- 1Norwegian College of Fishery Science, The Arctic University of Norway (UiT), Tromsø, Norway
- 2Department of Automation, and Process Engineering, The Arctic University of Norway (UiT), Tromsø, Norway
Under the individual vessel quota regulations, the expected economic return of a multi species fishery is influenced by an array of a multi-component choice such as targeted species, landings per haul, harvesting time and its location. The components of effort allocation decisions are further complicated by changes in the market conditions and the constant movements of fish between spawning and feeding habitats. Migratory behavior influences the dispersal of species, relative availability of fish and its composition, and the bycatch likelihood across different locations over the course of a fishing year. The objective of this article is to investigate the optimal allocation of fishing effort in the Norwegian bottom-trawl fleet within economically important species; cod, saithe, and haddock across three heavily trawled areas—including southern and northern parts of the west coast of Norway, and the high sea areas of the Arctic—to achieve maximum expected economic return, with respect to the individual vessel quota constraints and bycatch considerations. The results from a mixed integer non-linear optimization problem evidence that the spawning migration of Northeast Arctic cod along the northwest coast, effort allocation behavior of coastal fleet, together with institutional regulations necessitate the substitution of fishing effort across different fishing locations within the fishing season to maximize expected return. The results of our study further reveal that the Arctic region to target cod is the biggest contributor to annual fishing revenue. By contrast, conducting saithe fishery in the southwest of the Norwegian coast has the lowest economic contribution. The results from Monte Carlo simulation demonstrate that the proposed model is effective and applicable for effort allocation decision analysis.
Introduction
Economic theories predict that commercial fishing is undertaken to maximize expected economic return (Gordon, 1953, 1954), which is influenced by fish availability, measured by catch per unit of effort (CPUE) (Hilborn and Walters, 1992; Campbell, 2004) and market prices as well as the decisions underlying effort allocation such as when and where to fish and what to target (Béné and Tewfik, 2001; Birkenbach et al., 2020). Not only optimal distribution of fishing effort across time and space to achieve the highest expected economic return is an important goal for fishers, but it is also a means to a long-run economically viable fishing industry (Béné and Tewfik, 2001; van Oostenbrugge et al., 2002; Salas and Gaertner, 2004).
Ocean-going fishing vessels like the Norwegian bottom trawlers are quota-regulated and conduct multi-species fisheries. The main target species are Northeast Arctic (NEA) cod (Gadus morhua), saithe (Pollachius virens), and haddock (Melanogrammus aeglefinus), known as codfish (Birkenbach et al., 2020). These species constitute one of the most commercially valuable quota portfolios and comprise around 80% of total landings and revenue of this fleet (Norwegian Directorate of Fisheries, 2019). The deep-sea bottom-trawl fleet has been managed by Total Allowable Catches (TACs) and individual vessel quotas (IVQs) as a proportion of the TAC for each species (Standal and Asche, 2018). The TACs and IVQs are annually determined on a species-specific basis (Standal and Asche, 2018). Under this management scheme, overfishing is not allowed and trawlers with over-quota catches are penalized (Johnsen and Eliasen, 2011). Quotas are valid for 1 year.
The introduction of IVQs provides opportunities for fishers to maximize expected economic returns of quota portfolio. This is to say that having rights to predetermined shares of multiple fish stocks enable fishers to take their time within the fishing season, plan the utilization of the quota portfolio and allocate fishing effort during favorable times when CPUE and/or fish prices are high (Poos et al., 2010; Batsleer et al., 2015; Birkenbach et al., 2017). While quota management regime enables fishers to expand harvesting seasons and distribute effort more evenly throughout the fishing year, to avoid landings in a compressed season, market gluts and reduced prices (Grafton, 1996; Dupont et al., 2005; Grafton et al., 2006; Birkenbach et al., 2017), yet the optimal allocation of fishing effort to maximize expected return in multi species fishery is challenging.
Codfish are migratory species and constantly move between reproductive areas and feeding grounds. Every winter the codfish aggregate along the northwest coast of Norway to spawn (Trout, 1957; Pethon, 2005; Olsen et al., 2010). After spawning cod and haddock return to the high sea areas of the Barents Sea to feed (Trout, 1957; Olsen et al., 2010). The constant movements of codfish across different locations influence CPUE, species composition, and bycatch—the inadvertent capture of non-target species—thereby the expected returns (Poos et al., 2010; Asche et al., 2015; Batsleer et al., 2015). Codfish prices fluctuate by the changes in supply and demand sides as well as the interplay between them (Asche et al., 2002, 2004; Arnason et al., 2004; Nielsen et al., 2012; Alizadeh Ashrafi et al., 2020).
In the quota-regulated fisheries matching expected catch compositions with the remaining quotas to avoid overharvesting of the quota portfolio while aiming to maximize landing value of the quota portfolio is difficult (Copes, 1986; Branch et al., 2006; Sanchirico et al., 2006). The difficulty is aggravated by the spawning spatial overlay of codfish along the northwest coast of Norway during the winter months. The co-occurrence of spawning cod, saithe, and haddock in the same area increases the bycatch likelihood. In addition, the discarding of fish at sea is illegal; therefore, the entire catch including the non-target species needs to be landed. This means that the bycatch species are also counted against the corresponding stock quotas (Nærings og Fiskeridepartementet, 2009; Johnsen and Eliasen, 2011; Gullestad et al., 2015).
Additionally, the codfish are simultaneously harvested by different fleet groups. Even though assigning catch shares to fishers has ceased the competitive race to fish, harvesting behavior of one specific fleet could likely impact the effort allocation behavior for other fleets (Boyce, 1992; Alizadeh Ashrafi and Abe, 2021).
Given the complexity to characterize the optimal harvest of multi-species fishery, the aim of this paper is to find the optimal effort allocation to maximize the economic return in the codfish fishery operated by the trawl fleet in three heavily trawled regions, taking into account IVQ regulations and bycatch considerations. How to allocate the effort to maximize the expected return of the quota portfolio, is a typical decision-making problem. Different classes of optimization have been widely applied to make optimal decisions in complex decision-making problems in environmental studies (Mangel and Clark, 1988; Babcock and Pikitch, 2000). This article employs a mixed integer non-linear optimization to scrutinize the optimal seasonal harvest schedule to maximize the bio-economic revenue per unit of effort (RPUE) of the codfish portfolio. The application of optimization in effort allocation decisions in codfish fisheries provides a suitable framework as the combinations of bio-economic attributes for each species/area/time period are evaluated for their effects on expected returns given a fixed quota portfolio and maximal effort/unit time (Nocedal and Wright, 2006).
The results of this study provide trawlers opportunities to maximize landing value of the quota portfolio by offering them insights about where and when to fish what and how much to land per haul. It is now well admitted that fisheries management places greater prominence on fish behavior (Hilborn, 1985; Fulton et al., 2011), and the efficient regulations cannot be designed without investigation of fishers’ behavior (Wilen et al., 2002; Hilborn, 2007). Hence, the results of our study contribute to the improvement of fisheries management by assessing how trawlers might response to changes in biological, economic, and regulatory conditions.
Data
Trawling Area and Its Sub-Regions
The Norwegian trawlers predominantly catch cod, saithe, and haddock (Birkenbach et al., 2020). Figure 1 shows areas that are heavily trawled for cod, saithe, and haddock in the Norwegian waters. The Norwegian fisheries implement electronic monitoring technology to record and collects haul-based observations related to the geographic coordinates on the net set location and the location of lift. These data are gathered by the Norwegian Directorate of Fisheries. Using these data, we have plotted the spatial distribution of catch location of 53 active bottom-trawl boats over the period of 2011–2016 in Figure 1. A total of 68,356, 54,145, and 35,397 observations were collected for cod, saithe, and haddock fisheries, respectively.
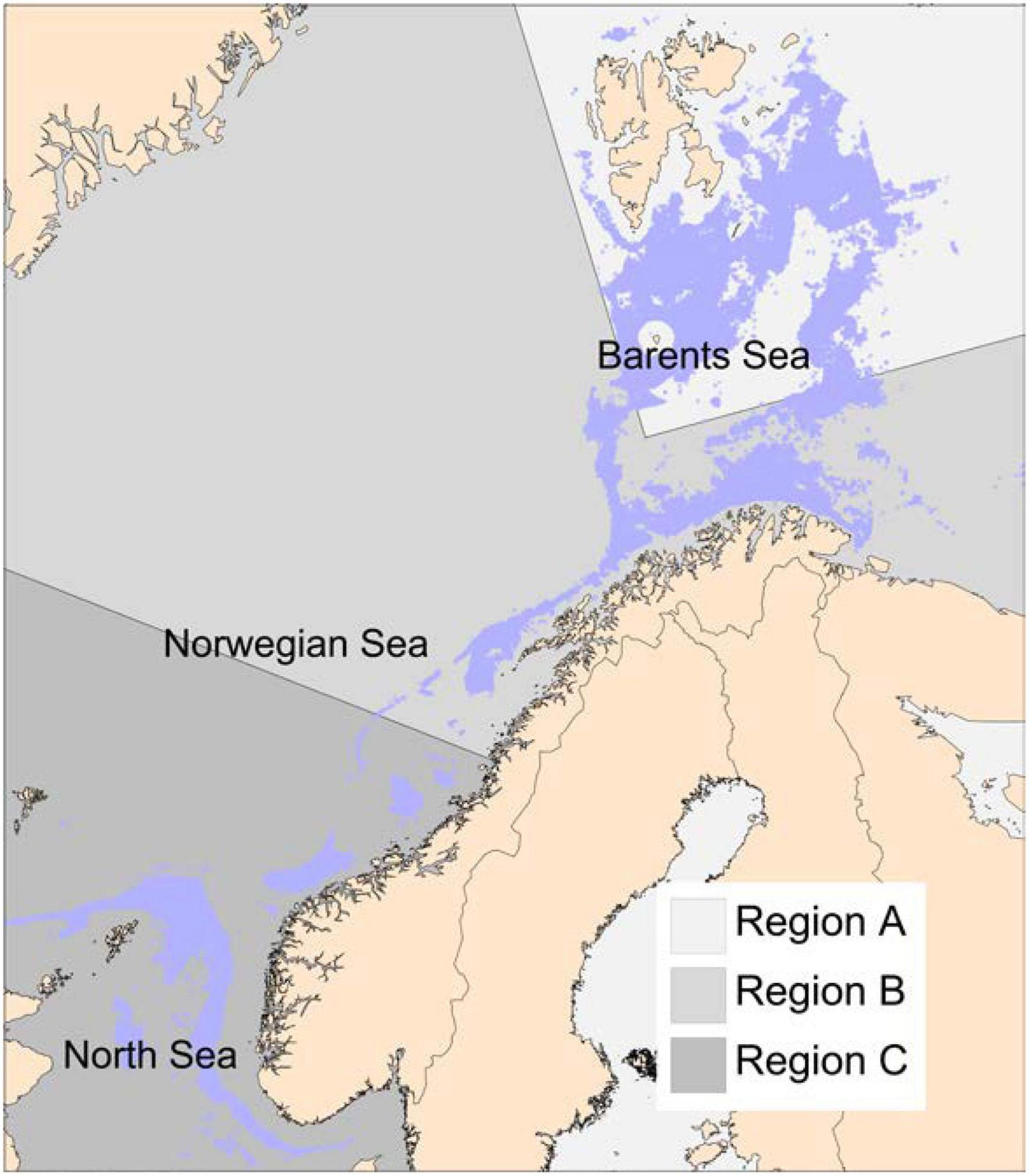
Figure 1. Schematic representation of the spatial distribution of the Norwegian bottom-trawl vessels. Purple dots show the catch locations in the codfish fishery based on the haul-level observations for 53 licensed trawl vessels over the period of 2011–2016. A total of 68,356, 54,145, and 35,397 observations were collected for cod, saithe, and haddock fisheries, respectively. The cod and haddock fisheries are prevalent in regions A and B, while the saithe fishery dominates in region C. The Norwegian Directorate of Fisheries.
As it seen from Figure 1, we have divided the west coast of Norway into two separate regions, and in total we consider three regions: A, B, and C. This division is based on the relative availability of codfish, a factor that stems from the feeding and spawning migration patterns over the course of a year. Region A is ascribed to the high sea areas of the Arctic, including Svalbard and Bear Island, where cod and haddock feed. Every winter, mature cod and haddock migrate southward and aggregate along the northwest coast of Norway to spawn, with a peak in March–April for cod and April–May for haddock (Bergstad et al., 1987; Olsen et al., 2010). The spawning of saithe along the west coast of Norway also begins in winter, and it peaks in February (Pethon, 2005; Olsen et al., 2010). Region B refers to the shallow waters off the northwest coast of Norway, where these three fish species aggregate to spawn during winter. Region C is located in the North Sea, where saithe is prevalent.
Construction of Fortnightly Data
A representative trawler aims to make optimal decisions underlying effort allocation to maximize the annual expected return of the codfish portfolio, while adhering to the quota constraints. Expected return of the quota portfolio is, however, unobservable due to the uncertain catch composition and the fish prices at the time of landing. We approximate expected return by the bio-economic RPUE. The RPUE is obtained from the multiplication of the CPUE and fish price. The value of CPUE reflects the fish availability (i.e., biological factor in fishers’ decision) and price of fish reflects the economic motivation to utilize the quota and allocate effort. To calculate the RPUE for each fishery, consisting of the main catch and the bycatch at a given time and in a specific region, we have obtained catch and effort observations as well as price data from two different sources. Based on the Norwegian fisheries regulations, fishers are allowed to sell the bycatch (Johnsen and Eliasen, 2011). Hence, the bycatch species could contribute critically to the expected returns.
We define each fishery (i.e., cod, saithe, and haddock fisheries) based on the catch composition. For different fisheries, catch composition can range from one dominant species combined with other species where they occupy less proportions in the total catch. For example, if the catch is dominated by cod, we consider this haul as cod fishery and the incidental catches of saithe and haddock as bycatch species.
The estimated catch and effort recordings for 53 trawl boats on a haul-to-haul basis have been obtained from fishers’ logbooks, which has been compiled by the Norwegian Directorate of Fisheries (Norwegian: Fiskeridirektoratet) for the period of 2011–2016. A total of 68,356, 54,145, and 35,397 observations have been collected for cod, saithe, and haddock fisheries, respectively. The spatiotemporal haul-based records in the data set are decomposed to the quantities of targeted fish and non-target species, enabling us to obtain the CPUE of the main and incidentally caught species. Because the main focus of this study is on cod, saithe, and haddock fisheries, we have only included the bycatch of saithe and haddock in the cod fishery, bycatch of cod and haddock in the saithe fishery, and bycatch of saithe and cod in haddock fishery. The catch is measured in tons, while fishing effort is measured by trawling hour. The CPUE is measured in tons of landed fish divided by hours of trawling.
In Figure 2, we have plotted the average of the total catch for 53 trawl vessels over the period of 2011–2016 on fortnightly basis.
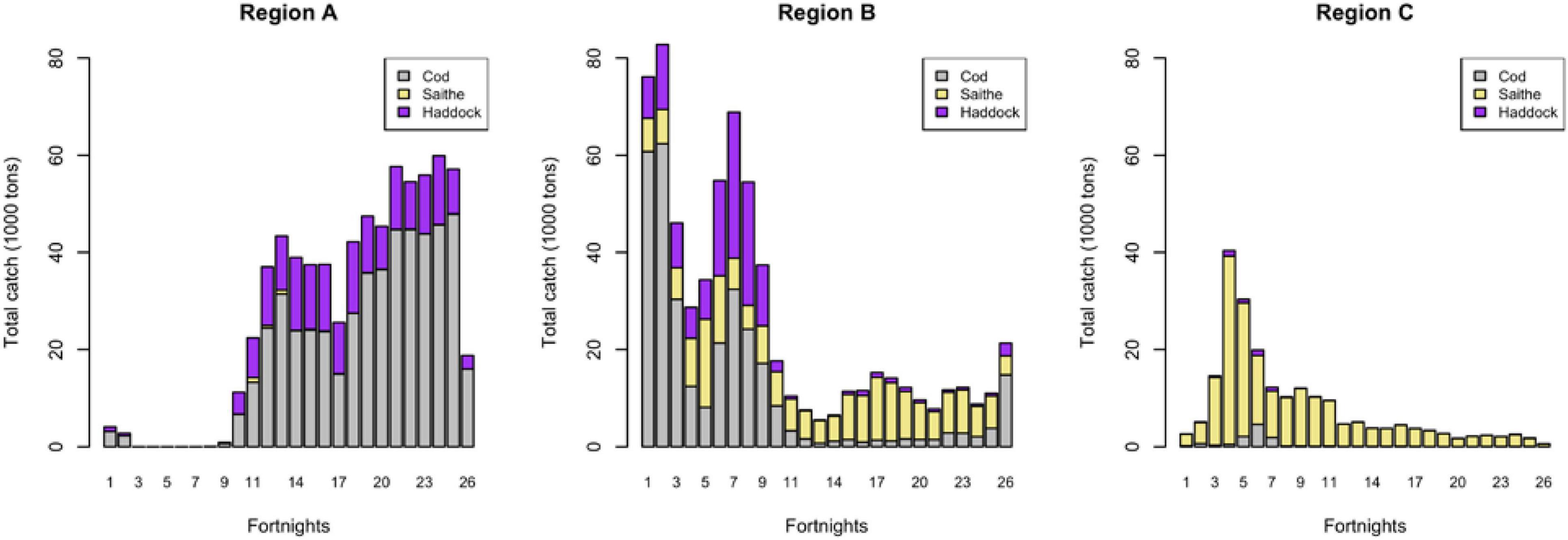
Figure 2. Average of the total catch of the codfish, harvested by trawl fleet, consist of 53 licensed vessels on fortnightly basis in regions A, B, and C over the period of 2011–2016. The total catch is measured in tons of fish. The Norwegian Directorate of Fisheries (Norwegian: Fiskeridirektoratet).
In the region A, landings of cod increase toward the end of the year, while an opposite catch pattern is observed for cod in the region B. The catch of cod and haddock have substantially fallen from the third fortnight (early February) in the region B. From the eleventh fortnight (May), the lowest catches were made in the region B for cod and haddock. The catch of saithe peaks in the region C at the beginning of the year.
Figure 3 shows the average of the total effort for 53 trawl vessels over the period of 2011–2016 on fortnightly basis.
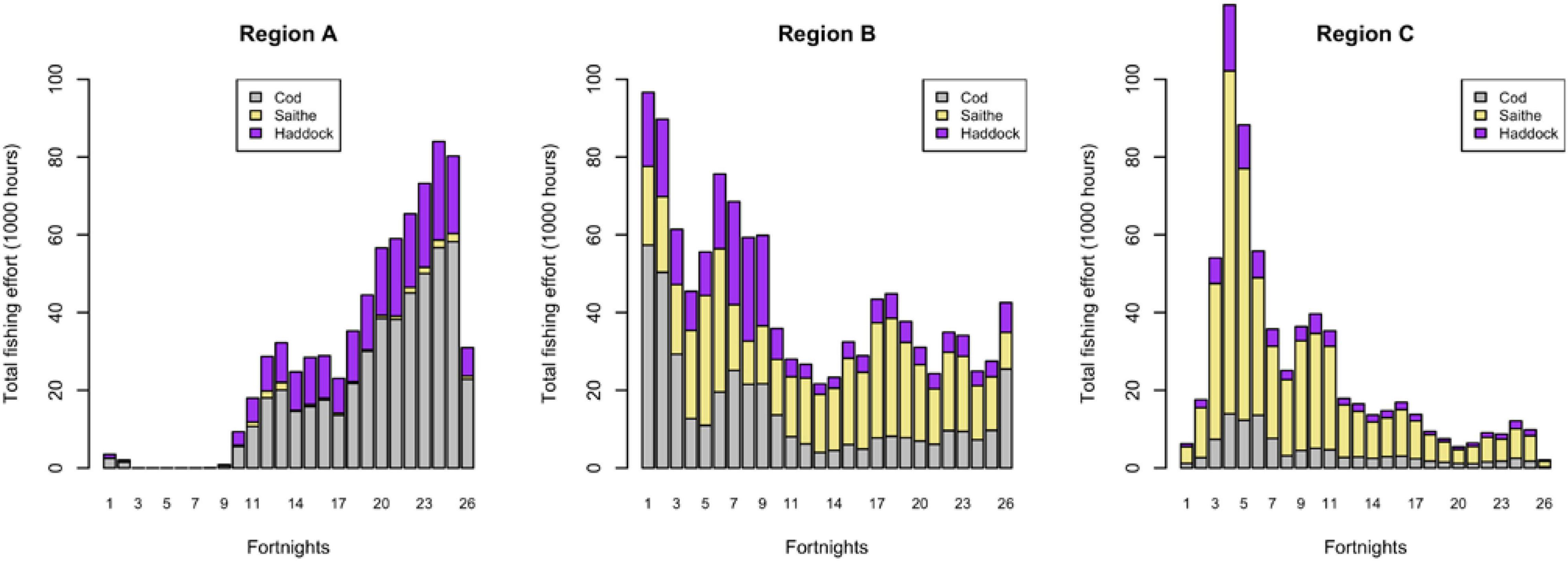
Figure 3. Average of the total effort allocation in the codfish in the trawl fleet, consist of 53 licensed vessels on fortnightly basis in regions A, B, and C over the period of 2011–2016. The effort is measured trawling hours. The Norwegian Directorate of Fisheries (Norwegian: Fiskeridirektoratet).
The pattern of effort allocation in Figure 3 is similar to the catch pattern, shown in Figure 2. The trawling hours in cod fishery show a dramatic increase in region A from May. The effort allocation in cod fishery in region B shows a decreasing pattern as time elapses. Concurrent to the reduction of trawling hours in cod fishery in region B, at the beginning of the year, the effort is intensified in saithe fishery in region C with its peak in February.
Figure 4 illustrates the average fortnightly variation in the observed CPUE within and between the selected species in the three selected regions over the period of 2011–2016. Non-target catches are also considered in the calculation of the CPUE of each fishery.
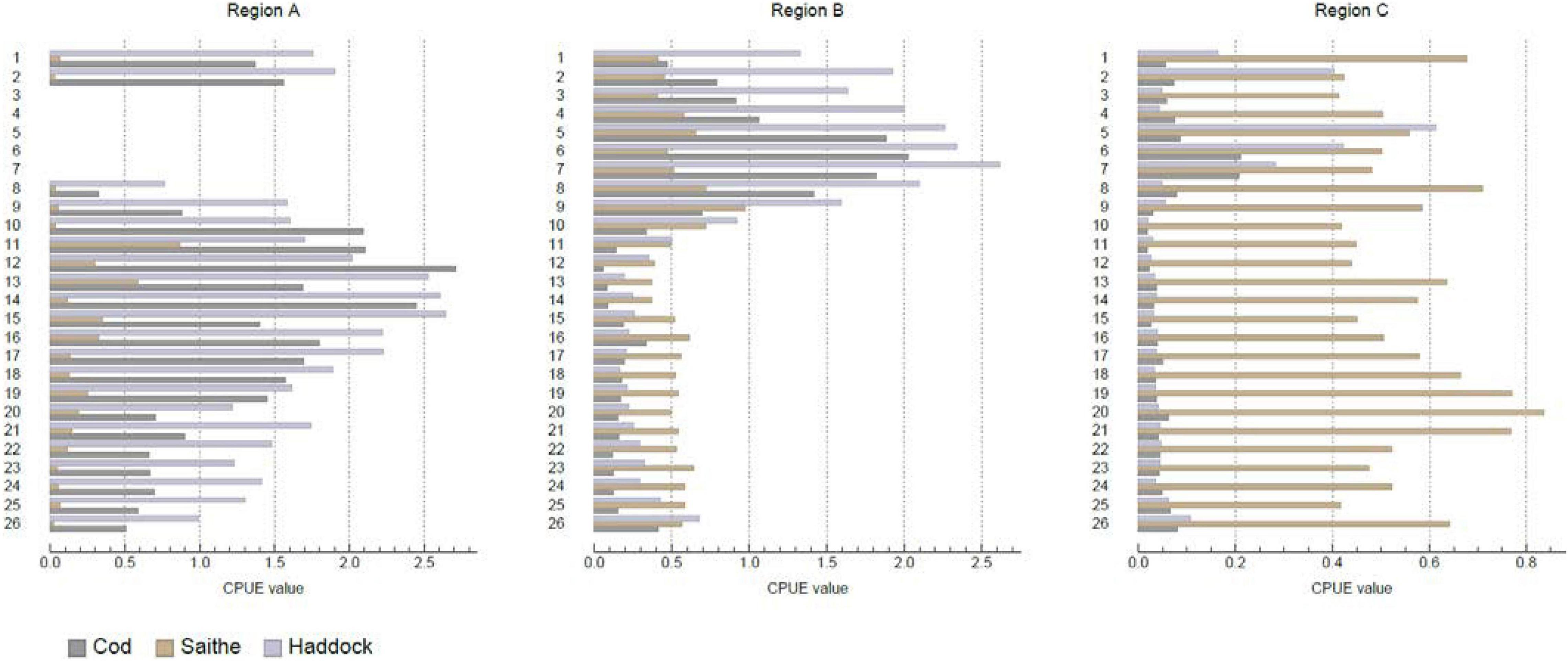
Figure 4. Average of fortnightly variation in the catch per unit of effort (CPUE) of cod, saithe, and haddock fisheries in regions A, B, and C. CPUE is measured in tons of fish caught per hour of trawling for 53 trawl vessels. The Norwegian Directorate of Fisheries (Norwegian: Fiskeridirektoratet).
As shown in Figure 4, cod and haddock are caught mostly in region A and B, while saithe is targeted in region C. In February and March, there is no fishing in region A, probably because of the less desirable climatic conditions of the Arctic. From April, cod and haddock fishery begins in region A because the weather conditions probably improve in the high sea areas the Arctic (i.e., sea-ice retreat and less wind chill). In region B, the CPUE of the cod and haddock fisheries follow a decreasing pattern toward the end of the year. The high CPUE of the cod and haddock fisheries at the beginning of the fishing year in region B might be associated with the spawning aggregation and increased catchability. The CPUE patterns of saithe in regions A and C are almost steady.
CPUE can be used to address the density of fish populations (Hilborn and Walters, 1992; Campbell, 2004). However, the constant changes in both biomass and species distributions over time and across various locations entails the idea of generating probabilistic CPUE. In Figure 5, we have shown the fortnightly variations in probabilistic CPUE in regions A, B, and C within a fishing year (i.e., the mathematical procedure to obtain probabilistic values of CPUE is explained in section “A Probabilistic Model of Spatiotemporal Catch Per Unit of Effort”). The probabilistic CPUE are used as input in the optimization model. The justification for utilizing the probabilistic CPUEs in the optimization model is explained in section A Probabilistic Model of Spatiotemporal Catch Per Unit of Effort.”
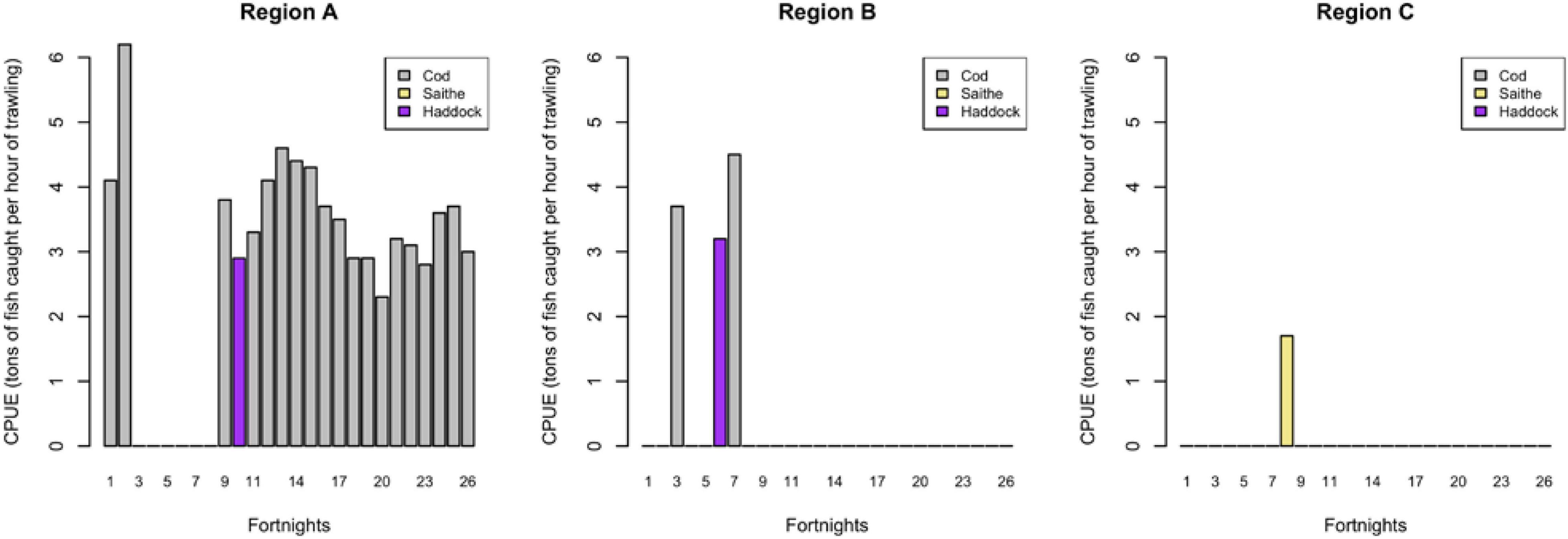
Figure 5. The bar graph shows the probabilistic catch per unit of effort (CPUE) in regions A, B, and C on a fortnightly basis. The probabilistic values of CPUE obtained from a convex combination of zero and a log-normally distributed random variable with mean and standard deviation depending on harvesting time, its location and target species.
As seen in Figure 5, region A is dominated by cod fishery. Within region A, if we set aside January which has the highest CPUE and February with no fishing activity, the CPUE of cod fishery is reasonably stable and remains around 4 tons per hour of trawling. In region B, cod and haddock fisheries are conducted. CPUE fluctuations are considerable, ranging from close to the zero to 5 tons of fish harvested in 1 h of trawling. Saithe fishing in region C is conducted only during the seventh fortnight (late March–early April), and its value is close to 2 tons per hour of trawling.
The Norwegian Fishermen’s Sales Organization (Norwegian: Norges Råfisklag) collects weekly ex-vessel prices received by the trawlers for the frozen cod, saithe, and haddock products. The Norwegian codfish trawl fleet in our study is equipped with manufacturing, processing, and freezing facilities, which enable them to freeze the harvested fish. For the purpose of this study, we have utilized the reported prices in Norwegian currency [Norwegian kroner (NOK)] covering the period of 2011–2016. Figure 6 illustrates the average fortnightly price per kilogram for the frozen cod, saithe, and haddock products over the period of 2011–2016.
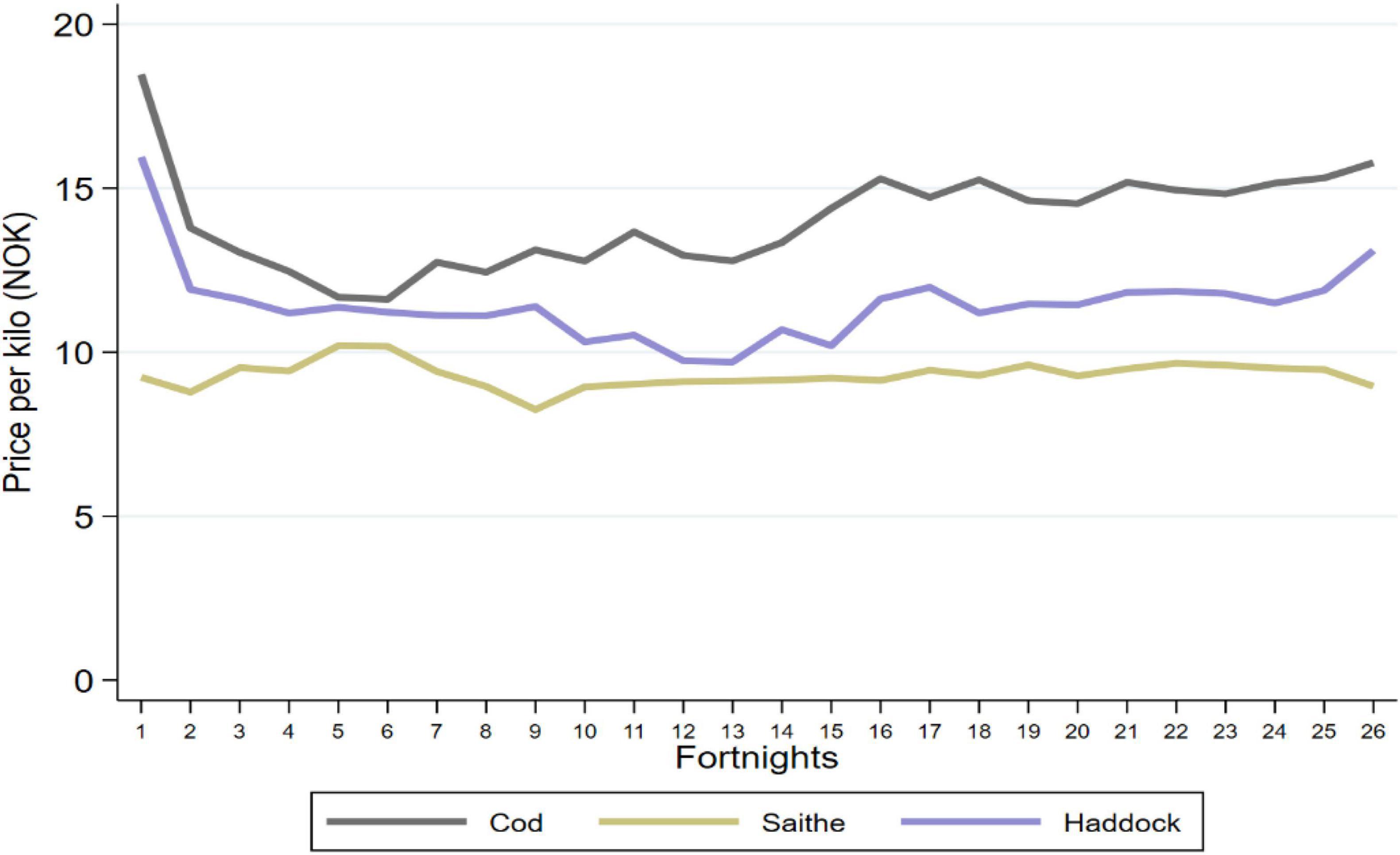
Figure 6. Average fortnightly prices per kilogram for the frozen cod, saithe, and haddock products received by the trawl fishers over the period of 2011–2016. Norwegian Fishermen’s Sale Organization (Norwegian: Norges Råfisklag).
As shown in Figure 6, cod and saithe are the most and least economically valuable species in the codfish fishery, respectively. The patterns of the price movements for cod and haddock are reasonably similar. As soon as the fishing season opens, the prices of cod and haddock decline. The reduction in the cod price is larger than the reduction in the haddock price. Cod and haddock receive higher prices toward the end of the fishing year. A contrasting pattern is observable for the saithe price at the beginning of the year, reaching its peak in March (fifth and sixth fortnights). The price of saithe remains stable toward the end of the year. Generally, the price of saithe does not fluctuate as much as the prices of cod and haddock.
For all analyses, the values of RPUE have been aggregated on a fortnightly basis across 53 vessels over the period of 2011–2016. The reason behind the choice of a fortnightly time resolution is related to the high cost of a frequent shift in the target species/location of fishing because of the vast geographical area shown in Figure 1. We believe that a 2-week time period is adequately long to justify the cost of switching between fisheries/locations (see also section “Model Assumptions”). Another relevant justification is to smooth out the random variation due to poor weather, bad luck, or other stochasticity. Moreover, physical characteristics of the trawl vessels such as longer vessel size, powerful engine, less susceptibility to the poor weather conditions, and the availability of processing and freezing facilities onboard enable them to take a 2-week-long fishing trips (Flaaten and Heen, 2004; Standal and Hersoug, 2015). The reason for considering 26 fortnights (i.e., equal to 1 year) is that the fish quotas are valid only for the given year.
Calculation of the Annual Quota Constraints
In a given year, a representative IVQ holder aims to maximize expected return subject to constraining quotas. In this section we explain how we obtain the assigned codfish quota for the representative trawler.
The Norwegian quota management system bans discarding commercial species; thus, fishers have to land the entire catch, including the incidental catch (Johnsen and Eliasen, 2011; Gullestad et al., 2015). To encourage compliance and prevent fishers from dumping non-target fish at sea, fishers are allowed to sell the bycatch (Johnsen and Eliasen, 2011). In 2009, the total landing obligation came into force by Marine Resources Act, mentioning that all the commercial fish species that are caught (i.e., including the main target and bycatch) are due to be counted against the corresponding stock quota (Nærings og Fiskeridepartementet, 2009; Johnsen and Eliasen, 2011).
In addition, overharvesting of the quota (i.e., including catch and bycatch) is illegal, meaning that fishers with catches in excess of their quota holdings are subject to confiscation and/or penalty (Johnsen and Eliasen, 2011; Gullestad et al., 2015). Considering the quota constraints and the fact that the bycatch is deducted from the corresponding quota, fishers need to choose the fishing location and season carefully, and account for the likelihood of the bycatch quantity of each species in the selected regions/fisheries over the course of a fishing year. For example, if a small portion of the quota for a specific species is left, the representative trawler has to redirect fishing effort away from that location/fishery because the remaining quota might be used as bycatch in the remaining fortnights. This means that in quota-regulated fisheries, fishers have to balance constantly the landed fish and the remaining quota to avoid over-utilization of the quota. Considering the above argument, the total catch for each species can be an adequate proxy for the allocated annual quotas.
In order to acquire the quota sizes for the representative trawler, first, we have found the average of total catch per boat. The Norwegian bottom-trawl vessels are relatively homogenous in terms of technology and holding quota portfolios. In addition, after the introduction of Individual Transferable Quota (ITQ) system in the Norwegian fisheries, trawl vessels have become bigger, owned by fewer companies (Anonymous, 2018). Under this circumstance, it is reasonable to expect similar catch patterns among the trawl vessels. Hence, averaging across vessels to find the quota sizes of the representative trawler does not concern us regarding loss of information.
Table 1 approximates the average annual quota allocation per trawl vessel in tons for three species over the period of 2011–2016. The cod quota is the largest in the codfish quota portfolio and has shown a moderate increase over the time, while the saithe quota has been reasonably steady. The haddock quota has shrunk from 2013 to 2015, but in 2016 it reached the same size as in 2011.
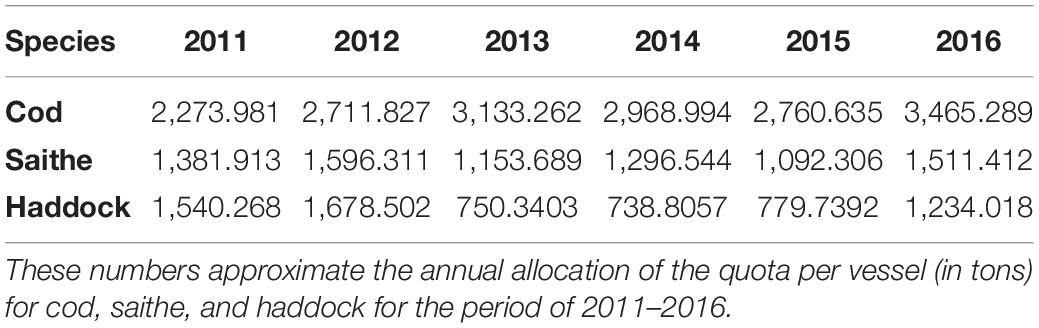
Table 1. Average of the total catch for cod, saithe, and haddock caught the trawl fleet over the period of 2011–2016.
Since quotas are valid for 1 year, to obtain the initial values for the codfish quotas for a given vessel in a given year, we have found the average of annual quota allocations for each species over 6 years, shown in Table 1.
The quota sizes are 2,885.66, 1,338.69, and 1,120.27 tons for cod, saithe, and haddock, respectively. Our optimization algorithm has been developed in a way that the maximizing harvest strategy is in line with the quota sizes and over-catch situations are avoided. For simplicity, we have rounded up the quota sizes for cod and saithe and rounded down the quota size for haddock. The implemented quota sizes in the optimization model for cod, saithe, and haddock fisheries are 3,000, 1,500, and 1,000 tons, respectively.
Materials and Methods
Modeling Assumptions
A number of assumptions have been made to build and to simplify our optimization model. In this study, we assume that a representative holder of the quota portfolio for cod, saithe, and haddock is an economically rational and compliant decision maker with perfect foresight who makes the decisions on when, where, and what to fish, and how much to land to balance the catch and the remaining quota, based on some notions of expected return.
Expected return is measured by the RPUE. Compliance is necessary in the utilization of the quota to assure that the representative fisher avoids over-harvesting the quota portfolio. We also assume that the trawl fisher operates in a full-time capacity and the fisher is fishing actively through the year (e.g., the vessel is not idle for maintenance, refurbishing, and the occasional repair). A switch between locations/fisheries takes place every 2 weeks (i.e., a fortnightly basis—every year consists of approximately 26 fortnights) to avoid the high cost of a shift between regions and/or fisheries (i.e., the trawling area is quite large, see Figure 1). In addition, the ability to process and freeze the fish onboard precludes trawlers from frequent port visits. We also assume that the representative trawler can fish only the main target species on a fortnightly basis. In addition, under the Norwegian quota management, just a small portion of the unused part of quotas is granted in the subsequent year. Hence, under this management scheme, a necessary condition to maximize expected return is the full utilization of the quota portfolio by the end of the fishing year. This assumption is underpinned by the annual profitability survey compiled by the Norwegian Directorate of Fisheries, which shows that trawlers fulfill their codfish portfolio by the end of the fishing year.1 We also disregard the transferability option of the quotas across vessels within a given fishing year because selling or buying the quota is a decision for the long run, while for the optimization model, we consider decisions over a year (i.e., short-term decision) and assume the quota as given. Furthermore, codfish quotas are highly valuable and there has been very limited transfer of these in recent years. Hence, although in principle quotas could be traded between firms at least annually, and thus provide an opportunity for short-term changes in quotas, this is not common in the Norwegian bottom-trawl fishery.
Additionally, in this study we only focus on the bycatch of saithe and haddock while targeting cod, incidental catches of cod and haddock while targeting saithe, and non-target cod and saithe while targeting haddock. The rationale behind this choice is due to the high commercial value of these species.
Since our study focuses on how variations in CPUE influence the decisions underlying effort allocation, we only consider the migratory behavior of codfish as a relevant biological feature of codfish. We consider any in-season stock dynamics, such as fish population growth rate as constant.
Formulation of a Multi-Region and Multi-Period Harvest Strategy
In this section, we formulate a mixed integer non-linear optimization model to identify the multi-locational and multi-periodic harvest strategy to maximize fishing expected return with the consideration of the quota constraints and bycatch species. As stated earlier, feeding and spawning migrations of codfish influence the bio-economic conditions (e.g., the CPUE, catch composition, and price) of the fishing locations and the expected returns over the course of a year. The representative trawler holds a quota portfolio of cod, saithe, and haddock and constantly displaces fishing effort to the locations/fisheries with a higher expected return. Mathematically speaking, the representative trawler chooses harvest location a, where Ais the set of possible fishing locations to target species j over the entire finite set of J at harvest time t over the entire finite set of T to achieve the maximum annual expected return. Prior to formulation of the optimization model, we specify the measurement for the expected return. We begin by Schaefer (1954) harvest function, written in Equation (1).
H(t,a,j) refers to the catch of fish species j, at time t in location a, measured in tons. E(t,a,j) is the allocated effort, measured in hours of trawling. X(t,a,j) is the population biomass of each species j at a specific location and time. The parameter q(j)is the catchability coefficient, for example, the portion of the available stock captured by one unit of effort; this parameter addresses the efficiency of a given fishery (Hilborn and Walters, 1992). The output elasticities with respect to the fishing effort and fish biomass are both 1. The harvest function in Equation (1) is further modified as show in Equation (2) to include the bycatch species as well.
The function H(t,a,j,k) now refers to the target species j, at time t in location a, while k refers to the species that it is actually caught. Hence, if jk, the catch composition includes bycatch. q(j,k) is the catchability coefficient of species k and j. Rewriting Equation (2) in terms of unit of effort yields Equation (3).
CPUE(t,a,j,k) refers to the CPUE of species k at time t and location a, when the main target is j. The harvest is divided by the total effort at time t, and not the spatial- and species-dependent effort, as it is the actual time spent fishing that is the denominator of interest, not where and when this time is allocated. From Equation (3), we see that the CPUE is proportional to stock abundance by the catchability coefficient q(j,k); hence, the CPUE could be used to reflect the variation in fish abundance. From the economic point of view, the CPUE addresses the productivity of a particular location/fishery (Hilborn and Walters, 1992).
The expected return is generated by catch size (CPUE) and fish price. When the representative fisher ventures into the sea for fishing, the ex-ante catch composition is uncertain. Moreover, the availability of processing and freezing capacities onboard enables trawlers to take long trips (i.e., approximately 2 weeks); hence, the price of fish when the fisher leaves the port might be different from the price at the time of landing. In this regard, we approximate the expected revenue through the bio-economic RPUE, obtained by Equation (4).
p(t,k) refers to the price per kilogram of species k caught at time t. In Equation (4), we have included the prices of the main catch and by catch species. The reason for including by catch species is that under the Norwegian fisheries management, fishers can sell the by catch species and generate revenue (Johnsen and Eliasen, 2011; Gullestad et al., 2015). The total RPUE (including both the main catch and the by catch) when fishing for species j at time t in location a is therefore represented by Equation (5).
To obtain the RPUE information for the representative trawler, the RPUE of each fishery is aggregated across 53 vessels over 6 years (2011–2016) on a fortnightly basis. Here, the usage of aggregated data to examine the optimal behavior of the representative trawler is not a concern, as we do not miss any critical information. As we have mentioned earlier, the codfish bottom-trawl vessels are reasonably homogenous in their physical characteristics and quota portfolios.
A Mixed Integer Non-linear Programming Model
A general non-linear optimization problem (NLP) can be stated as written in Equation (6).
J(x) is the objective function defining what is regarded as optimal, x is the optimization variable, ci(x)∀i∈ℰ is the equality constraint, and ci(x)∀i∈𝒥 is the inequality constraint (Nocedal and Wright, 2006). For an NLP, either the objective function or the constraints or both are non-linear. For a mixed integer optimization problem, the optimization variables consist of both continuous variables and variables that can only take integer values.
The problem described in this paper is to maximize the RPUE while taking into account constraints on quota (quotas are counted against the main and bycatch species) and fishing effort. The optimization problem is therefore formulated with the following objective function in Equation (7).
Inserting Equations (2), (3), and (5) into Equation (7) gives Equation (8).
Note that E(t) is the total effort at time t, i.e., the sum over a and j of E(t,a,j). The choice of optimization variable x is discussed below and finally defined in Equation (11). To decouple the spatial- and species-dependent effort E(t,a,j)from the spatial and species components a and j, we write E(t,a,j) as shown in Equation (9).
S(t,a,j) ∈ [0,1] is an integer variable defined as 1 if the representative trawler targets species j in location a at time t; otherwise, it is 0. E(t) is now the general amount of effort spent fishing at time t, regardless of location and species choices. This allows us to rewrite Equation (8) as Equation (10).
This change makes the objective function independent of the actual effort E(t), but dependent on the integer variable S(t,a,j), which states what and where to fish during each fortnight. The effort E(t), defining the hours spent each fortnight, will still be included in the optimization problem through constraints from the quota and the maximum number of hours spent fishing. The optimization variable x will therefore be a combination of the continuous variables E(t) and the integer variables S(t,a,j), making it a mixed integer optimization problem, as shown in Equation (11).
The constraints imposed on the objective function consist of quota constraints, effort constraints, spatial constraints, and species constraints. Each of these will be presented below. The quota constraint simply states that the fishing vessel must fulfill the quota of each species k (including bycatch), and it is implemented as an equality constraint in Equation (12).
H(k) is the total catch of species k (both as main catch and as bycatch) and Q(k) it the quota for species k. Based on the assumption stated in section “Modeling Assumptions,” we assume that the representative trawler needs to exhaust the quota portfolio to maximize annual fishing expected return.
The effort constraint states that the representative trawler cannot use more than a specific number of hours fishing each fortnight: Emax. In this paper, this is implemented as being equal for each fortnight, but it may very well vary. The effort constraint is implemented as an inequality constraint in Equation (13).
In this study, we have set a maximum of 15 h of trawling per day, resulting in Emax = 210 h of effort per fortnight. The spatial and species constraints are connected to the fact that the representative fisher can only be in one fishing location and target only one main species at any given time. This imposes the following inequality constraints on the integer variablesS(t,a,j), described in Equations (14) and (15).
Because the quota constraint in Equation (12) contains a product of the optimization variables E(t) and S(a,j,t), the optimization problem becomes non-linear, resulting in a mixed integer non-linear optimization problem.
To summarize, the implemented optimization problem has the objective function defined by Equation (10), the equality constraints defined by Equation (12), and the inequality constraints defined by Equations (13)–(15). In total, this gives 260 optimization variables, 3 equality constraints, and 182 inequality constraints. The optimization problem has been solved with the BONMIN MINLP-solver using CasADi (Andersson et al., 2019) and MATLAB software packages.
A Probabilistic Model of Spatiotemporal Catch per Unit of Effort
The CPUE is one of the components of the expected return (see Equation 4), which is obtained by the total catch divided by the total amount of allocated effort (here, trawling hours) employed to harvest the catch. The CPUE in each fishery changes spatially and temporally due to the changes in fish abundance and availability, environmental variables, or any unexpected perturbations in the marine environment (Hilborn and Walters, 1992; Campbell, 2004)—recall Equation (3). In addition, the prevalence and likelihood of bycatch varies by fishing season and fishing location. The variation in the CPUE introduces uncertainty in future catch composition (including bycatch) and expected returns. Hence, in the presence of uncertainty, it would seem useful to incorporate a probabilistic view of the CPUE in the optimization model, where each fishing effort is a random sample of an underlying probability distribution.
It seems that CPUE(t,a,j,k) can be reasonably approximated by a convex combination of zero and a log-normally distributed random variable with a mean and standard deviation depending on t,a,j,k. Mathematically, CPUE is then described by a probability density function f given by Equation (16).
α(t,a,j,k) is the probability of zero catch of the relevant species at the location a and time t. The parameter μ(t,a,j,k) denotes the mean value of the logarithm of the non-zero values of CPUE calculated from the data, while σ is the standard deviation of the logarithm of the non-zero CPUE values. To avoid a fully probabilistic optimization problem, the situation is simplified by computing and utilizing only the mean value of CPUE(t,a,j,k) in the model (Rubinstein and Kroese, 2016).
To test the sensitivity of the results of the optimization problem and the reliability of the model, a Monte Carlo simulation with each CPUE(t,a,j,k) independently modeled as a convex combination of zero and a log-normally distributed random variable has been performed. Such a probabilistic model should be used with care, because no correlation between variables has been accounted for, and there is a correlation between the main catch and by catches (Rubinstein and Kroese, 2016).
Results
Using the probabilistic CPUE in the mixed integer linear programming model, the optimization results are shown in Figures 7–9. The model maximizes annual expected return from holding a given codfish quota by choosing catch and effort sequences within the cod, saithe, and haddock fisheries across the three selected regions over the course of a fishing year (26 fortnights). Figure 7 shows the optimal allocation of a given quota portfolio over the course of a year across the cod, saithe, and haddock fisheries to maximize expected return.
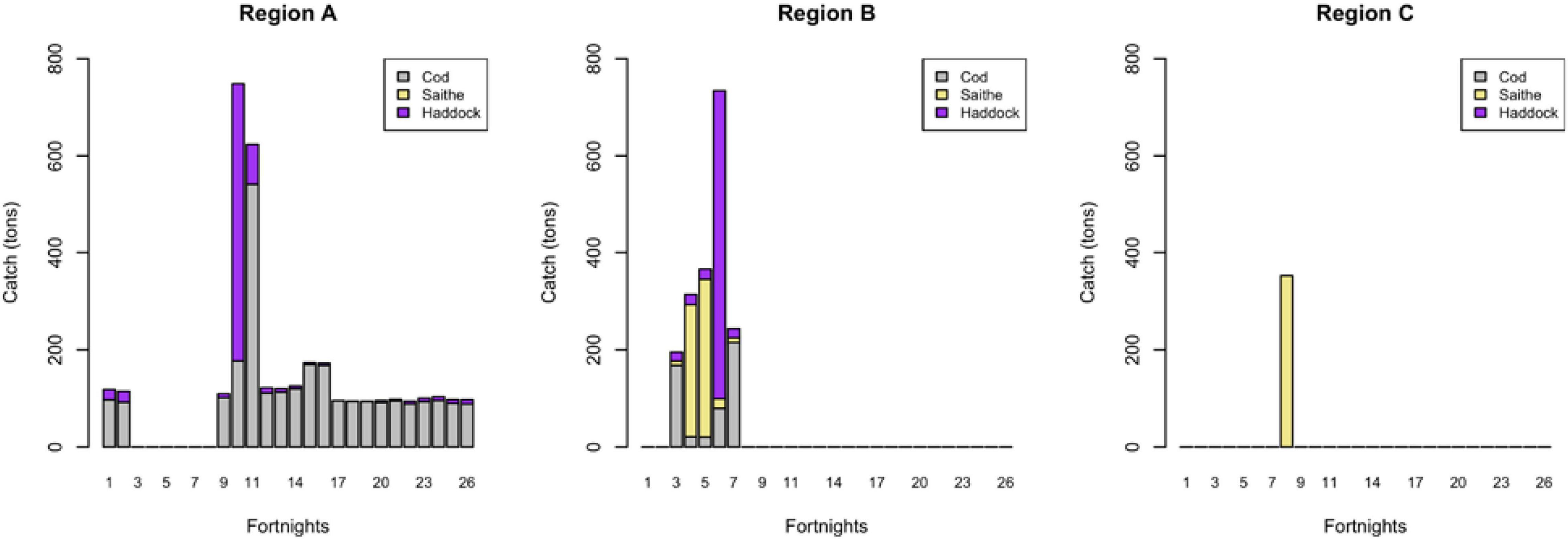
Figure 7. Optimal catch distribution and quota utilization in the cod, saithe, and haddock fisheries in the three selected regions over 26 fortnights.
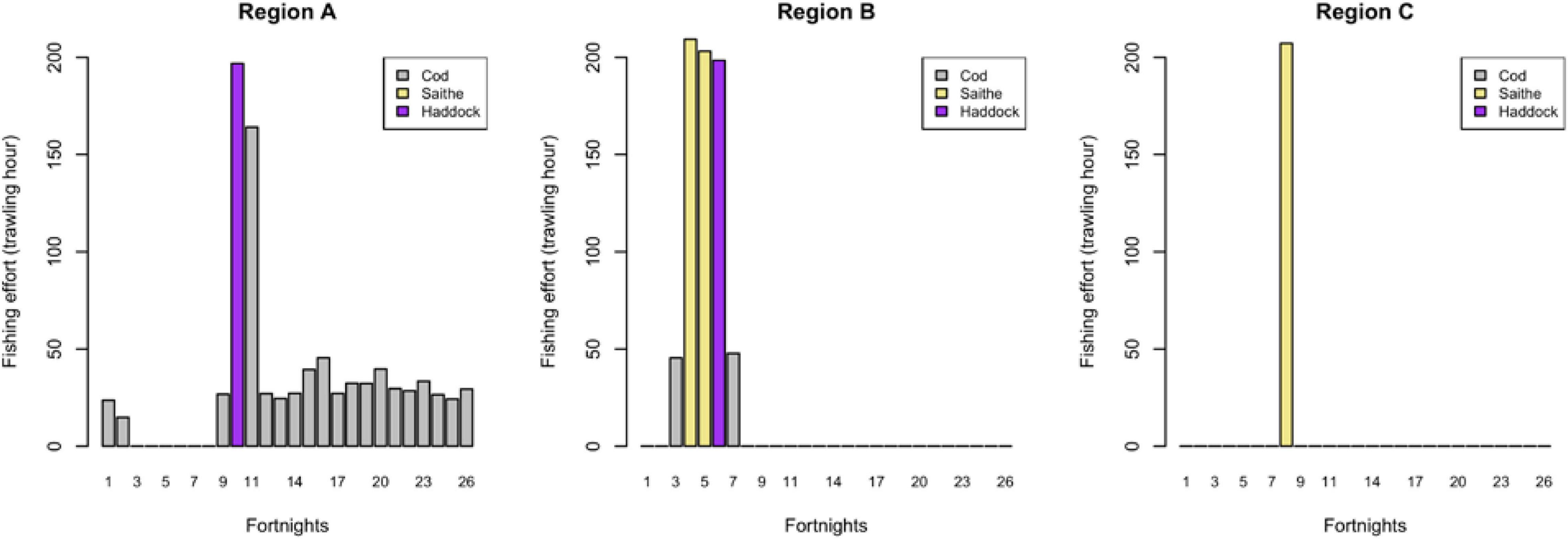
Figure 8. Optimal effort allocation in the cod, saithe, and haddock fisheries in the three selected regions over 26 fortnights.
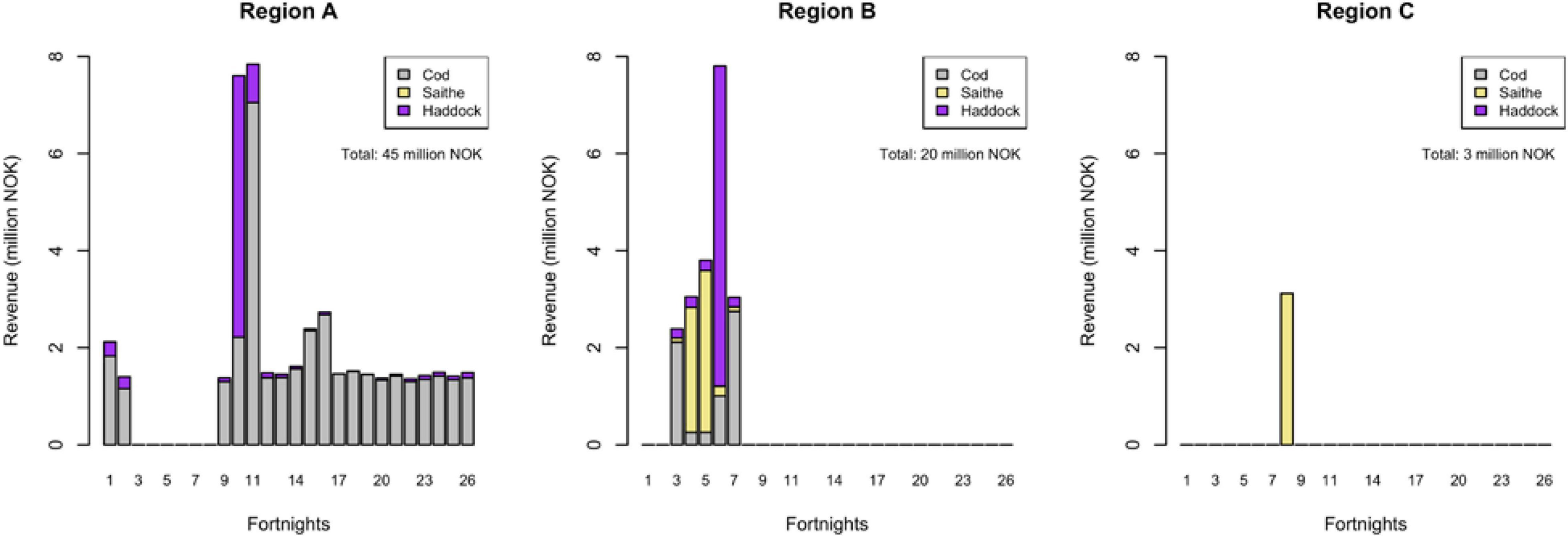
Figure 9. Maximum annual fishing revenue from the holding quota portfolio of the cod, saithe, and haddock fisheries decomposed into the three selected regions. The revenue shares of regions A, B, and C are 45, 20, and 3 million kroner (NOK), respectively.
As seen in Figure 7, an optimum trawler starts the fishing season by targeting cod in region A, with a small proportion of haddock bycatch. Thereafter, the representative trawler reallocates its fishing effort to region B and utilizes the cod, saithe, and haddock quotas. In the eighth fortnight, the effort is displaced to region C, and the main target species is saithe. From around May, the optimal trawler withdraws from region C and reallocates its fishing effort in region A, where it concentrates on fishing cod. The aggregated total catch is 3,117, 1,493, and 987 tons for cod, haddock, and saithe, respectively. This is a small excess catch of cod, which is well within the normal range of excess catch. This violation of the equality constraint is probably due to the heuristic nature of the BONMIN algorithm when dealing with non-convex optimization problems.
Figure 8 shows how fishing effort is allocated optimally to maximize expected return within a fishing year across the cod, saithe, and haddock fisheries. Unsurprisingly, the pattern of effort allocation resembles the catch pattern shown in Figure 7. An interesting fact is that the allocation of fishing effort has almost reached the maximum of 210 h (i.e., a fortnightly base) in the saithe and haddock fisheries, but not in the cod fishery. Evidently, as it is seen from Figure 8, an optimal trawl tends to spend less trawling time in the cod fishery each fortnight, despite the fact that he/she could have spent more time on trawling because the upper limit of effort on a fortnightly basis is 210 h.
Figure 9 shows the generated revenue from the adopted optimal harvest strategy. The total generated revenue is 68 million kroner, broken down by region and species. What is apparent from Figure 9 is the significant contribution of the cod fishery in the Arctic region to the fishing revenue. The generated revenue from the cod fishery in region A is approximately 45 million kroner, accounting for almost 70% of the total fishing revenue. The revenue from region B comes from mixed fisheries and the corresponding revenue share is 20 million kroner, accounting for approximately 30% of the total revenue. The source of revenue in region C is the saithe fishery, and its share is approximately 3 million kroner.
To validate our optimization results and assess the reliability of the model, we have performed a Monte Carlo simulation to see how the optimization results of the effort allocation differ from the simulation results. To this aim, the mean values of 100 Monte Carlo simulations with varying CPUEs have been obtained and compared with the mean value of the optimization result. As it shown in Figure 10, the trends are the same as our optimization solution. For example, no fishing has been conducted during fortnights 3–8 in region A. Conducting a mixed fishery is evident in region B. This finding underpins the robustness of our results.
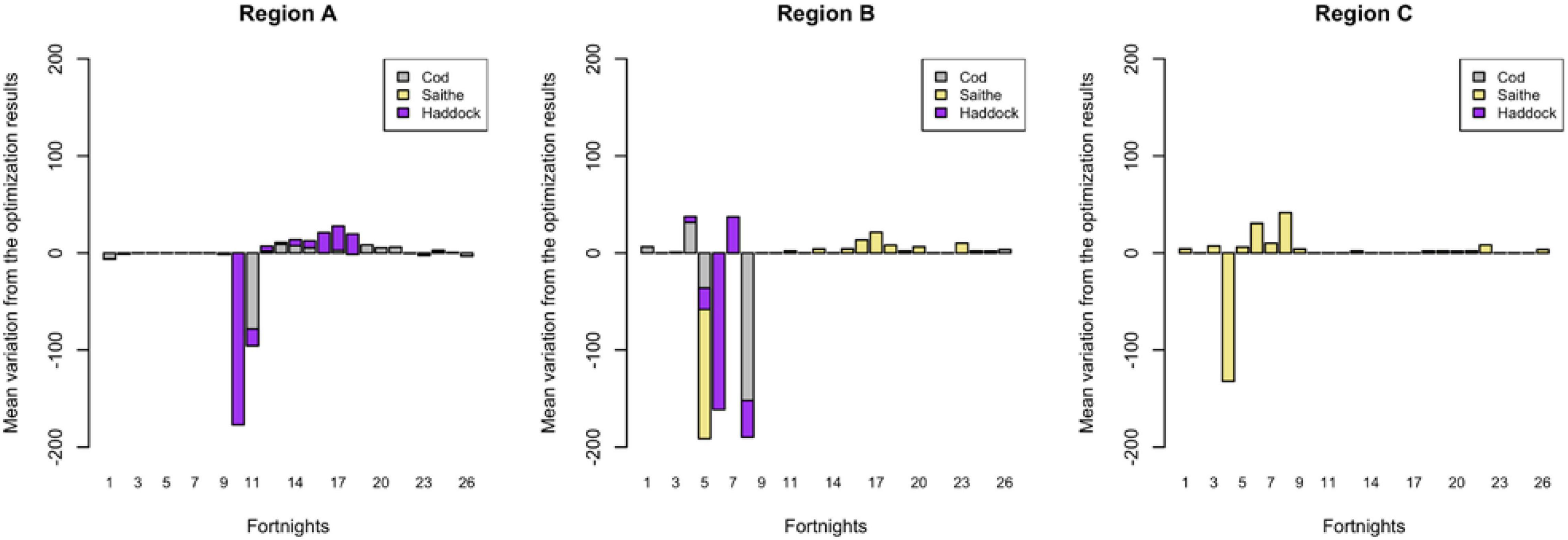
Figure 10. Mean variation from the optimization result. The numbers on the y-axis show the difference between the mean value of 100 Monte Carlo simulations and the main result presented in the paper.
Discussion
An optimal trawler starts fishing in the cod fishery in region A. There is one possible reason behind this harvest strategy. Early in the fishing season, cod is still plentiful in the Arctic area as the cod fish reach the spawning grounds along the northwest coast no earlier than March (Trout, 1957; Olsen et al., 2010). Moreover, at this time, the climatic condition of the Arctic is still suitable for the trawl vessels because the maximum sea ice extent of the Barents Sea generally occurs in March–April (fortnights 5–9) (Kvingedal, 2005; Årthun et al., 2012).
From the third fortnight (early February), an optimal trawler switches from the cod fishery in region A to region B, and becomes involved in a mixed fishery, where the quotas for cod, saithe, and haddock are utilized. A clear and concise explanation for the mixed fishery is most probably because of spawning aggregation of cod, saithe, and haddock along the northwest coast of Norway. This is to say that the overlap in the spatial distributions of the cod, saithe, and haddock increases the likelihood of catching non-target species and makes it more difficult for the fishers to decouple the target species from non-target species. Needless to say this makes it difficult for the fishers to balance the catch size and the remaining quota.
Surprisingly, at this time, an optimal trawler focuses on utilization of haddock and saithe quotas rather than the cod quota despite the fact that the availability (i.e., the CPUE) of cod is still relatively high (see Figure 4, middle panel). Even though the introduction of IVQ system has corrected “race to fish” incentives, it has not taken away the possible influences of fishers’ behavior on adopted harvest strategy of other counterparts (Boyce, 1992). Put differently, as soon as the fishery season opens, coastal fishers with different fishing gear such as gillnets cluster along the northwest coast of Norway to fish the cod quota, which is of high economic importance. Good availability of cod and the reduced cost of fishing caused by spawning aggregation (Sandberg, 2006; Hannesson, 2007) along the coast motivate the coastal fishers to fish the cod quota during the winter (Hermansen and Dreyer, 2010; Alizadeh Ashrafi et al., 2020). Moreover, unlike the trawl vessels that are flexible in terms of effort allocation across space, coastal boats are geographically less mobile, a factor that hinders them from off-shore fishing in the Arctic area to target cod and haddock. Hence, aggregation of cod along the coast creates a great opportunity for them to fish their quotas. In essence, the coastal fishing fleet receives a 65–80% share of the TAC for codfish (Asche et al., 2014; Birkenbach et al., 2020). The sudden increase in supply and large amount of landed cod lower the price of cod because the cod price is sensitive to the quantity supplied (Arnason et al., 2004; Birkenbach et al., 2020; see also Figure 6). The reduction in cod price discourages the optimal trawler to catch the cod quota, meaning that it is no longer profitable for the trawler to use the cod quota in region B. It seems that targeting saithe and haddock in region B and fishing saithe in region C are secondary resources to which an optimal trawler switches when the price of cod is reduced.
In region B, a small portion of cod is fished as the main target species, despite the low prices. This could be explained by the fact that quotas need to be exhausted by the end of the year. This means that, even though IVQs enable fishers to fish flexibly over the course of a year by responding to the changes in price and CPUE, the time at which price and CPUE values are higher could be an important driver on fishers’ decision-making process. The price of cod is higher toward the end of the year (see Figure 6) and waiting too long to utilize cod quota increases the risk of underutilization of cod quota and economic loss because of inability to exhaust the quota. Hence, an optimal trawl might choose to fish a small portion of cod in region B in winter to avoid potential economic loss.
Withdrawing from the cod fishery despite the reduction in the cost of harvesting signals that the reduction in price outweighs the reduction in the cost of fishing. This result is in accordance with the study of Alizadeh Ashrafi et al. (2020). The authors found that the magnitude in price reduction in cod is larger than the reduction in the cost during the spawning aggregation in winter, which in turn yields negative returns. Another rationale for the choice of region B to conduct fishing in early February is that as time elapses toward March, the maximum Arctic sea-ice extent begins take to shape (Kvingedal, 2005; Årthun et al., 2012), a factor that could increase the risk of fishing in region A and might impede the allocation of fishing effort. The negative effect of ice in the Barents Sea on trawlers’ behavior is confirmed by Alizadeh Ashrafi and Abe (2021).
In the eighth fortnight, an optimal trawler reallocates fishing effort in region C to target saithe to exhaust the remaining quota. This optimization result is consistent with the findings from Birkenbach et al. (2020), in which a profit-maximizing trawl fisher exhausts the saithe quota during a relatively short period of time in the winter. One possible reason for exhausting saithe quota during a short period of winter (fortnights 4-5-8) could be the fact that the CPUE of the saithe does not vary considerably (see Figure 4), which in turn might lead to more stable prices (see Figure 6). Another reason for the invariant price of saithe could be that this variable is not responsive to the changes in supplied and demanded quantities (Birkenbach et al., 2020). Thus, the invariant price of saithe and reduced price of cod underlie the decision to substitute cod with saithe in the winter.
From the ninth fortnight onward, an optimal trawler redistributes in region A to target cod and utilize the remaining quota. At this time, cod swims northward to the high sea areas of the Arctic to feed. This decision underlying effort allocation is driven by an economically rational behavior as the price of cod starts to rise from May (see Figure 6), which contributes to the increase in the expected returns. A possible explanation for the increase in the price of cod is that at this time coastal fishers have already harvested their cod quotas; hence, cod landings are limited. Related to this, Asche et al. (2002) and Arnason et al. (2004) have found that the cod price is very responsive with respect to the changes in landings. Moreover, from around May, the melting of sea ice provides better climatic conditions for fishing in the Arctic region. The capability of trawl vessels to conduct off-shore fishing in the high sea areas of the Artic indisputably shapes the harvest strategy. In essence, we see that the trawlers tend to spread the cod catch over the course of a year and they purposefully prefer not to allocate maximum fishing effort each fortnight (see Figure 8). Birkenbach et al. (2020) argued that the rationale behind this harvest strategy is due to the downward-sloping demand curve for cod.
The results of this study are beneficial for the trawlers by showing them how to optimally allocate fishing effort to maximize the expected return of the quota portfolio. Even though the introduction of IVQ system is a necessary condition to increase expected return, lack of knowledge about when and where to fish what and how much to land leads to a non-optimal harvest strategy. Additionally, an understanding of trawlers’ effort allocation could address the pressure on the sea bottom, habitat disruption and ecological detriment as this fishing technique can cause damage on the seabed (He and Winger, 2010). To attenuate of the possible erosion on the seabed appropriate management actions could be proposed and implemented. Hence, the results of our study contribute to environmentally and economically sustainable fisheries in the long-term.
The optimization model used in this study can be applied to different quota-managed multi-species fisheries to improve the effort allocation decisions, remove excessive effort, and consequently enhance the economic status of the fisheries by making modifications in the previously adopted harvest strategy.
Conclusion
Large industrial vessels like bottom trawlers fish to increase expected return. It is conventionally assumed that fishers displace their fishing effort according to the expected economic returns from fishing in the available fisheries and locations. Effort allocation is a multi-faceted process, and the decisions underlying optimum effort allocation and utilization of the quota portfolio are determined by a multitude of interconnected factors such as when, where, and what to fish, and how much of the quota for each fishery to utilize. Each of these components carries a tradeoff between the potential returns against anticipated costs.
The specific characteristics of the Norwegian codfish fishery amplify the difficulty to adopt a harvest pattern to achieve the maximum economic yield. The Norwegian trawlers hold a fishing portfolio for multiple species with heterogeneous biological and/or market conditions. In addition, the migratory behavior of the codfish over a vast geographical area and constant variation in fish abundance influence the catch composition and expected return. The spawning aggregation of cod, saithe, and haddock along the northwest coast and the joint harvest of codfish by coastal fishers during the winter months further complicates the identification of the optimal harvest schedule to maximize expected return. Moreover, these fisheries are managed through separate catch quotas; hence, fishers need to balance constantly the catch and the remaining quota to take advantages of varying bio-economic conditions while adhering to the quota constraints.
Given the above-mentioned complexity, the objective of this paper was to investigate how to allocate fishing effort optimally and to use the quota portfolio in the Norwegian trawl fleet across three different locations to target codfish within a fishing season to achieve maximum annul expected return, using the RPUE as a measurement of economic expected return. We have solved the multi-location and multi-period harvest problem through developing a mixed integer non-linear optimization model. Our findings show that the migratory behavior of NEA cod to spawn along the northwest coast of Norway and to feed in the high sea areas of the Arctic, the congregation of coastal boats during spawning season, and the freedom in spatial expansion and quota regulations influence the optimal redistribution of fishing effort across time and space. The outcomes of this study further disclose that the cod fishery in the Barents Sea and the saithe fishery in the North Sea are the most and least productive fisheries, respectively.
We believe the findings of this study will be useful for fishers by providing recommendations on how to utilize their fishing rights in a revenue-maximizing manner. The results of this study are also beneficial for the fisheries managers because they provide some insight about the motivation behind the adopted behavior of the trawlers to assess and to advise how possible changes in regulatory scheme might influence the harvest pattern.
Data Availability Statement
The catch and effort data are openly available in the Norwegian Directorate of Fisheries at https://www.fiskeridir.no/. Fish prices are publicly available in the Norwegian Fishermen’s Sale Organization at https://www.rafisklaget.no/.
Author Contributions
TA conceived the ideas, suggested the methodology, and collected the data. AE and AN analyzed the data and developed the model. AE did the optimization. AN did Monte Carlo simulation to add stochasticity into the optimization model. TA, AE, and AN wrote the manuscript. All authors contributed critically to the drafts and gave final approval for publication.
Conflict of Interest
The authors declare that the research was conducted in the absence of any commercial or financial relationships that could be construed as a potential conflict of interest.
Publisher’s Note
All claims expressed in this article are solely those of the authors and do not necessarily represent those of their affiliated organizations, or those of the publisher, the editors and the reviewers. Any product that may be evaluated in this article, or claim that may be made by its manufacturer, is not guaranteed or endorsed by the publisher.
Supplementary Material
The Supplementary Material for this article can be found online at: https://www.frontiersin.org/articles/10.3389/fmars.2021.738912/full#supplementary-material
Footnotes
- ^ https://www.fiskeridir.no/Yrkesfiske/Dokumenter/Reguleringsmoetet2/Hoeringer-av-reguleringer-for-2021-reguleringsmoetet
References
Alizadeh Ashrafi, T., and Abe, K. (2021). Intra- and inter-temporal effort allocation and profit-maximizing strategy of trawl fishery. ICES J. Mari. Sci. 78, 2943–2957.
Alizadeh Ashrafi, T., Eide, A., and Hermansen, Ø (2020). Spatial and temporal distributions in the norwegian cod fishery. Nat. Resour. Mod. 33:e12276. doi: 10.1111/1365-2656.12678
Andersson, J. A., Gillis, J., Horn, G., Rawlings, J. B., and Diehl, M. (2019). CasADi: a software framework for nonlinear optimization and optimal control. Math. Prog. Comput. 11, 1–36.
Arnason, R., Sandal, L. K., Steinshamn, S. I., and Vestergaard, N. (2004). Optimal feedback controls: comparative evaluation of the cod fisheries in denmark, iceland, and norway. Am. J. Agric. Econ. 86, 531–542.
Årthun, M., Eldevik, T., Smedsrud, L., Skagseth, Ø, and Ingvaldsen, R. (2012). Quantifying the influence of atlantic heat on barents sea ice variability and retreat. J. Climate 25, 4736–4743.
Asche, F., Bjørndal, M. T., and Bjørndal, T. (2014). Development in fleet fishing capacity in rights based fisheries. Mar. Policy 44, 166–171.
Asche, F., Chen, Y., and Smith, M. D. (2015). Economic incentives to target species and fish size: prices and fine-scale product attributes in norwegian fisheries. ICES J. Mar. Sci. 72, 733–740.
Asche, F., Gordon, D. V., and Hannesson, R. (2002). Searching for price parity in the European whitefish market. Appl. Econ. 34, 1017–1024. doi: 10.1080/00036840110061965
Asche, F., Gordon, D. V., and Hannesson, R. (2004). Tests for market integration and the law of one price: the market for whitefish in France. Mar. Resour. Econ. 19, 195–210. doi: 10.1086/mre.19.2.42629428
Babcock, E. A., and Pikitch, E. K. (2000). A dynamic programming model of fishing strategy choice in a multispecies trawl fishery with trip limits. Can. J. Fish. Aquatic Sci. 57, 357–370.
Batsleer, J., Hamon, K. G., van Overzee, H. M., Rijnsdorp, A. D., and Poos, J. (2015). High-grading and over-quota discarding in mixed fisheries. Reviews in Fish Biology and Fisheries 25, 715–736.
Béné, C., and Tewfik, A. (2001). Fishing effort allocation and fishermen’s decision making process in a multi-species small-scale fishery: analysis of the conch and lobster fishery in turks and caicos islands. Hum. Ecol. 29, 157–186.
Bergstad, O., Jørgensen, T., and Dragesund, O. (1987). Life history and ecology of the gadoid resources of the barents sea. Fish. Res. 5, 119–161. doi: 10.1016/0165-7836(87)90037-3
Birkenbach, A. M., Cojocaru, A. L., Asche, F., Guttormsen, A. G., and Smith, M. D. (2020). Seasonal harvest patterns in multispecies fisheries. Environ. Resour. Econ. 75, 631–655. doi: 10.1007/s10640-020-00402-7
Birkenbach, A. M., Kaczan, D. J., and Smith, M. D. (2017). Catch shares slow the race to fish. Nature 544, 223–226. doi: 10.1038/nature21728
Boyce, J. R. (1992). Individual transferable quotas and production externalities in a fishery. Nat. Resour. Mod. 6, 385–408.
Branch, T. A., Hilborn, R., Haynie, A. C., Fay, G., Flynn, L., Griffiths, J., et al. (2006). Fleet dynamics and fishermen behavior: lessons for fisheries managers. Can. J. Fish. Aquatic Sci. 63, 1647–1668. doi: 10.1139/f06-072
Campbell, R. A. (2004). CPUE standardisation and the construction of indices of stock abundance in a spatially varying fishery using general linear models. Fish. Res. 70, 209–227.
Copes, P. (1986). A critical review of the individual quota as a device in fisheries management. Land Econ. 62, 278–291. doi: 10.2307/3146392
Dupont, D. P., Fox, K. J., Gordon, D. V., and Grafton, R. Q. (2005). Profit and price effects of multi-species individual transferable quotas. J. Agric. Econ. 56, 31–57. doi: 10.1111/j.1477-9552.2005.tb00121.x
Flaaten, O., and Heen, K. (2004). Fishing vessel profitability and local economic link obligations—the case of Norwegian trawlers. Mar. Policy 28, 451–457.
Fulton, E. A., Smith, A. D., Smith, D. C., and van Putten, I. E. (2011). Human behaviour: the key source of uncertainty in fisheries management. Fish Fisher. 12, 2–17. doi: 10.1111/j.1467-2979.2010.00371.x
Gordon, H. S. (1953). An economic approach to the optimum utilization of fishery resources. J. Fisher. Board Can. 10, 442–457. doi: 10.1111/j.1865-1682.2012.01368.x
Gordon, H. S. (1954). The economic theory of a common-property resource: the fishery. J. Political Econ. 62, 178–203.
Grafton, R. Q. (1996). Individual transferable quotas: theory and practice. Rev. Fish Biol. Fisher. 6, 5–20. doi: 10.1007/bf00058517
Grafton, R. Q., Arnason, R., Bjørndal, T., Campbell, D., Campbell, H. F., Clark, C. W., et al. (2006). Incentive-based approaches to sustainable fisheries. Can. J. Fisher. Aquatic Sci. 63, 699–710.
Gullestad, P., Blom, G., Bakke, G., and Bogstad, B. (2015). The “discard ban package”: experiences in efforts to improve the exploitation patterns in norwegian fisheries. Mar. Policy 54, 1–9.
He, P., and Winger, P. D. (2010). “Effect of trawling on the seabed and mitigation measures to reduce impact,” in Behavior of Marine Fishes: Capture Processes and Conservation Challenges, ed. P. He (Ames Iowa: Wiley), 295–314.
Hannesson, R. (2007). A note on the”stock effect”. Mar. Resour. Econ. 22, 69–75. doi: 10.1086/mre.22.1.42629536
Hermansen, Ø, and Dreyer, B. (2010). Challenging spatial and seasonal distribution of fish landings—the experiences from rural community quotas in norway. Mar. Policy 34, 567–574.
Hilborn, R. (1985). Fleet dynamics and individual variation: why some people catch more fish than others. Can. J. Fisher. Aquatic Sci. 42, 2–13.
Hilborn, R. (2007). Managing fisheries is managing people: what has been learned? Fish Fisher. 8, 285–296.
Hilborn, R., and Walters, C. J. (1992). Quantitative Fisheries Stock Assessment: Choice, Dynamics and Uncertainty. New York: Chapman and Hall.
Johnsen, J. P., and Eliasen, S. (2011). Solving complex fisheries management problems: what the EU can learn from the nordic experiences of reduction of discards. Mar. Policy 35, 130–139. doi: 10.1016/j.marpol.2010.08.011
Kvingedal, B. (2005). Sea-Ice Extent and Variability in the Nordic Seas, 1967—2002 Ph. D, Thesis. Bergen: University of Bergen.
Mangel, M., and Clark, C. W. (1988). Dynamic Modeling in Behavioral Ecology. Princton: Princeton University Press.
Nielsen, M., Smit, J., and Guillen, J. (2012). Price effects of changing quantities supplied at the integrated European fish market. Mar. Resour. Econ. 27, 165–180. doi: 10.5950/0738-1360-27.2.165
Nocedal, J., and Wright, S. (2006). Numerical Optimization. New York: Springer Science & Business Media.
Norwegian Directorate of Fisheries (2019). Profitability Survey of the Norwegian fishing fleet. Bergen: Norwegian Directorate of Fisheries.
Nærings og Fiskeridepartementet (2009). Lov Om Forvaltning Av Viltlevande Marine Ressursar (Havressurslova). Norsk Lovtidend avd I, 6. Bergen: Norwegian Directorate of Fisheries.
Olsen, E., Aanes, S., Mehl, S., Holst, J. C., Aglen, A., and Gjøsæter, H. (2010). Cod, haddock, saithe, herring, and capelin in the barents sea and adjacent waters: a review of the biological value of the area. ICES J. Mar. Sci. 67, 87–101.
Poos, J. J., Bogaards, J., Quirijns, F., Gillis, D., and Rijnsdorp, A. (2010). Individual quotas, fishing effort allocation, and over-quota discarding in mixed fisheries. ICES J. Mar.ine science 67, 323–333.
Rubinstein, R. Y., and Kroese, D. P. (2016). Simulation and the Monte Carlo method. New Jersey: John Wiley & Sons.
Salas, S., and Gaertner, D. (2004). The behavioural dynamics of fishers: management implications. Fish Fisher. 5, 153–167.
Sanchirico, J. N., Holland, D., Quigley, K., and Fina, M. (2006). Catch-quota balancing in multispecies individual fishing quotas. Mar. Policy 30, 767–785.
Sandberg, P. (2006). Variable unit costs in an output-regulated industry: the fishery. Appl. Econ. 38, 1007–1018. doi: 10.1080/00036840500405912
Schaefer, M. B. (1954). Some aspects of the dynamics of populations important to the management of the commercial marine fisheries. Int. Am. Trop. Tuna Commission Bulletin 1, 23–56.
Standal, D., and Asche, F. (2018). Hesitant reforms: the Norwegian approach towards ITQ’s. Mar. Policy 88, 58–63. doi: 10.1016/j.marpol.2017.11.005
Standal, D., and Hersoug, B. (2015). Shaping technology, building society; the industrialization of the Norwegian cod fisheries. Mar. Policy 51, 66–74.
Trout, G. (1957). The bear island cod: migrations and movement. Ministry Agric. Food Fisher. Investigat. Ser. 2:21.
van Oostenbrugge, J. A., Bakker, E., van Densen, W. L., Machiels, M. A., and van Zwieten, P. A. (2002). Characterizing catch variability in a multispecies fishery: implications for fishery management. Can. J. Fisher. Aquatic Sci. 59, 1032–1043. doi: 10.1139/f02-078
Keywords: trawl fishery, codfish fishery, expected revenue, spatiotemporal dynamics, effort allocation, individual vessel quota
Citation: Alizadeh Ashrafi T, Ersdal AM and Nordli AS (2022) A Multi-Region and Multi-Period Harvest Schedule of the Trawl Fleet. Front. Mar. Sci. 8:738912. doi: 10.3389/fmars.2021.738912
Received: 19 August 2021; Accepted: 15 December 2021;
Published: 21 January 2022.
Edited by:
Brett W. Molony, Oceans and Atmosphere (CSIRO), AustraliaReviewed by:
Célia M. Teixeira, Center for Marine and Environmental Sciences (MARE), PortugalSatoshi Yamazaki, University of Tasmania, Australia
Copyright © 2022 Alizadeh Ashrafi, Ersdal and Nordli. This is an open-access article distributed under the terms of the Creative Commons Attribution License (CC BY). The use, distribution or reproduction in other forums is permitted, provided the original author(s) and the copyright owner(s) are credited and that the original publication in this journal is cited, in accordance with accepted academic practice. No use, distribution or reproduction is permitted which does not comply with these terms.
*Correspondence: Tannaz Alizadeh Ashrafi, dGFubmF6LmFsaXphZGVoQHVpdC5ubw==