- 1Griffith Climate Change Response Program, Griffith University, Gold Coast, QLD, Australia
- 2Whales & Climate Research Program, Griffith University, Gold Coast, QLD, Australia
- 3Coastal and Marine Research Centre, Griffith University, Gold Coast, QLD, Australia
- 4Cities Research Institute, Griffith University, Gold Coast, QLD, Australia
- 5Department of Earth Sciences, Stellenbosch University, Stellenbosch, South Africa
- 6Centre for Sustainable Oceans, Faculty of Applied Sciences, Cape Peninsula University of Technology, Cape Town, South Africa
- 7Marine and Antarctic Research Centre for Innovation and Sustainability (MARIS), University of Cape Town, Cape Town, South Africa
- 8Department of Oceanography, University of Cape Town, Cape Town, South Africa
Humpback whales, Megaptera novaeangliae, are a highly migratory species exposed to a wide range of environmental factors during their lifetime. The spatial and temporal characteristics of such factors play a significant role in determining suitable habitats for breeding, feeding and resting. The existing studies of the relationship between oceanic conditions and humpback whale ecology provide the basis for understanding impacts on this species. Here we have determined the most relevant environmental drivers identified in peer-reviewed literature published over the last four decades, and assessed the methods used to identify relationships. A total of 148 studies were extracted through an online literature search. These studies used a combined estimated 105,000 humpback whale observations over 1,216 accumulated study years investigating the relationship between humpback whales and environmental drivers in both Northern and Southern Hemispheres. Studies focusing on humpback whales in feeding areas found preferences for areas of upwelling, high chlorophyll-a concentration and frontal areas with changes in temperature, depth and currents, where prey can be found in high concentration. Preferred calving grounds were identified as shallow, warm and with slow water movement to aid the survival of calves. The few studies of migration routes have found preferences for shallow waters close to shorelines with moderate temperature and chlorophyll-a concentration. Extracting information and understanding the influence of key drivers of humpback whale behavioral modes are important for conservation, particularly in regard to expected changes of environmental conditions under climate change.
Introduction
Humpback whales (Megaptera novaeangliae, hereafter referred to as HWs) conduct one of the longest annual migrations of any mammal (Stone Florez-Gonzalez and Katona, 1990; Stevick et al., 2011). HWs are found in all oceans, migrating between high latitude feeding grounds in summer and tropical and sub-tropical breeding grounds in winter (Dawbin, 1966; Clapham and Mead, 1999). Many HW populations are generally showing marked increases to protection from last century’s severe whaling pressure (Chittleborough, 1965; Findlay, 2001; Noad et al., 2019). Northern and Southern Hemisphere HW populations are generally distinct, but some genetic and photographic evidence suggest a small level of interchange (Baker et al., 1990; Stone Florez-Gonzalez and Katona, 1990; Garrigue et al., 2007; Rasmussen et al., 2007). Currently, at least 16 HW populations are recognized worldwide including some sub-populations (Jackson et al., 2015), with whales utilizing coastal and open ocean habitats in both hemispheres. In the Southern Hemisphere, the species is distributed in seven breeding populations including sub-populations, from A to G (IWC, 1998, 2011).
A wide range of environmental conditions are encountered in these habitats, and HWs are able to identify and adapt to preferred conditions for their most important life stages or behavioral ‘modes’ herein referred to as breeding, feeding, migrating, and resting. Given the different habitats used and their mobility, migratory species have spatially broad ecological niches (Guisan and Zimmermann, 2000). Underlying reasons behind HW habitat selection are still debated (Corkeron and Connor, 1999). Different habitats may be favored depending on whether migration occurs to optimize energy budgets and thermoregulation (Brodie, 1975), calf growth and survivorship (Clapham, 1996), protection from predation (Naessig and Lanyon, 2004; Steiger, 2008) or feeding (Andrews-Goff et al., 2018).
Large-scale spatial patterns are well documented for migration (Dawbin, 1966; Garrigue et al., 2000), feeding (Owen et al., 2017) and breeding grounds (Smith et al., 2012); however, smaller-scale patterns of habitat use, cues that induce a switch between behavioral modes and the factors influencing the choice for each of these areas, are not as well understood (Franklin, 2014; Valani et al., 2020). Some studies suggest little or no influence of changing oceanographic conditions (currents, temperature, and salinity) on large scale migrations (Horton et al., 2020), while others suggest that HWs shift the timing of migration in response to oceanographic conditions (Ramp et al., 2015; Avila et al., 2019; Kershaw et al., 2021).
Some of the drivers that have been identified to influence HW habitat selection include bathymetry (Dalla Rosa et al., 2012), sea surface temperature (SST) (Dransfield et al., 2014), distance to coast/offshore (Burrows et al., 2012), hydrodynamics (Tynan et al., 2005), variations in chlorophyll-a concentration as a proxy for prey (Fiedler et al., 1998), salinity (Gregr and Trites, 2001), tides (Chenoweth et al., 2011), and the extent of the sea ice (Bombosch et al., 2014; Andrews-Goff et al., 2018). Large scale climate phenomena such the El Niño-Southern Oscillation (ENSO) or Southern Annular Mode (SAM) were used in some studies as a proxy for changes in oceanographic variables such as SST (Derville et al., 2019; Groß et al., 2020). Some of these variables are highly dynamic and have been locally changing over time, as environmental conditions are being altered by climate change, affecting all parts of the ocean (Freer et al., 2017). A comprehensive understanding of the influence of environmental conditions on this highly mobile species is required to enable any prediction of climate change and other anthropogenic effects on HWs (Meynecke and Meager, 2016; Fournet et al., 2018; Bolin et al., 2019; Meynecke et al., 2020; Santora et al., 2020; Suryan et al., 2021), and to design effective management plans for their protection (Doniol-Valcroze et al., 2007; Azzellino et al., 2014), including the development of dynamic protected areas (Maxwell et al., 2020).
Previous research has shown that HWs and other cetaceans demonstrate sensitivity toward changes in oceanographic variables, whether natural or climate-change induced (van Weelden et al., 2021). This includes the arrival of HWs in the Gulf of Lawrence shifted by 1 day per year over a 25-year period (1984–2010), resulting in significant earlier arrival on that feeding ground (Ramp et al., 2015). Encounter rates of HW groups that contained at least one mother with calf dropped by 76.5% in the Hawaii breeding grounds between 2013 and 2018, a period that coincides with a marine heat-wave observed in the area (Cartwright et al., 2019). The species also shifted its distribution and diet (from krill to schooling fish) with varying oceanographic and ecological conditions (Fleming et al., 2016; Becker et al., 2019), when SST in the California Current System increased. In a study from Oceania (population E), HWs also showed local responses to SST anomalies in their distribution in breeding grounds in Oceania with a maximum temperature tolerance at 28°C (Derville et al., 2019). Recent changes in HW feeding patterns have been observed off the west coast of South Africa, with large aggregations of 20–200 individuals involved in a novel feeding behavior (‘supergroups’) during October–November in the southern Benguela Upwelling System, which is located well north of their expected Antarctic feeding grounds (Findlay et al., 2017; Cade et al., 2021).
Given climate-induced changes in the ecosystem, including consequences to prey availability for the HWs, their reproduction can also be affected, e.g., as lower numbers of calves are observed following years of decreased prey biomass (Seyboth et al., 2021). Observed and projected changes in climatic variables include increasing ocean temperatures, changing ocean currents, rising sea levels, reduction in sea ice, more frequent and intense unpredictable weather events, and changes to the distribution and abundance of species that are prey items for HWs (Flores et al., 2012; McBride et al., 2014). Such changes need to be understood in the context of other human ocean resource-use impacts, including noise, physical and chemical pollutants, unsustainable extraction and habitat modification (Figure 1). It is anticipated that climate change will lead to a decline in the large-scale supply of nutrients due to enhanced upper water stratification in polar waters and consequently reduce the marine primary productivity driving food availability for HWs (Tulloch et al., 2018). HWs require high concentrations of prey to maximize energy efficiency while feeding (Piatt et al., 1989; Goldbogen et al., 2008). Therefore, preferred feeding ground habitats (Nicol et al., 2000; Doniol-Valcroze et al., 2007; Ressler et al., 2015), and opportunistic feeding in other locations (Visser et al., 2011), would occur where environmental conditions increase marine productivity and aggregation of prey (e.g., prior high chlorophyll-a concentration, upwelling and fronts). HWs can exhibit substantial flexibility in behaviors and prey selection and are suspected to have historically adapted their foraging behavior to new prey species when environmental conditions were unfavorable to common target prey species (Benson et al., 2002; Fleming et al., 2016). Habitat use during feeding may be heavily driven by immediate ocean conditions (Rockwood et al., 2020). Disentangling feeding-related movements driven by changing environmental cues and intrinsic/learned behavior remains an ongoing challenge (e.g., Weinrich, 1998), compounded by the need to disentangle past exploitation and current environmental change drivers (Nicol et al., 2008). Movement patterns can also be influenced by a variety of intra-specific social interactions (Ersts and Rosenbaum, 2003; Craig et al., 2014) or by human activity in particular in breeding areas (Cartwright et al., 2012) making the disentanglement from environmental change drivers more complex. There is evidence of range expansion in breeding grounds as populations recover from decades of commercial whaling (Mobley et al., 1999; Johnston et al., 2007; Lammers et al., 2011) or maybe as a result of environmental changes (Torre-Williams et al., 2019).
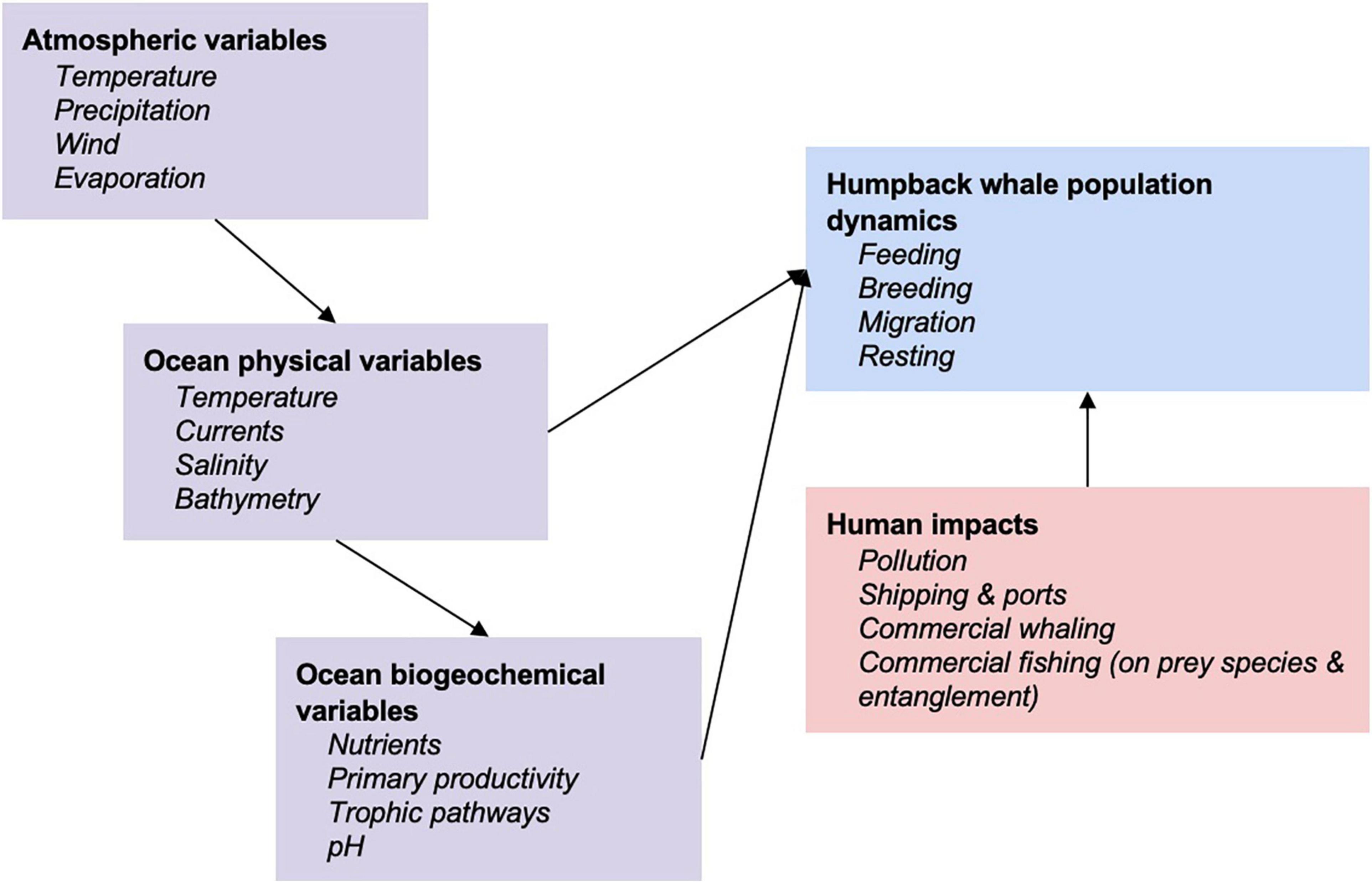
Figure 1. Conceptual framework to a systems approach of understanding humpback whale responses to environmental variables and human impacts (adapted from Meynecke et al., 2020).
In this review, we aim to extract combinations of environmental variables emphasizing on physical variables (with the addition of chlorophyll) that determine HW distribution and movements during behavioral modes of migration, breeding, feeding and resting by systematically analyzing accessible peer reviewed literature on the subject. Highlighting shared variables across populations using different habitats may provide insight into conservation efforts for understudied populations and assist with the modeling of species distribution. We also aim to identify commonly tested variables and how they influence HW behavioral modes, and outline the various methods applied to investigate the relationships. This synthesis in turn, highlights where further research is required.
Methods
We set out to review the relationship between HWs and environmental drivers by compiling the literature from online databases. We consulted Web of Knowledge, Google Scholar and Scopus. Searches for scientific literature were conducted up until 1st January 2021. Searches included multiple combinations of keywords (Table 1), with qualifying publications containing at least one word from each of the following categories: target species, environmental parameters tested (predictor variables) and the type of effect/behavior observed (response variables).
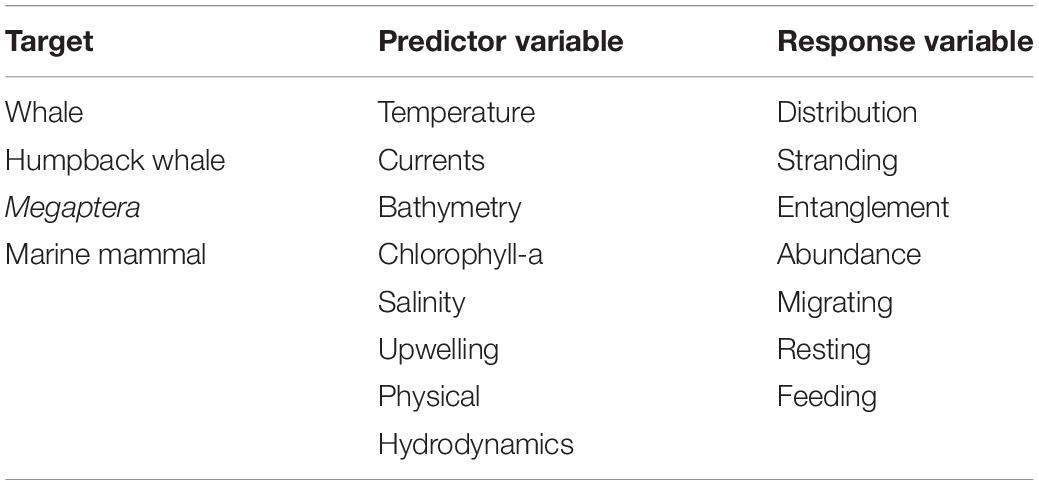
Table 1. Combination of keywords used in online search for literature related to humpback whales and environmental variables.
All parameters describing physical environmental conditions were included, and those that were very similar but differently termed were grouped together (e.g., “distance to the coast” and “distance offshore”). If results were presented in a paper without specific details on the statistical analyses, these studies were still included. Studies that lacked clear descriptive information on relationships of whales with environmental factors or were focused on prey only were excluded. We excluded studies focusing on prey only, because projections of prey availability are often spatially and temporally limited, whereas projections of SST or even chlorophyll are more readily available. Studies focusing on prey only often have different objectives to those investigating abiotic drivers and would benefit from an independent review.
The selection was based on inclusion of keywords in either, title, abstract or main text and if results on HW and environmental drivers were presented. The summary included details about the study publication (authors, year and journal of publication), timing (season and year), and location (hemisphere, country and hydrographic region) of research, the species studied (when other species besides HWs were included), method and platform of observation (whale watching boat, other type of vessel, photo ID, aerial, land-based, acoustic, tag, whaling records or stranding records), mode of behavior or effect observed (migrating, breeding, feeding, resting, stranding, entanglement), sample size (including the number of survey years per study), the range of environmental parameters investigated (including the methods of measurements used and the relationships observed), and finally whether climate change or global warming (considered as synonyms) was specifically mentioned or investigated. Publications did not necessarily include details for every category, and publications could have multiple features in some categories (e.g., both feeding and migrating behavior was observed). If the total sample size of HWs was not explicitly mentioned, then this was estimated based on the details provided and noted in the overview table.
Details about the location of fieldwork were recorded to allow comparisons between countries or regions and hemispheres, and between areas of similar/contrasting hydrography, such as eastern and western boundary currents, polar and tropical areas, or central oceanic waters. The environmental parameters investigated included temperature, hydrodynamics (this represented studies investigating the effects of currents, upwelling, etc.), chlorophyll concentration, salinity, bathymetry, distance offshore, tides and weather. As this review focuses mainly on abiotic parameters with the addition of chlorophyll concentration, prey was not included as a category in the database; however, if publications added prey as a variable or concluded that particular environmental parameters were indirectly related to cetacean distribution by concentrating prey, this was recorded and included in the review.
Results
Overview of Studies and Summary of Findings
A total of 148 studies that investigated the relationships between HWs and their surrounding physical environmental conditions were extracted (Supplementary Table 1). An additional 31 studies were disregarded after detailed assessment; in these cases, environmental drivers were not explicitly defined, discussed, not the focus of the study or no analyses were performed to investigate relationships.
A trend over time (1981–2020) showed an increase in online accessible peer reviewed studies investigating HW relationships with environmental drivers. In particular, in the past 4 years between 10 and 18 studies have been published per year. Climate change has also been addressed more often in recent years proportionally to the total number of publications (Figure 2). Forty-three studies mentioned climate change, climate shift or global warming (e.g., Bassoi et al., 2019) and two studies used climate projections in their analysis (Thompson et al., 2012; Derville et al., 2019).
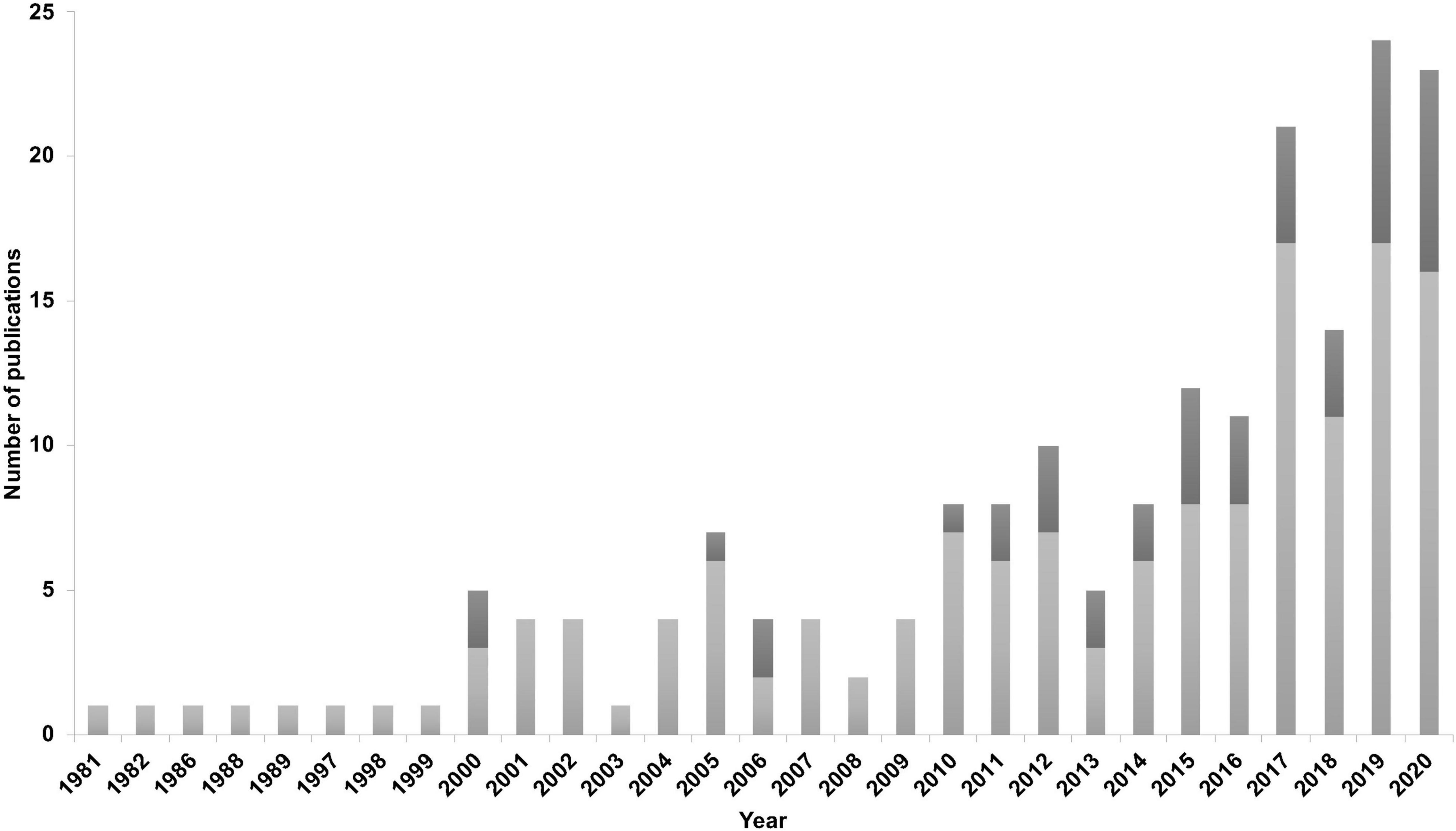
Figure 2. Number of retrieved studies investigating humpback whales and environmental drivers between 1981 and 2020. Dark gray bars indicate mention of climate change or climate shift in the studies.
Most studies were conducted in regions attributed to the United States, followed by Australia, Antarctica and Brazil (Figure 3). This regional bias also reflects the bias of the populations studied. The North-eastern Pacific populations were subject to a high number of studies (39), followed by population G and the North Atlantic populations (both in 20 studies) and population A (14). In the Southern Hemisphere, population E was included in a higher number of studies (12) and all other populations less than 10 times. However, Southern and Northern Hemisphere populations have been equally represented in the selected studies (78 and 75 times, respectively, counting double the few studies conducted worldwide).
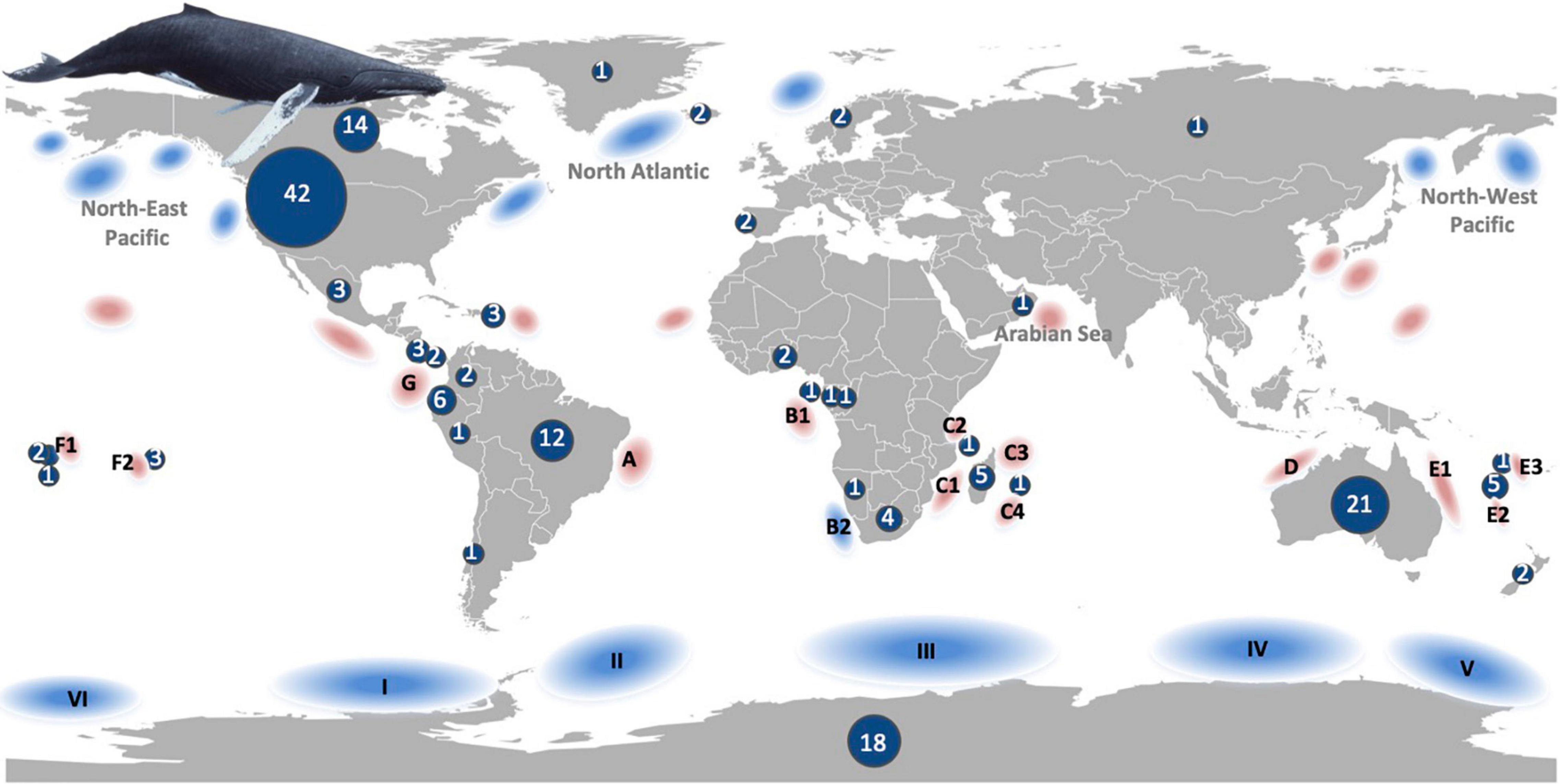
Figure 3. Humpback whale populations (at least 16 distinct populations shown as combined North-East Pacific, North Atlantic, Arabian Sea, North-West Pacific for the Northern Hemisphere and A-G breeding populations for the Southern Hemisphere) with approximate breeding (red) and feeding (blue) areas (classification based on Jackson et al., 2015). The number of studies extracted from 148 publications that investigated environmental drivers and humpback whale distribution are shown by country and separately for Antarctica.
The most accessible regions for HW research occurred near the coast or in the vicinity of islands. Coastal habitats were therefore included in half (74) of the studies while open ocean habitats were only subject to investigation in 22 studies with 5 involving satellite tags or remote sensing. Overall, our review has stronger contributions from HWs populations in the North-East Pacific, North Atlantic and G population and from studies conducting data collection in coastal waters. This is important to note when ranking the environmental drivers in order of relative importance. Certain environmental drivers were tested more often simply because they were the most likely to be applicable for coastal habitats and the particular HW populations studied.
The majority of studies (91) used dedicated survey vessels to collect data. Less common were studies using photo ID and acoustics (12 studies each), land-based surveys (16) and aerial surveys (17). Citizen science or whale watch boats were used in 18 studies and satellite tagging in 26 studies. Some studies (34) used more than one source of data. The number of HW records used for each study varied greatly between 5 (satellite tagging, Curtice et al., 2015) and over 10,000 records (citizen science, Stack et al., 2019).
Feeding in relation to environmental variables was the focus of a high number of retrieved studies (63) and consequently investigations were carried out mostly during summer months (Northern or Southern Hemispheres) (84) followed by winter (78), spring (47) and autumn (35). Migration was explicitly mentioned in 55 studies and resting mentioned in 19 studies. Relationships between entanglements and strandings and environmental drivers were mentioned in 7 studies (e.g., Meynecke and Meager, 2016; Volep et al., 2017). In a number of studies (69) other cetaceans were included in the analyses with up to 35 other species of which fin whales (Balaenoptera physalus) and minke whales (Balaenoptera acutorostrata and Balaenoptera bonaerensis) were most often included (Supplementary Figure 1).
Methods Applied Relating Environmental Drivers to Humpback Whale Distribution
The majority of studies included in this review used multivariate analyses whereas remaining studies adopted a range of other statistical approaches investigating single variable responses or described relationships based on field observations. Generalized Linear Models (GLMs) were most often applied (39), followed by General Additive Models (GAMs) (28), regression and correlation analyses (21), Maximum Entropy Models (MAXENT) (10), and other statistical approaches (7), such as Mann–Kendall trend test or Mann–Whitney U Test. Some studies have focused on a descriptive evaluation of the relationships, e.g., through visual representation showing the overlap of sightings with environmental variables (17) and others have focused on the analyses of density estimates that included variables as a function of sighting probability using Distance sampling (Thomas et al., 2010) (8) (Figure 4). Multivariate models have become increasingly popular with nearly half of all retrieved studies between 2010 and 2020 using GLMs, GAMs or MAXENT (Becker et al., 2010; De Rock et al., 2019). In general, multivariate models can capture complex interactions better than other statistical approaches, address auto- and cross-correlation of variables; and therefore, provide a more comprehensive representation of real-life ecosystems (Digby and Kempton, 2012). Such modeling approaches have become more attractive due to the availability of larger data sets and improved computational power allowing for faster processing.
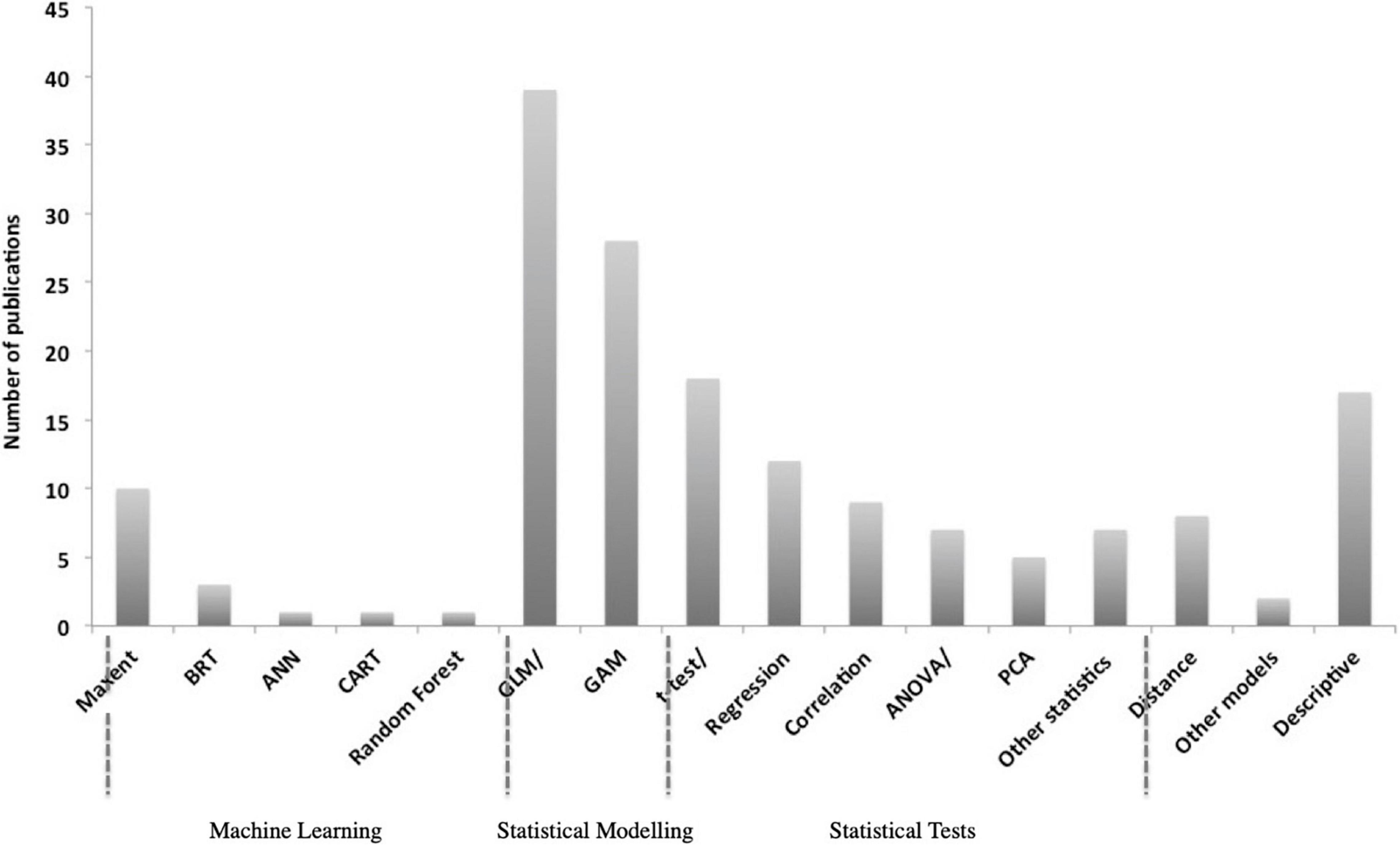
Figure 4. Tendency in the use of methods in order of complexity applied to study humpback whale relationships with environmental drivers extracted from 148 publications. Only the most frequent used methods are presented here based on retrieved studies between 1981–2020 and divided into machine learning techniques, statistical models and tests, and a category that includes usage of Distance software, other seldomly applied models (e.g., occupancy model or relative environmental suitability models) and descriptive assessments.
Overview of Environmental Data Sources and Whale Records in Reviewed Literature
A variety of open-source datasets e.g., through the National Oceanic and Atmospheric Administration (NOAA) Data Catalog1 and other datasets have been frequently used to investigate environmental drivers of HW distributions (Shelden and Rugh, 2010; Guidino et al., 2014; Garrigue et al., 2015). Each approach has its benefits and their selection largely depends on suitability of the data and the purpose of the studies.
Temporal and spatial resolution of the environmental data determines what type of analysis may be possible. Availability of marine environmental data with a large temporal (decades) and spatial resolution (global) is still very limited. In terms of Earth Observations, satellite SST and sea ice concentration have been one of the few variables available for the past 40 years since 1979 (Merchant et al., 2019). SST can be obtained from remote sensing data from AVHRR (Advanced Very High-Resolution Radiometer Pathfinder) or MODIS (Moderate Resolution Imaging Spectroradiometer). For instance, resolution in the studies ranged from 1 km grids (e.g., Doniol-Valcroze et al., 2007) to 28 km grids (e.g., Derville et al., 2019) and from daily (e.g., Picanço et al., 2009) to annual averages (Kaschner et al., 2006).
Variables such as bathymetry, slope, distance to shore and in most cases terrain type are reliable measures to determine suitable habitats as they vary little over time. Typical units for these are measurements of length, angle or categorical values for terrain type (e.g., “rugged or smooth”). Bathymetry has predominantly been derived from bathymetric charts ranging in 50 m2 to 1 km2 resolution on predefined grids (Lindsay et al., 2016; Lodi et al., 2020). In some regions, topography data were available from government agencies (e.g., hydrographic services) and related variables such as slope were calculated from bathymetric charts. Distance to shore was usually calculated using coastline data (Tardin et al., 2019). The dynamic distance to sea ice edge on feeding grounds (can be defined by the border of minimum 50% sea ice coverage) was obtained from the United States National Snow and Ice Data Center (NSIDC). The majority of environmental variables change over timescales that range from days to years. Traditionally, biotic and abiotic measurements were taken during HW surveys at sea, only covering a small area and timeframe. Larger areas over longer periods were sampled, for example through the Array for Real-time Geostrophic Oceanography (ARGO) Program providing temperature, conductivity and pressure from floating data loggers as well as current velocities (Roemmich et al., 2019). Early on, SST data was measured either as point data or continuously from survey vessels (Smith et al., 1986) but in recent years in situ temperature data as well as its gradient over depth were generally obtained using CTDs (Conductivity, Temperature, and Depth logger), in some studies to 1 km depth (Hazen et al., 2009; Munger et al., 2009; Keen, 2017).
Chlorophyll concentration was typically obtained through on-board fluorometers (Keen, 2017) or from daily to monthly remote sensing data through MODIS Aqua (9 km grid resolution), SeaWiFS (Sea-viewing Wide Field-of-view Sensor) or MERIS (Medium Resolution Imaging Spectrometer; 4 km grids) (e.g., García-Morales et al., 2017). Generally, a finer temporal and spatial resolution is desirable for chlorophyll concentrations due to its fine scale variation in time (days) and space (Wernand et al., 2013). In some studies, prey density or species that were considered potential prey were included in the analyses (Nowacek et al., 2011). Associated data on these were region specific and either collected during surveys through netting or echo sounder or derived from commercial landings. While prey density is the most promising variable to define feeding events and feeding habitat, it is also not available over large spatial and temporal scales (Siegel and Watkins, 2016). This is complicated further as HWs have been known to shift prey species depending on prey availability (Fleming et al., 2016; Owen et al., 2017).
For information on surface currents, ARGO drifter buoys were used in more recent studies (Horton et al., 2011). Daily sea surface current velocity (m/s) data were typically obtained from the Copernicus Marine Environment Monitoring Service (CMEMS) or when available from regional models (e.g., Bluelink) (Bolin et al., 2019; Riekkola et al., 2019) or the regional oceanic modeling system (ROMS) (Shchepetkin and McWilliams, 2005). Upwelling indices provided by NOAA or derived in situ using AD (Acoustic Doppler Current Profiler) have also been used (Nicol et al., 2000; Shelden and Rugh, 2010). Tide charts or regional tidal models were utilized to derive tidal data, such as its range or constituent.
Weather related variables such as wind speed/direction, sea state, air pressure and visibility were in most cases collected during observation or derived from open-source databases. Riekkola et al. (2019) used SSH data in meters (55 km2 or 27 km2 grids) derived from Copernicus Marine Environment Monitoring Service (CMEMS). Large scale climate indices like SOI, PDO, ONI (Oceanic Niño Index), SAM and NPGO were available from meteorological services and applied on a monthly or annual temporal resolution (e.g., Dransfield et al., 2014; Avila et al., 2019; Cartwright et al., 2019; Groß et al., 2020).
Most (91) of the HW records in the studies presented here were derived from dedicated scientific surveys (e.g., Salden, 1988) that included some form of abundance estimations (as opposed to presence data only). However, scientific surveys are expensive and are often limited in time, space and effort. Deriving data from multiple sources can overcome data scarcity (Pacifici et al., 2017). Citizen science data has become increasingly relevant and has allowed studies to cover larger temporal scales often with presence only data (Tiago et al., 2017; Derville et al., 2019; Stack et al., 2019; Valani et al., 2020). Other solutions to gain higher spatial and temporal resolution of whale records were the use of multi-species data sets collected as part of reoccurring scientific surveys (Chenoweth et al., 2011).
Satellite tagging of HWs can provide many details of an individual over large areas and a good temporal resolution. However, the limited number of whales observed as presence only data (often under 20 per study) limits the extrapolation of observations to whole populations. On the other hand, datasets covering thousands of records lack detailed information on individuals and are often limited to a certain region.
Overview of Applied Methodologies in Reviewed Literature
A classical approach to study marine species distribution patterns is by applying types of linear regression (e.g., multiple linear regressions by least squares, ordinary least squares models or general linear models) (e.g., Baker and Herman, 1981). Linear regression is easy to apply fitting linearly separable datasets. However, linear regression and correlation analyses are limited by their assumption of linearity, use of dependent variables and sensitivity to outliers (Smith and Santos, 2020). Other used statistical approaches to test environmental variables with species presence are Paired t-tests, Mann–Whitney U-test, G-test, and ANOVAs. All have similar limitations as linear regression analyses. Some studies have used ‘Distance sampling’ that also allows for assessing the influence of habitat variables on abundance estimates (Thomas et al., 2010) but this technique is designed to overcome sampling constraints in surveys and not to analyze relationships of species distribution with environmental variables.
To overcome some of the above limitations, species distribution models (SDMs) have been used. Generally, they can be described as mathematical tools allowing the description and prediction of distribution patterns of species and comprise several modeling techniques/algorithms [e.g., GAMs, GLMs, boosted regression trees (BRTs), random forests (RFs) and MAXENT]. Various studies have provided insights in the different types of SDMs and their performance (Derville et al., 2018; Smith et al., 2020b).
The choice of a specific SDM varies greatly and depends upon the study goal, the availability of data (e.g., occurrence data, need to simulate pseudo-absence, functional traits) and the assumptions and constraints applied. Generally, the use of SDMs comprise the following four steps: (i) model selection, (ii) model implementation, (iii) model validation and (iv) model error and uncertainty estimation. The first step is model selection, which includes implementation of statistical procedures (e.g., cross-validation, stepwise selection) to select the set of predictor variables. In the second step, the selected model predicts a spatial pattern, which can include parametric models (e.g., GAMs and GLMs) or machine learning techniques (e.g., BRTs, RFs, and MAXENT). Validation involves the use of performance measures [e.g., Area under the Curve (AUC), specificity, goodness-of-fit, and regression] to estimate the validity of the model output. The last step quantifies the errors and uncertainties that may derive from insufficient data and model misspecification.
For example, the choice for parametric models (GLMs and GAMs) depends mainly on the type of input data, with GAMs capable of handling species data with a widespread distribution. This makes GAMs often more flexible as simulation models than GLMs. However, this has consequences for robustness, with GLMs being more robust than GAMs.
More recently, machine (e.g., MAXENT, BRTs, and RFs) and deep (Artificial Neural Networks, Purdon et al., 2020) learning techniques are being used for SDMs, which allow the testing and fitting of multiple interactions among predictors and are tolerant of outliers, collinearity, and irrelevant predictors. Importantly, these techniques do not require strong assumptions prior to model selection. Taking into account the benefits and constraints of different SDMs, some studies have compared their performance on cetacean distribution [e.g., GLMs vs. GAMs; GAMs vs. MAXENT, Fiedler et al. (2018); BRTs vs. MAXENT vs. GLMs vs. GAMs, Derville et al. (2018)]. Machine learning techniques showed excellent explanatory performance when discriminating between presence and absence, but poorer performance when predicting on independent data (Oppel et al., 2012; Becker et al., 2020). The inferior predictive performance has been attributed to machine learning techniques overfitting to a greater degree than parametric models.
Boosted regression trees and ensemble approaches (e.g., Abrahms et al., 2019 on blue whales) have the ability to automatically fit interactions between predictor variables. On the contrary, in GAMs interactions between predictor variables must be explicitly defined when fitting. Different authors found contrary evidence between advantages and disadvantages of using BRTs and GAMs. Elith et al. (2008) suggested that an advantage of BRTs over GAMs is that they could handle sharp discontinuities when modeling species with distributions that occupied only a small proportion of the sample’s environmental space. Becker et al. (2020) found the opposite, with GAMs performing well and BRTs exhibiting poor predictive ability. The study suggests that both models should be used and that caution should be taken when applying BRTs to anomalous novel data and when including spatial terms (latitude and longitude) in the suite of potential predictors.
Among presence-only data models, MAXENT is the most popular. This technique is capable of incorporating model complexity while preventing overfitting and is appropriate to predict areas of potential species occurrence based on comparison with automatically generated background data. An important limitation that affects the accuracy of presence-only modeling relates to biases in the occurrence localities. For instance, Fiedler et al. (2018) compared MAXENT vs. GAMs performances for four large cetaceans by applying presence-absence or presence only data. Both methods produced very similar predictions when background data points were selected from observed absences. However, when presence-only data was modeled with pseudo-absences the spatial pattern of predictions was considerably altered. Its output can thus be interpreted in one of two ways, depending on sampling assumptions. If the data are assumed to be a random sample in space, it can predict relative occurrence probability but not occurrence probability. And if the data are assumed to be a random sample of individuals, it can predict relative occurrence data. In light of the availability of larger data sets, machine and deep learning methods utilizing Artificial Intelligence are becoming more popular as powerful methods for analyzing complex ecological relationships (Figure 4).
Main Environmental Drivers and Their Relationships With Humpback Whales
We have identified some main environmental drivers from the reviewed studies which include: bathymetry, temperature, distance offshore, chlorophyll, hydrodynamics, weather, salinity, tide, ice, turbidity, terrain and magnetic fields (Figure 5). A number of other variables fall under these main drivers and have been grouped accordingly. Details on all variables used in the reviewed studies can be found in Supplementary Table 1.
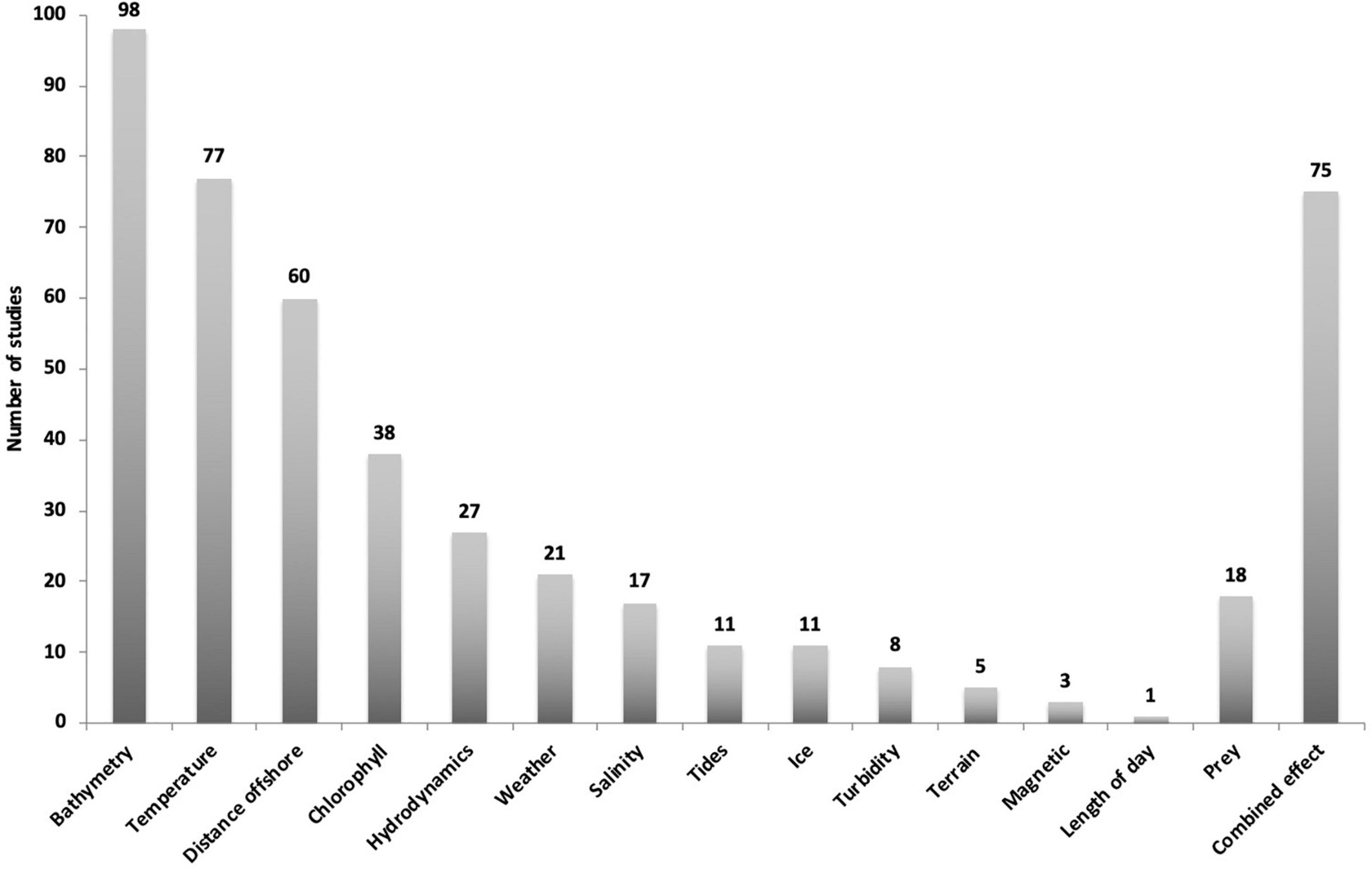
Figure 5. Overview of environmental driver categories from 148 publications investigating humpback whales and relationships with environmental drivers. Most studies tested more than one variable with 75 studies testing combined effects.
Bathymetry, temperature, distance to shore and their associated variables were the most common parameters investigated, being included in 137 studies. Fewer publications have included the effects of variables such as chlorophyll concentration, hydrodynamics, weather, salinity, and tides. Out of the 148 studies, bathymetry and associated variables were investigated 98 times, SST 77 times and distance to shore or ice edge 60 times. The categories turbidity (8), terrain type (5) and magnetic fields (3) were the least tested and 18 studies included prey as an additional variable (Figure 5).
The same environmental drivers can play very different roles for HWs depending on the whale’s behavioral mode and are related to the specific regional conditions. The regions used by HWs have contrasting characteristics (SST between −2 and 5°C in Arctic or Antarctic waters and 23–27°C along tropical coastlines and open ocean). Bathymetry has been a reliable predictor for the location of breeding grounds (Smith et al., 2012) but influences migration to a lesser degree (Horton et al., 2011). Hydrodynamics such as current speed and upwelling are more relevant for feeding activities. Environmental drivers are often correlated and overlap, which complicates analyses (Figure 6).
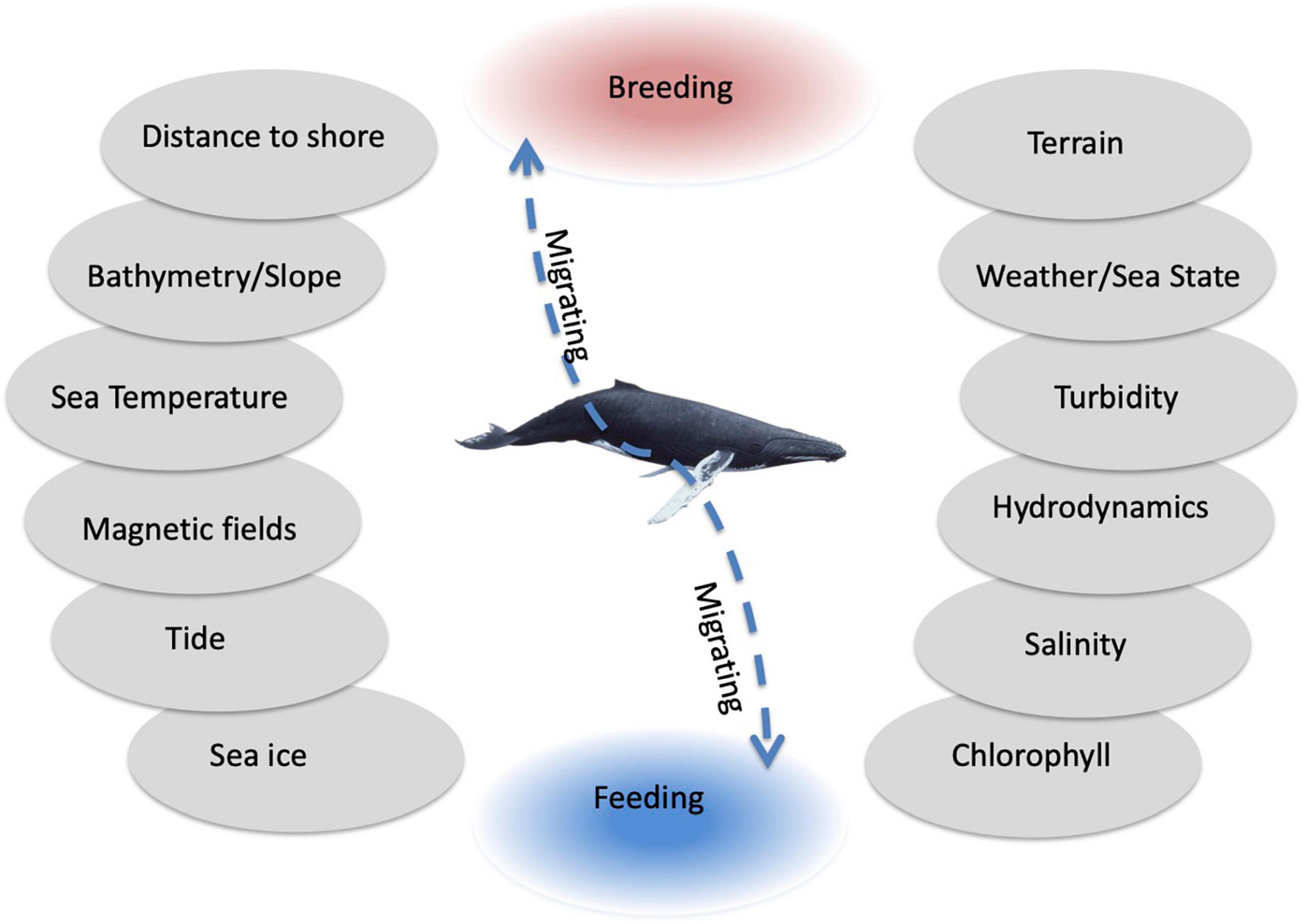
Figure 6. The main environmental drivers in order of relevance to humpback whale breeding (red), migration (dashed line), and feeding (blue) from bottom to top (e.g., sea ice concentration and chlorophyll being more important for feeding and distance to shore and terrain being more important for breeding).
We have grouped together the 73 studies that claimed to isolate the impact of one single driver or that analyzed only one variable (Figure 7). In many of these, bathymetry and temperature and associated variables (e.g., slope, SST) were individually investigated and the majority found them to significantly influence parts of the HW behavioral modes and habitat selection. A detailed overview of each study and their investigated variables is presented in the Supplementary Data. In the following paragraphs we will provide an overview of the findings for each variable category.
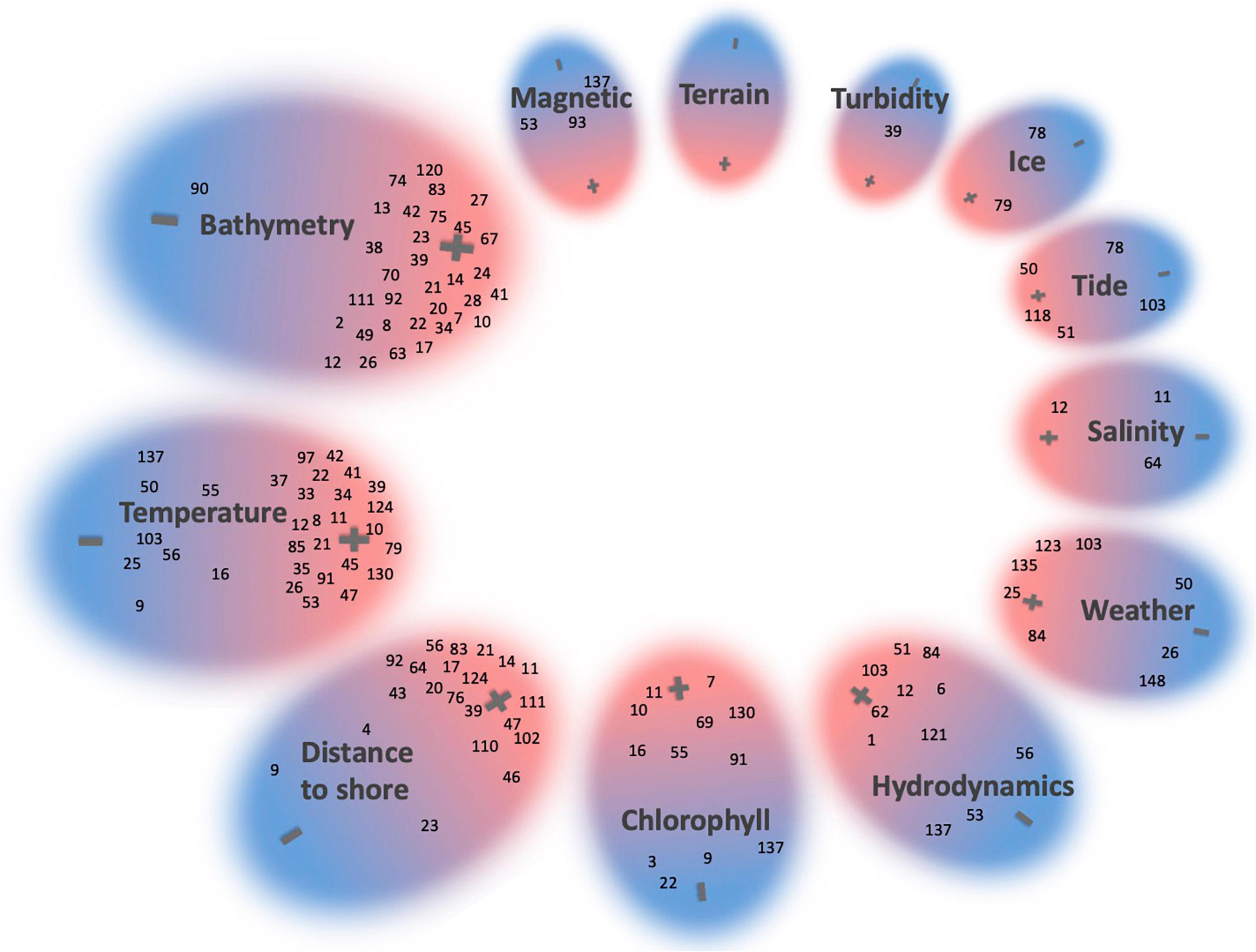
Figure 7. A representation of 73 studies assessing HWs and single environmental drivers. A “+” indicates that an influence of the variable was found and a “–” that no influence of that particular variable was found. Studies identified a weak relationship were placed in the middle. If more than one variable was analyzed, studies were assigned to multiple variables (e.g., temperature, bathymetry, and distance). The size of circles is related to the number of studies testing that particular variable. Studies were indexed (see Supplementary Data for a list of references).
Environmental Drivers Influencing Humpback Whales Behavior Modes
Feeding
The diet of HWs in general includes different types of prey (fish, krill, squid, and copepods) (Baker et al., 1985; Clapham and Palsboll, 1997; Hauser et al., 2010). Some populations have shown plasticity in their diet and adapting to prey availability (Gavrilchuk et al., 2014; Fleming et al., 2016) with different feeding techniques such as lunge feeding (Ware et al., 2011), bubble net feeding (Friedlaender et al., 2011), bottom feeding (Hain et al., 1995), pectoral herding (Kosma et al., 2019) and feeding in groups (Friedlaender et al., 2011; Findlay et al., 2017), or feeding individually (Ware et al., 2011). Such feeding at different trophic levels compounds investigation of environmental drivers, particularly with lags in environmental changes, primary and secondary productivity. The type and strength of relationships between physical variables and HW distribution in feeding areas depends on the type of selection and region, making the establishment and generalization of relationships with physical drivers challenging. There are also lag effects between drivers such as upwelling and prey availability and observed feeding events (or abundance in a feeding area), and synergistic effects from different variables. Measuring the relative importance of each for HWs distribution can be a difficult task. For example, HWs in the Southern Hemisphere predominantly feed on Antarctic krill (Euphausia superba) (Bettridge et al., 2015) whereas HWs in the Northern Hemisphere mostly feed on fish species (Evans, 1987).
Despite these challenging aspects, there are a number of variables that evidently are more reliable predictors for feeding grounds than others. For example, high relative abundance of HWs was observed in cooler waters (e.g., Orgeira et al., 2017) near the boundaries of major currents (Bestley et al., 2019) and regions of upwelling (e.g., Thompson et al., 2012) with high chlorophyll concentration (Tynan et al., 2005).
Some studies have found relationships between feeding activities and particular ranges of depths and temperature. In a multi-species study by Calambokidis et al. (2004) from the west coast of the United States, most whales, including HWs, were sighted between 100–200 m depth contours (8.4 km from shelf) in relatively colder areas (mean of 13.9°C) where upwelling occurred. This is similar to the findings of Dransfield et al. (2014) who showed that the highest HW counts were associated with an SST between 12 and 14°C on this coast. However, Burrows et al. (2016) found no significant relationship between depth and feeding in a multi-species study from California (United States) indicating contrasting results for depth and temperature variables. Further north in the Bering Sea, Zerbini et al. (2016) found that HW abundance was low in shallow depths, and generally high at intermediate and greater depths (200 m). Feeding dives of up to 200 m were also reported from Digital Acoustic Recording Tags (D-tags) attached to HWs near West Greenland (Bejder et al., 2019) and 300 m in the West Antarctic Peninsula (Nowacek et al., 2011). A study in Antarctica by Bombosch et al. (2014) found that SSH (Sea Surface Height Anomaly) (19.6% of deviation explained) and SST gradient (13%) were the best explanatory factors in the final distribution model. Independently of depth, temperature effects were investigated in most of these studies, with mixed results but generally a positive relationship with cooler waters was found (Nicol et al., 2000; Barendse et al., 2010).
Chlorophyll concentration was often included for investigation as a proxy for prey availability and was often more accessible than data on prey, by being accessed through remote sensed satellite data or measured in situ during surveys. Various studies have shown relationships between chlorophyll concentration and potential HW prey species (Atkinson et al., 2008; Kershaw et al., 2021). Higher HW abundance was observed in conjunction with high chlorophyll concentrations in feeding areas such as fjords in Canada (Keen et al., 2018). Owen et al. (2019) showed that chlorophyll concentration was significantly correlated with the broad-scale foraging behavior of five tagged HWs in East Antarctica, similar to Bestley et al. (2019) indicating the role of persistent primary production in foraging behavior. A mixed species study from the west coast in South Africa showed higher abundance in waters with relative high chlorophyll-a concentrations in summer (around 4 mg/m3) (Purdon et al., 2020). However, a study from Antarctica by Andrews-Goff et al. (2018) showed no direct relation between seasonal chlorophyll-a and HW foraging habitat when using chlorophyll climatology as a proxy for primary production. This is likely due in part to the inability of satellites to measure productivity of closely ice-associated habitats. Additionally, persistent cloud cover necessitates averaging of remotely sensed chlorophyll measurements (here across a 3-month period) and this has been leading to the loss of temporal information.
Among the least considered variables were ebb and flood tide, and internal waves, for which no significant relationship with feeding was found by Pineda et al. (2015), and dimethyl sulfide (DMS), a chemical released in areas of high marine productivity for which a relationship with HW behavior was found in Iceland and Madagascar, but not in Antarctica (Bouchard et al., 2019). Flood tide was a driver for feeding events of HWs in the Gulf of Maine (Hazen et al., 2009) and Alaska (Barlow et al., 2019) where feeding on fish occurs in tidal influenced bays and fjords.
Overall, environmental drivers for feeding behavior of HWs are region-specific and are best described as a combination of multiple factors. For instance, Riekkola et al. (2019) identified distance to the ice edge (with 2-months lag), SSH and the specific period of the year/region as relevant predictors of the behavioral state of HWs within their Southern Ocean feeding grounds. Similarly, large scale weather drivers such as the Southern Annular Mode (SAM) or Antarctic Oscillation were found to explain almost 24% of the variability of fatty acids (related to type and amount of food intake) in HWs over a 10-year period of the E1 population feeding in East Antarctica influencing migration patterns (Groß et al., 2020). In the Northern Hemisphere, at the feeding area at Cordell Bank north of San Francisco (United States), HW habitat use was associated with fluorescence, temperature and salinity. In addition, bathymetry, distance to the shelf break, distance to islands, coast and four climate indices (Upwelling Index – UI, Pacific Decadal Oscillation – PDO, North Pacific Gyre Oscillation – NPGO, and Southern Oscillation Index – SOI) also remained as significant covariates in the final GLM (Rockwood et al., 2020). In the Atlantic, Ramp et al. (2015) were able to determine a link with the North Atlantic Oscillation (NAO) index, reinforcing the overall role of the main climate patterns as significant variables in explaining feeding behavior.
A number of studies added prey abundance as variables to improve the predictive capacity of their models. This method, however, requires longer time series due to high variability in prey abundance and such times series are often difficult to obtain. For example, the best results from a GAM included SST and krill abundance for explaining isotopic signatures in HW sampled in the California Current (United States, Fleming et al., 2016) and a 40% contribution (in a second GLMM after some covariates were removed) of herring spawning and HW calving rate was determined for the Gulf of St Lawrence (United States) feeding area (Kershaw et al., 2021).
Breeding
Breeding grounds include areas with activities such as calving, nursing and mating. Breeding grounds are typically identified by shallow (<50 m), warm (21–28°C) and calm waters, close to the coast where HWs spend several weeks to give birth and nurse their newborns (Jenner et al., 2001; Smith et al., 2012; Bruce et al., 2014; Irvine et al., 2018). They can extend along the expanse of the migratory corridor rather than in precise identified locations. In fact, a number of studies have demonstrated that breeding behaviors have occurred outside recognized breeding grounds for HW populations (Bruce et al., 2014; Lucena et al., 2015; Irvine et al., 2018). HWs can calf every 2 years depending on feeding success (Baker and Herman, 1987; Torre-Williams et al., 2019) and the gestation period is around 12 months (Chittleborough, 1958).
Bathymetry is the driver most often identified with HW calving, breeding and resting grounds. This includes associated variables such as bed slope (e.g., Sleeman et al., 2007; Chou et al., 2020). Generally, there is consistency of the findings throughout all populations. Most studies reported significant relationships with depth ranging between 20 and 50 m. Steep, continental slopes and deep waters are generally avoided by HWs when breeding. These patterns of habitat preferences were also reflected in individual movements recorded through satellite tracking (Garrigue et al., 2020). New Caledonia singletons and pods with calves preferred depths of less than 20 m (Derville et al., 2019) and in the Great Barrier Reef the preferred depth range was between 30 and 58 m (Smith et al., 2020a). These findings were similar for the population G in Costa Rica and Peru (20–50 m depth) (May-Collado et al., 2005; Guidino et al., 2014). Breeding areas in offshore reef environments appear to be slightly deeper with preferences found around 50 m (Garrigue et al., 2020) likely because deeper waters are close by compared to that of breeding areas on continental shelves.
Sea surface temperature preferences for breeding grounds generally ranged from 19 to 28°C. There are some regional differences with 21–23°C reported for the Great Barrier Reef (Smith et al., 2020a), 22.3–27.8°C for Oceania (Derville et al., 2019), 19.4–26°C for Brazil (Tardin et al., 2019), average 24.6°C for breeding grounds of population G (Rasmussen et al., 2007), any temperature above 20°C for population D on the west coast of Australia (Burton, 2001) and above 21°C for Hawaii (Johnston et al., 2007). The highest tolerated temperature is believed to be around 27.7°C (Derville et al., 2019). However, there may also be different temperature preferences both within and among breeding ground sites (Beaugrand and Kirby, 2016).
Given the identified shallow water preference for mother-calf pairs, it is not surprising that the related variable distance offshore (or for reef and island environments: distance to reef or island) showed significant relationships in a number of studies (e.g., Ersts and Rosenbaum, 2003; Lindsay et al., 2016). Breeding behavior and high HW abundance in breeding grounds generally occurs in close proximity of a few kilometers (<10 km) to continents, islands or reefs consistently throughout a number of regions and populations worldwide. Mothers and calves are found predominantly within 1–5 km to shore (Salden, 1988; Ersts and Rosenbaum, 2003). However, in shallow bays preferences for distance to shore can be much further from the shoreline (e.g., up to 70 km, Chou et al., 2020), indicating that shallow slopes and bathymetry are better variables to describe suitable breeding areas than coastal distance. Other variables considered when investigating breeding areas were SSH and wind speed, both indicators of mesoscale circulation and turbulence. Calmer conditions allow calves to remain close to their mothers and may assist with nursing and lower overall energy expenditure (Whitehead and Moore, 1982; Martins et al., 2001; Oviedo and Solís, 2008). An example is the Bay of Guinea (West Africa) which is a large open embayment where HWs exhibit breeding behavior in low wind speed (5.9 ± 1.3 m/s) over several months (Chou et al., 2020). Such conditions are expected in bays and areas protected by reefs and islands.
Resting
Resting occurs during breeding, feeding and migration [described as prolonged surfacing periods, logging, reduced swimming speed and permanence in the same area for days or even weeks (Franklin et al., 2011)]. Prolonged periods of resting mostly occur during migration by mother-calf pairs for nursing, and to reduce energetically expensive associations with competitive male groups (Ersts and Rosenbaum, 2003; Franklin et al., 2017). Calm, shallow (<50 m) waters are utilized as resting places by mothers and calves during their journey to summer grounds (Franklin et al., 2011; Valani et al., 2020). Only a handful of studies have focused on environmental preferences for resting behavior and resting areas, likely because they are not as easy to define as feeding or breeding grounds. Resting areas are found in protected waters with shallow depth and calm surface conditions (Meynecke et al., 2013). Water depths and distance to shore have therefore been significant variables to describe resting behavior (e.g., Stack et al., 2019). The preferences for resting areas are similar to those of breeding areas, with resting mother-calf pairs found in 30 m or less depth of water (Valani et al., 2020) and in close proximity to shore often in open or closed embayments (Franklin et al., 2011; Bruce et al., 2014). Short periods of resting may also occur during offshore migration and feeding but limited information is available, with information mainly coming from direct observation or tagging (Weinstein et al., 2017). Lower SST (relative to surrounding waters) may be preferred during resting along the migratory corridor (Reinke et al., 2016; Tardin et al., 2019). For example, differences between northern and southern migration characteristics along the Agulhas Current were identified in South Africa (Findlay and Best, 1996; Findlay et al., 2011).
Migrating
Migratory behavior can be defined as any direct movement over prolonged time in the same direction, at steady swimming speed and excluding breeding, feeding and resting (Andrews-Goff et al., 2018). Mother-calf pairs will travel significantly slower (e.g., in the range of 2–4 km/h) than other pods (e.g., in the range of 4–5 km/h) (Noad and Cato, 2007).
Migration is likely a result of energetic advantages, allowing whales to take advantage of seasonally abundant prey resources in cooler waters and maximize reproductive success in warmer waters (Clapham, 2000; Rasmussen et al., 2007). The timing of migration and breeding is separated by maturity, sex, and reproductive cycle (Chittleborough, 1965; Dawbin, 1966; Craig et al., 2003). Valsecchi et al. (2010) also identified the selection of different migratory routes and the potential for sub-structure within populations in Australia. Craig and Herman (1997) identified that the timing of migration has shown to vary due to different energy requirements within HW cohorts in that males are more likely to maximize movements during migration for mating opportunities. Genetic and photographic data, and historic whaling data have shown a higher abundance of males along migratory corridors and breeding grounds, which may also be related to catch selection and observation bias (Chittleborough, 1958, 1965; Clapham, 1992; Brown et al., 1995; Craig et al., 2003). In contrast, females minimize energetic costs during migration and may not migrate every year (Best et al., 1995) if not breeding.
Mother-calf pairs are found closer to shore during migration and have more stop overs and resting than singletons or pods without calves (Bejder et al., 2019). Generally, HWs stay in proximity to shore and within the continental shelf while migrating near continents (e.g., Best and Ross, 1996; Paton and Kniest, 2011). Calambokidis et al. (2019) estimated that HWs spend the majority of their migration time within 30 m of the sea surface (90% at night and 69% during daytime). However, deep dives (400 m) have been reported during offshore migration (Derville et al., 2020). Model analyses found a relationship with distance to coast in Brazil and Chile but not with bathymetry (Viddi et al., 2010; Tardin et al., 2019). Burton (2001) also reported an avoidance of hypersaline waters in the Western Australia migration route. Once HWs leave the coast or reefs, there are limited available data on their migratory behavior other than historic whaling data, satellite data from individuals or some observations from offshore surveys. During migration in offshore environments no particular preference for bathymetry has been found (Rosenbaum and Collins, 2006). However, seamounts may play an important role in navigation. Derville et al. (2020) reported spatially structured movements of satellite-tagged HW around shallow seamounts (<200 m). Residence time significantly increased with proximity to shallow seamounts, while dive depth increased in the vicinity of seafloor ridges. This is in line with another satellite-tagging study for population C, where mixed cohorts traveled significantly faster during deep-water transit than shallow-water transit over 1–71 day deployment duration (Dulau et al., 2017). Minimal or no contribution of SST or chlorophyll to migratory behavior was found (Tardin et al., 2019; Groß et al., 2020; Horton et al., 2020). Stephenson et al. (2020) found SST to be a third-degree model contributor (with mixed layer depth and slope being first and second contributor respectively) in a multi-species study from New Zealand, but current literature on HWs does not appear to support a strong influence of SST on migratory behavior.
Considering that HWs cover vast areas of open sea, they also cross various currents and are exposed to strong weather conditions. A direct influence of weather on HW migratory behavior has not been documented to the best of our knowledge, while currents are the most investigated drivers. HWs have shown evidence of utilizing currents flowing in the direction of travel (Baker and Herman, 1981) and avoiding those flowing in the opposite direction (Findlay and Best, 1996; Burton, 2001; Findlay et al., 2011), while larger scale studies (covering oceans) showed no effect of currents suggesting that HWs are able to compensate and remain on direct migration paths (Horton et al., 2011, 2020). The influence of currents could also be dependent on the cohort with mother-calf pairs needing more resting time than other cohorts. Increased entanglements in near shore shark nets (400–500 m from shore) by mother-calf pairs as a proxy for higher number of whales were related to the pathway of the East Australian Current (EAC), with entanglements significantly increasing when the EAC was closer to shore (Volep et al., 2017; Bolin et al., 2019). This is a similar response to what Burton (2001) discovered off the west coast of Australia, where whales were resting outside major currents.
Discussion
This review synthesized 148 studies investigating HWs and their relationships with environmental variables. From these studies we determined the most frequently tested drivers and extracted relationships that are most related to each of the HW’s behavioral modes considered here (feeding, breeding, resting, and migrating). Information provided in this review allows for a more comprehensive understanding on how these drivers determine HW behavior across regions and populations. It also provides important information for modeling HW movements.
Bathymetry and distance to shore were consistently determined throughout the literature as the most important variables for HW breeding in both hemispheres (e.g., Ersts and Rosenbaum, 2003; Félix and Botero-Acosta, 2011; Garrigue et al., 2011) with nursing groups found in shallower waters close to shore with gentle bed slopes (Whitehead and Moore, 1982; Mignucci-Giannoni, 1998; Oviedo and Solís, 2008; Cartwright et al., 2012; Craig et al., 2014). Preferred values for SST were less important than bathymetry in areas ranging from subtropical to tropical waters (19–28°C) (Rasmussen et al., 2012; Tardin et al., 2019). The role of weather-related variables such as SSH and sea state were not often investigated and further studies on these variables are recommended (Chou et al., 2020).
While resting areas have some similarities with breeding areas (bathymetry range, proximity to coast, reduced wind and wave forces, and degree of bed slope) questions remain if preferred conditions for resting areas are similar throughout all populations and whether HWs can shift their resting locations without compromising their energy budgets. There is evidence that resting areas in semi-enclosed bays facing the migration stream are used by population sub-groups and for short (days) or long (weeks) periods of time (Franklin, 2014).
Research in feeding areas has revealed a preference for strong gradients of temperature and currents (frontal zones) (Hamazaki, 2002; Bassoi et al., 2019). Environments with high chlorophyll concentration and steeper bed slopes were further preferred while feeding (Laidre et al., 2010; Santora et al., 2010; Friedlaender et al., 2011). High prey concentrations are often associated with these conditions (Schweigert et al., 2013) and other cetaceans have been observed feeding in regions with similar conditions (Griffin, 1999; Gannier and Praca, 2007). The successful use of chlorophyll concentration as a predictor variable for feeding varies spatially and relies on an interpretation of the complex relationship and lagged effect with HW prey at multiple trophic levels, as previously mentioned.
Only a few studies tested relationships with salinity and these generally suggested a preference for more saline waters during feeding (Smith et al., 1986; Gregr and Trites, 2001; Tynan et al., 2005; Dalla Rosa et al., 2012; Dransfield et al., 2014). This may be explained by the presence of cold, saline, nutrient-rich water rising to the surface during upwelling, and increasing productivity (Fiedler et al., 1998; Calambokidis and Barlow, 2004; Thompson et al., 2012). Cetaceans are believed to sense salt concentration through taste (Feng et al., 2014) and may be able to use it as a cue when searching for food (Bouchard et al., 2019). Opportunistic feeding has also been documented during migration (Stockin and Burgess, 2005; Danilewicz et al., 2008), indicating that certain environmental cues, such as high levels of DMS, can lead to feeding events (Bouchard et al., 2019). The importance of drivers is highly dependent on prey preferences and reflects the regional differences of HW populations. However, further research into hydrodynamics and biogeochemical processes can provide some of the missing links (e.g., time lag effects) to predict feeding events (Fiedler et al., 1998; Calambokidis and Barlow, 2004; Thompson et al., 2012). Such research will also assist in linking prey dynamics with fine-scale responses of HWs to environmental conditions (Tulloch et al., 2019; Meynecke et al., 2020).
The majority of studies reviewed here have focused on feeding and breeding areas, while migration and resting received less attention. However, HWs spend up to half of their life migrating (Dawbin, 1966), and many migration routes pass alongside highly developed coastlines risking negative human interaction. The cues or triggers for migration are not well understood and it remains speculative as to whether environmental factors drive HWs to leave feeding and breeding grounds at a particular time. It will likely be a combination of environmental triggers, physiology and behavior (social and learned). Other species such as blue whales showed earlier arrival in feeding grounds related to colder sea surface temperature anomalies from the previous season that correlated with greater krill biomass the following year (Szesciorka et al., 2020).
It is worth further investigating the importance of distinct oceanographic features (e.g., canyons and seamounts), fine scale oceanographic processes and the soundscape to determine relevant environmental drivers or human impacts on migration behavior. As technology advances, data of known relevant drivers including SST, salinity and chlorophyll will become available in higher resolution for open ocean environments which in turn will allow for more fine scale analyses.
The role of magnetic and gravitational field variables in combination with the position of the sun has shown limited evidence for determining migration routes but deserves further attention (Horton et al., 2011, 2020). As shown in other migrating animals, the main course and direction are likely a learned behavior (maternally directed site fidelity). However, diversions from this learned behavior have also been documented frequently and will be more evident in the future through automated fluke matching (Felix et al., 2020).
Challenges and Future Directions
As outlined in this review, a multifaceted approach will provide the best understanding of the relationships between HWs and a suite of environmental drivers. This includes the use of multi-species studies which are suitable to provide an overview of feeding aggregations, and help to define large-scale patterns and regions of high cetacean abundance (Ingman et al., 2021) but are limited in terms of extracting relevant relationships at the species level. The often very-detailed satellite tagging studies help with specific preferences of cohorts and individuals and give insights into unknowns, e.g., navigation during migration or maximum depths for feeding as well as fine scale habitat preferences. The less common studies on strandings and environmental drivers showing relationships of wind patterns and cooler waters with higher number of strandings can give insights into long-term trends of migration patterns in coastal waters (Evans et al., 2005; Meager and Limpus, 2014; Meynecke and Meager, 2016).
Our review identified that preferred conditions vary between populations (e.g., due to different prey preferences, varying temperature preferences for breeding areas). Other factors such as learned behavior may play an important role and can or could be more relevant in habitat selection than environmental drivers (Barendse et al., 2013). The importance of early experience and maternal influence on the return of HWs to traditional feeding grounds have been documented through individual return rates and population genetics (Baker et al., 2013; Whitehead and Rendell, 2021). Hence, HWs might not disperse to areas with suitable environmental conditions that may have been erased from the cultural memory of individuals due to whaling (Hauser et al., 2010) or that may be too remote or are emerging. Disentangling the effect of learned behavior from the effect of environmental drivers is challenging but may be achieved in future studies through fluke matching and individual records of thousands of HWs. Promising platforms using A.I. to train algorithms (e.g., Flukebook and Happy Whale) and dataset assembly through citizen science open new possibilities in this field of research. Further genetic (Apprill et al., 2014; Schmitt et al., 2014), isotope (Fleming et al., 2016) and fatty acid (Groß et al., 2020) studies related to environmental drivers will add to a better understanding of environmental drivers and relationships with HWs. Further testing and use of SDMs based on machine learning will improve predictive capacity and allow for constant as well as fast-changing variables over time to be included. However, a careful fitting and validation is important and requires extensive datasets (Reisinger et al., 2021).
Improving predictive capacity is particularly relevant for understanding the role of human impacts that might change HW behavior, forcing them to move to alternative, potentially less suitable, habitats (Corkeron, 1995) or altering recovery rates of populations. An area suitable for breeding, feeding or migration may be avoided or is degraded due to vessel traffic (Guzman et al., 2013), noise pollution (Au and Green, 2000; Laist et al., 2001; Weilgart, 2007), dredging (Todd et al., 2015), fishing (Gribble et al., 1998; Clapham and Mead, 1999), chemical inputs (Remili et al., 2020) and climate change (Jackson et al., 2001; Chilvers et al., 2005). These kinds of impacts need to be considered when undertaking habitat suitability studies or studies investigating predictive capacity of environmental drivers. A good understanding of the influence of environmental drivers is required to enable modeling of future impacts including climate change (Figure 1). Considering the variabilities that the marine ecosystem is currently subjected to and other factors as the HW populations recovery, such modeling is complex.
Disentangling the natural variability of distribution patterns from climate change is not straightforward. Combining long-term data sets spanning several decades and big data set analyses will make this more feasible in the future. Although there is some recognition of the impacts of climate change on HWs (e.g., Ramp et al., 2015), there are many knowledge gaps in the influence of climate variability on HW feeding, breeding and migration distributions. Given the temperature tolerance for HWs, small changes in SST due to climate change are likely not going to have major impacts on breeding grounds as long as suitable habitats below 28°C are accessible (Derville et al., 2019). Significant changes in ocean circulation patterns and sea ice are predicted as a result of climate change in feeding areas, which may result in changes to the timing of prey availability as well as the size, density and locations of important foraging areas (Nicol et al., 2000, 2008; Ramp et al., 2015). In the rapidly warming Western Antarctica Peninsula, there is evidence that krill are being replaced by salps, which are not a suitable diet for whales (Plum et al., 2020). Less predictable occurrence of prey and reduced densities would increase the time and energy cost of feeding. A possible adaptive response could be feeding outside traditional feeding grounds (Findlay et al., 2017) or reducing length and time of migration by shifting calving grounds closer to feeding grounds (Torre-Williams et al., 2019). A separate review of HW relationships with prey for different regions is recommended to provide a more in-depth overview of available studies.
Most of the responses of HWs to climate change are still unknown. In order to tackle the complex impacts of climate change on environmental variables and consequently on HWs, a multi- and transdisciplinary research approach is needed (Meynecke et al., 2020). With new time series of previously unavailable or limited data for environmental drivers (such as data from autonomous devices), we are also likely to see an increase of new variables in the future studies. It raises the question of whether environmental drivers have been selected because they were the most likely to relate with a HW’s behavioral mode or because they were the most accessible and promising at the time. Some variables included here (e.g., DMS proxies for feeding areas) are still undertested and need to be further investigated regarding their influence on the distribution of the species populations and/or on the species behavior in specific regions. Other environmental drivers that will hopefully be subject to increased research in the future include nutrient and links to food abundance in the ice-covered ocean (Meyer et al., 2020) given the anticipated changes in polar regions (Turner and Marshall, 2011).
Conclusion
Determining the type and strength of relationships between environmental drivers and HWs continues to be of great relevance. Despite increasing research in this field over recent years, a number of unknowns remain in terms of both the physical and biological domains of this inter-disciplinary issue. However, increasing availability of multi-variate data streams, and analytical advancements for parametrization of models and in particular for predicting anthropogenic impacts have started to provide much-needed contexts for comprehensive assessments of environmental drivers. The findings highlighted in this review can provide the basis for future research by addressing the identified gaps. Determining environmental conditions that increase the risk of entanglements (Santora et al., 2020), vessel strikes (Redfern et al., 2017; Smith et al., 2020a), noise pollution (Erbe et al., 2019), impacts from whale tourism (Sprogis et al., 2020), climate change impacts (Meynecke et al., 2020), identifying where new habitats could arise and when currently used habitats might become unsuitable, would enable better protection of this iconic species. Elevated protection of current and future critical habitat and a plan for flexible protection zones are needed in light of the many challenges ahead.
Author Contributions
J-OM, JdB, and J-LB contributed to conception and design of the study and organized the database. JdB and ES wrote sections of the manuscript. BM, KF, MV, AR, and J-OM contributed funding. All authors contributed to manuscript revision, read, and approved the submitted version.
Funding
This work was supported by a grant to Griffith University from a private charitable trust as part of the Whales & Climate Research Program.
Conflict of Interest
The authors declare that the research was conducted in the absence of any commercial or financial relationships that could be construed as a potential conflict of interest.
Publisher’s Note
All claims expressed in this article are solely those of the authors and do not necessarily represent those of their affiliated organizations, or those of the publisher, the editors and the reviewers. Any product that may be evaluated in this article, or claim that may be made by its manufacturer, is not guaranteed or endorsed by the publisher.
Acknowledgments
The authors like to thank the many colleagues who contributed to the ongoing discussion of this research.
Supplementary Material
The Supplementary Material for this article can be found online at: https://www.frontiersin.org/articles/10.3389/fmars.2021.720774/full#supplementary-material
Footnote
References
Abrahms, B., Welch, H., Brodie, S., Jacox, M. G., Becker, E. A., Bograd, S. J., et al. (2019). Dynamic ensemble models to predict distributions and anthropogenic risk exposure for highly mobile species. Divers. Distrib. 25, 1182–1193. doi: 10.1111/ddi.12940
Andrews-Goff, V., Bestley, S., Gales, N. J., Laverick, S. M., Paton, D., Polanowski, A. M., et al. (2018). Humpback whale migrations to Antarctic summer foraging grounds through the southwest Pacific Ocean. Sci. Rep. 8:12333. doi: 10.1038/s41598-018-30748-4
Apprill, A., Robbins, J., Eren, A. M., Pack, A. A., Reveillaud, J., Mattila, D., et al. (2014). Humpback whale populations share a core skin bacterial community: towards a health index for marine mammals? PLoS One 9:e90785. doi: 10.1371/journal.pone.0090785
Atkinson, A., Siegel, V., Pakhomov, E. A., Rothery, P., Loeb, V., Ross, R. M., et al. (2008). Oceanic circumpolar habitats of Antarctic krill. Mar. Ecol. Prog. Ser. 362, 1–23. doi: 10.3354/meps07498
Au, W., and Green, M. (2000). Acoustic interaction of humpback whales and whale-watching boats. Mar. Environ. Res. 49, 469–481. doi: 10.1016/s0141-1136(99)00086-0
Avila, I. C., Dormann, C. F., García, C., Payán, L. F., and Zorrilla, M. X. (2019). Humpback whales extend their stay in a breeding ground in the Tropical Eastern Pacific. ICES J. Mar. Sci. 77, 109–118. doi: 10.1093/icesjms/fsz251
Azzellino, A., Fossi, M. C., Gaspari, S., Lanfredi, C., Lauriano, G., Marsili, L., et al. (2014). An index based on the biodiversity of cetacean species to assess the environmental status of marine ecosystems. Mar. Environ. Res. 100, 94–111. doi: 10.1016/j.marenvres.2014.06.003
Baker, C. S., and Herman, L. M. (1981). Migration and local movement of humpback whales (Megaptera novaeangliae) through Hawaiian waters. Can. J. Zool. 59, 460–469. doi: 10.1139/z81-067
Baker, C. S., and Herman, L. M. (1987). Alternative population estimates of humpback whales (Megaptera-Novaeangliae) in Hawaiian Waters. Can. J. Zool. Revue Can. Zool. 65, 2818–2821. doi: 10.1139/z87-426
Baker, C. S., Herman, L. M., Perry, A., Lawton, W. S., Straley, J. M., and Straley, J. H. (1985). Population characteristics and migration of summer and late-season humpback whales (Megaptera-Novaeangliae) in Southeastern Alaska. Mar. Mamm. Sci. 9, 304–323 doi: 10.1111/j.1748-7692.1985.tb00018.x
Baker, C. S., Palumbi, S. R., Lambertsen, R. H., Weinrich, M. T., Calambokidis, J., and Obrien, S. J. (1990). Influence of seasonal migration on geographic-distribution of mitochondrial-DNA haplotypes in humpback whales. Nature 344, 238–240. doi: 10.1038/344238a0
Baker, C. S., Steel, D., Calambokidis, J., Falcone, E., Gonz?Lez-Peral, U., Barlow, J., et al. (2013). Strong maternal fidelity and natal philopatry shape genetic structure in North Pacific humpback whales. Mar. Ecol. Prog. Ser. 494, 291–306. doi: 10.3354/meps10508
Barendse, J., Best, P. B., Carvalho, I., and Pomilla, C. (2013). Mother knows best: occurrence and associations of resighted humpback whales suggest maternally derived fidelity to a southern hemisphere coastal feeding ground. PLoS One 8:e81238. doi: 10.1371/journal.pone.0081238
Barendse, J., Best, P. B., Thornton, M., Pomilla, C., Carvalho, I., and Rosenbaum, H. C. (2010). Migration redefined? Seasonality, movements and group composition of humpback whales Megaptera novaeangliae off the west coast of South Africa. Afr. J. Mar. Sci. 32, 1–22.
Barlow, D. R., Fournet, M., and Sharpe, F. (2019). Incorporating tides into the acoustic ecology of humpback whales. Mar. Mamm. Sci. 35, 234–251. doi: 10.1111/mms.12534
Bassoi, M., Acevedo, J., Secchi, E. R., Aguayo-Lobo, A., Dalla Rosa, L., Torres, D., et al. (2019). Cetacean distribution in relation to environmental parameters between Drake Passage and northern Antarctic Peninsula. Polar Biol. 43, 1–15. doi: 10.1007/s00300-019-02607-z
Beaugrand, G., and Kirby, R. R. (2016). Quasi-deterministic responses of marine species to climate change. Clim. Res. 69, 117–128. doi: 10.3354/cr01398
Becker, E. A., Carretta, J. V., Forney, K. A., Barlow, J., Brodie, S., Hoopes, R., et al. (2020). Performance evaluation of cetacean species distribution models developed using generalized additive models and boosted regression trees. Ecol. Evol. 10, 5759–5784. doi: 10.1002/ece3.6316
Becker, E. A., Forney, K. A., Redfern, J. V., Barlow, J., Jacox, M. G., Roberts, J. J., et al. (2019). Predicting cetacean abundance and distribution in a changing climate. Divers. Distrib. 25, 626–643. doi: 10.1111/ddi.12867
Becker, E., Forney, K., Ferguson, M., Foley, D., Smith, R., Barlow, J., et al. (2010). Comparing California Current cetacean–habitat models developed using in situ and remotely sensed sea surface temperature data. Mar. Ecol. Prog. Ser. 413, 163–183. doi: 10.3354/meps08696
Bejder, L., Videsen, S., Hermannsen, L., Simon, M., Hanf, D., and Madsen, P. T. (2019). Low energy expenditure and resting behaviour of humpback whale mother-calf pairs highlights conservation importance of sheltered breeding areas. Sci. Rep. 9:771. doi: 10.1038/s41598-018-36870-7
Benson, S. R., Croll, D. A., Marinovic, B. B., Chavez, F. P., and Harvey, J. T. (2002). Changes in the cetacean assemblage of a coastal upwelling ecosystem during El Niño 1997–98 and La Niña 1999. Prog. Oceanogr. 54, 279–291.
Best, P. B., and Ross, G. J. B. (1996). Whale observations from the Knysna Heads, 1903–1906. South Afr. J. Mar. Sci. 17, 305–308. doi: 10.2989/025776196784158626
Best, P. B., Sekiguchi, K., and Findlay, K. P. (1995). A suspended migration of humpback whales Megaptera novaeangliae on the west coast of South Africa. Mar. Ecol. Prog. Ser. Oldendorf 118, 1–12. doi: 10.3354/meps118001
Bestley, S., Andrews-Goff, V., Van Wijk, E., Rintoul, S. R., Double, M. C., and How, J. (2019). New insights into prime Southern Ocean forage grounds for thriving Western Australian humpback whales. Sci. Rep. 9:13988. doi: 10.1038/s41598-019-50497-2
Bettridge, S. O. M., Baker, C. S., Barlow, J., Clapham, P., Ford, M. J., Gouveia, D., et al. (2015). Status Review of the Humpback Whale (Megaptera novaeangliae) Under the Endangered Species Act. NOAA-TM-NMFS-SWFSC-540. Washington, DC: National Oceanic and Atmospheric Administration.
Bolin, J. A., Schoeman, D. S., Pizà-Roca, C., and Scales, K. L. (2019). A current affair: entanglement of humpback whales in coastal shark-control nets. Rem. Sens. Ecol. Conserv. 6, 1–10.
Bombosch, A., Zitterbart, D. P., Van Opzeeland, I., Frickenhaus, S., Burkhardt, E., Wisz, M. S., et al. (2014). Predictive habitat modelling of humpback (Megaptera novaeangliae) and Antarctic minke (Balaenoptera bonaerensis) whales in the Southern Ocean as a planning tool for seismic surveys. Deep Sea Res. Part I Oceanogr. Res. Pap. 91, 101–114. doi: 10.1016/j.dsr.2014.05.017
Bouchard, B., Barnagaud, J.-Y., Poupard, M., Glotin, H., Gauffier, P., Torres Ortiz, S., et al. (2019). Behavioural responses of humpback whales to food-related chemical stimuli. PLoS One 14:e0212515. doi: 10.1371/journal.pone.0212515
Brodie, P. F. (1975). Cetacean energetics, an overview of intrespecific size variation. Ecology 56, 152–161. doi: 10.2307/1935307
Brown, M. R., Corkeron, P. J., Hale, P. T., Schultz, K. W., and Bryden, M. (1995). Evidence for a sex-segregated migration in the humpback whale (Megaptera novaeangliae). Proc. R. Soc. Lond. Ser. B Biol. Sci. 259, 229–234. doi: 10.1098/rspb.1995.0034
Bruce, E., Albright, L., Sheehan, S., and Blewitt, M. (2014). Distribution patterns of migrating humpback whales (Megaptera novaeangliae) in Jervis Bay, Australia: a spatial analysis using geographical citizen science data. Applied Geography 54, 83–95. doi: 10.1016/j.apgeog.2014.06.014
Burrows, J. A., Harvey, J. T., Newton, K. M., Croll, D. A., and Benson, S. R. (2012). Marine mammal response to interannual variability in Monterey Bay, California. Mar. Ecol. Prog. Ser. 461, 257–271. doi: 10.3354/meps09712
Burrows, J., Johnston, D., Straley, J., Chenoweth, E., Ware, C., Curtice, C., et al. (2016). Prey density and depth affect the fine-scale foraging behavior of humpback whales Megaptera novaeangliae in Sitka Sound, Alaska, USA. Mar. Ecol. Prog. Ser. 561, 245–260. doi: 10.3354/meps11906
Burton, C. (2001). Historical and recent distribution of humpback whales in Shark Bay, Western Australia. Mem. Qld. Museum 47, 599–612.
Cade, D. E., Seakamela, S. M., Findlay, K. P., Fukunaga, J., Kahane-Rapport, S. R., Warren, J. D., et al. (2021). Predator-scale spatial analysis of intra-patch prey distribution reveals the energetic drivers of rorqual whale super-group formation. Funct. Ecol. 35, 894–908. doi: 10.1111/1365-2435.13763
Calambokidis, J., and Barlow, J. (2004). Abundance of blue and humpback whales in the eastern North Pacific estimated by capture-recapture and line-transect methods. Mar. Mamm. Sci. 20, 63–85. doi: 10.1111/j.1748-7692.2004.tb01141.x
Calambokidis, J., Fahlbusch, J. A., Szesciorka, A. R., Southall, B. L., Cade, D. E., Friedlaender, A. S., et al. (2019). Differential vulnerability to ship strikes between day and night for blue, fin, and humpback whales based on dive and movement data from medium duration archival tags. Front. Mar. Sci. 6:543. doi: 10.3389/fmars.2019.00543
Calambokidis, J., Steiger, G. H., Ellifrit, D. K., Troutman, B. L., and Bowlby, C. E. (2004). Distribution and abundance of humpback whales (Megaptera novaeangliae) and other marine mammals off the northern Washington coast. Fish. Bull. 102, 563–580.
Cartwright, R., Gillespie, B., Labonte, K., Mangold, T., Venema, A., Eden, K., et al. (2012). Between a rock and a hard place: habitat selection in female-calf humpback whale (Megaptera novaeangliae) pairs on the Hawaiian breeding grounds. PLoS One 7:e38004. doi: 10.1371/journal.pone.0038004
Cartwright, R., Venema, A., Hernandez, V., Wyels, C., Cesere, J., and Cesere, D. (2019). Fluctuating reproductive rates in Hawaii’s humpback whales, Megaptera novaeangliae, reflect recent climate anomalies in the North Pacific. R. Soc. Open Sci. 6:181463. doi: 10.1098/rsos.181463
Chenoweth, E. M., Gabriele, C. M., and Hill, D. F. (2011). Tidal influences on humpback whale habitat selection near headlands. Mar. Ecol. Prog. Ser. 423, 279–289. doi: 10.3354/meps08891
Chilvers, B. L., Lawler, I. R., Macknight, F., Marsh, H., Noad, M., and Paterson, R. (2005). Moreton Bay, Queensland, Australia: an example of the co-existence of significant marine mammal populations and large-scale coastal development. Biol. Conserv. 122, 559–571. doi: 10.1016/j.biocon.2004.08.013
Chittleborough, R. (1958). The breeding cycle of the female humpback whale, Megaptera nodosa (Bonnaterre). Mar. Freshw. Res. 9, 1–18. doi: 10.1071/mf9580001
Chittleborough, R. G. (1965). Dynamics of Two Populations of the Humpback Whale, Megaptera novaeangliae (Borowsk). Aust. J. Mar. Freshw. Res. 16, 33–128. doi: 10.1071/mf9650033
Chou, E., Kershaw, F., Maxwell, S. M., Collins, T., Strindberg, S., and Rosenbaum, H. C. (2020). Distribution of breeding humpback whale habitats and overlap with cumulative anthropogenic impacts in the Eastern Tropical Atlantic. Divers. Distrib. 26, 549–564. doi: 10.1111/ddi.13033
Clapham, P. J. (1992). Age at attainment of sexual maturity in humpback whales, Megaptera novaeangliae. Can. J. Zool. 70, 1470–1472. doi: 10.1139/z92-202
Clapham, P. J. (1996). The social and reproductive biology of Humpback Whales: an ecological perspective. Mamm. Rev. 26, 27–49. doi: 10.1111/j.1365-2907.1996.tb00145.x
Clapham, P. J. (2000). “Cetacean societies Ill,” in The Humpback Whale, eds J. C. Mann, R. C. Connor, P. L. Tyack, and H. Whitehead (Chicago, IL: University of Chicago Press). doi: 10.1098/rsif.2015.0676
Clapham, P. J., and Mead, J. (1999). Megaptera novaeangliae. Mamm. Species 604, 1–9. doi: 10.2307/3504352
Clapham, P. J., and Palsboll, P. J. (1997). Molecular analysis of paternity shows promiscuous mating in female humpback whales (Megaptera novaeangliae, Borowski). Proc. Biol. Sci. 264, 95–98. doi: 10.1098/rspb.1997.0014
Corkeron, P. J. (1995). Humpback whales (Megaptera-Novaeangliae) in Hervey Bay, Queensland – behavior and responses to whale-watching vessels. Can. J. Zool. Revue Can. Zool. 73, 1290–1299. doi: 10.1139/z95-153
Corkeron, P. J., and Connor, R. C. (1999). Why do baleen whales migrate? Mar. Mamm. Sci. 15:1228. doi: 10.1111/j.1365-294X.2008.04069.x
Craig, A. S., and Herman, L. M. (1997). Sex differences in site fidelity and migration of humpback whales (Megaptera novaeangliae) to the Hawaiian Islands. Can. J. Zool. 75, 1923–1933. doi: 10.1139/z97-822
Craig, A. S., Herman, L. M., Gabriele, C. M., and Pack, A. A. (2003). Migratory timing of humpback whales (Megaptera novaeangliae) in the central North Pacific varies with age, sex and reproductive status. Behaviour 140, 981–1001. doi: 10.1163/156853903322589605
Craig, A. S., Herman, L. M., Pack, A. A., and Waterman, J. O. (2014). Habitat segregation by female humpback whales in Hawaiian waters: avoidance of males? Behaviour 151, 613–631. doi: 10.1163/1568539x-00003151
Curtice, C., Johnston, D. W., Ducklow, H., Gales, N., Halpin, P. N., and Friedlaender, A. S. (2015). Modeling the spatial and temporal dynamics of foraging movements of humpback whales (Megaptera novaeangliae) in the Western Antarctic Peninsula. Mov. Ecol. 3:13. doi: 10.1186/s40462-015-0041-x
Dalla Rosa, L., Ford, J. K., and Trites, A. W. (2012). Distribution and relative abundance of humpback whales in relation to environmental variables in coastal British Columbia and adjacent waters. Continent. Shelf Res. 36, 89–104. doi: 10.1016/j.csr.2012.01.017
Danilewicz, D., Tavares, M., Moreno, I. B., Ott, P. H., and Trigo, C. C. (2008). Evidence of feeding by the humpback whale (Megaptera novaeangliae) in mid-latitude waters of the western South Atlantic. JMBA2 Biodiv. Records 2:e88.
Dawbin, W. H. (1966). The Seasonal Migratory Cycle of Humpback Whales. Berkeley, CA: University of California Press.
De Rock, P., Elwen, S. H., Roux, J., Leeney, R., James, B., Visser, V., et al. (2019). Predicting large-scale habitat suitability for cetaceans off Namibia using MinxEnt. Mar. Ecol. Prog. Ser. 619, 149–167. doi: 10.3354/meps12934
Derville, S., Torres, L. G., Albertson, R., Andrews, O., Baker, C. S., Carzon, P., et al. (2019). Whales in warming water: assessing breeding habitat diversity and adaptability in Oceania’s changing climate. Glob. Change Biol. 25, 1466–1481. doi: 10.1111/gcb.14563
Derville, S., Torres, L. G., Iovan, C., and Garrigue, C. (2018). Finding the right fit: comparative cetacean distribution models using multiple data sources and statistical approaches. Divers. Distrib. 24, 1657–1673. doi: 10.1111/ddi.12782
Derville, S., Torres, L. G., Zerbini, A. N., Oremus, M., and Garrigue, C. (2020). Horizontal and vertical movements of humpback whales inform the use of critical pelagic habitats in the western South Pacific. Sci. Rep. 10:4871. doi: 10.1038/s41598-020-61771-z
Digby, P. G. N., and Kempton, R. A. (2012). Multivariate Analysis of Ecological Communities. Dordrecht: Springer.
Doniol-Valcroze, T., Berteaux, D., Larouche, P., and Sears, R. (2007). Influence of thermal fronts on habitat selection by four rorqual whale species in the Gulf of St. Lawrence. Mar. Ecol. Prog. Ser. 335, 207–216. doi: 10.3354/meps335207
Dransfield, A., Hines, E., Mcgowan, J., Holzman, B., Nur, N., Elliott, M., et al. (2014). Where the whales are: using habitat modeling to support changes in shipping regulations within National Marine Sanctuaries in Central California. Endanger. Species Res. 26, 39–57. doi: 10.3354/esr00627
Dulau, V., Pinet, P., Geyer, Y., Fayan, J., Mongin, P., Cottarel, G., et al. (2017). Continuous movement behavior of humpback whales during the breeding season in the southwest Indian Ocean: on the road again! Mov. Ecol. 5:11. doi: 10.1186/s40462-017-0101-5
Elith, J., Leathwick, J. R., and Hastie, T. (2008). A working guide to boosted regression trees. J. Anim. Ecol. 77, 802–813. doi: 10.1111/j.1365-2656.2008.01390.x
Erbe, C., Marley, S. A., Schoeman, R. P., Smith, J. N., Trigg, L. E., and Embling, C. B. (2019). The effects of ship noise on marine mammals—a review. Front. Mar. Sci. 6:606. doi: 10.3389/fmars.2019.00606
Ersts, P. J., and Rosenbaum, H. C. (2003). Habitat preference reflects social organization of humpback whales (Megaptera novaeangliae) on a wintering ground. J. Zool. 260, 337–345. doi: 10.1017/s0952836903003807
Evans, K., Thresher, R., Warneke, R., Bradshaw, C. J., Pook, M., Thiele, D., et al. (2005). Periodic variability in cetacean strandings: links to large-scale climate events. Biol. Lett. 1, 147–150. doi: 10.1098/rsbl.2005.0313
Felix, F., Abras, D. R., Cheeseman, T., Haase, B., Santos, J. D., Marcondes, M. C. C., et al. (2020). A new case of interoceanic movement of a humpback whale in the southern hemisphere: the El nino link. Aquat. Mamm. 46:578+. doi: 10.1578/am.46.6.2020.578
Félix, F., and Botero-Acosta, N. (2011). Distribution and behaviour of humpback whale mother–calf pairs during the breeding season off Ecuador. Mar. Ecol. Prog. Ser. 426, 277–287. doi: 10.3354/meps08984
Feng, P., Zheng, J., Rossiter, S. J., Wang, D., and Zhao, H. (2014). Massive losses of taste receptor genes in toothed and baleen whales. Genome Biol. Evol. 6, 1254–1265. doi: 10.1093/gbe/evu095
Fiedler, P. C., Redfern, J. V., Forney, K. A., Palacios, D. M., Sheredy, C., Rasmussen, K., et al. (2018). Prediction of large whale distributions: a comparison of presence–absence and presence-only modeling techniques. Front. Mar. Sci. 5:419. doi: 10.3389/fmars.2018.00419
Fiedler, P. C., Reilly, S. B., Hewitt, R. P., Demer, D., Philbrick, V. A., Smith, S., et al. (1998). Blue whale habitat and prey in the California Channel Islands. Deep Sea Res. Part II Top. Stud. Oceanogr. 45, 1781–1801. doi: 10.1038/s41598-019-52792-4
Findlay, K. P. (2001). A review of humpback whale catches by modern whaling operationsin the Southern Hemisphere. Mem. Qld. Museum 47, 411–420.
Findlay, K. P., and Best, P. B. (1996). Estimates of the numbers of humpback whales observed migrating past Cape Vidal, South Africa, 1988-1991. Mar. Mamm. Sci. 12, 354–370. doi: 10.1111/j.1748-7692.1996.tb00589.x
Findlay, K. P., Best, P. B., and Meÿer, M. A. (2011). Migrations of humpback whales past Cape Vidal, South Africa, and an estimate of the population increase rate (1988–2002). Afr. J. Mar. Sci. 33, 375–392. doi: 10.2989/1814232x.2011.637345
Findlay, K. P., Seakamela, S. M., Meyer, M. A., Kirkman, S. P., Barendse, J., Cade, D. E., et al. (2017). Humpback whale “super-groups”–A novel low-latitude feeding behaviour of Southern Hemisphere humpback whales (Megaptera novaeangliae) in the Benguela Upwelling System. PLoS One 12:e0172002. doi: 10.1371/journal.pone.0172002
Fleming, A. H., Clark, C. T., Calambokidis, J., and Barlow, J. (2016). Humpback whale diets respond to variance in ocean climate and ecosystem conditions in the California Current. Glob. Change Biol. 22, 1214–1224. doi: 10.1111/gcb.13171
Flores, H., Atkinson, A., Kawaguchi, S., Krafft, B. A., Milinevsky, G., Nicol, S., et al. (2012). Impact of climate change on Antarctic krill. Mar. Ecol. Prog. Ser. 458, 1–19.
Fournet, M. E. H., Matthews, L. P., Gabriele, C. M., Haver, S., Mellinger, D. K., and Klinck, H. (2018). Humpback whales Megaptera novaeangliae alter calling behavior in response to natural sounds and vessel noise. Mar. Ecol. Prog. Ser. 607, 251–268. doi: 10.3354/meps12784
Franklin, T., Franklin, W., Brooks, L., and Harrison, P. (2017). Site-specific female-biased sex ratio of humpback whales (Megaptera novaeangliae) during a stopover early in the southern migration. Can. J. Zool. 96, 533–544. doi: 10.1139/cjz-2017-0086
Franklin, T., Franklin, W., Brooks, L., Harrison, P., Baverstock, P., and Clapham, P. (2011). Seasonal changes in pod characteristics of eastern Australian humpback whales (Megaptera novaeangliae), Hervey Bay 1992–2005. Mar. Mamm. Sci. 27, E134–E152.
Franklin, W. (2014). Abundance, Population Dynamics, Reproduction, Rates of Population Increase and Migration Linkages of Eastern Australian Humpback Whales (Megaptera novaeangliae) Utilising Hervey Bay, Queensland. Ph.D. thesis. Lismore, NSW: Southern Cross University.
Freer, J. J., Partridge, J. C., Tarling, G. A., Collins, M. A., and Genner, M. J. (2017). Predicting ecological responses in a changing ocean: the effects of future climate uncertainty. Mar. Biol. 165:7. doi: 10.1007/s00227-017-3239-1
Friedlaender, A., Bocconcelli, A., Wiley, D., Cholewiak, D., Ware, C., Weinrich, M., et al. (2011). Underwater components of humpback whale bubble-net feeding behaviour. Behaviour 148, 575–602. doi: 10.1163/000579511x570893
Gannier, A., and Praca, E. (2007). SST fronts and the summer sperm whale distribution in the north-west Mediterranean Sea. J. Mar. Biol. Assoc. U. K. 87, 187–193. doi: 10.1017/s0025315407054689
García-Morales, R., Pérez-Lezama, E. L., and Shirasago-Germán, B. (2017). Influence of environmental variability on distribution and relative abundance of baleen whales (suborder Mysticeti) in the Gulf of California. Mar. Ecol. 38:e12479. doi: 10.1111/maec.12479
Garrigue, C., Baker, S., Constantine, R., Poole, M., Hauser, N., Clapham, P., et al. (2007). “Interchange of humpback whales in Oceania (South Pacific), 1999 to 2004,” in Paper Presented at the Scientific Committee of the International Whaling Commission, Anchorage, AK.
Garrigue, C., Clapham, P. J., Geyer, Y., Kennedy, A. S., and Zerbini, A. N. (2015). Satellite tracking reveals novel migratory patterns and the importance of seamounts for endangered South Pacific humpback whales. R. Soc. Open Sci. 2:150489. doi: 10.1098/rsos.150489
Garrigue, C., Derville, S., Bonneville, C., Baker, C. S., Cheeseman, T., Millet, L., et al. (2020). Searching for humpback whales in a historical whaling hotspot of the Coral Sea, South Pacific. Endanger. Species Res. 42, 67–82. doi: 10.3354/esr01038
Garrigue, C., Forestell, P. H., Greaves, J., Gill, P., Naessig, P. J., Patenaude, N. M., et al. (2000). Migratory movements of humpback whales (Megaptera nevaeangliae) between New Caledonia, East Australia and New Zealand. J. Cetacean Res. Manage. 2, 111–115.
Garrigue, C., Franklin, T., Constantine, R., Russel, K., Burns, D., Poole, M., et al. (2011). First assessment of interchange of humpback whales between Oceania and the East coast of Australia. J. Cetacean Res. Manage. Special Issue 3, 269–274. doi: 10.47536/jcrm.vi.314
Gavrilchuk, K., Lesage, V., Ramp, C., Sears, R., Bérubé, M., Bearhop, S., et al. (2014). Trophic niche partitioning among sympatric baleen whale species following the collapse of groundfish stocks in the Northwest Atlantic. Mar. Ecol. Prog. Ser. 497, 285–301. doi: 10.3354/meps10578
Goldbogen, J. A., Calambokidis, J., Croll, D. A., Harvey, J. T., Newton, K. M., Oleson, E. M., et al. (2008). Foraging behavior of humpback whales: kinematic and respiratory patterns suggest a high cost for a lunge. J. Exp. Biol. 211, 3712–3719. doi: 10.1242/jeb.023366
Gregr, E. J., and Trites, A. W. (2001). Predictions of critical habitat for five whale species in the waters of coastal British Columbia. Can. J. Fish. Aquat. Sci. 58, 1265–1285. doi: 10.1139/f01-078
Gribble, N. A., Mcpherson, G., and Lane, B. (1998). Effect of the Queensland Shark Control Program on non-target species: whale, dugong, turtle and dolphin: a review. Mar. Freshw. Res. 49, 645–651. doi: 10.1071/mf97053
Griffin, R. B. (1999). Sperm Whale Distributions and Community ecology associated with a warmcore ring off Georges Bank. Mar. Mamm. Sci. 15, 33–51. doi: 10.1111/j.1748-7692.1999.tb00780.x
Groß, J., Virtue, P., Nichols, P. D., Eisenmann, P., Waugh, C. A., and Bengtson Nash, S. (2020). Interannual variability in the lipid and fatty acid profiles of east Australia-migrating humpback whales (Megaptera novaeangliae) across a 10-year timeline. Sci. Rep. 10:18274. doi: 10.1038/s41598-020-75370-5
Guidino, C., Llapapasca, M. A., Silva, S., Alcorta, B., and Pacheco, A. S. (2014). Patterns of spatial and temporal distribution of humpback whales at the Southern Limit of the Southeast Pacific Breeding Area. PLoS One 9:e112627. doi: 10.1371/journal.pone.0112627
Guisan, A., and Zimmermann, N. E. (2000). Predictive habitat distribution models in ecology. Ecol. Model. 135, 147–186. doi: 10.1016/s0304-3800(00)00354-9
Guzman, H. M., Gomez, C. G., Guevara, C. A., and Kleivane, L. (2013). Potential vessel collisions with Southern Hemisphere humpback whales wintering off Pacific Panama. Mar. Mamm. Sci. 29, 629–642.
Hain, J. H. W., Ellis, S. L., Kenney, R. D., Clapham, P. J., Gray, B. K., Weinrich, M. T., et al. (1995). Apparent bottom feeding by humpback-whales on stellwagen bank. Mar. Mamm. Sci. 11, 464–479. doi: 10.1111/j.1748-7692.1995.tb00670.x
Hamazaki, T. (2002). Spatiotemporal prediction models of cetacean habitats in the mid-western North Atlantic Ocean (from Cape Hatteras, North Carolina, USA to Nova Scotia, Canada). Mar. Mamm. Sci. 18, 920–939. doi: 10.1111/j.1748-7692.2002.tb01082.x
Hauser, N., Zerbini, A. N., Geyer, Y., Heide-Jørgensen, M.-P., and Clapham, P. (2010). Movements of satellite-monitored humpback whales, Megaptera novaeangliae, from the cook islands. Mar. Mamm. Sci. 26, 679–685. doi: 10.1111/j.1748-7692.2009.00363.x
Hazen, E. L., Friedlaender, A. S., Thompson, M. A., Ware, C. R., Weinrich, M. T., Halpin, P. N., et al. (2009). Fine-scale prey aggregations and foraging ecology of humpback whales Megaptera novaeangliae. Mar. Ecol. Prog. Ser. 395, 75–89. doi: 10.3354/meps08108
Horton, T. W., Holdaway, R. N., Zerbini, A. N., Hauser, N., Garrigue, C., Andriolo, A., et al. (2011). Straight as an arrow: humpback whales swim constant course tracks during long-distance migration. Biol. Lett. 7, 674–679. doi: 10.1098/rsbl.2011.0279
Horton, T. W., Zerbini, A. N., Andriolo, A., Danilewicz, D., and Sucunza, F. (2020). Multi-Decadal humpback whale migratory route fidelity despite oceanographic and geomagnetic change. Front. Mar. Sci. 7:414. doi: 10.3389/fmars.2020.00414
Ingman, K., Hines, E., Mazzini, P. L. F., Rockwood, R. C., Nur, N., and Jahncke, J. (2021). Modeling changes in baleen whale seasonal abundance, timing of migration, and environmental variables to explain the sudden rise in entanglements in California. PLoS One 16:e0248557. doi: 10.1371/journal.pone.0248557
Irvine, L. G., Thums, M., Hanson, C. E., Mcmahon, C. R., and Hindell, M. A. (2018). Evidence for a widely expanded humpback whale calving range along the Western Australian coast. Mar. Mamm. Sci. 34, 294–310. doi: 10.1111/mms.12456
IWC (1998). Annex G-Report of the sub-committee on the comprehensive assessment of Southern hemisphere humpback whales. Rep. Int. Whal. 48, 170–118.
IWC (2011). Final Report on the Assessment of the Southern Hemisphere Humpback Whale Breeding Stock B. Paper SC/63/Rep 6. Cambridge: International Whaling Commission.
Jackson, J. A., Ross-Gillespie, A., Butterworth, D. S., Findlay, K., Holloway, S., Robbins, J., et al. (2015). Southern Hemisphere Humpback Whale Comprehensive Assessment — A Synthesis and Summary: 2005-2015. Report (SC/66a/SH/3). Cambridge: International Whaling Commission Scientific Committee.
Jackson, R. B., Carpenter, S. R., Dahm, C. N., Mcknight, D. M., Naiman, R. J., Postel, S. L., et al. (2001). Water in a changing world. Ecol. Appl. 11, 1027–1045.
Jenner, K. C., Jenner, M.-N. M., and Mccabe, K. A. (2001). Geographical and temporal movements of humpback whales in western Australian waters. APPEA J. 41, 749–765. doi: 10.1071/aj00044
Johnston, D., Chapla, M., Williams, L., and Mattila, D. (2007). Identification of humpback whale Megaptera novaeangliae wintering habitat in the Northwestern Hawaiian Islands using spatial habitat modeling. Endanger. Species Res. 3, 249–257.
Kaschner, K., Watson, R., Trites, A., and Pauly, D. (2006). Mapping world-wide distributions of marine mammal species using a relative environmental suitability (RES) model. Mar. Ecol. Prog. Series 316, 285–310. doi: 10.3354/meps316285
Keen, E. M. (2017). Aggregative and feeding thresholds of sympatric rorqual whales within a fjord system. Ecosphere 8:e01702. doi: 10.1002/ecs2.1702
Keen, E. M., Wray, J., Pilkington, J. F., Thompson, K. L., and Picard, C. R. (2018). Distinct habitat use strategies of sympatric rorqual whales within a fjord system. Mar. Environ. Res. 140, 180–189. doi: 10.1016/j.marenvres.2018.06.014
Kershaw, J. L., Ramp, C. A., Sears, R., Plourde, S., Brosset, P., Miller, P. J. O., et al. (2021). Declining reproductive success in the Gulf of St. Lawrence’s humpback whales (Megaptera novaeangliae) reflects ecosystem shifts on their feeding grounds. Glob. Change Biol. 27, 1027–1041. doi: 10.1111/gcb.15466
Kosma, M. M., Werth, A. J., Szabo, A. R., and Straley, J. M. (2019). Pectoral herding: an innovative tactic for humpback whale foraging. R. Soc. Open Sci. 6:191104. doi: 10.1098/rsos.191104
Laidre, K. L., Heide-Jørgensen, M. P., Heagerty, P., Cossio, A., Bergström, B., and Simon, M. (2010). Spatial associations between large baleen whales and their prey in West Greenland. Mar. Ecol. Prog. Ser. 402, 269–284. doi: 10.3354/meps08423
Laist, D. W., Knowlton, A. R., Mead, J. G., Collet, A. S., and Podesta, M. (2001). Collisions between ships and whales. Mar. Mamm. Sci. 17, 35–75. doi: 10.1111/j.1748-7692.2001.tb00980.x
Lammers, M. O., Fisher-Pool, P. I., Au, W. L., Meyer, C. G., Wong, K. B., and Brainard, R. E. (2011). Humpback whale Megaptera novaeangliae song reveals wintering activity in the Northwestern Hawaiian Islands. Mar. Ecol. Prog. Ser. 423, 261–268. doi: 10.3354/meps08959
Lindsay, R. E., Constantine, R., Robbins, J., Mattila, D. K., Tagarino, A., and Dennis, T. E. (2016). Characterising essential breeding habitat for whales informs the development of large-scale Marine Protected Areas in the South Pacific. Mar. Ecol. Prog. Ser. 548, 263–275. doi: 10.3354/meps11663
Lodi, L., Tardin, R., and Maricato, G. (2020). Modeling cetacean habitat use in an urban coastal area in southeastern Brazil. Mar. Ecol. Prog. Ser. 642, 227–240. doi: 10.3354/meps13313
Lucena, M. B., Barbosa, M. C., Sissini, M. N., and Sazima, I. (2015). Out of the mainstream: humpback whale calving site and associated fishes at an oceanic island off Brazil. Mar. Biodivers. 46, 27–28. doi: 10.1007/s12526-015-0357-1
Martins, C. C. A., Morete, M. E., Engel, M. H., Freitas, A. C., Secchi, E. R., and Kinas, P. G. (2001). Aspects of habitat use patterns of humpback whales in the Abrolhos bank, Brazil, breeding ground. Mem. Qld. Museum 47, 563–570.
Maxwell, S. M., Gjerde, K. M., Conners, M. G., and Crowder, L. B. (2020). Mobile protected areas for biodiversity on the high seas. Science 367, 252–254. doi: 10.1126/science.aaz9327
May-Collado, L., Gerrodette, T., Calambokidis, J., Rasmussen, K., and Sereg, I. (2005). Patterns of cetacean sighting distribution in the Pacific Exclusive Economic Zone of Costa Rica based on data collected from 1979-2001. Rev. Biol. Trop. 53, 249–263.
McBride, M. M., Dalpadado, P., Drinkwater, K. F., Godø, O. R., Hobday, A. J., Hollowed, A. B., et al. (2014). Krill, climate, and contrasting future scenarios for Arctic and Antarctic fisheries. ICES J. Mar. Sci. 71, 1934–1955. doi: 10.1093/icesjms/fsu002
Meager, J. J., and Limpus, C. (2014). Mortality of inshore marine mammals in eastern Australia is predicted by freshwater discharge and air temperature. PLoS One 9:e94849. doi: 10.1371/journal.pone.0094849
Merchant, C. J., Embury, O., Bulgin, C. E., Block, T., Corlett, G. K., Fiedler, E., et al. (2019). Satellite-based time-series of sea-surface temperature since 1981 for climate applications. Sci. Data 6:223. doi: 10.1038/s41597-019-0236-x
Meyer, B., Atkinson, A., Bernard, K. S., Brierley, A. S., Driscoll, R., Hill, S. L., et al. (2020). Successful ecosystem-based management of Antarctic krill should address uncertainties in krill recruitment, behaviour and ecological adaptation. Commun. Earth Environ. 1:28.
Meynecke, J. O., Vindenes, S., and Teixeira, D. (2013). “Monitoring humpback whale (Megaptera novaeangliae) behaviour in a highly Urbanised coastline: Gold Coast, Australia,” in Global Challenges in Integrated Coastal Zone Management, eds E. Moksness, E. Dahl, and J. Støttrup (Chichester: Wiley), 101–113. doi: 10.1002/9781118496480.ch8
Meynecke, J.-O., and Meager, J. J. (2016). Understanding strandings: 25 years of humpback whale (Megaptera novaeangliae) strandings in Queensland, Australia. J. Coast. Res. 75, 897–902. doi: 10.2112/si75-180.1
Meynecke, J.-O., Seyboth, E., De Bie, J., Menzel Barraqueta, J.-L., Chama, A., Prakash Dey, S., et al. (2020). Responses of humpback whales to a changing climate in the Southern Hemisphere: priorities for research efforts. Mar. Ecol. 41:e12616.
Mignucci-Giannoni, A. A. (1998). Zoogeography of Cetaceans off Puerto Rico and the Virgin Islands. Caribb. J. Sci. 34, 173–190.
Mobley, J. J. R., Bauer, G. B., and Herman, L. M. (1999). Changes over a ten-year interval in the distribution and relative abundance of humpback whales (Megaptera novaeangliae) wintering in Hawaiian waters. Aquat. Mamm. 25, 63–72.
Munger, L. M., Camacho, D., Havron, A., Campbell, G., Calambokidis, J., Douglas, A., et al. (2009). Baleen whale distribution relative to surface temperature and zooplankton abundance off Southern California, 2004–2008. Calif. Coop. Oceanic Fish. Investig. Rep. 50, 155–168.
Naessig, P. J., and Lanyon, J. M. (2004). Levels and probable origin of the predatory scarring on humpback whales (Megaptera novaeangliae) in east Australian waters. Wildl. Res. 31, 163–170. doi: 10.1071/wr03086
Nicol, S., Pauly, T., Bindoff, N. L., Wright, S., Thiele, D., Hosie, G. W., et al. (2000). Ocean circulation off east Antarctica affects ecosystem structure and sea-ice extent. Nature 406, 504–507. doi: 10.1038/35020053
Nicol, S., Worby, A., and Rebecca, L. (2008). Changes in the Antarctic sea ice ecosystem: potential effects on krill and baleen whales. Mar. Freshw. Res. 59, 361–382. doi: 10.1071/mf07161
Noad, M. J., and Cato, D. H. (2007). Swimming speeds of singing and non-singing humpback whales during migration. Mar. Mamm. Sci. 23, 481–495. doi: 10.1111/j.1748-7692.2007.02414.x
Noad, M. J., Kniest, E., and Dunlop, R. A. (2019). Boom to bust? Implications for the continued rapid growth of the eastern Australian humpback whale population despite recovery. Popul. Ecol. 61, 198–209. doi: 10.1002/1438-390x.1014
Nowacek, D. P., Friedlaender, A. S., Halpin, P. N., Hazen, E. L., Johnston, D. W., Read, A. J., et al. (2011). Super-Aggregations of Krill and humpback whales in Wilhelmina Bay, Antarctic Peninsula. PLoS One 6:e19173. doi: 10.1371/journal.pone.0019173
Oppel, S., Meirinho, A., Ramírez, I., Gardner, B., O’connell, A. F., Miller, P. I., et al. (2012). Comparison of five modelling techniques to predict the spatial distribution and abundance of seabirds. Biol. Conserv. 156, 94–104. doi: 10.1016/j.biocon.2011.11.013
Orgeira, J. L., González, J. C., Jiménez, Y. G., and Benítez, L. M. (2017). Occurence of fin and humpback whales in the Scotia Sea and the protected marine area of the South Orkney. Mastozool. Neotrop. 24, 135–143.
Oviedo, L., and Solís, M. (2008). Underwater topography determines critical breeding habitat for humpback whales near Osa Peninsula, Costa Rica: implications for Marine Protected Areas. Rev. Biol. Trop. 56, 591–602. doi: 10.15517/rbt.v56i2.5610
Owen, K., Jenner, K. C. S., Jenner, M.-N. M., Mccauley, R. D., and Andrews, R. D. (2019). Water temperature correlates with baleen whale foraging behaviour at multiple scales in the Antarctic. Mar. Freshw. Res. 70, 19–32. doi: 10.1071/mf17288
Owen, K., Kavanagh, A. S., Warren, J. D., Noad, M. J., Donnelly, D., Goldizen, A. W., et al. (2017). Potential energy gain by whales outside of the Antarctic: prey preferences and consumption rates of migrating humpback whales (Megaptera novaeangliae). Polar Biol. 40, 277–289. doi: 10.1007/s00300-016-1951-9
Pacifici, K., Reich, B. J., Miller, D. A. W., Gardner, B., Stauffer, G., Singh, S., et al. (2017). Integrating multiple data sources in species distribution modeling: a framework for data fusion∗. Ecology 98, 840–850. doi: 10.1002/ecy.1710
Paton, D. A., and Kniest, E. (2011). Population growth of Australian east Coasthumpback whales, observed from Cape Byron, 1998 to 2004. J. Cetacean Res. Manage. Spec. Issue 3, 261–268. doi: 10.47536/jcrm.vi.316
Piatt, J. F., Methven, D. A., Burger, A. E., Mclagan, R. L., Mercer, V., and Creelman, E. (1989). Baleen whales and their prey in a coastal environment. Can. J. Zool. 67, 1523–1530. doi: 10.1126/science.aax9044
Picanço, C., Carvalho, I., and Brito, C. (2009). Occurrence and distribution of cetaceans in São Tomé and Príncipe tropical archipelago and their relation to environmental variables. J. Mar. Biol. Assoc. U. K. 89, 1071–1076. doi: 10.1017/s0025315409002379
Pineda, J., Starczak, V., Da Silva, J. C., Helfrich, K., Thompson, M., and Wiley, D. (2015). Whales and waves: humpback whale foraging response and the shoaling of internal waves at Stellwagen Bank. J. Geophys. Res. Oceans 120, 2555–2570. doi: 10.1002/2014jc010564
Plum, C., Hillebrand, H., and Moorthi, S. (2020). Krill vs salps: dominance shift from krill to salps is associated with higher dissolved N:P ratios. Sci. Rep. 10:5911. doi: 10.1038/s41598-020-62829-8
Purdon, J., Shabangu, F. W., Pienaar, M., Somers, M. J., and Findlay, K. (2020). Cetacean species richness in relation to anthropogenic impacts and areas of protection in South Africa’s mainland Exclusive Economic Zone. Ocean Coast. Manage. 197:105292. doi: 10.1016/j.ocecoaman.2020.105292
Ramp, C., Delarue, J., Palsbøll, P. J., Sears, R., and Hammond, P. S. (2015). Adapting to a warmer ocean—seasonal shift of baleen whale movements over three decades. PLoS One 10:e0121374. doi: 10.1371/journal.pone.0121374
Rasmussen, K., Calambokidis, J., and Steiger, G. H. (2012). Distribution and migratory destinations of humpback whales off the Pacific coast of Central America during the boreal winters of 1996–2003. Mar. Mamm. Sci. 28, E267–E279.
Rasmussen, K., Palacios, D. M., Calambokidis, J., Saborío, M. T., Dalla Rosa, L., Secchi, E. R., et al. (2007). Southern Hemisphere humpback whales wintering off Central America: insights from water temperature into the longest mammalian migration. Biol. Lett. 3, 302–305. doi: 10.1098/rsbl.2007.0067
Redfern, J. V., Moore, T. J., Fiedler, P. C., De Vos, A., Brownell, R. L. Jr., Forney, K. A., et al. (2017). Predicting cetacean distributions in data-poor marine ecosystems. Divers. Distrib. 23, 394–408. doi: 10.1111/ddi.12537
Reinke, J., Lemckert, C., and Meynecke, J.-O. (2016). Coastal fronts utilized by migrating humpback whales, Megaptera novaeangliae, on the Gold Coast, Australia. J. Coastal Res. 75, 552–557. doi: 10.2112/si75-111.1
Reisinger, R. R., Friedlaender, A. S., Zerbini, A. N., Palacios, D. M., Andrews-Goff, V., Dalla Rosa, L., et al. (2021). Combining regional habitat selection models for large-scale prediction: circumpolar habitat selection of southern ocean humpback whales. Rem. Sens. 13:2074. doi: 10.3390/rs13112074
Remili, A., Gallego, P., Pinzone, M., Castro, C., Jauniaux, T., Garigliany, M.-M., et al. (2020). Humpback whales (Megaptera novaeangliae) breeding off Mozambique and Ecuador show geographic variation of persistent organic pollutants and isotopic niches. Environ. Pollut. 267:115575. doi: 10.1016/j.envpol.2020.115575
Ressler, P. H., Dalpadado, P., Macaulay, G. J., Handegard, N., and Skern-Mauritzen, M. (2015). Acoustic surveys of euphausiids and models of baleen whale distribution in the Barents Sea. Mar. Ecol. Prog. Ser. 527, 13–29. doi: 10.3354/meps11257
Riekkola, L., Andrews-Goff, V., Friedlaender, A., Constantine, R., and Zerbini, A. N. (2019). Environmental drivers of humpback whale foraging behavior in the remote Southern Ocean. J. Exp. Mar. Biol. Ecol. 517, 1–12. doi: 10.1016/j.jembe.2019.05.008
Rockwood, R. C., Elliott, M. L., Saenz, B., Nur, N., and Jahncke, J. (2020). Modeling predator and prey hotspots: management implications of baleen whale co-occurrence with krill in Central California. PLoS One 15:e0235603. doi: 10.1371/journal.pone.0235603
Roemmich, D., Alford, M. H., Claustre, H., Johnson, K., King, B., Moum, J., et al. (2019). On the Future of Argo: a Global, Full-Depth, Multi-Disciplinary Array. Front. Mar. Sci. 6:439. doi: 10.3389/fmars.2019.00439
Rosenbaum, H., and Collins, T. (2006). The ecology, population characteristics and conservation efforts for humpback whales (Megaptera noavaeangliae) on their wintering grounds in the coastal waters of Gabon. Bull. Biol. Soc. Washington 12, 219–228.
Salden, D. R. (1988). Humpback whale encounter rates offshore of Maui, Hawaii. J. Wildl. Manage. 52, 301–304. doi: 10.2307/3801238
Santora, J. A., Mantua, N. J., Schroeder, I. D., Field, J. C., Hazen, E. L., Bograd, S. J., et al. (2020). Habitat compression and ecosystem shifts as potential links between marine heatwave and record whale entanglements. Nat. Commun. 11:536. doi: 10.1038/s41467-019-14215-w
Santora, J. A., Reiss, C. S., Loeb, V. J., and Veit, R. R. (2010). Spatial association between hotspots of baleen whales and demographic patterns of Antarctic krill Euphausia superba suggests size-dependent predation. Mar. Ecol. Prog. Ser. 405, 255–269. doi: 10.3354/meps08513
Schmitt, N. T., Double, M. C., Jarman, S. N., Gales, N., Marthick, J. R., Polanowski, A. M., et al. (2014). Low levels of genetic differentiation characterize Australian humpback whale (Megaptera novaeangliae) populations. Mar. Mamm. Sci. 30, 221–241. doi: 10.1111/mms.12045
Schweigert, J. F., Thompson, M., Fort, C., Hay, D. E., Therriault, T. W., and Brown, L. N. (2013). Factors linking Pacific herring (Clupea pallasi) productivity and the spring plankton bloom in the Strait of Georgia, British Columbia, Canada. Prog. Oceanogr. 115, 103–110. doi: 10.1016/j.pocean.2013.05.017
Seyboth, E., Félix, F., Lea, M.-A., Dalla Rosa, L., Watters, G. M., Reid, K., et al. (2021). Influence of krill (Euphausia superba) availability on humpback whale (Megaptera novaeangliae) reproductive rate. Mar. Mamm. Sci. 37, 1498–1506.
Shchepetkin, A. F., and McWilliams, J. C. (2005). The regional oceanic modeling system (ROMS): a split-explicit, free-surface, topography-following-coordinate oceanic model. Ocean Model. 9, 347–404. doi: 10.1029/2007JC004602
Shelden, K. E., and Rugh, D. J. (2010). Forty years of winter: cetaceans observed during the southbound migration of gray whales, Eschrichtius robustus, near Granite Canyon, central California. Mar. Fish. Rev. 72, 1–19.
Siegel, V., and Watkins, J. L. (2016). “Distribution, biomass and demography of antarctic krill, Euphausia superba,” in Biology and Ecology of Antarctic Krill, ed. V. Siegel (Cham: Springer International Publishing), 21–100. doi: 10.1007/978-3-319-29279-3_2
Sleeman, J. C., Meekan, M. G., Wilson, S. G., Jenner, C., Jenner, M. N., Boggs, G. S., et al. (2007). Biophysical correlates of abundances of marine megafauna at Ningaloo Reef, Western Australia. Mar. Freshw. Res. 58, 608–623. doi: 10.1071/mf06213
Smith, A. B., and Santos, M. J. (2020). Testing the ability of species distribution models to infer variable importance. Ecography 43, 1801–1813. doi: 10.1111/ecog.05317
Smith, J. N., Grantham, H. S., Gales, N., Double, M. C., Noad, M. J., and Paton, D. (2012). Identification of humpback whale breeding and calving habitat in the Great Barrier Reef. Mar. Ecol. Prog. Ser. 447, 259–272. doi: 10.3354/meps09462
Smith, J. N., Kelly, N., and Renner, I. W. (2020b). Validation of presence-only models for conservation planning and the application to whales in a multiple-use marine park. Ecol. Appl. Early View 31:e02214. doi: 10.1002/eap.2214
Smith, J. N., Kelly, N., Childerhouse, S., Redfern, J. V., Moore, T. J., and Peel, D. (2020a). Quantifying ship strike risk to breeding whales in a multiple-use marine park: the great barrier reef. Front. Mar. Sci. 7:67. doi: 10.3389/fmars.2020.00067
Smith, R., Dustan, P., Au, D., Baker, K., and Dunlap, E. (1986). Distribution of cetaceans and sea-surface chlorophyll concentrations in the California Current. Mar. Biol. 91, 385–402. doi: 10.1007/bf00428633
Sprogis, K. R., Bejder, L., Hanf, D., and Christiansen, F. (2020). Behavioural responses of migrating humpback whales to swim-with-whale activities in the Ningaloo Marine Park, Western Australia. J. Exp. Mar. Biol. Ecol. 522:151254. doi: 10.1016/j.jembe.2019.151254
Stack, S., Currie, J., Mccordic, J., Machernis, A., and Olson, G. (2019). Distribution patterns of east Australian humpback whales (Megaptera novaeangliae) in Hervey Bay, Queensland: a historical perspective. Aust. Mammal. 42, 16–27. doi: 10.1071/am18029
Steiger, G. H. E. A. (2008). Geographic variation in killer whale attacks on humpback whales in the North Pacific: implications for predation pressure. Endanger. Species Res. 4, 247–256. doi: 10.3354/esr00078
Stephenson, F., Goetz, K., Sharp, B. R., Mouton, T. L., Beets, F. L., Roberts, J., et al. (2020). Modelling the spatial distribution of cetaceans in New Zealand waters. Divers. Distrib. 26, 495–516. doi: 10.1111/ddi.13035
Stevick, P. T., Neves, M. C., Johansen, F., Engel, M. H., Allen, J., Marcondes, M. C. C., et al. (2011). A quarter of a world away: female humpback whale moves 10 000 km between breeding areas. Biol. Lett. 7, 299–302. doi: 10.1098/rsbl.2010.0717
Stockin, K. A., and Burgess, E. A. (2005). Opportunistic feeding of an adult humpback whale (Megaptera novaeangliae) migrating along the coast of southeastern Queensland, Australia. Aquat. Mamm. 31, 120–123. doi: 10.1578/am.31.1.2005.120
Stone Florez-Gonzalez, L., and Katona, S. G. (1990). Whale migration record. Nat. Lond. 346:705. doi: 10.1038/346705a0
Suryan, R. M., Arimitsu, M. L., Coletti, H. A., Hopcroft, R. R., Lindeberg, M. R., Barbeaux, S. J., et al. (2021). Ecosystem response persists after a prolonged marine heatwave. Sci. Rep. 11:6235.
Szesciorka, A. R., Ballance, L. T., Široviæ, A., Rice, A., Ohman, M. D., Hildebrand, J. A., et al. (2020). Timing is everything: drivers of interannual variability in blue whale migration. Sci. Rep. 10:7710. doi: 10.1038/s41598-020-64855-y
Tardin, R. H., Chun, Y., Jenkins, C. N., Maciel, I. S., Simão, S. M., and Alves, M. A. S. (2019). Environment and anthropogenic activities influence cetacean habitat use in southeastern Brazil. Mar. Ecol. Prog. Ser. 616, 197–210. doi: 10.3354/meps12937
Thomas, L., Buckland, S. T., Rexstad, E. A., Laake, J. L., Strindberg, S., Hedley, S. L., et al. (2010). Distance software: design and analysis of distance sampling surveys for estimating population size. J. Appl. Ecol. 47, 5–14. doi: 10.1111/j.1365-2664.2009.01737.x
Thompson, S. A., Sydeman, W. J., Santora, J. A., Black, B. A., Suryan, R. M., Calambokidis, J., et al. (2012). Linking predators to seasonality of upwelling: using food web indicators and path analysis to infer trophic connections. Prog. Oceanogr. 101, 106–120. doi: 10.1016/j.pocean.2012.02.001
Tiago, P., Ceia-Hasse, A., Marques, T. A., Capinha, C., and Pereira, H. M. (2017). Spatial distribution of citizen science casuistic observations for different taxonomic groups. Sci. Rep. 7:12832. doi: 10.1038/s41598-017-13130-8
Todd, V. L. G., Todd, I. B., Gardiner, J. C., Morrin, E. C. N., Macpherson, N. A., Dimarzio, N. A., et al. (2015). A review of impacts of marine dredging activities on marine mammals. ICES J. Mar. Sci. 72, 328–340. doi: 10.1093/icesjms/fsu187
Torre-Williams, L., Martinez, E., Meynecke, J. O., Reinke, J., and Stockin, K. A. (2019). Presence of newborn humpback whale (Megaptera novaeangliae) calves in Gold Coast Bay, Australia. Mar. Freshw. Behav. Physiol. 52, 199–216. doi: 10.1080/10236244.2019.1671769
Tulloch, V. J. D., Plagányi, ÉE., Brown, C., Richardson, A. J., and Matear, R. (2019). Future recovery of baleen whales is imperiled by climate change. Glob. Change Biol. 25, 1263–1281. doi: 10.1111/gcb.14573
Tulloch, V. J. D., Plagányi, ÉE., Matear, R., Brown, C. J., and Richardson, A. J. (2018). Ecosystem modelling to quantify the impact of historical whaling on Southern Hemisphere baleen whales. Fish Fish. 19, 117–137. doi: 10.1111/faf.12241
Turner, J., and Marshall, G. J. (2011). Climate Change in the Polar Regions. Cambridge: Cambridge University Press.
Tynan, C. T., Ainley, D. G., Barth, J. A., Cowles, T. J., Pierce, S. D., and Spear, L. B. (2005). Cetacean distributions relative to ocean processes in the northern California Current System. Deep Sea Res. Part II Top. Stud. Oceanogr. 52, 145–167. doi: 10.1016/j.dsr2.2004.09.024
Valani, R., Meynecke, J.-O., and Olsen, M. T. (2020). Presence and movement of humpback whale (Megaptera novaeangliae) mother-calf pairs in the Gold Coast, Australia. Mar. Freshw. Behav. Physiol. 53, 251–263. doi: 10.1080/10236244.2020.1850177
Valsecchi, E., Corkeron, P. J., Galli, P., Sherwin, W., and Bertorelle, G. (2010). Genetic evidence for sex-specific migratory behaviour in western South Pacific humpback whales. Mar. Ecol. Prog. Ser. 398, 275–286. doi: 10.3354/meps08280
van Weelden, C., Towers, J. R., and Bosker, T. (2021). Impacts of climate change on cetacean distribution, habitat and migration. Clim. Change Ecol. 1:100009. doi: 10.1016/j.ecochg.2021.100009
Viddi, F. A., Hucke-Gaete, R., Torres-Florez, J. P., and Ribeiro, S. (2010). Spatial and seasonal variability in cetacean distribution in the fjords of northern Patagonia, Chile. ICES J. Mar. Sci. 67, 959–970. doi: 10.1093/icesjms/fsp288
Visser, F., Hartman, K. L., Pierce, G. J., Valavanis, V. D., and Huisman, J. (2011). Timing of migratory baleen whales at the Azores in relation to the North Atlantic spring bloom. Mar. Ecol. Prog. Ser. 440, 267–279. doi: 10.3354/meps09349
Volep, E., Carroll, A., Strauss, D., Meynecke, J.-O., and Kobashi, D. (2017). Effect of environmental conditions on cetacean entanglements: a case study from the Gold Coast, Australia. Mar. Freshw. Res. 68, 1977–1987. doi: 10.1071/mf16302
Ware, C., Friedlaender, A. S., and Nowacek, D. P. (2011). Shallow and deep lunge feeding of humpback whales in fjords of the West Antarctic Peninsula. Mar. Mamm. Sci. 27, 587–605. doi: 10.1111/j.1748-7692.2010.00427.x
Weilgart, L. S. (2007). The impacts of anthropogenic ocean noise on cetaceans and implications for management. Can. J. Zool. 85, 1091–1116. doi: 10.1016/j.marpolbul.2018.01.014
Weinrich, M. (1998). Early experience in habitat choice by humpback whales (Megaptera novaeangliae). J. Mammal. 79, 163–170. doi: 10.2307/1382851
Weinstein, B. G., Double, M., Gales, N., Johnston, D. W., and Friedlaender, A. S. (2017). Identifying overlap between humpback whale foraging grounds and the Antarctic krill fishery. Biol. Conserv. 210, 184–191. doi: 10.1016/j.biocon.2017.04.014
Wernand, M. R., Van Der Woerd, H. J., and Gieskes, W. W. C. (2013). Trends in ocean colour and chlorophyll concentration from 1889 to 2000, Worldwide. PLoS One 8:e63766. doi: 10.1371/journal.pone.0063766
Whitehead, H., and Moore, M. J. (1982). Distribution and movements of West Indian humpback whales in winter. Can. J. Zool. 60, 2203–2211. doi: 10.1139/z82-282
Whitehead, H., and Rendell, L. (2021). “Mammals of the ocean” in The Cultural Lives of Whales and Dolphins (Chicago, IL: University of Chicago Press), 45–66.
Zerbini, A. N., Friday, N. A., Palacios, D. M., Waite, J. M., Ressler, P. H., Rone, B. K., et al. (2016). Baleen whale abundance and distribution in relation to environmental variables and prey density in the Eastern Bering Sea. Deep Sea Res. Part II Top. Stud. Oceanogr. 134, 312–330. doi: 10.1016/j.dsr2.2015.11.002
Keywords: climate change, oceanography, cetacean, distribution drivers, environmental change, Megaptera novaeangliae, occurrence
Citation: Meynecke J-O, de Bie J, Barraqueta J-LM, Seyboth E, Dey SP, Lee SB, Samanta S, Vichi M, Findlay K, Roychoudhury A and Mackey B (2021) The Role of Environmental Drivers in Humpback Whale Distribution, Movement and Behavior: A Review. Front. Mar. Sci. 8:720774. doi: 10.3389/fmars.2021.720774
Received: 04 June 2021; Accepted: 22 October 2021;
Published: 10 November 2021.
Edited by:
David M. P. Jacoby, Lancaster University, United KingdomReviewed by:
Elizabeth McHuron, University of Washington, United StatesLenin Enrique Oviedo Correa, Centro de Investigación de Cetáceos Costa Rica, Costa Rica
Copyright © 2021 Meynecke, de Bie, Barraqueta, Seyboth, Dey, Lee, Samanta, Vichi, Findlay, Roychoudhury and Mackey. This is an open-access article distributed under the terms of the Creative Commons Attribution License (CC BY). The use, distribution or reproduction in other forums is permitted, provided the original author(s) and the copyright owner(s) are credited and that the original publication in this journal is cited, in accordance with accepted academic practice. No use, distribution or reproduction is permitted which does not comply with these terms.
*Correspondence: Jan-Olaf Meynecke, o.meynecke@griffith.edu.au