- 1Institute of Coastal Systems-Analysis and Modeling, Helmholtz-Zentrum Hereon, Geesthacht, Germany
- 2Nansen Environmental and Remote Sensing Center, and Bjerknes Centre for Climate Research, Bergen, Norway
- 3Institute of Oceanography, Center for Earth System Research and Sustainability (CEN), Universität Hamburg, Hamburg, Germany
- 4Alfred Wegener Institute, Bremerhaven, Germany
- 5Royal Netherlands Institute for Sea Research (NIOZ), Texel, Netherlands
- 6Max Planck Institute for Meteorology, Hamburg, Germany
Predicting the ambient environmental conditions in the coming several years to one decade is of key relevance for elucidating how deep-sea habitats, like for example sponge habitats, in the North Atlantic will evolve under near-future climate change. However, it is still not well known to what extent the deep-sea environmental properties can be predicted in advance. A regional downscaling prediction system is developed to assess the potential predictability of the North Atlantic deep-sea environmental factors. The large-scale climate variability predicted with the coupled Max Planck Institute Earth System Model with low-resolution configuration (MPI-ESM-LR) is dynamically downscaled to the North Atlantic by providing surface and lateral boundary conditions to the regional coupled physical-ecosystem model HYCOM-ECOSMO. Model results of two physical fields (temperature and salinity) and two biogeochemical fields (concentrations of silicate and oxygen) over 21 sponge habitats are taken as an example to assess the ability of the downscaling system to predict the interannual to decadal variations of the environmental properties based on ensembles of retrospective predictions over the period from 1985 to 2014. The ensemble simulations reveal skillful predictions of the environmental conditions several years in advance with distinct regional differences. In areas closely tied to large-scale climate variability and ice dynamics, both the physical and biogeochemical fields can be skillfully predicted more than 4 years ahead, while in areas under strong influence of upper oceans or open boundaries, the predictive skill for both fields is limited to a maximum of 2 years. The simulations suggest higher predictability for the biogeochemical fields than for the physical fields, which can be partly attributed to the longer persistence of the former fields. Predictability is improved by initialization in areas away from the influence of Mediterranean outflow and areas with weak coupling between the upper and deep oceans. Our study highlights the ability of the downscaling regional system to predict the environmental variations at deep-sea benthic habitats on time scales of management relevance. The downscaling system therefore will be an important part of an integrated approach towards the preservation and sustainable exploitation of the North Atlantic benthic habitats.
Introduction
The deep sea, which encompasses depths below around 200 m, was initially considered to be a “marine desert” with low density and biomass of benthic species (Sanders and Hessler, 1969). Today we know that it can host a variety of unique ecosystems like for example cold-water coral (CWC) reefs and sponge grounds (Roberts et al., 2006; Hogg et al., 2010; Cathalot et al., 2015). In the deep North Atlantic Ocean, numerous benthic habitats widely spread across a broad spectrum of geomorphological features such as shelves, slopes, seamounts, mid-ocean ridges, canyons and fjords (Cárdenas and Rapp, 2015; Roberts et al., 2018; Kazanidis et al., 2019a,b,c; Meyer et al., 2020a, b), which are associated with a wide range of depths through the mesopelagic and bathyal zones and even at abyssal and hadal depths (Vacelet and Custódio, 2007; Hestetun et al., 2019). These deep-sea habitats have gained increasing attention in recent years from both ecological and conservation perspectives, as they are found to be vital for the well-being of the entire ocean and biosphere (e.g., Sweetman et al., 2017; Levin et al., 2019). Sponge habitats and CWCs, for example, provide ecosystem goods and services like the provision of habitats and food sources for associated benthic and pelagic species (Bett and Rice, 1992; Maldonado et al., 2017; Hawkes et al., 2019) and thereby sustain hotspots of biodiversity in the deep sea (Klitgaard and Tendal, 2004; Buhl-Mortensen et al., 2010; Beazley et al., 2013, 2015; Maldonado et al., 2017; Meyer et al., 2019). Further they are of fundamental importance for the benthic–pelagic coupling and marine biogeochemical cycling (Pile and Young, 2006; Oevelen et al., 2009; Smith et al., 2009; Maldonado et al., 2019).
The deep-sea environmental conditions, including but not limited to the physical (e.g., temperature, salinity, current velocity, and bottom shear) and biogeochemical properties (e.g., nutrients and oxygen concentrations), are crucial constrains of the biodiversity, distribution, and connectivity patterns of the deep-sea habitats (e.g., Levin, 2003; Woulds et al., 2007; Yasuhara and Danovaro, 2016; Puerta et al., 2020). For instance, temperature influences metabolic rates and enzyme activities in organisms, resulting in different temperature envelopes for their species thriving (Hochachka and Somero, 1968; Yasuhara and Danovaro, 2016). Salinity, together with temperature, defines the water density — a key parameter with which the occurrence of some important habitats, for example CWCs in the northeastern Atlantic, is found to be correlated (Dullo et al., 2008). In addition, temperature and salinity are often used as proxies of water masses that are associated with circulation patterns, which is a key physical process in influencing the distribution and density of the benthic habitats through transporting food or larvae (Puerta et al., 2020; Roberts et al., 2021). Inorganic nutrients are crucial components for the construction of organisms, with silicate as an example being an essential element for building the skeleton of most deep-sea sponges (Howell et al., 2016; Maldonado et al., 2020, 2021). Oxygen is an important electron acceptor for biogeochemical processes and therefore fundamental for aerobic life. If oxygen decreases below a certain threshold, organisms experience a variety of stressors, limiting their distributions like for example in oxygen minimum zones (Vaquer-Sunyer and Duarte, 2008; Keeling et al., 2010). Those environmental conditions vary among different benthic habitats. As a result, the deep-sea species have their own preferred ranges of environmental properties, connected to their optimal growth and feeding conditions and the spreading or retention of larvae (e.g., Klitgaard and Tendal, 2004; Roberts et al., 2021). For instance, the reef-forming CWCs Lophelia pertusa (as well as the non-reef-forming CWC species Desmophyllum dianthus which is significantly similar to Lophelia molecularly and morphologically, Addamo et al., 2016) and Madrepora oculata are mainly found at a temperature range of 4–12°C (Naumann et al., 2014), while octocorals survive at a narrower and lower temperature range of 1.6–6.1°C (Buhl-Mortensen et al., 2015). The boreal sponge grounds with Geodia barretti, Stryphnus ponderosus, and Stelletta normani are only rarely occurring at temperatures lower than 3°C, whereas cold-water sponge grounds with Geodia parva, Geodia hentscheli, Geodia phlegraei, Stelletta rhaphidiophora, and Schaudinnia rosea can live in waters below zero degrees (Hogg et al., 2010; Roberts et al., 2021).
Emerging evidences show that changes in environmental conditions will affect habitat integrity and representativeness, and thus will alter species distributions and interactions (Meyer et al., 2015; Pecl et al., 2017; Puerta et al., 2020). For instance, Blacker (1957, 1965) mapped changes in the distribution of sponges off West Spitzbergen and he ascribed the changes to a stronger inflow of Atlantic water, which was reflected in a change of water mass properties. Erekovsky (1995) found a nearly seven-fold decrease of sponges in the Barents Sea between 1920–1950 and 1960–1980 which was presumed to be related to a shift in the polar front (Klitgaard and Tendal, 2004). Even though the response of deep-sea habitats to environmental changes varies among species and locations (e.g., Buhl-Mortensen et al., 2015; Hanz et al., 2020, 2021; Puerta et al., 2020), it is suggested that many of the habitats are often living in a fragile equilibrium. They are likely not able to adapt to abrupt changes in the environment because of their slow growth rates and long life spans (Yasuhara et al., 2008, 2014; Roark et al., 2009; Morrison et al., 2020).
Climate change and anthropogenic activities are bringing significant changes in the environmental properties of the deep ocean, which are likely to move beyond the limits of past natural variability by the end of 21st century (Purkey and Johnson, 2010; Levin and Le Bris, 2015; Sweetman et al., 2017; Perez et al., 2018). Such unprecedented environmental changes could further provoke a cascade of deleterious ecological consequences like sponge demise (Sweetman et al., 2017). In fact, footprints of climate change on deep-sea environmental conditions have already been reported in recent decades (Purkey and Johnson, 2010; Stramma et al., 2010; Morato et al., 2020), which in turn have raised substantial pressures on the deep-sea habitats (e.g., Yasuhara et al., 2016; Strand et al., 2017). In 2006 and 2008, mass mortalities events of the deep-water sponge Geodia barretti at the Tisler Reef on the sill of the Kosterfjord along the Norwegian shelf were observed to coincide with a series of uncharacteristically large and rapid increases in bottom water temperature, which exceeded the typical short-term physiological limits of the host reef, principally Lophelia pertusa (Guihen et al., 2012). It was inferred that the unexpected temperature increase was associated with climate change and that future temperature shock events at this site might have a swift and negative impact on the local CWC reef ecosystems. Johnson et al. (2018) predicted that in a 20–50 year timeframe most of the deep-sea Area-Based Management Tools (ABMTs) in the North Atlantic would be affected by climate change, implying that significant changes of the deep-sea ecosystem might take place in the near future. Because of the high vulnerability of these deep-sea habitats to the effects of anthropogenic disturbance and climate change, the benthic invertebrates, e.g., CWC reefs and sponge habitats, are classified as ‘vulnerable marine ecosystems (VMEs)’ by the Food and Agriculture Organization of the United Nations (FAO, 2009), which are in need of enhanced conservation and protection on account of their longevity and expected long recovery times after disturbance (Levin et al., 2019).
In face of ongoing environmental changes, addressing the increasing pressures placed on the deep-sea habitats is an urgent task requiring thorough evaluation of cumulative impacts (Johnson et al., 2018). To date, numbers of studies, including modeling prediction endeavors, have been conducted to investigate the possible impacts of future climate change on deep-sea benthic ecosystems (Knudby et al., 2013; Sweetman et al., 2017; Beazley et al., 2018, 2021; Bell et al., 2018; Johnson et al., 2018; Morato et al., 2020; Pearman et al., 2020; Puerta et al., 2020). However, most of the studies were based on environmental conditions that were projected into the end of the 21st century with a broad range of assumptions on future greenhouse gas emissions, world population growth and technology development (Riahi et al., 2011; Van Vuuren et al., 2011). Those climate projections explain well the long-term trends while there is low confidence in the interannual to decadal variability at a given point in time (Befort et al., 2020; Lee et al., 2021). Therefore, the estimated deep-sea ecosystem status is scenario dependent and potentially presents a high degree of uncertainty at short timescales (Payne et al., 2016; Beazley et al., 2021). By far, few studies regarding the impacts of near-term environmental changes (i.e., over the next years to one decade in the future) on the benthic habitats have been reported, mostly because that reliable predictions of the environment variations in the near-term future are lacking (Johnson et al., 2017). One exception is Johnson et al. (2018), who drew the information of environmental changes over the next 30 years from a compilation of climate projection (IPCC 5th Assessment Report) covering the North Atlantic and other published works with varying sub-regional focuses (Johnson et al., 2017). In spite of the compilation work, they pointed out that more precise and detailed oceanographic data are needed.
In view of a practical protection and sustainable management of the deep-sea habitats, effective and reliable predictions of the natural and anthropogenic-derived variations of the deep-sea environmental factors in the coming years to one decade is required to inform management decisions (Tommasi et al., 2017). Decadal oceanic predictions, encompassing predictions on annual, multi-annual to decadal timescales, are proven to be a suitable approach for this goal (Borchert et al., 2019). A major advantage of decadal predictions compared to centennial climate projections is that the former can integrate the known knowledge from the past into the future—the predictability can be assessed by performing retrospective forecasts of the historical period and comparing them against subsequent observations (Smith et al., 2019). The system is credible for forecasting the future once it shows skillful predictions in the past (Brune et al., 2018; Borchert et al., 2019; Smith et al., 2019). However, to our knowledge, it is still poorly known how many years in advance the environmental conditions at the North Atlantic deep-sea habitats can be predicted, owing to the fact that there is no operational decadal predictions dedicated to the deep sea. To close this knowledge gap, we make a first trial to assess the predictability of the deep-sea environmental factors with a novel downscaling regional decadal prediction system with the main focus on the deep North Atlantic Ocean.
We choose the coupled regional physical-ecosystem model (HYCOM-ECOSMO) for the regional downscaling, with particular considerations of the deep-sea dynamics. For instance, in the physical model, HYCOM (Bleck, 2002), isopycnal levels facilitate good conservation of water-mass and tracer properties in the deep ocean, representing an advantage for simulations focusing on deep-sea habitats at depth (Winther and Evensen, 2006). The ecosystem model, ECOSMO (Daewel and Schrum, 2013), includes a single layer of sediments with parameterizations for processes of settling, resuspension and burial (when sediment can no longer be resuspended) happening at the bottom ocean (Samuelsen et al. submitted to this issue, under review). The model has been shown the capability of reproducing the observed states of the environmental properties at the deep North Atlantic Ocean over the historical period of 1948–2014 (Samuelsen et al., 2019; Samuelsen et al. submitted to this issue, under review). We therefore use the same model configuration with Samuelsen et al. (2019) in our study to perform the decadal predictions.
Specifically, in this study we aim at (1) setting up a downscaling prediction system with retrospective predictions of the marine environmental factors over the period of 1985–2014; (2) assessing the predictability of selected environmental factors at the deep-sea habitats. For assessing the predictability, we choose sponge habitats as examples, which are key components of benthic marine ecosystems in the deep North Atlantic from intertidal to abyssal depths (e.g., Rice et al., 1990; Klitgaard and Tendal, 2004; Beazley et al., 2013). In this study, 21 sponge habitats are selected, covering almost all of the biggest known sponge habitats through the North Atlantic (Figure 1 and Tables 1, 2). In addition, we choose four parameters (temperature, salinity, concentrations of silicate and oxygen) for detailed analysis since they are the most important predictor variables in species distribution models (e.g., Knudby et al., 2013; Beazley et al., 2018; Morato et al., 2020; Rodríguez-Basalo et al., 2021) and have shown to influence the benthic habitats at present as mentioned earlier in this section. Therefore, they are used to represent the physical and biogeochemical fields.
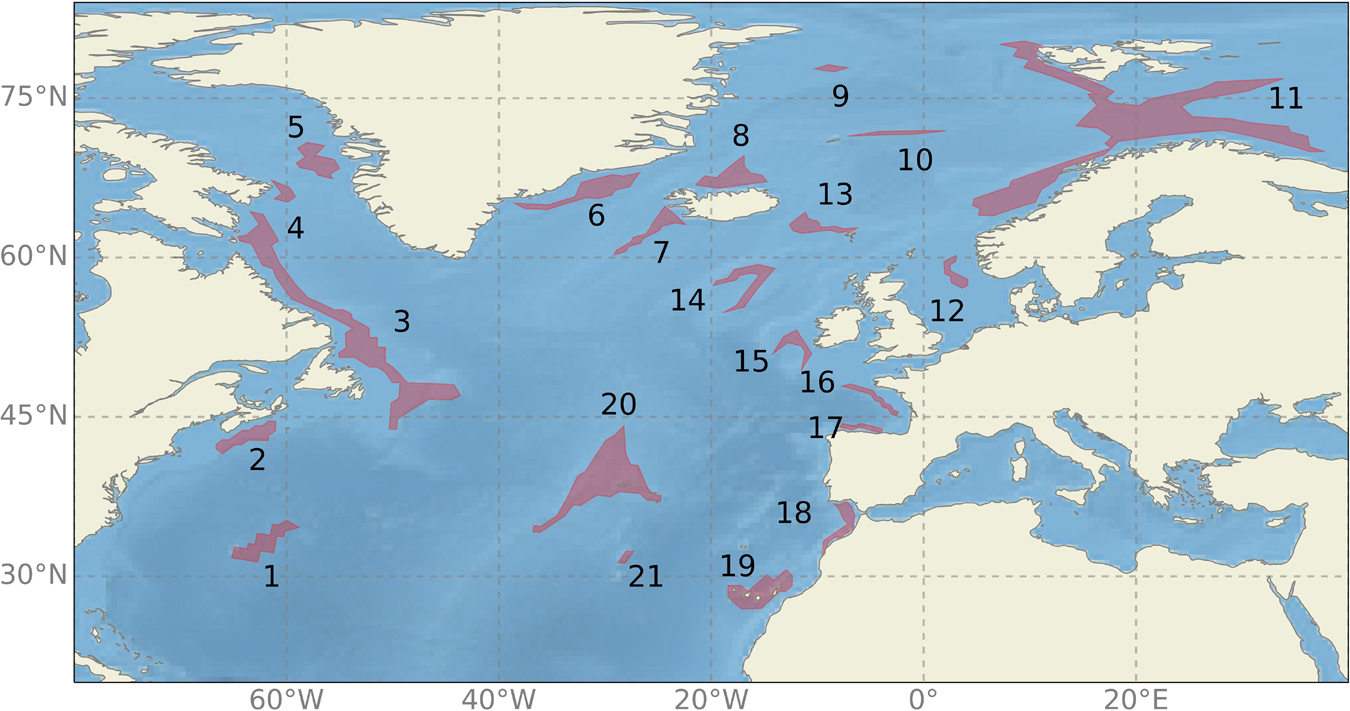
Figure 1. Map of the North Atlantic Ocean including part of the Arctic Ocean showing the locations of the 21 sponge habitats studied in this paper. The habitats are ordered from 1 to 21, with the corresponding number marked on the map.
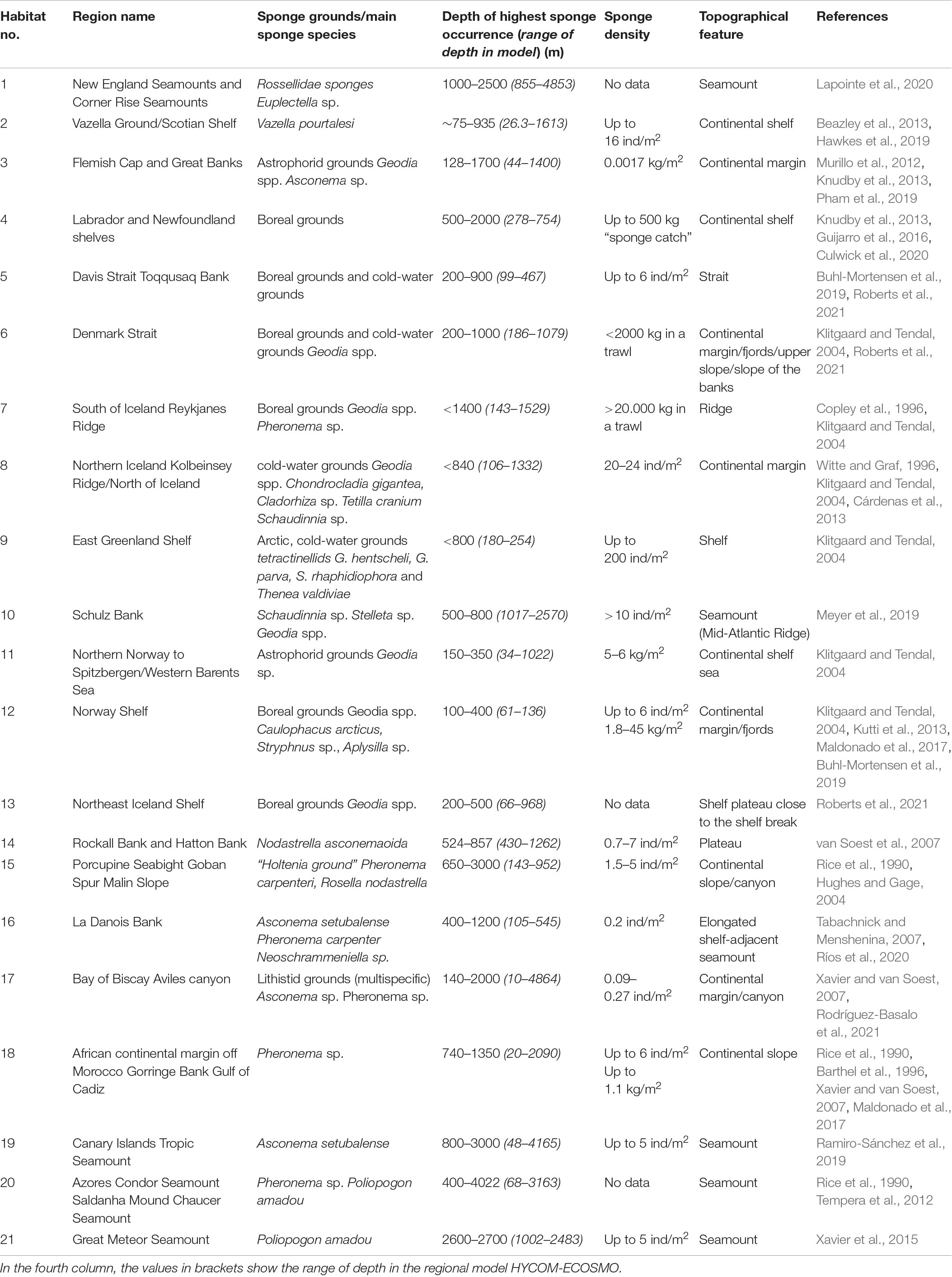
Table 1. The habitat number given in our study and the corresponding name, sponge grounds/main sponge species, depths of highest sponge occurrence, sponge density, topographical feature, and related references.
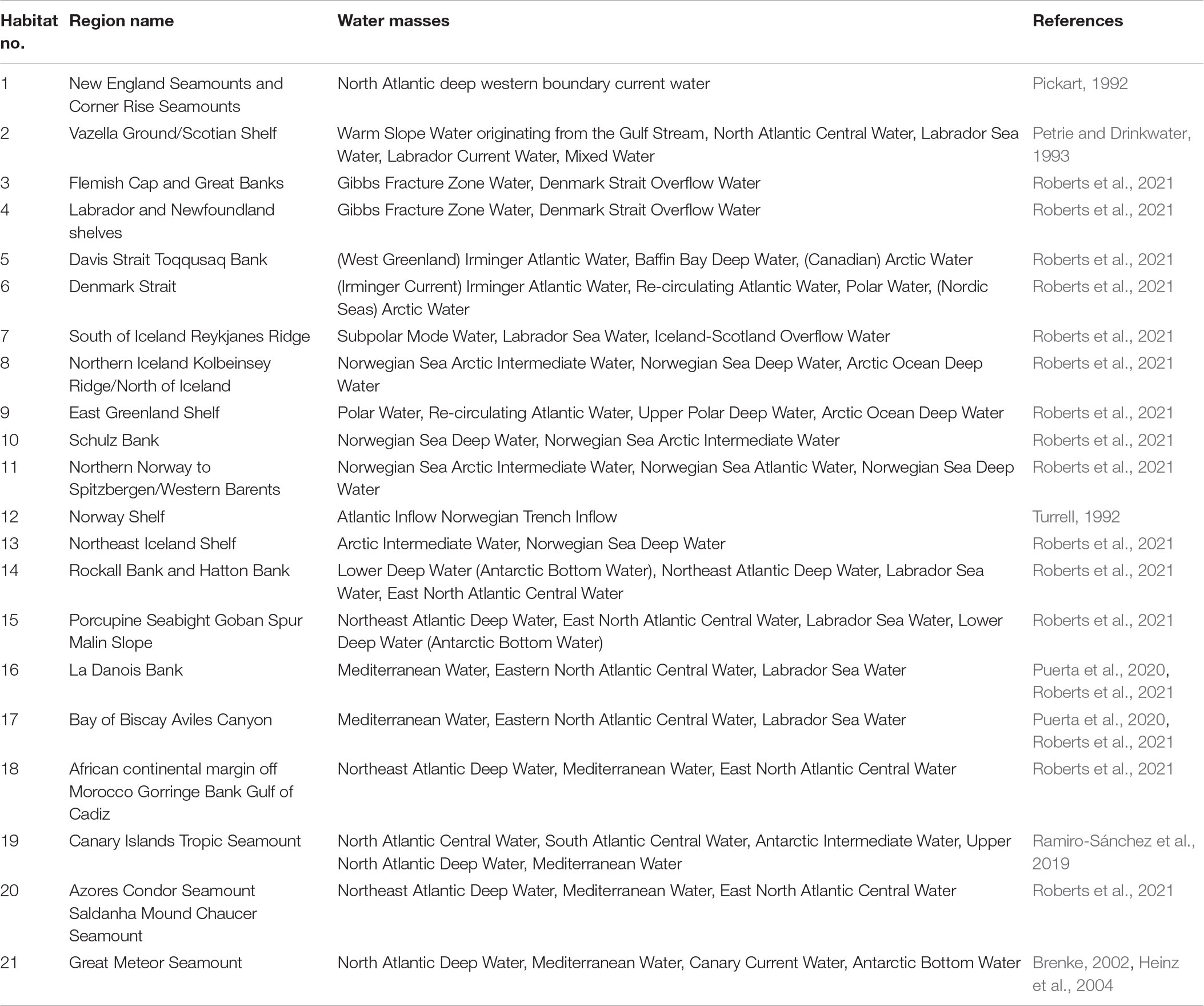
Table 2. The habitat number given in our study, the corresponding name, the associated water masses and related references.
This paper is organized as follows. First, we introduce the downscaling strategy and the methods used to assess the predictability. Subsequently, the potential predictability of the four environmental factors is evaluated over the 21 sponge habitats. Then the possible factors influencing the predictability, the limitations and potentials of the prediction, as well as the applicability of the prediction in the deep-sea habitat management are discussed, which are followed by a final conclusion.
Model and Methods
Model Description
The downscaling prediction is performed based on a regional coupled physical-ecosystem model, covering part of the southern Atlantic and the whole domain of the North Atlantic and the Arctic Ocean (Figure 2). The regional model is forced at the ocean surface and lateral boundaries by output from decadal climate predictions, which are based on fully coupled global earth system models (ESMs), so that large-scale variability of the climate is dynamically downscaled to the regional model domain.
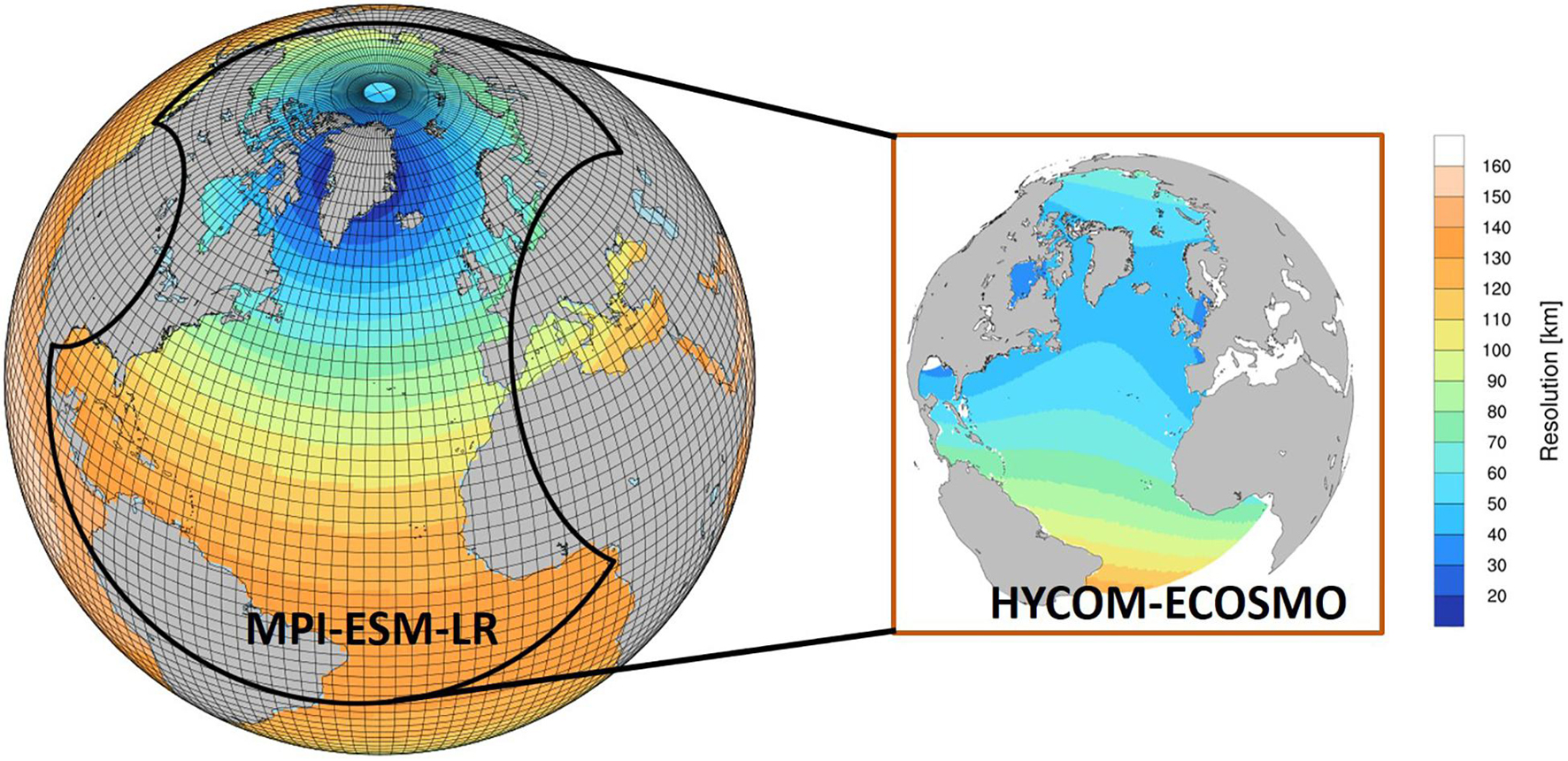
Figure 2. Sketch of the downscaling prediction system. Decadal predictions with the coupled Max Planck Institute Earth System Model with low-resolution configuration (MPI-ESM-LR) provide surface and lateral boundary conditions to the regional coupled physical-ecosystem model HYCOM-ECOSMO. Colors illustrate the horizontal resolution of the ocean models. Grid lines over the global model show the grid of the atmospheric model. Note not all grid-lines are shown.
The regional model, HYCOM-ECOSMO, consists of the physical ocean model Hybrid Coordinate Ocean Model (HYCOM; Bleck, 2002), including a sea ice component, and the ECOSystem Model (ECOSMO) which is a medium-complexity biogeochemical model (Daewel and Schrum, 2013). HYCOM uses a curvilinear grid with a horizontal resolution of approximately 20–70 km. Similar with Sakov et al. (2012) and Samuelsen et al. (2015), the model uses hybrid coordinates in vertical, with z-levels in the upper ocean and mixed layer and isopycnal levels in the deep ocean. Consequently, the vertical layers will be re-distributed along with changes in the deep ocean density fields. The upper five layers are fixed as z-coordinates, ensuring that the vertical resolution of the upper ocean is maintained, which is particularly important for resolving the gradient of light in the upper ocean when computing primary production. At the open boundaries, which are placed between the equator and 30°S in the south, at the Strait of Gibraltar in the east and at the Bering Strait in the north, we mimic the treatments used in Hansen and Samuelsen (2009) and Samuelsen et al. (2015) to set a relaxation zone of 20 model grids, over which the modeled physical fields (temperature, salinity and density) and biogeochemical fields (alkalinity, DIC, nitrate, phosphate, silicate and oxygen) are relaxed toward prescribed fields. The relaxation is strongest at the outer grid cell and is linearly decreased toward the interior of the model, with a relaxation time-scale on the outer grid cell of 20 days.
ECOSMO resolves four nutrients (nitrate, phosphate, silicate, and ammonium), two types of phytoplankton functional groups (diatoms and flagellates) as well as two classes of zooplankton functional groups (herbi- and omnivorous). In addition, particulate and dissolved organic material, and oxygen are included. In the model, constant element ratios consistent with the classical Redfield stoichiometry is applied (Tett et al., 1985). The three nutrients (nitrate, phosphate, and silicate) are tracked in the bottom sediments using simplified 0d bulk parametrizations for sedimentation, resuspension, and remineralization in the sediment layer. ECOSMO is online coupled with HYCOM, sharing the same model grids. A detailed description of the model can be found in Samuelsen et al. (submitted to this issue, under review).
The global decadal climate prediction used in this study is based on the coupled Max Planck Institute Earth System Model with low-resolution configuration (MPI-ESM-LR baseline-1, Pohlmann et al., 2013). The ocean component of MPI-ESM-LR uses bipolar configuration, with one pole over Greenland and another over Antarctica, leading to the spatial resolution ranging from 15 km around Greenland to 185 km in the tropical area (Jungclaus et al., 2013). The capability of this ESM to predict the variability in the upper ocean of the North Atlantic several years in advance has been identified both for the physical states (e.g., Müller et al., 2014; Polkova et al., 2019) and for the biogeochemistry (e.g., Li et al., 2016).
Simulations
Three sets of simulations are involved in the downscaling prediction system: the reconstruction simulation, the ensemble of initialized simulations and the ensemble of uninitialized simulations. A schematic diagram showing the regional simulations as well as the relevant global simulations are illustrated in Figure 3.
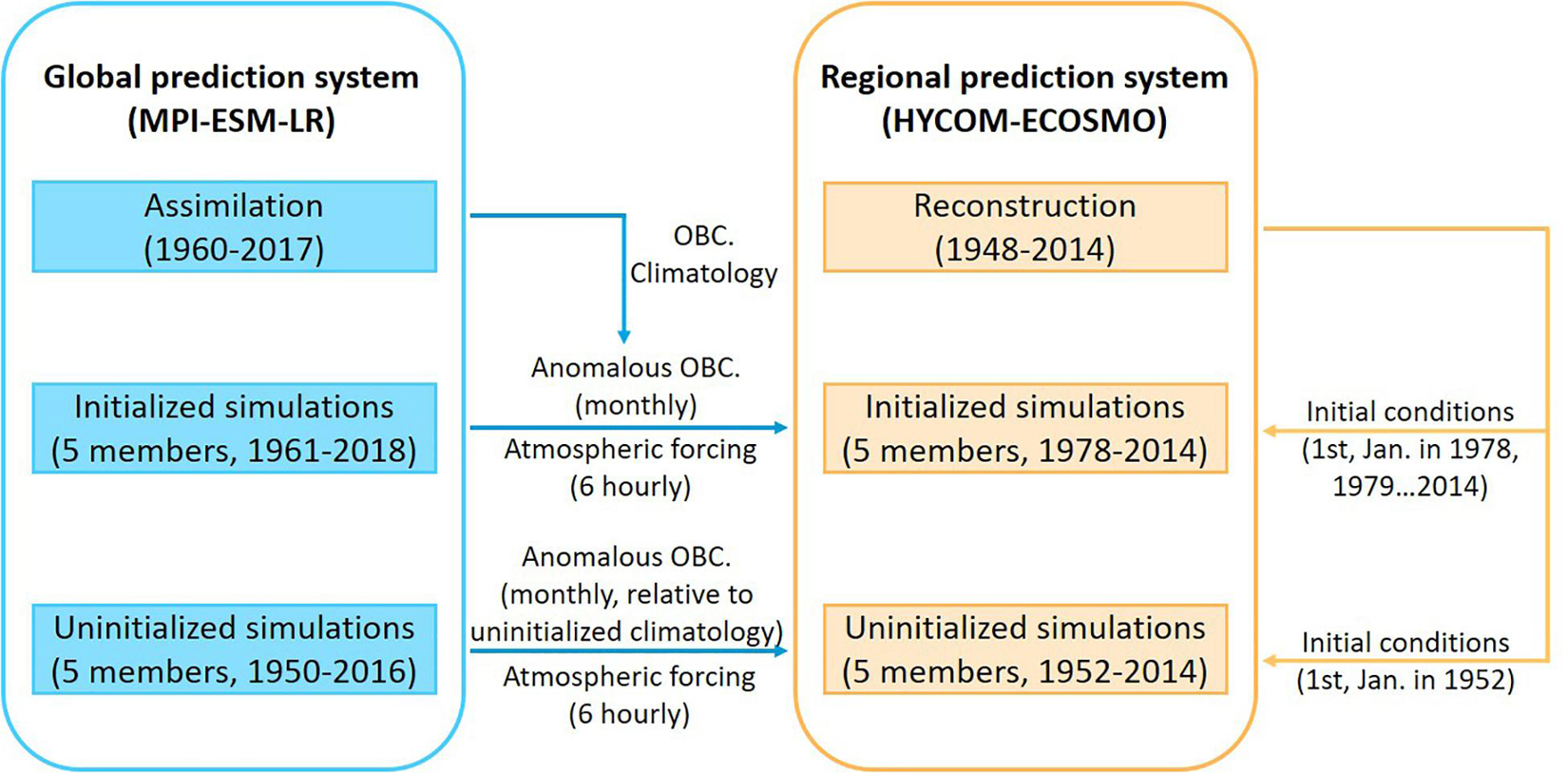
Figure 3. Schematic diagram describing how the three sets of simulations with the regional prediction system are built. Blue box: Ensembles of initialized and uninitialized simulations from the global prediction system (MPI-ESM-LR) provide 6-hourly atmospheric forcing and monthly anomalous open boundary conditions (OBC) to the corresponding ensembles of initialized and uninitialized simulations with the regional prediction system (HYCOM-ECOSMO). The anomaly fields of the initialized simulations from MPI-ESM-LR are based on the assimilation run, while the anomaly fields of the uninitialized simulations from MPI-ESM-LR are based on their own climatology. Orange box: Reconstruction with HYCOM-ECOSMO provides initial conditions for the initialized and uninitialized simulations within the same regional prediction system.
Based on the availability of the forcing data, the simulation period differs among the three sets of regional simulations. Results over a common period of 1985–2014 are used for analysis of the predictability. The three sets of simulations are described as follows:
(i) A 67-year (1948–2014) simulation (referred to “reconstruction simulation” hereafter) is used to provide a long, consistent time series of the environmental conditions at deep-sea sponge habitats in the North Atlantic. In this simulation, a global high-resolution atmospheric reanalysis product based on the atmospheric model ECHAM6 (Schubert-Frisius and Feser, 2015) is used to provide forcing to the regional model at the ocean surface. The modeled temperature, salinity and density at the open boundaries are relaxed toward climatological monthly fields from the Generalized Digital Environment Model (GDEM; Carnes, 2009), while the modeled nutrients (nitrate, phosphate, and silicate) and oxygen are relaxed toward World Ocean Atlas (WOA2013) monthly climatology (Garcia et al., 2013a, b). The simulation has been well validated by being compared with observations at the same location and on the same date (Samuelsen et al., 2019), providing us confidence that this simulation is able to reproduce the interannual to decadal variations of the environmental properties in the simulation period.
(ii) An ensemble of five initialized prediction simulations (referred to “initialized simulations” hereafter), is started from the reconstruction simulation on 1 January in each year over the period 1978–2014. Each simulation has a length of 8 years. Six-hourly atmospheric forcing is obtained from five out of ten randomly selected members of the initialized simulations of MPI-ESM-LR. In this implementation, the five regional simulations have identical initial conditions. Perturbations, represented by the differences of the atmospheric forcing among the five global members, quickly transfer to the regional ocean through the air-sea interactions. In order to include the effect of large-scale variability, which may propagate through the open boundaries into the regional model domain, the modeled temperature, salinity and density at the relaxation zones are relaxed toward the corresponding MPI-ESM-LR monthly fields of the initialized simulations. To avoid possible climatic shock between the reconstruction and the initialized simulations, we apply a bias-correction method to include both the observed climatology and the variability from the global prediction system mimicking the methods described in Pozo Buil et al. (2021) as follows:
where OBC stands for the open boundary conditions toward which the modeled fields are relaxed, OBCclim stands for the GDEM climatologically monthly fields, OBCanom. is the monthly anomaly of the MPI-ESM-LR initialized simulations, which is obtained by subtracting the climatologically monthly mean of the MPI-ESM-LR assimilation (Figure 3), i is the ensemble member from 1 to 5 and t is the month from 1 to 12. Boundaries of biogeochemical variables are relaxed toward the WOA2013 monthly climatology.
(iii) An ensemble of five uninitialized prediction simulations (referred to “uninitialized simulations” hereafter) is performed for the period 1952–2014, which is started from the reconstruction simulation on 1 January 1952 and forced with 6-hourly atmospheric data from five out of ten randomly selected members of the uninitialized simulations of MPI-ESM-LR. As our analysis focuses on the period of 1985–2014, we assume that the model has lost the memory of the initial conditions after more than 30 years of integration. The treatment of the open boundaries is the same as in the initialized simulations but with the calculation of the monthly anomalies based on the climatology of the MPI-ESM-LR uninitialized simulations.
The above three sets of regional simulations are used to assess the skill of retrospective predictions of the four variables. The results will provide confidence for a further attempt to forecast the future evolution of environmental conditions in the forthcoming years to one decade.
Study Sites
In this work, we focus on 21 sponge habitats in the North Atlantic (Figure 1). Those habitats represent good geographical coverage of the North Atlantic and a diversity of species (Table 1). The locations of those habitats are obtained from the EU Project ‘‘SponGES1”. They are first digitalized and then mapped on our model grids. Detailed information about the individual habitat, including the name, the dominant sponge species, the depth ranges, the sponge density, the topographical feature and the corresponding references is listed in Table 1. The main water masses that each sponge habitat is associated with are listed in Table 2.
Despite the fact that some areas are shallower than 200 m and may not represent the deep sea, they are still considered in the analysis because of their importance in hosting sponge habitats that are of great interest to the sponge communities. For instance, the Scotian Shelf off Nova Scotia (Habitat 2), with a range of depth from 75 m to 935 m (Table 1), is home to a globally unique arrogation of the glass sponge Vazella pourtalesii. Management measures to protect sponge habitats (and CWCs as well) have been implemented in this area such as bottom-fishery closures implemented by Fisheries and Oceans Canada (Breeze and Fenton, 2007; Beazley et al., 2021). Thus, assessments of the predictability of the environmental variables are meaningful. Sponge habitats in part of the Arctic Ocean are also considered. Note that the model depths at each habitat are not always in the same range with the observations (Table 1) because of the inconsistent locations between observations and the model, as well as that the model resolution is not sufficient to resolve the fine structures of the topography.
Analysis Methods
Our analysis is based on the annual mean values at the bottom layer of the water column over the sponge habitats. The bottom layer is represented by the last isopycnal layer of the model.
For the initialized simulations, we first construct time series from the 8-year long individual simulation as a function of lead time. For instance, the time series of lead year 1 is obtained by concatenating the first lead year of every 8-year long initialized simulation and thus covers a period of 1978–2014. This method applies to lead year 1 to 8, resulting in eight time series over 1978–2014, 1979–2015, …, 1985–2021, respectively. The time series of the anomalies in the common period 1985–2014 are used for the following statistical analysis. Anomalies of the three types of simulations are calculated by subtracting their respective climatological means. For the uninitialized simulations, the climatology is calculated from their ensemble mean, while for the initialized simulations with additionally respect to the lead time. The time series are all linearly detrended with the long-term trend being removed to emphasize the predictability in interannual to decadal variability.
Owing to the lack of adequate observational data to represent the interannual to decadal variability, the predictions are verified against the reconstruction simulation to quantify the potential predictive skills. In this way, we assume the modeled fields in the reconstruction simulation are proxies of the historical variability. Therefore, we present in this paper the potential predictability, an upper limit of the predictability, which is obtained by considering a perfect reconstruction of the reality (Boer et al., 2013). The comparison between the initialized and uninitialized simulations is used to assess the improvement of the prediction due to initialization.
We quantify the predictive skill with the anomaly correlation coefficient (ACC) and the normalized mean absolute error (NMAE). The ACC has an emphasis on evaluating the phasing of the predictions against the reconstruction and is calculated between the ensemble means of the prediction simulations (both for uninitialized and initialized simulations) and the reconstruction (Brady et al., 2020):
where x′ is the anomaly of the prediction ensemble mean, y′ is the anomaly of the reconstruction, and N is the number of years (30 years from 1985 to 2014). The ACC is a function of lead time in the initialized simulations. A nonparametric bootstrapping approach with 500 bootstrap samples following Goddard et al. (2013) is used to quantify the significance of the correlation skill with the null hypothesis that the two time series are uncorrelated.
The NMAE is used to assess the divergence between mean trajectories of the prediction simulations and reconstruction and is a measure of the accuracy in predicting anomaly magnitudes (Brady et al., 2020). It is the mean absolute error (MAE) of the ensemble mean relative to the reconstruction, then normalized by the interannual standard deviation of the reconstruction. MAE is used here instead of bias metrics such as the root mean square error (RMSE), as it is more accurate in assessing average model bias (Willmott and Matsuura, 2005).
where σ′y is the standard deviation of the reconstruction over the assessing period. NMAE increases as the prediction error grows. When NMAE < 1, the prediction error falls within the variability of the reconstruction and we determine that the prediction is accurate.
Based on the ACC and NMAE, we further define potential predictability horizon (referred to ‘predictability horizon’ hereafter) as the time at which skillful prediction is lost (Séférian et al., 2014). In this study, it is identified as the maximum lead year of the initialized simulations for which the ACC is significantly positive at 95% and the NMAE is significantly smaller than 1. Note that when determining the predictability horizon, we don’t stick to the comparison between the initialized and uninitialized simulations. The comparison of the two prediction simulations is used to assess the benefit of initialization.
Finally, we classify the sponge habitats into five categories based on the predictability horizon in the physical fields (temperature and salinity) and biogeochemical fields (concentrations silicate and oxygen). The five categories are:
1) High predictability in both physical (Phy.) and biogeochemical (BGC) fields (referred to “High Phy.&BGC”) – the predictability horizon is longer than (including) 4 years for at least one variable in each of the two fields (physical and biogeochemical);
2) High predictability only in biogeochemical fields (referred to “High BGC”) – the predictability horizon is longer than (including) 4 years for at least one variable in the biogeochemical fields but no such long predictability in the physical fields;
3) High predictability only in physical fields (referred to “High Phy.”) – the predictability horizon is longer than (including) 4 years for at least one variable in the physical fields but no such long predictability in the biogeochemical fields;
4) Moderate predictability in physical and biogeochemical fields (referred to “Moderate Phy.&BGC”) – predictability horizon in both two fields is less than 4 years, there are at least one variable in the two fields having predictability of longer than 2 years.
5) Low predictability in both physical and biogeochemical fields (referred to “Low Phy.&BGC”) – no variable has predictability of longer than 2 years.
Results
Potential Predictive Skill at the Lead Time of 2 Years
The potential predictability (referred to ‘predictability’ hereafter for simplicity) is first assessed with the predictive skill at a lead time of 2 years (Figures 4–6). The initialized simulations produce skillful predictions of bottom temperature, salinity, concentrations of silicate and oxygen at a large portion of sponge habitats, as illustrated by the maps of the ACCs (Figures 4A–D). The ACCs of the four variables share similar spatial patterns, with high (>0.8) ACCs in the subtropical area (Habitats 1, 19–21), the subpolar gyre (SPG, 60°W–10°W, 50°N–65°N) area (Habitats 6–8, 13–14) and along the North American and Greenland margin (Habitats 2–5). In those areas, the correlations with the initialized simulations are significantly higher than those with the uninitialized simulations, implying improved predictability due to initialization (Figure 4 and Supplementary Figure S1). In contrast, there are no significant correlation skills at sponge habitats along the European margin (Habitats 12, 15–18), as well as the Habitat 10 in the Nordic Sea in the initialized simulations, indicating no predictability at those areas. In the uninitialized simulations, the correlations over most of the sponge habitats are low and seldom significantly different from zero (Figures 4E–H). Exceptions are found in the SPG area and the Newfoundland and Labrador margin (Habitats 3–7, 14) for silicate and oxygen, as relatively high correlations emerge even without initializations (Figures 4G,H). The high correlations in the uninitialized simulations indicate that certain predictability in those two variables rises from external forcing or internal variations, and imply that the predictability in those two variables will be maintained to longer periods than temperature and salinity, which is corroborated later by the potential predictability horizon.
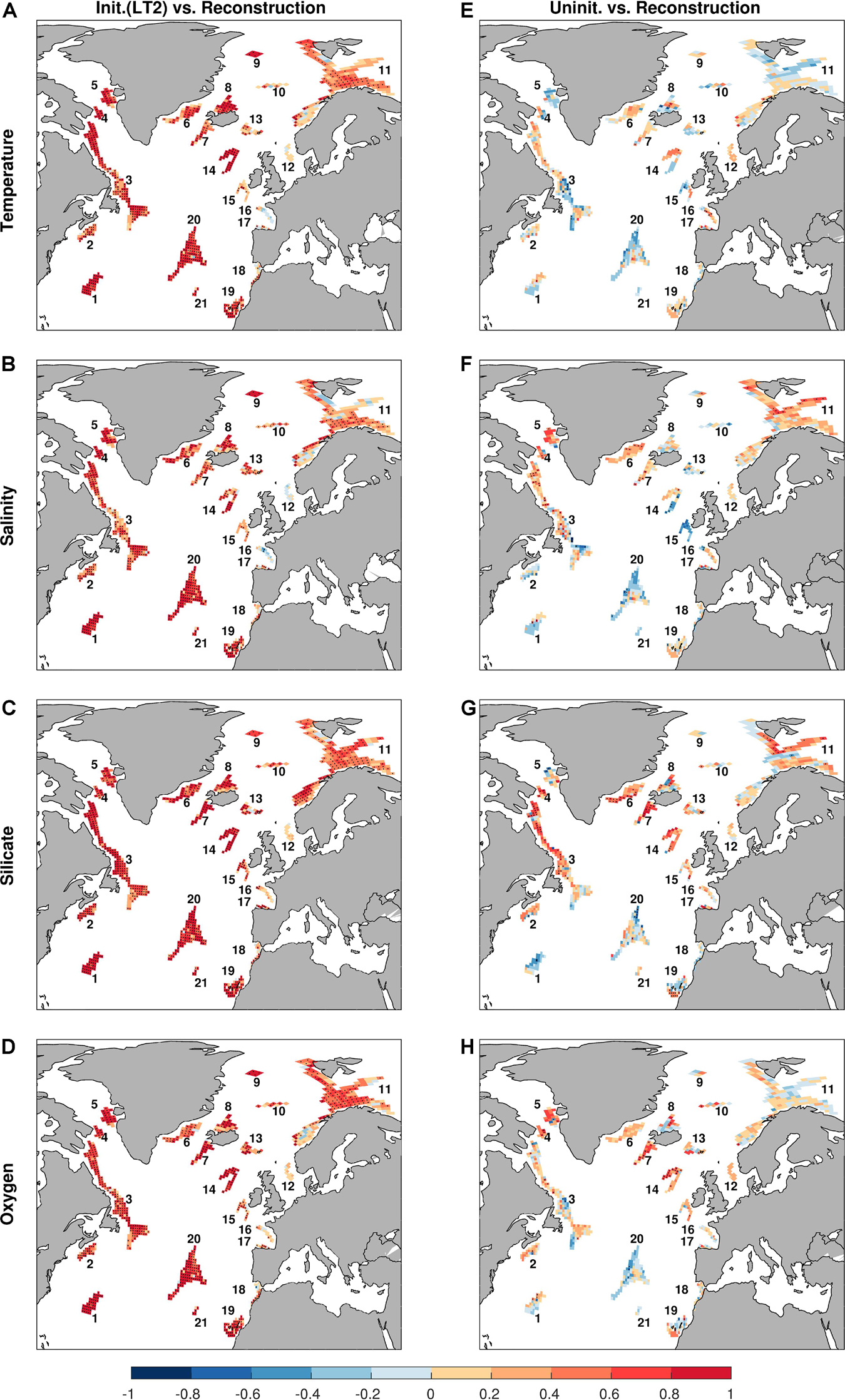
Figure 4. Anomaly correlation coefficients (ACCs) for temperature (A,E), salinity (B,F), silicate (C,G), and oxygen (D,H). Left column (A–D): correlations between the initialized simulations at a lead time of 2 years and the reconstruction. Right column (E–H): correlations between the uninitialized simulations and the reconstruction. Stippling denotes statistically significant correlations at the 95% level based on a bootstrap approach with 500 bootstrapped resamples.
The spatial distribution of the MAEs of the predictions against the reconstruction indicated by the NMAEs coincides with the ACCs, as high NMAEs are corresponding with low ACCs (Figures 4, 5). At sponge habitats along the North American margin and open waters of the Atlantic as well as along the African margin (Habitats 1–10, 13–14, 19–21) in the initialized simulations, the NMAEs are significantly smaller than 1 (Figures 5A–D), indicating that the mean error of the prediction primarily falls within the magnitude of the interannual variability. At those habitats, the prediction errors are smaller in the initialized simulations than in the uninitialized simulations, providing additional evidence for the improved predictive skill due to initialization (Figure 5 and Supplementary Figure S1). In contrast, the NMAEs at the sponge habitats along the European margin (Habitats 11–12, 15–18) are close to or even higher than 1 in both the initialized and uninitialized simulations, showing no significant benefit from initialization.
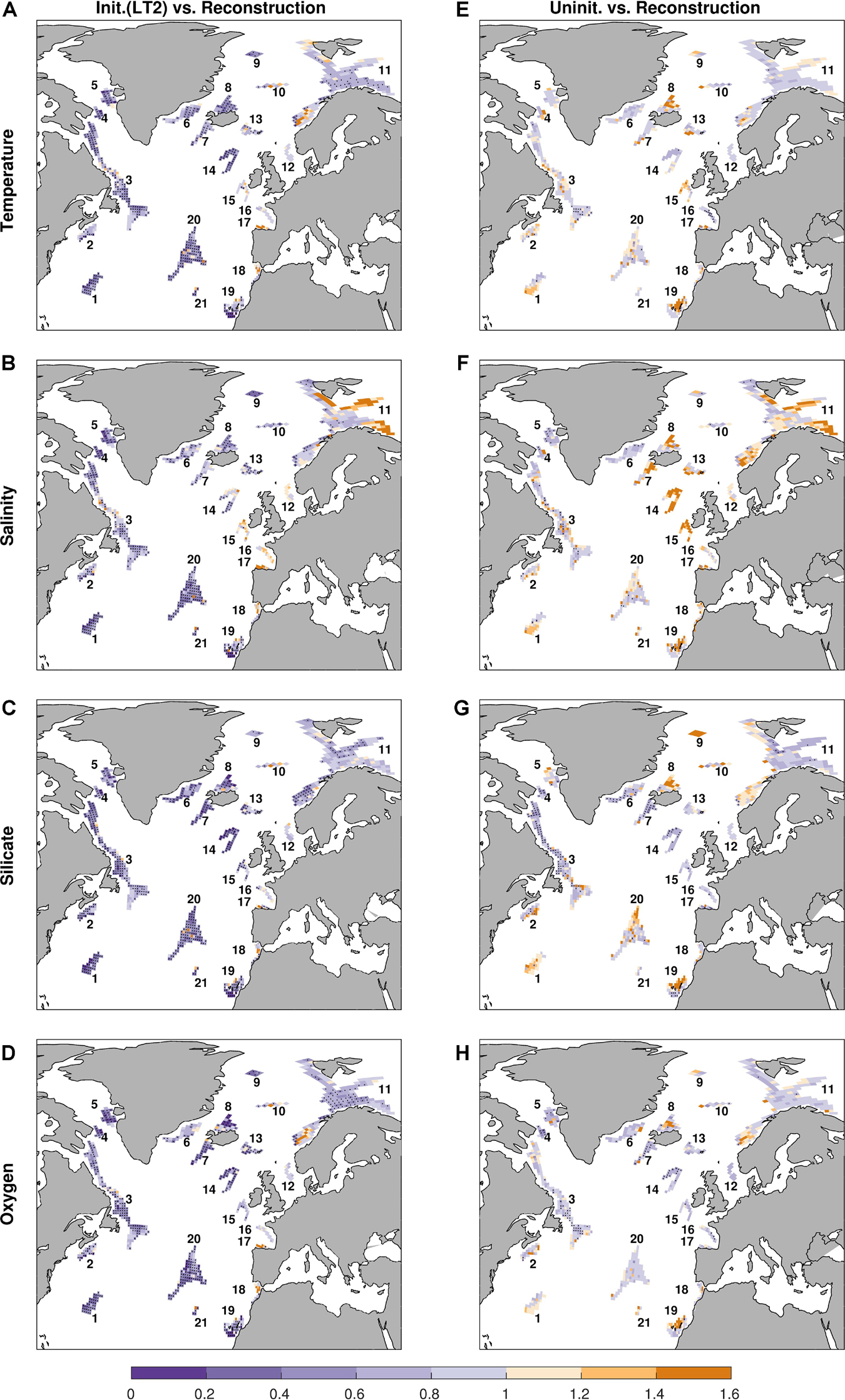
Figure 5. Normalized mean absolute errors (NMAEs) for temperature (A,E), salinity (B,F), silicate (C,G), and oxygen (D,H). Left column (A–D): NMAEs of initialized simulations at a lead time of 2 years. Right column (E–H): NMAEs of uninitialized simulations. Stippling denotes NMAEs less than 1 at the 95% significance level based on a bootstrap approach with 500 bootstrapped resamples.
The improved predictive skills of the interannual to decadal variations due to initialization is directly reflected by the detrended time series of the four variables (Figure 6). Here we show the time series at Habitat 11 and 14 (see Figure 1), dominated by interannual and decadal variability, respectively. We use the time series with a lead time of 2 years with the initialized simulations to analyze the source of the improved predictive skill. At Habitat 11, the temperature and oxygen are dominated by interannual variations (Figures 6A,D) as illustrated by the reconstruction. Such variations are well captured by the initialized simulations in terms of both the phasing and amplitude. In contrast, the uninitialized simulations fail to predict such variations but only show a smoother temporal evolution. For silicate, the simulated increase in the uninitialized simulations is delayed by about 10 years as compared with the reconstruction (Figure 6C). The weak or even absence of predictive skill in the uninitialized simulations indicates that the uninitialized simulations mostly capture only the long-term trend in response to the external forcing such as climate evolution caused by rising carbon emissions, and that the initialized simulations, in addition to tracking the long-term trend, are capable of maintaining the interannual to decadal variations for some years. The reconstructed variability of salinity is rather weak, varying within a limited range of ∼0.03. Neither the initialized nor the uninitialized simulations capture the variability well (Figure 6B). At Habitat 14, the time series of the variables demonstrate significant decadal variability (Figures 6E–H). Predictions with the initialized simulations agree well with those reconstructed decadal variations. The uninitialized simulations show chronological increasing/decreasing trends with maxima/minima at around the year 2000, leading to a biased phasing of the variations in particular before 2000.
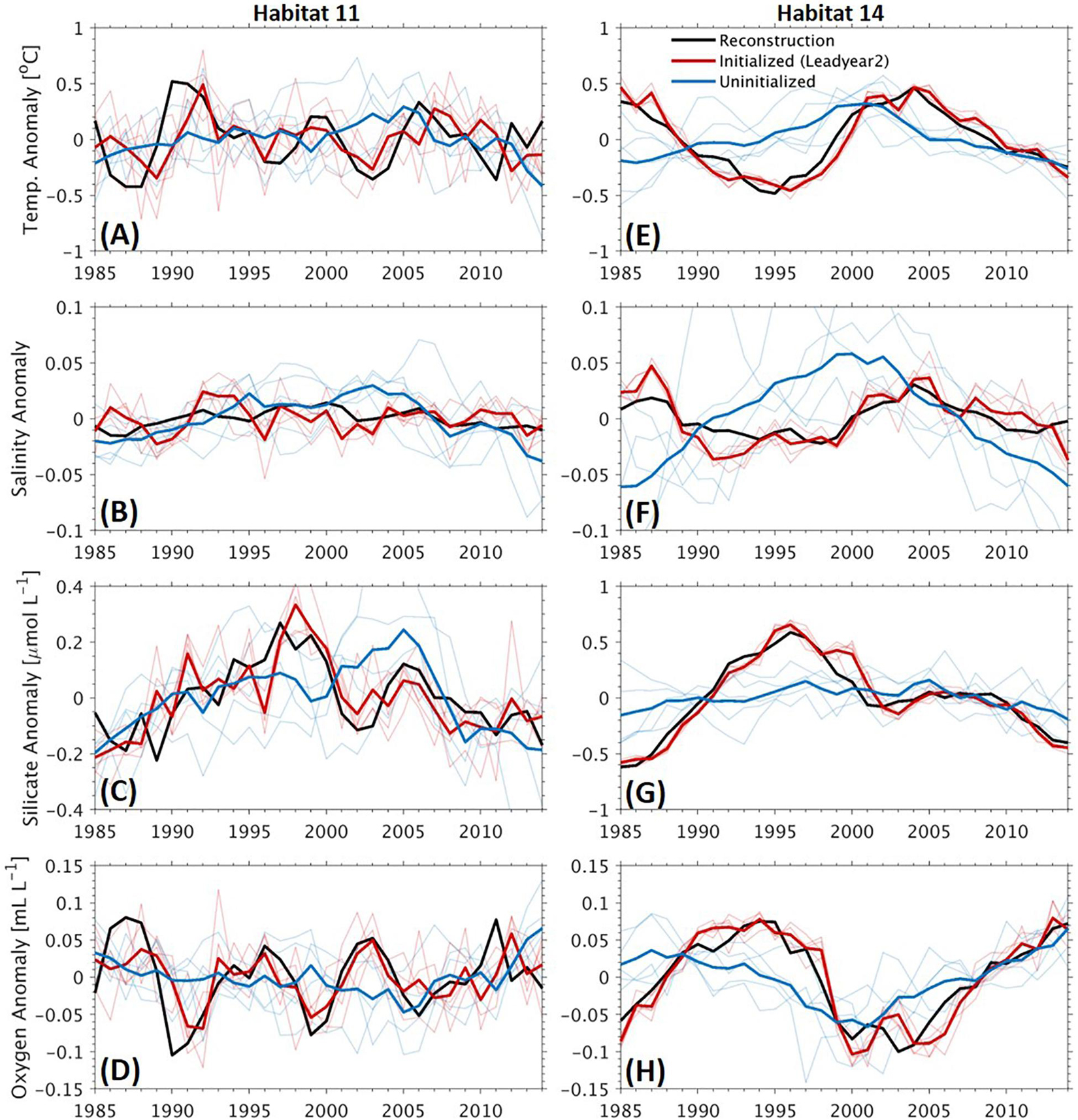
Figure 6. Time series of detrended anomalies of annual mean temperature (A,E), salinity (B,F), concentrations of silicate (C,G), and oxygen (D,H) at sponge habitats 11 (A–D) and 14 (E–H). Bold black lines: reconstruction, bold red/blue lines: ensemble means of the initialized/uninitialized simulations at a lead time of 2 years, thin red/blue lines: individual members of the initialized/uninitialized simulations. The locations of the two habitats can be seen in Figure 1.
Potential Predictability Horizon
The predictability of the four variables degrades along with lead times, as is evidenced by the comparison of time series at lead times of 2 years (Figure 6) and of 4 years (Figure 7). After 2 years, the initialized simulations, on the one hand, lose their ability to capture the variations in the reconstruction (e.g., temperature and oxygen at Habitat 11 at lead year 4, Figures 7A,D) and, on the other hand, generate artificial variations that do not exist in the reconstruction (e.g., salinity at Habitat 14 at lead year 4, Figure 7F). The predictability of the low-frequency (decadal to multi-decadal) variations is retained longer than the high-frequency (interannual to multi-year) variations. The degradation of the predictability is also evidenced in the decreasing of the ACCs and the increasing of the NMAEs along with the lead times (Figures 8, 9).
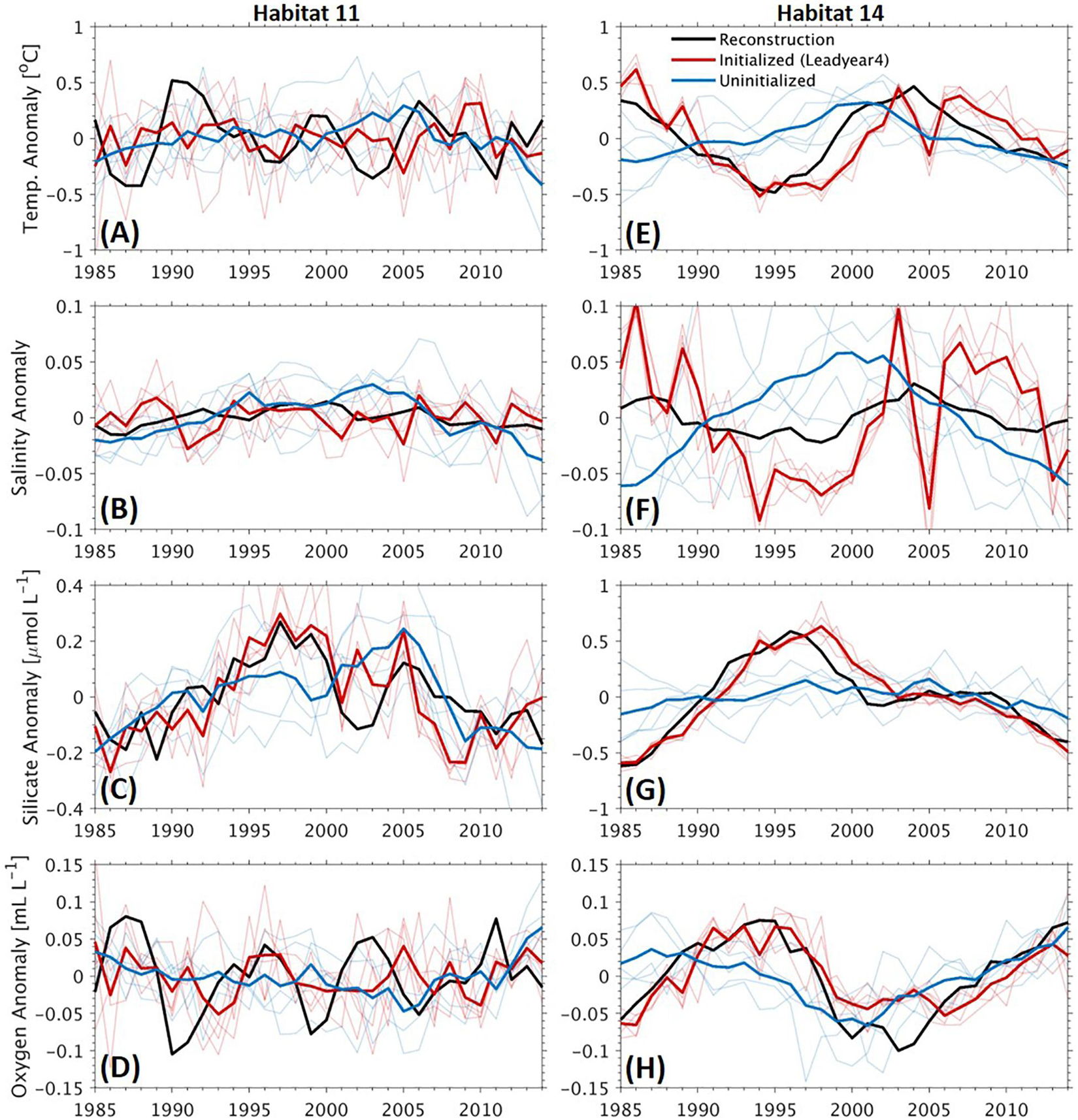
Figure 7. Time series of detrended anomalies of annual mean temperature (A,E), salinity (B,F), concentrations of silicate (C,G), and oxygen (D,H) at sponge habitats 11 (A–D) and 14 (E–H). Bold black lines: reconstruction, bold red/blue lines: ensemble means of the initialized/uninitialized simulations at a lead time of 4 years, thin red/blue lines: individual members of the initialized/uninitialized simulations. The locations of the two habitats can be seen in Figure 1.
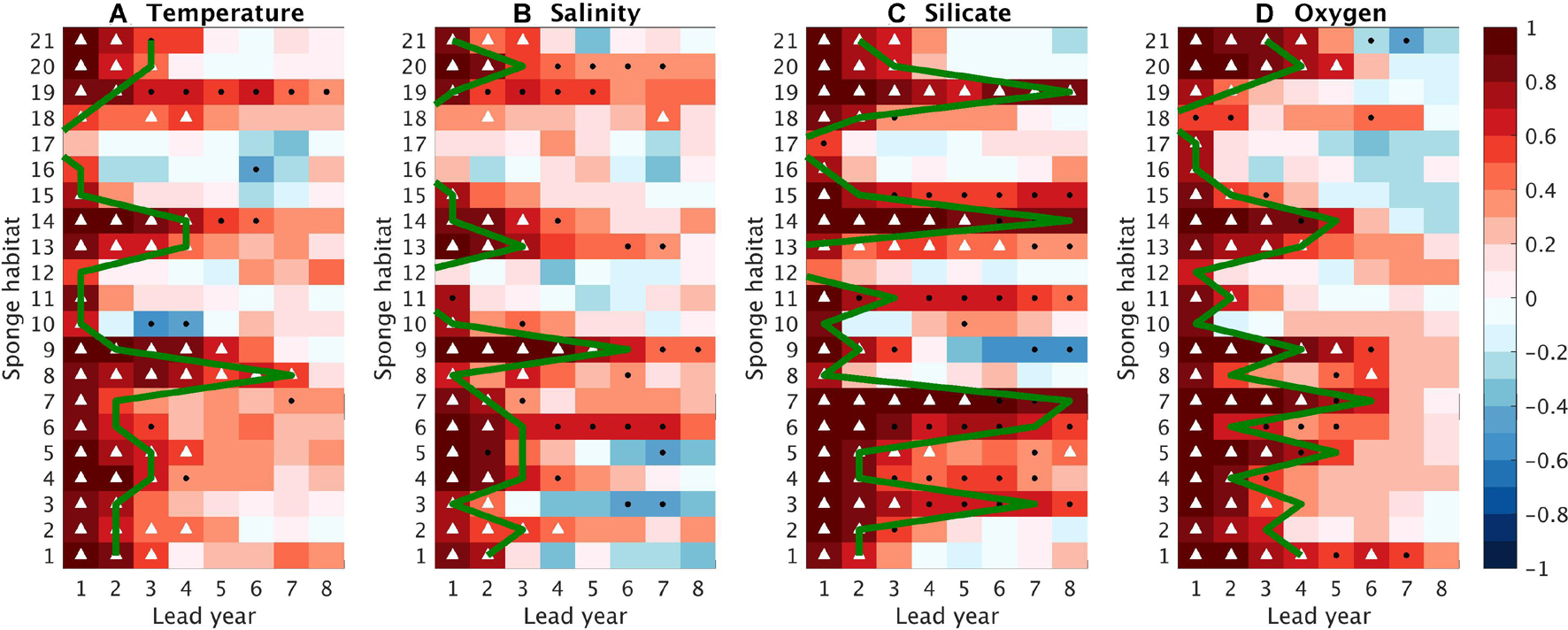
Figure 8. Anomaly correlation coefficients (ACCs, in colors) as a function of lead time (x axis) and sponge habitat (y axis) for (A) Temperature, (B) Salinity, (C) Silicate, and (D) Oxygen. White triangles indicate that the ACCs are significant and significantly exceed the ACCs with the uninitialized simulations; black dots denote that the ACCs are significant but not significantly larger than the uninitialized simulations. Green lines indicate the predictability horizon, which is defined as the last lead year when ACCs are significant and the NMAEs are significantly smaller than 1. Significance is tested based on a bootstrap approach with 500 bootstrapped resamples.
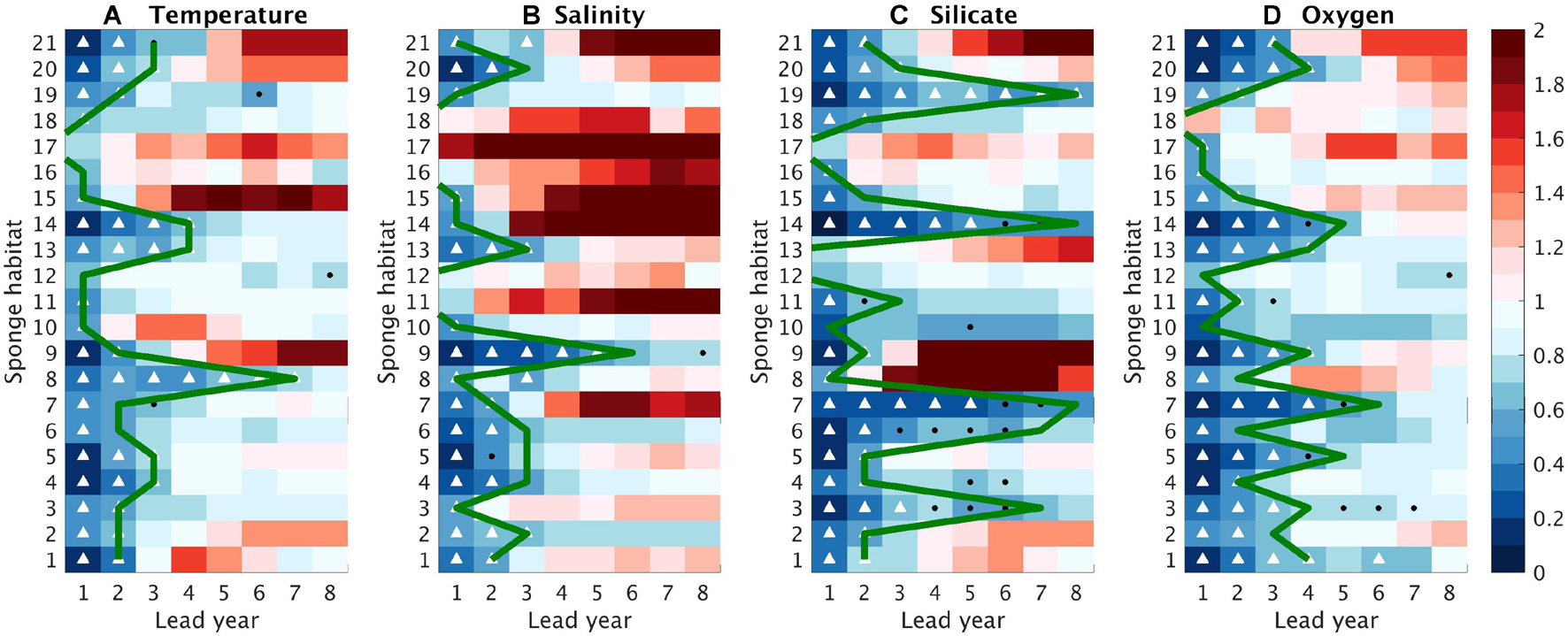
Figure 9. Normalized mean absolute errors (NMAEs) as a function of lead time (x axis) and sponge habitat (y axis) for (A) Temperature, (B) Salinity, (C) Silicate, and (D) Oxygen. White triangles indicate that the NMAEs are significantly smaller than 1 and significantly smaller than the NMAEs with the uninitialized simulations; black dots denote that the NMAEs are significantly smaller than 1but not significantly smaller than the uninitialized simulations. NMAE > 1 is considered as no predictability. Green lines, identical with the green lines in Figure 8, indicate the predictability horizon, which is defined as the last lead year when ACCs are significant and the NMAEs are significantly smaller than 1. Significance is tested based on a bootstrap approach with 500 bootstrapped resamples.
The predictability horizon of the four variables from the initialized simulations varies among sponge habitats and variables (Figures 8, 9). In this study, the predictability horizon is more constrained by the NMAEs. For instance, high ACCs with 95% significance last until the lead time of 4 years for salinity at Habitats 14, but the predictability horizon is only 1 year because of the rapid increase of NMAE (Figures 8, 9). We find robust high/low predictability of the environmental properties at certain sponge habitats, e.g., at Habitat 14, three out of the four variables can be predicted up to 4 years in advance while at Habitat 17, the predictive skills for all the four variables are lost within 2 years. In addition, we find that in a large part of the sponge habitats (e.g., Habitats 1–3, 5–7, 11, 14–15, 19–21), the predictability last longer in the biogeochemical fields than in the physical fields.
The map of the categories illustrates a distinct regional difference in the predictability (Figure 10). High predictability, defined as predictability horizon of more than 4 years, exists in both physical and biogeochemical fields at two habitats inside the North Atlantic SPG (Habitats 13, 14) and one on the East Greenland Shelf (Habitat 9). High predictability of the biogeochemical fields is also found at numbers of habitats either spreading along the western margins (Habitats 3, 5–7) or in the open ocean (Habitats 1, 20) with additional one along the African margin (Habitat 19). For those habitats, the predictability of the physical fields is less than 4 years. In contrast, at Habitat 8, north of the Iceland, the physical fields can be predicted more than 4 years ahead while the biogeochemical fields are less predictable. Some habitats (Habitats 2, 4, 11) located in the marginal seas are characterized by moderate predictability, as at least one field can be predicted more than 2 years in advance but none of the variables are skillfully predictable for more than 4 years. There are six habitats, with five located in the European margin (Habitats 12, 15–18) and one located in the open Nordic Sea (Habitat 10), subject to low predictable environmental conditions, as both the physical and biogeochemical fields lose their predictive skill quickly within 2 years.
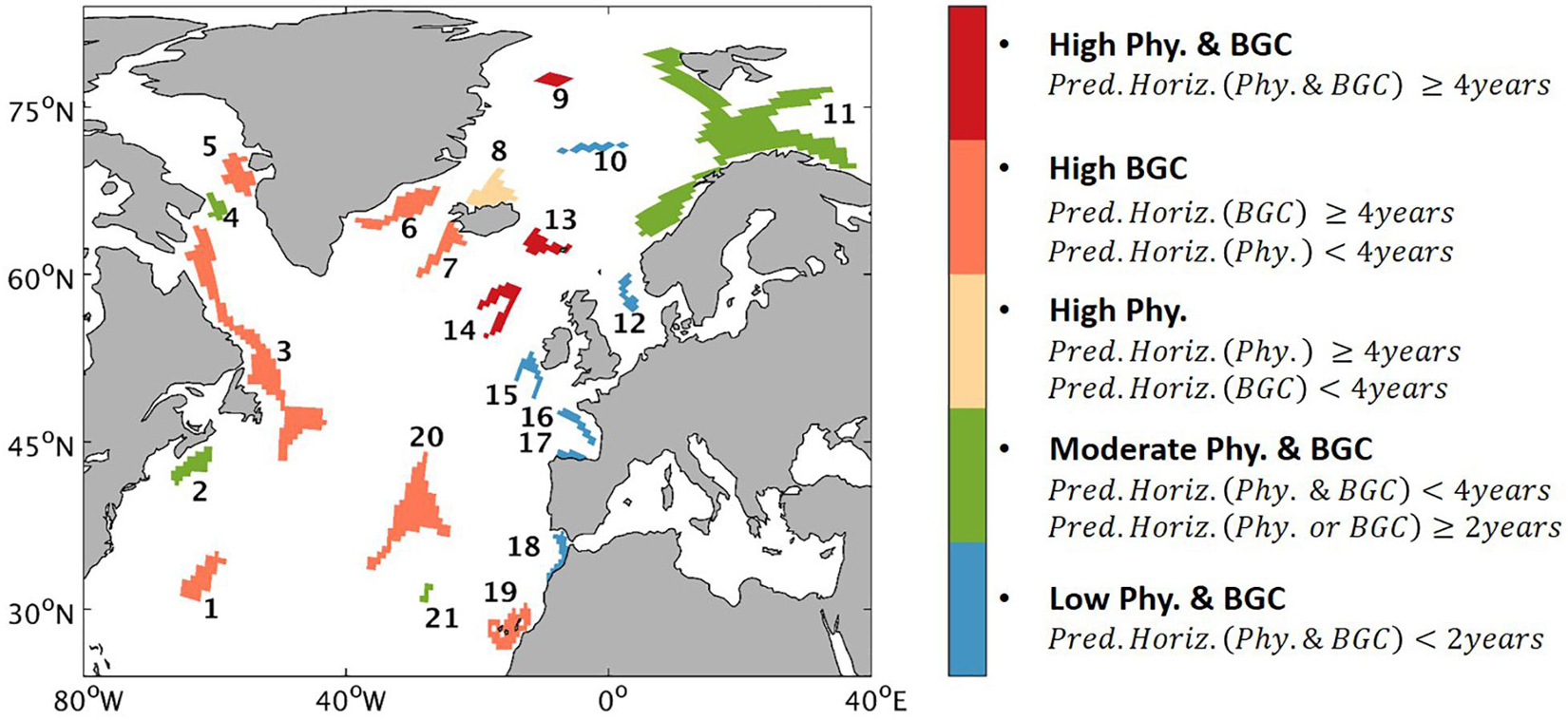
Figure 10. Map of the sponge habitats showing the five categories of predictability. (1) High predictability in both physical (Phy.) and biogeochemical (BGC) fields (referred to “High Phy.&BGC”) – the predictability horizon is longer than (including) 4 years for at least one variable in each of the two fields (physical and biogeochemical). (2) High predictability only in biogeochemical fields (referred to “High BGC”) – the predictability horizon is longer than (including) 4 years for at least one variable in the biogeochemical fields but no such long predictability in the physical fields. (3) High predictability only in physical fields (referred to “High Phy.”) – the predictability horizon is longer than (including) 4 years for at least one variable in the physical fields but no such long predictability in the biogeochemical fields. (4) Moderate predictability in physical and biogeochemical fields (referred to “Moderate Phy.&BGC”) – predictability horizon in both two fields is less than 4 years, there are at least one variable in the two fields having predictability of longer than 2 years. (5) Low predictability in both physical and biogeochemical fields (referred to “Low Phy.&BGC”) – no variable has predictability of longer than 2 years.
Discussion
Mechanisms Underlying the Spatial Patterns of the Predictability
The sponge habitats involved in this study are subject to a diversity of geographical and geomorphological features and depths (Table 1), which are associated with substantial spatial variability in their hydrographic settings like water masses, local circulation patterns, interactions with upper oceans. Those differences suggest different underlying mechanisms describing/limiting the predictability.
High predictability for both the physical and the biogeochemical fields is found at sponge habitats in the SPG area (Habitats 13 and 14). A close coherence between the winter North Atlantic Oscillation (NAO) index and decadal changes in the deep-water temperature and salinity in the SPG area was identified during 1950–2000, which was attributed to the NAO-induced regulation of the relative contribution of cold fresh subpolar water and warm saline subtropical water to the deep-water formation (Sarafanov, 2009). The dominating water masses (e.g., Northeast Atlantic Deep Water) in these areas (Table 2) are integral to the Atlantic meridional overturning circulation (AMOC) (Rhein et al., 2011). This implies that the high predictive skills of the deep-sea environmental properties are largely prompted by NAO-AMOC related climate variability. In addition, we notice that Habitat 9, which is shallow with a mean depth of 200 m, free from the AMOC influence and covered by sea ice most of the year (Rudels, 1995), is characterized by high predictability for both the physical and biogeochemical fields. This skillful predictability arises probably from sea ice processes, as suggested by Jacox et al. (2020) that persistence of sea ice can cascade into predictability of the marine ecosystems.
Low predictability is found in the Gulf of Cadiz (Habitat 18), a region under the influence of the Mediterranean outflow. In the regional model, the simulated Mediterranean outflow is constrained by the restoring fields provided by the global predictions, as the eastern open boundary is placed near the Strait of Gibraltar (Figure 2). However, the water masses of the Mediterranean outflow are not well described by the global model because of its low resolution in the Mediterranean Sea (Marsland et al., 2003; Jungclaus et al., 2013; Malanotte-Rizzoli et al., 2014). The bias carried by the global model further propagates into the North Atlantic along the pathway of the Mediterranean outflow from the Strait of Gibraltar (Habitat 18) into the Bay of Biscay (Habitats 16, 17) and further reaches the southwest of Porcupine bank (Habitat 15) through a combination of narrow slope currents and mixing processes with the North Atlantic Current (Iorga and Lozier, 1999a, b; Bower et al., 2002), which in turn leads to low predictability of the environmental properties in those areas (Habitats 15–17). An opposite case is the Habitat 19, which receives less influence from the Mediterranean outflow (Iorga and Lozier, 1999a, b; Pastor et al., 2012). As a result, both the physical and biogeochemical fields at this site show high correlations between the initialized simulations with the reconstruction, albeit that the predictability of temperature and salinity is more constrained by high NMAEs.
In the lower layers of the ocean, a vigorous coupling with the upper ocean will likely degrade the predictability of the variations of the bottom environment, since the unpredictable signal of the atmosphere will cascade into the lower layers via the vertical exchange (e.g., Boer, 2004; Fransner et al., 2020). Thus, the low predictability at Habitat 12, the shallowest area among the 21 habitats, is attributed to the mean depth of 93 m, which suggests a profound impact of the atmospheric forcing through strong mixing between the ocean surface and bottom (Mathis et al., 2015). Although this habitat is influenced by the Atlantic inflow, which is found significantly correlated with large-scale atmosphere circulation NAO (Hjøllo et al., 2009), predictability is not sustained long in this area.
In Habitat 10 (near the Schulz Bank), the bottom ocean is occupied by the Norwegian Sea Deep Water (Roberts et al., 2021). This water mass, a mixture of relatively cold and fresh Greenland Sea Deep Water with warmer, saltier Eurasian Basin Deep Water from the Arctic Ocean, can be traced only for a short distance (Swift and Koltermann, 1988). In addition, the mixture is highly variable, as the formation of the Greenland Sea Deep Water is depending on the convective processes that are highly associated with fluctuating atmospheric conditions (Blindheim and Rey, 2004). As a result, the water properties are less predictable and show low predictability.
Over a large fraction of the habitats, the predictive skills for the biogeochemical fields are higher than for the physical fields. Similar findings in the upper ocean have been reported by several studies (e.g., Séférian et al., 2014; Li et al., 2016). The reasons for this finding are under investigation. Enhanced persistence likely plays a role. In this study, the persistence of silicate, estimated to 3 years, is longer than that of temperature and salinity maintaining only to 1 year, suggesting a longer memory of silicate variations than the physical fields in the deep ocean (Supplementary Figure S2).
It is suggested that the deep ocean is expected to be more predictable than the surface layer, supported by the explanation that the deep ocean is not directly coupled to the high-frequency atmosphere, the variability of which is relatively unpredictable (Frölicher et al., 2020). The increasing of the predictability with depth is evidenced on global or basin scales within a perfect modeling framework (Frölicher et al., 2020). However, this does not guarantee high predictability of the deep sea on smaller spatial scales such as at sponge habitats in this study. The spatial patterns of the predictability (Figures 8–10) reveal that different regional processes operating on a range of spatio-temporal scales are playing important roles in controlling/limiting the predictability. A good understanding of the local processes such as circulation patterns, mass transport and interactions with atmosphere/sediment helps to explain the predictability and thus to provide insights into the possible improvement of the current prediction systems. For instance, it is widely realized that the global coupled models don’t capture the spatial scales required to correctly represent the Mediterranean ocean climate (Somot et al., 2008; Sasaki et al., 2020), since this climate is strongly driven by regional-scale topography and local winds, which are not resolved until the spatial resolution reaches certain values (Li, 2006). Therefore, an extension of the regional model domain to cover the whole Mediterranean Sea with an increased horizontal resolution is a promising way to better describe the water properties of the Mediterranean outflow and thus will probably improve the ability to predict the environmental factors at Habitats 15–18.
Prediction Into the Future
In this study, our analysis is based on a retrospective prediction (hindcast) in the past 30 years. We gain confidence from the analysis that our regional prediction system can be used to predict deep-sea environmental conditions in the near future, which is important for evaluating whether the environmental conditions are moving beyond the levels of ‘typical’ or natural variability that the sponges may have adapted to tolerate (Roberts et al., 2021). Currently the extension of the historical prediction into the future is limited by the length of the atmospheric forcing used to drive the reconstruction, which ends in the early 2015. It is promising to apply the prediction system into the future once the continued production of the atmospheric forcing with the related model is ready (Frauke Feser, personal communication).
Benchmark of Observation-Based Assessment
In assessing predictive skills, we are challenged by the lack of observations. The potential predictability, as deduced by comparing the prediction simulations against the reconstruction, is the upper limit of the predictability horizon with a given prediction system (e.g., Séférian et al., 2014). Such predictability horizons are hardly obtained in a real forecast, since it would require a perfect initialization and nudging of all fields in the model (Boer et al., 2013). Alternatively, the effective predictability horizon, requiring observationally based products to verify the prediction skill (Penny et al., 2019), provides a more restrictive and unbiased estimate of the predictability (Séférian et al., 2014).
However, the amount of observations in the deep sea, especially in specific benthic habitat, is constrained due to the high cost and limited technological capabilities (Levin et al., 2019). While there are short-term monitoring time-series at some habitats (e.g., Hanz et al., 2021), long-term monitoring time series are missing at most sponge habitats. The existing collective database, such as World Ocean Database (WOD, Boyer et al., 2018) and GLODAPv2 (Olsen et al., 2016) are possible sources of long-term time series. However, caution should be taken when interpreting those data since available observations are usually not equally or even normally distributed in space nor in time (Schrum et al., 2006). For instance, the variability displayed by the time series of the bottom temperature at Habitat 14 extracted from WOD, is mainly associated with the depths and dates of the observations, as high temperatures are coincided with shallow depths (Supplementary Figure S3). The variability shown by this time series is therefore not representative of the interannual variability, which can not be simply compared with the modeled annual mean. In the model validation section of Samuelsen et al. (2019), the station observations were compared directly to the model result from the same date and location to avoid the issue discussed above. It is also demonstrated by IPCC 6th Assessment Report that the estimated predictive skill can be degraded by errors in observational dataset used for verification, suggesting that skill may tend to be underestimated, particularly for variables whose observational uncertainties are relatively large (Lee et al., 2021). In this sense, we don’t show the comparison of the prediction against observations.
Implications of the Prediction System in Deep-Sea Benthic Habitats Preservation and Management
The assessment in this study provides a synoptic view of the spatial patterns of the predictability of deep-sea environmental factors over the widespread sponge habitats in the North Atlantic, providing the sponge community an indication to what extent the prediction of the environmental conditions can be used. For instance, reconstructed decadal variations of the four variables at the Rockall Bank (Habitat 14) demonstrate a tendency of the sponge habitat toward a status of being exposed to multiple stressors (simultaneous warming and decreasing silicate and oxygen concentrations) during 1995–2005. Although no negative impact of such change on the sponge habitat was documented in the past, we deduce that similar changes might occur in the future and are likely to be intensified by anthropogenic-induced climate change. Those changes in the near future might lead to a possibility that the environmental conditions move beyond the historical natural variability and become severe threats to the sponges. The high predictability of 4 years allows an effective prediction of such possible changes. Once the phase of change is predicted as continuously increasing/decreasing in temperature and salinity/silicate and oxygen in the coming 4 years, alert can be provided to the related management. This is of particular importance also for other benthic habitats living there, for instance the CWC reefs, the distribution of which are closely linked with environmental variations such as large-scale circulation patterns that can be traced by oceanographical variables (Schulz et al., 2020). In recent years, marine heat waves have been reported more frequently under climate change (Frölicher et al., 2018). The marine heat waves might affect deep-sea ecosystems, particularly for the CWCs and sponges inhabiting shallower waters in the northern areas of the North Atlantic such as the Norwegian area (Habitat 12) (Guihen et al., 2012). Despite the low predictability of temperature (1 year) in that region (Figures 8–10), it is still meaningful to predict the bottom temperature 1 year ahead to identify the possible occurrence of marine heat waves and mass mortality events, thus allowing reactions to mitigate damage.
Species distribution models, predicting the possible degree of habitats loss or gain under future climate change, offer a powerful tool in the deep sea for the effective management and conservation (Pearman et al., 2020). Those predictive models, based upon species-environment relationships, require accurately predicted environmental factors as important predictor variables (Guisan and Zimmermann, 2000). However, many knowledge gaps remaining in the biological processes and oceanographic dynamics hamper the models’ credibility (Puerta et al., 2020). In particular, the use of present-day database (e.g., World Ocean Atlas) or the long-term climate projection to provide oceanographic parameters to the species distribution models are likely to raise additional sources of uncertainty (Burgos et al., 2020). Several studies have emphasized the importance of incorporating oceanographic data to improve the predictive capability of benthic species distribution models (e.g., Pearman et al., 2020). Environmental variables required by the habitats prediction vary among species, locations and models (e.g., Knudby et al., 2013; Beazley et al., 2018; Morato et al., 2020; Pearman et al., 2020). In addition, it is suggested that the deep-sea ecosystems are likely to be impacted by multiple stressors (Büscher et al., 2017). Our downscaling prediction system describes more environmental variables than we analyzed here, e.g. currents, other nutrients (nitrate, phosphate), primary production, pH, particulate organic carbon flux and so on. Therefore, the system has potentials to offer predictions for more variables and locations, and thus facilitate the prediction of a wider range of deep-sea benthic species like CWC reefs which are also prevailing in the North Atlantic for example on the Rockall Bank (Habitat 14). In that sense, our prediction will finally help to set up management strategies like how to improve the current deep-sea management tools, e.g., ABMTs (Johnson et al., 2018) and how to adapt the sustainable development to climate changes (Armstrong et al., 2019).
Conclusion
We have made an attempt for the first time to assess the potential predictability of the interannual to decadal variations of the deep-sea environmental conditions in the North Atlantic under the ongoing climate change. The assessments are based on a novel operational downscaling regional decadal prediction system dedicated to the deep-sea dynamics. Model results of four environmental parameters (temperature, salinity, concentrations of silicate and oxygen) over 21 sponge habitats are used as an example to assess the predictability. Our results demonstrate the potential of the regional model to predict the environmental conditions multiple years in advance in the deep North Atlantic Ocean.
Based on our analysis, we draw the following conclusions:
1. Variations of both physical (temperature and salinity) and biogeochemical (concentrations of silicate and oxygen) conditions are potentially predictable several years in advance.
2. The predictability of the four variables is subject to distinct regional differences, with the potential predictability horizon varying from less than 2 years for all variables at sponge habitats along the European margin to more than 4 years in the SPG area and the Arctic Ocean.
3. In areas with high predictability, the variations of the environmental conditions are associated with ocean overturning structures (e.g., AMOC) that are regulated by large-scale climate variability such as the NAO or with the persistence of sea ice, while in areas with low predictability, the deep-sea environmental variations are under remarkable influence of the atmosphere or the local circulations such as the Mediterranean outflow.
4. The biogeochemical fields are more predictable than the physical fields at a large portion of the sponge habitats, partly due to the longer persistence of biogeochemical fields than the physical counterparts.
5. Predictability is significantly improved by initialization in areas with weak air-sea coupling and areas free from the influence of the Mediterranean outflow.
Overall, our analysis demonstrates the capacity of the downscaling regional system for skillfully predicting the variations of the environmental conditions at deep-sea sponge habitats in the near future. The assessment of the predictability can be applied to other ocean parameters (e.g., pH and other nutrients) and at other benthic habitats (e.g., CWC reefs). The downscaling prediction system can therefore help to improve management in the deep-sea ecosystems on interannual to decadal timescales.
Data Availability Statement
The raw data supporting the conclusions of this article will be made available by the authors, without undue reservation.
Author Contributions
CS, UD, and FL designed this study. FL, CS, UD, SB, and JB developed the downscaling prediction system and designed the simulations. FL conducted the numerical simulations supported by AS and UD. The analysis was performed by FL supported by SB and CS. The result interpretation and discussion were conducted by FL and UH. Inputs for the regional model from MPI-ESM-LR simulations were provided by HP. FL led the writing of the manuscript with inputs from all co-authors, who discussed the results and commented on the manuscript at every stage. All authors contributed to the article and approved the submitted version.
Funding
FL and AS are supported by project SponGES (Grant Agreement No. 679849). JB and CS are supported by project CLICCS (Grant Agreement No. 390683824). JB and SB are supported by Copernicus (contract C3S-330). The MPI-ESM-LR simulations were performed within the German Federal Ministry for Education and Research (BMBF) project MiKlip under Grant Number FKZ 01LP1519A.
Conflict of Interest
The authors declare that the research was conducted in the absence of any commercial or financial relationships that could be construed as a potential conflict of interest.
Publisher’s Note
All claims expressed in this article are solely those of the authors and do not necessarily represent those of their affiliated organizations, or those of the publisher, the editors and the reviewers. Any product that may be evaluated in this article, or claim that may be made by its manufacturer, is not guaranteed or endorsed by the publisher.
Acknowledgments
This research has been performed in the scope of the SponGES project, which received funding from the European Union’s Horizon 2020 Research and Innovation Program under Grant Agreement No. 679849. The study is a contribution to the Deutsche Forschungsgemeinschaft (DFG, German Research Foundation) under Germany’s Excellence Strategy—EXC 2037 “Climate, Climatic Change, and Society” (CLICCS)—Project: 390683824, and a contribution to the Center for Earth System Research and Sustainability (CEN) of Universität Hamburg. JB and SB are supported by Copernicus Climate Change Service, funded by the EU, under contract C3S-330. The MPI-ESM-LR simulations were performed within the German Federal Ministry for Education and Research (BMBF) project MiKlip under Grant Number FKZ 01LP1519A. Computational resources were made available by the German Climate Computing Center (DKRZ). We thank Dr. Hongmei Li and Dr. Vimal Koul for their big help in analyzing the model results. We thank the two reviewers for their constructive and helpful comments for improving the manuscript.
Supplementary Material
The Supplementary Material for this article can be found online at: https://www.frontiersin.org/articles/10.3389/fmars.2021.703297/full#supplementary-material
Footnotes
References
Addamo, A. M., Vertino, A., Stolarski, J., García-Jiménez, R., Taviani, M., and Machordom, A. (2016). Merging scleractinian genera: the overwhelming genetic similarity between solitary Desmophyllum and colonial Lophelia. BMC Evol. Biol. 16:108. doi: 10.1186/s12862-016-0654-8
Armstrong, C. W., Vondolia, G. K., Foley, N. S., Henry, L. A., Needham, K., and Ressurreição, A. (2019). Expert assessment of risks posed by climate change and anthropogenic activities to ecosystem services in the deep North Atlantic. Front. Mar. Sci. 6:158. doi: 10.3389/fmars.2019.00158
Barthel, D., Tendal, O. S., and Thiel, H. (1996). A wandering population of the hexactineliid sponge pheronema carpenteri on the continental slope off morocco, Northwest Africa. Mar. Ecol. 17, 603–616. doi: 10.1111/j.1439-0485.1996.tb00420.x
Beazley, L. I., Kenchington, E. L., Murillo, F. J., and Sacau, M. D. M. (2013). Deep-sea sponge grounds enhance diversity and abundance of epibenthic megafauna in the Northwest Atlantic. ICES J. Mar. Sci. 70, 1471–1490. doi: 10.1093/icesjms/fst124
Beazley, L., Kenchington, E., Yashayaev, I., and Murillo, F. J. (2015). Drivers of epibenthic megafaunal composition in the sponge grounds of the Sackville Spur, northwest Atlantic. Deep Sea Res. 1 Oceanogr. Res. Pap. 98, 102–114. doi: 10.1016/j.dsr.2014.11.016
Beazley, L., Wang, Z., Kenchington, E., Yashayaev, I., Rapp, H. T., Xavier, J. R., et al. (2018). Predicted distribution of the glass sponge vazella pourtalesi on the scotian shelf and its persistence in the face of climatic variability. PLoS One 13:e0205505. doi: 10.1371/journal.pone.0205505
Beazley, L., Kenchington, E., Murillo, F. J., Brickman, D., Wang, Z., Davies, A. J., et al. (2021). Climate change winner in the deep sea? Predicting the impacts of climate change on the distribution of the glass sponge Vazella pourtalesii. Mar. Ecol. Prog. Ser. 657, 1–23. doi: 10.3354/meps13566
Bell, J. J., Bennett, H. M., Rovellini, A., and Webster, N. S. (2018). Sponges to be winners under near-future climate scenarios. Bioscience 68, 955–968. doi: 10.1093/biosci/biy142
Befort, D. J., O’Reilly, C. H., and Weisheimer, A. (2020). Constraining projections using decadal predictions. Geophys. Research Lett. 47:e2020GL087900.
Bett, B. J., and Rice, A. L. (1992). The influenceof hexactinellid sponge (Pheronema carpenteri) spicules on the patchy distribution of macrobenthos in the porcupine seabight (bathyal ne atlantic). Ophelia 36, 217–226. doi: 10.1080/00785326.1992.10430372
Blacker, R. W. (1957). Benthic animals as indicators of hydrographic conditions and climatic change in Svalbard waters. Fish. Investig. 20, 1–49.
Blacker, R. W. (1965). Recent Changes in the Benthos of the West Spitzbergen Fishing Grounds. Vancouver, BC: International Commission for the Northwest Atlantic Fisheries special Publication.
Bleck, R. (2002). An oceanic general circulation model framed in hybrid isopycnic-Cartesian coordinates. Ocean Model. 4, 55–88.
Blindheim, J., and Rey, F. (2004). Water-mass formation and distribution in the Nordic Seas during the 1990s. ICES J. Mar. Sci. 61, 846–863. doi: 10.1016/j.icesjms.2004.05.003
Boer, G. J. (2004). Long time-scale potential predictability in an ensemble of coupled climate models. Clim. Dyn. 23, 29–44. doi: 10.1007/s00382-004-0419-8
Boer, G. J., Kharin, V. V., and Merryfield, W. J. (2013). Decadal predictability and forecast skill. Clim. Dyn. 41, 1817–1833. doi: 10.1007/s00382-013-1705-0
Borchert, L. F., Düsterhus, A., Brune, S., Müller, W. A., and Baehr, J. (2019). Forecast-oriented assessment of decadal hindcast skill for North Atlantic SST. Geophys. Res. Lett. 46, 11444–11454. doi: 10.1029/2019gl084758
Bower, A. S., Le Cann, B., Rossby, T., Zenk, W., Gould, J., Speer, K., et al. (2002). Directly measured mid-depth circulation in the northeastern North Atlantic Ocean. Nature 419, 603–607. doi: 10.1038/nature01078
Boyer, T. P., Baranova, O.K. Coleman, C., Garcia, H. E., Grodsky, A., Locarnini, R. A., et al. (2018). “World ocean database 2018,” in NOAA Atlas NESDIS 87. and GLODAPv2, ed. A. V. Mishonov (Silver Spring, MD: National Centers for Environmental InformationOcean Climate Laboratory).
Brady, R. X., Lovenduski, N. S., Yeager, S. G., Long, M. C., and Lindsay, K. (2020). Skillful multiyear predictions of ocean acidification in the California current system. Nat. Commun. 11:2166.
Breeze, H., and Fenton, D. G. (2007). Designing management measures to protect cold-water corals off Nova Scotia, Canada. Bull. Mar. Sci. 81, 123–133.
Brenke, N. (2002). “The benthic community of the Great Meteor Bank,” in Proceedings of the ICES Annual Science Conference and ICES Centenary, (Copenhagen).
Brune, S., Düsterhus, A., Pohlmann, H., Müller, W. A., and Baehr, J. (2018). Time dependency of the prediction skill for the North Atlantic subpolar gyre in initialized decadal hindcasts. Clim. Dyn. 51, 1947–1970. doi: 10.1007/s00382-017-3991-4
Buhl-Mortensen, L., Vanreusel, A., Gooday, A. J., Levin, L. A., Priede, I. G., Buhl-Mortensen, P., et al. (2010). Biological structures as a source of habitat heterogeneity and biodiversity on the deep ocean margins. Mar. Ecol. 31, 21–50. doi: 10.1111/j.1439-0485.2010.00359.x
Buhl-Mortensen, L., Olafsdottir, S. H., Buhl-Mortensen, P., Burgos, J. M., and Ragnarsson, S. A. (2015). Distribution of nine cold-water coral species (Scleractinia and Gorgonacea) in the cold temperate North Atlantic: effects of bathymetry and hydrography. Hydrobiologia 759, 39–61. doi: 10.1007/s10750-014-2116-x
Buhl-Mortensen, L., Burgos, J. M., Steingrund, P., Buhl-Mortensen, P., Ólafsdóttir, S. H., and Ragnarsson, S. Á (2019). Vulnerable Marine Ecosystems (VMEs): Coral and Sponge VMEs in Arctic and Sub-Arctic Waters–Distribution and Threats. Copenhagen: Nordic Council of Ministers.
Burgos, J. M., Buhl-Mortensen, L., Buhl-Mortensen, P., Ólafsdóttir, S. H., Steingrund, P., Ragnarsson, S. Á, et al. (2020). Predicting the distribution of indicator taxa of vulnerable marine ecosystems in the Arctic and Sub-arctic Waters of the Nordic Seas. Front. Mar. Sci. 7:131. doi: 10.3389/fmars.2020.00131
Büscher, J. V., Form, A. U., and Riebesell, U. (2017). Interactive effects of ocean acidification and warming on growth, fitness and survival of the cold-water coral Lophelia pertusa under different food availabilities. Front. Mar. Sci. 4:101. doi: 10.3389/fmars.2017.00101
Carnes, M. R. (2009). Description and Evaluation of GDEM-V 3.0. Mississippi, MS: Naval Research Lab Stennis Space Center Ms Oceanography Divdtic.
Cathalot, C., Van Oevelen, D., Cox, T. J., Kutti, T., Lavaleye, M., Duineveld, G., et al. (2015). Cold-water coral reefs and adjacent sponge grounds: hotspots of benthic respiration and organic carbon cycling in the deep sea. Front. Mar. Sci. 2:37. doi: 10.3389/fmars.2015.00037
Cárdenas, P., Rapp, H. T., Klitgaard, A. B., Best, M., Thollesson, M., and Tendal, O. S. (2013). Taxonomy, biogeography and DNA barcodes of Geodia species (Porifera, Demospongiae, Tetractinellida) in the Atlantic boreo-arctic region. Zool. J. Linn. Soc. 169, 251–311. doi: 10.1111/zoj.12056
Cárdenas, P., and Rapp, H. T. (2015). Demosponges from the Northern Mid-Atlantic Ridge shed more light on the diversity and biogeography of North Atlantic deep-sea sponges. J. Mar. Biol. Assoc. U.K. 95, 1475–1516. doi: 10.1017/s0025315415000983
Copley, J. T. P., Tyler, P. A., Sheader, M., Murton, B. J., and German, C. R. (1996). Megafauna from sublittoral to abyssal depths along the Mid-Atlantic Ridge south of Iceland. Oceanol. Acta 19, 549–559.
Culwick, T., Phillips, J., Goodwin, C., Rayfield, E. J., and Hendry, K. R. (2020). Sponge density and distribution constrained by fluid forcing in the deep sea. Front. Mar. Sci. 7:395. doi: 10.3389/fmars.2020.00395
Daewel, U., and Schrum, C. (2013). Simulating long-term dynamics of the coupled North Sea and Baltic Sea ecosystem with ECOSMO II: model description and validation. J. Mar. Syst. 119, 30–49. doi: 10.1016/j.jmarsys.2013.03.008
Dullo, W. C., Flögel, S., and Rüggeberg, A. (2008). Cold-water coral growth in relation to the hydrography of the Celtic and Nordic European continental margin. Mar. Ecol. Prog. Ser. 371, 165–176. doi: 10.3354/meps07623
Erekovsky, A. V. (1995). Materials to the faunistic study of the White and Barents seas sponges. 5. quantitative distribution. Berl. Geowissenschaftliche Abh. 16, 709–714.
FAO (2009). International Guidelines for the Management of Deep-sea Fisheries in the High Seas. Rome: Food and Agriculture Organization.
Fransner, F., Counillon, F., Bethke, I., Tjiputra, J., Samuelsen, A., Nummelin, A., et al. (2020). Ocean biogeochemical predictions—initialization and limits of predictability. Front. Mar. Sci. 7:386. doi: 10.3389/fmars.2020.00386
Frölicher, T. L., Fischer, E. M., and Gruber, N. (2018). Marine heatwaves under global warming. Nature 560, 360–364. doi: 10.1038/s41586-018-0383-9
Frölicher, T. L., Ramseyer, L., Raible, C. C., Rodgers, K. B., and Dunne, J. (2020). Potential predictability of marine ecosystem drivers. Biogeosciences 17, 2061–2083. doi: 10.5194/bg-17-2061-2020
Garcia, H. E., Boyer, T. P., Locarnini, R. A., Antonov, J. I., Mishonov, A. V., Baranova, O. K., et al. (2013a). World Ocean Atlas 2013. Volume 3, Dissolved Oxygen, Apparent Oxygen Utilization, and Oxygen Saturation. Silver Spring, MD: National Oceanographic Data Center.
Garcia, H. E., Locarnini, R. A., Boyer, T. P., Antonov, J. I., Baranova, O. K., Zweng, M. M., et al. (2013b). World Ocean Atlas 2013. Volume 4, Dissolved Inorganic Nutrients (Phosphate, Nitrate, Silicate). Silver Spring, MD: National Oceanographic Data Center.
Goddard, L., Kumar, A., Solomon, A., Smith, D., Boer, G., Gonzalez, P., et al. (2013). A verification framework for interannual-to-decadal predictions experiments. Clim. Dyn. 40, 245–272. doi: 10.1007/s00382-012-1481-2
Guihen, D., White, M., and Lundälv, T. (2012). Temperature shocks and ecological implications at a cold-water coral reef. Mar. Biodivers. Rec. 5:e68.
Guijarro, J., Beazley, L., Lirette, C., Kenchington, E., Wareham, V., Gilkinson, K., et al. (2016). Species Distribution Modelling of Corals and Sponges from Research Vessel Survey Data in the Newfoundland and Labrador Region for use in the Identification of Significant Benthic Areas. Ottawa, OTT: Fisheries and Oceans Canada.
Guisan, A., and Zimmermann, N. E. (2000). Predictive habitat distribution models in ecology. Ecol. Model. 135, 147–186. doi: 10.1016/s0304-3800(00)00354-9
Hansen, C., and Samuelsen, A. (2009). Influence of horizontal model grid resolution on the simulated primary production in an embedded primary production model in the Norwegian Sea. J. Mar. Syst. 75, 236–244. doi: 10.1016/j.jmarsys.2008.10.004
Hanz, U., Beazley, L., Kenchington, E., Duineveld, G., Rapp, H. T., and Mienis, F. (2020). Seasonal variability in near-bed environmental conditions in the Vazella pourtalesii glass sponge grounds of the Scotian Shelf. Front. Mar. Sci. 7:1179. doi: 10.3389/fmars.2021.696777
Hanz, U., Roberts, E. M., Duineveld, G., Davies, A., van Haren, H., Rapp, H. T., et al. (2021). Long-term observations reveal environmental conditions and food supply mechanisms at an Arctic deep-sea sponge ground. J. Geophys. Res. 126, e2020JC016776.
Hawkes, N., Korabik, M., Beazley, L., Rapp, H. T., Xavier, J. R., and Kenchington, E. (2019). Glass sponge grounds on the Scotian Shelf and their associated biodiversity. Mar. Ecol. Prog. Ser. 614, 91–109. doi: 10.3354/meps12903
Heinz, P., Ruepp, D., and Hemleben, C. (2004). Benthic foraminifera assemblages at great meteor seamount. Mar. Biol. 144, 985–998. doi: 10.1007/s00227-003-1257-7
Hestetun, J. T., Rapp, H. T., and Pomponi, S. (2019). Deep-sea carnivorous sponges from the Mariana Islands. Front. Mar. Sci. 6:371. doi: 10.3389/fmars.2019.00371
Hjøllo, S. S., Skogen, M. D., and Svendsen, E. (2009). Exploring currents and heat within the North Sea using a numerical model. J. Mar. Syst. 78, 180–192. doi: 10.1016/j.jmarsys.2009.06.001
Hochachka, P. W., and Somero, G. N. (1968). The adaptation of enzymes to temperature. Comp. Biochem. Phys. 27, 659–668. doi: 10.1016/0010-406x(68)90605-1
Hogg, M. M., Tendal, O. S., Conway, K. W., Pomponi, S. A., Gutt, J., Krautter, M., et al. (2010). Deep-Sea Sponge Grounds: Reservoirs of Biodiversity. Cambridge: World Conservation Monitoring Centre.
Howell, K. L., Piechaud, N., Downie, A. L., and Kenny, A. (2016). The distribution of deep-sea sponge aggregations in the North Atlantic and implications for their effective spatial management. Deep Sea Res. 1 Oceanogr. Res. Pap. 115, 309–320. doi: 10.1016/j.dsr.2016.07.005
Hughes, D. J., and Gage, J. D. (2004). Benthic metazoan biomass, community structure and bioturbation at three contrasting deep-water sites on the northwest European continental margin. Prog. Oceanogr. 63, 29–55. doi: 10.1016/j.pocean.2004.09.002
Iorga, M. C., and Lozier, M. S. (1999a). Signatures of the Mediterranean outflow from a North Atlantic climatology: 1. Salinity and density fields. J. Geophys. Res. Oceans 104, 25985–26009. doi: 10.1029/1999jc900115
Iorga, M. C., and Lozier, M. S. (1999b). Signatures of the Mediterranean outflow from a North Atlantic climatology: 2. Diagnostic velocity fields. J. Geophys. Res. Oceans 104, 26011–26029. doi: 10.1029/1999jc900204
Jacox, M. G., Alexander, M. A., Siedlecki, S., Chen, K., Kwon, Y. O., Brodie, S., et al. (2020). Seasonal-to-interannual prediction of North American coastal marine ecosystems: Forecast methods, mechanisms of predictability, and priority developments. Prog. Oceanogr. 183:102307. doi: 10.1016/j.pocean.2020.102307
Johnson, D., Ferreira, M. A., and Kenchington, E. (2017). Report on Priorities for an Expert Assessment of North Atlantic MPAs, EBSAs, and VMEs in ABNJ. Contribution to the EU H2020 Atlas Project, Project Deliverable 7 2. Victoria, VIC: ATLAS.
Johnson, D., Ferreira, M. A., and Kenchington, E. (2018). Climate change is likely to severely limit the effectiveness of deep-sea ABMTs in the North Atlantic. Mar. Policy 87, 111–122. doi: 10.1016/j.marpol.2017.09.034
Jungclaus, J. H., Fischer, N., Haak, H., Lohmann, K., Marotzke, J., Matei, D., et al. (2013). Characteristics of the ocean simulations in the max planck institute ocean model (MPIOM) the ocean component of the MPI-Earth system model. J. Adv. Model. Earth Syst. 5, 422–446. doi: 10.1002/jame.20023
Kazanidis, G., Vad, J., Henry, L. A., Neat, F., Berx, B., Georgoulas, K., et al. (2019a). Distribution of deep-sea sponge aggregations in an area of multisectoral activities and changing oceanic conditions. Front. Mar. Sci. 6:163. doi: 10.3389/fmars.2019.00163
Kazanidis, G., Vad, J., Henry, L.-A., Neat, F., Berx, B., Georgoulas, K., et al. (2019b). Environmental and biological data from deep-sea sponge aggregations in the Faroe-Shetland Channel Nature Conservation Marine Protected Area. PANGAEA doi: 10.1594/PANGAEA.897592
Kazanidis, G., Vad, J., Henry, L.-A., Neat, F., Berx, B., Georgoulas, K., et al. (2019c). Seabed images and corresponding environmental data from deep-sea sponge aggregations in the Faroe-Shetland Channel Nature Conservation Marine Protected Area. PANGAEA doi: 10.1594/PANGAEA.897604
Keeling, R. F., Körtzinger, A., and Gruber, N. (2010). Ocean deoxygenation in a warming world. Annu. Rev. Mar. Sci. 2, 199–229. doi: 10.1146/annurev.marine.010908.163855
Klitgaard, A. B., and Tendal, O. S. (2004). Distribution and species composition of mass occurrences of large-sized sponges in the northeast Atlantic. Prog. Oceanogr. 61, 57–98. doi: 10.1016/j.pocean.2004.06.002
Knudby, A., Kenchington, E., and Murillo, F. J. (2013). Modeling the distribution of Geodia sponges and sponge grounds in the Northwest Atlantic. PLoS One 8:e82306. doi: 10.1371/journal.pone.0082306
Kutti, T., Bannister, R. J., and Fosså, J. H. (2013). Community structure and ecological function of deep-water sponge grounds in the traenadypet MPA—Northern Norwegian continental shelf. Cont. Shelf Res. 69, 21–30.
Lapointe, A., Watling, L., and Gontz, A. M. (2020). “Deep-sea benthic megafaunal communities on the New England and corner rise seamounts, Northwest Atlantic Ocean,” in Seafloor Geomorphology as Benthic Habitat, eds P. Harris and E. Baker (Amsterdam: Elsevier), 917–932.
Lee, J. Y., Marotzke, J., Bala, G., Cao, L., Corti, S., Dunne, J. P., et al. (2021). “Future global climate: scenario-based projections and near-term information,” in Climate Change 2021: The Physical Science Basis. Contribution of Working Group I to the Sixth Assessment Report of the Intergovernmental Panel on Climate Change, eds V. Masson-Delmotte, P. Zhai, A. Pirani, S. L. Connors, C. Péan, S. Berger, et al. (Cambridge: Cambridge University Press).
Levin, L. A. (2003). Oxygen minimum zone benthos: adaptation and community response to hypoxia. Oceanogr. Mar. Biol. Annu. Rev. 41, 1–45.
Levin, L. A., and Le Bris, N. (2015). The deep ocean under climate change. Science 350, 766–768. doi: 10.1126/science.aad0126
Levin, L. A., Bett, B. J., Gates, A. R., Heimbach, P., Howe, B. M., Janssen, F., et al. (2019). Global observing needs in the deep ocean. Front. Mar. Sci. 6:241. doi: 10.3389/fmars.2019.00241
Li, H., Ilyina, T., Müller, W. A., and Sienz, F. (2016). Decadal predictions of the North Atlantic CO 2 uptake. Nat. Commun. 7:11076.
Li, L. Z. (2006). Atmospheric GCM response to an idealized anomaly of the Mediterranean sea surface temperature. Clim. Dyn. 27, 543–552. doi: 10.1007/s00382-006-0152-6
Malanotte-Rizzoli, P., Artale, V., Borzelli-Eusebi, G. L., Brenner, S., Crise, A., Gacic, M., et al. (2014). Physical forcing and physical/biochemical variability of the Mediterranean Sea: a review of unresolved issues and directions for future research. Ocean Sci. 10, 281–322. doi: 10.5194/os-10-281-2014
Maldonado, M., Aguilar, R., Bannister, R. J., Bell, J. J., Conway, K. W., Dayton, P. K., et al. (2017). Sponge Grounds as Key Marine Habitats: a Synthetic Review of Types, Structure, Functional Roles, and Conservation Concerns. Cham: Springer International Publishing.
Maldonado, M., López-Acosta, M., Sitjà, C., García-Puig, M., Galobart, C., Ercilla, G., et al. (2019). Sponge skeletons as an important sink of silicon in the global oceans. Nat. Geosci. 12, 815–822. doi: 10.1038/s41561-019-0430-7
Maldonado, M., López-Acosta, M., Beazley, L., Kenchington, E., Koutsouveli, V., and Riesgo, A. (2020). Cooperation between passive and active silicon transporters clarifies the ecophysiology and evolution of biosilicification in sponges. Sci. Adv. 6:eaba9322. doi: 10.1126/sciadv.aba9322
Maldonado, M., Beazley, L., López-Acosta, M., Kenchington, E., Casault, B., Hanz, U., et al. (2021). Massive silicon utilization facilitated by a benthic-pelagic coupled feedback sustains deep-sea sponge aggregations. Limnol. Oceanogr. 66, 366–391. doi: 10.1002/lno.11610
Marsland, S. J., Haak, H., Jungclaus, J. H., Latif, M., and Röske, F. (2003). The max-planck-institute global ocean/sea ice model with orthogonal curvilinear coordinates. Ocean Model. 5, 91–127. doi: 10.1016/S1463-5003(02)00015-X
Mathis, M., Elizalde, A., Mikolajewicz, U., and Pohlmann, T. (2015). Variability patterns of the general circulation and sea water temperature in the North Sea. Prog. Oceanogr. 135, 91–112. doi: 10.1016/j.pocean.2015.04.009
Meyer, H. K., Roberts, E. M., Mienis, F., and Rapp, H. T. (2020a). Megafauna Abundances Observed During a Sponges Cruise With RV G.O. Sars and ROV Ægir 6000 in Sognefjord, Norway. Brussels: Data Publisher for Earth & Environmental Science.
Meyer, H. K., Roberts, E. M., Mienis, F., and Rapp, H. T. (2020b). Megafauna AbundancesWith Corresponding Environmental and Nutrient Variables From a Sponges Cruise With RV G.O. Sars and ROV Ægir 6000 From Two ROV Dives in Sognefjord, Norway. Brussels: Data Publisher for Earth & Environmental Science.
Meyer, H. K., Roberts, E. M., Rapp, H. T., and Davies, A. J. (2019). Spatial patterns of arctic sponge ground fauna and demersal fish are detectable in autonomous underwater vehicle (AUV) imagery. Deep Sea Res. 1 Oceanogr. Res. Pap. 153:103137.
Meyer, K. S., Sweetman, A. K., Young, C. M., and Renaud, P. E. (2015). Environmental factors structuring Arctic megabenthos—a case study from a shelf and two fjords. Front. Mar. Sci. 2:22. doi: 10.3389/fmars.2015.00022
Morato, T., González-Irusta, J. M., Dominguez-Carrió, C., Wei, C. L., Davies, A., Sweetman, A. K., et al. (2020). Climate-induced changes in the suitable habitat of cold-water corals and commercially important deep-sea fishes in the North Atlantic. Glob. change Biol. 26, 2181–2202.
Morrison, K. M., Meyer, H. K., Roberts, E. M., Rapp, H. T., Colaço, A., and Pham, C. K. (2020). The first cut is the deepest: trawl effects on a deep-sea sponge ground are pronounced four years on. Front. Mar. Sci. 7:1059. doi: 10.3389/fmars.2020.605281
Müller, W. A., Pohlmann, H., Sienz, F., and Smith, D. (2014). Decadal climate predictions for the period 1901–2010 with a coupled climate model. Geophys. Res. Lett. 41, 2100–2107. doi: 10.1002/2014GL059259
Murillo, F. J., Muñoz, P. D., Cristobo, J., Ríos, P., González, C., Kenchington, E., et al. (2012). Deep-sea sponge grounds of the flemish cap, flemish pass and the grand banks of newfoundland (Northwest Atlantic Ocean): distribution and species composition. Mar. Biol. Res. 8, 842–854. doi: 10.1080/17451000.2012.682583
Naumann, M. S., Orejas, C., and Ferrier-Pagès, C. (2014). Species-specific physiological response by the cold-water corals Lophelia pertusa and Madrepora oculata to variations within their natural temperature range. Deep Sea Res. 2 Top. Stud. Oceanogr. 99, 36–41. doi: 10.1016/j.dsr2.2013.05.025
Oevelen, D. V., Duineveld, G., Lavaleye, M., Mienis, F., Soetaert, K., and Heip, C. H. (2009). The cold-water coral community as hotspot of carbon cycling on continental margins: a food-web analysis from Rockall Bank (northeast Atlantic). Limnol. Oceanogr. 54, 1829–1844. doi: 10.4319/lo.2009.54.6.1829
Olsen, A., Key, R. M., van Heuven, S., Lauvset, S. K., Velo, A., Lin, X., et al. (2016). The global ocean data analysis project version 2 (GLODAPv2) – an internally consistent data product for the world ocean. Earth Syst. Sci. Data 8, 297–323. doi: 10.5194/essd-8-297-2016
Pastor, M. V., Peña-Izquierdo, J., Pelegrí, J. L., and Marrero-Díaz, Á (2012). Meridional changes in water mass distributions off NW Africa during November 2007/2008. Cienc. Mar. 38, 223–244. doi: 10.7773/cm.v38i1b.1831
Payne, M. R., Barange, M., Cheung, W. W., MacKenzie, B. R., Batchelder, H. P., Cormon, X., et al. (2016). Uncertainties in projecting climate-change impacts in marine ecosystems. ICES J. Mar. Sci. 73, 1272– 1282.
Pearman, T. R. R., Robert, K., Callaway, A., Hall, R., Iacono, C. L., and Huvenne, V. A. (2020). Improving the predictive capability of benthic species distribution models by incorporating oceanographic data–Towards holistic ecological modelling of a submarine canyon. Prog. Oceanogr. 184:102338.
Pecl, G. T., Araújo, M. B., Bell, J. D., Blanchard, J., Bonebrake, T. C., Chen, I. C., et al. (2017). Biodiversity redistribution under climate change: impacts on ecosystems and human well-being. Science 355:eaai9214.
Penny, S. G., Akella, S., Balmaseda, M. A., Browne, P., Carton, J. A., Chevallier, M., et al. (2019). Observational needs for improving ocean and coupled reanalysis, S2S prediction, and decadal prediction. Front. Mar. Sci. 6:391. doi: 10.3389/fmars.2019.00391
Perez, F. F., Fontela, M., García-Ibáñez, M. I., Mercier, H., Velo, A., Lherminier, P., et al. (2018). Meridional overturning circulation conveys fast acidification to the deep Atlantic Ocean. Nature 554, 515–518. doi: 10.1038/nature25493
Petrie, B., and Drinkwater, K. (1993). Temperature and salinity variability on the Scotian Shelf and in the Gulf of Maine 1945–1990. J. Geophys. Res. Oceans 98, 20079–20089. doi: 10.1029/93jc02191
Pham, C. K., Murillo, F. J., Lirette, C., Maldonado, M., Colaço, A., Ottaviani, D., et al. (2019). Removal of deep-sea sponges by bottom trawling in the Flemish Cap area: conservation, ecology and economic assessment. Sci. Rep. 9:15843.
Pickart, R. S. (1992). Water mass components of the North Atlantic deep western boundary current. Deep Sea Res. A. Oceanogr. Res. Pap. 39, 1553–1572. doi: 10.1016/0198-0149(92)90047-w
Pile, A. J., and Young, C. M. (2006). The natural diet of a hexactinellid sponge: benthic–pelagic coupling in a deep-sea microbial food web. Deep Sea Res. 1 Oceanogr. Res. Pap. 53, 1148–1156. doi: 10.1016/j.dsr.2006.03.008
Pohlmann, H., Mueller, W. A., Kulkarni, K., Kameswarrao, M., Matei, D., Vamborg, F. S. E., et al. (2013). Improved forecast skill in the tropics in the new MiKlip decadal climate predictions. Geophys. Res. Lett. 40, 5798–5802.
Polkova, I., Brune, S., Kadow, C., Romanova, V., Gollan, G., Baehr, J., et al. (2019). Initialization and ensemble generation for decadal climate predictions: a comparison of different methods. J. Adv. Model. Earth Sys. 11, 149–172. doi: 10.1029/2018MS001439
Pozo Buil, M., Jacox, M. G., Fiechter, J., Alexander, M. A., Bograd, S. J., Curchitser, E. N., et al. (2021). A dynamically downscaled ensemble of future projections for the California current system. Front. Mar. Sci. 8:324. doi: 10.3389/fmars.2021.612874
Puerta, P., Johnson, C., Carreiro-Silva, M., Henry, L. A., Kenchington, E., Morato, T., et al. (2020). Influence of water masses on the biodiversity and biogeography of deep-sea benthic ecosystems in the North Atlantic. Front. Mar. Sci. 7:239. doi: 10.3389/fmars.2020.00239
Purkey, S. G., and Johnson, G. C. (2010). Warming of global abyssal and deep Southern Ocean waters between the 1990s and 2000s: Contributions to global heat and sea level rise budgets. Journal of Climate 23, 6336–6351. doi: 10.1175/2010jcli3682.1
Rhein, M., Kieke, D., Hüttl-Kabus, S., Roessler, A., Mertens, C., Meissner, R., et al. (2011). Deep water formation, the subpolar gyre, and the meridional overturning circulation in the subpolar North Atlantic. Deep Sea Res. 2 Top. Stud. Oceanogr. 58, 1819–1832. doi: 10.1016/j.dsr2.2010.10.061
Ramiro-Sánchez, B., González-Irusta, J. M., Henry, L. A., Cleland, J., Yeo, I., Xavier, J. R., et al. (2019). Characterization and mapping of a deep-sea sponge ground on the tropic seamount (Northeast Tropical Atlantic): implications for spatial management in the high seas. Front. Mar. Sci. 6:278. doi: 10.3389/fmars.2019.00278
Riahi, K., Rao, S., Krey, V., Cho, C., Chirkov, V., Fischer, G., et al. (2011). RCP 8.5—A scenario of comparatively high greenhouse gas emissions. Clim. Change 109, 33–57. doi: 10.1007/s10584-011-0149-y
Rice, A. L., Thurston, M. H., and New, A. L. (1990). Dense aggregations of a hexactinellid sponge, Pheronema carpenteri, in the porcupine seabight (northeast Atlantic Ocean), and possible causes. Prog. Oceanogr. 24, 179–196. doi: 10.1016/0079-6611(90)90029-2
Ríos, P., Prado, E., Carvalho, F. C., Sánchez, F., Rodríguez-Basalo, A., Xavier, J. R., et al. (2020). Community composition and habitat characterization of a rock sponge aggregation (Porifera, Corallistidae) in the Cantabrian Sea. Front. Mar. Sci. 7:578. doi: 10.3389/fmars.2020.00578
Roark, E. B., Guilderson, T. P., Dunbar, R. B., Fallon, S. J., and Mucciarone, D. A. (2009). Extreme longevity in proteinaceous deep-sea corals. Proc. Natl. Acad. Sci. U.S.A. 106, 5204–5208. doi: 10.1073/pnas.0810875106
Roberts, E. M., Mienis, F., Rapp, H. T., Hanz, U., Meyer, H. K., and Davies, A. J. (2018). Oceanographic setting and short-timescale environmental variability at an Arctic seamount sponge ground. Deep Sea Res. 1 Oceanogr. Res. Pap. 138, 98–113. doi: 10.1016/j.dsr.2018.06.007
Roberts, E. M., Bowers, D. G., Meyer, H. K., Samuelsen, A., Rapp, H. T., and Cárdenas, P. (2021). Water masses constrain the distribution of deep-sea sponges in the North Atlantic Ocean and Nordic Seas. Mar. Ecol. Prog. Ser. 659, 75–96. doi: 10.3354/meps13570
Roberts, J. M., Wheeler, A. J., and Freiwald, A. (2006). Reefs of the deep: the biology and geology of cold-water coral ecosystems. Science 312, 543–547. doi: 10.1126/science.1119861
Rodríguez-Basalo, A., Prado, E., Sánchez, F., Ríos, P., Gómez-Ballesteros, M., and Cristobo, J. (2021). High resolution spatial distribution for the hexactinellid sponges Asconema setubalense and Pheronema carpenteri in the Central Cantabrian Sea. Front. Mar. Sci. 8:129. doi: 10.3389/fmars.2021.612761
Rudels, B. (1995). The thermohaline circulation of the Arctic Ocean and the Greenland Sea. Philos. Trans. R. Soc. Lond. A 352, 287–299. doi: 10.1098/rsta.1995.0071
Sakov, P., Counillon, F., Bertino, L., Lisæter, K. A., Oke, P. R., and Korablev, A. (2012). TOPAZ4: an ocean-sea ice data assimilation system for the North Atlantic and Arctic. Ocean Sci. 8, 633–656. doi: 10.5194/os-8-633-2012
Samuelsen, A., Hansen, C., and Wehde, H. (2015). Tuning and assessment of the HYCOM-NORWECOM V2. 1 biogeochemical modeling system for the North Atlantic and Arctic oceans. Geosci. Model Dev. 8, 2187–2202.
Samuelsen, A., Schrum, C., Daewel, U., Castano-Primo, R., and Yumruktepe, V. Ç (2019). SponGES Deliverable 7.6 - Report on Historic Climate and Ecosystem Data. Berkeley, CA: National Energy Research Scientific Computing Center.
Sarafanov, A. (2009). On the effect of the North Atlantic Oscillation on temperature and salinity of the subpolar North Atlantic intermediate and deep waters. ICES J. Mar. Sci. 66, 1448–1454. doi: 10.1093/icesjms/fsp094
Sasaki, H., Kida, S., Furue, R., Aiki, H., Komori, N., Masumoto, Y., et al. (2020). A global eddying hindcast ocean simulation with OFES2. Geosci. Model Dev. 13, 3319–3336. doi: 10.5194/gmd-13-3319-2020
Schubert-Frisius, M., and Feser, F. (2015). Global High Resolution Climate Reconstruction with ECHAM6 using the spectral nudging technique, run by Helmholtz-Zentrum Geesthacht. Hamburg: World Data Center for Climate.
Schulz, K., Soetaert, K., Mohn, C., Korte, L., Mienis, F., Duineveld, G., et al. (2020). Linking large-scale circulation patterns to the distribution of cold water corals along the eastern Rockall Bank (northeast Atlantic). J. Mar. Syst. 212:103456.
Schrum, C., Alekseeva, I., and John, M. S. (2006). Development of a coupled physical–biological ecosystem model ECOSMO: part I: model description and validation for the North Sea. J. Mar. Syst. 61, 79–99.
Séférian, R., Bopp, L., Gehlen, M., Swingedouw, D., Mignot, J., Guilyardi, E., et al. (2014). Multiyear predictability of tropical marine productivity. Proc. Natl. Acad. Sci. U.S.A. 111, 11646–11651. doi: 10.1073/pnas.1315855111
Smith, D. M., Eade, R., Scaife, A. A., Caron, L. P., Danabasoglu, G., DelSole, T. M., et al. (2019). Robust skill of decadal climate predictions. NPJ Clim. Atmos. Sci. 2:13.
Smith, K. L., Ruhl, H. A., Bett, B. J., Billett, D. S. M., Lampitt, R. S., and Kaufmann, R. S. (2009). Climate, carbon cycling, and deep-ocean ecosystems. Proc. Natl. Acad. Sci. U.S.A. 106, 19211–19218. doi: 10.1073/pnas.0908322106
Somot, S., Sevault, F., Déqué, M., and Crépon, M. (2008). 21st century climate change scenario for the Mediterranean using a coupled atmosphere–ocean regional climate model. Glob. Planet. Change 63, 112–126. doi: 10.1016/j.gloplacha.2007.10.003
Stramma, L., Schmidtko, S., Levin, L. A., and Johnson, G. C. (2010). Ocean oxygen minima expansions and their biological impacts. Deep Sea Res. 1 Oceanogr. Res. Pap. 57, 587–595. doi: 10.1016/j.dsr.2010.01.005
Strand, R., Whalan, S., Webster, N. S., Kutti, T., Fang, J. K. H., Luter, H. M., et al. (2017). The response of a boreal deep-sea sponge holobiont to acute thermal stress. Sci. Rep. 7:1660.
Sweetman, A. K., Thurber, A. R., Smith, C. R., Levin, L. A., Mora, C., Wei, C. L., et al. (2017). Major impacts of climate change on deep-sea benthic ecosystems. Elementa 4:5.
Swift, J. H., and Koltermann, K. P. (1988). The origin of Norwegian Sea deep water. J. Geophys. Res. Oceans 93, 3563–3569. doi: 10.1029/jc093ic04p03563
Tabachnick, K. R., and Menshenina, L. L. (2007). Revision of the genus Asconema (Porifera: Hexactinellida: Rossellidae). J. Mar. Biol. Assoc. U.K. 87, 1403–1429. doi: 10.1017/s0025315407058158
Tempera, F., Giacomello, E., Mitchell, N. C., Campos, A. S., Henriques, A. B., Bashmachnikov, I., et al. (2012). “Mapping condor seamount seafloor environment and associated biological assemblages (Azores, Ne Atlantic),” in Proceedings of the Seafloor Geomorphology as Benthic Habitat, (Amsterdam: Elsevier), 807–818. doi: 10.1016/b978-0-12-385140-6.00059-1
Tett, P., Droop, M. R., and Heaney, S. I. (1985). The Redfield ratio and phytoplankton growth rate. J. Mar. Biol. Assoc. U.K. 65, 487–504. doi: 10.1017/s0025315400050566
Tommasi, D., Stock, C. A., Hobday, A. J., Methot, R., Kaplan, I. C., Eveson, J. P., et al. (2017). Managing living marine resources in a dynamic environment: the role of seasonal to decadal climate forecasts. Prog. Oceanogr. 152, 15–49.
Turrell, W. R. (1992). New hypotheses concerning the circulation of the northern North Sea and its relation to North Sea fish stock recruitment. ICES J. Mar. Sci. 49, 107–123. doi: 10.1093/icesjms/49.1.107
Vacelet, J., and Custódio, M. R. (2007). Diversity and evolution of deep-sea carnivorous sponges. Porifera research: biodiversity, innovation and sustainability. Série Livros 28, 107–115.
van Soest, R. W., Cleary, D. F., de Kluijver, M. J., Lavaleye, M. S., Maier, C., and van Duyl, F. C. (2007). Sponge diversity and community composition in Irish bathyal coral reefs. Contrib. Zool. 76, 121–142. doi: 10.1163/18759866-07602005
Van Vuuren, D. P., Edmonds, J., Kainuma, M., Riahi, K., Thomson, A., Hibbard, K., et al. (2011). The representative concentration pathways: an overview. Clim. Change 109, 5–31. doi: 10.1007/s10584-011-0148-z
Vaquer-Sunyer, R., and Duarte, C. M. (2008). Thresholds of hypoxia for marine biodiversity. Proc. Natl. Acad. Sci. U.S.A. 105, 15452–15457. doi: 10.1073/pnas.0803833105
Willmott, C. J., and Matsuura, K. (2005). Advantages of the mean absolute error (MAE) over the root mean square error (RMSE) in assessing average model performance. Clim. Res. 30, 79–82. doi: 10.3354/cr030079
Winther, N. G., and Evensen, G. (2006). A hybrid coordinate ocean model for shelf sea simulation. Ocean Model. 13, 221–237. doi: 10.1016/j.ocemod.2006.01.004
Witte, U., and Graf, G. (1996). Metabolism of deep-sea sponges in the Greenland-Norwegian Sea. J. Exp. Mar. Biol. Ecol. 198, 223–235. doi: 10.1016/0022-0981(96)00006-8
Woulds, C., Cowie, G. L., Levin, L. A., Andersson, J. H., Middelburg, J. J., Vandewiele, S., et al. (2007). Oxygen as a control on sea floor biological communities and their roles in sedimentary carbon cycling. Limnol. Oceanogr. 52, 1698–1709.
Xavier, J., and van Soest, R. (2007). Demosponge fauna of Ormonde and Gettysburg Seamounts (Gorringe Bank, north-east Atlantic): diversity and zoogeographical affinities. J. Mar. Biol. Assoc. U.K. 87, 1643–1653. doi: 10.1017/s0025315407058584
Xavier, J. R., Tojeira, I., and Van Soest, R. W. (2015). On a hexactinellid sponge aggregation at the Great Meteor seamount (North-east Atlantic). J. Mar. Biol. Assoc. U.K. 95, 1389–1394.
Yasuhara, M., Cronin, T. M., Demenocal, P. B., Okahashi, H., and Linsley, B. K. (2008). Abrupt climate change and collapse of deep-sea ecosystems. Proc. Natl. Acad. Sci. U.S.A. 105, 1556–1560. doi: 10.1073/pnas.0705486105
Yasuhara, M., Okahashi, H., Cronin, T. M., Rasmussen, T. L., and Hunt, G. (2014). Response of deep-sea biodiversity to abrupt deglacial and H olocene climate changes in the N orth A tlantic O cean. Glob. Ecol. Biogeogr. 23, 957–967. doi: 10.1111/geb.12178
Yasuhara, M., and Danovaro, R. (2016). Temperature impacts on deep-sea biodiversity. Biol. Rev. 91, 275–287.
Keywords: sponge habitat, deep-sea, predictability, regional downscaling prediction, interannual to decadal variability, environmental conditions
Citation: Liu F, Daewel U, Samuelsen A, Brune S, Hanz U, Pohlmann H, Baehr J and Schrum C (2021) Can Environmental Conditions at North Atlantic Deep-Sea Habitats Be Predicted Several Years Ahead? ——Taking Sponge Habitats as an Example. Front. Mar. Sci. 8:703297. doi: 10.3389/fmars.2021.703297
Received: 30 April 2021; Accepted: 29 September 2021;
Published: 28 October 2021.
Edited by:
Ellen Kenchington, Bedford Institute of Oceanography (BIO), CanadaReviewed by:
Patricia Puerta, Oceanographic Center of the Balearic Islands, Spanish Institute of Oceanography (IEO), SpainFuru Mienis, Royal Netherlands Institute for Sea Research (NIOZ), Netherlands
Copyright © 2021 Liu, Daewel, Samuelsen, Brune, Hanz, Pohlmann, Baehr and Schrum. This is an open-access article distributed under the terms of the Creative Commons Attribution License (CC BY). The use, distribution or reproduction in other forums is permitted, provided the original author(s) and the copyright owner(s) are credited and that the original publication in this journal is cited, in accordance with accepted academic practice. No use, distribution or reproduction is permitted which does not comply with these terms.
*Correspondence: Feifei Liu, ZmVpZmVpLmxpdUBoZXJlb24uZGU=