- 1Arctic and Aquatic Research Division, Fisheries and Oceans Canada, Winnipeg, MB, Canada
- 2Demersal and Benthic Sciences Division, Maurice-Lamontagne Institute, Fisheries and Oceans Canada, Mont-Joli, QC, Canada
- 3Department of Oceanography, Dalhousie University, Halifax, NS, Canada
- 4Department of Physical Oceanography, Woods Hole Oceanographic Institution, Woods Hole, MA, United States
- 5Laboratoire d’Océanographie de Villefranche, Sorbonne Université, Villefranche-sur-mer, France
- 6ArcticNet, Québec Océan, Takuvik, Departement de Biologie, Université Laval, Quebec City, QC, Canada
- 7Institute of Marine Research, His, Norway
- 8School of Biological Sciences, Oceans Institute, The University of Western Australia, Perth, WA, Australia
- 9Centre for Earth Observation Science, Clayton H. Riddell Faculty of Environment, Earth, and Resources, University of Manitoba, Winnipeg, MB, Canada
- 10Centre for Arctic Knowledge and Exploration, Canadian Museum of Nature, Ottawa, ON, Canada
Climate change is transforming marine ecosystems through the expansion and contraction of species’ ranges. Sea ice loss and warming temperatures are expected to expand habitat availability for macroalgae along long stretches of Arctic coastlines. To better understand the current distribution of kelp forests in the Eastern Canadian Arctic, kelps were sampled along the coasts for species identifications and percent cover. The sampling effort was supplemented with occurrence records from global biodiversity databases, searches in the literature, and museum records. Environmental information and occurrence records were used to develop ensemble models for predicting habitat suitability and a Random Forest model to predict kelp cover for the dominant kelp species in the region – Agarum clathratum, Alaria esculenta, and Laminariaceae species (Laminaria solidungula and Saccharina latissima). Ice thickness, sea temperature and salinity explained the highest percentage of kelp distribution. Both modeling approaches showed that the current extent of arctic kelps is potentially much greater than the available records suggest. These modeling approaches were projected into the future using predicted environmental data for 2050 and 2100 based on the most extreme emission scenario (RCP 8.5). The models agreed that predicted distribution of kelp in the Eastern Canadian Arctic is likely to expand to more northern locations under future emissions scenarios, with the exception of the endemic arctic kelp L. solidungula, which is more likely to lose a significant proportion of suitable habitat. However, there were differences among species regarding predicted cover for both current and future projections. Notwithstanding model-specific variation, it is evident that kelps are widespread throughout the area and likely contribute significantly to the functioning of current Arctic ecosystems. Our results emphasize the importance of kelp in Arctic ecosystems and the underestimation of their potential distribution there.
Introduction
Kelp forests dominate shallow rocky coasts in cold-water regions (Wernberg et al., 2019). These forests are dominated by large brown algae in the order Laminariales (Alongi, 2018). Where abundant, kelps form marine forests that provide a number of important ecosystem services: creating habitat, serving as food and cover for pelagic and benthic organisms, acting as nursery grounds for fish and other fauna, supporting complex food webs through nutrient filtration, coastal protection, and carbon sequestration through detritus export to deeper waters (Krause-Jensen and Duarte, 2016; Eger et al., 2021). Kelp forests are found along 28% of the world’s coastline (Starko et al., 2021) and are present in 43% of the world’s marine ecoregions (Krumhansl et al., 2016), covering ∼1,500,000 km2 (Jayathilake and Costello, 2020).
Climate change and human activities are altering environmental conditions with habitat loss occurring at a rapid rate in coastal zones, destabilizing habitat-forming species (Halpern et al., 2015), including documented recent declines in kelp forests globally (Duarte et al., 2018; Wernberg et al., 2018). In the Arctic, the loss of sea ice (and consequently increased light availability) and warming temperatures are predicted to increase the geographic extent and depth range of marine vegetation, with 145,093 km2 of suitable habitat projected for this type of species (Krause-Jensen et al., 2020). Coastal erosion from melting sea ice, fragmenting permafrost, and unusually high glacial inputs are, however, increasing sediment loads and freshwater inputs in high-latitude coastal zones (Fritz et al., 2017), which could result in direct kelp die-offs (Konar et al., 2017; Filbee-Dexter et al., 2019) or offset positive impacts of increased light and warmer temperatures (Bonsell and Dunton, 2018). Long-term research from Greenland, Russia, and Norway suggests a warmer Arctic with less sea ice may support higher kelp productivity and biomass by expanding the northern range and lower depth limit of these species (Filbee-Dexter et al., 2019; Krause-Jensen et al., 2020). However, the degree to which these changes will positively affect kelps will likely vary regionally, and depend on the extent of glacial ice melt, permafrost collapse, turbidity increase, and salinity reductions in coastal areas (Bartsch et al., 2016; Bonsell and Dunton, 2018; Traiger and Konar, 2018).
The Arctic is the epicenter of the global climate crisis, warming at least twice as fast as the global average (Niemi et al., 2019; Ballinger et al., 2020). It is predicted that, without taking action to reduce global warming, the Arctic will be ice-free each summer before 2050 (Hwang et al., 2020). The Canadian Arctic is warming at a rate three times the global average (Flato et al., 2019) and the greatest reductions of sea ice cover duration and concentration have been observed there (Stammerjohn et al., 2012; Mudryk et al., 2018; Derksen et al., 2019). The Eastern Canadian Arctic has been highlighted as lacking historical baseline data generally (Archambault et al., 2010) and for kelps, specifically (Krumhansl et al., 2016), with sampling efforts for the latter only recently increasing through documentation of kelp forests along Arctic and subarctic coastlines between Ellesmere Island and Labrador and along coasts in Lancaster Sound, Ungava Bay, Hudson Bay, Baffin Bay, and Resolute Bay (Lee, 1973; Adey and Hayek, 2011; Filbee-Dexter et al., 2019; Bringloe et al., 2020; Starko et al., 2021). This critical knowledge gap is particularly worrisome given rapid ongoing environmental changes along Arctic coasts (AMAP, 2017). Many uncertainties are related to this knowledge gap. For example, the exact number of macroalgal species (including kelp) is not certain, and there are different estimates in the literature, ranging from 160 species at the Arctic scale (Bluhm et al., 2011), but 210 at the Canadian Arctic scale (Archambault et al., 2010). It is of particular interest to evaluate the current state of these ecosystems before substantial changes occur (and can no longer be measured or estimated). Anticipating these changes and understanding these new ecosystems and their functioning is a key priority for northern communities.
Modeling techniques are useful to fill gaps and make predictions to help understand and predict where species may be found and the rate and direction of predicted changes. Species distribution models (SDM) have been used to predict the distribution of suitable habitat for kelp at local (Gorman et al., 2013; Bajjouk et al., 2015; Young et al., 2015), regional (Raybaud et al., 2013; Assis et al., 2016; Wilson et al., 2019) and global/Arctic (Müller et al., 2009; Assis et al., 2018a; Jayathilake and Costello, 2020; Krause-Jensen et al., 2020) scales. For the Arctic region, some of these works include projections in the Eastern Canadian Arctic (Müller et al., 2009; Assis et al., 2018a), but none of them have used data directly from the field or focused on arctic populations. In fact, kelp are poorly represented in studies on the processes driving changes in habitat suitability and species distributions (Melo-Merino et al., 2020). Ensemble modeling is a widely used SDM tool (Hao et al., 2019) that has been shown to reduce single-model bias (Araújo and New, 2007) by aggregating the results of multiple models in a combined final mean prediction (Araújo and New, 2007). An integrated ensemble approach is especially important in cases where future projections are being calculated, increasing the level of confidence in the results (Guisan et al., 2017).
Changes in environmental conditions can affect not only the suitability of kelp habitat, but also the abundance of the species that are present. Macroalgal abundance can be estimated in terms of density, biomass and percent cover (e.g., Gorman et al., 2013; Bajjouk et al., 2015; Young et al., 2015; Krumhansl et al., 2016; van Son et al., 2020). However, quantitatively calculating these various estimates of abundance for currently unstudied areas and future scenarios cannot be done using most SDM tools, which rely on presence/absence input data. The Random Forest model is the natural candidate for this sort of investigation due to its ability to model numeric results and its very low computational cost that allows for much higher replication of model runs.
Using a combination of these two modeling approaches (ensemble models for habitat suitability supplemented with Random Forest for kelp cover), this paper aims to identify the primary environmental drivers and make spatial predictions of habitat suitability and cover of kelps in the Eastern Canadian Arctic under current conditions and a future extreme climate-change scenario.
Materials and Methods
Study Region
The majority of the Canadian coastline is rocky (Frederick et al., 2016), with erosional shorelines that are wave-dominated (Nyberg and Howell, 2016). Much of the nearshore shallow region is affected by ice scour, generally preventing rich bottom flora from establishing (Stewart and Lockhart, 2005). This study focuses on the Eastern Canadian Arctic (Figure 1). The largest portion of the area of interest is the Hudson Bay Complex (i.e., James Bay, Hudson Bay, Hudson Strait and Foxe Basin), which is relatively shallow (150-m mean depth) (Prinsenberg, 1986) (Figure 1) and receives large volumes of freshwater runoff and advected arctic marine waters (Stewart and Lockhart, 2005; and references therein). Variations in local atmospheric conditions largely control sea-ice cover variability (Hochheim and Barber, 2014; and references therein). The deepest and most extensive ocean corridor (650-m deep) in the region is from the Labrador Sea north through the central Davis Strait and Baffin Bay, and west into Lancaster Sound (Tang et al., 2004; Jørgensen et al., 2005).
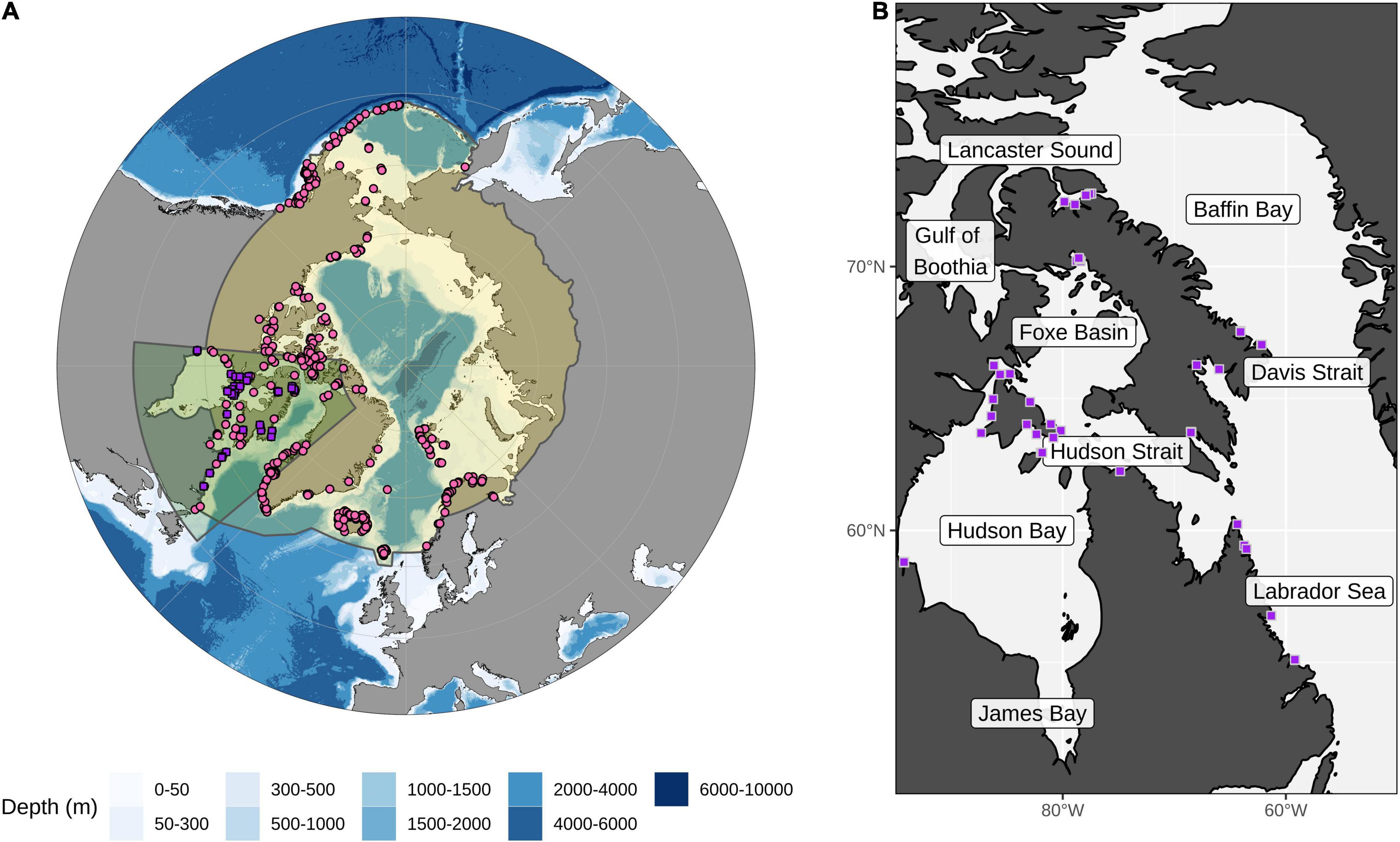
Figure 1. Map of the Arctic and the focal area for this study. (A) The Arctic region is shown in yellow with the focal area of the study highlighted with a green polygon. Presence data points used in the ensemble model are shown as pink circles and purple squares (for details on the complete set of presence points included for each species see Supplementary Figure 1). (B) The study area shows the recent Arctic field campaign collection points (purple squares) for kelp coverage data used in the Random Forest and presence points for ensemble models (for details on sampling sites see Supplementary Table 1). Regions discussed in the text are labeled.
Species Data
The kelp species selected for this study were: (1) Agarum clathratum Dumortier, 1822; (2) Alaria esculenta (Linnaeus) Greville, 1830; (3) Laminaria solidungula J. Agardh, 1868; and (4) Saccharina latissima (Linnaeus) C. E. Lane, C. Mayes, Druehl and G. W. Saunders, 2006. For the analysis to quantify kelp cover, S. latissima and L. solidungula were grouped into the ‘Laminariaceae’.
The taxonomy of kelp species has been in flux for the last couple of decades with some changes even at the genus level (Lane et al., 2006; McDevit and Saunders, 2010; Starko et al., 2019). The species included in this study were no exception to these reorganizations and taxonomic challenges.
We identified name changes and possible misclassifications for each species of interest in this study. Some historical specimens of A. clathratum were labeled as Agarum cribrosum Bory de Saint-Vincent, 1826, a synonym (Silva, 1991). For S. latissima, some taxonomic synonyms we encountered included Laminaria agardhii Kjellman, 1877, Laminaria groenlandica Rosenvinge, 1893, L. saccharina (Linnaeus) J. V. Lamouroux, 1813, and Saccharina groenlandica (Rosenvinge) C. E. Lane, C. Mayes, L. Druehl and G. W. Saunders, 2006 (Guiry and Guiry, 2020). For the purposes of this study, we considered Saccharina longicruris (Bachelot Pylaie) Kuntze, 1891 as equivalent to S. latissima (McDevit and Saunders, 2010).
The species in the Eastern Canadian Arctic and North Atlantic Ocean now known as Hedophyllum nigripes (J. Agardh) Starko, Lindstrom and Martone, 2019, had in the past been attributed to S. groenlandica, but Longtin and Saunders (2015) have shown that S. groenlandica is a synonym of S. latissima. Moreover, in terms of misidentifications, digitate forms of Hedophyllum nigripes can be mistaken for Laminaria digitata, while non-digitate forms of H. nigripes can be mistaken for S. latissima (Longtin and Saunders, 2015). Therefore, non-digitate kelps identified as S. groenlandica could be either S. latissima or H. nigripes, while digitate kelps identified as L. digitata could be H. nigripes. Our best efforts were done to separate these records to model the predicted distributions. Initially, H. nigripes and L. digitata occurrence locations were used to build individual models, but the resulting predictions were not robust or biologically meaningful. The scant occurrence points led to additional problems, such as adding a spatial bias in the model with training and testing points not being independent. Hence, these species were not included in the final analysis.
For records where it was not possible to trace an image or identification, a decision was made to trust the source, but it is acknowledged that there could be issues regarding taxonomic identifications in these cases.
Kelp Occurrence
Occurrence data of kelp species were compiled using museum data records of kelp specimens from the National Herbarium of Canada at the Canadian Museum of Nature (CANA). Each was accompanied by a picture of the specimen, enabling identification if a record needed to be renamed according to the most updated taxonomic nomenclature. We also compiled information from datasets collected from field campaigns that took place between 2011 and 2020 in different regions of the Eastern Canadian Arctic (North Baffin Island, Hudson Strait, Roes Welcome Sound, Foxe Basin, Baffin Bay, Davis Strait, Labrador Sea, and Ellesmere Island). Divers and drop cameras were used to take pictures and/or make videos along transects to identify kelp (see details in Supplementary Table 1). To complement this dataset and increase the number of occurrence points, biodiversity databases such as the Global Biodiversity Information Facility (GBIF1) and Ocean Biogeographic Information System (OBIS2) were used. Additionally, a literature search was done to complete occurrence records in places where there were gaps in the information already gathered (Northeastern and Southwestern Greenland, Svalbard, and some regions of the Barents and White seas) (Borum et al., 2002; Hop et al., 2016; Schoenrock et al., 2018; Filbee-Dexter et al., 2019; Ronowicz et al., 2020). We also contacted kelp experts (e.g., G. Saunders) who provided occurrence records from their lab databases (Supplementary Figure 1, but also see Supplementary Table 2 for the complete raw dataset).
To limit over-prediction, duplicate records were removed and only a single presence record was retained for each grid cell (see Section “Environmental Data” for details on grid size). Occurrence points were considered at the same resolution as the corresponding environmental layers (García-Roselló et al., 2015), and data were rarefied to provide an unbiased sample of points using the SDMtoolbox in ArcGIS (Brown et al., 2017). This was calculated using the ‘Spatially Rarefy Occurrence Data for SDMs’ tool, where spatially autocorrelated occurrence points are removed. All points were verified to ensure that they were in sea grids. Kelp normally grows along the coast in the intertidal zone down to depths of approximately 30–40 m (Wernberg et al., 2019). However, some occurrences were located within inland grids. For these occurrences, any that were up to two grid cells inland were moved to the closest sea grid using the Near Proximity tool in ArcMap v10.2.2. Any points further inland were removed from the database.
All retained occurrence points were then clipped to only include those that were from Arctic environments (using the Arctic region delimited by the Arctic Monitoring and Assessment Programme, AMAP) (Figure 1A and Supplementary Figure 1) to ensure that the data used for modeling was focused on cold-adapted kelp populations. Populations that are distributed on the extreme ends of their temperature ranges can have local adaptations to marginal climatic conditions present in those places, so this approach excluded occurrence data from southern limits of these species that could skew the model (Angilletta et al., 2006; Trivedi et al., 2008; Vale et al., 2014; Bennett et al., 2015). Furthermore, the relative contribution of environmental variables used can also depend on the geographic scale to which the model is fit (Nyström Sandman et al., 2013). Models that are fitted regionally have been shown to perform better when the interest is to model marginal populations (Vale et al., 2014; Guisan et al., 2017). This is because, for widespread species, model accuracy can be reduced and distribution can be overestimated due to different ecological preferences among subpopulations within the species range (Stockwell and Peterson, 2002; Hernandez et al., 2006). This consideration is particularly important in our case, since arctic kelp are part of arctic marine biomes that consist of unique assemblages in polar waters (Bringloe et al., 2020) and the species selected for this study are known to have true arctic populations (Müller et al., 2009).
Kelp Cover
Previous and recent research campaigns across the Eastern Canadian Arctic (Filbee-Dexter et al., 2021) collected kelp cover data that allow habitat suitability and percent cover of kelp species to be modeled and predicted throughout this region. Abundance of kelp can be measured in several ways, with biomass per area, number of individuals per area (i.e., density) and percent cover being the three most common. Although biomass per area provides the best measure of algal standing stock, most of the data combined in the present study and used to estimate kelp abundance were derived from videos or photographs of the kelp canopy and seafloor. Because it is not possible to extract either biomass or density reliably from photographs, percent cover was chosen to maximize the number of sites for which data could be obtained. All sampling campaigns collected video or still images of the seafloor at comparable depths either by SCUBA diving or using a drop camera or remotely deployed camera system (for further details on sampling campaigns see Supplementary Table 1). Video transects were analyzed by taking frame grabs (10 – 12 per transect) at regularly spaced intervals along the video (∼20 – 30 s depending on total video time). Only high-quality images with a clear view of the canopy or substratum were used. Photographs were analyzed for percent cover of all kelp species and other macroalgae using ImageJ (Schindelin et al., 2012) and the point-count method to calculate percent cover by overlaying 49 evenly spaced points on each image and identifying the seaweeds (or substratum) under each point. In some videos it was difficult to distinguish between L. solidungula and S. latissima so these species were grouped into the single category “Laminariaceae spp.”.
Environmental Data
Environmental data layers were used as input for modeling habitat suitability and cover of kelp in the Eastern Canadian Arctic. These layers were downloaded at a global scale from Bio-ORACLE v2.13 (Assis et al., 2018b) and then cropped to the Arctic region. Bio-ORACLE layers are created from pre-processed global ocean re-analyses of a combination of satellite and in situ observations to calculate monthly averages for present conditions (roughly 2000–2014) (Assis et al., 2018b). The resolution of these environmental layers was 5 arcmin (approximately 9.2 km at the equator). A set of 9 environmental layers were initially included to model the present conditions in the Arctic and were composed of long-term mean values of minimum and maximum records per year (except for surface temperature – see explanation below) of variables at the maximum depth of the grid cell and/or sea surface values. Environmental layers that were included in the study were based on knowledge about variables highlighted as being important limiting factors for kelp taxa and that have been used in other modeling studies (Zacher et al., 2009; Bosch et al., 2018). These variables included sea surface temperature, sea surface salinity, ice thickness, bottom current velocity, dissolved oxygen, photosynthetically available radiation (PAR), and bottom minerals and nutrients (iron, nitrate, and phosphate). A sensitivity test was done to identify a priori the type of temperature layer that is most significant for kelp (the one that, by itself, best explains known kelp distribution) by calculating individual Generalized Linear Models for each species using only minimum, maximum and mean sea surface and bottom temperature. This analysis was limited to temperature layers since it is the primary variable assumed to limit the distribution of species (Spence and Tingley, 2020). Only maximum sea surface temperature showed good predictor performance on its own and was thus included in the set of predictors. Bathymetry and land distance were obtained from Aquamaps4 (Kaschner et al., 2016) at the same resolution as the Bio-ORACLE layers. These two layers were used as masks for both modeling approaches (see below) after the models were run and were used to filter out pixels that were too deep or too far from land to have any real likelihood of supporting kelp. Besides, these two variables are indirect predictors, which are expected to be weaker at larger extents and are only appropriate for use at local scales (Austin, 2002; Nyström Sandman et al., 2013). Fine-scale measured variables become predictors with less predictive accuracy over a wider geographical scale (Nyström Sandman et al., 2013). Any pixels deeper than 50 m or more than 15 km from the coastline were removed from the final results. Supplementary Figure 2 shows the workflow for environmental variables selection.
Modeling Approaches
Variable Selection
The inclusion of correlated variables may lead to errors in the model and not correspond to real physiological tolerances of a species (Marcelino and Verbruggen, 2015). Hence, collinearity of the set of 9 variables was tested by applying a combination of the Variance Inflation Factor (VIF) in R (Naimi et al., 2014) and Pearson correlation coefficient (PCC) (Supplementary Figure 2). The VIF method can detect hidden correlation structures that are often not seen through pairwise correlations (Guisan et al., 2017). After the stepwise elimination of highly inflating variables using the selection criterion VIF > 10, some remaining variables were still clearly correlated, and PCC (0.8) was applied. Only six variables were thus retained for model predictions: (1) maximum surface temperature, (2) mean surface salinity, (3) mean ice thickness, (4) mean bottom iron, (5) mean bottom phosphate, and (6) mean bottom current velocity (Supplementary Figure 2).
Note that after models were run, the variable importance across models for each species were averaged and raw values rescaled to range from 0 to 1 to compare and select the top few most important variables.
Habitat Suitability: Ensemble Models
Predicted habitat suitability was calculated and modeled using ensemble models with biomod2 v3.4.6 (Thuiller et al., 2020) within R v4.0.3 (R Core Team, 2020). Five modeling techniques known to be robust and perform well in this type of approaches were run: Maximum Entropy (MaxEnt), Generalized Linear Model (GLM), Random Forest (RF), Artificial Neural Network (ANN), and Generalized Additive Model (GAM). Detailed information on these models is available in Thuiller et al. (2020).
Prediction of suitable species habitat uses two types of information as input: (i) occurrence data (species known occurrence points) complemented with the generation of pseudo-absence/background points, and (ii) environmental variables as data layers. Model evaluation was done using cross-validation with 70% of the occurrence points chosen randomly and used to train the model and the other 30% to test it. Five replicates of 1,000 random pseudo-absence points were generated to build several sets of pseudo-absences to prevent sampling bias (Guisan et al., 2017). The use of a smaller numbers of pseudo-absences is suggested when there are limited numbers of presence points in a species’ database (Barbet-Massin et al., 2012).
The five models used to build the final ensemble model were run with default setting options. A complete set of default options can be found in the biomod2 package under the function ‘Print_Default_ModelingOptions()’. Replicate model runs were done five times, and the average was used as the final outcome of the models, which was then converted to binary values of suitable and unsuitable habitat. This binary classification was based on the maximum training sensitivity plus specificity threshold since it is known to produce the most accurate predictions (Jiménez-Valverde and Lobo, 2007; Liu et al., 2013). True Skill Statistic (TSS), the Receiver Operating Curve (ROC), False Alarm Ratio (FAR), Accuracy, and Success Ratio (SR) were used to assess the accuracy of predictions (see Supplementary Table 3 for more information on model evaluation metrics). Models with a TSS score < 0.7 were excluded from the final ensemble predictions as suggested as an evaluation metric quality threshold by Guisan et al. (2017). This measures ranges from −1 to +1, with statistically excellent model performance being indicated by a minimum of 0.7 (Allouche et al., 2006).
Cover: Random Forest
Of the models used in the ensemble that can provide the continuous numerical outputs needed to estimate kelp cover (%), Random Forests are the least computationally expensive and tend to be the most accurate (Li and Wang, 2013). Kelp cover was modeled with this single modeling technique as it allowed for many more repetitions to be performed than an ensemble model. The same environmental layers used for the ensemble model were used here to create 1,000 independent Random Forests, each grown with 200 trees (i.e., branches). Results of each individual Random Forest were trained on a random set of 70% of the abundance data and tested on the remaining 30%. The cover values per pixel for these 1,000 models were averaged to produce the final results. Running this higher number of models allowed for a more meaningful calculation of the variance in the cover projected by the models.
An important difference between the continuous Random Forest and the presence-only ensemble approach is that only the cover values found within the study area (Figure 1A, green box, and Figure 1B, purple squares) were used for the Random Forests, such that the environmental data fed to the Random Forests was restricted to this area as well. Projections of kelp cover were presented only in the regions where habitat suitability was predicted.
Future Projections
After running, testing, and validating predictive models of current kelp habitat suitability and cover, these predictions were projected into the future under global change scenarios. Environmental layers representing future global change were obtained from Bio-ORACLE v2.1 for the most extreme emission scenario (RCP 8.5) for the years 2050 and 2100. Under this extreme emission future scenario without climate policies, increasing greenhouse gas emissions are projected to create a median temperature anomaly of 4.9°C relative to pre-industrial levels by the end of the century (Riahi et al., 2007; Moss et al., 2010) and an ice-free Arctic during the summer season by 2050 (Hwang et al., 2020). All of the variables that were used for running the models for present conditions were also used for models that were projected into the future, with the caveat that only temperature, salinity, ice thickness and current velocity data layers changed for projected future scenarios (Supplementary Figure 2).
Results
Importance of Variables
Of the six environmental variables that were used as explanatory predictors for habitat suitability and cover, each was, in different proportions, included as one of the top four most important predictors for at least one species and for one of the models (Figure 2 and Supplementary Table 4). Four environmental predictors (temperature, ice cover, salinity, and phosphate) were selected for habitat suitability predictions, but their relative importance varied by species. Environmental predictors for cover were more variable and included all six variables. Ice thickness and salinity were the most important predictors for all combinations of species and methodologies, together explaining up to 62% of kelp habitat suitability and 44% of kelp cover (Figure 2 and Supplementary Table 4). Temperature and phosphate were also important (mainly for habitat suitability), while iron and current velocity were only important for cover (Figure 2).
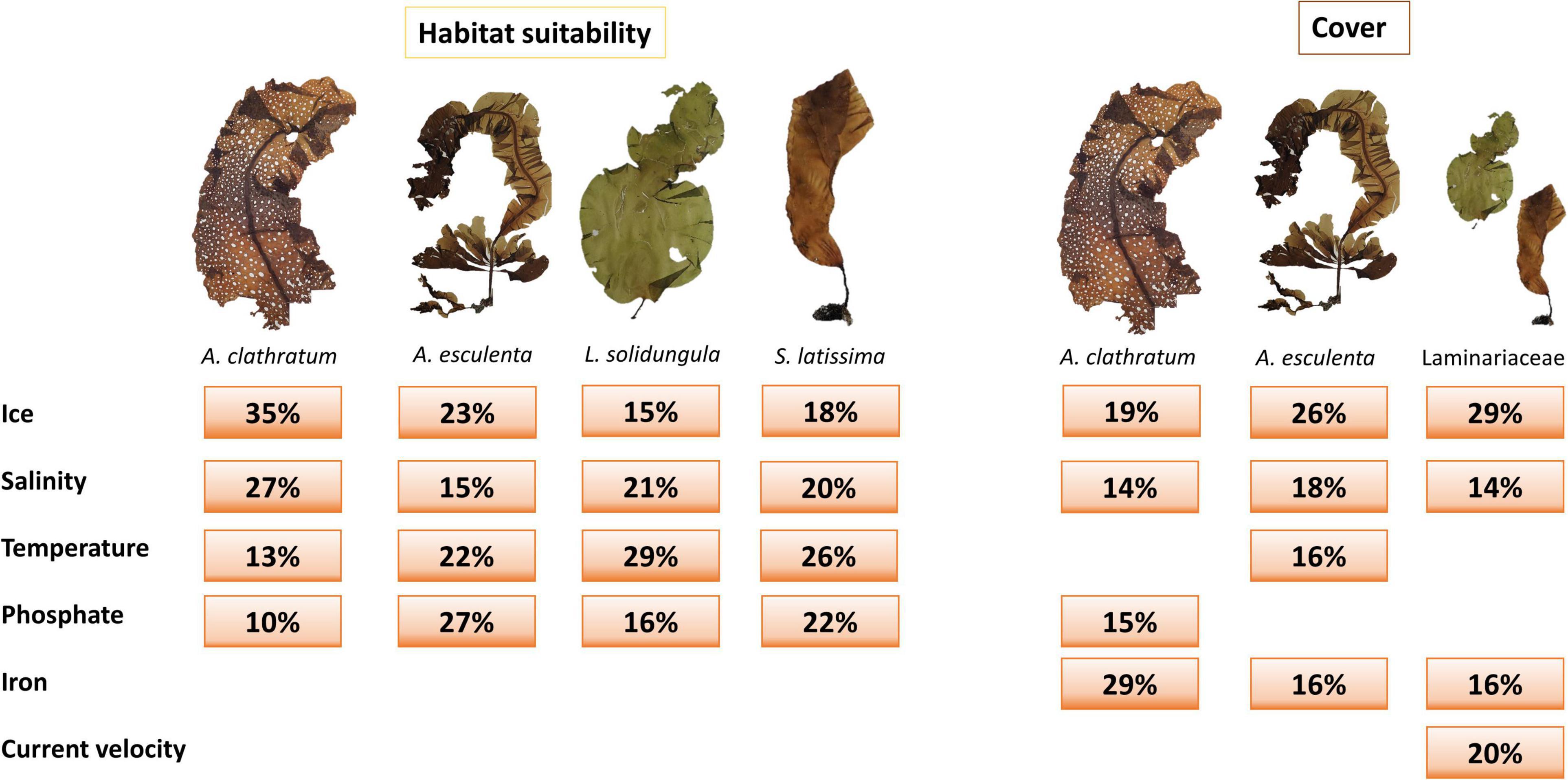
Figure 2. Environmental variable importance (rounded in%, highlighting the% contribution of that variable that explains the modeled distribution) for species and model runs (habitat suitability: Ensemble models, cover: Random Forest). Pictures of the species were taken from the herbarium of the Canadian Museum of Nature (CANA) (details in Supplementary Table 4).
Looking at response curves of environmental variables across all species for ensemble models, the most notable link between variable importance in habitat suitability for L. solidungula is that it decreases at a slower pace in regions of greater ice thickness (together with S. latissima) and is less tolerant to higher temperatures (Figure 3A). This differs to the other species, where predicted habitat suitability is highest in regions with less ice. This is most strongly pronounced for A. clathratum, partially explaining why it is projected to increase so much in future change scenarios. The response curves for the Random Forest models (Figure 3B) tend to be noisier than for the ensembles (Figure 3A), with multiple local optima per species and variable. Generally, the Random Forests predict higher percent cover for Laminariaceae than for the other species. This is due to the higher percent cover values in the raw data and it should be noted that this is a different scale than the binary presence/absence results of the ensemble models.
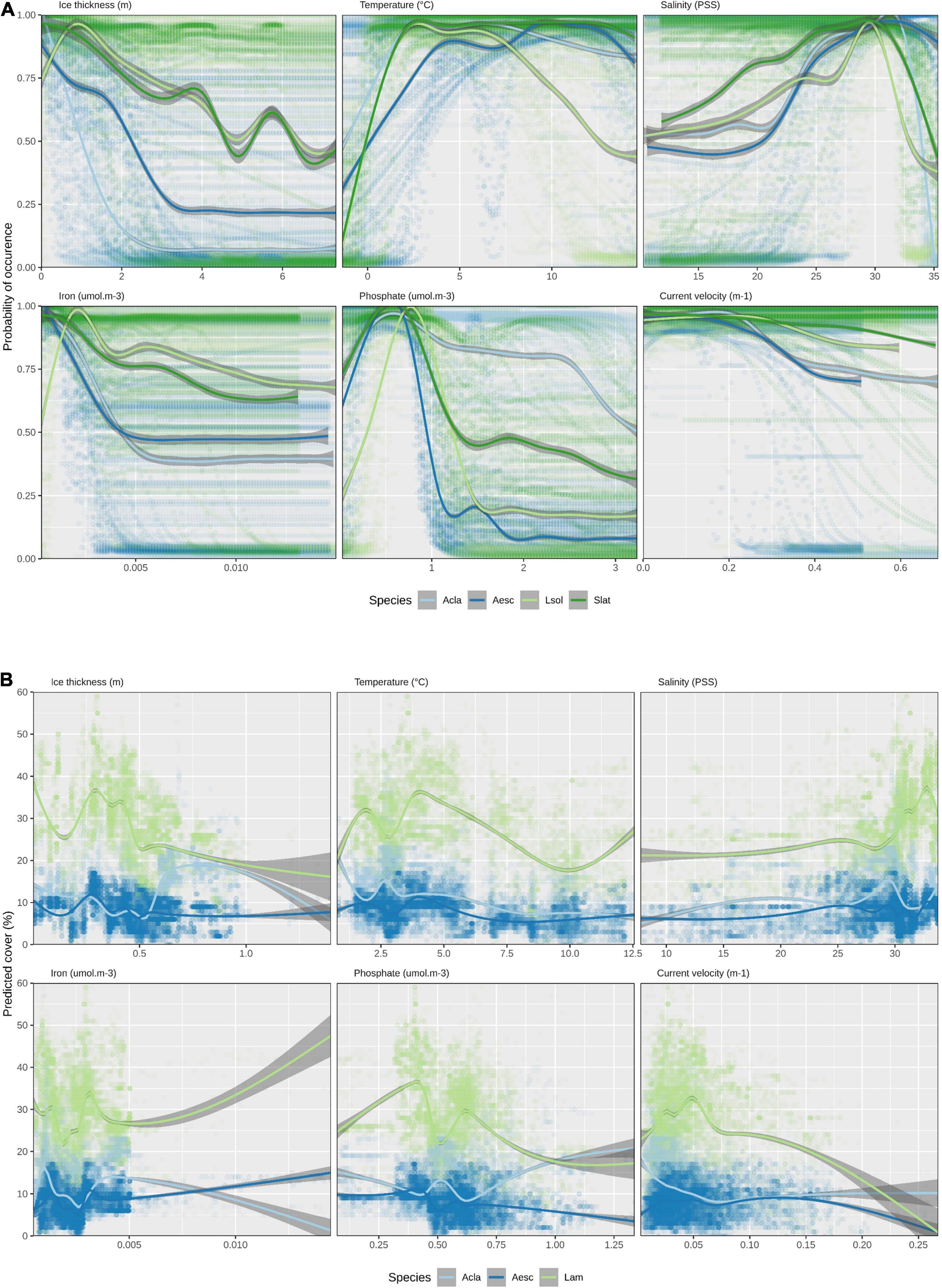
Figure 3. Environmental response curves (generalized additive model) across all species and models. (A) Ensemble models for habitat suitability and (B) Random Forest for percent cover (Mean ice thickness, maximum surface temperature, mean surface salinity, mean bottom iron, mean bottom phosphate, mean bottom current velocity). Acla, Agarum clathratum; Aesc, Alaria esculenta; Lsol, Laminaria solidungula; Slat, Saccharina latissima; Lam, Laminariaceae. Note that values for the X- and Y-axes differ between the (A) ensemble and (B) Random Forest model panels and that the species shown between the two modeling approaches differ, as explained in the methods. The standard error for the GAMs are shown as dark gray ribbons.
Predicted Suitability
The area of projected present-day suitable habitat for L. solidungula is the highest of the four modeled species at 269,000 km2, while the lowest is for A. esculenta at 183,000 km2. Almost all of the study area is predicted to be suitable for at least one of the species modeled, with the exception of James Bay, where none of the kelps are predicted to be present. In total, the estimated area of suitable habitat considering all modeled species in the Eastern Canadian Arctic was 312,000 km2.
Habitat suitability throughout the Eastern Canadian Arctic is projected to increase for all modeled species except for L. solidungula (Figure 4). The greatest gain in range of suitable habitat is projected for A. clathratum by 2100 at +39,000 km2 (Figure 4A), with expansion projected southward along the coast of Hudson Bay, westward along the Arctic Archipelago seen in the study region, and eastward along the north and west coasts of Greenland. There are some minor losses in suitable habitat projected in the Gulf of Boothia, west of Baffin Island. Although most of the coastal regions in Hudson Bay are projected to become more suitable for A. clathratum in the future, there is no suitable habitat projected in James Bay (Figure 4A).
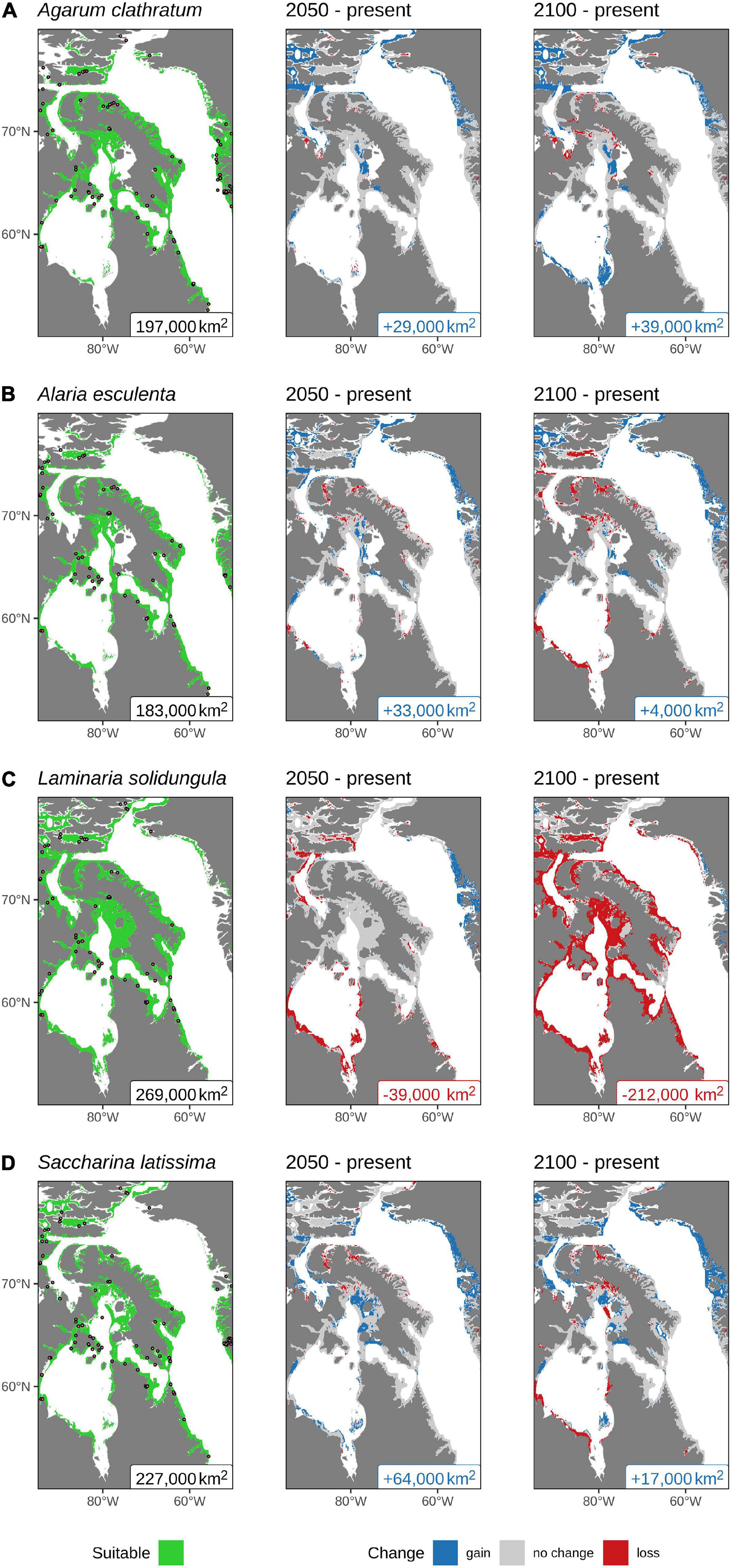
Figure 4. Predicted suitable habitat calculated using the results from the ensemble model projections of kelp presence in the Eastern Canadian Arctic: (A) Agarum clathratum; (B) Alaria esculenta; and Laminariaceae spp.: (C) Laminaria solidungula; and (D) Saccharina latissima under present (left panel), 2050 (middle panel), and 2100 (right panel). The presence data used in the models are shown with dots (left panel), and the colored text labels at the bottom of each panel show the total surface area (rounded to the nearest 1,000 km2) projected to be suitable habitat for the given species. All values are based on an assumed depth limit of 30 m. For clarity, all pixels within 15 km of land or 50-m deep are shown.
For A. esculenta, a net gain in the range of suitable habitat is predicted along the coast of western Greenland and westward along the Arctic Archipelago (Figure 4B). Minor losses in suitable habitat in Hudson Bay, Foxe Basin, and the fjords of Baffin Island appear in the 2050 projection and increase in size by 2100. Of the four modeled species, A. esculenta shows the lowest overall change in projected suitable habitat, with a projected gain in area of +33,000 km2 over the present area by 2050, but only a further increase of +4,000 km2 by 2100.
Laminaria solidungula is projected to have the greatest loss in suitable habitat by 2100 (−212,000 km2, Figure 4C). The 2050 projection shows that most of Hudson Bay and the upper arm of the Northwest Passage will no longer be suitable for L. solidungula while some new suitable habitat may occur along the west coast of Greenland. Any reprieve observed in 2050 will, however, be lost by 2100 when it is projected that all but the very northernmost portion of this species’ current estimated extent will no longer be suitable (Figure 4C). This makes the projected suitable habitat of L. solidungula by far the smallest of the four species by 2100.
The other Laminariaceae species modeled in this study, S. latissima, shows a contrasting trend relative to L. solidungula. It is projected to have the largest gain in suitable habitat in 2050 at +64,000 km2 and the second largest gain in area of suitable habitat, after A. clathratum, by 2100 at +17,000 km2. The projected area of present day suitable habitat is the second highest, although the large projected increases in the future throughout much of the study area project that S. latissima will have the largest area of suitable habitat in both 2050 and 2100 (Figure 4D). Declines are projected for some areas (e.g., north of Baffin Bay, Foxe Basin and Hudson Bay) by 2100. In general, suitable habitat is projected to occur in the northernmost reaches of the study area and is expected to persist into the future. As the ocean warms and ice recedes, the model projects that S. latissima will gain suitable habitat along much of the west coast of Greenland and the northern arm of the Northwest Passage.
Predicted Cover in Suitable Regions
Of the study area regions that are projected in the present day to provide suitable habitats for A. clathratum, the densest cover (%) is predicted along the northeast coast of Baffin Island, with an average net cover of 5% (Figure 5A). As the range of projected suitable habitat for this kelp expands in 2050, the density of cover is predicted to increase along the north coast of Baffin Island and somewhat within Foxe Basin, although the overall cover is predicted to either remain the same or decrease throughout the habitable range, with no overall change. The areas that show decreases in cover in 2050 are projected to remain low in 2100, but much of the coastline in the southern half of the study area where suitable habitat is projected to expand is predicted to see increased cover. However, these changes nearly balance out with a net change of −1% in cover overall across the study area.
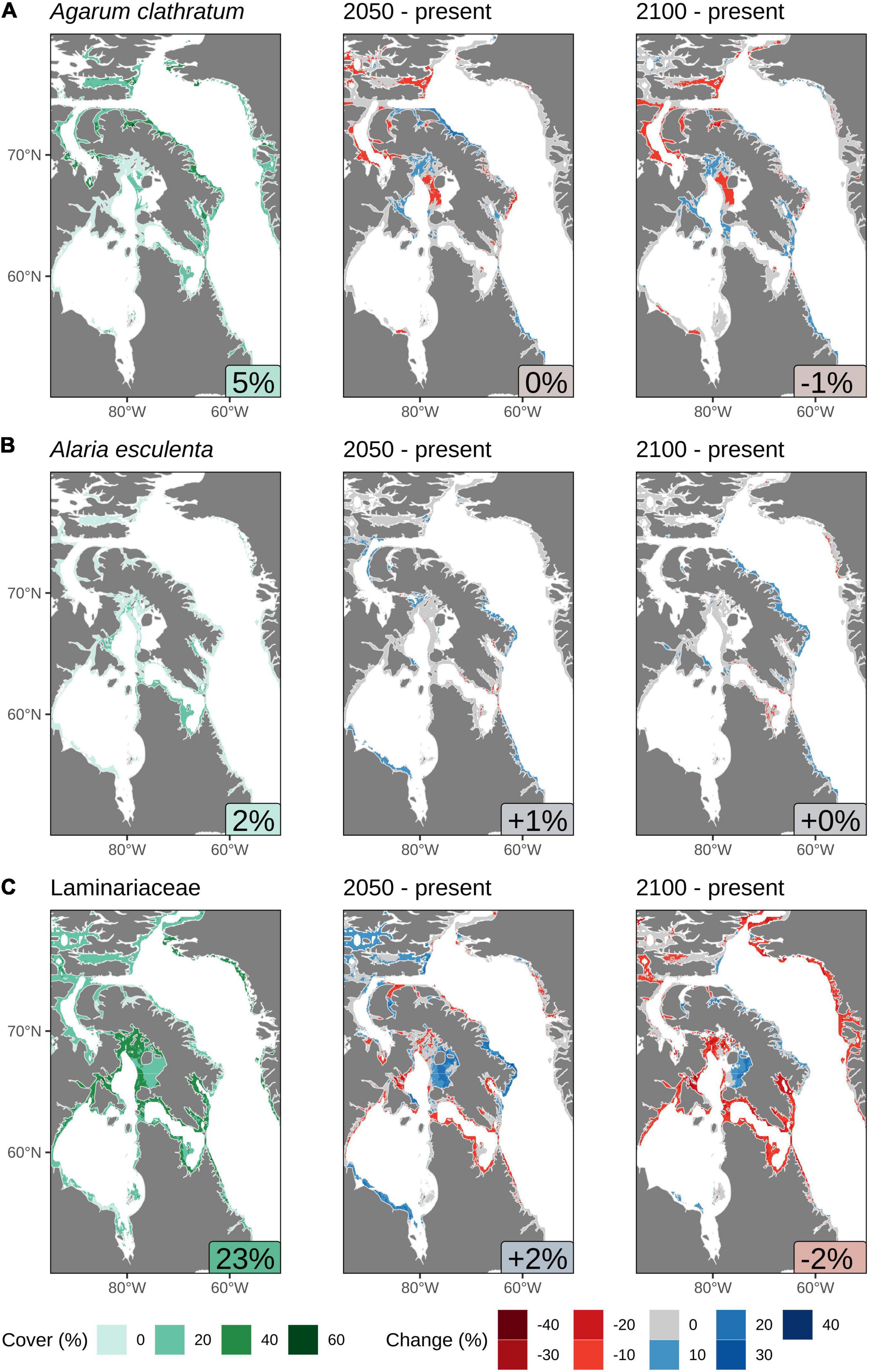
Figure 5. Predicted cover (%) calculated in regions of suitable habitat using the results from the Random Forest model in the Eastern Canadian Arctic: (A) Agarum clathratum, (B) Alaria esculenta, and (C) Laminariaceae spp. The text labels in the bottom right of the panels in the left column show the average % cover in suitable habitat areas. The text column in the center and right columns show the average change in the % cover for that projected year. Note that these changes are not large due to much of the suitable habitat having a projected change in cover of 0%. All values shown are based on calculations of an assumed depth limit of 30 m, but for clarity, pixels are shown with a depth limit of 50 m or if they are within 15 km of land.
Much of the predicted expansion in suitable habitat for A. esculenta in the northern regions of the study area (Figure 4B) is not predicted to be accompanied by increases in cover, and the current average cover in suitable habitats is only 2% (Figure 5B). Increases in cover by 2050 are projected along the southern shore of Hudson Bay, the northern arm of the Northwest Passage, the east coast of Baffin Island, and the Labrador coast. By 2100, the increased cover along the Labrador coast is projected to persist, while that along much of the east coast of Baffin Island is projected to increase further. Some minor decreases in cover are projected within Hudson Strait by both 2050 and 2100. Overall, an increase in cover of 1% is projected by 2050, but increasing losses in Hudson Strait by 2100 reduce the gain back to +0%.
As described above, it was necessary to combine the model results for Laminariaceae species. The projected suitable habitat for these two species differs substantially (Figures 4C,D), with L. solidungula predicted to all but disappear from the Eastern Canadian Arctic by 2100, whereas S. latissima should see gains in suitable habitat area. It is thus not surprising that combining these two species with very different fates into a single Random Forest model produced quite convoluted results. The average present-day projections for the cover of Laminariaceae spp. is 23%, which is by far the highest of all of the modeled species (Figure 5C). Projecting to 2050 shows that most of the projected habitable area will experience great increases or decreases in cover, but only an overall change of +2%. By 2100, most of the gains seen by 2050 will be reversed and the model projects overall losses of cover, with an average of −2%. Note that this projected loss is likely the effect of L. solidungula, having losses that are proportionally greater than predicted habitat gains in S. latissima. Unfortunately we cannot separate these effects based on percent cover projections (Figure 5C), although it may be inferred from the habitat suitability projections (Figures 4C,D).
Predictions of percent cover from the Random Forest models show higher average cover values (Supplementary Figure 3) when results are not filtered by habitat suitability predictions as shown here (Figure 5), although the northeastern part of Baffin Island is the region where there is predicted gain in percent cover for all species. The whole Eastern Arctic is predicted to suffer considerable environmental changes (e.g., reduced ice thickness and sea surface salinity and increased sea surface temperature) (Figure 6), but the northeastern coast of Baffin Island shows the least pronounced changes. In this region, maximum temperatures do not change as much as the other regions and ice persists, indicating that the northeastern coast of Baffin Island may act as a refugia with conditions similar to present day.
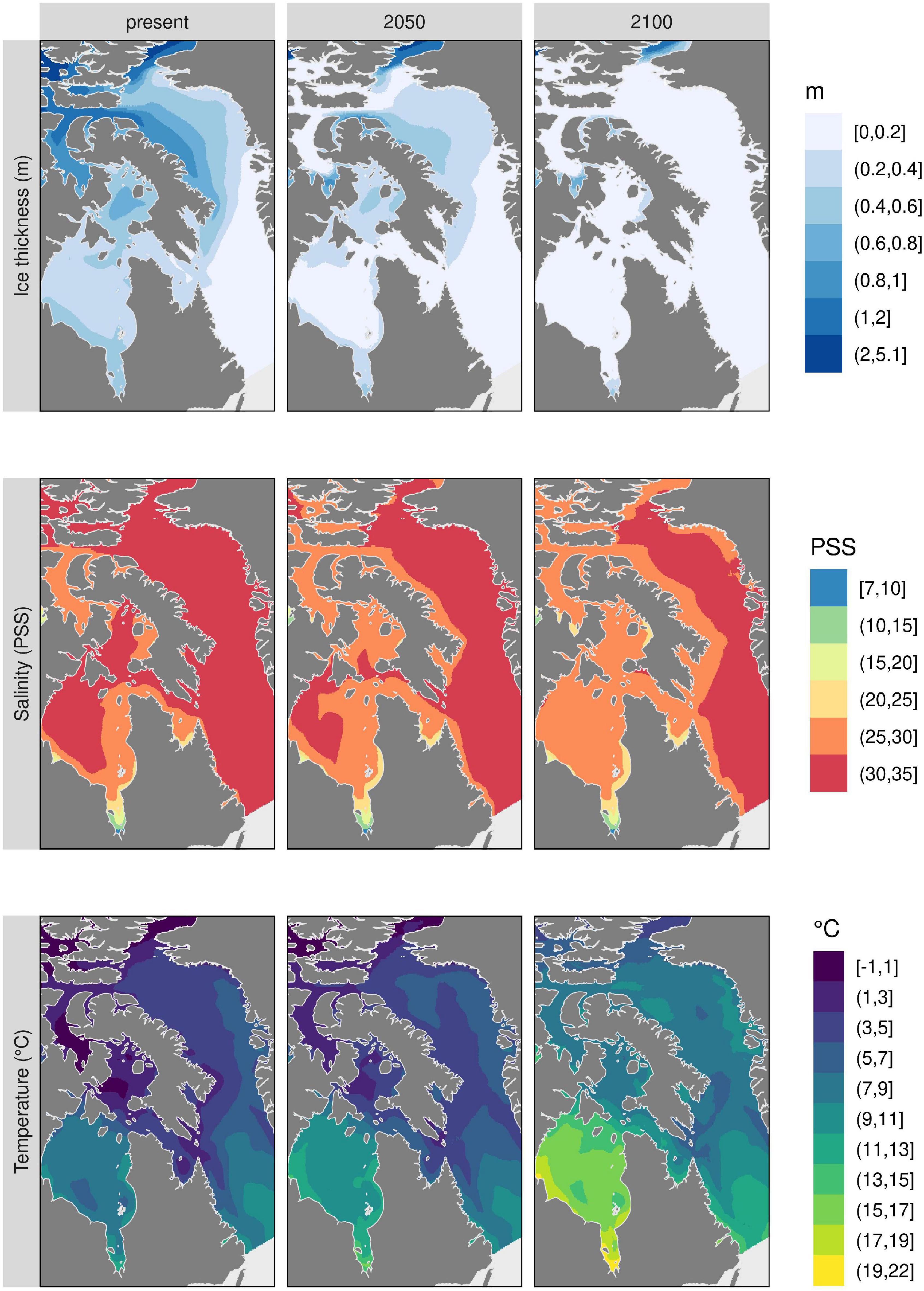
Figure 6. Top three environmental predictors used in kelp models. These layers have been modified from the original source (Bio-ORACLE v2.1; Assis et al., 2018b) to show changes through time in mean ice thickness, mean sea surface salinity, and maximum sea surface temperature.
Model Accuracy
As ensemble models are made up of the average of many models, it is important to consider the quality of individual models, potentially removing models from the ensemble that do not have a TSS score of at least 0.7. Briefly, the only model that never failed was RF, contrasting with MaxEnt that was the one that failed the most. The complete set of model evaluation metrics across species and models are provided in Supplementary Table 3, and the range of scores for the various models that made up the ensembles in Supplementary Figure 4A.
Rather than filtering the individual Random Forest cover models based on TSS tests, all of the outputs from the validation portion of the 1,000 model runs were averaged together by pixel and the ranges in accuracy were documented (Supplementary Figure 4B). In general, by comparing percent cover results and model accuracy for all species, we observed that the Random Forest models underperformed at predicting areas with cover higher than 30%, and almost never predicted model covers greater than 60% even though observed values of 100% cover exist in the dataset (see summary of Supplementary Figure 4B). Thus, actual percent cover throughout the Eastern Canadian Arctic is likely much higher than the results shown here.
As seen in Supplementary Figure 5, S. latissima was the one with higher values of standard errors given the proportion of models that had <0.7 TSS values (Supplementary Figure 4A). For the Laminariaceae there is near perfect agreement within the ensemble models about the habitat suitability in the deeper waters of the Labrador Sea and Baffin Bay. With the exception of L. solidungula, there is also good model agreement about the habitat suitability along the coast of Labrador.
Discussion
In this study we show the predicted current status and changes in suitable habitat and cover of the main kelp forest forming species in the Eastern Canadian Arctic. In general, most of the coastal regions of the Eastern Canadian Arctic, with the exception of James Bay, provide suitable habitat for kelp under current environmental conditions. Thus, the potential extent of Arctic kelps is much greater than the breadth of the heretofore sampled sites. This potential suitable habitat of over 312,000 km2 in the Eastern Canadian Arctic alone would significantly increase the current estimate for subtidal macroalgae in the entire Arctic, which is 675,000 km2, depth clipped 0–30 m (Krause-Jensen et al., 2020). It would also likely increase the estimated global distribution of kelp forests, which is currently estimated to be between 1,469,900 and 2,500,000 km2 (Krause-Jensen and Duarte, 2016; Filbee-Dexter, 2020; Jayathilake and Costello, 2020), because these global estimates have underestimated the extent of suitable habitat in the Arctic. This large extent of suitable habitat is likely due to both the long coastline created by the many islands, fjords and bays within the archipelago and surrounding continental coasts (representing ∼10% of the world’s coastlines depending on the resolution used), and the wide shallow coastal zone that often extends 10 s of km from the shoreline within the depth limits of macroalgae. Numerous uncertainties surround this estimate and prevent direct comparisons between global models, but we have considerable data for this region that has been added. Our results highlight the importance of including this vast region – which has not been counted in global models - in our understanding and assessments of the global status and trends of the world’s kelp forests.
There is strong evidence that the kelp extent in the Eastern Canadian Arctic is undergoing significant changes. Suitable habitat is predicted to increase under an extreme climate change scenario (RCP 8.5) for most of the modeled species, except for the Arctic endemic L. solidungula, which is predicted to lose a considerable proportion of suitable habitat in most of this region, giving place to species such as S. latissima that are not exclusively Arctic species and are also distributed widely in more temperate regions. We predicted up to 60% cover in areas of suitable habitat in the present, with changes in patterns of cover under future scenarios varying by kelp species, ranging from 40% gain to 40% loss in cover. Models show cover loss to be greatest for Laminariaceae spp. mainly due to the associated loss predicted for L. solidungula. Much smaller net average changes in cover (from −2% to +2%) are predicted as changes among areas largely balance out.
Variables
Sea surface temperature and ice thickness were retained for most of the species and algorithms used, contributing up to 48% to species model predictions. This is in agreement with other studies showing that temperature is one of the main predictors that contribute significantly to explaining kelp distribution (Assis et al., 2016; Jayathilake and Costello, 2020; Krause-Jensen et al., 2020). Kelps are sensitive to warming temperatures, which can often be a stressor at warm range edges along temperate coasts (Duarte et al., 2018; Wernberg et al., 2018; Filbee-Dexter et al., 2021), and we have shown that, in our study, L. solidungula was the species with the greatest sensitivity to higher temperatures (which translates later into the species having the highest predicted loss in area). However, many kelp species in the Arctic experience temperatures close to their lower thermal limit and demonstrate improved recruitment and growth under 1–3°C increases (Filbee-Dexter et al., 2019). Such responses will vary across kelp species and subpopulations (Bringloe et al., 2020), with more cold-adapted species, such as A. esculenta and L. solidungula, becoming limited when temperatures exceed 10°C. Changes in temperature translate into loss of sea ice, but it is difficult to predict with certainty how these changes will affect kelp given that the rate of change in sea ice extension may be collectively underestimated by climate models (Peng et al., 2020). In addition to the direct relationship with warming, the presence of sea ice can have a direct mechanical effect on kelp. Sea ice thickness can limit distribution due to ice abrasion and changes in light exposure (Conlan et al., 1998; Wiencke, 2009; Clark et al., 2013). Our results show that L. solidungula is the species with greatest tolerance to the presence of ice and regions where ice persists under climate change scenarios are likely where this species will find refugia. Although we used a common depth cut-off in the distribution models, the presence of thick sea ice has been shown to limit the lower depth limit of kelps (Krause-Jensen et al., 2012), potentially resulting in overestimation of areal extents in the more northern regions in our study area.
Salinity was also among the top three variables explaining the distribution of kelp. Melting ice and glaciers can reduce salinity and this increase in fresh water can be an important stressor for kelp (Spurkland and Iken, 2011; Traiger and Konar, 2018), negatively affecting osmotic and ionic levels as well as the photosynthetic apparatus (Kirst, 1990; Kirst and Wiencke, 1995). This stress can also affect early life stages, and thus the complete kelp life cycle (Lind and Konar, 2017). Consequently, kelp populations could be highly impacted by the predicted changes in salinity in the Hudson Bay region under climate change scenarios, given that it is known that river discharge in the region can influence the system (Déry et al., 2018).
A considerable proportion of the percent cover for all species was also explained by iron (up to 29% of variable importance). Iron is an essential nutrient for primary production and may be a limiting factor in marine systems, including macroalgae (Price, 1968; Johnson et al., 1997; Anton et al., 2018). For consistency, the same environmental variables were used in both modeling approaches when results from the two methodologies were merged. However, the scale at which processes act may differ, affecting the most relevant variables explaining suitability as compared to cover. We think that iron may play an important role in predicting abundance (% cover) at a local scale but have less of a role in predicting the general distribution of the species (at a broader scale). Iron deficiency can decrease growth of certain algae (Miller et al., 2016; Shao et al., 2020; and references therein). However, iron-related experimental work has been best studied for phytoplankton, and much less-so for multicellular macroalgae (Suzuki et al., 1995; Miller et al., 2014). That being said, iron has been shown to play a very important role in the growth, reproduction, gametogenesis, and pigment synthesis of Laminaria and Saccharina spp. (e.g., Motomura and Sakai, 1984; Suzuki et al., 1994; Lewis et al., 2013; Iwai et al., 2015).
Originally, there were variables that were not retained in the models since they were highly correlated (e.g., phosphate was highly correlated to nitrates). Thus, PAR, dissolved oxygen and nitrate could also explain the distribution/cover of kelp, but their roles cannot be distinguished from the other variables.
Habitat Suitability
A northward expansion of habitat suitability is predicted for all species, except for L. solidungula. This general pattern can be largely explained by the increase in temperature and decrease of sea ice to the north, creating more suitable habitat. This northward expansion is in accordance with historical trends and models for subtidal Arctic macroalgae with past environmental predictions as shown by Krause-Jensen et al. (2020). They predicted that comparing past (1940–1950) with present (2000–2017) conditions would show a gain in habitat suitability in our study area, mainly situated in northern Hudson Bay and around Baffin Island. This seems to be the general trend from the past to the present, with further northward expansion in the future, as we show here, potentially accelerating due to global change-related temperature increases. However, although not considered in our region of study, local stressors and regional variations (and not only global drivers) may affect kelp forest dynamics (Krumhansl et al., 2016; Krause-Jensen et al., 2020).
The case of L. solidungula differs from the other kelps as models predict a very high loss of area of suitable habitat (−79% suitable habitat in 2100 compared to predicted present habitat). A possible explanation is that L. solidungula is a truly Arctic species occurring in cold water masses near the freezing point (Hooper, 1984) and even the most southern populations are adapted to small annual temperature ranges (Müller et al., 2009). Our results for L. solidungula agree with the work by Müller et al. (2009), who predicted extensions in northern areas without permanent ice cover and retreats in Hudson Bay and southern Baffin Island, Labrador and Newfoundland. The other three species display broader distribution patterns that could allow them to have more plastic physiological responses to warmer temperatures (Bartsch et al., 2008). In addition, L. solidungula can withstand light limitation for long periods (Dunton and Schell, 1986), depending only on photosynthetic radiation during ice break-up at intermediate ice concentrations (Bonsell and Dunton, 2018). Hence, light would not be a limiting factor for its predicted northward extension whereas the other species would benefit from earlier ice break-ups for their photosynthetic radiation requirements. At the same time, as an Arctic endemic species predicted to lose habitat in more southern regions, L. solidungula may not have many other northern locations to where it could expand.
In general, James Bay was not predicted to provide suitable habitat for any of the modeled kelp species, which may be explained by the particularities of the region relative to the rest of the assessment area. James Bay is characterized by considerable freshwater runoff from the surrounding land mass, together with a great amount of river borne sediments, with mud bottoms dominating most of the bay (Stewart and Lockhart, 2005; Nozais et al., 2021). It is generally shallow (rarely deeper than 50 m) and has historically had vast subtidal meadows of eelgrass, Zostera marina, along its coastlines (Stewart and Lockhart, 2005). Current and future salinity conditions likely play a major role in creating environmental conditions that are currently and will not in the future be suitable for kelp. As highlighted in the results and in Section “Variables,” salinity is one of the most important variables that explain kelp distribution, and kelp tend to have limited development and survival in regions with low salinity (Taylor, 1954).
Only Arctic populations of kelp species were included as model inputs, which could be a factor affecting the predicted patterns. The four kelp species considered in this study are known to have a boreal/Arctic distribution (Filbee-Dexter et al., 2019; Wernberg et al., 2019). Modeling populations that are adapted to colder environments should more accurately predict the distribution of these algae in the study region. One assumption of SDM is that species observations are suited to the model and aim of the study (Guisan et al., 2017). In other words, selection criteria for species observations must correspond to the question to be addressed. We wished to make predictions of the current distribution pattern of Arctic populations and in the future under a global change scenario. Marine benthic ecosystems in the Arctic may have evolved as Arctic assemblages rather than being an extension of cold-tolerant flora from temperate ecoregions (Bringloe et al., 2020). Given that Arctic macroalgae seem to be characterized by populations that are genetically distinct from conspecifics in less northern areas (Bringloe et al., 2020), focusing on Arctic populations seemed the logical choice.
Cover
Net changes in kelp cover through time generally balanced out in our predictions (increasing in some regions while decreasing in others at a similar pace and extension). This suggests that although the overall suitable habitat for kelp is expanding, local or regional drivers of kelp abundance in the Eastern Canadian Arctic are predicted to display a more mixed response to climate change. Interestingly, the regions where all species are predicted to gain some percent cover is in the northern sections of Baffin Island, where predictions of climate change in the Eastern Arctic region are considered to change less drastically compared to the other locations in the study regions. For example, the decline in sea ice cover has been relatively slow over the past few decades (Stammerjohn et al., 2012), meaning this region could act as a refugia for kelp and thus is likely important for endemic species.
Kelp cover can be a proxy measure for kelp biomass, individual size and productivity. The pattern of regional increases and decreases in abundance agrees with trends at a global scale from a time series of kelp abundance over the past half-century described by Krumhansl et al. (2016). They found no detectable change in kelp abundance in 35% of the global ecoregions assessed in their study, and decreases in kelp abundance for 38% of ecoregions, with proportional changes in the Arctic ecoregions they examined being positive but very close to zero. Nevertheless, Arctic ecosystems were largely underrepresented in the ecoregions included in this global analysis (only Beaufort Sea and East Greenland Shelf were among the 34 ecoregions examined), making it difficult to know if this pattern is applicable to our assessment region. In our study area, Laminariaceae spp. were predicted to decline the most in average cover (−2%). This could be because L. solidungula, which will experience more suboptimal conditions, is driving the overall trend for Laminariaceae spp. In contrast, our estimations of little to no change in kelp cover do not agree with those of increased kelp abundance in the Arctic measured in the field (Krause-Jensen et al., 2012). It is possible that the geographic and oceanographic complexity of the region, with resulting gradients in nutrients, salinity, sea ice polynyas and varying seafloor substrata create a complex regional pattern of kelp abundance in the eastern Canadian Arctic, that results in localized regions of gains and losses in abundance.
Model Limitations
We chose ensemble models to use a more inclusive methodology that uses the average from a set of models rather than results from any single model. We found this approach to be the most robust for addressing our questions, although every model has its limitations to consider. SDM is a correlative approach incorporating only environmental information related to the species’ known occurrence. This, in some ways, disregards the physiology of the species. Work by Gamliel et al. (2020) has suggested that when physiology is incorporated in SDMs, model predictions display smaller changes in distributions, translating this into smaller range shifts than previous predictions. This further supports our choice of using only Arctic distributional data. One method of including organismal physiology in this type of analysis is by integrating macroalgal phenology to improve predictions (Chefaoui et al., 2019), but this was beyond the scope of the current work.
For our study, we also used different methodologies, each with distinct assumptions and considerations that need to be taken into account, to address the two different aspects of distribution and cover. For example, the exact regions and data points used differed between methodologies. Because the ensemble model used presence data and environmental layers for the full Arctic range, whereas the Random Forest models were restricted to abundance data that were collected in situ from sites in the study area accessible by divers, the latter did not include data from areas with high ice thickness. In contrast, data for the ensemble models included museum records from CANA that specifically sampled from under the ice. In our study, the importance of different environmental layers varied depending on the modeling approach. These differences can be related to the scale at which species respond to the environment, and thus the resolution at which the species - environment relationship is analyzed can confound comparisons (Mertes and Jetz, 2018). In addition, the extent of the study area can change the importance of an environmental variable (Nyström Sandman et al., 2013; Guisan et al., 2017). Thus, the differences found between our two modeling approaches can be related to the scale of the process being modeled (cover predicting results at a local scale vs. habitat suitability predicting at a regional/global scale).
Low performance of some models can be explained by these kelp species having widespread distributions. Possible local and/or regional adaptations can result in poorer performing models since more specific responses (at the physiological level for example) can be related to processes happening in the local environment (in a polar environment for example) (Stockwell and Peterson, 2002; Nyström Sandman et al., 2013). We endeavored to minimize this effect by only using occurrence points from Arctic populations (see above), although some models nonetheless had low performance. This discrepancy could be related to the relationship between distribution extent and niche ranges, since better predictions are expected from species with narrow niches (Nyström Sandman et al., 2013). Another explanation could be related to the diversity of algorithms and base assumptions for each model included in the ensemble method. The data included in each model (occurrence data points and number of pseudo-absences/background) was the same for all algorithms to standardize inputs. However, the number of absences, pseudo-absences, or background data that are appropriate for inclusion in a given model has a degree of uncertainty since pseudo-absences do not correspond to true absences, and the assumptions behind each model can influence outcomes (Sillero, 2011).
Perspectives
Kelps are foundation species that form important marine habitats. As such, the predicted distribution of these algae not only highlights the distribution of this foundation species group, but also underlines their likely ecological importance in this under-studied region. The predicted expansion of kelp species to more northern regions in the Arctic may thus create new opportunities to the potential benefit of other species that may arrive through range expansion or as novel introductions (i.e., non-indigenous species). Changes in habitat and cover of these species may also trigger changes to the entire ecosystem since they are very important food and habitat-provision resources for many benthic organisms (Dunton and Schell, 1986; Debenham, 2005). Effects could be extended beyond where kelps grow. For example, a large proportion of kelp production is exported to deeper waters (estimated at 82% of the primary productivity), becoming a very important food source where it settles and potentially plays an important role in marine carbon sequestration, calculated to exceed that sequestered by angiosperms in coastal habitats (Krause-Jensen and Duarte, 2016; Krause-Jensen et al., 2018).
Native boreal, non-indigenous, and invasive species could likely expand their ranges as these high-latitude regions become more suitable due to global change (Goldsmit et al., 2020) such that species from temperate regions are expected to have greater success in establishing if they are introduced to high-latitude regions (Krause-Jensen and Duarte, 2014). In addition, a recent horizon-scanning exercise for marine invasive species in the Hudson Bay complex identified macroalgae as one of the taxonomic groups with highest risk of invasion in the region (Goldsmit et al., 2021). Invasive macroalgae have been found to produce ecological impacts even when they are outside of their thermal range of origin (at the lower limit of the known minimum temperatures in their native ranges) (Bennett et al., 2021). Together, these characteristics suggest that the region could face major risks in the near future.
More than just environmental factors can affect kelp presence and abundance (our models show regions of habitat suitability and cover considering only the relationship with environmental predictors). These predictions could be highly affected by biological factors and interactions such as grazing and kelp colonization. The presence of herbivores such as urchins can greatly modify the prevalence of kelp (Estes and Duggins, 1995), but grazing vulnerability varies according to kelp species, size, and age, among other factors (Gagnon et al., 2005; Ng and Micheli, 2020). Additionally, kelp are generally considered to have a restricted dispersal capacity modified by ocean circulation and currents (Brennan et al., 2014), although many factors related to the environmental conditions, time and disturbance may play a very important role in dispersal and colonization (Reed et al., 1992, 1997). These processes are highly variable among kelp species; for example, A. esculenta is considered to be more of an opportunistic species, is capable of long distance dispersal, and is often the first species to colonize disturbed sites (Vadas, 1968; Himmelman et al., 1983). Colonization of newly suitable habitat by kelp forests, therefore, requires that kelp propagules be transported to these areas, and this process will vary based on source population proximity and species composition, as well as environmental factors and direction of the currents. Including biological processes such as herbivory and recruitment in modeled predictions was beyond the scope of our work, hence the importance of interpreting our analyses only in terms of habitat suitability predictions which consider environmental variables. Future work should focus on combining our predicted habitat suitability with an examination of these important biological processes, in order to move from habitat suitability to a possible future realized extent of kelp forests in the Eastern Canadian Arctic.
Conclusion
The contemporary global decline of kelp forests was the main subject of a recent horizon scan of global biological conservation issues (Sutherland et al., 2020). In this context, the results of our study highlight the need for appropriate management actions for this natural resource, as suggested by other researchers (Filbee-Dexter et al., 2019; Krause-Jensen et al., 2020). There remains, however, a great need for the collection of additional field data in the Canadian Arctic (including the Eastern Canadian Arctic) to better understand the current extent of kelp forests in the region. However, our findings highlight that kelp in this region could account for a considerable proportion of the total kelp forest area globally, but that it has been historically underestimated. Although current predictions are somewhat uncertain, the possible expansion of kelp forests should provide new habitats for fish and other marine organisms, increased carbon storage, and a suite of other ecosystem services along Arctic coastlines. This finding may be a silver lining of a changing climate because the appearance of kelp forests along the coastlines of the Eastern Canadian Arctic will bring with them an increase in primary productivity and potentially other commercially valuable species (Filbee-Dexter et al., 2019; Eger et al., 2021). It may therefore be advisable that marine resource managers in the areas where increased habitat suitability of kelp is predicted be ready to manage and develop this resource, whether it be due to increases to existing stocks or the novel appearance of these species and the ecosystems for which they are a foundation.
Data Availability Statement
The original contributions presented in the study are included in the article/Supplementary Material, further inquiries can be directed to the corresponding author. The R code written for the habitat suitability (ensemble models) and cover (Random Forest) may be found at: https://github.com/robwschlegel/ArcticKelp.
Author Contributions
JG and RS contributed substantially to this work and share first authorship, they both contributed to the formal analysis, development of the methodology, programming, and results visualization. RS was in charge of the implementation of the computer code, testing, and maintaining the code components. JG was in charge of writing the original draft of the manuscript. All authors contributed to the conceptualization and to the research investigation process at different levels and/or moments. KF-D, KM, AS, KH, and CWM produced data and participated in data curation. KF-D, LJ, CJM, AS, CWM, KH, and PA provided resources. KF-D, LJ, CWM, KH, and PA were in charge of funding acquisition and supervision. KF-D and PA were the project administrators. All authors contributed to the final version of the manuscript.
Funding
This work was supported by ArcticNet (P101 ArcticKelp), Fisheries and Oceans Canada Arctic Climate Change Adaptation Strategy, Arctic Science and Aquatic Invasive Species Monitoring and Research Funds, the Natural Sciences and Engineering Research Council (NSERC), NRCan Polar Continental Shelf Program Support, Canadian Aquatic Invasive Species Network (CAISN), the Nunavut Marine Region Wildlife Management Board (NWMB), Quebec-Ocean, and the Ocean Frontier Institute through an award from the Canada First Research Excellence Fund, the Marine Environmental Observation, Prediction and Response Network of Centres of Excellence’s (MEOPAR-NCE) Southampton Island Marine Ecosystem Project, and the Belmont Forum–BiodivERsA’s De-icing of Arctic Coasts: critical or new opportunities for marine biodiversity and Ecosystem Services (ACCES). KF-D was supported by the Australian Research Council (DE190100692).
Conflict of Interest
The authors declare that the research was conducted in the absence of any commercial or financial relationships that could be construed as a potential conflict of interest.
Publisher’s Note
All claims expressed in this article are solely those of the authors and do not necessarily represent those of their affiliated organizations, or those of the publisher, the editors and the reviewers. Any product that may be evaluated in this article, or claim that may be made by its manufacturer, is not guaranteed or endorsed by the publisher.
Acknowledgments
We thank K. Shoenrock, I. Hendriks, M. Ronowicz, and G. Saunders for sharing species occurrence information. We also thank Pauline Fortin for helping to collate museum species data and Gabrielle Martineau for helping to extract species identification and cover data from video recordings, local people and organizations for providing sampling support: D. Kaludjak, K. Lindell, A. Williams, and J. Williams (community of Iqaluit); C. Alaku, C. Kadjulik, K. Ningiurluut, K. Okituk, C. Okituk, L. Yuliusie, and A. Keatainak (community of Salluit); A. Allianaq, T. R. Avingaq, G. Illupaalik, J. Kukkik, A. Kutiq, and T. A. Taqqaugaq (communities of Igloolik and Hall Beach); Mittimatalik, Igloolik, Hall Beach, and Amaruq Hunters and Trappers; the Qaqqalik Landholding Corporation; Churchill Northern Studies Center; Nunavut Research Institute and the Nunavut Fisheries Association, and the people that helped in the field: professional divers and people in charge of logistics and who helped taking samples and analyzing in the lab. We recognize the Traditional Inhabitants of both ceded and unceded territory on which this research was conducted, including the Mittimatalingmiut (Pond Inlet), other Nunavummiut (Nunavut), and Nunavimmiut (Nunavik) Inuit. We acknowledged that gains in contemporary knowledge invariably build on a history of race, gender, and sexual orientation discrimination.
Supplementary Material
The Supplementary Material for this article can be found online at: https://www.frontiersin.org/articles/10.3389/fmars.2021.742209/full#supplementary-material
Footnotes
References
Adey, W. H., and Hayek, L. A. C. (2011). Elucidating marine biogeography with macrophytes: quantitative analysis of the North Atlantic supports the thermogeographic model and demonstrates a distinct subarctic region in the Northwestern Atlantic. Northeast. Nat. 18, 1–128. doi: 10.1656/045.018.m801
Allouche, O., Tsoar, A., and Kadmon, R. (2006). Assessing the accuracy of species distribution models: prevalence, kappa and the true skill statistic (TSS). J. Appl. Ecol. 43, 1223–1232. doi: 10.1111/j.1365-2664.2006.01214.x
AMAP (2017). Snow, Water, Ice and Permafrost in the Arctic (SWIPA) 2017. Orway: Arctic Monitoring and Assessment Programme (AMAP).
Angilletta, M. J. Jr., Bennett, A. F., Guderley, H., Navas, C. A., Seebacher, F., and Wilson, R. S. (2006). Coadaptation: a unifying principle in evolutionary thermal biology. Physiol. Biochem. Zool. 79, 282–294. doi: 10.1086/499990
Anton, A., Hendriks, I. E., Marba, N., Krause-Jensen, D., Garcias-Bonet, N., and Duarte, C. M. (2018). Iron deficiency in seagrasses and macroalgae in the Red Sea is unrelated to latitude and physiological performance. Front. Mar. Sci. 5:74. doi: 10.3389/fmars.2018.00074
Araújo, M. B., and New, M. (2007). Ensemble forecasting of species distributions. Trends Ecol. Evol. 22, 42–47. doi: 10.1016/j.tree.2006.09.010
Archambault, P., Snelgrove, P. V., Fisher, J. A., Gagnon, J. M., Garbary, D. J., Harvey, M., et al. (2010). From sea to sea: canada’s three oceans of biodiversity. PLoS One 5:e12182. doi: 10.1371/journal.pone.0012182
Assis, J., Araújo, M. B., and Serrão, E. A. (2018a). Projected climate changes threaten ancient refugia of kelp forests in the North Atlantic. Glob. Change Biol. 24, e55–66. doi: 10.1111/gcb.13818
Assis, J., Tyberghein, L., Bosch, S., Verbruggen, H., Serrão, E. A., and De Clerck, O. (2018b). Bio-ORACLE v2. 0: extending marine data layers for bioclimatic modelling. Glob. Ecol. Biogeogr. 27, 277–284. doi: 10.1111/geb.12693
Assis, J., Lucas, A. V., Bárbara, I., and Serrão, E. Á (2016). Future climate change is predicted to shift long-term persistence zones in the cold-temperate kelp Laminaria hyperborea. Mar. Environ. Res. 113, 174–182. doi: 10.1016/j.marenvres.2015.11.005
Austin, M. P. (2002). Spatial prediction of species distribution: an interface between ecological theory and statistical modelling. Ecol. Model. 157, 101–118. doi: 10.1016/S0304-3800(02)00205-3
Bajjouk, T., Rochette, S., Laurans, M., Ehrhold, A., Hamdi, A., and Le Niliot, P. (2015). Multi-approach mapping to help spatial planning and management of the kelp species L. digitata and L. hyperborea: case study of the Molène Archipelago. Brittany. J. Sea Res. 100, 2–21. doi: 10.1016/j.seares.2015.04.004
Ballinger, T. J., Overland, J. E., Wang, M., Bhatt, U. S., Hanna, E., Hanssen-Bauer, I., et al. (2020). Arctic Report Card 2020: surface Air Temperature. United States: NOAA. doi: 10.25923/gcw8-2z06
Barbet-Massin, M., Jiguet, F., Albert, C. H., and Thuiller, W. (2012). Selecting pseudo-absences for species distribution models: how, where and how many? Methods Ecol. Evol. 3, 327–338. doi: 10.1111/j.2041-210X.2011.00172.x
Bartsch, I., Paar, M., Fredriksen, S., Schwanitz, M., Daniel, C., Hop, H., et al. (2016). Changes in kelp forest biomass and depth distribution in Kongsfjorden, Svalbard, between 1996–1998 and 2012–2014 reflect Arctic warming. Polar Biol. 39, 2021–2036. doi: 10.1007/s00300-015-1870-1
Bartsch, I., Wiencke, C., Bischof, K., Buchholz, C. M., Buck, B. H., Eggert, A., et al. (2008). The genus Laminaria sensu lato: recent insights and developments. Eur. J. Phycol. 43, 1–86. doi: 10.1080/09670260701711376
Bennett, S., Santana-Garcon, J., Marbà, N., Jorda, G., Anton, A., Apostolaki, E. T., et al. (2021). Climate-driven impacts of exotic species on marine ecosystems. Glob. Ecol. Biogeogr. 30, 1043–1055. doi: 10.1111/geb.13283
Bennett, S., Wernberg, T., Arackal Joy, B., de Bettignies, T., and Campbell, A. H. (2015). Central and rear-edge populations can be equally vulnerable to warming. Nat. Commun. 6:10280. doi: 10.1038/ncomms10280
Bluhm, B. A., Gebruk, A. V., Gradinger, R., Hopcroft, R. R., Huettmann, F., Kosobokova, K. N., et al. (2011). Arctic marine biodiversity: an update of species richness and examples of biodiversity change. Oceanography 24, 232–248. doi: 10.5670/oceanog.2011.75
Bonsell, C., and Dunton, K. H. (2018). Long-term patterns of benthic irradiance and kelp production in the central Beaufort Sea reveal implications of warming for Arctic inner shelves. Progr. Oceanogr. 162, 160–170. doi: 10.1016/j.pocean.2018.02.016
Borum, J., Pedersen, M., Krause-Jensen, D., Christensen, P., and Nielsen, K. (2002). Biomass, photosynthesis and growth of Laminaria saccharina in a high-arctic fjord. NE Greenland. Mar. Biol. 141, 11–19. doi: 10.1007/s00227-002-0806-9
Bosch, S., Tyberghein, L., Deneudt, K., Hernandez, F., and De Clerck, O. (2018). In search of relevant predictors for marine species distribution modelling using the MarineSPEED benchmark dataset. Divers. Distrib. 24, 144–157. doi: 10.1111/ddi.12668
Brennan, G., Kregting, L., Beatty, G. E., Cole, C., Elsäßer, B., Savidge, G., et al. (2014). Understanding macroalgal dispersal in a complex hydrodynamic environment: a combined population genetic and physical modelling approach. J. R. Soc. Interf. 11:20140197. doi: 10.1098/rsif.2014.0197
Bringloe, T. T., Verbruggen, H., and Saunders, G. W. (2020). Unique biodiversity in Arctic marine forests is shaped by diverse recolonization pathways and far northern glacial refugia. Proc. Natl. Acad. Sci. U. S. A. 117, 22590–22596. doi: 10.1073/pnas.2002753117
Brown, J. L., Bennett, J. R., and French, C. M. (2017). SDMtoolbox 2.0: the next generation Python-based GIS toolkit for landscape genetic, biogeographic and species distribution model analyses. PeerJ. 5:e4095. doi: 10.7717/peerj.4095
Chefaoui, R. M., Serebryakova, A., Engelen, A. H., Viard, F., and Serrão, E. A. (2019). Integrating reproductive phenology in ecological niche models changed the predicted future ranges of a marine invader. Divers. Distrib. 25, 688–700. doi: 10.1111/ddi.12910
Clark, G. F., Stark, J. S., Johnston, E. L., Runcie, J. W., Goldsworthy, P. M., Raymond, B., et al. (2013). Light-driven tipping points in polar ecosystems. Glob. Change Biol. 19, 3749–3761. doi: 10.1111/gcb.12337
Conlan, K. E., Lenihan, H. S., Kvitek, R. G., and Oliver, J. S. (1998). Ice scour disturbance to benthic communities in the Canadian High Arctic. Mar. Ecol. Progr. Ser. 166, 1–16. doi: 10.3354/meps166001
Debenham, C. (2005). Multiple stable isotopic analyses of the Boulder Patch, a High Arctic Kelp Community: trophic and Temporal Perspectives. United States: University of Alaska Fairbanks. 75.
Derksen, C., Burgess, D., Duguay, C., Howell, S., Mudryk, L., Smith, S., et al. (2019). “Changes in snow, ice, and permafrost across Canada” in Canada’s Changing Climate Report. eds E. Bush and D. S. Lemmen (Canada: Government of Canada). 194–260. doi: 10.4095/308279
Déry, S. J., Stadnyk, T. A., MacDonald, M. K., Koenig, K. A., and Guay, C. (2018). Flow alteration impacts on Hudson Bay river discharge. Hydrol. Proces. 32, 3576–3587. doi: 10.1002/hyp.13285
Duarte, B., Martins, I., Rosa, R., Matos, A. R., Roleda, M. Y., Reusch, T. B., et al. (2018). Climate change impacts on seagrass meadows and macroalgal forests: an integrative perspective on acclimation and adaptation potential. Front. Mar. Sci. 5:190. doi: 10.3389/fmars.2018.00190
Dunton, K. H., and Schell, D. M. (1986). A seasonal carbon budget for the kelp Laminaria solidungula in the Alaskan high Arctic. Mar. Ecol. Progr. Ser. 31, 57–66. doi: 10.3354/meps031057
Eger, A., Marzinelli, E., Baes, R., Blain, C., Blamey, L., Carnell, P., et al. (2021). The economic value of fisheries, blue carbon, and nutrient cycling in global marine forests. Ecoevorxiv doi: 10.32942/osf.io/n7kjs
CrossRef Full Text | Available online at: https://ecoevorxiv.org/n7kjs/ (accessed June 15, 2021).
Estes, J. A., and Duggins, D. O. (1995). Sea otters and kelp forests in Alaska: generality and variation in a community ecological paradigm. Ecol. Monogr. 65, 75–100. doi: 10.2307/2937159
Filbee-Dexter, K. (2020). Ocean forests hold unique solutions to our current environmental crisis. One Earth 2, 398–401. doi: 10.1016/j.oneear.2020.05.004
Filbee-Dexter, K., MacGregor, K. A., Lavoie, C., Garrido, I., Goldsmit, J., De La Guardia, L. C., et al. (2021). Sea ice and substratum shape extensive kelp forests in the Canadian Arctic. EcoEvoRxiv [Preprint]. doi: 10.32942/osf.io/t82cf
Filbee-Dexter, K., Wernberg, T., Fredriksen, S., Norderhaug, K. M., and Pedersen, M. F. (2019). Arctic kelp forests: diversity, resilience and future. Glob. Planet. Change 172, 1–14. doi: 10.1016/j.gloplacha.2018.09.005
Flato, G., Gillett, N., Arora, V., Cannon, A., and Anstey, J. (2019). “Modelling future climate change. Chapter 3” in Canada’s Changing Climate Report. eds E. Bush and D. S. Lemmen (Ottawa: Government of Canada). 74–111. doi: 10.4095/327808
Frederick, J. M., Thomas, M. A., Bull, D. L., Jones, C. A., and Roberts, J. D. (2016). The Arctic Coastal Erosion Problem. United States: Sandia National Laboratories. doi: 10.2172/1431492
Fritz, M., Vonk, J. E., and Lantuit, H. (2017). Collapsing arctic coastlines. Nat. Clim. Change 7, 6–7. doi: 10.1038/nclimate3188
Gagnon, P., Johnson, L. E., and Himmelman, J. H. (2005). Kelp patch dynamics in the face of intense herbivory: stability of Agarum clathratum (Phaeophyta) stands and associated flora on urchin barrens. J. Phycol. 41, 498–505. doi: 10.1111/j.1529-8817.2005.00078.x
Gamliel, I., Buba, Y., Guy-Haim, T., Garval, T., Willette, D., Rilov, G., et al. (2020). Incorporating physiology into species distribution models moderates the projected impact of warming on selected Mediterranean marine species. Ecography 43, 1090–1106. doi: 10.1111/ecog.04423
García-Roselló, E., Guisande, C., Manjarrés-Hernández, A., González-Dacosta, J., Heine, J., Pelayo-Villamil, P., et al. (2015). Can we derive macroecological patterns from primary Global Biodiversity Information Facility data? Glob. Ecol. Biogeogr. 24, 335–347. doi: 10.1111/geb.12260
Goldsmit, J., McKindsey, C. W., Schlegel, R. W., Stewart, D. B., Archambault, P., and Howland, K. L. (2020). What and where? Predicting invasion hotspots in the Arctic marine realm. Glob. Change Biol. 26, 4752–4771. doi: 10.1111/gcb.15159
Goldsmit, J., McKindsey, C. W., Stewart, D. B., and Howland, K. L. (2021). Screening for high-risk marine invaders in the Hudson Bay region, Canadian Arctic. Front. Ecol. Evol. 9:61. doi: 10.3389/fevo.2021.627497
Gorman, D., Bajjouk, T., Populus, J., Vasquez, M., and Ehrhold, A. (2013). Modeling kelp forest distribution and biomass along temperate rocky coastlines. Mar. Biol. 160, 309–325. doi: 10.1007/s00227-012-2089-0
Guisan, A., Thuiller, W., and Zimmermann, N. E. (2017). Habitat Suitability and Distribution Models: with Applications in R. Cambridge: Cambridge University Press. doi: 10.1017/9781139028271
Halpern, B. S., Frazier, M., Potapenko, J., Casey, K. S., Koenig, K., Longo, C., et al. (2015). Spatial and temporal changes in cumulative human impacts on the world’s ocean. Nat. Commun. 6, 1–7. doi: 10.1038/ncomms8615
Hao, T., Elith, J., Guillera-Arroita, G., and Lahoz-Monfort, J. J. (2019). A review of evidence about use and performance of species distribution modelling ensembles like BIOMOD. Divers. Distrib. 25, 839–852. doi: 10.1111/ddi.12892
Hernandez, P. A., Graham, C. H., Master, L. L., and Albert, D. L. (2006). The effect of sample size and species characteristics on performance of different species distribution modeling methods. Ecography 29, 773–785. doi: 10.1111/j.0906-7590.2006.04700.x
Himmelman, J. H., Cardinal, A., and Bourget, E. (1983). Community development following removal of urchins, Strongylocentrotus droebachiensis, from the rocky subtidal zone of the St. Lawrence Estuary, eastern Canada. Oecologia 59, 27–39.
Hochheim, K. P., and Barber, D. G. (2014). An update on the ice climatology of the Hudson Bay system. Arct. Antarct. Alp. Res. 46, 66–83. doi: 10.1657/1938-4246-46.1.66
Hooper, R. G. (1984). Functional adaptations to the polar environment by the Arctic kelp. Laminaria solidungula. Br. Phycol. J. 19:194.
Hop, H., Kovaltchouk, N. A., and Wiencke, C. (2016). Distribution of macroalgae in Kongsfjorden. Svalbard. Polar Biol. 39, 2037–2051. doi: 10.1007/s00300-016-2048-1
Hwang, B., Aksenov, Y., Blockley, E., Tsamados, M., Brown, T., Landy, J., et al. (2020). Impacts of climate change on Arctic sea ice. Mar. Clim. Change Impacts Partners. Sci. Rev. 2020, 208–227.
Iwai, H., Fukushima, M., Motomura, T., Kato, T., and Kosugi, C. (2015). Effect of iron complexes with seawater extractable organic matter on oogenesis in gametophytes of a brown macroalga (Saccharina japonica). J. Appl. Phycol. 27, 1583–1591. doi: 10.1007/s10811-014-0479-z
Jayathilake, D. R., and Costello, M. J. (2020). A modelled global distribution of the kelp biome. Biol. Conserv. 252:108815. doi: 10.1016/j.biocon.2020.108815
Jiménez-Valverde, A., and Lobo, J. M. (2007). Threshold criteria for conversion of probability of species presence to either–or presence–absence. Acta Oecol. 31, 361–369. doi: 10.1016/j.actao.2007.02.001
Johnson, K. S., Gordon, R. M., and Coale, K. H. (1997). What controls dissolved iron concentrations in the world ocean? Mar. Chem. 57, 137–161. doi: 10.1016/S0304-4203(97)00043-1
Jørgensen, O. A., Hvingel, C., Møller, P. R., and Treble, M. A. (2005). Identification and mapping of bottom fish assemblages in Davis Strait and southern Baffin Bay. Can. J. Fish. Aquat. Sci. 62, 1833–1852. doi: 10.1139/f05-101
Kaschner, K., Kesner-Reyes, K., Garilao, C., Rius-Barile, J., Rees, T., and Froese, R. (eds) (2016). AquaMaps: predicted range Maps for Aquatic Species. Available online at: www.aquamaps.org, Version 08/2016 (accessed August 2017).
Kirst, G. O. (1990). Salinity tolerance of eukaryotic marine algae. Annu. Rev. Plant Biol. 41, 21–53. doi: 10.1146/annurev.pp.41.060190.000321
Kirst, G. O., and Wiencke, C. (1995). Ecophysiology of polar algae. Oceanogr. Literat. Rev. 12:1094. doi: 10.1111/j.0022-3646.1995.00181.x
Konar, B., Edwards, M. S., Bland, A., Metzger, J., Ravelo, A., Traiger, S., et al. (2017). A swath across the great divide: kelp forests across the Samalga Pass biogeographic break. Cont. Shelf Res. 143, 78–88. doi: 10.1016/j.csr.2017.06.007
Krause-Jensen, D., Archambault, P., Assis, J., Bartsch, I., Bischof, K., Filbee-Dexter, K., et al. (2020). Imprint of climate change on pan-Arctic marine vegetation. Front. Mar. Sci. 7:617324. doi: 10.3389/fmars.2020.617324
Krause-Jensen, D., and Duarte, C. M. (2016). Substantial role of macroalgae in marine carbon sequestration. Nat. Geosci. 9, 737–742. doi: 10.1038/ngeo2790
Krause-Jensen, D., Lavery, P., Serrano, O., Marbà, N., Masque, P., and Duarte, C. M. (2018). Sequestration of macroalgal carbon: the elephant in the Blue Carbon room. Biol. Lett. 14:20180236. doi: 10.1098/rsbl.2018.0236
Krause-Jensen, D., and Duarte, C. M. (2014). Expansion of vegetated coastal ecosystems in the future Arctic. Front. Mar. Sci. 1:77. doi: 10.3389/fmars.2014.00077
Krause-Jensen, D., Marbà, N., Olesen, B., Sejr, M. K., Christensen, P. B., Rodrigues, J., et al. (2012). Seasonal sea ice cover as principal driver of spatial and temporal variation in depth extension and annual production of kelp in Greenland. Glob. Change Biol. 18, 2981–2994. doi: 10.1111/j.1365-2486.2012.02765.x
Krumhansl, K. A., Okamoto, D. K., Rassweiler, A., Novak, M., Bolton, J. J., Cavanaugh, K. C., et al. (2016). Global patterns of kelp forest change over the past half-century. Proc. Natl. Acad. Sci. U. S. A. 113, 13785–13790. doi: 10.1073/pnas.1606102113
Lane, C. E., Mayes, C., Druehl, L. D., and Saunders, G. W. (2006). A multi-gene molecular investigation of the kelp (Laminariales, Phaeophyceae) supports substantial taxonomic re-organization. J. Phycol. 42, 493–512. doi: 10.1111/j.1529-8817.2006.00204.x
Lee, R. K. S. (1973). General ecology of the Canadian Arctic benthic marine algae. Arctic 26, 32–43. doi: 10.14430/arctic2894
Lewis, R. J., Green, M. K., and Afzal, M. E. (2013). Effects of chelated iron on oogenesis and vegetative growth of kelp gametophytes (Phaeophyceae). Phycol. Res. 61, 46–51. doi: 10.1111/j.1440-1835.2012.00667.x
Li, X., and Wang, Y. (2013). Applying various algorithms for species distribution modelling. Integr. Zool. 8, 124–135. doi: 10.1111/1749-4877.12000
Lind, A. C., and Konar, B. (2017). Effects of abiotic stressors on kelp early life-history stages. Algae 32, 223–233. doi: 10.4490/algae.2017.32.8.7
Liu, C., White, M., and Newell, G. (2013). Selecting thresholds for the prediction of species occurrence with presence-only data. J. Biogeogr. 40, 778–789. doi: 10.1111/jbi.12058
Longtin, C. M., and Saunders, G. W. (2015). On the utility of mucilage ducts as a taxonomic character in Laminaria and Saccharina (Phaeophyceae)–the conundrum of S. groenlandica. Phycologia 54, 440–450. doi: 10.2216/15-19.1
Marcelino, V. R., and Verbruggen, H. (2015). Ecological niche models of invasive seaweeds. J. Phycol. 51, 606–620. doi: 10.1111/jpy.12322
McDevit, D. C., and Saunders, G. W. (2010). A DNA barcode examination of the Laminariaceae (Phaeophyceae) in Canada reveals novel biogeographical and evolutionary insights. Phycologia 49, 235–248. doi: 10.2216/PH09-36.1
Melo-Merino, S. M., Reyes-Bonilla, H., and Lira-Noriega, A. (2020). Ecological niche models and species distribution models in marine environments: a literature review and spatial analysis of evidence. Ecol. Model. 415:108837. doi: 10.1016/j.ecolmodel.2019.108837
Mertes, K., and Jetz, W. (2018). Disentangling scale dependencies in species environmental niches and distributions. Ecography 41, 1604–1615. doi: 10.1111/ecog.02871
Miller, E. P., Auerbach, H., Schünemann, V., Tymon, T., and Carrano, C. J. (2016). Surface binding, localization and storage of iron in the giant kelp Macrocystis pyrifera. Metallomics 8, 403–411. doi: 10.1039/C6MT00027D
Miller, E. P., Böttger, L. H., Weerasinghe, A. J., Crumbliss, A. L., Matzanke, B. F., Meyer-Klaucke, W., et al. (2014). Surface-bound iron: a metal ion buffer in the marine brown alga Ectocarpus siliculosus J. Exp. Bot. 65, 585–594. doi: 10.1093/jxb/ert406
Moss, R. H., Edmonds, J. A., Hibbard, K. A., Manning, M. R., Rose, S. K., Van Vuuren, D. P., et al. (2010). The next generation of scenarios for climate change research and assessment. Nature 463, 747–756. doi: 10.1038/nature08823
Motomura, T., and Sakai, Y. (1984). Ultrastructural studies of gametogenesis in Laminaria angustata (Laminariales, Phaeophyta) regulated by iron concentration in the medium. Phycologia 23, 331–343. doi: 10.2216/i0031-8884-23-3-331.1
Mudryk, L. R., Derksen, C., Howell, S., Laliberté, F., Thackeray, C., Sospedra-Alfonso, R., et al. (2018). Canadian snow and sea ice: historical trends and projections. Cryosphere 12, 1157–1176. doi: 10.5194/tc-12-1157-2018
Müller, R., Laepple, T., Bartsch, I., and Wiencke, C. (2009). Impact of oceanic warming on the distribution of seaweeds in polar and cold-temperate waters. Bot. Mar. 52, 617–638. doi: 10.1515/BOT.2009.080
Naimi, B., Hamm, N. A., Groen, T. A., Skidmore, A. K., and Toxopeus, A. G. (2014). Where is positional uncertainty a problem for species distribution modelling? Ecography 37, 191–203. doi: 10.1111/j.1600-0587.2013.00205.x
Ng, C. A., and Micheli, F. (2020). Size-dependent vulnerability to herbivory in a coastal foundation species. Oecologia 193, 199–209. doi: 10.1007/s00442-020-04655-3
Niemi, A., Ferguson, S., Hedges, K., Melling, H., Michel, C., Ayles, B., et al. (2019). “State of Canada’s Arctic seas” in Canadian Technical Report of Fisheries and Aquatic Science. (Canada: Fisheries and Oceans Canada Freshwater Institute).
Nozais, C., Vincent, W. F., Belzile, C., Gosselin, M., Blais, M.-A., Canáriod, J., et al. (2021). The Great Whale River ecosystem: ecology of a subarctic river and its receiving waters in coastal Hudson Bay, Canada. Ecoscience doi: 10.1080/11956860.2021.1926137
Nyberg, B., and Howell, J. A. (2016). Global distribution of modern shallow marine shorelines. Implications for exploration and reservoir analogue studies. Mar. Petrol. Geol. 71, 83–104. doi: 10.1016/j.marpetgeo.2015.11.025
Nyström Sandman, A., Wikström, S. A., Blomqvist, M., Kautsky, H., and Isaeus, M. (2013). Scale-dependent influence of environmental variables on species distribution: a case study on five coastal benthic species in the Baltic Sea. Ecography 36, 354–363. doi: 10.1111/j.1600-0587.2012.07053.x
Peng, G., Matthews, J. L., Wang, M., Vose, R., and Sun, L. (2020). What do global climate models tell us about future arctic sea ice coverage changes? Climate 8:15. doi: 10.3390/cli8010015
Price, C. A. (1968). Iron compounds and plant nutrition. Annu. Rev. Plant Physiol. 19, 239–248. doi: 10.1146/annurev.pp.19.060168.001323
Prinsenberg, S. J. (1986). The circulation pattern and current structure of Hudson Bay. Can. Inland Seas 44, 187–203. doi: 10.1016/S0422-9894(08)70903-6
R Core Team. (2020). R: a Language and Environment for Statistical Computing. Austria: R Foundation for Statistical Computing.
Raybaud, V., Beaugrand, G., Goberville, E., Delebecq, G., Destombe, C., Valero, M., et al. (2013). Decline in kelp in west Europe and climate. PLoS One 8:e66044. doi: 10.1371/journal.pone.0066044
Reed, D. C., Amsler, C. D., and Ebeling, A. W. (1992). Dispersal in kelps: factors affecting spore swimming and competency. Ecology 73, 1577–1585.
Reed, D. C., Anderson, T. W., Ebeling, A. W., and Anghera, M. (1997). The role of reproductive synchrony in the colonization potential of kelp. Ecology 78, 2443–2457.
Riahi, K., Grübler, A., and Nakicenovic, N. (2007). Scenarios of long-term socio-economic and environmental development under climate stabilization. Technol. Forecast. Soc. Change 74, 887–935. doi: 10.1016/j.techfore.2006.05.026
Ronowicz, M., Włodarska-Kowalczuk, M., and Kukliński, P. (2020). Glacial and depth influence on sublittoral macroalgal standing stock in a high-Arctic fjord. Cont. Shelf Res. 194:104045. doi: 10.1016/j.csr.2019.104045
Schindelin, J., Arganda-Carreras, I., Frise, E., Kaynig, V., Longair, M., Pietzsch, T., et al. (2012). Fiji: an open-source platform for biological-image analysis. Nat. Methods 9, 676–682. doi: 10.1038/nmeth.2019
Schoenrock, K. M., Stachnik, Ł, Vad, J., Kamenos, N. A., Pearce, D., Rea, B., et al. (2018). “Distribution of benthic communities in a fjord-marine system in Southwestern Greenland, with focus on algal dominated habitats” in Proceedings of the International Youth Scientific and Practical Conference, “Arctic Research: from Extensive to Integrated Development. (United Kingdom: University of Glasgow).
Shao, Z., Shuai, L., Cheng, H., Wu, Z., You, F., Zhang, H., et al. (2020). Influence of iron and carbon on the occurrence of Ulva prolifera (Ulvophyceae) in the Yellow Sea. Region. Stud. Mar. Sci. 36:101224. doi: 10.1016/j.rsma.2020.101224
Sillero, N. (2011). What does ecological modelling model? A proposed classification of ecological niche models based on their underlying methods. Ecol. Model. 222, 1343–1346. doi: 10.1016/j.ecolmodel.2011.01.018
Silva, P. C. (1991). Nomenclatural remarks on Agarum (Laminariaceae, Phaeophyceae). Japan. J. Phycol. 39, 217–221.
Spence, A. R., and Tingley, M. W. (2020). The challenge of novel abiotic conditions for species undergoing climate-induced range shifts. Ecography 43, 1571–1590. doi: 10.1111/ecog.05170
Spurkland, T., and Iken, K. (2011). Salinity and irradiance effects on growth and maximum photosynthetic quantum yield in subarctic Saccharina latissima (Laminariales, Laminariaceae). Bot. Mar. 54, 355–365. doi: 10.1515/bot.2011.042
Stammerjohn, S., Massom, R., Rind, D., and Martinson, D. (2012). Regions of rapid sea ice change: an inter-hemispheric seasonal comparison. Geophys. Res. Lett. 39. doi: 10.1029/2012GL050874
Starko, S., Gomez, M. S., Darby, H., Demes, K. W., Kawai, H., Yotsukura, N., et al. (2019). A comprehensive kelp phylogeny sheds light on the evolution of an ecosystem. Mol. Phylogenet. Evol. 136, 138–150. doi: 10.1016/j.ympev.2019.04.012
Starko, S., Wilkinson, D. P., and Bringloe, T. T. (2021). Recent global model underestimates the true extent of Arctic kelp habitat. Biol. Conserv. 257:109082. doi: 10.1016/j.biocon.2021.109082
Stewart, D. B., and Lockhart, W. L. (2005). An overview of the Hudson Bay marine ecosystem. Can. Techn. Rep. Fish. Aquat. Sci. 2586:vi + 487.
Stockwell, D. R., and Peterson, A. T. (2002). Effects of sample size on accuracy of species distribution models. Ecol. Model. 148, 1–13. doi: 10.1016/S0304-3800(01)00388-X
Sutherland, W. J., Dias, M. P., Dicks, L. V., Doran, H., Entwistle, A. C., Fleishman, E., et al. (2020). A horizon scan of emerging global biological conservation issues for 2020. Trends Ecol. Evol. 35, 81–90. doi: 10.1016/j.tree.2019.10.010
Suzuki, Y., Kuma, K., and Matsunaga, K. (1994). Effect of iron on oogonium formation, growth rate and pigment synthesis of Laminaria japonica (Phaeophyta). Fish. Sc. 60, 373–378. doi: 10.2331/fishsci.60.373
Suzuki, Y., Kuma, K., and Matsunaga, K. (1995). Bioavailable iron species in seawater measured by macroalga (Laminaria japonica) uptake. Mar. Biol. 123, 173–178. doi: 10.1007/BF00350337
Tang, C. C., Ross, C. K., Yao, T., Petrie, B., DeTracey, B. M., and Dunlap, E. (2004). The circulation, water masses and sea-ice of Baffin Bay. Progr. Oceanogr. 63, 183–228. doi: 10.1016/j.pocean.2004.09.005
Thuiller, W., Georges, D., Engler, R., and Breiner, F. (2020). Biomod2: Ensemble Platform for Species Distribution Modeling. R package version 3.4.6. Available online at: https://CRAN.R-project.org/package=biomod2 (accessed July 2020).
Traiger, S. B., and Konar, B. (2018). Mature and developing kelp bed community composition in a glacial estuary. J. Exp. Mar. Biol. Ecol. 501, 26–35. doi: 10.1016/j.jembe.2017.12.016
Trivedi, M. R., Berry, P. M., Morecroft, M. D., and Dawson, T. P. (2008). Spatial scale affects bioclimate model projections of climate change impacts on mountain plants. Glob. Change Biol. 14, 1089–1103.
Vadas, R. L. Sr. (1968). The Ecology of Agarum and the Kelp Bed Community. USA: University of Washington.
Vale, C. G., Tarroso, P., and Brito, J. C. (2014). Predicting species distribution at range margins: testing the effects of study area extent, resolution and threshold selection in the Sahara–Sahel transition zone. Divers. Distrib. 20, 20–33. doi: 10.1111/ddi.12115
van Son, T. C., Nikolioudakis, N., Steen, H., Albretsen, J., Furevik, B. R., Elvenes, S., et al. (2020). Achieving reliable estimates of the spatial distribution of kelp biomass. Front. Mar. Sci. 7:107. doi: 10.3389/fmars.2020.00107
Wernberg, T., Coleman, M. A., Bennett, S., Thomsen, M. S., Tuya, F., and Kelaher, B. P. (2018). Genetic diversity and kelp forest vulnerability to climatic stress. Sci. Rep. 8, 1–8. doi: 10.1038/s41598-018-20009-9
Wernberg, T., Krumhansl, K., Filbee-Dexter, K., and Pedersen, M. F. (2019). “Status and trends for the world’s kelp forests” in World Seas: an Environmental Evaluation, 2nd Edn. ed. C. Sheppard (United Kingdom: Academic Press). 57–78. doi: 10.1016/B978-0-12-805052-1.00003-6
Wiencke, C. (2009). Biology of Polar Benthic Algae, ed. C. Wiencke (Berlin: Gruyter De), 337. doi: 10.1515/9783110229714
Wilson, K. L., Skinner, M. A., and Lotze, H. K. (2019). Projected 21st-century distribution of canopy-forming seaweeds in the Northwest Atlantic with climate change. Divers. Distrib. 25, 582–602. doi: 10.1111/ddi.12897
Young, M., Ierodiaconou, D., and Womersley, T. (2015). Forests of the sea: predictive habitat modelling to assess the abundance of canopy forming kelp forests on temperate reefs. Remote Sens. Environ. 170, 178–187. doi: 10.1016/j.rse.2015.09.020
Keywords: Laminariales, polar, ensemble model, species distribution model (SDM), climate change, shallow subtidal benthic
Citation: Goldsmit J, Schlegel RW, Filbee-Dexter K, MacGregor KA, Johnson LE, Mundy CJ, Savoie AM, McKindsey CW, Howland KL and Archambault P (2021) Kelp in the Eastern Canadian Arctic: Current and Future Predictions of Habitat Suitability and Cover. Front. Mar. Sci. 18:742209. doi: 10.3389/fmars.2021.742209
Received: 15 July 2021; Accepted: 16 September 2021;
Published: 07 October 2021.
Edited by:
Guillem Chust, Technological Center Expert in Marine and Food Innovation (AZTI), SpainReviewed by:
Leire Ibaibarriaga Contreras, Marine Research Division, Technology Center Expert in Marine and Food Innovation (AZTI), SpainGregory N. Nishihara, Nagasaki University, Japan
Copyright © 2021 Goldsmit, Schlegel, Filbee-Dexter, MacGregor, Johnson, Mundy, Savoie, McKindsey, Howland and Archambault. This is an open-access article distributed under the terms of the Creative Commons Attribution License (CC BY). The use, distribution or reproduction in other forums is permitted, provided the original author(s) and the copyright owner(s) are credited and that the original publication in this journal is cited, in accordance with accepted academic practice. No use, distribution or reproduction is permitted which does not comply with these terms.
*Correspondence: Jesica Goldsmit, amVzaWNhLmdvbGRzbWl0QGRmby1tcG8uZ2MuY2E=; amVzaWNhZ29sZHNtaXRAZ21haWwuY29t
†These authors have contributed equally to this work and share first authorship