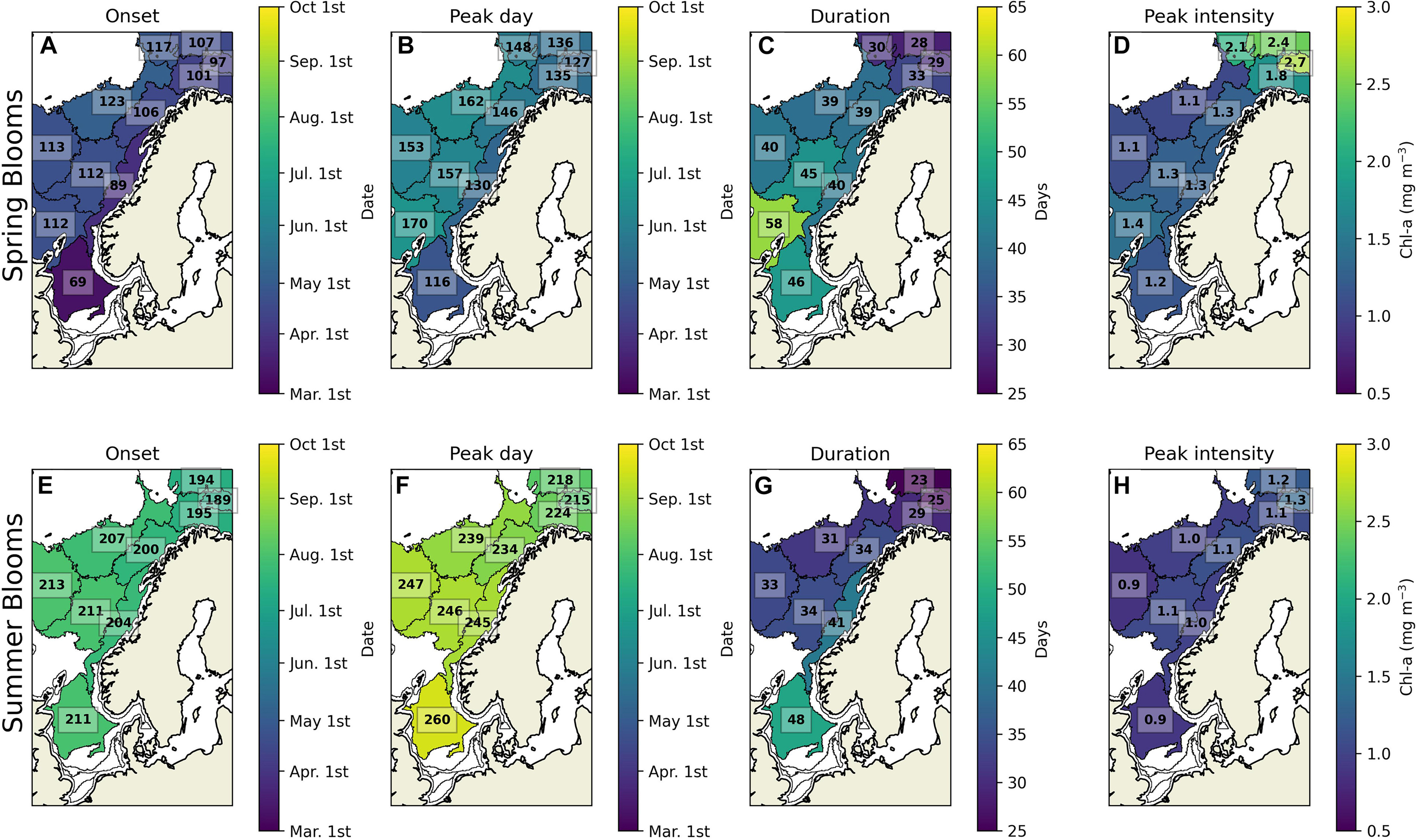
Figure 4. Climatology of spring and summer blooms phenology. The subplots are the spring bloom (A) onset, (B) peak day, (C) duration, and (D) intensity; the summer blooms (E) onset, (F) peak day, (G) duration, and (H) intensity. The numbers in the onset and peak day are Julian days; in the duration are in days; in the peak intensity are in Chl-a (mg m–3). Sub-regions where quantity is untrustworthy are masked in white.
The timing and intensity of summer blooms have lower spatial discrepancies than for the spring blooms. The summer bloom begins on average from July 8th in the Barents Sea to August 1st in the Norwegian Sea and it ends from August 3rd in the Barents Sea to September 17th in the North Sea. The blooms last from 23 days in the Barents Sea to 48 days in the North Sea. Finally, the summer blooms intensity varies between 0.9 and 1.2 mg m–3.
Bloom Phenology Trends
The spring bloom timing does not significantly change in the North and the Norwegian seas over the 21 years study period, but the spring bloom onset advances and the duration increases in the Barents Sea (Figure 5 and Supplementary Figure 2). In sub-regions 1 and 3, the blooms onset advances by −0.74-day year–1, and in sub-regions 3 and 4, the blooms duration increases by 0.49-day year–1. Furthermore, the intensity of spring blooms increases in the Norwegian Sea (Supplementary Figure 3) in sub-regions 5 and 7, with a rate of 0.02 and 0.01 mg m–3 year–1, respectively.
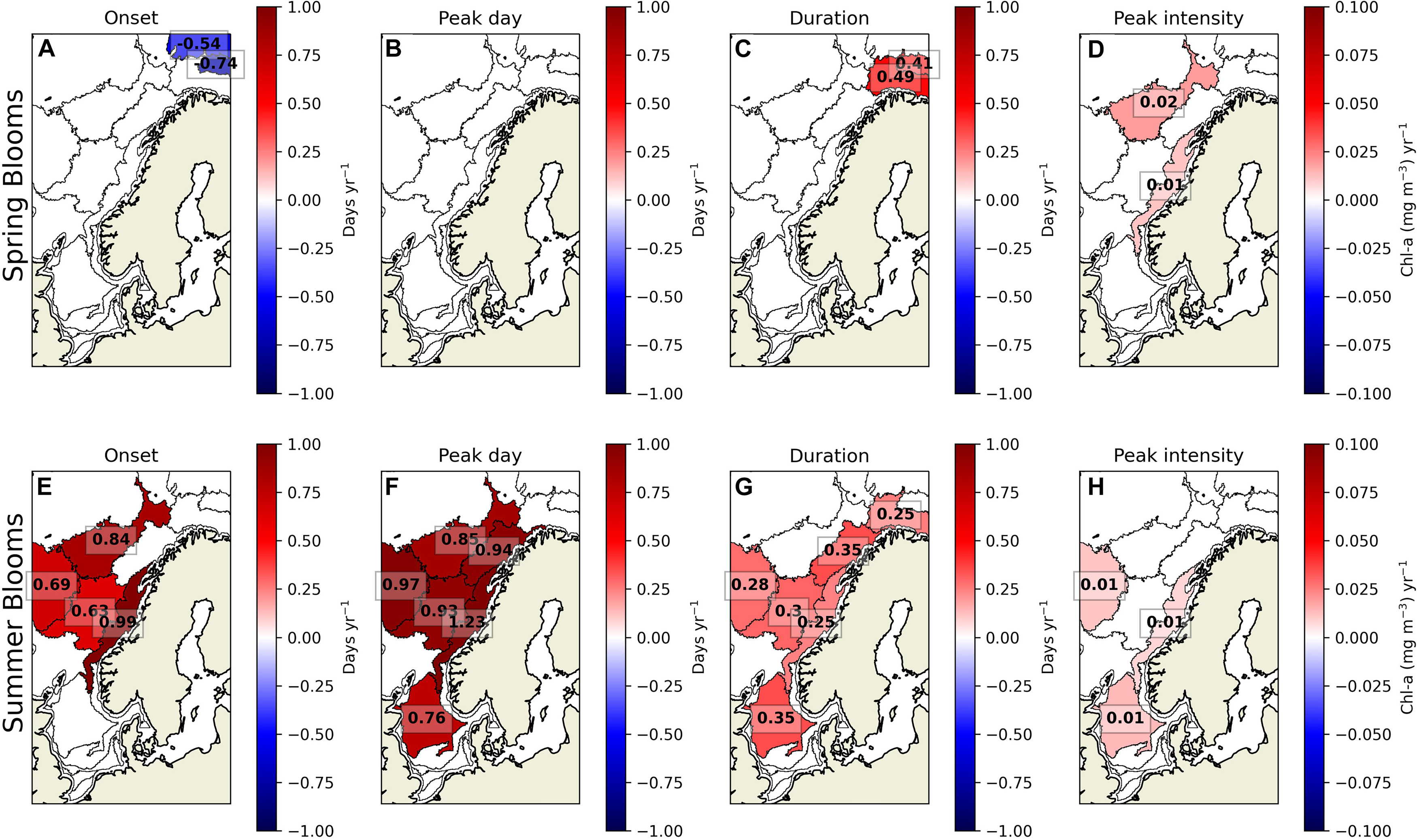
Figure 5. The trends of spring and summer blooms phenology from 2000 to 2020. The subplots are the spring bloom (A) onset, (B) peak day, (C) duration, and (D) intensity; the summer blooms (E) onset, (F) peak day, (G) duration, and (H) intensity. Sub-regions where trends are insignificant (α > 0.05) are masked in white.
The summer blooms significantly change from the North Sea and the Barents Sea. The summer blooms onset and peak day is delayed by 1.25-day year–1 in sub-regions 5, 6, 7, 8, 9, and 11. The duration of the blooms also increases at a rate up to 0.35-day year–1 in sub-regions 4, 6, 7, 8, 9, and 12. Last, the intensity of blooms increases in sub-regions 7, 9, and 12 with a rate of 0.025 mg m–3 year–1.
Interannual Detrended Correlation
The MLD interannual variability correlates with the spring bloom timing and intensity in the North, Norwegian, and Barents seas (Figure 6). The correlation varies between 0.51 and 0.74 with the bloom onset and peak day and between −0.54 and −0.62 with the intensity. Thus, despite a study arguing that the spring bloom is not triggered by the shallowing of the ML and it starts in winter when the MLD is maximum (Behrenfeld, 2010), our results show that MLD seems to regulate the timing of spring blooms. The only exceptions are in sub-regions 2, 7, and 10, where MLD does not correlate with the spring bloom phenology.
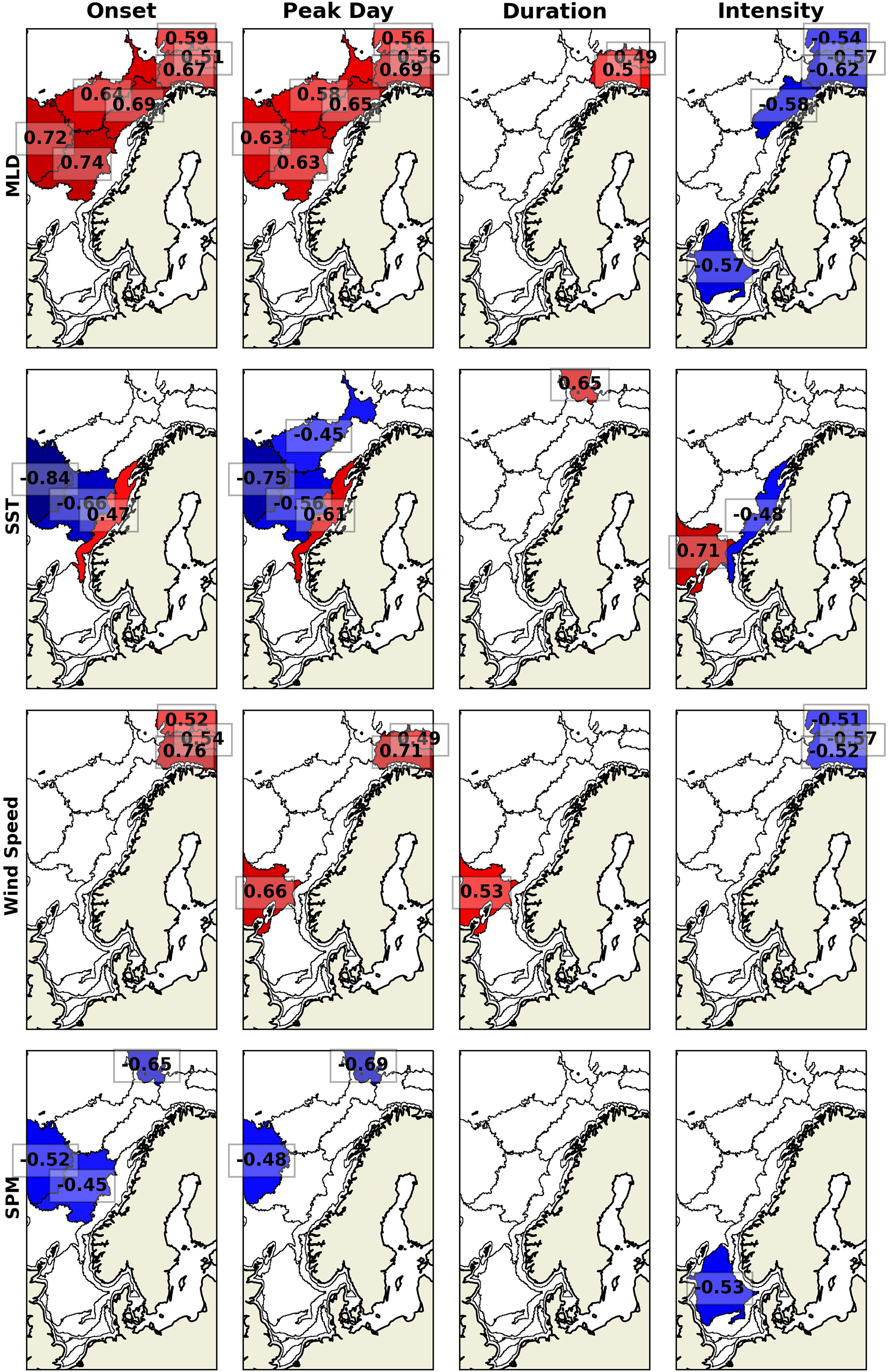
Figure 6. Detrended interannual correlation between the spring bloom phenology and MLD, SST, wind speed, and SPM. Red colors are positive correlations, blue colors are negative correlations and white colors are used for insignificant correlations (α > 0.05).
Sea surface temperature correlates with the spring blooms timing and intensity in the Norwegian Sea but with inverse relationships depending on the region. On one hand, warmer waters are correlated with later and weaker spring blooms in sub-region 7. On the other hand, warmer waters are correlated with earlier spring blooms in sub-regions 5, 8, and 9, and stronger spring blooms in sub-region 10. Wind speed and SPM are correlated with the spring blooms just in a few regions. Wind speed is significantly correlated with the Barents Sea spring bloom timing and intensity, where weaker winds relate to earlier onsets and peak days and more intense blooms. SPM is correlated to spring bloom timing in sub-regions 2, 8, and 9, and with the intensity only in the North Sea, where low SPM concentrations relate to stronger spring blooms.
The summer bloom correlates with physical and biogeochemical parameters only in a few sub-regions (Figure 7). Shallower MLD correlates with the earlier summer blooms onset and peak day in sub-regions 8 and 9. Warmer SST correlates with later and longer summer blooms in sub-regions 6, 7, 8, and 12, and with less intense blooms in sub-regions 3 and 8. Last, wind speed only correlates with summer blooms intensity in sub-region 6, and SPM only correlates with summer blooms intensity in sub-regions 4, 5, and 6.
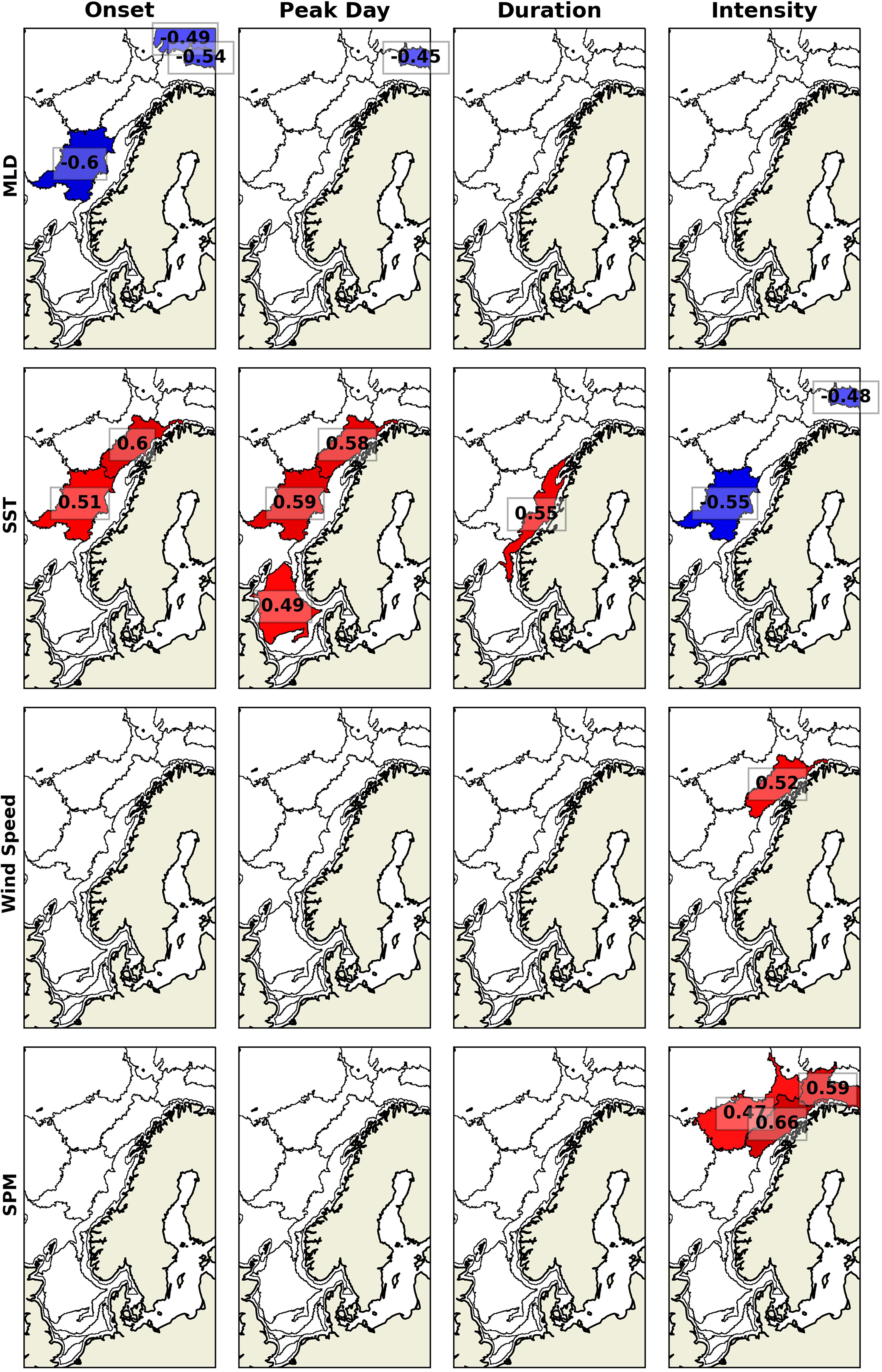
Figure 7. Detrended interannual correlation between the summer bloom phenology and MLD, SST, wind speed, and SPM. Red colors are positive correlations, blue colors are negative correlations and white colors are used for insignificant correlations (α > 0.05).
It should be noted that other remotely sensed and modeled parameters have been considered but were not presented to keep the paper concise. For spring bloom, photosynthetically available radiation (PAR) has significant correlations in sub-region 4 in a similar manner to MLD–albeit weaker. For summer blooms, the correlations with PAR are at the edge of significance and somewhat counter-intuitive–with higher PAR linked to shorter and weaker summer blooms. Euphotic depth (Zeu) and light attenuation coefficient (Kd) were also considered. These are inherently related to the algae blooms–i.e., Zeu and Kd change the blooms (Sverdrup, 1953) at the same time the Chl-a change the Zeu and Kd (Kirk, 2011). Thus, we retrieved high correlations. However, the correlations showed that higher biomass blooms are correlated to higher Kd and lower Zeu, meaning that the blooms probably control the interannual variability of Kd and Zeu during the bloom rather than the contrary. For this reason, PAR, Kd, and Zeu are not presented in this study.
Discussion
Summer Bloom Delay
In the trends analysis, it appears that summer blooms are getting delayed from 2000 to 2020. However, the possible causes of this delay are unclear to us. The spring bloom exhausts the nutrients on the surface, and associated with other factors such as zooplankton grazing, leads to the ending of spring bloom. In the poor nutrient waters of summer, the increase of MLD results in an upwell of nutrients required for a summer or autumn bloom (Glen Harrison et al., 2013; Wihsgott et al., 2019). Therefore, we would expect that changes in the summer bloom phenology are related to the MLD. For example, Martinez et al. (2011) suggested that a possible cause for the weaker autumn bloom in the North Atlantic (30–50°N) was a delayed increase of MLD during the autumn of the 2000s. We computed the MLD trend during the summer bloom and down to 4 weeks before the onset. Our results showed that there had been no significant trend in the MLD from 2000 to 2020. Besides, we also computed the trends of SST, SPM and wind speed, and none showed a significant trend.
The lack of trends in MLD, SST, SPM and wind speed suggests that other factors that are not assessed in this study may be influencing the delay of summer blooms. For example, bio-advection of phytoplankton and zooplankton pressure. A recent study showed that bio-advection caused by a faster intrusion of the North Atlantic Current is causing the phytoplankton Emiliania huxleyi to increase in the Barents Sea (Oziel et al., 2020). In the North Atlantic, the predominant zooplankton species has been changing (Beaugrand, 2002), and the new species biomass increases during autumn (Planque and Fromentin, 1996). Martinez et al. (2011) suggested that the new predominant zooplankton species increased the grazing pressure in the autumn, probably leading to a weaker bloom in the 2000s. Likewise, the delay of summer blooms found in this study could be related to the bio-advection of phytoplankton or changes in the zooplankton community structure. Still, no firm conclusion can be held yet on the reason of the delayed summer blooms. This will be the topic of a follow-up study.
Barents Sea: Stronger, Earlier and Shorter Spring Blooms Driven by Shallower Mixed Layer
The intensity of spring blooms in the Barents Sea (sub-regions 1, 3, and 4) showed the highest interannual variability of the study area (Supplementary Figure 3). For example, varying from 1.2 mg m–3 in 2008 to 4.1 mg m–3 in 2002 in sub-region 3. Besides, the intensity of spring blooms is negatively correlated with the onset, peak day and duration (R < −0.44), meaning that the spring blooms in the Barents Sea are typically either early, short and strong or late, long and weak.
Two dynamical processes contribute to the nutrient loads in the surface waters of the southern and central Barents Sea: the lateral inflow of Atlantic waters that come from NwAC and the vertical mixing when the ML is deeper during winter (Wassmann et al., 2006). In March, the deep ML makes the phosphate, nitrate and silicate evenly distributed in the water column, reaching 0.85, 11.2, and 4.5 μM, respectively (Reigstad et al., 2002). It was shown that springs with a deeper ML are correlated with higher nitrate concentrations (Olsen et al., 2003). However, deep ML and high nitrate concentrations do not necessarily lead to stronger spring blooms. Our results suggest that a shallower ML is correlated to stronger spring blooms. Stratified waters reduce the phytoplankton sinking below the euphotic zone, which may be critical in the Barents Sea, where one of the predominant phytoplankton taxa is Diatom that quickly sinks due to its dense cell walls of silica (Degerlund and Eilertsen, 2010). The high concentration of nutrients available at the beginning of the bloom in March (Reigstad et al., 2002) could be sufficient for developing the spring blooms, while interannual variability of MLD would control the intensity and the timing of the blooms. Nevertheless, a reduced level of nutrients available in years of shallower ML can explain why stronger spring blooms end sooner.
The seasonal ML shallowing during spring is driven by the SST increase from solar radiation and the freshening of water coming from sea ice melting, interactions with the fresher fjord water systems and inflow of the NCC (Drinkwater et al., 2003; Olsen et al., 2003; Loeng and Drinkwater, 2007). In the sub-regions 1, 3, and 4, we found that the interannual variability of MLD is significantly correlated with wind speed (R = 0.58) and freshwaters discharge of Tana River (R = −0.65) during the bloom. This indicates that calm waters and high discharge of freshwaters may lead to shallower ML, resulting in stronger spring blooms. This also explains why weaker winds correlate with earlier and stronger spring blooms in the Barents Sea.
Mixed Layer Depth and Sea Surface Temperature Influence on the Spring Bloom Phenology in the Norwegian Sea
Surface heating from solar radiation and freshening of the coastal waters leads to the shallowing ML during the spring in the Norwegian Sea, and the spring bloom starts when the MLD reaches a lower depth than the Sverdrup critical depth (Sverdrup, 1953). The MLD has been shown to influence the spatial variability of spring blooms onset (Naustvoll et al., 2020). Our results show that MLD is also a possible driver of the interannual variability of bloom onset and peak day. The positive correlation between MLD and the spring blooms onset and peak day in the sub-regions 5, 6, 8, and 9 (R > 0.58) suggests that a shallower ML favor earlier spring blooms. In sub-regions 5, 8, and 9, interannual SST is correlated with MLD (R < −0.46), and consequently, SST is also correlated with the spring bloom timing. In sub-region 6, only the Målselva River inflow has a significant correlation with MLD (R = −0.55). Therefore, warmer years during the spring could lead to shallower ML and earlier blooms from the middle to the northwestern Norwegian Sea, while the spring bloom in sub-region 6 behaves similarly to spring blooms in the Barents Sea.
The SST is correlated with the spring blooms in sub-regions 7 and 10 without the influence of MLD. In both sub-regions, MLD has not correlated with SST and the spring blooms as observed in the remaining Norwegian Sea. Besides, the spring bloom intensity of both sub-regions shows an inverse relationship with SST. Warmer waters are correlated with stronger blooms in sub-region 10, whereas colder waters are correlated with earlier and stronger blooms in sub-region 7. Without the influence of SST on MLD interannual variability, two hypotheses could explain why warmer waters are correlated with stronger spring blooms in sub-region 10. First, warmer waters increase the growth rate of phytoplankton (Eppley, 1972; Moisan et al., 2002; Bissinger et al., 2008), and during the spring bloom, a higher growth rate could support the bloom to reach higher Chl-a biomass. Second, warmer waters could indicate a higher intrusion of Atlantic waters from the North Atlantic Current. If Atlantic waters are associated with the input of nutrients in sub-region 10, more nutrients could lead the spring bloom to higher Chl-a biomass.
Regarding sub-region 7, warm Atlantic waters trapped in mesoscale anticyclonic eddies were found to delay the spring blooms in the Norwegian Shelf (Hansen et al., 2010). However, the spring bloom delay in the eddy was probably caused by the delayed shallowing of the ML, and our results have not shown a relationship between SST and MLD in sub-region 7. Thus, we have not found a plausible explanation why colder waters could relate to earlier and stronger spring blooms in this region.
Shallower Mixed Layer Drives Clearer Waters and Stronger Spring Blooms in the North Sea
The average spring bloom intensity in the sub-region 12 ranges from 0.9 to 1.5 mg m–3, and it is significant correlated with MLD and SPM. A shallower ML reduces the phytoplankton sinking to deeper waters, and a lower SPM concentration increases the euphotic zone and the light available to the algae photosynthesis. Moreover, we also found that SPM is correlated with MLD during the spring bloom (R = −0.44). A previous study showed that surface SPM variability in the North Sea is correlated with the bed shear stress caused by oceanic waves, but only for months with a deep ML (Wilson and Heath, 2019). In March, deep ML allows the bed shear stress to increase the surface SPM concentration (R2 = 0.47). In April, the MLD decreases and the bead shear stress is no longer correlated with the surface SPM variability. The climatological spring bloom in sub-region 12 starts on March 10th and ends on April 26th, and this implies that the relation between MLD, bed shear stress and SPM are strongly correlated for almost half of the bloom period. Therefore, the compound influence of SPM and MLD was found to explain the intensity of spring blooms. Years of shallower ML reduces the phytoplankton sinking and could lead to more transparent waters, resulting in more sunlight available to photosynthesis and, hence, stronger spring blooms.
Conclusion
This study presents an exhaustive analysis of the regional spring and summer blooms phenology for a region that extends from the Barents, Norwegian to the North seas using an extended and novel clustering analysis. The regional climatology and trend of the phytoplankton blooms phenology, as well as a primary analysis of the co-variability of potential drivers with interannual variability, are presented and discussed. In the Barents Sea, we found that low wind amplitude and increased freshening lead to springs with shallower ML, and more stratified waters are related to spring blooms starting earlier and reaching higher biomass during the peak. In the Norwegian Sea, we found that SST has an opposed correlation with spring blooms. There, warmer waters are correlated with earlier and higher biomass blooms from the north of Scotland to the off-shore central parts of the Norwegian Sea, but lower temperatures are correlated with earlier and higher biomass blooms in the eastern Norwegian Sea. In the North Sea, springs with shallower ML limits the SPM increase by bottom shear stress, favoring an environment of more stratified and clearer waters, correlating well with blooms of higher biomass. Last, we also found a rapid delay in the onset and increased biomass of the summer blooms during the past 21 years in almost the entire study region. The summer blooms are starting later, ending later, getting longer and reaching higher Chl-a concentrations.
Data Availability Statement
Publicly available datasets were analyzed in this study. This data can be found here: OC:CCI: https://www.oceancolour.org/, CMEMS: https://marine.copernicus.eu/, GlobColour: https://www.globcolour.info/, and NMDC: https://nmdc.no/.
Author Contributions
ES, FC, JB, AK, and LP contributed to the conception and design of the study. ES organized the database, performed the statistical analysis, and wrote the first draft of the manuscript. ES and FC performed the data processing. FC and JB supervised the data processing and statistical analysis. All authors contributed to the interpretation of the results of this work, manuscript revision, read, and approved the submitted version.
Funding
ES is a holder of an institute research fellowship (INSTSTIP) funded by the basic institutional funding through Norwegian Research Council (#318085). FC acknowledges the Trond Mohn Foundation under project number: BFS2018TMT01. JB acknowledges the NFR Climate Futures (309562). NK acknowledges the Nansen Legacy project (276730). This work has also received a grant for computer time from the Norwegian Program for super computing (NOTUR2, project number nn9039k) and a storage grant (NORSTORE, NS9039k).
Conflict of Interest
The authors declare that the research was conducted in the absence of any commercial or financial relationships that could be construed as a potential conflict of interest.
Publisher’s Note
All claims expressed in this article are solely those of the authors and do not necessarily represent those of their affiliated organizations, or those of the publisher, the editors and the reviewers. Any product that may be evaluated in this article, or claim that may be made by its manufacturer, is not guaranteed or endorsed by the publisher.
Acknowledgments
We want to thank Jiping Xie for making the TOPAZ4 MLD data available to us. We also want to thank the Norwegian Institute of Marine Research for making the in situ Chl-a data available. JB is member of Sorbonne University as associate professor.
Supplementary Material
The Supplementary Material for this article can be found online at: https://www.frontiersin.org/articles/10.3389/fmars.2021.746327/full#supplementary-material
Footnotes
- ^ https://marine.copernicus.eu/
- ^ https://www.globcolour.info/
- ^ https://www.nve.no/
- ^ https://nmdc.no/
References
Arthur, D., and Vassilvitskii, S. (2007). “k-means++: the advantages of careful seeding,” in Proceedings of the Eighteenth Annual ACM-SIAM Symposium on Discrete Algorithms, SODA, (New Orleans), 11.
Asch, R. G., Stock, C. A., and Sarmiento, J. L. (2019). Climate change impacts on mismatches between phytoplankton blooms and fish spawning phenology. Glob. Chang. Biol. 25, 2544–2559. doi: 10.1111/gcb.14650
Bailey, S. W., and Werdell, P. J. (2006). A multi-sensor approach for the on-orbit validation of ocean color satellite data products. Remote Sens. Environ. 102, 12–23. doi: 10.1016/j.rse.2006.01.015
Beaugrand, G. (2002). Reorganization of North Atlantic marine copepod biodiversity and climate. Science (80-.). 296, 1692–1694. doi: 10.1126/science.1071329
Behrenfeld, M. J. (2010). Abandoning sverdrup’s critical depth hypothesis on phytoplankton blooms. Ecology 91, 977–989. doi: 10.1890/09-1207.1
Bissinger, J. E., Montagnes, D. J. S., Sharples, J., and Atkinson, D. (2008). Predicting marine phytoplankton maximum growth rates from temperature: improving on the Eppley curve using quantile regression. Limnol. Oceanogr. 53, 487–493. doi: 10.4319/lo.2008.53.2.0487
Bleck, R. (2002). An oceanic general circulation model framed in hybrid isopycnic-Cartesian coordinates. Ocean Model. 4, 55–88. doi: 10.1016/S1463-5003(01)00012-9
Blondeau-Patissier, D., Tilstone, G. H., Martinez-Vicente, V., and Moore, G. F. (2004). Comparison of bio-physical marine products from SeaWiFS, MODIS and a bio-optical model with in situ measurements from Northern European waters. J. Opt. A Pure Appl. Opt. 6, 875–889. doi: 10.1088/1464-4258/6/9/010
Brody, S. R., Lozier, M. S., and Dunne, J. P. (2013). A comparison of methods to determine phytoplankton bloom initiation. J. Geophys. Res. Ocean. 118, 2345–2357. doi: 10.1002/jgrc.20167
Calton, B. (2020). ESA Ocean Colour Climate Change Initiative – Phase 3 Product User Guide for v5. 0 Dataset. Paris: ESA.
Chang, F. H., Anderson, C., and Boustead, N. C. (1990). First record of a Heterosigma (Raphidophyceae) bloom with associated mortality of cage-reared salmon in Big Glory Bay, New Zealand. New Zeal. J. Mar. Freshw. Res. 24, 461–469. doi: 10.1080/00288330.1990.9516437
De Cauwer, V., Ruddick, K., Park, Y.-J., Nechad, B., and Kyramarios, M. (2004). Optical Remote Sensing in Support of Eutrophication Monitoring in the Southern North Sea. European Association of Remote Sensing Laboratories (EARSeL), 208–221.
Degerlund, M., and Eilertsen, H. C. (2010). Main species characteristics of Phytoplankton spring blooms in NE Atlantic and Arctic Waters (68–80° N). Estuaries Coasts 33, 242–269. doi: 10.1007/s12237-009-9167-7
Drinkwater, K. F., Belgrano, A., Borja, A., Conversi, A., Edwards, M., Greene, C. H., et al. (2003). “The response of marine ecosystems to climate variability associated with the north atlantic oscillation,” in The North Atlantic Oscillation: Climatic Significance and Environmental Impact, eds J. W. Hurrell, Y. Kushnir, G. Ottersen, and M. Visbeck (Washington, DC: American Geophysical Union), 211–234.
Eisma, D., Johnston, R., and Cadogan, J. I. G. (1987). The North Sea: an overview. Philos. Trans. R. Soc. London. B, Biol. Sci. 316, 461–485. doi: 10.1098/rstb.1987.0032
Eldevik, T., Nilsen, J. E., Iovino, D., Anders Olsson, K., Sandø, A. B., and Drange, H. (2009). Observed sources and variability of Nordic seas overflow. Nat. Geosci. 2, 406–410. doi: 10.1038/ngeo518
Evensen, G. (2003). The Ensemble Kalman Filter: theoretical formulation and practical implementation. Ocean Dyn. 53, 343–367. doi: 10.1007/s10236-003-0036-9
Ferreira, A. S. A., Hátún, H., Counillon, F., Payne, M. R., and Visser, A. W. (2015). Synoptic-scale analysis of mechanisms driving surface chlorophyll dynamics in the North Atlantic. Biogeosciences 12, 3641–3653. doi: 10.5194/bg-12-3641-2015
Folkestad, A., Pettersson, L. H., and Durand, D. D. (2007). Inter-comparison of ocean colour data products during algal blooms in the Skagerrak. Int. J. Remote Sens. 28, 569–592. doi: 10.1080/01431160600821044
Furevik, T., Bentsen, M., Drange, H., Johannessen, J. A., and Korablev, A. (2002). Temporal and spatial variability of the sea surface salinity in the Nordic Seas. J. Geophys. Res. Ocean. 107, 1–16. doi: 10.1029/2001jc001118
Giannini, F., Hunt, B. P. V., Jacoby, D., and Costa, M. (2021). Performance of OLCI Sentinel-3A satellite in the Northeast Pacific coastal waters. Remote Sens. Environ. 256:112317. doi: 10.1016/j.rse.2021.112317
Glen Harrison, W., Yngve Børsheim, K., Li, W. K. W., Maillet, G. L., Pepin, P., Sakshaug, E., et al. (2013). Phytoplankton production and growth regulation in the Subarctic North Atlantic: a comparative study of the Labrador Sea-Labrador/Newfoundland shelves and Barents/Norwegian/Greenland seas and shelves. Prog. Oceanogr. 114, 26–45. doi: 10.1016/j.pocean.2013.05.003
Gobler, C. J. (2020). Climate change and harmful algal blooms: insights and perspective. Harmful Algae 91:101731. doi: 10.1016/j.hal.2019.101731
Gohin, F. (2011). Annual cycles of chlorophyll-a, non-algal suspended particulate matter, and turbidity observed from space and in-situ in coastal waters. Ocean Sci. 7, 705–732. doi: 10.5194/os-7-705-2011
Good, S., Fiedler, E., Mao, C., Martin, M. J., Maycock, A., Reid, R., et al. (2020). The current configuration of the OSTIA system for operational production of foundation sea surface temperature and ice concentration analyses. Remote Sens. 12, 1–20. doi: 10.3390/rs12040720
Hansen, C., Kvaleberg, E., and Samuelsen, A. (2010). Anticyclonic eddies in the Norwegian Sea; their generation, evolution and impact on primary production. Deep Sea Res. Part I Oceanogr. Res. Pap. 57, 1079–1091. doi: 10.1016/j.dsr.2010.05.013
Harrison, P. J., Piontkovski, S., and Al-Hashmi, K. (2017). Understanding how physical-biological coupling influences harmful algal blooms, low oxygen and fish kills in the Sea of Oman and the Western Arabian Sea. Mar. Pollut. Bull. 114, 25–34. doi: 10.1016/j.marpolbul.2016.11.008
Holm-Hansen, O., and Riemann, B. (1978). Chlorophyll a Determination: improvements in Methodology. Oikos 30:438. doi: 10.2307/3543338
Hunke, E. C., and Dukowicz, J. K. (1997). An Elastic–Viscous–Plastic model for sea ice dynamics. J. Phys. Oceanogr. 27, 1849–1867. doi: 10.1175/1520-04851997027<1849:AEVPMF<2.0.CO;2
Kaartvedt, S., Johnsen, T. M., Aksnes, D. L., Lie, U., and Svendsen, H. (1991). Occurrence of the toxic phytoflagellate Prymnesium parvum and associated fish mortality in a Norwegian fjord system. Can. J. Fish. Aquat. Sci. 48, 2316–2323. doi: 10.1139/f91-272
Kent, M., Whyte, J., and LaTrace, C. (1995). Gill lesions and mortality in seawater pen-reared Atlantic salmon Salmo salar associated with a dense bloom of Skeletonema costatum and Thalassiosira species. Dis. Aquat. Organ. 22, 77–81. doi: 10.3354/dao022077
Kirk, J. T. O. (2011). Light and Photosynthesis in Aquatic Ecosystems. Cambridge: Cambridge University Press.
Landsberg, J. H., Hendrickson, J., Tabuchi, M., Kiryu, Y., Williams, B. J., and Tomlinson, M. C. (2020). A large-scale sustained fish kill in the St. Johns River, Florida: a complex consequence of cyanobacteria blooms. Harmful Algae 92:101771. doi: 10.1016/j.hal.2020.101771
Leblanc, K., Quéguiner, B., Diaz, F., Cornet, V., Michel-Rodriguez, M., Durrieu de Madron, X., et al. (2018). Nanoplanktonic diatoms are globally overlooked but play a role in spring blooms and carbon export. Nat. Commun. 9:953. doi: 10.1038/s41467-018-03376-9
Legendre, L. (1990). The significance of microalgal blooms for fisheries and for the export of particulate organic carbon in oceans. J. Plankton Res. 12, 681–699. doi: 10.1093/plankt/12.4.681
Loeng, H., and Drinkwater, K. (2007). An overview of the ecosystems of the Barents and Norwegian Seas and their response to climate variability. Deep. Res. Part II Top. Stud. Oceanogr. 54, 2478–2500. doi: 10.1016/j.dsr2.2007.08.013
MacQueen, J. (1967). “Some methods for classification and analysis of multivariate observations,” in Proceeding of the Fifth Berkeley Symposium (Berkeley), 281–297.
Martinez, E., Antoine, D., D’Ortenzio, F., and De Boyer Montégut, C. (2011). Phytoplankton spring and fall blooms in the North Atlantic in the 1980s and 2000s. J. Geophys. Res. Ocean. 116, 1–11. doi: 10.1029/2010JC006836
Merchant, C. J., Embury, O., Bulgin, C. E., Block, T., Corlett, G. K., Fiedler, E., et al. (2019). Satellite-based time-series of sea-surface temperature since 1981 for climate applications. Sci. Data 6, 1–18. doi: 10.1038/s41597-019-0236-x
Mohd-Din, M., Abdul-Wahab, F., Mohamad, S. E., Jamaluddin, H., Shahir, S., Ibrahim, Z., et al. (2020). Prolonged high biomass diatom blooms induced formation of hypoxic-anoxic zones in the inner part of Johor Strait. Environ. Sci. Pollut. Res. 27, 42948–42959. doi: 10.1007/s11356-020-10184-6
Moisan, J. R., Moisan, T. A., and Abbott, M. R. (2002). Modelling the effect of temperature on the maximum growth rates of phytoplankton populations. Ecol. Modell. 153, 197–215. doi: 10.1016/S0304-3800(02)00008-X
Morel, A., and Prieur, L. (1977). Analysis of variations in ocean color1. Limnol. Oceanogr. 22, 709–722. doi: 10.4319/lo.1977.22.4.0709
Mork, M. (1981). Circulation phenomena and frontal dynamics of the Norwegian coastal current. Philos. Trans. R. Soc. London. Ser. A, Math. Phys. Sci. 302, 635–647. doi: 10.1098/rsta.1981.0188
Naustvoll, L. J., Melle, W., Klevjer, T., Drinkwater, K. F., Strand, E., and Knutsen, T. (2020). Dynamics of phytoplankton species composition, biomass and nutrients in the North Atlantic during spring and summer - A trans-Atlantic study. Deep Sea Res. Part II Top. Stud. Oceanogr. 180:104890. doi: 10.1016/j.dsr2.2020.104890
Neveux, J., Delmas, D., Romano, J., Algarra, P., Ignatiades, L., Herbland, A., et al. (1990). Comparison of chlorophyll and phaeopigment determinations by spectrophotometric, fluorometric, spectrofluorometric and HPLC methods. Mar. Microb. Food Webs 4, 217–238.
Olsen, A., Johannessen, T., and Rey, F. (2003). On the nature of the factors that control spring bloom development at the entrance to the Barents Sea and their interannual variability. Sarsia 88, 379–393. doi: 10.1080/00364820310003145
Opdal, A. F., Lindemann, C., and Aksnes, D. L. (2019). Centennial decline in North Sea water clarity causes strong delay in phytoplankton bloom timing. Glob. Chang. Biol. 25, 3946–3953. doi: 10.1111/gcb.14810
Oziel, L., Baudena, A., Ardyna, M., Massicotte, P., Randelhoff, A., Sallée, J. B., et al. (2020). Faster Atlantic currents drive poleward expansion of temperate phytoplankton in the Arctic Ocean. Nat. Commun. 11, 1–8. doi: 10.1038/s41467-020-15485-5
Perry, R. K. (1986). “Bathymetry,” in The Nordic Seas, ed. B. G. Hurdle (New York, NY: Springer New York), 211–236.
Petrenko, D., Pozdnyakov, D., Johannessen, J., Counillon, F., and Sychov, V. (2013). Satellite-derived multi-year trend in primary production in the Arctic Ocean. Int. J. Remote Sens. 34, 3903–3937. doi: 10.1080/01431161.2012.762698
Pettersson, L. H., and Pozdnyakov, D. (2013). Monitoring of Harmful Algal Blooms. Berlin: Springer Berlin Heidelberg.
Planque, B., and Fromentin, J. (1996). Calanus and environment in the eastern North Atlantic. I. Spatial and temporal patterns of C. finmarchicus and C. helgolandicus. Mar. Ecol. Prog. Ser. 134, 101–109. doi: 10.3354/meps134101
Platt, T., Fuentes-Yaco, C., and Frank, K. T. (2003). Spring algal bloom and larval fish survival off Nova Scotia. Nature 423, 398–399.
Pozdnyakov, D. V., Pettersson, L. H., and Korosov, A. A. (2017). Exploring the Marine Ecology From Space. Cham: Springer International Publishing.
Ramsay, J. O., and Silverman, B. W. (2005). Functional Data Analysis. New York, NY: Springer New York.
Reigstad, M., Wassmann, P., Wexels Riser, C., Øygarden, S., and Rey, F. (2002). Variations in hydrography, nutrients and chlorophyll a in the marginal ice-zone and the central Barents Sea. J. Mar. Syst. 38, 9–29. doi: 10.1016/S0924-7963(02)00167-7
Reynolds, R. A., Stramski, D., and Mitchell, B. G. (2001). A chlorophyll-dependent semianalytical reflectance model derived from field measurements of absorption and backscattering coefficients within the Southern Ocean. J. Geophys. Res. Ocean. 106, 7125–7138. doi: 10.1029/1999JC000311
Rousseeuw, P. J. (1987). Silhouettes: a graphical aid to the interpretation and validation of cluster analysis. J. Comput. Appl. Math. 20, 53–65. doi: 10.1016/0377-0427(87)90125-7
Sakov, P., Counillon, F., Bertino, L., Lisæter, K. A., Oke, P. R., and Korablev, A. (2012). TOPAZ4: an ocean-sea ice data assimilation system for the North Atlantic and Arctic. Ocean Sci. 8, 633–656. doi: 10.5194/os-8-633-2012
Sathyendranath, S., Brewin, R. J. W., Brockmann, C., Brotas, V., Calton, B., Chuprin, A., et al. (2019). An ocean-colour time series for use in climate studies: the experience of the ocean-colour climate change initiative (OC-CCI). Sensors (Switzerland) 19:4285. doi: 10.3390/s19194285
Student. (1908). Probable error of a Correlation Coefficient. Biometrika 6, 302–310. doi: 10.2307/2331474
Sundby, S., Drinkwater, K. F., and Kjesbu, O. S. (2016). The North Atlantic spring-bloom system-where the changing climate meets the winter dark. Front. Mar. Sci. 3:28. doi: 10.3389/fmars.2016.00028
Sverdrup, H. U. (1953). On conditions for the vernal blooming of phytoplankton. ICES J. Mar. Sci. 18, 287–295. doi: 10.1093/icesjms/18.3.287
Tangen, K. (1977). Blooms of gyrodinium aureolum (dinophygeae) in north european waters, accompanied by mortality in marine organisms. Sarsia 63, 123–133. doi: 10.1080/00364827.1977.10411330
Tilstone, G. H., Peters, S. W. M., van der Woerd, H. J., Eleveld, M. A., Ruddick, K., Schönfeld, W., et al. (2012). Variability in specific-absorption properties and their use in a semi-analytical ocean colour algorithm for MERIS in North Sea and Western English Channel Coastal Waters. Remote Sens. Environ. 118, 320–338. doi: 10.1016/j.rse.2011.11.019
Townsend, D. W., and Cammen, L. M. (1987). Potential importance of the timing of spring plankton blooms to benthic-pelagic coupling and recruitment of juvenile demersal fishes. Biol. Oceanogr. 5, 215–228. doi: 10.1080/01965581.1987.10749514
Vikebø, F. B., Korosov, A., Stenevik, E. K., Husebø, A., and Slotte, A. (2012). Spatio-temporal overlap of hatching in Norwegian spring-spawning herring and the spring phytoplankton bloom at available spawning substrata. ICES J. Mar. Sci. 69, 1298–1302. doi: 10.1038/278097a0
Vikebø, F. B., Strand, K. O., and Sundby, S. (2019). Wind intensity is key to phytoplankton spring bloom under climate change. Front. Mar. Sci. 6:518. doi: 10.3389/fmars.2019.00518
Wassmann, P., Reigstad, M., Haug, T., Rudels, B., Carroll, M. L., Hop, H., et al. (2006). Food webs and carbon flux in the Barents Sea. Prog. Oceanogr. 71, 232–287. doi: 10.1016/j.pocean.2006.10.003
Wihsgott, J. U., Sharples, J., Hopkins, J. E., Woodward, E. M. S., Hull, T., Greenwood, N., et al. (2019). Observations of vertical mixing in autumn and its effect on the autumn phytoplankton bloom. Prog. Oceanogr. 177:102059. doi: 10.1016/j.pocean.2019.01.001
Wilson, R. J., and Heath, M. R. (2019). Increasing turbidity in the North Sea during the 20th century due to changing wave climate. Ocean Sci. 15, 1615–1625. doi: 10.5194/os-15-1615-2019
Xie, J., Bertino, L., Counillon, F., Lisæter, K. A., and Sakov, P. (2017). Quality assessment of the TOPAZ4 reanalysis in the Arctic over the period 1991–2013. Ocean Sci. 13, 123–144. doi: 10.5194/os-13-123-2017
Keywords: phytoplankton, bloom phenology, Barents Sea, Norwegian Sea, North Sea, remote sensing, spring algae bloom, summer algae bloom
Citation: Silva E, Counillon F, Brajard J, Korosov A, Pettersson LH, Samuelsen A and Keenlyside N (2021) Twenty-One Years of Phytoplankton Bloom Phenology in the Barents, Norwegian, and North Seas. Front. Mar. Sci. 8:746327. doi: 10.3389/fmars.2021.746327
Received: 23 July 2021; Accepted: 12 October 2021;
Published: 10 November 2021.
Edited by:
Hjálmar Hátún, Faroe Marine Research Institute (FAMRI), Faroe IslandsReviewed by:
Rajdeep Roy, Indian Space Research Organisation, IndiaTomonori Isada, Hokkaido University, Japan
Copyright © 2021 Silva, Counillon, Brajard, Korosov, Pettersson, Samuelsen and Keenlyside. This is an open-access article distributed under the terms of the Creative Commons Attribution License (CC BY). The use, distribution or reproduction in other forums is permitted, provided the original author(s) and the copyright owner(s) are credited and that the original publication in this journal is cited, in accordance with accepted academic practice. No use, distribution or reproduction is permitted which does not comply with these terms.
*Correspondence: Edson Silva, ZWRzb24uc2lsdmFAbmVyc2Mubm8=