- 1National Center for Ecological Analysis and Synthesis, University of California, Santa Barbara, Santa Barbara, CA, United States
- 2Future Earth, School of Global Environmental Sustainability, Colorado State University, Fort Collins, CO, United States
- 3California Sea Grant, Scripps Institution of Oceanography, University of California, San Diego, San Diego, CA, United States
- 4CSIRO, Oceans and Atmosphere, Hobart, TAS, Australia
- 5Institute for Marine and Antarctic Studies, University of Tasmania, Hobart, TAS, Australia
- 6Scientific Committee on Oceanic Research, Robinson Hall, College of Earth, Ocean and Atmosphere, University of Delaware, Newark, DE, United States
- 7Departamento de Estudios Ambientales, Universidad Simón Bolívar, Caracas, Venezuela
- 8Earth Ocean and Ecological Sciences, School of Environmental Sciences, University of Liverpool, Liverpool, United Kingdom
- 9NOAA, U.S. Integrated Ocean Observing System, Silver Spring, MD, United States
- 10School of Earth and Environmental Sciences, University of Queensland, St Lucia, QLD, Australia
- 11U.S. Marine Mammal Commission, Bethesda, MD, United States
- 12Northwest Association of Networked Ocean Observing Systems, Applied Physics Laboratory, University of Washington, Seattle, WA, United States
- 13Pacific Community, Fisheries, Aquaculture and Marine Ecosystems, Noumea, New Caledonia
- 14Intergovernmental Oceanographic Commission of UNESCO, Ocean Biodiversity Information System, Oostende, Belgium
- 15North Pacific Marine Science Organization, Sidney, BC, Canada
- 16Department of Biology, University of Pisa, CoNISMa, Pisa, Italy
- 17South African Institute for Aquatic Biodiversity, Grahamstown, South Africa
- 18Department of Zoology and Entomology, Rhodes University, Grahamstown, South Africa
- 19Core Science Systems, Denver Federal Center, U.S. Geological Survey, Denver, CO, United States
- 20GEOMAR, Helmholtz Centre for Ocean Research/Helmholtz Metadata Collaboration, Bremen, Germany
- 21Brazilian Future Ocean Panel, São Paulo, Brazil
- 22Research Institute for Global Change, Japan Agency for Marine-Earth Science and Technology, Yokosuka, Japan
- 23BirdLife International, David Attenborough Building, Cambridge, United Kingdom
- 24Tennenbaum Marine Observatories Network and Marine GEO Program, Smithsonian Institution, Edgewater, MD, United States
- 25Stanford Center for Ocean Solutions, Stanford University, Stanford, CA, United States
- 26Scripps Institution of Oceanography, University of California, San Diego, La Jolla, CA, United States
- 27Alfred Wegener Institute Helmholtz Centre for Polar and Marine Research Section Polar Biological Oceanography, Bremerhaven, Germany
- 28Institute for Marine Sciences and Ocean Sciences Department, University of California, Santa Cruz, Santa Cruz, CA, United States
- 29Marine Biological Association, Plymouth, United Kingdom
- 30Institute for Marine Remote Sensing, College of Marine Science, University of South Florida, St Petersburg, FL, United States
- 31NOAA Fisheries – Office of Science and Technology, Silver Spring, MD, United States
- 32CORDIO East Africa, Mombasa, Kenya
- 33UN Environment Programme World Conservation Monitoring Centre, Cambridge, United Kingdom
- 34International Water Management Institute, Colombo, Sri Lanka
- 35Institute of Marine Research, Bergen, Norway
- 36Coastal Policy and Humanities Research, Vancouver, BC, Canada
- 37Global Biodiversity Information Facility, København, Denmark
- 38Ocean Conservancy, Santa Cruz, CA, United States
Maintaining healthy, productive ecosystems in the face of pervasive and accelerating human impacts including climate change requires globally coordinated and sustained observations of marine biodiversity. Global coordination is predicated on an understanding of the scope and capacity of existing monitoring programs, and the extent to which they use standardized, interoperable practices for data management. Global coordination also requires identification of gaps in spatial and ecosystem coverage, and how these gaps correspond to management priorities and information needs. We undertook such an assessment by conducting an audit and gap analysis from global databases and structured surveys of experts. Of 371 survey respondents, 203 active, long-term (>5 years) observing programs systematically sampled marine life. These programs spanned about 7% of the ocean surface area, mostly concentrated in coastal regions of the United States, Canada, Europe, and Australia. Seagrasses, mangroves, hard corals, and macroalgae were sampled in 6% of the entire global coastal zone. Two-thirds of all observing programs offered accessible data, but methods and conditions for access were highly variable. Our assessment indicates that the global observing system is largely uncoordinated which results in a failure to deliver critical information required for informed decision-making such as, status and trends, for the conservation and sustainability of marine ecosystems and provision of ecosystem services. Based on our study, we suggest four key steps that can increase the sustainability, connectivity and spatial coverage of biological Essential Ocean Variables in the global ocean: (1) sustaining existing observing programs and encouraging coordination among these; (2) continuing to strive for data strategies that follow FAIR principles (findable, accessible, interoperable, and reusable); (3) utilizing existing ocean observing platforms and enhancing support to expand observing along coasts of developing countries, in deep ocean basins, and near the poles; and (4) targeting capacity building efforts. Following these suggestions could help create a coordinated marine biodiversity observing system enabling ecological forecasting and better planning for a sustainable use of ocean resources.
Introduction
Marine ecosystems provide essential services to society, including food security, livelihoods, recreation, and nature-based climate solutions (Benway et al., 2019; König et al., 2019; Winther et al., 2020; Estes et al., 2021). Indeed, the ocean is opening new economic frontiers, and has been projected to provide over 3 trillion USD of added value to the global economy by 2030 (OECD, 2016). This demand for services is driving an increase in uses of the ocean at the same time that a rapidly changing climate is impacting marine ecosystems in ways that we don’t yet understand very well, limiting our ability to forecast and properly manage such uses in a sustainable manner (McCauley et al., 2015; Golden et al., 2017). Such an understanding requires information founded on scientific observations. Long-term and spatially representative measurements of marine ecosystems are vital to: (1) detect seasonal, annual, and decadal climate variability and trends, (2) distinguish between natural and human-induced change, (3) understand the causes of change (e.g., ocean warming relative to extractive industries), (4) understand ecological mechanisms (e.g., food web interactions) and consequences of change (including adaptive capabilities), and (5) improve coupled physical, biogeochemical, and ecological forecasting. Such information underpins marine ecosystem management, conservation and development efforts, informs indicators of progress toward globally-agreed upon goals and targets, and is fundamental to achieve socially equitable and ecologically sustainable ocean economies (Benway et al., 2019; Rayner et al., 2019). Collecting this information and sharing it with stakeholders worldwide requires a coordinated, integrated ocean observing system, as recognized by many international entities and processes, including the United Nations Decade of Ocean Science for Sustainable Development (2021–2030; Ryabinin et al., 2019; Heymans et al., 2020) and the High Level Panel for a Sustainable Ocean Economy (Winther et al., 2020).
Previous studies have inventoried biodiversity data and observations globally (Costello et al., 2010; Appeltans et al., 2016; Edgar et al., 2017), regionally in the Mediterranean (Tintoré et al., 2019), Pacific Ocean (Koslow and Couture, 2015), and along the coast of South America (Miloslavich et al., 2011; Cruz-Motta et al., 2020), and for fishes (Mora et al., 2008), as well as biodiversity time series (Dornelas et al., 2018). But there has been no detailed global assessment of active, long-term (>5 years), and systematic marine biological observing programs. Such an assessment is needed to inform strategic attempts to coordinate and fill gaps in the development of an observing system for marine biodiversity.
Coordination of long-term measurements on regional and global scales requires prioritization and standardization of observations facilitated through the implementation of ocean observing frameworks (Lindstrom et al., 2012; Tanhua et al., 2019a). Information needs can be met through products underpinned by specific, essential variables. The Essential Ocean Variables (EOVs; acronyms used throughout the manuscript are referenced in Table 1) and Essential Biodiversity Variables (EBVs), both of which have been advanced through observing frameworks, represent feasible (cost-effective), and high impact (scientific, policy, and societal relevance) examples of such variables (Pereira et al., 2013; Miloslavich et al., 2018; Muller-Karger et al., 2018a). Monitoring of these variables to address local information needs allows for the aggregation of data to characterize regional, global, and longer-term variation and trends, and assists in understanding how local changes fit in a regional and global context.
Currently, global observing systems for ocean physics (Sloyan et al., 2019) and biogeochemistry (Bakker et al., 2012; Tilbrook et al., 2019) are fairly well developed and coordinated internationally. A global ocean observing system (GOOS) for marine biodiversity, which is to be integrated with physics and biogeochemistry observing systems, is in the initial phase of development (Bax et al., 2019; Canonico et al., 2019). The biological EOVs defined in this observing system focus on the status and trends of habitats (e.g., cover and composition of hard corals, seagrasses, mangroves, and macroalgae), which are consistent with the International Union for Conservation of Nature Global Ecosystem Typology (Keith et al., 2020), and broad functional groups representing marine ecosystem components (e.g., diversity and biomass of microbes, phytoplankton, zooplankton, and the distribution and abundance of benthic invertebrates, fishes, sea turtles, seabirds, and marine mammals; Miloslavich et al., 2018). Biological EOVs have been proposed to address local and national needs and for tracking the progress of global agreements, such as the Convention on Biological Diversity (CBD), the United Nations 2030 Agenda for Sustainable Development (SDGs), and the United Nations Convention on Climate Change (UNFCCC; Miloslavich et al., 2018). Ocean color and acoustic characteristics of the environment (ocean sound) are now recognized as EOVs by multiple disciplines since they encompass a multitude of ecological properties of the ocean. The EOV framework allows for improved global data coordination for both EOVs and marine EBVs, since the data collected for EOVs are used to compute marine EBVs (Muller-Karger et al., 2018a).
Here, we build upon the identification and validation of biological EOVs (Miloslavich et al., 2018) by: (1) identifying the existing long-term biological observing programs that could serve as the foundation of an integrated system; (2) conducting a gap analysis to determine if the existing system infrastructure, sites, and operational environment will provide sufficient global coverage to support a robust biological ocean observing system (Bax et al., 2019); and (3) assessing the degree of coordination and connectivity among the existing components of the biological ocean observing system.
Materials and Methods
Criteria for Inclusion
Marine biodiversity studies often focus on local scales, particular habitats or taxa, and can be of limited duration due to cost, feasibility, and suitability for specific study goals. Their contribution to sustained and consistent long-term monitoring is therefore highly variable. For the purposes of this study, we identified observing programs that systematically sampled marine organisms in situ (as opposed to only via remote sensing, thus the ocean color EOV was not included in this study) for periods longer than 5 years. Remotely sensed measurements were excluded from this survey because they are more easily coordinated across large, global scales and because satellite remote sensing ultimately requires in situ verification.
Building an Inventory of Observing Programs
To identify an initial list of long-term, active marine biodiversity observing programs globally, we first identified long-term biological observing programs from previous studies (see Buttigieg et al., 2018; Miloslavich et al., 2018; Duffy et al., 2019) and from interviews with network leads and other domain experts, resulting in the identification of 254 programs. We then obtained contact information for all contributors of data relevant to biological EOVs in the Ocean Biodiversity Information System (OBIS, 2021; n = 1,491) using the OBIS Application Programming Interface (API)1. A preliminary survey was sent to these contacts to identify long-term biological monitoring programs and associated program leads, resulting in the identification of an additional 202 biological observing programs. We further searched for biological EOV keywords (microbes, phytoplankton, zooplankton, fishes, benthic invertebrates, turtles, seabirds, marine mammals, seagrasses, mangroves, macroalgae, hard corals, and ocean sound) in various online repositories and directories such as Dynamic Ecological Information Management System (deims.org/), Biological and Chemical Oceanography Data Management Office (bco-dmo.org), Pangaea Data publisher (pangaea.de), United Kingdom Directory of Marine Observing Programs datasheet (ukdmos.org), and European Directory of the Initial Ocean-Observing Systems (EDIOS, seadatanet.org/Metadata/EDIOS-Observing-systems) adding an additional 208 programs to our data pool. Finally, further programs were identified via the Long-term Ecological Research Network (lternet.edu) and Deep Ocean Observing System (deepoceanobserving.org) websites, the International Group for Marine Ecological Time Series (igmets.net; O’Brien et al., 2017), and the Alfred Wegener Institute. Collectively, these efforts generated a list of 891 biological observing entities globally, of which we identified valid contact information for 643 (Supplementary Material 1).
Survey Administration
An online survey of multiple choice and descriptive questions was sent to the primary contacts of the 643 individual observing entities to collect qualitative and quantitative information on each program. The survey was open from November 2019 to April 2020. Survey questions were developed based on the attributes recommended by the Observation Coordination Group of the Joint Technical Commission for Oceanography and Marine Meteorology (JCOMM, 2018), now called the Joint WMO-IOC Collaborative Board, which is responsible for the international coordination of oceanographic and marine meteorological observations, data management, and services. These attributes helped in our evaluation of programs in the context of global system readiness guidelines. We included questions on which biological EOVs were sampled, spatial extent of sampling, funding sources, sampling duration and frequency, if the programs were part of coordinating networks, whether the programs followed FAIR (findable, accessible, interoperable and reusable; Wilkinson et al., 2016) data principles, standards and best practices, and if the observing programs conducted capacity development (Supplementary Material 2). In addition, we collected information on the type of observing entity (e.g., primary data collector, data aggregator, data product developer, data user, and directory of observing programs) to ensure that we were including only programs that serve as foundational data providers, referred to as observing programs, in our analyses (definitions of terms and a conceptual model are depicted in Figure 1).
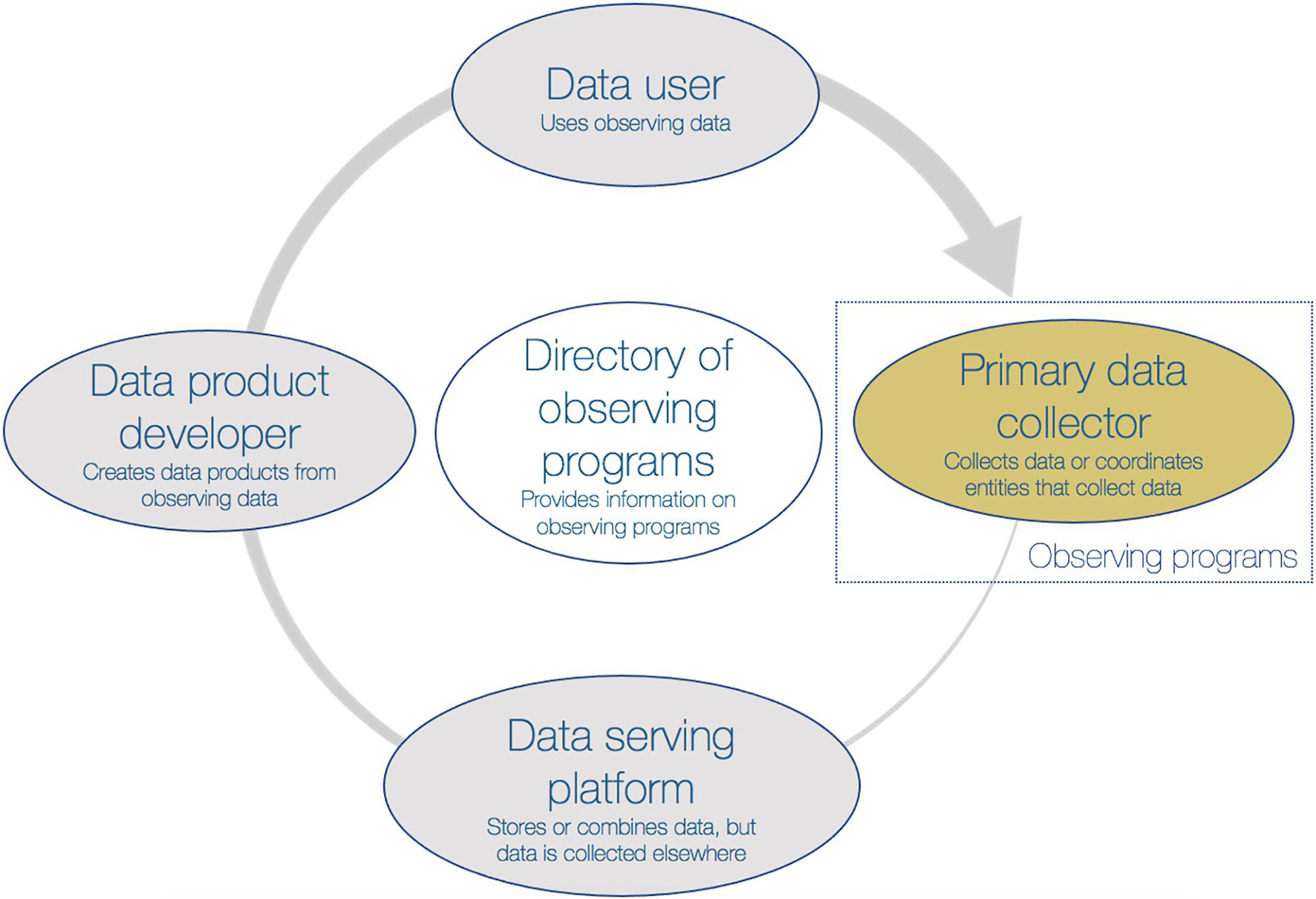
Figure 1. Types of observing entities within the context of the idealized ocean observing data lifecycle. Primary data collectors (yellow oval) can be individual programs or networks of data collectors (referred to as observing programs in this study). The data collected then feed into data serving platforms, such as repositories or aggregators, where they can be accessed by data product developers and then used by data users. Ideally, the data users then help guide refinement of the choice and methods of data collected to answer relevant scientific and societal questions to ensure a “fit for purpose” observing system. A directory of observing programs can provide essential information related to the observing entities in order to understand the key actors involved in the ocean observing data lifecycle. Definitions for each type of observing entity are provided below each category in the figure and are included in Supplementary Material 2 (Question 14). The thickness of the gray arrow indicates the value of the data, which increases as data are shared and used. The results of this study focus on the primary data collectors (observing programs; yellow oval in the dotted blue box) which could directly contribute data to a global ocean observing system.
Data Analysis: Summary Statistics, Spatial, and Network Analyses
Of the 643 observing entities that were sent the survey, 371 completed the survey, and of those programs that completed the survey 203 programs were primary data providers that were currently active, long-term (5 or more years old), and systematically sampled at least one of the EOVs (see section “Results”). All analyses and visualizations were conducted only on the primary data collectors (Figure 1, yellow oval) active, long-term observing programs that systematically sampled marine life (n = 203), using the statistical software R (Version 3.5.0, R Core Team, 2018).
Spatial Analyses
For the 203 active, long-term programs, geographic information was not available for 11 programs, and these were removed from further spatial analyses. 64 programs shared information directly on their sampling regions (polygons, points, or linestrings). Where only images such as jpeg files of sampling locations were available (n = 10), we manually extracted the coordinates using the R package “digitize” (Poisot, 2011). For the remaining programs (n = 118), we used the best available information on long-term sampling locations from published or online data. Spatial data were projected to the geodetic coordinate system EPSG:4326. To calculate spatial area, data were rasterized and aggregated to 0.5° grid cells (about 55 km2 at the equator) to align with the spatial resolution of common environmental source layers. Thus, any 0.5° grid cell that had a time series point in it was considered to have been sampled. Total ocean area and sampling area were calculated by taking the median cell size and multiplying by the number of cells. The sampling area divided by the total ocean area was used to determine the percentage of the global ocean covered by biological sampling. In addition, the percentage of the ocean covered by sampling was validated by calculating the proportion of cells with sampling over the total number of cells in the raster of the global ocean. To calculate the area of the global neritic, coastal zone (<200 m depth) around each continent, we used the General Bathymetric Chart of the Oceans gridded bathymetry data and developed a raster from 0 to 200 m depth (GEBCO compilation group, 2020). Spatial data manipulation and analyses were performed using the “sf” (Pebesma, 2018), “raster” (Hijmans, 2017), “fasterize” (Ross, 2020), “gdalUtils” (Greenberg and Mattiuzzi, 2018), “rgdal” (Bivand et al., 2019), “rgeos” (Bivand and Rundel, 2018), “lwgeom” (Pebesma, 2020), “geojsonsf” (Cooley, 2019), “geojson” (Chamberlain and Ooms, 2019), and “geojsonlint” (Chamberlain and Teucher, 2019) R packages.
To identify areas where we may have missed long-term observing programs, we compared the spatial coverage of programs from our survey against all identified records corresponding to the biological EOVs from OBIS and the Global Biodiversity Information Facility (GBIF), including taxa classifications from the coastal and marine records of the World Register of Marine Species (WoRMS Editorial Board, 2021): Biota, Animalia, Plantae, Fungi, Protozoa, Bacteria/Monera, Chromista, and Archaea (accessed OBIS: 8 July 2020, GBIF: 4 August 2020; Satterthwaite and Waller, 2020). Time series observations archived in OBIS and GBIF were aggregated into 0.5° grid cells and filtered to retain only individual datasets that had annually replicated samples in the same area for 5 or more years since 2014. The presence of long-term biological sampling (5 or more years) within different jurisdictions was determined by overlaying a map of 230 Exclusive Economic Zones (EEZs) onto program spatial areas (Flanders Marine Institute (VLIZ), 2019). To assess sampling bias and identify potential gaps for all EOVs across the entire global ocean and for habitat EOVs within the coastal zone, we applied Kolmogorov–Smirnov tests to compare sampled distributions to actual distributions for latitude and depth.
Network Analyses
To understand and illustrate linkages between observing programs, large coordinating networks, and data serving platforms, responses to the survey were mapped and analyzed using the “igraph” package in R (Csardi and Nepusz, 2006). Networks are composed of nodes and edges. The nodes correspond to the observing programs, coordinating networks, or data serving platforms and the edges correspond to directional connections (i.e., data transfer) between observing programs and the corresponding coordinating network (edge based on coordination) or data aggregators (edge based on data flow). Edges (connections) between nodes were unweighted and based on survey responses to open-ended survey questions (Supplementary Material 2). Edges between two data serving platforms were obtained from an online search of the website for each data serving platform, along with personal communications with relevant project leads in cases where the connection could not be found or was unclear. Edges were directed to indicate direction of data transfer (e.g., between a program and a data serving node or between two data serving nodes).
Results
We received responses from 58% (371 out of 643) of observing systems identified. Of the 371 total observing systems surveyed, 203 were currently active, long-term (5 or more years old), and systematically sampled at least one of the biological EOVs. The information, including the spatial extent, about these observing programs is available via a public metadata portal that is in development as part of the GOOS2. The ocean observing community can interact with and update the metadata portal via a feedback form and an administrator will update the information based on the feedback received. We encourage this community to provide further input and to keep this valuable resource up to date.
Biological Essential Ocean Variables Sampled by Existing Long-Term Biological Ocean Observing Programs
Phytoplankton (n = 98) was the most common biological EOV sampled by the 203 observing programs followed by zooplankton (n = 89), fishes (n = 88), benthic invertebrates (n = 83), marine mammals (n = 67), macroalgae (n = 55), seabirds (n = 39), microbes (n = 38), seagrasses (n = 33), hard corals (n = 26), ocean sound (n = 22), sea turtles (n = 20), and mangroves (n = 7).
Spatial Extent of Existing Long-Term Biological Ocean Observing Programs
Long-term observing programs from our survey covered 6% of the global surface ocean. Adding long-term datasets (>5 years) in OBIS and GBIF not identified in survey responses added an additional 1% coverage of the ocean, thus bringing the total ocean covered by biological observations to 7% (Figure 2). Of the total 7%, about 5% came from programs which monitored within EEZ boundaries with the remainder in Areas Beyond National Jurisdiction (ABNJ). Sampling was unevenly distributed throughout the global ocean across latitudes (D = 0.23, p < 0.001) and depths (D = 0.21, p < 0.001; Figure 3). Regions around 50 degrees north and south were relatively over-sampled, whereas under-sampled regions included mid-latitudes around the equator and the Arctic (Figure 3A) and within the bathyal, abyssal, and hadal regions of the ocean where the seafloor is deeper than 2,500 m (Figure 3B). Most of the ocean lacked long-term biological observations, including most of the open ocean as well as along the coasts of some parts of South America, Eastern Europe, Asia, parts of Oceania, and Africa. Considering all nations with a coastline, 22% had no identified sampling programs within their EEZ, including certain EEZs off Eastern and Western Africa, South America, in the South Pacific, the Caspian Sea (Figure 2). The much higher amount of sampling in waters less than 200 m is at least partly an artifact of habitat EOVs being restricted to, or more commonly found in the coastal and nearshore ocean.
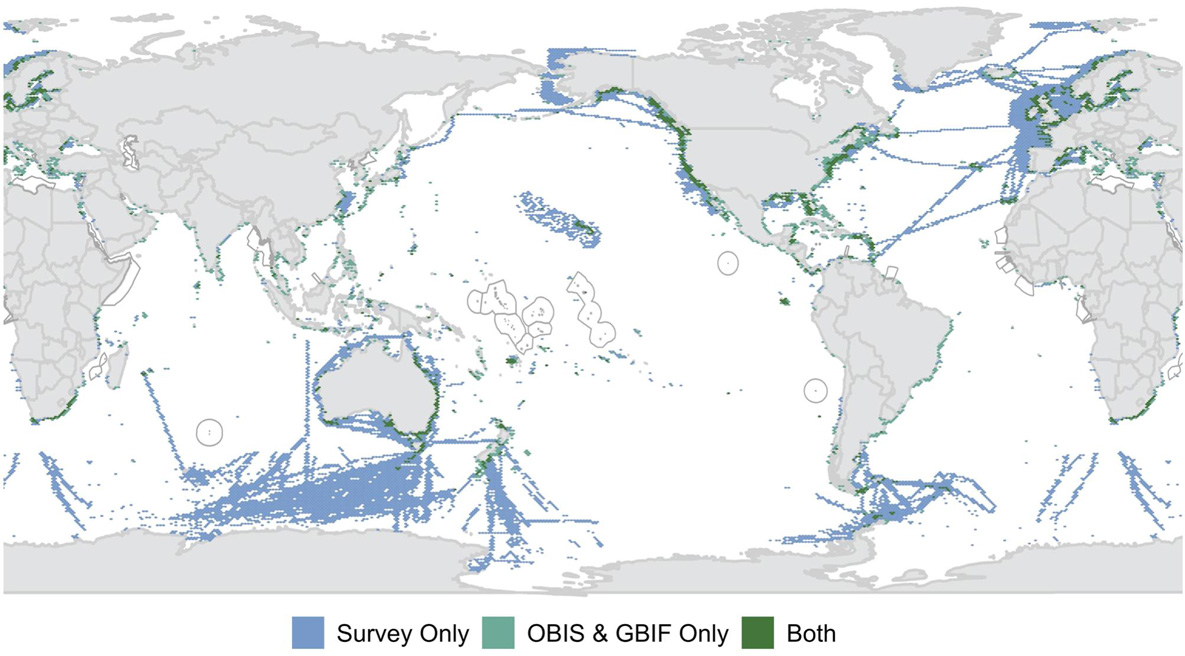
Figure 2. Spatial coverage of known active, long-term biological observations globally (colored regions). Color indicates biological observations identified from the survey only (blue- 5% of ocean surface), from datasets in the Ocean Biodiversity Information System (OBIS) and the Global Biodiversity Information System (GBIF) only (teal- 1% of ocean surface), and those identified in both sources (green- 1% of ocean surface; map displayed across ≥ 0.5° grid cell: about 55 km2 at the equator). Gray lines show Exclusive Economic Zones (EEZ; 200 nm) of nations with no known biological Essential Ocean Variable (EOV) sampling according to this study.
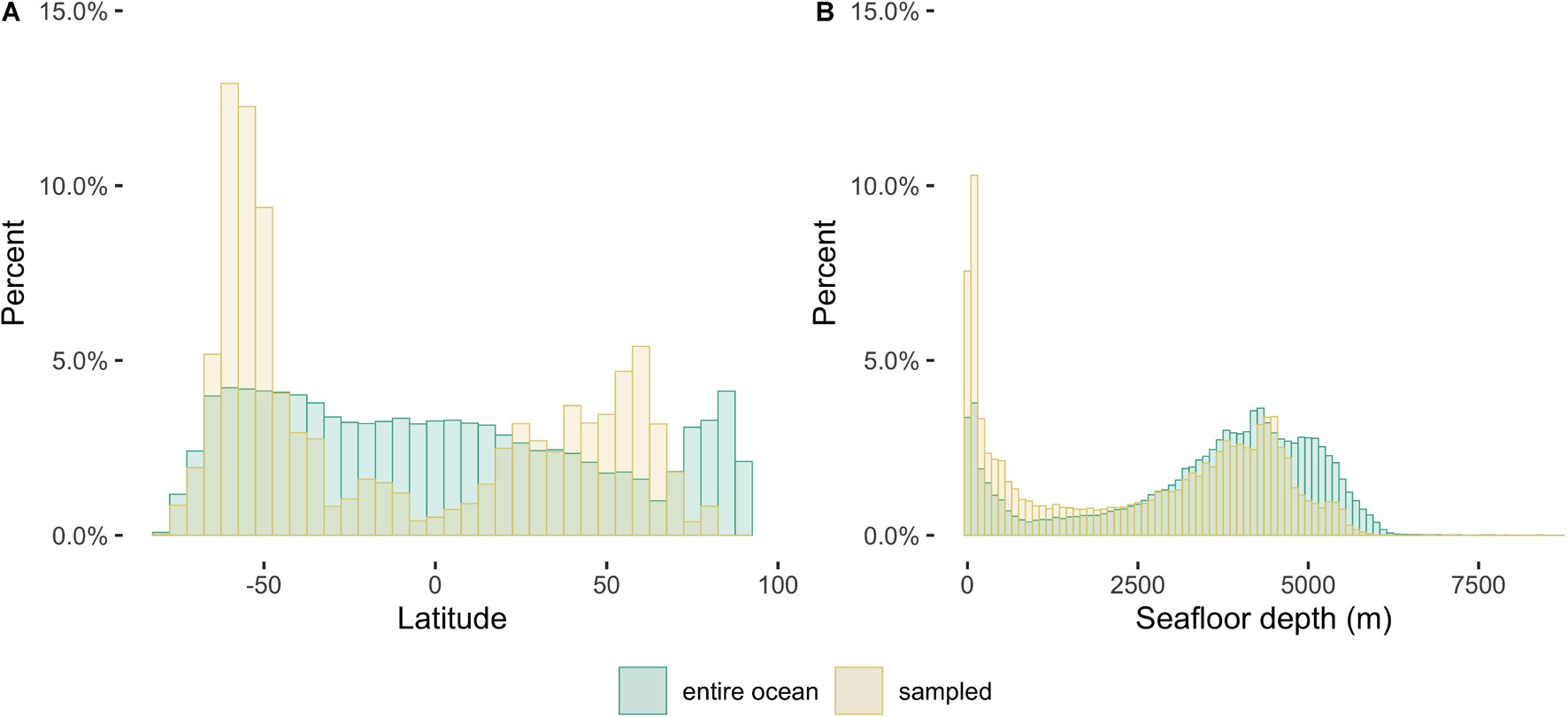
Figure 3. Histograms of latitude (A) and seafloor depth (B) for the global ocean area (“entire ocean”) compared with areas sampled by observing programs in this study (“sampled”). Results include the spatial information from the surveyed programs and the datasets from the Ocean Biodiversity Information System (OBIS) and the Global Biodiversity Information System (GBIF). Bin widths are 5 degrees for latitude (A) and 100 meters for seafloor depth (B). Regions that were under sampled are characterized by the entire ocean (blue bars) being greater than the sampled areas (gold bars), with the converse for oversampled areas.
According to our survey, approximately 6% of the coastal zone (<200 m depth) was covered by observing programs sampling habitat EOVs, such as seagrasses, mangroves, hard corals, and macroalgae. Habitat sampling was also unevenly distributed throughout the global coastal zone with respect to latitude (D = 0.36, p < 0.001) and depth (D = 0.11, p < 0.001).
Sustainability of Existing Biological Ocean Observations
Diversity of Funding Sources
Funding for the 203 active, long-term observing programs identified in our study came from government, civil society or volunteers, private sector, or grants to academic/research institutions. Over half of the programs were funded by a single sector (68%; n = 139), with the rest funded by multiple sectors (32%; n = 74; Figure 4). Of programs funded by a single sector, 61 programs were funded solely by government funds and 55 programs by academic grants.
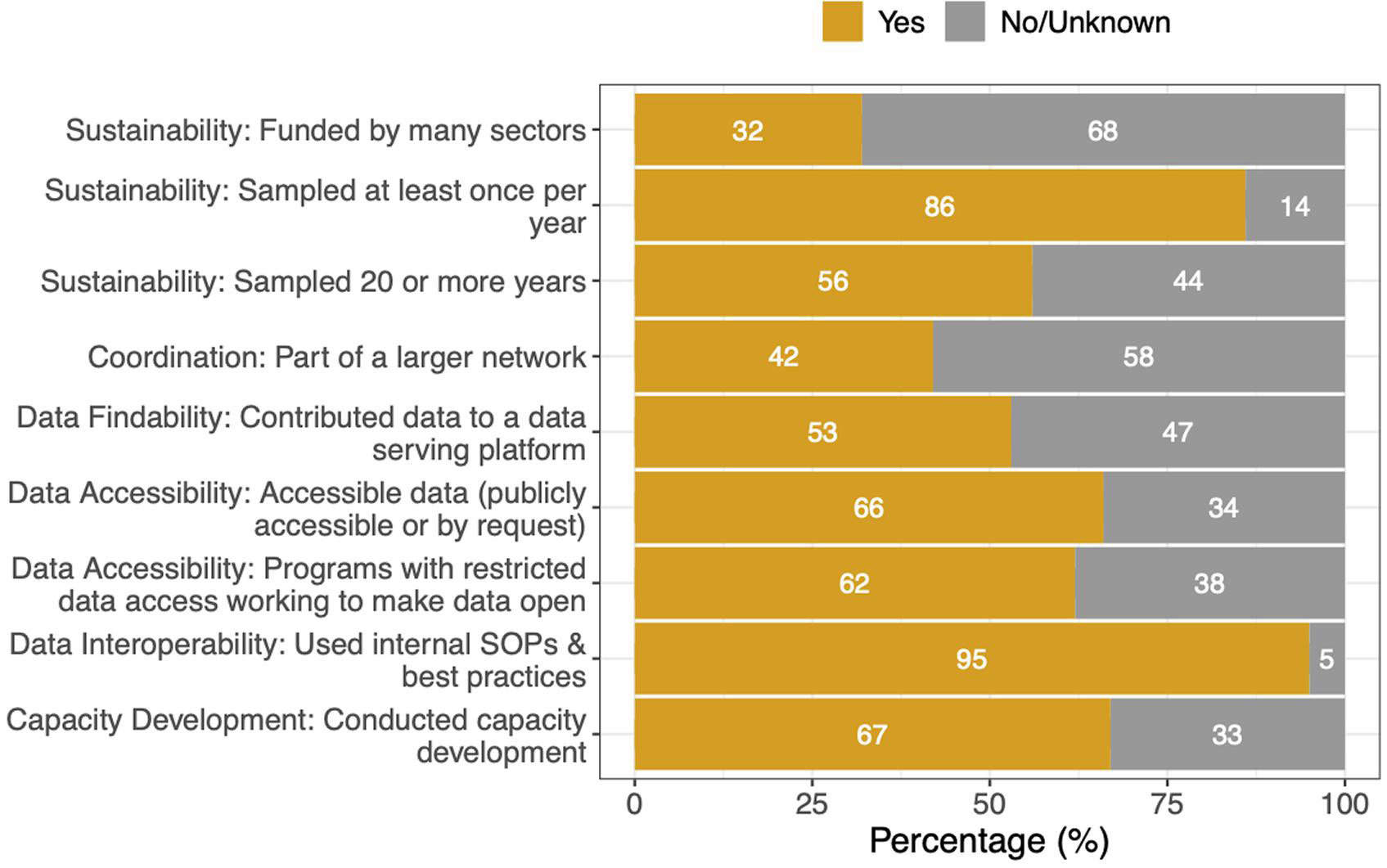
Figure 4. Bar plot based on survey responses displaying the 203 active, long-term observing programs sustainability (based on funding, sampling frequency, and duration), coordination, data management (including findable, accessible, and interoperable data), and existing capacity development efforts.
Sampling Duration and Frequency of Long-Term Biological Observing Programs
Over half (56%; n = 114) of the long-term (>5 years) biological observing programs have been sampling for more than 20 years and most of the long-term programs sampled at least once per year (86%; n = 174; Figure 4). Some of the currently active, longest-running biological observing programs from our survey are the Wetland Bird Survey, that monitors non-breeding water birds in the United Kingdom (since 1947), the California Cooperative Oceanic Fisheries Investigations (CalCOFI), that studies ecosystem dynamics in the California Current ecosystem (since 1949), and the Marine Biodiversity and Climate Change Project (MarClim), that samples rocky intertidal sites in the United Kingdom (since 1952).
Coordination of Existing Biological Ocean Observations
Overarching Coordination Networks
Of the 203 active long-term biological observing programs, under half (42%; n = 86) indicated that they were part of a larger coordinating observing network (Figure 4). Of these, 68 programs were part of at least one of eight large national and international coordinating networks identified by survey participants: GOOS, the Marine Biodiversity Observation Network (MBON), LTER, International LTER (ILTER), the U.S. Integrated Ocean Observing System (IOOS), Integrated Marine Observing System, OceanSITES, and the Global Alliance of Continuous Plankton Recorder (CPR) Surveys.
FAIR Data Standards
Findable Data: Tools Used by Existing Biological Observing Programs to Provide Data Access
Over half of the marine biological observing programs (53%; n = 108) contributed their data into a data repository, aggregator, or other data serving platform (Figure 4). The data serving platforms that were directly connected to the most data providers were global and regional in scope, such as OBIS, IOOS Regional nodes, GBIF, DataONE, National Biodiversity Network (NBN), and ERDDAP (Figure 5). ERRDAP was included because for some programs an ERDDAP serves as the primary location where observing programs provide online access to their data, so in essence, an ERDDAP acts as a repository, even though it is a framework for sharing data. In addition, although ERDDAP encompasses many different instances, we treated all ERRDAPs as broadly part of the same system. The data serving platforms that were directly or indirectly connected (through another data server as an intermediary) to the most data providers were GBIF, Atlas of Living Australia, European Marine Observation and Data Network, OBIS, DataONE, IOOS regional nodes, Archive for Marine Species and Habitats Data (DASSH), NBN, Global Archive, and ERDDAP. Of the 108 active, systematic long-term programs that contributed data into a data serving platform, 73 programs contributed data into at least one of the 18 most common data serving platforms (common refers to connections with three or more data contributors; Figure 5).
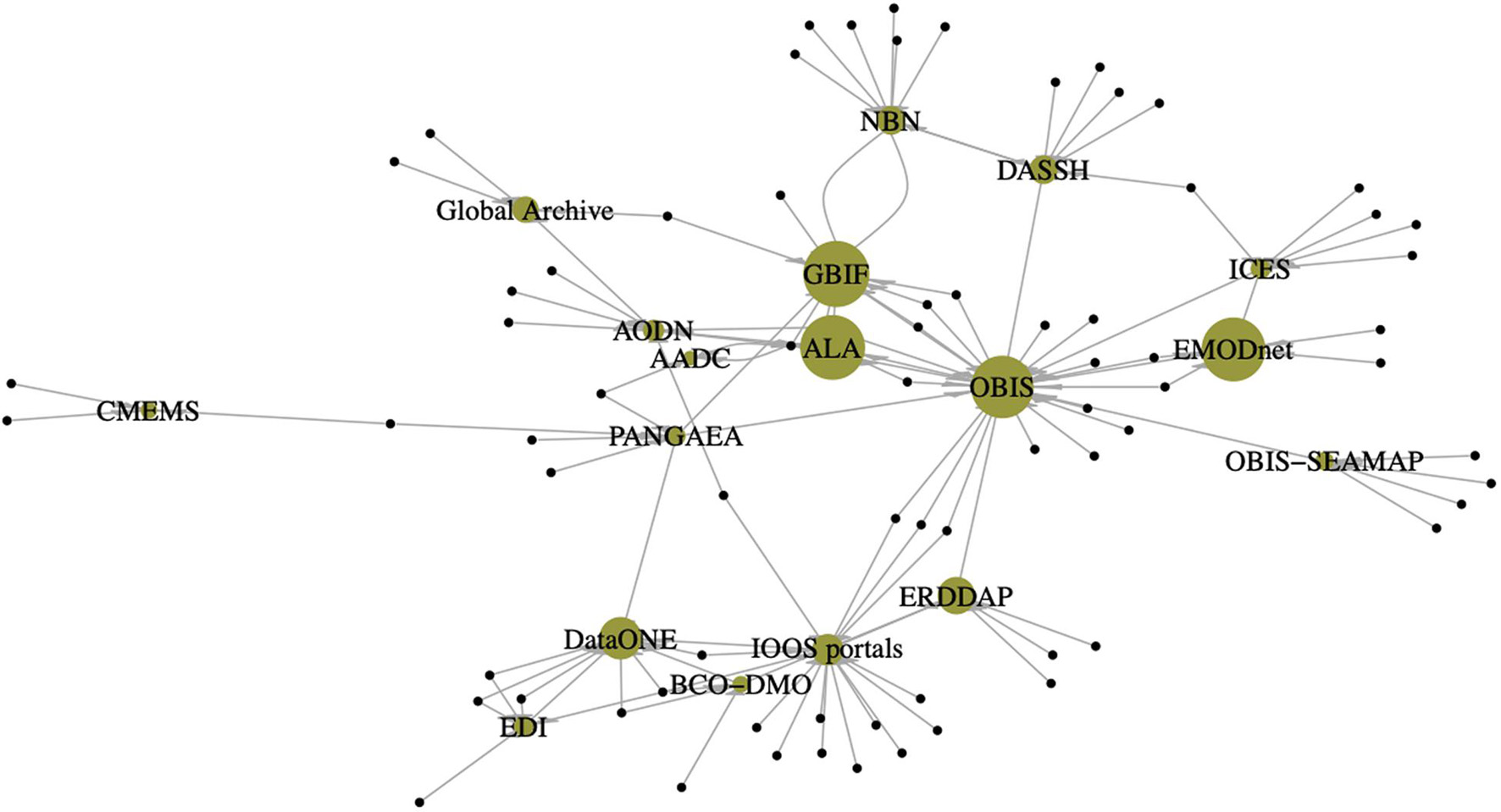
Figure 5. Network displaying data repositories, aggregators, or other data serving platforms (green circles; n = 18) and observing programs (small black circles; n = 73). Data servers and associated observing programs were only included in instances where three or more observing programs indicated that they provided data to a given data serving platform, in order to clearly display the most common data servers. The node size is scaled by the number of observing programs that provide data to a given data server, whether directly or through another intermediary. Larger nodes indicate data serving platforms with higher numbers of biological observing program datasets, whether the data were provided directly from the observing program or indirectly to the repository from another data repository (Acronyms are defined in Table 1).
Accessible Data: Data Access of Existing Biological Observing Programs
Two-thirds of the marine biological observing programs (66%; n = 135) responded that they had accessible data, either publicly accessible data (n = 49) or accessible by request (n = 86; Figure 4). The other one-third of programs evaluated (34%; n = 68) had limited data access because of a moratorium associated with data use or a secure login (n = 26), the data provider and another entity (e.g., contractor) only had access to the data (n = 18), only the data provider had access to the data (n = 17), or unknown data restrictions (n = 7).
Of observing programs that had limited access due to a moratorium, restricted, or fully restricted access (n = 68), nearly two-thirds stated that they were working to make their data fully open (62%; n = 42), a quarter were not working to make their data open (25%; n = 17), and the remaining did not respond (13%; n = 9; Figure 4). Of those that were not working to make their data open, the main reason for not making their data open was the lack of sufficient resources, such as funding and personnel (n = 6). Other reasons (n = 11) included: requests from data providers to keep data closed (e.g., due to fear of improper use or attribution by potential users); funding, institutional, or national policies in place that hinder data from being made available by the network (e.g., due to the possibility of data being used for commercial gain); a lack of incentives to share data; data are kept private or embargoed to be used for scientific, thesis, and other research studies; responsibilities associated with data sharing remain with individual researchers involved in the network; or a lack of an implemented data policy.
Of the 6% of the ocean covered by surveyed biological observing programs, most (5.3%) had accessible biological data from active, long-term observing programs (Figure 6; pink areas). Most accessible data were concentrated off the coasts of Western Europe, United States, Canada, Australia, Southern Africa, and around the Hawaiian Islands. In addition, accessible data existed in some parts of the Southern Ocean including near Drake Passage and south of Australia, along with some parts of the South Pacific. Half of one percent (0.5%) of the ocean was covered by observing programs working to make their data open (Figure 6; purple areas) and was generally concentrated in similar regions to those with open data. Most of the regions that had inaccessible data and were not working to make data open (0.2% of the ocean) were off of the northern part of North America, eastern India, southeastern tip of Australia, and China (Figure 6; teal areas).
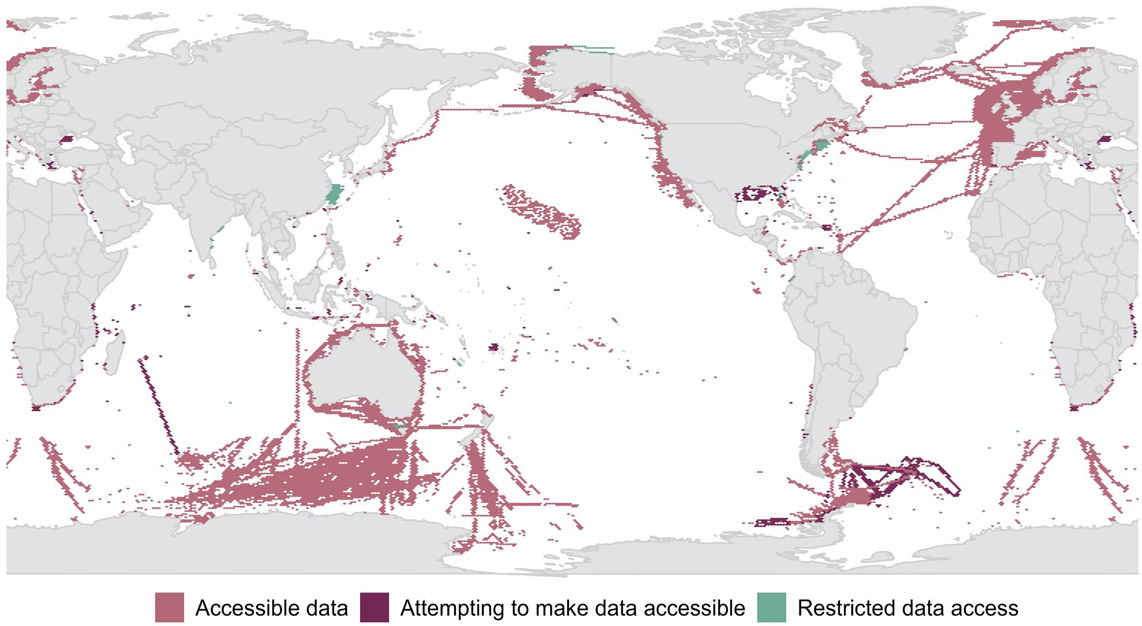
Figure 6. Spatial coverage of the 192 observing programs surveyed that had detailed spatial data and were currently active, had been sampling for 5 or more years, and sampled at least one EOV systematically (map displayed across ≥ 0.5° grid cell: about 55 km2 at the equator). Color differentiates data access, including data that are accessible whether publicly available online or accessible by request (pink), programs that are attempting to make data accessible (purple), and programs that have restricted data access (teal).
Interoperable and Reusable Data: Best Practices and Data and Metadata Standards Used by Existing Biological Observing Programs
Most of the biological observing programs (95%; n = 193) stated that they used standard operating procedures (SOPs) or protocols for data collection within the context of an individual program (Figure 4). Yet only one-tenth of these programs (n = 21) shared protocols with another program. Specifically, five programs shared the CPR Survey protocols (Richardson et al., 2006) and four programs shared methods in the HELCOM COMBINE Manual. The remaining programs shared methods with at least one other program including the CalCOFI methods (CalCOFI Methods, 2021), the ORCA protocol, guidelines from the Convention for the Conservation of Antarctic Marine Living Resources, the Superabundant, Abundant, Common, Frequent, Occasional and Rare abundance scale (Hiscock, 1990), benthic Baited Remote Underwater Video guide (Langlois et al., 2020), or the Atlantic Zonal Monitoring Program sampling protocol (Mitchell et al., 2002).
The data and metadata standards and specifications used by biological observing programs included various mutually compatible formats depending on the type of data and workflows. For example, Darwin Core for biological diversity data (Darwin core3), the Genomics Standards Consortium Minimum Information about any Sequence (MIxS) for genomic data (The National Microbiome Initiative4), and Open Geospatial Consortium standards for spatial information (OGC, 2021). Ecological Metadata Language was listed as a metadata standard for ecological research (Jones et al., 2019) and Network Common Data Form (NetCDF) was listed as a community standard for data sharing (Unidata5).
Capacity Development and Technology Transfer Supported by Existing Biological Observing Programs
Developing lasting human, institutional, and technical capacity underpins the long-term sustainability of observing programs, since recruiting, training, and mentorship of ocean professionals, novel innovations, and technological advancements are essential to ensure that observing systems adapt, evolve, and grow in response to changing needs over time (Bax et al., 2018). Of the 203 active long-term programs, over two-thirds (67%; n = 136) conducted capacity development and technology transfer (Figure 4). Examples included training for early career ocean observing professionals and citizen scientists, testing or hosting new instruments and sensors, and supporting projects focused on observing system technology development.
Discussion
Our global analysis identified 203 long-term biological observing programs that covered ∼7% of the global ocean (Figure 2). Based on our study, we suggest four key steps that can increase the sustainability, connectivity, and spatial coverage of biological EOVs in the global ocean: (1) sustain existing long-term observing programs to understand long-term trends and processes and encourage better communication and coordination among programs thus building connectivity across larger spatial scales; (2) promote and strive for FAIR data and the convergence toward common methods and community practices to ensure that observing data can be integrated across scales and domains; (3) expand biological ocean observations to fill gaps in sampling, including along coasts of developing countries, in deep ocean basins, and in the Arctic Ocean to enhance global coverage; and (4) leverage existing capacity development and technology transfer efforts to promote sustainability and broader coverage (Figure 7).
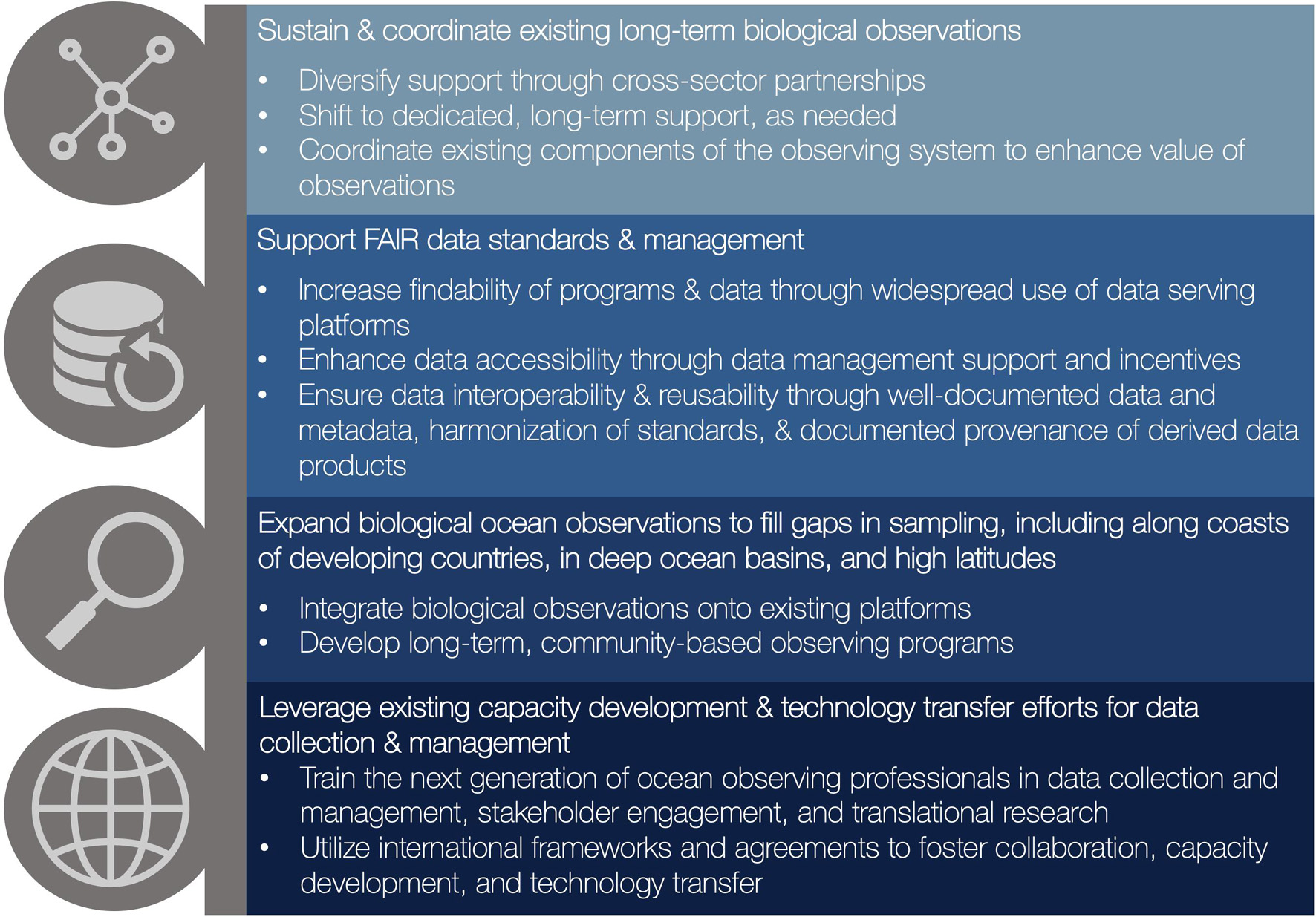
Figure 7. Possible future directions for a sustained, coordinated biological ocean observing system based on the results of this study.
Sustain and Coordinate Existing Long-Term Biological Observations
The biological EOVs identified in this study have been sampled for over 20 years, and in some cases over 70 years, and provide the foundation for a globally coordinated, sustained observing system. Additional consideration may be required for deep sea observations including deep pelagic areas (Danovaro et al., 2020). However, the sustainability of ocean observations is constrained by limited support and cross-sector cooperation (Hedge et al., 2017; Weller et al., 2019). While some programs have been sampling at regular intervals for decades despite the lack of dedicated resourcing, the current structures and policies generally discourage the maintenance of longer-term programs (Boero et al., 2015). For example, we found that nearly two-thirds of programs were supported by only one sector, indicating that there is a need to diversify sources for most programs thereby enhancing long-term security. Diversifying sources of support requires that ocean observations address scientific and societal needs to ensure their utility to a range of stakeholders (Mackenzie et al., 2019). For example, biological ocean observations can inform societal questions related to food security (e.g., fisheries and aquaculture); human health (e.g., harmful algal blooms, bacterial contamination, and medicines); coastal protection and carbon sequestration (e.g., coastal and nearshore natural habitats); as well as tourism, recreation, and well-being (e.g., marine biodiversity, culturally important species; Bax et al., 2019).
Improved coordination and connection among the existing programs within the global observing system is needed to develop a truly integrated global observing system. This improved coordination could involve networking and connecting observing programs that are sampling comparable parameters, in the same region, or within similar time periods. Integrating observing data could enable an enhanced understanding of phenomena across different scales, including across time periods (e.g., long-term climate driven changes versus short term variability), spatial regions (e.g., local versus global processes), and variables sampled (e.g., biophysical coupling). This enhanced understanding could increase the value of long-term data collections to academic, government, civil society, and industry stakeholders. For example, a sustained, coordinated observing system is necessary for use in environmental impact assessments, strategic environmental assessments, sustainability assessments, and for monitoring compliance during development activities. Many coordinating bodies already exist within the ocean observing field (MBON Duffy et al., 2013; IOOS Snowden J. et al., 2019; ILTER Muelbert et al., 2019), and have been working on large-scale efforts to coordinate biological monitoring through frameworks such as the EOVs and EBVs (Miloslavich et al., 2018; Muller-Karger et al., 2018a). However, over half of observing programs surveyed here were not part of a larger coordinating network. Thus, efforts could ensure that observing programs are aware of coordinating networks, that there are sufficient opportunities to become part of coordinating networks, and that the value and benefit of increased connectivity is clearly demonstrated and communicated.
Support FAIR Data Standards and Data Management and Convergence Toward Community Practices
In order for ocean time-series data to be effectively used, integrated, and connected, data management should follow the FAIR data principles, ensuring that data are Findable, Accessible, Interoperable, and Reusable (Wilkinson et al., 2016; Tanhua et al., 2019b).
Increase Findability of Programs and Data by Using and Further Connecting Existing Data Repositories and Data Serving Platforms
Programs
There were programs that we did not capture in our analysis, either due to (1) programs not responding to the survey, such as in cases where programs were located in countries where English was not the primary language or in cases where the contributors did not speak English (Nuñez and Amano, 2021), or (2) programs not appearing on our initial list, such as in cases where the program was not publishing data in platforms established by the international observing community (e.g., OBIS, GBIF). For example, we only included data collected in association with fisheries such as fishery independent surveys (e.g., Moriarty et al., 2019), fisheries-dependent monitoring programs, or from observers on fishing vessels (e.g., Nicol et al., 2013), when the fisheries data were included in OBIS or GBIF.
Data
Just over half of our survey respondents identified that they contribute their data to a data aggregator, repository, or other data serving platform, indicating that there are many observing datasets that are not easily findable. The repositories most connected to long-term biological observing programs, OBIS and GBIF, were also the most connected elements in the broader biodiversity informatics landscape (Bingham et al., 2017). OBIS has expanded beyond species occurrences to maintain the link between co-occurring measurements of environmental properties and biological observations as well as important sampling information (gear, area, volume, duration, and units; De Pooter et al., 2017), providing improved functionality to use abundance and biomass metrics, and OBIS has been identified as an essential component of EOV and EBV frameworks (Muller-Karger et al., 2018a).
We suggest that an effective way to increase the findability of data from smaller, individual programs online could be to provide the incentives, knowledge, training, and tools required to link to a relevant data serving platform (e.g., exemplar notebooks and workflows to align data to Darwin Core standards). Incentives may include greater visibility of programs, therefore increasing access to support, and greater ability to meet the expectations of supporters (e.g., some agencies require that data are made publicly available). In addition, we found some connectivity between data serving platforms (Figure 5; gray arrows between green data provider nodes), meaning that there is some degree of data exchange happening across data serving platforms. However, efforts could continue to move toward data sharing across platforms and data interoperability among data and standards so that the otherwise fragmented data systems can become more easily integrated, and individual observing program components become more broadly findable and thereby usable. An example of this type of interoperable data “system of systems” is the data infrastructure, SeaDataNet, which connects over a hundred data centers across Europe to enable access to otherwise distributed data, metadata, and data products (Tanhua et al., 2019b).
Enhance Data Accessibility Through Data Management Support and Incentives
Open data, and more broadly open science, accelerates and advances scientific and societal research (Reichman et al., 2011). About two-thirds of the active biological observing programs had available data, whether easily accessible or by request, which covered about 5.3% of the global surface ocean. This general lack of availability suggests that, at present, data from a fraction of the surface ocean is available for use in developing integrated global datasets that could be used to provide relevant societal indicators. Given that the remainder of observing programs had restricted access to their data in some way, and many more programs were not identified because their data were not available in common data serving platforms for marine biodiversity, further effort could provide the opportunity to move toward a culture of open data to ensure that we are maximizing the utility of data collected across all observing systems.
The main data-sharing barriers reported by observing programs were the lack of sufficient resources (e.g., funding, personnel, or knowledge), incentives or disincentives for sharing data (e.g., fear of improper use), or the ability to share data (e.g., data policies). These barriers align with previous research dating back nearly a decade (Reichman et al., 2011; Pendleton et al., 2019). Cultural and technical solutions have been developed to address data access challenges (Pendleton et al., 2019), including metrics to incentivize data sharing, such as an ocean data impact factor, or digital community currencies that could reward data providers on various aspects of the data (e.g., quantity, quality, or transparency; Pendleton et al., 2019). Technical solutions could include ledger-based technologies that uniquely identify data and associate the data with a specific author or owner to aid in tracking the data from the original source to various uses, thereby ensuring proper attribution and credit (Pendleton et al., 2019). For example, many repositories and data serving platforms provide a unique link, or “digital object identifier” (DOI), for each dataset download, so that data users can cite one DOI within which all associated datasets and their respective DOIs are also cited. As datasets continue to become more publishable, citable, and widely used, they will be further valued as an essential part of research and scholarship (Reichman et al., 2011; see Edgar and Stuart-Smith, 2014 for an example). Fast-tracking efforts to develop and propagate these attribution technologies by the global informatics community could incentivize sharing. In addition, providing data management support and data sharing platforms could facilitate an increase in the availability of long-term biodiversity observations.
Ensure Data Interoperability and Reusability Through Well-Documented Data and Metadata, Harmonized Use of Standards, Convergence of Practices, and Documented Provenance of Derived Data Products
Few biological observing programs in our study shared SOPs, standardized workflows or best practices (though see Langlois et al., 2020), which suggests that efforts could help develop tools that facilitate the comparison of data collected using different methods. Although bringing heterogeneous datasets together remains a challenge (König et al., 2019), well-documented data and metadata, harmonization of standards and vocabulary, and documented provenance of derived data products are essential to ensure data interoperability and reuse (Snowden D. et al., 2019; Tanhua et al., 2019b). For example, internationally-accepted standards and schemas, such as Darwin Core and standards from the International Organization for Standardization (ISO), provide a way to harmonize some biological EOV data (Benson et al., 2018).
In addition, new ontologies and semantic mediation tools are being developed that enable automated data harmonization and technologies are being developed to perform “crosswalks” between standards, vocabularies, and formats (Snowden D. et al., 2019; Tanhua et al., 2019b). Some international observing networks are working on these efforts by establishing and implementing standard survey approaches, data and metadata standards, co-developing data management workflows and shared vocabularies, contributing best practice publications, and developing complementary methods and resulting data formats that are feasible with varying levels of capacity (e.g., Muller-Karger et al., 2018a; Canonico et al., 2019; Pearlman et al., 2019). For example, a categorical ranking of methods for a specific parameter (e.g., optimal/good/acceptable) can be used as a tool to encourage the expansion of ocean observations in a manner that is consistent with available resources and capacity (Benway et al., 2013).
Specifically, future efforts could work toward convergence in the ocean observing community on data management and formatting standards for interoperability on molecular observations such as eDNA (for example, developing a DNA derived extension to Darwin Core that is interoperable with the MIxS standard), as well as for optics, imaging, animal tracking and biologging (see Sequeira et al., 2021), and passive and active acoustic observations of biological variables. In addition, existing and planned observing programs and data management organizations could work closely with the Ocean Best Practices System (OBPS) of the Intergovernmental Oceanographic Commission to help define, promote, and adopt standard operating practices to help organize the vast and diverse field of biological data and lead to strategies that advance interoperability (Pearlman et al., 2019).
Expand Ocean Observations to Fill Gaps in Biological Sampling, Including Along Coasts of Developing Countries, in Deep Ocean Basins, and Near the Poles
We were unable to identify active, long-term programs collecting biological observations in most of the surface ocean (∼93%) with gaps in biological observations off the coasts of parts of South America, Eastern Europe and the Caspian Sea, Asia, Oceania, Africa, the Arctic, and in the deep oceans and ABNJ. The coverage of global biological ocean observations follows a similar pattern to the global geographical coverage of marine and land-based time series identified in several studies (Titley et al., 2017; Dornelas et al., 2018). Moreover, these regions of the world’s ocean stand out in terms of considering the influence of environmental drivers in fisheries management, although efforts are still restricted to rather few species (Skern-Mauritzen et al., 2016). Maintaining biological EOVs is especially important under climate change, since stock productivity is mostly negatively affected by climate change, though examples do exist where ocean warming might improve the degree of sustainable landings (FAO, 2020). The spatial gaps in the coverage of long-term observations off the coasts of many countries highlights the challenge faced by nations in supporting sustained observations of their marine environment, despite the importance of ocean observations for food security and the general economy (Buckwell et al., 2020).
The ABNJ and the deep sea are the largest habitats on Earth. As with many remote regions, but also because the deep ocean is so vast, there are very few biodiversity and other biological observations in waters deeper than 1,000 m and the deep benthos (Webb et al., 2010; Levin et al., 2019; Danovaro et al., 2020). With biodiversity beyond national jurisdiction being an ongoing area of negotiations at the United Nations, there is an increasing need for a systematic, scientific understanding of these offshore regions. Efforts to further develop observing systems in many of the major ocean basins and seas could be beneficial. While several projects are currently underway to census current observing programs and further develop observation capacity in ABNJs, resourcing remains an impediment (Deep Ocean- Levin et al., 2019; Arctic and Antarctic- Lee et al., 2019; Smith G. C. et al., 2019; Straneo et al., 2019; Atlantic Ocean- deYoung et al., 2019; tropical Atlantic Ocean- Foltz et al., 2019; Indian Ocean- Hermes et al., 2019; North Pacific Ocean- Barth et al., 2019; tropical Pacific Ocean Smith N. et al., 2019, Yellow Sea- Kim et al., 2019; and Southern Ocean- Newman et al., 2019).
Integrate Emerging Biodiversity Sensor Technologies to Increase the Spatial Coverage of Observations
Emerging biodiversity sensor technologies (e.g., Imaging FlowCytobot, CytoBuoy, Video Plankton Recorder, passive, and active acoustic sensors) hold great promise for adding to existing observing platforms that may already be sampling in areas but that have a limited range of biological observations (Wang et al., 2019). These sensors can document biodiversity and therefore considerably augment already routinely used sensors (e.g., for chlorophyll). Sensors could be added onto existing marine and observing infrastructure such as buoys, ships, gliders, saildrones, cabled underwater observatories, undersea telecommunications cables (SMART Cables- Howe et al., 2019), opportunistic vessels via the Ships of Opportunity Program (e.g., Escobar-Flores et al., 2020), oil and gas platforms, as well as on marine animals (e.g., Fedak, 2013; Hays et al., 2018; Harcourt et al., 2019; March et al., 2020).
However, adding biodiversity sensors onto existing infrastructure for routine and long-term operation is not a trivial task due to challenges in both technological integration and the management of the resulting data sets. Data emanating from high throughput sensors are rarely usable “as is,” have complex metadata, and require time consuming and server intensive bioinformatics to make them usable (Buck et al., 2019). In addition, optical and acoustic data produce very large volumes of data which adds difficulty in data analysis and sharing. Further, different systems may use different protocols and standards to disseminate data. Therefore, at present it is still difficult to make such data at least partially comparable with existing data sets based on conventional methodologies (Buck et al., 2019). However, automated detection and classification methodologies are rapidly advancing and have been shown to be highly reliable (Marini et al., 2018).
Lastly, in situ observations of physical, biogeochemical, and biological EOVs from all platforms can be especially powerful when combined with satellite observations. Once satellite observations are validated against in situ sampling, biological satellite data products can be computed globally and shared openly (Muller-Karger et al., 2018b), providing valuable information for the underrepresented regions identified in this study. Increasing sub-surface, in situ observations [e.g., animal borne sensors, environmental metabarcoding (eDNA), imaging flow cytometry, and bio-acoustics] can greatly improve the value of satellite surface measurements (Jacox et al., 2015; Keates et al., 2020).
Long-Term Investments in Community-Based Observing Programs
Building a network that is truly global will require long-term participation from local communities and institutions (Stuart-Smith et al., 2017; Miloslavich et al., 2019; Edgar et al., 2020). Local engagement of communities in observing and monitoring, including through citizen science, community-based observing, and inclusion of indigenous, traditional, and local ecological knowledge (Berkes et al., 1994; Alessa et al., 2016), has gained momentum in recent years due to the increasing recognition of the value of observations from local observers, the awareness of the benefit of community participation in environmental management, and the conceptual shift toward environmental systems being complex, interconnected social-ecological systems (Conrad and Hilchey, 2011; Griffith et al., 2018). In addition, the development of cost-effective, user-friendly monitoring technologies, such as smartphone applications, and ways to deliver the information has facilitated more widespread community engagement in observing (Andrachuk et al., 2019). Community-based efforts may consist of collaborative partnerships between researchers, place-based observers, government officials, and community organizations (Griffith et al., 2018) and may be focused on community needs and interests (Johnson et al., 2015). Mutually beneficial efforts could deliver real value to local stakeholders and could include two-way knowledge sharing, co-development of funding proposals, project plans, educational resources, and the sharing of information (Kaiser et al., 2019).
Leverage Existing Capacity Development and Technology Transfer Efforts
Developing lasting human, institutional, and systemic capacity is the foundation of a long-term biological ocean observing system that supports a sustainable future (Bax et al., 2018). We found that around two-thirds of surveyed observing programs are engaged in some form of capacity development and technology transfer initiatives, which provides an opportunity to leverage these existing efforts to train observing personnel, especially early career professionals, on topics related to data collection and management, stakeholder engagement, and translational research. Yet, developing local capacity both in terms of personnel (i.e., technical skills) and infrastructure (i.e., computers) for ocean observing requires a multi-faceted approach with involvement from the public and private sector alike. For example, technological transfer of observation tools, such as drones, can require government approval, greater breadth of language offerings from businesses (i.e., drone companies), accessibility to scientific literature and publishing, and general data findability and accessibility (Hsu et al., 2021). Most importantly, establishing long-lasting partnerships is crucial for technology transfer, and beneficial training workshops could consider cultural, participant and instructor requirements, and local resource limitations (Miloslavich et al., 2019). While large systematic changes can greatly facilitate general technological transfer, building local capacity ultimately demands a great degree of customization to the community and observation tool in question.
In addition to capacity development and technology transfer in existing observing programs, there are international frameworks and agreements that can help to foster collaboration, capacity development, and technology transfer among observing programs in different countries, and especially between countries with varying levels of income. For example, there are provisions for capacity building and technology transfer in the United Nations Convention for the Law of the Sea (UNCLOS), as well as in other international agreements such as the UNFCCC, the CBD, in the Sustainable Development Goals, and through the Intergovernmental Oceanographic Commission. International coordinating frameworks, such as the United Nations Decade of Ocean Science for Sustainable Development (2021–2030), can also provide a catalyst for diverse engagement and collaborations (Ryabinin et al., 2019).
Conclusion
In summary, existing long-term biological observing programs could be improved with sustained support and better coordination, including through the adoption of FAIR data standards. Sustaining observing programs can be achieved by continuing to improve the relevance of ocean observations, such as through cross-sector partnerships and a shift toward longer-term support that are directed toward resource management and application. In addition, better connectivity among programs could be encouraged, including through existing coordinating networks (e.g., GOOS, MBON, and the Global Coral Reef Monitoring Network), and new networks when needed. FAIR data standards can be encouraged by providing the incentives, knowledge, training, and tools required to serve data online and to follow best practices (e.g., IOC’s OBPS; Pearlman et al., 2019), data and metadata standards, controlled vocabularies, and web enabled standards. In addition, we could continue to expand biological ocean observations to fill sampling gaps by integrating biological observing sensors onto existing platforms and by investing in partnerships with local communities through community-based observing programs. Finally, observing programs can be further sustained and enhanced by using international frameworks to improve collaboration and by leveraging existing education and training efforts that are customized for the unique needs of each region and context (Figure 7).
This study serves as a baseline which can be used to assess progress toward coordinating marine biodiversity measurements globally, such as within GOOS and MBON (Miloslavich et al., 2018); to inform sustainable development initiatives (Malone et al., 2014; Ryabinin et al., 2019); to underpin the development of national sustainable ocean economies; and for measuring progress toward global goals and targets such as the Convention on Biological Diversity’s post-2020 biodiversity framework, the United Nations Sustainable Development Goals, and a new legally-binding instrument under UNCLOS on marine biodiversity beyond national jurisdiction.
Future work could prioritize efforts to further catalog the global observing assets by focusing on specific EOVs as well as particular regions to identify additional observing programs that were not initially findable but could contribute to a globally coordinated ocean observing system. While we cannot expect to monitor the entire ocean, efforts could be made to ensure that sampling is representative of essential species and habitats across the global ocean and nations’ reporting requirements. Species EOVs include mobile species and sampling could be established to accommodate their biogeography, which is not well known for many taxa. Habitat EOVs are already included or proposed for national reporting. Effective coverage for these EOVs could be at the level that enables countries with these habitats to understand their status and trends sufficiently to manage them effectively and report on their progress.
There remains a substantial gap between the current capacity for global observations of marine resources and the potential for sustained observing programs that are comprehensive and accessible. Efforts may need to be prioritized if we are to meet the challenge of reliable and sustained observation of biological diversity, especially in biodiversity-rich developing countries where observing capacity may be lacking and marine resources may be under strong pressure. By integrating physical, biogeochemical, biological, and socio-economic variables across our global ocean, we can achieve a more comprehensive understanding of marine ecosystem processes in a rapidly changing climate and improve our capacity to conserve and sustainably use the ocean’s living heritage that supports us.
Data Availability Statement
Code and data for this manuscript are available at: https://github.com/evsatt/Final_GOOS_BioEco. Data are included in Supplementary Material.
Author Contributions
All authors supported the conceptualization, data collection/curation, and/or data analyses for this research. ESa, NB, PM, LR, GC, DD, SS, KE, and RC contributed to the design of methods, visualizations, and the initial draft. All authors contributed substantially to the writing, review, and editing of the final manuscript. PM, NB, and DD acquired funding for this research.
Funding
This work was supported by the Program for Early-stage Grants Advancing Sustainability Science (PEGASuS) 2: Ocean Sustainability Program, a partnership of Future Earth, National Center for Ecological Analysis and Synthesis (NCEAS), and Colorado State University. We are thankful to contributions by members of the U.S. Marine Biodiversity Observation Network (MBON) under the U.S. National Ocean Partnership Program (NASA, NOAA, U.S. IOOS, BOEM, and ONR) through NASA grant NNX14AP62A (“National Marine Sanctuaries as Sentinel Sites for a Demonstration Marine Biodiversity Observation Network”) and NOAA Award NA19NOS0120199 (“Implementing a Marine Biodiversity Observation Network in South Florida to Advance Ecosystem-Based Management”). We also thank contributions from the MBON Pole to Pole of the Americas program funded by NASA under the A.50 AmeriGEO Work Program of the Group on Earth Observations (Grant Number 80NSSC18K0318). This is contribution 91 from the Smithsonian Institution’s Tennenbaum Marine Observatories Network and Marine GEO program. ESa was supported by the PEGASuS 2: Ocean Sustainability Program funded in part by the Gordon and Betty Moore Foundation’s Science Program and the NOMIS Foundation. LR received support from BYONIC (ERC award number 724289). The GOOS Biology and Ecosystems Panel is funded by AIMS, CSIRO, and IOC/UNESCO and hosted by Australia’s Integrated Marine Observing System (IMOS) – IMOS is enabled by the National Collaborative Research Infrastructure Strategy (NCRIS). It is operated by a consortium of institutions as an unincorporated joint venture, with the University of Tasmania as Lead Agent. RC was supported by NOAA Sea Grant’s 2019 John A. Knauss Marine Policy Fellowship Program. AH received support from the Pew Charitable Trusts and the Baum Foundation. Partial support for FM-K was provided by NOAA and the U.S. Integrated Ocean Observing System/IOOS Program Office (award number NA19NOS0120199), NASA (awards numbers NNX14AP62A and 80NSSC20K0017), and NSF (award number 1728913). JD was supported by the Tennenbaum Marine Observatories Network and NSF award OCE-1829922. CS was supported by NSF (award #1758047) and the PEGASuS 2: Ocean Sustainability Program funded in part by the Gordon and Betty Moore Foundation’s Science Program and the NOMIS Foundation. LW and SP were supported by UNEP-WCMC’s Proteus Partnership. L-MR was supported by the CGIAR Research Program on Water, Land, and Ecosystems by Funders contributing to the CGIAR Trust Fund (https://www.cgiar.org/funders/). OK was supported by the IMR. RS-S was supported by an Australian Research Council Fellowship (FT190100599). LB-C was supported by the University of Pisa under grant PRA-2020-76. PB was supported by the Helmholtz Metadata Collaboration and the Frontiers in Arctic Marine Monitoring (FRAM) program of the Alfred Wegener Institute, Helmholtz Centre for Polar and Marine Research. RK contributed as part of the GlobalHAB Research Program funded by the Intergovernmental Oceanographic Commission (IOC) and the Scientific Committee on Oceanic Research (SCOR).
Conflict of Interest
The authors declare that the research was conducted in the absence of any commercial or financial relationships that could be construed as a potential conflict of interest.
Publisher’s Note
All claims expressed in this article are solely those of the authors and do not necessarily represent those of their affiliated organizations, or those of the publisher, the editors and the reviewers. Any product that may be evaluated in this article, or claim that may be made by its manufacturer, is not guaranteed or endorsed by the publisher.
Acknowledgments
We are grateful to the members of the biological observing community that helped us to identify biological observing programs globally and the 371 biological observing program leaders and managers who provided invaluable information by completing the survey on biological ocean observations. We would like to thank Josh Tewksbury, Heath Kelsey, Zoë Bax, and Simon Thrush for participating in the foundational conversations to develop the ideas presented in the manuscript and Ben Halpern for his guidance and support. We are grateful for the Program for Early-stage Grants Advancing Sustainability Science (PEGASuS) 2: Ocean Sustainability Program, a partnership of Future Earth, National Center for Ecological Analysis and Synthesis (NCEAS), and Colorado State University for providing the support for the working groups and meetings. In addition, we appreciate the generous contributions from the National Center for Ecological Analysis and Synthesis (NCEAS) and the IOC/UNESCO Global Ocean Observing System (GOOS) to publish this as an openly accessible manuscript. We would also like to thank Roland Viger and Robin White from USGS and two reviewers for their insightful and helpful comments to improve the manuscript.
Supplementary Material
The Supplementary Material for this article can be found online at: https://www.frontiersin.org/articles/10.3389/fmars.2021.737416/full#supplementary-material
Supplementary Material 1 | List of all programs initially contacted.
Supplementary Material 2 | PDF of survey questions used for this study.
Footnotes
- ^ https://api.obis.org/
- ^ https://bioeco-dev.obis.org/
- ^ https://dwc.tdwg.org/
- ^ gensc.org/mixs/
- ^ unidata.ucar.edu/software/netcdf/
References
Alessa, L., Kliskey, A., Gamble, J., Fidel, M., Beaujean, G., and Gosz, J. (2016). The role of Indigenous science and local knowledge in integrated observing systems: moving toward adaptive capacity indices and early warning systems. Sustainab. Sci. 11, 91–102. doi: 10.1007/s11625-015-0295-7
Andrachuk, M., Marschke, M., Hings, C., and Armitage, D. (2019). Smartphone technologies supporting community-based environmental monitoring and implementation: a systematic scoping review. Biol. Conserv. 237, 430–442. doi: 10.1016/j.biocon.2019.07.026
Appeltans, W., Dujardin, F., Flavell, M., Miloslavich, P., and Webb, T. J. (2016). Biodiversity Baselines in the GlobalOcean. Available online at: http://www.vliz.be/en/imis?module=ref&refid=284433 (accessed December 30, 2020).
Bakker, D. C. E., Pfeil, B., Olsen, A., Sabine, C. L., Metzl, N., Hankin, S., et al. (2012). Global data products help assess changes to ocean carbon sink. EOS Trans. Am. Geophys. Union 93, 125–126. doi: 10.1029/2012EO120001
Barth, J. A., Allen, S. E., Dever, E. P., Dewey, R. K., Evans, W., Feely, R. A., et al. (2019). Better regional ocean observing through cross-national cooperation: a case study from the northeast pacific. Front. Mar. Sci. 6:93. doi: 10.3389/fmars.2019.00093
Bax, N. J., Appeltans, W., Brainard, R., Duffy, J. E., Dunstan, P., Hanich, Q., et al. (2018). Linking capacity development to goos monitoring networks to achieve sustained ocean observation. Front. Mar. Sci. 5:346. doi: 10.3389/fmars.2018.00346
Bax, N. J., Miloslavich, P., Muller-Karger, F. E., Allain, V., Appeltans, W., Batten, S. D., et al. (2019). A response to scientific and societal needs for marine biological observations. Front. Mar. Sci. 6:395. doi: 10.3389/fmars.2019.00395
Benson, A., Brooks, C. M., Canonico, G., Duffy, E., Muller-Karger, F., Sosik, H. M., et al. (2018). Integrated observations and informatics improve understanding of changing marine ecosystems. Front. Mar. Sci. 5:428. doi: 10.3389/fmars.2018.00428
Benway, H. M., Lorenzoni, L., White, A. E., Fiedler, B., Levine, N. M., Nicholson, D. P., et al. (2019). Ocean time series observations of changing marine ecosystems: an era of integration, synthesis, and societal applications. Front. Mar. Sci. 6:393. doi: 10.3389/fmars.2019.00393
Benway, H. M., Telszewski, M., and Lorenzoni, L. (2013). Improving intercomparability of marine biogeochemical time series. EOS Trans. Am. Geophys. Union 94, 160–160. doi: 10.1002/2013EO170004
Berkes, F., Folke, C., and Gadgil, M. (1994). “Traditional ecological knowledge, biodiversity, resilience and sustainability,” in Biodiversity Conservation: Problems and Policies. Papers from the Biodiversity Programme Beijer International Institute of Ecological Economics Royal Swedish Academy of Sciences, eds C. A. Perrings, K.-G. Mäler, C. Folke, C. S. Holling, and B.-O. Jansson (Netherlands: Springer), 269–287. doi: 10.1007/978-94-011-1006-8_15
Bingham, H., Doudin, M., Weatherdon, L., Despot-Belmonte, K., Wetzel, F., Groom, Q., et al. (2017). The biodiversity informatics landscape: elements, connections and opportunities. Res. Ideas Outcomes 3:e14059. doi: 10.3897/rio.3.e14059
Bivand, R., and Rundel, C. (2018). rgeos: Interface to Geometry Engine – Open Source (‘GEOS’). R package version 0.3-28. Available online at: https://CRAN.R-project.org/package=rgeos (accessed September 8, 2019).
Bivand, R., Keitt, T., and Rowlingson, B. (2019). rgdal: Bindings for the ‘Geospatial’ Data Abstraction Library. R package version 1.4-6. Available online at: https://CRAN.R-project.org/package=rgdal (accessed October 14, 2019).
Boero, F., Kraberg, A. C., Krause, G., and Wiltshire, K. H. (2015). Time is an affliction: why ecology cannot be as predictive as physics and why it needs time series. J. Sea Res. 101, 12–18. doi: 10.1016/j.seares.2014.07.008
Buck, J. J. H., Bainbridge, S. J., Burger, E. F., Kraberg, A. C., Casari, M., Casey, K. S., et al. (2019). Ocean data product integration through innovation-the next level of data interoperability. Front. Mar. Sci. 6:32. doi: 10.3389/fmars.2019.00032
Buckwell, A. J., Fleming, C., Smart, J. C. R., Ware, D., and Mackey, B. (2020). Challenges and sensitivities in assessing total ecosystem service values: lessons from vanuatu for the pacific. J. Environ. Dev. 29, 329–365. doi: 10.1177/1070496520937033
Buttigieg, P. L., Fadeev, E., Bienhold, C., Hehemann, L., Offre, P., and Boetius, A. (2018). Marine microbes in 4D — using time series observation to assess the dynamics of the ocean microbiome and its links to ocean health. Curr. Opin. Microbiol. 43, 169–185. doi: 10.1016/j.mib.2018.01.015
CalCOFI Methods (2021). Available online at: https://calcofi.org/ccpublications/calcofi-methods.html (accessed October 5, 2021).
Canonico, G., Buttigieg, P. L., Montes, E., Muller-Karger, F. E., Stepien, C., Wright, D., et al. (2019). Global observational needs and resources for marine biodiversity. Front. Mar. Sci. 6:367. doi: 10.3389/fmars.2019.00367
Chamberlain, S., and Ooms, J. (2019). geojson: Classes for ‘GeoJSON’. R package version 0.3.2. Available online at: https://CRAN.R-project.org/package=geojson (accessed October 20, 2019).
Chamberlain, S., and Teucher, A. (2019). geojsonlint: Tools for Validating ‘GeoJSON’. R package versionn 0.3.0. Available online at: https://CRAN.R-project.org/package=geojsonlint (accessed October 15, 2019).
Conrad, C. C., and Hilchey, K. G. (2011). A review of citizen science and community-based environmental monitoring: issues and opportunities. Environ. Monit. Assess. 176, 273–291. doi: 10.1007/s10661-010-1582-5
Cooley, D. (2019). geojsonsf: GeoJSON to Simple Feature Converter. R package version 1.3.0. Available online at: https://CRAN.R-project.org/package=geojsonsf (accessed October 10, 2019).
Costello, M. J., Coll, M., Danovaro, R., Halpin, P., Ojaveer, H., and Miloslavich, P. (2010). A census of marine biodiversity knowledge, resources, and future challenges. PLoS One 5:e12110. doi: 10.1371/journal.pone.0012110
Cruz-Motta, J. J., Miloslavich, P., Guerra-Castro, E., Hernández-Agreda, A., Herrera, C., Barros, F., et al. (2020). Latitudinal patterns of species diversity on South American rocky shores: local processes lead to contrasting trends in regional and local species diversity. J. Biogeogr. 47, 1966–1979. doi: 10.1111/jbi.13869
Csardi, G., and Nepusz, T. (2006). The Igraph Software Package for Complex Network Research. InterJournal Complex Syst. 1695, 1–9.
Danovaro, R., Fanelli, E., Aguzzi, J., Billett, D., Carugati, L., Corinaldesi, C., et al. (2020). Ecological variables for developing a global deep-ocean monitoring and conservation strategy. Nat. Ecol. Evol. 4, 181–192. doi: 10.1038/s41559-019-1091-z
Darwin core (n.d.). Available online at: https://dwc.tdwg.org/ (accessed March 2, 2021).
De Pooter, D., Appeltans, W., Bailly, N., Bristol, S., Deneudt, K., Eliezer, M., et al. (2017). Toward a new data standard for combined marine biological and environmental datasets—Expanding OBIS beyond species occurrences. Biodiversity Data Journal 5:e10989. doi: 10.3897/BDJ.5.e10989
deYoung, B., Visbeck, M., de Araujo Filho, M. C., Baringer, M. O., Black, C., Buch, E., et al. (2019). An integrated all-atlantic ocean observing system in 2030. Front. Mar. Sci. 6:428. doi: 10.3389/fmars.2019.00428
Dornelas, M., Antão, L. H., Moyes, F., Bates, A. E., Magurran, A. E., Adam, D., et al. (2018). BioTIME: a database of biodiversity time series for the Anthropocene. Global Ecol. Biogeogr. 27, 760–786. doi: 10.1111/geb.12729
Duffy, J. E., Amaral-Zettler, L. A., Fautin, D. G., Paulay, G., Rynearson, T. A., Sosik, H. M., et al. (2013). Envisioning a marine biodiversity observation network. BioScience 63, 350–361. doi: 10.1525/bio.2013.63.5.8
Duffy, J. E., Benedetti-Cecchi, L., Trinanes, J., Muller-Karger, F. E., Ambo-Rappe, R., Boström, C., et al. (2019). Toward a coordinated global observing system for seagrasses and marine macroalgae. Front. Mar. Sci. 6:317. doi: 10.3389/fmars.2019.00317
Edgar, G. J., Alexander, T. J., Lefcheck, J. S., Bates, A. E., Kininmonth, S. J., Thomson, R. J., et al. (2017). Abundance and local-scale processes contribute to multi-phyla gradients in global marine diversity. Sci. Adv. 3:e1700419. doi: 10.1126/sciadv.1700419
Edgar, G. J., and Stuart-Smith, R. D. (2014). Systematic global assessment of reef fish communities by the Reef Life Survey program. Sci. Data 1:140007. doi: 10.1038/sdata.2014.7
Edgar, G. J., Cooper, A., Baker, S. C., Barker, W., Barrett, N. S., Becerro, M. A., et al. (2020). Establishing the ecological basis for conservation of shallow marine life using Reef Life Survey. Biol. Conserv. 252:108855. doi: 10.1016/j.biocon.2020.108855
Escobar-Flores, P. C., O’Driscoll, R. L., Montgomery, J. C., Ladroit, Y., and Jendersie, S. (2020). Estimates of density of mesopelagic fish in the Southern Ocean derived from bulk acoustic data collected by ships of opportunity. Polar Biol. 43, 43–61. doi: 10.1007/s00300-019-02611-3
Estes, M., Anderson, C., Appeltans, W., Bax, N., Bednaršek, N., Canonico, G., et al. (2021). Enhanced monitoring of life in the sea is a critical component of conservation management and sustainable economic growth. Mar. Policy 132:104699. doi: 10.1016/j.marpol.2021.104699
Fedak, M. A. (2013). The impact of animal platforms on polar ocean observation. Deep Sea Res. Part II Topic. Stud. Oceanogr. 88–89, 7–13. doi: 10.1016/j.dsr2.2012.07.007
Flanders Marine Institute (VLIZ) (2019). Maritime Boundaries Geodatabase: Maritime Boundaries and Exclusive Economic Zones(200nm) [Data set]. Ostend: VLIZ. doi: 10.14284/386
Foltz, G. R., Brandt, P., Richter, I., Rodríguez-Fonseca, B., Hernandez, F., Dengler, M., et al. (2019). The tropical atlantic observing system. Front. Mar. Sci. 6:206. doi: 10.3389/fmars.2019.00206
GEBCO compilation group (2020). Gridded Bathymetry Data (General Bathymetric Chart of the Oceans). (n.d.). GEBCO. Available online at: https://www.gebco.net/data_and_products/gridded_bathymetry_data/ (accessed March 2, 2021).
Golden, J. S., Virdin, J., Nowacek, D., Halpin, P., Bennear, L., and Patil, P. G. (2017). Making sure the blue economy is green. Nat. Ecol. Evol. 1, 1–3. doi: 10.1038/s41559-016-0017
Greenberg, J. A., and Mattiuzzi, M. (2018). gdalUtils: Wrappers for the Geospatial Data Abstraction Library (GDAL) Utilities. R package version 2.0.1.14. Available online at: https://CRAN.R-project.org/package=gdalUtils (accessed December 8, 2019).
Griffith, D. L., Alessa, L., and Kliskey, A. (2018). Community-based observing for social–ecological science: lessons from the Arctic. Front. Ecol. Environ. 16:S44–S51. doi: 10.1002/fee.1798
Harcourt, R., Sequeira, A. M. M., Zhang, X., Roquet, F., Komatsu, K., Heupel, M., et al. (2019). Animal-borne telemetry: an integral component of the ocean observing toolkit. Front. Mar. Sci. 6:326. doi: 10.3389/fmars.2019.00326
Hays, G. C., Alcoverro, T., Christianen, M. J. A., Duarte, C. M., Hamann, M., Macreadie, P. I., et al. (2018). New tools to identify the location of seagrass meadows: marine grazers as habitat indicators. Front. Mar. Sci. 5:9. doi: 10.3389/fmars.2018.00009
Hedge, P., Molloy, F., Sweatman, H., Hayes, K. R., Dambacher, J. M., Chandler, J., et al. (2017). An integrated monitoring framework for the great barrier reef world heritage area. Mar. Policy 77, 90–96. doi: 10.1016/j.marpol.2016.12.014
Hermes, J. C., Masumoto, Y., Beal, L. M., Roxy, M. K., Vialard, J., Andres, M., et al. (2019). A sustained ocean observing system in the Indian ocean for climate related scientific knowledge and societal needs. Front. Mar. Sci. 6:355. doi: 10.3389/fmars.2019.00355
Heymans, J. J., Bundy, A., Christensen, V., Coll, M., de Mutsert, K., Fulton, E. A., et al. (2020). The ocean decade: a true ecosystem modeling challenge. Front. Mar. Sci. 7:554573. doi: 10.3389/fmars.2020.554573
Hijmans, R. J. (2017). raster: Geographic Data Analysis and Modeling. R package version 2.6-7. Available online at: https://CRAN.R-project.org/package=raster (accessed September 21, 2019).
Hiscock, K. (1990). Marine Nature Conservation Review: Methods. Joint Nature Conservation Committee, Peterborough, Nature Conservancy Council, CSD Report, No. 1072. (Marine Nature Conservation Review Report, No. MNCR/OR/5). Peterborough: Joint Nature Conservation Committee.
Howe, B. M., Arbic, B. K., Aucan, J., Barnes, C. R., Bayliff, N., Becker, N., et al. (2019). Smart cables for observing the global ocean: science and implementation. Front. Mar. Sci. 6:424. doi: 10.3389/fmars.2019.00424
Hsu, A. J., Pruckner, S., Satterthwaite, E. V., Weatherdon, L. V., Hadley, K., and Nguyen, E. T. T. (2021). Challenges and recommendations for equitable use of aerial tools for mangrove research. Front. Mar. Sci. 8:277. doi: 10.3389/fmars.2021.643784
Jacox, M. G., Edwards, C. A., Kahru, M., Rudnick, D. L., and Kudela, R. M. (2015). The potential for improving remote primary productivity estimates through subsurface chlorophyll and irradiance measurement. Deep Sea Res. Part II Top. Stud. Oceanogr. 112, 107–116. doi: 10.1016/j.dsr2.2013.12.008
Johnson, N., Alessa, L., Behe, C., Danielsen, F., Gearheard, S., Gofman-Wallingford, V., et al. (2015). The contributions of community-based monitoring and traditional knowledge to arctic observing networks: reflections on the state of the field. Arctic 68, 28–40.
Jones, M. B., O’Brien, M., Mecum, B., Boettiger, C., Schildhauer, M., Maier, M., et al. (2019). Ecological Metadata Language version 2.2.0. KNB Data Repository. doi: 10.5063/F11834T2
Kaiser, B. A., Hoeberechts, M., Maxwell, K. H., Eerkes-Medrano, L., Hilmi, N., Safa, A., et al. (2019). The importance of connected ocean monitoring knowledge systems and communities. Front. Mar. Sci. 6:309. doi: 10.3389/fmars.2019.00309
Keates, T. R., Kudela, R. M., Holser, R. R., Hückstädt, L. A., Simmons, S. E., and Costa, D. P. (2020). Chlorophyll fluorescence as measured in situ by animal-borne instruments in the northeastern Pacific Ocean. J. Mar. Syst. 203:103265. doi: 10.1016/j.jmarsys.2019.103265
Keith, D., Ferrer, J. R., Nicholson, E., Bishop, M. J., Polidoro, B., Ramírez-Llodra, E., et al. (2020). The IUCN Global Ecosystem Typology v1.01: Descriptive profiles for Biomes and Ecosystem Functional Groups. Gland: IUCN
Kim, Y. S., Jang, C. J., Noh, J. H., Kim, K.-T., Kwon, J.-I., Min, Y., et al. (2019). A yellow sea monitoring platform and its scientific applications. Front. Mar. Sci. 6:601. doi: 10.3389/fmars.2019.00601
König, C., Weigelt, P., Schrader, J., Taylor, A., Kattge, J., and Kreft, H. (2019). Biodiversity data integration—The significance of data resolution and domain. PLoS Biol. 17:e3000183. doi: 10.1371/journal.pbio.3000183
Koslow, J. A., and Couture, J. (2015). Pacific Ocean observation programs: gaps in ecological time series. Mar. Policy 51, 408–414. doi: 10.1016/j.marpol.2014.09.003
Langlois, T., Goetze, J., Bond, T., Monk, J., Abesamis, R. A., Asher, J., et al. (2020). A field and video annotation guide for baited remote underwater stereo-video surveys of demersal fish assemblages. Methods Ecol. Evol. 11, 1401–1409. doi: 10.1111/2041-210X.13470
Lee, C. M., Starkweather, S., Eicken, H., Timmermans, M.-L., Wilkinson, J., Sandven, S., et al. (2019). A framework for the development, design and implementation of a sustained Arctic Ocean observing system. Front. Mar. Sci. 6:451. doi: 10.3389/fmars.2019.00451
Levin, L. A., Bett, B. J., Gates, A. R., Heimbach, P., Howe, B. M., Janssen, F., et al. (2019). Global observing needs in the deep ocean. Front. Mar. Sci. 6:241. doi: 10.3389/fmars.2019.00241
Lindstrom, E. J., Gunn, A., Fischer, A., McCurdy, L. K., and Glover, and the Task Team for an Integrated Framework for Sustained Ocean Observing (2012). Framework for Ocean Observing. Paris: UNESCO, doi: 10.5270/OceanObs09-FOO.
Mackenzie, B., Celliers, L., Assad, L. P., de, F., Heymans, J. J., Rome, N., et al. (2019). The role of stakeholders in creating societal value from coastal and ocean observations. Front. Mar. Sci. 6:137. doi: 10.3389/fmars.2019.00137
Malone, T. C., DiGiacomo, P. M., Gonçalves, E., Knap, A. H., Talaue-McManus, L., and de Mora, S. (2014). A global ocean observing system framework for sustainable development. Mar. Policy 43, 262–272. doi: 10.1016/j.marpol.2013.06.008
March, D., Boehme, L., Tintoré, J., Vélez-Belchi, P. J., and Godley, B. J. (2020). Towards the integration of animal-borne instruments into global ocean observing systems. Global Change Biol. 26, 586–596. doi: 10.1111/gcb.14902
Marini, S., Fanelli, E., Sbragaglia, V., Azzurro, E., Del Rio Fernandez, J., and Aguzzi, J. (2018). Tracking fish abundance by underwater image recognition. Sci. Rep. 8:13748. doi: 10.1038/s41598-018-32089-8
McCauley, D. J., Pinsky, M. L., Palumbi, S. R., Estes, J. A., Joyce, F. H., and Warner, R. R. (2015). Marine defaunation: animal loss in the global ocean. Science 347:1255641. doi: 10.1126/science.1255641
Miloslavich, P., Klein, E., Díaz, J. M., Hernández, C. E., Bigatti, G., Campos, L., et al. (2011). Marine biodiversity in the Atlantic and Pacific coasts of South America: knowledge and gaps. PLoS One 6:e14631. doi: 10.1371/journal.pone.0014631
Miloslavich, P., Bax, N. J., Simmons, S. E., Klein, E., Appeltans, W., Aburto-Oropeza, O., et al. (2018). Essential ocean variables for global sustained observations of biodiversity and ecosystem changes. Global Change Biol. 24, 2416–2433. doi: 10.1111/gcb.14108
Miloslavich, P., Seeyave, S., Muller-Karger, F., Bax, N., Ali, E., Delgado, C., et al. (2019). Challenges for global ocean observation: the need for increased human capacity. J. Oper. Oceanogr. 12(suppl. 2), S137–S156. doi: 10.1080/1755876X.2018.1526463
Mitchell, M. R., Harrison, G., Pauley, K., Gagné, A., Maillet, G., and Strain, P. (2002). Atlantic zonal monitoring program sampling protocol. Can. Tech. Rep. Hydrogr. Ocean Sci. 223, iv + 23.
Mora, C., Tittensor, D. P., and Myers, R. A. (2008). The completeness of taxonomic inventories for describing the global diversity and distribution of marine fishes. Proc. R. Soc. B Biol. Sci. 275, 149–155. doi: 10.1098/rspb.2007.1315
Moriarty, M., Greenstreet, S. P. R., Rasmussen, J., and de Boois, I. (2019). Assessing the state of demersal fish to address formal ecosystem based management needs: making fisheries independent trawl survey data ‘fit for purpose.’. Front. Mar. Sci. 6:162. doi: 10.3389/fmars.2019.00162
Muelbert, J. H., Nidzieko, N. J., Acosta, A. T. R., Beaulieu, S. E., Bernardino, A. F., Boikova, E., et al. (2019). Ilter – the international long-term ecological research network as a platform for global coastal and ocean observation. Front. Mar. Sci. 6:527. doi: 10.3389/fmars.2019.00527
Muller-Karger, F. E., Hestir, E., Ade, C., Turpie, K., Roberts, D. A., Siegel, D., et al. (2018a). Satellite sensor requirements for monitoring essential biodiversity variables of coastal ecosystems. Ecol. Appl. 28, 749–760. doi: 10.1002/eap.1682
Muller-Karger, F. E., Miloslavich, P., Bax, N. J., Simmons, S., Costello, M. J., Sousa Pinto, I., et al. (2018b). Advancing marine biological observations and data requirements of the complementary essential ocean variables (Eovs) and essential biodiversity variables (Ebvs) frameworks. Front. Mar. Sci. 5:211. doi: 10.3389/fmars.2018.00211
Newman, L., Heil, P., Trebilco, R., Katsumata, K., Constable, A., van Wijk, E., et al. (2019). Delivering sustained, coordinated, and integrated observations of the southern ocean for global impact. Front. Mar. Sci. 6:433. doi: 10.3389/fmars.2019.00433
Nicol, S. J., Allain, V., Pilling, G. M., Polovina, J., Coll, M., Bell, J., et al. (2013). An ocean observation system for monitoring the affects of climate change on the ecology and sustainability of pelagic fisheries in the Pacific Ocean. Clim. Change 119, 131–145. doi: 10.1007/s10584-012-0598-y
Nuñez, M. A., and Amano, T. (2021). Monolingual searches can limit and bias results in global literature reviews. Nat. Ecol. Evol. 5, 264–264. doi: 10.1038/s41559-020-01369-w
O’Brien, T. D., Lorenzoni, L., Isensee, K., and Valdés, L.(Eds) (2017). What are Marine Ecological Time Series Telling us About the Ocean? A Status Report. iOC-UNESCO, IOC TEchnical Series, No. 129. 297. Paris: IOC-UNESCO.
OBIS (2021). Ocean Biodiversity Information System. Intergovernmental Oceanographic Commission of UNESCO. Available online at: www.obis.org (accessed July 5, 2021).
OECD (2016). The Ocean Economy in 2030. Available online at: https://www.oecd.org/environment/the-ocean-economy-in-2030-9789264251724-en.html (accessed March 2, 2021).
OGC (2021). OGC Standards and Resources. Available online at: https://www.ogc.org/standards (accessed March 2, 2021).
Pearlman, J., Bushnell, M., Coppola, L., Karstensen, J., Buttigieg, P. L., Pearlman, F., et al. (2019). Evolving and sustaining ocean best practices and standards for the next decade. Front. Mar. Sci. 6:277. doi: 10.3389/fmars.2019.00277
Pebesma, E. (2018). Simple features for R: standardized support for spatial vector data. R J. 10, 439–446. doi: 10.32614/RJ-2018-009
Pebesma, E. (2020). lwgeom: Bindings to Selected ‘liblwgeom’ Functions for Simple Features. R package version 0.2-1. Available online at: https://CRAN.R-project.org/package=lwgeom (accessed March 25, 2020).
Pendleton, L. H., Beyer, H., Estradivari, Grose, S. O., Hoegh-Guldberg, O., Karcher, D. B., et al. (2019). Disrupting data sharing for a healthier ocean. ICES J. Mar. Sci. 76, 1415–1423. doi: 10.1093/icesjms/fsz068
Pereira, H. M., Ferrier, S., Walters, M., Geller, G. N., Jongman, R. H. G., Scholes, R. J., et al. (2013). Essential biodiversity variables. Science 339, 277–278. doi: 10.1126/science.1229931
Poisot, T. (2011). The digitize package: extracting numerical data from scatterplots. R J. 3, 25–26.
R Core Team (2018). R: A Language and Environment for Statistical Computing. Vienna: R Foundation for Statistical Computing.
Rayner, R., Jolly, C., and Gouldman, C. (2019). Ocean observing and the blue economy. Front. Mar. Sci. 6:330. doi: 10.3389/fmars.2019.00330
Reichman, O. J., Jones, M. B., and Schildhauer, M. P. (2011). Challenges and opportunities of open data in ecology. Science 331, 703–705. doi: 10.1126/science.1197962
Richardson, A. J., Walne, A. W., John, A. W. G., Jonas, T. D., Lindley, J. A., Sims, D. W., et al. (2006). Using continuous plankton recorder data. Prog. Oceanogr. 68, 27–74. doi: 10.1016/j.pocean.2005.09.011
Ross, N. (2020). fasterize: Fast Polygon to Raster Conversion. R package version 1.0.2. Available online at: https://CRAN.R-project.org/package=fasterize (accessed May 1, 2020).
Ryabinin, V., Barbière, J., Haugan, P., Kullenberg, G., Smith, N., McLean, C., et al. (2019). The UN decade of ocean science for sustainable development. Front. Mar. Sci. 6:470. doi: 10.3389/fmars.2019.00470
Satterthwaite, E., and Waller, J. (2020). GBIF Custom Download of All Ocean Data From: Establishing the Foundation for the Global Observing System for Marine Life [Data set]. Geneva, Switzerland: Zenodo. doi: 10.35000/tbxp-kt36
Sequeira, A. M. M., O’Toole, M., Keates, T. R., McDonnell, L. H., Braun, C. D., Hoenner, X., et al. (2021). A standardisation framework for bio-logging data to advance ecological research and conservation. Methods Ecol. Evol. 12, 996-1007. doi: 10.1111/2041-210X.13593
Skern-Mauritzen, M., Ottersen, G., Handegard, N. O., Huse, G., Dingsør, G. E., Stenseth, N. C., et al. (2016). Ecosystem processes are rarely included in tactical fisheries management. Fish Fish. 17, 165–175. doi: 10.1111/faf.12111
Sloyan, B. M., Wilkin, J., Hill, K. L., Chidichimo, M. P., Cronin, M. F., Johannessen, J. A., et al. (2019). Evolving the physical global ocean observing system for research and application services through international coordination. Front. Mar. Sci. 6:449. doi: 10.3389/fmars.2019.00449
Smith, G. C., Allard, R., Babin, M., Bertino, L., Chevallier, M., Corlett, G., et al. (2019). Polar ocean observations: a critical gap in the observing system and its effect on environmental predictions from hours to a season. Front. Mar. Sci. 6:429. doi: 10.3389/fmars.2019.00429
Smith, N., Kessler, W. S., Cravatte, S., Sprintall, J., Wijffels, S., Cronin, M. F., et al. (2019). Tropical pacific observing system. Front. Mar. Sci. 6:31. doi: 10.3389/fmars.2019.00031
Snowden, D., Tsontos, V. M., Handegard, N. O., Zarate, M., O’ Brien, K., Casey, K. S., et al. (2019). Data interoperability between elements of the global ocean observing system. Front. Mar. Sci. 6:442. doi: 10.3389/fmars.2019.00442
Snowden, J., Hernandez, D., Quintrell, J., Harper, A., Morrison, R., Morell, J., et al. (2019). The U. S. Integrated ocean observing system: governance milestones and lessons from two decades of growth. Front. Mar. Sci. 6:242. doi: 10.3389/fmars.2019.00242
Straneo, F., Sutherland, D. A., Stearns, L., Catania, G., Heimbach, P., Moon, T., et al. (2019). The case for a sustained greenland ice sheet-ocean observing system(Grioos). Front. Mar. Sci. 6:138. doi: 10.3389/fmars.2019.00138
Stuart-Smith, R. D., Edgarm, G. J., Barrett, N. S., Bates, A. E., Baker, S. C., Bax, N. J., et al. (2017). Assessing national biodiversity trends for rocky and coral reefs through the integration of citizen science and scientific monitoring programs. Bioscience 67, 134–146. doi: 10.1093/biosci/biw180
Tanhua, T., McCurdy, A., Fischer, A., Appeltans, W., Bax, N., Currie, K., et al. (2019a). What we have learned from the framework for ocean observing: evolution of the global ocean observing system. Front. Mar. Sci. 6:471. doi: 10.3389/fmars.2019.00471
Tanhua, T., Pouliquen, S., Hausman, J., O’Brien, K., Bricher, P., de Bruin, T., et al. (2019b). Ocean fair data services. Front. Mar. Sci. 6:440. doi: 10.3389/fmars.2019.00440
Tilbrook, B., Jewett, E. B., DeGrandpre, M. D., Hernandez-Ayon, J. M., Feely, R. A., Gledhill, D. K., et al. (2019). An enhanced ocean acidification observing network: from people to technology to data synthesis and information exchange. Front. Mar. Sci. 6:337. doi: 10.3389/fmars.2019.00337
Tintoré, J., Pinardi, N., Álvarez-Fanjul, E., Aguiar, E., Álvarez-Berastegui, D., Bajo, M., et al. (2019). Challenges for sustained observing and forecasting systems in the Mediterranean sea. Front. Mar. Sci. 6:568. doi: 10.3389/fmars.2019.00568
Titley, M. A., Snaddon, J. L., and Turner, E. C. (2017). Scientific research on animal biodiversity is systematically biased towards vertebrates and temperate regions. PLoS One 12:e0189577. doi: 10.1371/journal.pone.0189577
Wang, Z. A., Moustahfid, H., Mueller, A. V., Michel, A. P. M., Mowlem, M., Glazer, B. T., et al. (2019). Advancing observation of ocean biogeochemistry, biology, and ecosystems with cost-effective in situ sensing technologies. Front. Mar. Sci. 6:519. doi: 10.3389/fmars.2019.00519
Webb, T. J., Berghe, E. V., and O’Dor, R. (2010). Biodiversity’s big wet secret: the global distribution of marine biological records reveals chronic under-exploration of the deep pelagic ocean. PLoS One 5:e10223. doi: 10.1371/journal.pone.0010223
Weller, R. A., Baker, D. J., Glackin, M. M., Roberts, S. J., Schmitt, R. W., Twigg, E. S., et al. (2019). The challenge of sustaining ocean observations. Front. Mar. Sci. 6:105. doi: 10.3389/fmars.2019.00105
Wilkinson, M. D., Dumontier, M., Aalbersberg, I. J., Appleton, G., Axton, M., Baak, A., et al. (2016). The FAIR Guiding Principles for scientific data management and stewardship. Sci. Data 3:160018. doi: 10.1038/sdata.2016.18
Winther, J.-G., Dai, M., Rist, T., Hoel, A. H., Li, Y., Trice, A., et al. (2020). Integrated ocean management for a sustainable ocean economy. Nat. Ecol. Evol. 4, 1451–1458. doi: 10.1038/s41559-020-1259-6
WoRMS Editorial Board (2021). World Register of Marine Species. doi: 10.14284/170 Available online at: http://www.marinespecies.org (accessed August 1, 2020).
Keywords: biodiversity, global coordination, long term observations, time series, environmental monitoring, sustainability, essential ocean variables, climate change
Citation: Satterthwaite EV, Bax NJ, Miloslavich P, Ratnarajah L, Canonico G, Dunn D, Simmons SE, Carini RJ, Evans K, Allain V, Appeltans W, Batten S, Benedetti-Cecchi L, Bernard ATF, Bristol S, Benson A, Buttigieg PL, Gerhardinger LC, Chiba S, Davies TE, Duffy JE, Giron-Nava A, Hsu AJ, Kraberg AC, Kudela RM, Lear D, Montes E, Muller-Karger FE, O’Brien TD, Obura D, Provoost P, Pruckner S, Rebelo L-M, Selig ER, Kjesbu OS, Starger C, Stuart-Smith RD, Vierros M, Waller J, Weatherdon LV, Wellman TP and Zivian A (2021) Establishing the Foundation for the Global Observing System for Marine Life. Front. Mar. Sci. 8:737416. doi: 10.3389/fmars.2021.737416
Received: 06 July 2021; Accepted: 24 September 2021;
Published: 25 October 2021.
Edited by:
Laura Lorenzoni, National Aeronautics and Space Administration (NASA), United StatesReviewed by:
Michael William Lomas, Bigelow Laboratory for Ocean Sciences, United StatesCarrie Wall, University of Colorado, Boulder, United States
Copyright © 2021 Satterthwaite, Bax, Miloslavich, Ratnarajah, Canonico, Dunn, Simmons, Carini, Evans, Allain, Appeltans, Batten, Benedetti-Cecchi, Bernard, Bristol, Benson, Buttigieg, Gerhardinger, Chiba, Davies, Duffy, Giron-Nava, Hsu, Kraberg, Kudela, Lear, Montes, Muller-Karger, O’Brien, Obura, Provoost, Pruckner, Rebelo, Selig, Kjesbu, Starger, Stuart-Smith, Vierros, Waller, Weatherdon, Wellman and Zivian. This is an open-access article distributed under the terms of the Creative Commons Attribution License (CC BY). The use, distribution or reproduction in other forums is permitted, provided the original author(s) and the copyright owner(s) are credited and that the original publication in this journal is cited, in accordance with accepted academic practice. No use, distribution or reproduction is permitted which does not comply with these terms.
*Correspondence: Erin V. Satterthwaite, esatterthwaite@ucsd.edu