- 1Marine and Fluvial Systems, Faculty of Engineering Technology, University of Twente, Enschede, Netherlands
- 2Faculty of Geo-Information Science and Earth Observation, University of Twente, Enschede, Netherlands
- 3Department of Estuarine and Delta Systems, NIOZ Royal Netherlands Institute for Sea Research, Yerseke, Netherlands
- 4Department of Geography, National University of Singapore, Singapore, Singapore
- 5Centre for Nature-Based Climate Solutions, National University of Singapore, Singapore, Singapore
- 6National Institute of Water and Atmospheric Research, Hamilton, New Zealand
Integration of mangroves in projects to reduce coastal flood risk is increasingly being recognised as a sustainable and cost-effective alternative. In addition to the construction of conventional hard flood protection infrastructure, mangroves not only contribute to attenuating flood events (functionality), they also recover in, and adapt to, a changing climate (persistence). The implementation of mangroves in flood risk reduction, however, remains complex. This is because the innate functionality and persistence of mangroves depend on a range of environmental conditions. Importantly, mangroves may collapse when environmental impacts or climatic changes exceed key system thresholds, bringing uncertainty into a situation where failure could endanger lives and livelihoods. The uncertainties in mangrove functionality and persistence can be dealt with by (1) improving insights in how ecological and physical processes affect mangrove functionality and persistence across scales, (2) advancing tools to accurately assess and predict mangrove functionality and persistence, and (3) adopting an adaptive management approach combined with appropriate engineering interventions to enhance mangrove functionality and persistence. Here, we review existing evidence, monitoring techniques and modelling approaches from the viewpoint of mangrove functionality and persistence. Inspired by existing guidelines for Nature-based Solutions (NbS) to reduce flood risk, we provide an operationalization for this new approach. In addition, we identify where further research efforts are required for the practical application of mangroves in coastal flood risk management. Key aspects in the variability and uncertainty of the functionality and persistence of mangroves are their failure and recovery mechanisms, which are greatly site- and storm-specific. We propose five characteristic damage regimes that result in increasing reductions of mangrove functionality as well as post-storm recovery periods. Further research on the quantification of these regimes and their thresholds is required for the successful integration of mangroves in coastal flood risk management. Ultimately, the key challenge is the development of adaptive management strategies to optimise long-term mangrove functionality and persistence, or their resilience. Such adaptive strategies should be informed by continued mangrove functionality and persistence assessments, based on continued monitoring and modelling of key mangrove thresholds, and supported through well-established guidelines.
1. Introduction
Hundreds of millions of people are exposed to the risk of coastal flooding due to the compounding effects of tides, storms, tropical cyclones and tsunamis (Kulp and Strauss, 2019). This exposure is expected to increase with sea level rise (SLR) and the increasing frequency and intensity of tropical cyclones (Woodruff et al., 2013; Kirezci et al., 2020; Taherkhani et al., 2020). Rapid coastal population growth and urbanization is also increasing the vulnerability to, and consequences of, flood events, especially in the tropics (Neumann et al., 2015). Meanwhile, coastal wetland ecosystems that can reduce flood risk (Arkema et al., 2013; Van Coppenolle and Temmerman, 2020), are in rapid decline due to their exploitation and conversion for other land-use purposes. To reduce the flood risk exposure in low-elevation coastal zones, particularly in developing economies, it is critical to develop and implement sustainable and cost-effective solutions that reduce coastal flood risk.
Mangrove forests can contribute to the reduction of coastal flood risk in low-elevation coastal zones (Hochard et al., 2019; Menéndez et al., 2020). Due to their presence, extent and characteristic above-ground aerial root systems, they can help reduce surge levels and attenuate wind waves. Moreover, mangroves can provide a variety of co-benefits to coastal communities and beyond, that are embedded in the United Nations Sustainable Development Goals (SDGs). Mangroves contribute to food provision (SDG-2) by providing an important habitat for fish and shellfish (Carrasquilla-Henao and Juanes, 2017); to economic development (SDG-8) by supporting ecotourism (Spalding and Parrett, 2019); and to climate action (SDG-13) by effectively sequestrating carbon (Ezcurra et al., 2016). As such, mangroves act as a broad Nature-based Solution (NbS) to both climate change adaptation and mitigation. Their application to reduce coastal flood risk is also increasingly supported worldwide through numerous initiatives that seek to incorporate coastal ecosystem protection and restoration into coastal engineering and management.
There is increasing interest in the application of mangroves to contribute to flood risk reduction along with a range of engineering structures, in hybrid-engineering solutions (Sutton-Grier et al., 2015). To capitalize on this interest and successfully and effectively integrate mangroves in coastal flood risk management, we need to understand mangrove dynamics and thresholds, and the optimal conditions for mangroves to reduce flood risk. In particular, design and implementation guidelines will help understand how mangroves can be incorporated into broader flood risk reduction solutions. Guidelines translate scientific knowledge of mangrove dynamics into a language that stakeholders and users, such as engineers and decision makers, can work with, while providing insights in future research required to better incorporate mangroves in flood risk projects.
Management (i.e., decision making) and engineering of projects to reduce coastal flood risk are typically executed in well-defined sequential processes. Firstly, the management process assesses the flood risk exposure of a coastal community and determines the necessity of flood protection infrastructure to prevent or mitigate flood events. Secondly, the engineering process concerns the design, construction and maintenance of this infrastructure. The infrastructure is initially designed to have functionality for flood risk reduction, that is of a sufficient capacity to avoid and/or mitigate the effects of flood events. The infrastructure must also have sufficient persistence, that is the capacity to maintain its functionality for a certain period of time. After implementation, regular monitoring is required to assess the infrastructure functionality over time. The monitoring requirement recognizes the large variability in geomorphological and socio-economic developments along shorelines. Furthermore, predictive models are typically used to explore effects of anticipated extreme conditions or environmental change on the functionality and persistence of the infrastructure. In case the infrastructure functionality cannot be guaranteed for the design life, then (additional) engineering interventions may need to be considered.
Mangroves have a functional capacity to reduce coastal flood risk, but also have innate persistent capacities that traditional hard-engineering infrastructure is lacking (Gedan et al., 2011; Cheong et al., 2013; Temmerman et al., 2013; Bouma et al., 2014). The functionality of mangroves is provided by the structure of their above-ground biomass and aerial roots that dampens hydrodynamic flows and dissipates hydrodynamic energy of waves (Marois and Mitsch, 2015). Mangroves also have innate persistence due to their adaptive ecosystem engineering capacity to adjust to changing environmental conditions (Jones et al., 1994, 1997). Their natural regeneration and sediment trapping capacity enables mangroves to (1) stabilise shorelines (Krauss et al., 2014; Woodroffe et al., 2016), (2) recover from impacts of extreme events (Krauss and Osland, 2020), and (3) adapt to longer-term changes in environmental conditions (Schuerch et al., 2018; Saintilan et al., 2020). Hence, mangroves have the potential to offer a shoreline-stabilising, impact-resilient and climate-adaptive strategy to reduce coastal flood risk. In doing so, mangroves can provide additional values for society while implementation and maintenance costs could be substantially lower compared to hard-engineering infrastructure (Borsje et al., 2011; Narayan et al., 2016).
Coastal flood risk management requires reliable systems providing a predictable functionality with a well-defined persistence. However, the functionality of mangroves is highly non-linear in relation to forest width (Koch et al., 2009; Montgomery et al., 2019). Also, their persistence is limited by critical thresholds: mangroves may collapse when either impacts of extreme events (Sippo et al., 2018; Krauss and Osland, 2020), or changes in environmental conditions exceed their natural tolerance (Friess et al., 2012). Such non-linearities and thresholds to mangrove functionality and persistence complicate efforts to incorporate mangroves into coastal flood protection projects. This way, mangroves bring uncertainty into a situation where failure could endanger lives and livelihoods.
There is a fundamental difference in the variability and uncertainty of functionality and persistence between hard-engineering infrastructure and mangroves (Figure 1). This difference necessitates an adaptive management approach. Successful design and implementation of mangroves to reduce coastal flood risk requires (1) a mechanistic understanding of mangrove functionality and persistence, (2) accurate assessments of mangrove functionality and persistence, and (3) identification of design alternatives to enhance mangrove functionality or persistence. For conventional hard-engineering structures, mechanistic understanding, tools and interventions have been addressed and distilled in design guidelines that have been widely applied to manage coastal flood risk (USACE, 2001). By contrast, practical engineering and design guidelines for flood risk reduction using mangroves, or other NbS, based on a mechanistic understanding of these systems, have not yet been developed. This review aims at providing a comprehensive overview of the context and processes that determine mangrove functionality and persistence, which will allow for the definition, quantification and monitoring of locally suitable critical success factors for the implementation of mangroves to reduce coastal flood risk.
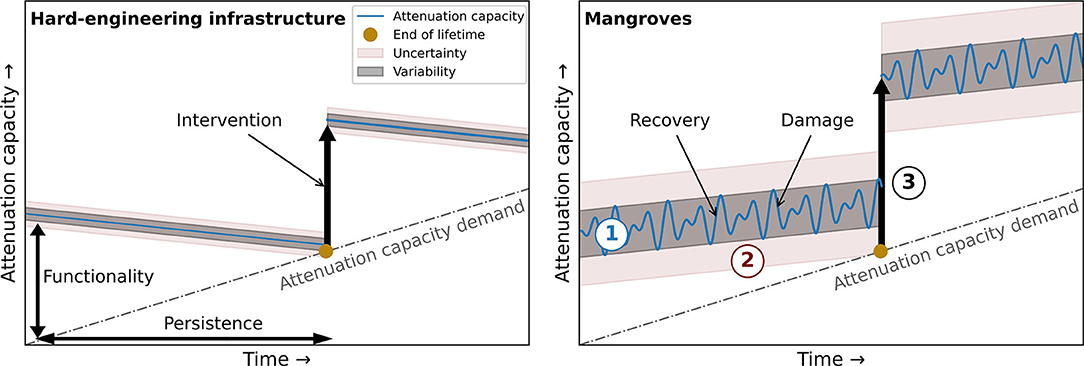
Figure 1. Variability and uncertainty in reducing flood risk with (A) hard-engineering flood defences and (B) mangroves. The utilization of mangroves requires (1) insights in the variability of mangrove functionality and persistence, (2) tools to estimate and predict mangrove functionality and persistence and (3) design alternatives that increase mangrove functionality and/or persistence. The attenuation capacity demanded of flood protection infrastructure is expected to increase due to the globally increasing flood risk as a result of sea-level rise and increasing human activity in the coastal zone. While mangroves have capacities to adapt to sea-level rise (i.e., the attenuation capacity increases gradually), hard-engineering infrastructure cannot (i.e., the attenuation capacity gradually decreases). The figure scales depend on the local physical and social circumstances in specific flood risk projects.
The review discusses the functionality and persistence of mangroves from the perspective of coastal flood risk reduction. Firstly, we discuss field evidence for, and the mechanistics of, mangrove functionality and persistence. Secondly, we present field monitoring and remote sensing techniques to monitor mangrove functionality and persistence. Thirdly, we discuss modelling tools that can aid in assessing and predicting the functionality and persistence of mangroves. Fourthly, we provide an overview of available guidelines for engineering and management with mangroves and provide an operationalization of these guidelines following the functionality and persistence approach and the evidence and tools discussed before. Before the conclusion, we discuss remaining challenges that require further study to be able to proceed to practical engineering and management strategies to reduce coastal flood risk with mangroves.
2. Field and Remote Sensing Evidence for Mangrove Functionality and Persistence
Biogeomorphic dynamics in mangroves are characterised by continuous interactions between hydrodynamic, morphological and ecological processes (Figure 2). The present state of a mangrove forest determines its capacity to attenuate hydrodynamic energy (i.e., wave and water level reduction) and resulting functionality. Mangrove forest persistence depends on how its present state changes over time, due to morphological changes (i.e., surface elevation changes relative to sea level) or changes in forest composition and extent (i.e., forest structure changes) resulting from ecological and/or physical processes (Figure 3).
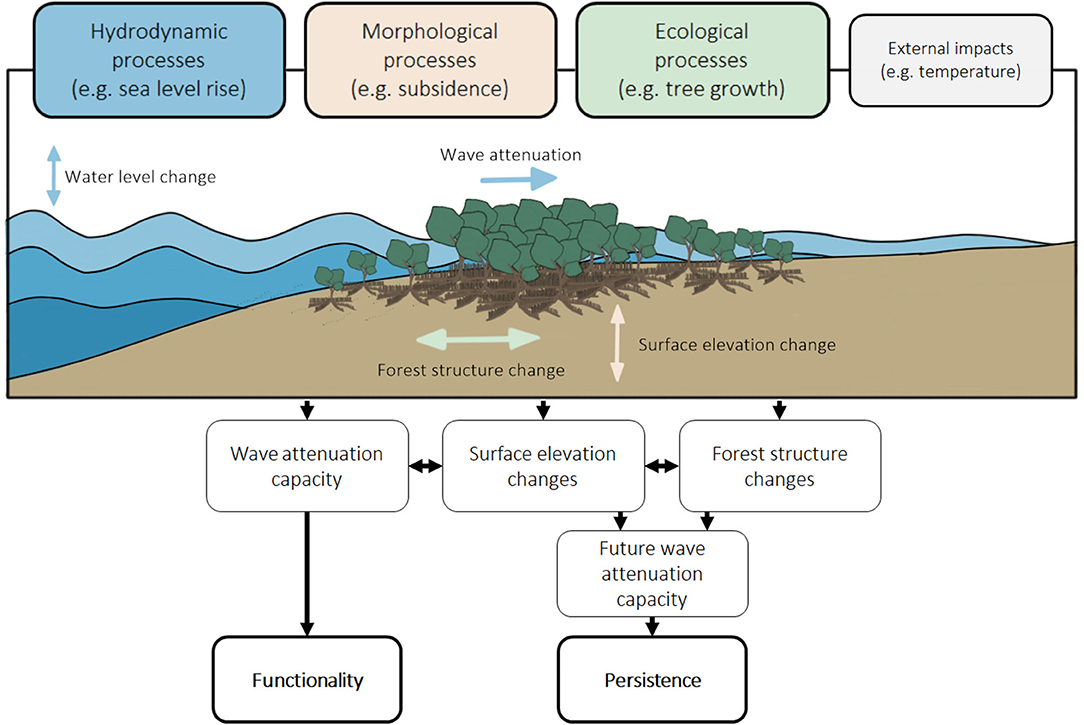
Figure 2. Interacting hydrodynamic, morphological and ecological processes in mangroves determine their functionality and persistence. These interactions are also affected by external impacts.
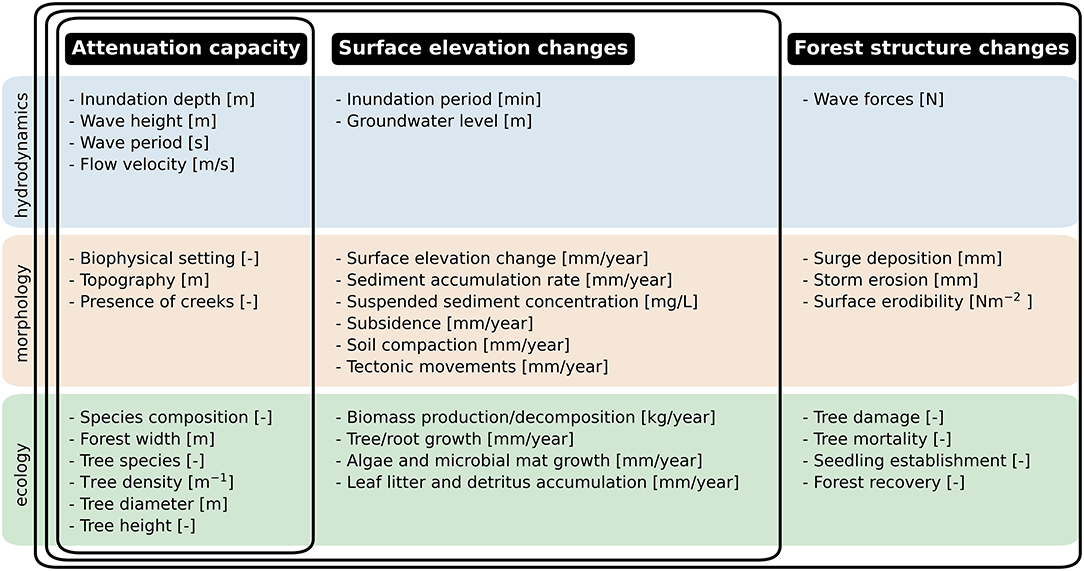
Figure 3. Hydrodynamic, morphological and ecological parameters of processes that determine the functionality and persistence of mangroves. The overlapping frames indicate which parameters are included for the attenuation capacity, surface elevation changes and forest structure changes, respectively.
Interactions between hydrodynamic, morphological and ecological processes occur across multiple temporal and spatial scales (Figure 4). This multi-scale interaction is the consequence of the different scales of the processes determining the attenuation capacity and changes in surface elevation and forest structure in mangroves. Three scale-levels are distinguished in these processes: the tree scale, focusing on local short-term dynamics around trees and tree patches; the forest scale, considering dynamics of entire transects through mangrove forests; and the ecosystem scale for the larger-scale environmental conditions of the mangroves (Figure 4). In this review, we focus on the tree and forest scales specifically, since flood risk reduction projects typically consider timescales up to decades and spatial scales within a manager's jurisdiction. Below, we review the relevant processes in mangroves for their functionality and persistence, of which a summarized overview is provided in Figure 3.
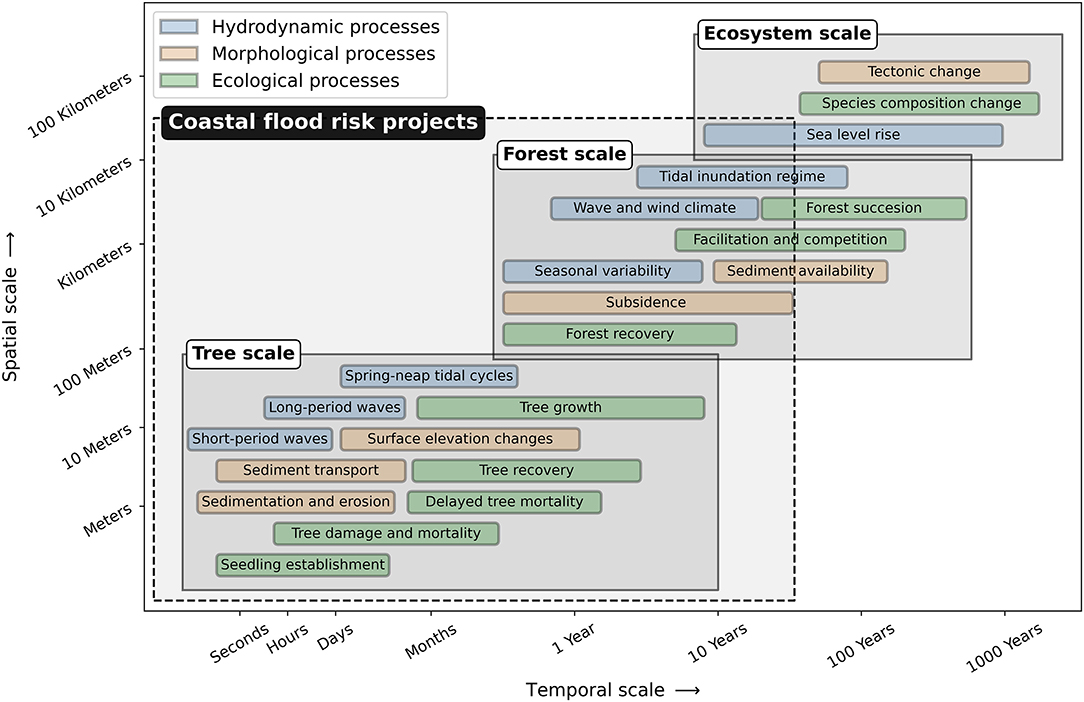
Figure 4. Spatial and temporal scales of interacting hydrodynamic, morphological and ecological processes in mangroves. A distinction is made between the most important processes that shape (1) trees and tree patches—tree scale, (2) forests along an elevation gradient—forest scale and (3) entire mangrove ecosystems—ecosystem scale. The spatial and temporal scale of coastal flood risk projects typically comprises the tree scale and forest scale.
2.1. Functionality: Wave Attenuation Capacity
The functionality of mangroves to reduce coastal flood risk depends on their capacity to attenuate propagating waves as they shoal onshore, thereby progressively reducing hydrodynamic exposure at the shore. Mangroves are exposed to different wave types characterised by their periods: gravity waves (1–20 s) such as wind waves; infragravity waves (20 s–5 min); long-period waves (5 min–12 h) such as tsunamis; ordinary tidal waves (12–24 h); and transtidal waves (>24 h) such as storm surges (Toffoli and Bitner-Gregersen, 2017). In estuaries, wave height and period are typically fetch-limited depending on wind direction and system attributes such as scale, bed elevation, tidal range (Mariotti and Fagherazzi, 2013; Green and Coco, 2014), whereas this is not the case on open muddy shores. Different coastal hazards may also generate different types and combinations of waves; for example, while an earthquake-induced tsunami produces long-period waves, tropical cyclones may generate a variety of waves such as gravity, infragravity and transtidal waves simultaneously. Although field evidence for the attenuation of shorter-period waves is increasing, field evidence is still limited for longer-period waves, such as for infragravity waves. As a result, the functionality of mangroves during high-magnitude flood events is yet to be quantified.
Previous studies indicate that the functionality of a mangrove forest depends on a number of hydrodynamic and biophysical attributes: inundation depth, wave height, wave period, flow velocity, intertidal topography, presence of creeks, forest width, tree species, tree stem density, tree stem diameter and tree height (Figure 3). Onshore propagating waves transform in shape and height, depending on subtidal and intertidal morphology. Waves can increase in height due to shoaling and decrease in height due to energy losses by bottom friction (i.e., skin friction) and drag caused by bed forms or other roughness elements (Green and Coco, 2014). The presence of mangrove trees imposes additional energy losses and enhances wave attenuation (Mazda et al., 1997a,b). The stems, branches, leaves and characteristic species-dependent aerial roots of mangroves all contribute to form drag. Additionally, mangroves can (locally) increase levels of turbulence, especially when flow velocities are high. In this way, they induce additional hydrodynamic energy losses (Furukawa and Wolanski, 1996; Norris et al., 2017).
For gravity waves, attenuation rates up to 0.012 per m (i.e., wave height reduces with 1.2% per m) of mangroves have been observed, depending on the inundation depth, tree species and tree density (Mazda et al., 1997a, 2006; Quartel et al., 2007; Horstman et al., 2014). Hence, mangroves can substantially reduce gravity wave energy, by up to 75% over a few tens of meters (Brinkman, 2006; Phuoc and Massel, 2006). Attenuation rates generally increase with wave height. Wave height therefore reduces non-linearly after entering mangroves. However, attenuation rates in mangroves reduce with increasing wave period. Because of this preferential attenuation of shorter-period waves, mean wave periods have been observed to increase across a mangrove forest (Horstman et al., 2014).
For ordinary tidal and trans-tidal waves, observed attenuation rates are two orders of magnitude smaller than for gravity waves, up to 2 cm per 100 m of mangroves, mainly depending on the presence of creeks (Krauss et al., 2009; Montgomery et al., 2018). Creeks penetrating mangrove forests provide low-resistance conduits for these long waves, and ordinary tidal waves have been found to even be amplified locally within creeks up to about 110% (Horstman et al., 2021); this study found that high-tide water levels within a 850-m long creek were attenuated by up to 1.2 cm per 100 m of channel length, whereas rates of up to 3.6 cm per 100 m were observed in the forest.
2.2. Persistence: Surface Elevation Changes
Surface elevation changes determine the persistence of mangrove forests primarily in two ways. Firstly, mangroves can gradually become submerged when the rate of surface elevation gain is not sufficient to keep pace with SLR. The ecosystem-scale persistence of mangroves depends on gaining sufficient surface elevation to keep up with sea-level rise (i.e., surface elevation capital; Lovelock et al., 2015b). Secondly, surface elevation changes due to excessive erosion or sedimentation can damage mangroves at the tree and forest scale when critical thresholds are exceeded. Instantaneous or gradual submergence increases the duration and depth of tidal inundations beyond species-specific physiological tolerances, and can result in mortality at the tree and forest scales (Figure 4). While insights in the persistence to SLR are increasing (Saintilan et al., 2020), limited field evidence of tree and forest scale surface elevation changes in a wide range of mangrove settings is available. This shortage of data hampers a comprehensive understanding of the processes determining surface elevation dynamics and their sensitivities and thresholds to environmental change.
Surface elevation changes in mangroves depend on hydroperiod, groundwater level, sediment accumulation, suspended sediment concentration, soil compaction, tectonic movements, above- and below-ground biomass production/decomposition, growth of algae and microbial mats and leaf litter and detritus accumulation (Figure 3). Mangroves are able to increase the surface elevation of their underlying substrate through either mineral-sediment accumulation, in-situ production of biomass, or a combination thereof (Krauss et al., 2014; Woodroffe et al., 2016; Swales et al., 2019). Sediments can accumulate in mangrove forests because the sediment carrying capacity of hydrodynamic flows reduces when their velocity reduces due to the enhanced vegetation drag. Sediment accumulation rates (SAR) in mangroves increase with suspended sediment concentrations (SSC) in the water, tidal inundation frequency, inundation depth and mangrove forest width (Furukawa and Wolanski, 1996; Horstman et al., 2015; Lovelock et al., 2015a; Swales et al., 2016; Willemsen et al., 2016). SAR also depend on the wave climate (Green and Coco, 2007) and typically reduce, or even become negative (i.e., erosion), with increasing wave energy (Norris et al., 2021). SAR show a large temporal and spatial variability (Van Santen et al., 2007; Smoak et al., 2013; Rogers et al., 2014; Swales et al., 2015, 2019), and vary across mangrove species and tree density (Furukawa and Wolanski, 1996; Krauss et al., 2003; Adame et al., 2010; Huxham et al., 2010; McKee, 2011; Howard et al., 2020). For example, SAR rates varied between 8.3 mm/year (Sonneratia alba) and 11.0 mm/year (Rhizophora spp.) in Micronesia, owing to different root structures and their effect on hydrodynamics (Krauss et al., 2003).
Surface elevation changes can also be controlled by surface and sub-surface biotic contributions. Growth of algae, microbial mats and accumulation of leaf litter and detritus on the forest surface can increase the surface elevation directly and/or indirectly by increasing SAR through the resulting increase of surface roughness (Cahoon et al., 2006; McKee, 2011). In the substrate, biomass production (i.e., root growth) can increase elevation, while losses have been attributed to biomass decomposition (Figure 3; Huxham et al., 2010; McKee, 2011; Lang'at et al., 2014).
Lastly, sub-surface physical contributions to surface elevation changes are soil compaction, ground water level change and tectonic movements (Rogers et al., 2005; Rogers and Saintilan, 2008; Lovelock et al., 2011a, 2015a). Lovelock et al. (2011a) found lower surface elevation gains in muddy mangroves (1.4 mm/year) than in sandy mangroves (5.9 mm/year) due to 8 mm/year of mud soil compaction. Sub-surface physical processes also show large temporal variability. For instance, the desiccation of soil in higher mangrove areas due to infrequent inundations can lead to varying soil compaction rates and surface elevation losses in time (Swales et al., 2019).
2.3. Persistence: Forest Structure Changes
The persistence of mangroves also depends on forest structure changes at the tree (e.g., tree mortality, inter-species competition) and forest (e.g., forest recovery, succession) scales (Figure 4). Individual trees and forests can become damaged when external impacts or surface elevation changes exceed certain thresholds: trees and tree patches can be defoliated, their branches can break or they can die off due to tree snapping, tree uprooting, burial of their aerial roots or tree blowdowns (Swales et al., 2007, 2015; Krauss and Osland, 2020). Field evidence of wind impact on trees is increasing, but field evidence of tree damage and/or tree mortality induced by wave action and associated instantaneous erosion or sedimentation remains very limited. Depending on the extent of the damage and the conditions of the remaining forest, mangrove trees and forests can recover. Ecosystem scale forest structure changes may also occur, by the adaptation of mangroves to e.g., climatic changes such as changes in temperature or rainfall (causing a shift in salinity) or migration of mangroves to areas suitable for seedling establishment.
Mangrove tree damage and mortality depends on storm severity (i.e., inundation depth, wave height, wind speed and wave forces), storm-event sediment deposition and/or erosion (i.e., surface erodibility), tree species, density and height (Figure 3). Primarily, tree damage in mangroves has been linked to the severity of tropical cyclones and the exposure of individual mangrove trees or stands (Paling et al., 2008; Doyle et al., 2009; Smith et al., 2009; Dutta et al., 2015; Feller et al., 2015; Long et al., 2016; Danielson et al., 2017) in mangrove forests that grow along coastlines exposed to cyclonic activity. Tree damage has been observed to increase rapidly when wind speeds of tropical cyclones exceed 130 km/h (Imbert, 2018). The exposure of mangrove trees to wind-induced structural damage was found to increase with increasing tree height (Milbrandt et al., 2006; Zhang et al., 2008, 2016; Salmo et al., 2013) and decreasing tree density (Vogt et al., 2012). Tree damage is also species-dependent (Steinke and Ward, 1989; Sherman et al., 2001; Kauffman and Cole, 2010). For example, higher mortality rates have been observed for Rhizophora spp., which were unable to self-support during large wind speeds (Macamo et al., 2016; Asbridge et al., 2018; Imbert, 2018). Rapid sedimentation and excess leaf litter (Steinke and Ward, 1989; Castañeda-Moya et al., 2010, 2020; Lovelock et al., 2011b) can also cause delayed mortality of mangrove trees, by smothering the above-ground aerial roots (Sherman et al., 2001; Paling et al., 2008; McKee and Vervaeke, 2009; Kauffman and Cole, 2010; Radabaugh et al., 2019). Tree species with pneumatophore roots (e.g., Avicennia spp.) or which are unable to sprout from living roots (e.g., Rhizophora spp.) are particularly vulnerable to rapid sedimentation (Macamo et al., 2016; Sidik et al., 2016; Asbridge et al., 2018; Imbert, 2018).
Mangrove forests can recover from damage to individual trees, but recovery rates and success decline with an increasing scale of damage. Forest recovery depends on tree damage, (delayed) tree mortality, surface elevation losses, the survival, delivery and establishment of seedlings and the forest health (Figure 3). The recovery process of storm-damaged mangroves can be impeded by post-storm surface elevation losses. Post-storm surface elevation losses can occur in forest gaps, resulting from tree loss. Rates of post-storm elevation loss of up to 32 mm/year have been reported (Lang'at et al., 2014). Rapid surface elevation losses triggered by storms may also impede propagule establishment and seedling recruitment (Cahoon et al., 2003; Vogt et al., 2012; Macamo et al., 2016; Asbridge et al., 2018), which is a critical step towards recovery of severely impacted mangroves with high tree mortality (Cahoon et al., 2003; Proffitt et al., 2006; Zhang et al., 2008). Seedling delivery may already be minimal after propagules and flowering material is dispersed during tropical cyclones (Steinke and Ward, 1989). The resilience and recovery of mangrove forests are further influenced by the ecological health and status of a mangrove forest prior to storm events. For example, deteriorated mangrove forests recover more slowly from severe tree damage caused by storms (Milbrandt et al., 2006; Harris et al., 2010; Primavera et al., 2016; Walcker et al., 2019).
3. Monitoring of Mangrove Functionality and Persistence
Measurements are required to assess the functionality and persistence of mangroves and to provide robust field evidence of their contribution to flood risk reduction. A range of field monitoring and remote sensing techniques are being used to measure hydrodynamic (section 3.1), morphological (section 3.2) and ecological (section 3.3) processes which are key for evaluating the functionality and persistence of mangroves (Figure 3). While field monitoring typically aims to obtain insights on tree- and forest-scale processes, remote-sensing techniques can provide insight on forest- and ecosystem-scale processes (Figure 4).
3.1. Hydrodynamic Processes and Extreme Weather Events
Hydrodynamic measurements in mangroves typically serve functionality assessments at the tree and forest scales. However, hydrodynamic processes also affect surface elevation changes and forest structure changes, related to their persistence (Figure 3). Hydrodynamic processes are often monitored in-situ by deploying field-monitoring equipment along a transect through the mangroves, for periods typically covering days-weeks. Long-term monitoring stations and remote sensing can be used for the monitoring of forest-to-ecosystem scale boundary conditions such as sea level and wave climate.
Inundation depths and periods are typically measured with autonomous low-frequency pressure sensors, or tide gauges (Mazda et al., 1997a; Phuoc and Massel, 2006; Quartel et al., 2007; Horstman et al., 2014; Montgomery et al., 2018; Swales et al., 2019). Alternatively, visual or automated observations of vertically placed staff gauges (Swales et al., 2019) or dyed cotton ropes (Ferwerda et al., 2007; Lovelock et al., 2015a) have been used. In addition, wave heights and periods of short-period waves have been measured with high-frequency pressure sensors (Mazda et al., 1997a; Brinkman, 2006; Horstman et al., 2014). In contrast to inundation depth measurements, short-period waves require a higher frequency (>2 Hz) to measure dynamic pressure differences in the water column.
Flow velocities have been measured with Electro-Magnetic Current (EMC) meters (Mazda et al., 1997a; Quartel et al., 2007; Van Santen et al., 2007), Acoustic Doppler Velocimeters (ADV) and Acoustic Doppler Current Profilers (ADCP) (Horstman et al., 2015, 2021; Willemsen et al., 2016). While the EMC and ADV provide the flow velocity at a single point, the ADCP can provide a velocity profile throughout the water column. Alternatively, downward-looking video cameras have been used to study flow velocities and patterns in mangroves (Furukawa and Wolanski, 1996). The latter technique provides spatial information on flow velocities at the water surface.
Ground water levels have been measured by means of the distance between the ground water table and the surface, through a pressure gauge positioned in a perforated plastic pipe (Rogers and Saintilan, 2008; Montgomery et al., 2018). Alternatively, rainfall measurements from nearby environmental stations have been used as an indicator for ground water levels (Rogers et al., 2005; Salmo et al., 2013; Swales et al., 2019).
Lastly, forest-to-ecosystem scale parameters such as sea level, wave conditions and wind climate have typically been obtained from nearby tide gauges, wave buoys or climate stations. Complemented by tide gauges, satellite altimetry measurements have also been shown to provide accurate measurements of the sea level over greater spatial extents (Church and White, 2011; Watson et al., 2015; Cipollini et al., 2017). Wind and wave climate (e.g., Izaguirre et al., 2011; Young and Donelan, 2018; Young and Ribal, 2019) and other essential climate variables can be obtained from satellite observations (e.g., Hollmann et al., 2013). Geostationary satellites, such as GOES and MeteoSat, can continuously monitor weather conditions and signal (tropical) depressions. In 2016, NASA's Cyclone Global Navigation Satellite System (CYGNSS) was launched, which employs a number of micro-satellites to measure (ocean) wind speed to improve extreme weather prediction (Ruf et al., 2016).
3.2. Morphology and Sediment Transport Processes
The present state morphology is an important indicator for the functionality of a mangrove forest, whereas temporal changes of the morphology due to sediment transport processes can provide indicators of its persistence. Both remote-sensing and field-monitoring techniques have been used to measure geomorphological and sediment transport processes in mangroves.
The present state morphology of mangrove forests have typically been obtained using remote-sensing of satellite imagery or aerial photogrammetry (e.g., in Krauss et al., 2003; Yuvaraj et al., 2014, respectively). Bhargava et al. (2021) applied remote sensing to observe the extent of the water surface and thus the topography relative to the water level. Additional morphological features, such as the presence of creeks or the intertidal topography in mangroves, have been obtained with (1) airborne or spaceborne remote-sensing with lidar (Zhang et al., 2008; Montgomery et al., 2018) or radar (Kuenzer et al., 2011), (2) field-scale remote sensing with drones (Beselly et al., 2021) and (3) field monitoring with RTK GPS and/or Total Station (Horstman et al., 2015; Leong et al., 2018; Walcker et al., 2019) or levelling tubes (Quartel et al., 2007; Van Santen et al., 2007). It is generally challenging to obtain topographic measurements in mangrove forests, as direct measurements are hampered by the dense vegetation and RTK GPS as well as remote sensing are affected by the tree canopy.
Sedimentation is measured over a range of time scales, from events or multiple days up to several months or years. Short-term accretion is typically measured using sediment traps, marker horizons, burial plates, sediment pins or sediment cores. Traps are thin canvas or plastic plates (Van Santen et al., 2007; Swales and Lovelock, 2020) or ceramic tiles (Horstman et al., 2015) and marker horizons consist of a white powdered feldspar clay layer (Cahoon and Turner, 1989). They are placed at the surface and from the deposited weight of sediments on the traps, or layer thickness on the markers, surface accretion can be determined. Burial plates work similarly, but a pin is pushed through the soil to measure the distance to plate installed at approximately 30 cm deep (Balke et al., 2013a). To measure not only accretion, but also erosion, sediment pins have been used. These are steel pins that are driven approximately 70-80 cm into the soil (Krauss et al., 2003; Huxham et al., 2010). Surface accretion due to storm surge deposition can be measured with cores (e.g., a Russian peat corer) (Smith et al., 2009; Castañeda-Moya et al., 2010, 2020), in which mangrove peat and storm deposits can be differentiated by means of their color. Overall, the above-mentioned weight, layer thickness or distances are measured either semi-continuous on temporal scales of days-weeks, or intermittent on temporal scales of months-years. The recently developed SED sensor forms a promising technique to monitor surface accretion and erosion in mangroves continuously on the timescale of months-years (Hu et al., 2020).
In addition to short-term accretion, long-term net sedimentation rates at the surface are determined with Pb10 – coring. Pb10 – coring is used to study sedimentary processes at decadal time scales, with dating of sediment deposits up to 100 years old (Quartel et al., 2007; Smoak et al., 2013; Swales et al., 2015).
Substrate elevation changes due to subsurface processes have been measured in the field, with surface elevation tables (SET) and more recently rod surface elevation tables (RSET, Cahoon et al., 2002b), and with satellite remote sensing. SETs and RSETs consist of a horizontal reference attached to a steel rod that is driven into the sediment down to the bedrock or to refusal (typically 10–25 m). Through the horizontal reference, fibre-glass pins can manually be lowered to measure the distance to the surface (Cahoon et al., 2002a). The benefit of SETs and RSETs is that they do not disturb the surface sediments and provide measurements of both mean and spatial variability in surface elevation changes at the tree scale. To obtain surface elevation changes both at the surface and in the substrate, the RSET approach is usually combined with the marker horizon (MH) technique. This way, the RSET-MH has become a widely applied methodology to measure elevation changes in mangroves (Webb et al., 2013; Cahoon, 2015). SET or RSET readings of surface and substrate elevation changes are typically collected at intervals of months to years. Alternatively, satellite remote sensing (radar interferometry) has been used to detect ecosystem-scale subsidence (Chaussard et al., 2013).
Sediment supply (for accretion) largely depends on suspended sediment concentrations (SSC) and hydroperiod. Measurements of SSC have been obtained from water samples (Furukawa and Wolanski, 1996; Adame et al., 2010) or indirectly from optical backscatter sensors (Phuoc and Massel, 2006; Van Santen et al., 2007; Balke et al., 2015) or acoustic backscatter sensors (Horstman et al., 2015; Venditti et al., 2016). It can be challenging to obtain reliable SSC measurements from optical and acoustic sensors, because they require calibration in controlled environments, which greatly depends on the type, size and colour of the sediment (Hoitink and Hoekstra, 2005; Downing, 2006). Alternatively, river discharge (Swales et al., 2019) or turbidity (Lovelock et al., 2015a) have been used as proxies for SSC and/or sediment supply. In turn, turbidity can be estimated from differences in water color on satellite imagery complemented with Secchi disk measurements (Gillis et al., 2017).
While measurements of surface elevation changes are abundant, there is limited knowledge of surface erosion in mangrove forests. The erodibility of the surface depends on a threshold bed shear stress: the critical shear stress for erosion (τe) and an erosion-excess bed shear stress gradient (M). M indicates how quickly erosion rates increase when the critical bed shear stress is exceeded. Both (τe) and M can be measured with the EROMES device (Stokes and Harris, 2015; Nguyen et al., 2019, 2020). By gradually increasing the rotational speed of a propeller in the EROMES tube, the flow velocity and exerted bed shear stress at the surface of the sediment sample in the tube follow. Thereafter, (τe) and M can be derived from the measurement of the turbidity in the tube (Stokes and Harris, 2015). Alternatively, the surface erodibility has been determined through the soil shear strength measured with a shear vane (McKee and Vervaeke, 2009). However, no direct relation between this soil shear strength, (τe) and M has been derived to date.
3.3. Forest Structure and Ecological Processes
Forest structure (i.e. width, composition, density) largely determines the functionality of mangroves. Hence, forest structure changes are important for their persistence. Mangrove forest structure has widely been measured by remote sensing. In addition, field monitoring has been performed to obtain tree scale measurements of relevant ecological processes. Forest width can be obtained from aerial photographs (e.g., Vogt et al., 2012) or satellite imagery (e.g., Long et al., 2016). These techniques can also provide additional insights on mangrove functionality through forest species composition, but additional field work may be required for ground referencing (e.g., Van Santen et al., 2007). Direct measurements are performed at the tree scale, typically by determining tree species, tree density, tree stem diameter, tree height and tree roots of representative trees in plots along a transect (e.g., Krauss et al., 2003; Horstman et al., 2014; Howard et al., 2020). Obtaining tree-scale data in support of mangrove functionality is labour intensive as measurements are generally made visually and/or manually. There are efforts being made to automize this data collection through photogrammetry (Liénard et al., 2016) or laser scanning, though still requiring active ground data collection.
Ecological processes are an indicator for the persistence of mangroves, since they can affect both the surface elevation and the forest structure. Tree-scale processes such as tree growth, root growth, growth of algae or microbial mats and accumulation of leaf litter and detritus are also typically obtained from field surveys. Tree growth has been measured with manual or recording dendrometer bands (i.e., stainless-steel bands which are placed around the tree stem) (Krauss et al., 2007; Lovelock et al., 2011b; Vilas et al., 2019) or by tagging and re-measuring individual trees (Feller et al., 2015). Root growth has been measured with root ingrowth bags (Cahoon et al., 2003; Lovelock et al., 2011a; McKee, 2011). These nylon mesh bags are filled with natural sediments, buried at the field site and later collected to measure the produced root biomass. The growth of algae or microbial mats at the surface may affect surface elevation change and can be measured from cut pneumatophore roots (Steinke and Ward, 1989) or by constructing surface screens (McKee, 2011). The obtained samples can be analysed for organic matter or Chlorophyll-a content (i.e., a proxy for algal biomass) (Horstman et al., 2018). Lastly, the contribution of leaf litter or detritus accumulation to surface elevation changes can be measured by using litter baskets (Steinke and Ward, 1989; Castañeda-Moya et al., 2010, 2020; Gladstone-Gallagher et al., 2016; Danielson et al., 2017).
The global distribution of mangrove forests have been mapped using optical and radar satellite remote sensing (Giri et al., 2011; Bunting et al., 2018). Forest- and ecosystem-scale persistence of mangroves has widely been studied with remote sensing, by monitoring forest structure changes due to tree damage and/or forest recovery in response to extreme weather events (Paling et al., 2008; Doyle et al., 2009; Vogt et al., 2012; Long et al., 2016; Macamo et al., 2016; Zhang et al., 2016; Walcker et al., 2019). These remote sensing studies typically relate forest structure to parameters such as the leaf area index (LAI) (Salmo et al., 2013; Feller et al., 2015), the normalized differential vegetation index (NDVI) (Long et al., 2016; Macamo et al., 2016; Walcker et al., 2019; Goldberg et al., 2020), the enhanced vegetation index (EVI), the land surface temperature (LST) or the foliage projective cover (FPC) (Dutta et al., 2015; Asbridge et al., 2018). Ground referencing is required to translate these indices into forest structure information. Lymburner et al. (2020) obtained long-term (decades) annual mangrove cover from optical satellite data complemented by lidar data. Zhang et al. (2016) concluded that the normalized differential moisture index (NDMI) provides a sound indicator to assess mangrove forest structure changes. Recent remote sensing approaches have even managed to detect tree species and tree height from space-borne images (Pham et al., 2019; Wang et al., 2019), and to estimate tree biomass from drone images (Jones et al., 2020). Simard et al. (2019) used SRTM SAR data, together with spaceborne lidar data, to map the maximum canopy height of mangroves worldwide. These tree- and forest-scale insights on mangrove forest structure are particularly helpful to assess the functionality and persistence of mangroves (Figure 4).
4. Models of Mangrove Functionality and Persistence
Models are essential in predicting and assessing the functionality and persistence of mangroves. Firstly, classification models are required to categorize mangroves for implementation in flood risk management (section 4.1). Secondly, empirical (section 4.2) and numerical (section 4.3) mangrove models can be used to simulate extreme flooding conditions (functionality), and to predict their long-term development (persistence) to assess the consequences of environmental and human-induced changes. While classification models for mangroves have been widely applied, the development of empirical and numerical models with predictive skill is an active field of research (e.g., Peters et al., 2020; Buffington et al., 2021). For instance, first numerical approaches for short-wave attenuation, tidal propagation and sediment accumulation in mangroves have been developed (e.g., Willemsen et al., 2016), but are lacking ecological processes such as tree growth and mortality. In contrast, mangrove forest growth models (e.g., Grueters et al., 2019) typically disregard morphological processes, while models for the morphological evolution of mangroves (e.g., Buffington et al., 2021) disregard hydrodynamic processes such as waves.
4.1. Classification Models
Classification models aim at obtaining first qualitative assessments of the functionality and persistence of mangroves by categorizing qualitative dimensionless parameters such as the biophysical setting and tree species. Classification models are applicable on ecosystem, forest and tree scales (Table 1). The biophysical setting of mangroves indicates the geomorphic setting as well as the dominant sedimentary processes (Worthington et al., 2020). In terms of sedimentary settings, mangroves can be classified as either terrigenous or carbonate. Terrigenous mangroves represent the majority of the world's mangrove cover and are dominated by fluvial sediment supply. In contrast, sediments in carbonate mangroves mainly come from calcareous sedimentation. In terms of geomorphic settings, deltaic, estuarine, lagoonal and open coast mangroves have been classified (Thom, 1984; Woodroffe, 1992; Worthington et al., 2020).
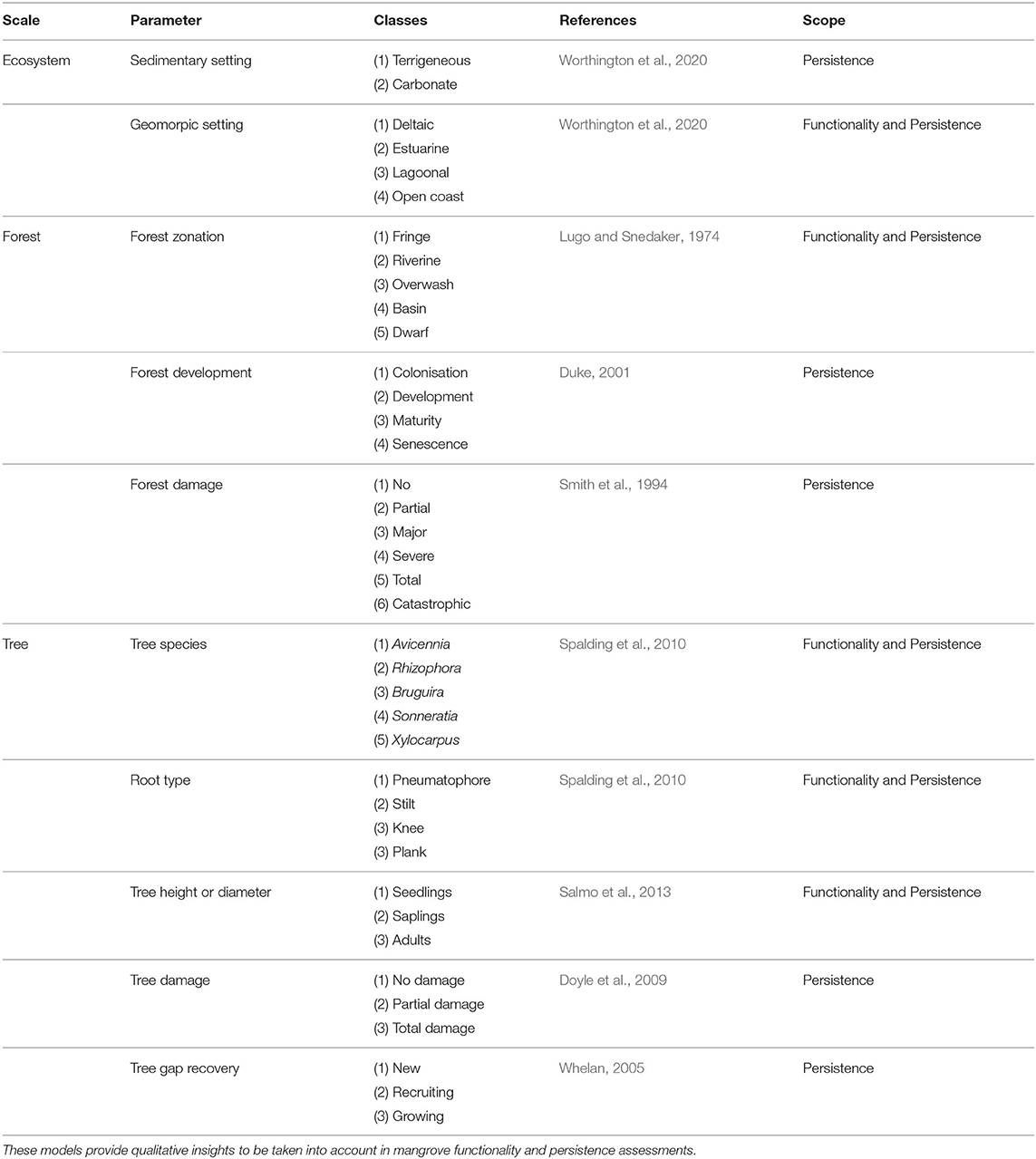
Table 1. Existing classification models for the categorization of mangroves in flood risk management.
At the smaller forest scale, mangroves consist of different topographic zones with characteristic species compositions, across for example fringe, riverine, overwash, basin and dwarf mangroves (Lugo and Snedaker, 1974). Topographic zones are often based on their respective distances to a river or open ocean, but they are typically also characterised by differences in tree species, density, height, elevation, tidal inundation regime and exposure to waves. Tidal inundation regime is a key control on tree species (Van Loon et al., 2016). Alternatively, topographic zones in mangroves have been named after the type of trees: scrub or mature forest (Adame et al., 2010).
To standardize the application of mangroves to reduce flood risk, tree scale parameters such as species, height and diameter need to be classified/generalized. Common mangrove species are from the following genera: Avicennia, Rhizophora, Bruguira, Sonneratia and Xylocarpus (Spalding et al., 2010). They are characteristised by different types of aerial root systems, such as pneumatophores for Avicennia, stilt roots for Rhizophora, knee roots for Bruguiera and plank roots for Xylocarpus. Tree height and diameter can be used to distinguish between seedlings (dbh <2.5 cm), saplings (height <1.3 m and dbh <5 cm) and adults (height > 1.3 m or dbh > 5 cm) (Vogt et al., 2012; Salmo et al., 2013), but also other thresholds or sub-classes have been used (e.g., Ferwerda et al., 2007; Radabaugh et al., 2019).
Lastly, important qualitative parameters to standardize for flood risk reduction are the tree damage, forest development and gap recovery. Tree damage has been classified with a variety of classification models at different scales (Table 1; Smith et al., 1994; Sherman et al., 2001). For instance, Smith et al. (1994) distinguishes between: (1) no damage, (2) partial damage (<25% defoliation), (3) major damage (25–50% defoliation, branches broken), (4) severe damage (>75% defoliation, trees fallen), (5) total damage (>50% of trees fallen) and (6) catastrophic damage (>75% of trees fallen). Duke (2001) considered a classification model for forest development and recovery, while Whelan (2005) discussed several conceptual gap recovery stages (Table 1).
4.2. Empirical Models
Empirical models are basic quantitative assessments of the functionality and persistence of mangroves, mostly focusing on the forest scale. Empirical models are particularly useful for preliminary assessments, when system knowledge is still incomplete (Table 2). Initial empirical models on the forest-scale functionality of mangroves relate wave attenuation in mangroves to a specific wave attenuation rate r. For short-period waves, an exponential decay in wave height H along a transect through mangroves was found (Table 2; Mazda et al., 2006). To estimate the attenuated wave height at a given location, the relation requires the incident wave height at the open/unvegetated boundary of the forest H0 and the distance inland of the forest fringe x. Wave attenuation rates for short-period waves have been observed of approximately 0.001–0.01 (see Table 1 in Horstman et al., 2014). For longer-period waves, a linear decay in the inundation depth h along a transect through the mangroves was found (Krauss et al., 2009). Similar to attenuation of short-period waves, this equation requires the inundation depth at the open boundary of the forest h0 and the related distance inland x. For long waves, observed attenuation rates ranged between 4.21·10−5 and 9.45·10−5 (Krauss et al., 2009).
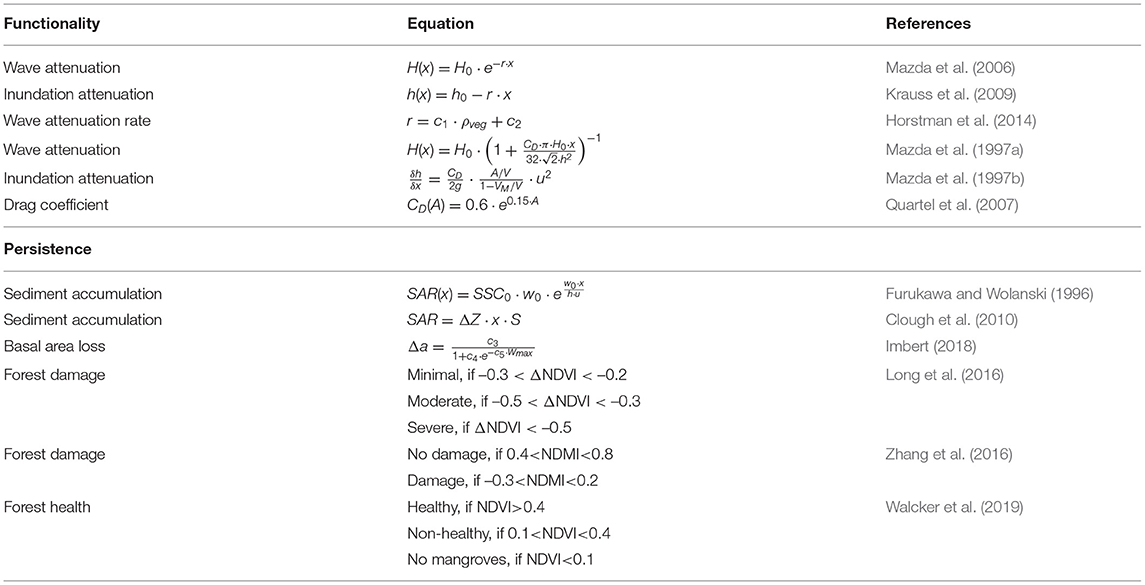
Table 2. Existing empirical models for preliminary quantitative assessments of mangrove functionality and persistence.
Additional empirical relations have been established to estimate forest-scale functionality, including the tree-scale characteristics of mangroves explicitly. Firstly, attenuation rates have been related to the volume of the mangrove vegetation per unit volume of water (ρveg) (Mazda et al., 1997b). ρveg was found to be directly related to the short-wave attenuation rate by means of two calibration coefficients (Table 2). Secondly, attenuation in mangroves can be estimated by means of applying the drag force concept. This way, the exerted drag forces of the mangrove trees on hydraulic flows are estimated (Morison et al., 1950) by allocating a drag force coefficient CD to the trees. Both above-mentioned approaches are similar, as indicated by the work of Quartel et al. (2007), who related CD to ρveg by means of the frontal area of the mangroves A. In general, defining CD for mangrove is challenging due to its species and depth variability.
For the persistence of mangroves, an empirical model was developed by Furukawa and Wolanski (1996) to estimate sediment accumulation rates along a transect through mangroves (i.e., forest scale). This model requires the depth-averaged sediment concentration at the seaward boundary of the forest SSC0, the settling velocity of the sediments ws, the inundation depth h and the flow velocity u. A widely applied model for assessing mangrove and wetland persistence to sea-level rise is the Sea Level Affecting Marshes Model (SLAMM; Li et al., 2015; Mogensen and Rogers, 2018). The model has implemented a simplistic empirical relation that assumes that the surface accretion rate is a function of relative elevation to mean sea level ΔZ, the distance from a river or tidal channel x and a salinity effect S (Table 2; Clough et al., 2010).
The persistence of mangroves also depends on expected forest structure changes. Imbert (2018) developed an empirical model to estimate the loss of trunk-basal area in mangroves Δa based on the maximum wind speeds that occurred during a tropical cyclone (Wmax; Table 2). The equation requires three calibration coefficients (c3−c5) which depend on the topographic zone of the forest and the tree species. Remote-sensing studies have made use of empirical relations between the forest structure (i.e., forest damage) and parameters such as NDVI. These relations have been either based on absolute values (Zhang et al., 2016; Walcker et al., 2019) or the variablity in NDVI (Long et al., 2016).
4.3. Numerical Models
Numerical mangrove models are developed to obtain quantitative predictions of the attenuation of extreme events (functionality) and the system's development under environmental changes and human (engineering) interventions (persistence). Numerical models provide predictions based on hydrodynamic, morphological and ecological processes that are solved in a quantitative framework.
4.3.1. Hydrodynamic Processes
Numerical wave models generally simulate propagation of short-period and longer-period waves with different approaches. Propagation of short-period waves is computed based on a wave-energy balance approach, in which mangroves are included as an additional energy loss term ϵv. A widely applied formulation for ϵv was developed by Mendez and Losada (2004):
Herein, ρ is the water density, N is the tree stem density, D is the tree stem diameter, g is the gravitational acceleration, k is the wave number, σ is the wave frequency, α is the relative vegetation height, h is the inundation depth and Hrms is the root-mean-square wave height. This formulation is currently implemented in numerical wave models such as SWAN (Booij et al., 1999; Suzuki et al., 2012) and XBeach (Roelvink et al., 2009). An example of a numerical study of short-wave attenuation in mangroves with Equation 1 is the study of van Wesenbeeck et al. (2016).
In contrast, propagation of long-period waves (>20 s) is simulated by solving the non-linear shallow water equations (continuity and momentum). In numerical models that simulate the propagation of longer-period waves (e.g., Delft3D, Telemac) the effects of mangroves have been implemented in the momentum equation in two different ways: (1) by increasing the bed roughness and (2) by including an additional momentum loss term. The first approach increases the actual bed shear stress due to surface roughness (τb) with a vegetation roughness component (τv) (c.f. Gourgue et al., 2020). The second approach reduces the flow momentum with a drag term based on vegetation properties such as density, diameter, height and drag coefficient (c.f. Brückner et al., 2019). The flow resistance concept is generally favoured in morphological simulations since an artificially increased bed roughness may result in over-estimations of bed shear stresses τb and hence of erosion rates (section 4.3.2 and Equation 4).
While the vegetation roughness (of fully submerged vegetation) is typically calculated with the formulation of Baptist et al. (2007) (Equation 2), the flow resistance coefficient λ for emergent vegetation can be calculated with Equation 3 (Deltares, 2020).
Zhang et al. (2012) and Menéndez et al. (2019) followed the artificial roughness approach by increasing the bed roughness coefficients (i.e., the Manning coefficient) from 0.04 to 0.14 to compensate for the presence of mangroves. The studies of Horstman et al. (2015) and Willemsen et al. (2016) describe tidal wave propagation and sedimentation in mangroves applying the flow resistance approach, where the physical properties of the mangroves are accounted for.
4.3.2. Surface Elevation Changes and Sediment Transport Processes
Surface elevation changes occurring due to surface dynamics (e.g., sedimentation) and sub-surface processes (e.g., subsidence) are generally solved with separate numerical approaches. For instance, the above-mentioned hydrodynamic models include sediment transport processes and resulting sediment deposition or erosion, but no sub-surface processes. A widely applied approach to calculate erosion and deposition rates of cohesive sediments are the Partheniades-Krone equations (Equations 4, 5 for erosion and deposition, respectively).
Herein, ER is the erosion rate, M is the erodibility of the bed, τb is the bed shear stress exerted by the flow and τe is the critical bed shear stress for erosion, DR is the deposition rate, ws is the settling velocity of the sediment, SSC is the suspended sediment concentration and τd is the critical bed shear stress for deposition. The Partheniades-Krone equations do not explicitly include the effects of mangroves on erosion or sedimentation. Instead, erosion (and resuspension) in mangroves is reduced because of a reduced bed shear stress exerted by the flow (τb), induced via the reduction in flow momentum.
The stabilizing effects of mangrove roots on the erodibility of the bed were included in the approach of Van Maanen et al. (2015), by increasing the critical threshold for erosion τe based on the presence of below-ground mangrove biomass (Equation 6). Their model also accounted for reduced subsidence ΔZorg based on a relation with the below-ground biomass (Equation 7). Hence, they artificially increased the erosion threshold and decreased the subsidence when mangroves were present, but no calibration or validation of this model was provided.
Herein, τe, 0 is the critical bed shear stress for erosion when no mangroves were present, Kcr is a constant of 0.1, Bb is the below-ground biomass, Bb, mature is the maximum below-ground biomass (1.2 kg/m2) and Korg is a constant of 1 mm/year (Van Maanen et al., 2015).
Model studies on the ecosystem-scale persistence of mangroves typically consider relative sea-level rise (i.e., the change in elevation of the forest floor with respect to the sea level), in response to absolute sea-level rise scenarios (IPCC, 2019). Instead of solving sediment transport processes, these model approaches typically calculate surface elevation changes based on the annually-averaged hydroperiod (e.g., SLAMM). Buffington et al. (2021) also included the effects of leaf litter deposition, root decomposition and soil compaction, all primarily related to the present species composition, on surface elevation changes in mangroves.
4.3.3. Forest Structure and Ecological Processes
Numerical models to simulate forest structure changes in mangroves generally distinguish between seedlings and trees. While seedling establishment models relate the establishment of seedlings to hydrodynamic and morphological processes, tree development models aim to simulate the tree life cycle including growth, competition and mortality.
The Windows of Opportunity (WoO) model quantifies three critical sequential life stages of seedlings: propagule delivery and root anchoring; seedling establishment; and seedling growth and survival (Balke et al., 2013b). Although propagule delivery depends on seasonal timing of propagule release with cycles of intertidal flat inundation and exposure, models have generally assumed unrestricted delivery during the fruiting season or year round (Balke et al., 2015). For seedling establishment, an inundation-free period of approximately 2–5 days is required for seedlings to anchor their roots in the soil (Balke et al., 2015). During the third life stage, growing seedlings gain resistance (i.e., τp) to detachment by bed erosion and thereby survive conditions up to a certain threshold of hydrodynamic and morphological stresses. The WoO model allocates a certain shear strength to seedlings after establishment (τp, 0). This strength gradually increases over time with the growth of the root system (root length, biomass) that is assumed to increase as a function of seedling age a. The WoO model is summarized by:
Herein, T1 and T2 are the required time periods in WoO-1 (seedling establishment) and WoO-2 (seedling growth), and c6 and c7 are calibration coefficients. Seedling detachment and resulting mortality is imposed when hydrodynamic and morphological stresses exceed the strength of seedlings.
Tree growth models consider tree growth, tree competition and tree mortality in relation to environmental conditions such as the availability of light, nutrients and salinity (Peters et al., 2020). These models are generally individual-based and consider the dynamics of individual trees, while forest-scale dynamics depend on the interaction between neighbouring trees (Vincenot et al., 2016). A widely applied equation in mangrove tree-growth models is the Shugart equation (Equation 9; Shugart, 1984; Chen and Twilley, 1998). The Shugart equation assumes tree growth is a function of existing tree diameter Di and height Hi, and is limited by a growth reduction factor freduction that is generally related to the nutrient availability and soil salinity (c.f. Grueters et al., 2014, 2019):
Herein, G is the growth rate, Dmax is the maximum tree diameter, Hmax is the maximum tree height, c8, c9, c10 are calibration parameters, freduction is the reduction factor due to availability of light, nutrients and salinity, and fcompetition is the reduction factor due to competition with neighbouring trees. Tree mortality is generally induced when tree growth falls below a certain threshold. This can occur either when trees are large, or because either growth reduction by inundation or competition have substantially increased. In the model of Van Maanen et al. (2015), the growth reduction factor was related to tidal-inundation frequency, which served as a proxy for soil salinity. Consequently, in their model the reduction factor was referred to as the inundation factor (finundation). Berger and Hildenbrandt (2000) proposed an additional growth-limiting competition factor, expressing the competition for resources between neighbouring trees. In general, a radius around trees is determined where individual tree growth is not measureably impaired by neighbouring trees. Growth rates are reduced once the radii of neighbouring trees begin to overlap. Recently, a mechanistic alternative to the Shugart equation is being developed, in which mangrove tree growth has been directly related to prevailing environmental conditions such as salinity and ground water flows (Peters et al., 2014, 2018; Bathmann et al., 2020).
Ecosystem-scale mangrove persistence models, such as the model developed by Buffington et al. (2021) and SLAMM also consider species composition changes. In contrast to solving the establishment, growth and mortality of individual trees, Buffington et al. (2021) consider a probability of random seedling establishment and logarithmic growth of the basal area coverage of trees. Changes in the species composition can then occur with sea-level rise, since the seedling establishment probability differs between mangrove species and depends on the relative elevation compared to sea level, or hydroperiod. Instead, the SLAMM model applies pre-defined relations between species composition (i.e., wetland vegetation type) and the relative elevation compared to sea level to simulate species composition changes (Mogensen and Rogers, 2018).
5. Guidelines for Incorporating Mangroves Into Flood Risk Reduction
A range of frameworks and guidelines exist for engineering and management to reduce flood risk with nature-based solutions (NbS). These guidelines mainly focus on the design (i.e., engineering) and implementation (i.e., management) processes, or the steps to take, whereas there is limited guidance on specific design considerations, or design support. Traditional engineering manuals such as the Coastal Engineering Manual (USACE, 2001) provide design support for traditional engineering infrastructure, but cannot always be applied to NbS. The standardization and implementation of mangroves to reduce coastal flood risk requires design support on the prediction and assessment of both mangrove functionality and persistence. Design support always requires field evidence (i.e., system knowledge; section 2) in combination with continuous learning and assessment of system dynamics (i.e. monitoring techniques; section 3) and input from design tools (i.e., modelling approaches; section 4).
5.1. Existing Frameworks and Guidelines for NbS
Frameworks developed for the design and implementation of NbS, such as PIANC (2011); De Vriend and Van Koningsveld (2012); USACE (2015); World Bank (2017) commonly present a clear sequence of key principles to be applied in the process of flood risk projects. Here we will briefly elaborate on the five key principles provided by the World Bank (2017), a comprehensive framework for the implementation of NbS to reduce coastal flood risk (Figure 5):
• Principle 1 - consider the larger system scale – is particularly important for NbS because natural dynamics (i.e., variability in functionality and persistence) are an intrinsic part of the solution (Figure 1).
• Principle 2 - assess the risks and benefits – recognises the fact that the risks and benefits of NbS (i.e., uncertainty in functionality and persistence) are more multi-dimensional compared to hard-engineering solutions.
• Principle 3 - develop a standardized performance evaluation – requires repeatable and transparent assessment methods of the performance of NbS in terms of functionality and persistence.
• Principle 4 - integrate with the principles of ecosystem conservation and restoration – recognises that ecosystem conservation and restoration principles must be integrated in NbS to ensure their functionality and persistence. For mangroves, a number of restoration guidelines have been produced, such as IUCN and WRI (2014); Lewis and Brown (2014); PTFCF (2018). Importantly, many of these guidelines advocate for the strong participation and inclusion of local communities.
• Principle 5 - manage the ecosystem adaptively – recognises that NbS need adaptive management, requiring continuous monitoring and frequent performance evaluations for maintained functionality and persistence.
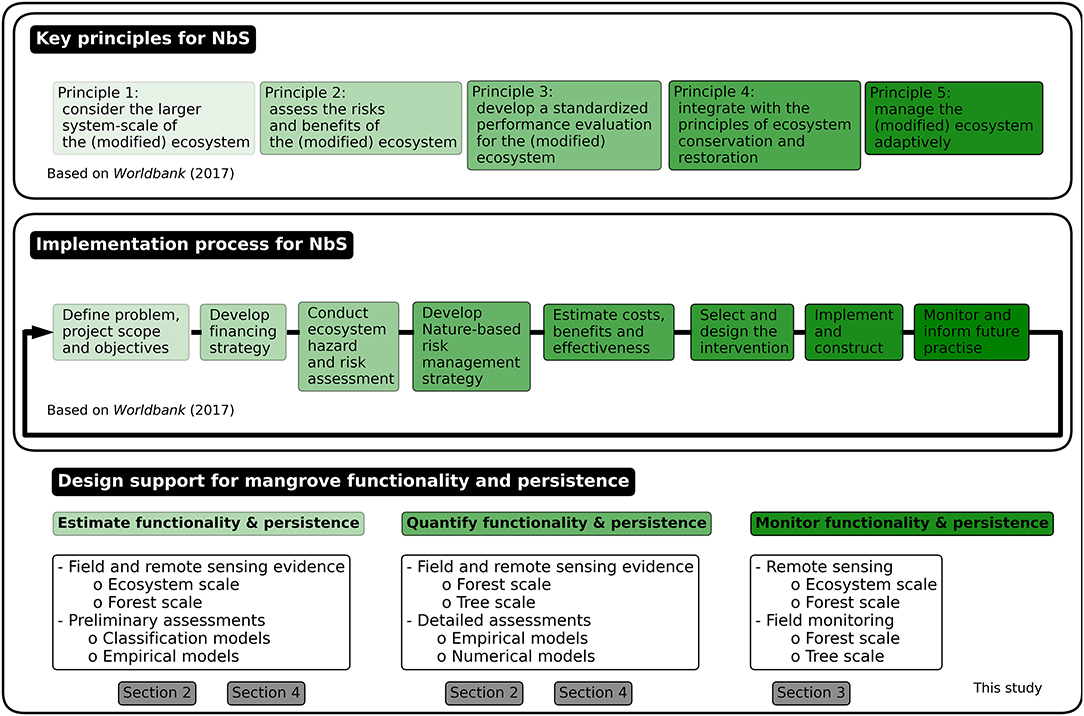
Figure 5. Key principles and implementation steps for NbS to reduce coastal flood risk, based on World Bank (2017). This review has addressed design support and tools for mangroves. The green shading indicates when these can be operationalized in existing frameworks and guidelines.
Guidelines for the reduction of flood risk with NbS describe the step-wise design or implementation process for these projects (Opperman, 2014; Spalding et al., 2014; UNEP, 2014; Bridges et al., 2015; NOAA, 2015; UNISDR, 2015; World Bank, 2016, 2017; Bilkovic et al., 2017; IPCC, 2019; van Eekelen and Bouw, 2020). Figure 5 presents the implementation steps described in World Bank (2017). In general, these steps are similar to those in traditional flood risk management guidelines such as the Coastal Engineering Manual (USACE, 2001). In contrast, the guidelines for NbS do not provide the same level of design guidance yet. Robust design support for the application of mangroves to reduce flood risk is thus not available (Schoonees et al., 2019). Hence, standardised assessments and design practices for the definition, quantification and monitoring of locally suitable critical success factors for the implementation of mangroves in coastal flood risk projects are yet to be developed.
5.2. Design Support for Mangrove Functionality and Persistence
The functionality and persistence of mangroves for coastal flood risk reduction need to be predicted and assessed throughout the complete design and implementation process. Figure 5 presents at what stages system knowledge from field and remote sensing evidence (existing data), monitoring (new data) and design tools (e.g., models) are needed in the design and implementation process.
The preliminary design phase (until the “estimate costs, benefits and effectiveness” step in Figure 5) requires input based on pre-existing knowledge of the system's functioning (section 2) at forest-to-ecosystem scales, in combination with classification and empirical models (sections 4.1 and 4.2, respectively). These inputs and models are instrumental for the preliminary assessment of the functionality and persistence of the considered mangrove forest. In these early design stages, potential alternative solutions to reduce coastal flood risk must also be considered, such as (1) doing nothing; (2) replacing mangroves with hard-engineering infrastructure; (3) complementing or enhancing mangroves with hard-engineering infrastructure in hybrid-engineering solutions. To assess what alternative has the highest likelihood of achieving the project objectives, design support is required at this stage. In general, the selected alternative will depend on the hydrodynamic forcing and the availability of natural resources such as sediments, nutrients and accommodation space (Schoonees et al., 2019), but also on the (perceived) urgency to reduce coastal flood risk in a sustainable manner (Morris et al., 2020). In mangroves, for instance, it must be understood whether the natural sediment supply is sufficient to keep pace with local SLR, and whether artificial sediment supply would be a viable alternative. The availability and development of design support is critical to come to such understanding.
The detailed design phase (in Figure 5 the “select and design” step) requires tree-to-forest scale system knowledge (section 2) as well as empirical (section 4.2) and numerical models (section 4.3). These stages require detailed site information and advanced (process-based) models to develop a functional and persistent solution in any particular area. This is done by detailed assessments of the selected alternative solutions at the forest scale. For instance, mangroves can be supported in a hybrid-engineering approach in several ways, by: (1) protection and restoration of existing mangroves; (2) additional flood protection infrastructure to increase mangrove functionality; (3) additional wave attenuation infrastructure to increase mangrove persistence at high-energy sites, or a combination of these approaches. The local hydrodynamic forcing and the availability of accommodation space are important factors that need to be considered at the forest scale when assessing (and enhancing) functionality and persistence of mangroves. Some design estimations have been developed, such as the estimated 150 m of convex mudflat that would be required to restore mangroves by placing wave attenuation structures to stimulate natural seedling establishment (Ecoshape, 2020). However, apart from the guidelines for mangrove restoration (e.g., Lewis and Brown, 2014), there are limited resources available to support mangrove functionality or persistence assessments, such as Ellison (2012), World Bank (2016), and World Bank (2019).
Monitoring of mangrove forests, in the field and via remote sensing, plays an essential role to inform the implementation process as well as to evaluate project outcomes (in Figure 5 the “monitor and inform future practice” step). Monitoring plays a key role to improve system understanding and to inform adaptive management over the design life of a project. In practice, monitoring should inform continued performance assessments on different scales to quantify the functionality and persistence of mangroves. As such, monitoring approaches need to aim at system thresholds that may affect mangrove functionality and persistence, while adaptive management strategies need to be ready in case any of these thresholds is (likely to be) exceeded (Lewis et al., 2016). The development of such monitoring approaches and management guidelines is one of the main challenges ahead (section 6). The multitude of ongoing mangrove restoration projects can provide a wealth of experiences and practices to develop these approaches and guidelines for future flood risk projects (Ellison et al., 2020).
6. Challenges to Mangroves for Flood Risk Reduction
6.1. Mangrove Functionality: Design Storm Conditions
The functionality of hard-engineering infrastructure has traditionally been designed and assessed by means of its design conditions or design storm: the most severe storm conditions in which the infrastructure is functional without structural failure. This threshold is also known as the Ultimate Limit State (ULS). Storm conditions that exceed the design storm of hard-engineering infrastructure can result in failure (Figure 6A) and induce associated flood damage on communities (i.e., social, economic, cultural activities) protected by this infrastructure. The design conditions or design storm concept is not applicable to mangroves, and this induces additional uncertainty in functionality assessments (Figure 1).
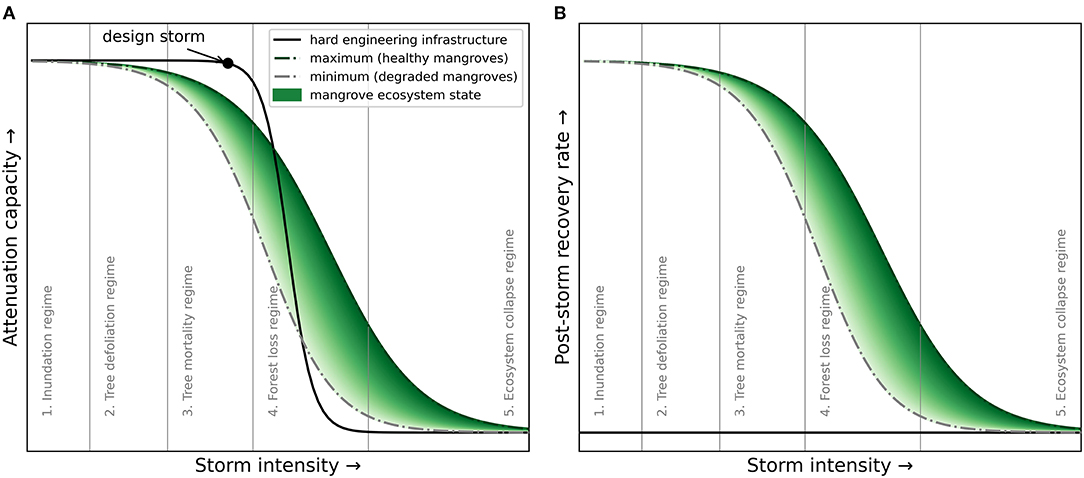
Figure 6. (A) Attenuation capacity of hard-engineering infrastructure and mangroves in relation to the intensity of storms and (B) the post-storm recovery rate of mangroves in relation to the storm intensity. The attenuation capacity of hard-engineering infrastructure reduces rapidly due to failure when the design storm intensity is exceeded. The attenuation capacity of mangroves reduces gradually depending on a range of site-specific thresholds-related failure mechanisms, the mangrove ecosystem state (i.e., health) and capacity to attenuate waves. Hard-engineering infrastructure cannot recover from storm damage, but mangroves can recover depending on the intensity of damage and the ecosystem state, amongst other site-specific conditions. Generally applicable storm-impact regimes (section 2.3) are indicated, but site-specific processes and thresholds need to be determined to apply mangroves in flood risk projects.
In contrast to the full protection offered by, for example, widely-implemented levees or dikes, the functionality of mangroves is dependent on the storm intensity. Mangroves may completely attenuate mild storms (i.e., limited wave heights and surge levels), but their storm attenuation capacity (i.e., functionality) gradually decreases with increasing storm intensity (Figure 6A). This decreasing functionality occurs due to the lower attenuation capacity of mangroves for longer-period waves (section 2.1), but also due to a range of increasingly severe natural failure mechanisms (e.g., tree mortality) (section 2.3). Moreover, this decreasing functionality is greatly site-specific depending on the local storm-induced disturbances (e.g., winds and waves) as well as the characteristics of the mangrove forest. In general, owing to the larger width and typical spatial heterogeneity of mangrove forests, failure mechanisms are assumed to occur more gradually compared to the ULS of hard-engineering infrastructure. Consequently, in flood risk reduction projects with mangroves, the design storm concept is not applicable.
The implementation of mangroves in coastal flood risk projects requires an understanding of mangrove functionality during extreme events and variations in mangrove functionality through time (Figure 6A). The evidence of the flood damage reduction due to mangroves during tsunamis remains circumstantial (Marois and Mitsch, 2015) and existing assessment models also do not include failure mechanisms, which substantially increases the uncertainty in mangrove functionality assessments. This uncertainty can be overcome by continued field and remote sensing monitoring of the key parameters at specific sites (Figure 3; e.g., wave height combined with forest width and tree density). These monitoring data on mangrove functionality and state under varying storm conditions can reduce the uncertainty of mangrove functionality and can also help improve assessment models. In addition, the temporal variability in mangrove functionality could be captured by an ecosystem state, or resilience, parameter indicating mangroves health and hence functionality (Figure 6A). This can for instance be achieved by relating mangrove functionality to remote sensing parameters, such as NDVI (Walcker et al., 2019), which then could be parameters of special interest to optimize in a long-term adaptive management approach (section 6.3).
6.2. Short-Term Mangrove Persistence: Failure and Recovery Mechanisms
Hard-engineering infrastructure has been developed as relatively rigid structures that do not change much with time. By contrast, mangrove functionality is continuously affected by failure and recovery processes. The temporal variability in functionality that can be caused by the occurrence of failure mechanisms in mangroves substantially increases the uncertainty in persistence assessments (Figure 1). To incorporate mangroves in coastal flood risk projects, a comprehensive understanding of the inherently site-specific failure and recovery mechanisms, their thresholds and signals of stress (i.e., early warning signals) is required. Present knowledge on the failure and recovery mechanisms in mangroves and their thresholds is limited due to a lack of systematic and site-specific field evidence. For instance, little is known about the mechanisms and quantity of bed erosion (or deposition) that is induced within mangrove forests by storms (section 2.2) and how this subsequently impacts mangrove trees (section 2.3). A comprehensive mangrove dynamics model with predictive skill for mangrove failure and recovery would be needed to reduce the uncertainty in mangrove persistence assessments (section 4.3).
We identify five conceptual storm impact-recovery regimes of mangroves, in order of increasing impact/spatial scale (Figure 6A): (1) tree-recovery regime; (2) tree-defoliation regime; (3) tree-mortality regime; (4) forest-loss regime; and (5) ecosystem-collapse regime. The post-storm recovery rate decreases when mangroves go through these increasingly severe damage regimes (Figure 6B). Mangrove trees that have experienced defoliation during storm events can recover relatively quickly (e.g., several months; Feller et al., 2015; Danielson et al., 2017; Radabaugh et al., 2019). By contrast, recovery from the tree-mortality regime is expected to take 2–5 years (e.g., Sherman et al., 2001; Long et al., 2016; Zhang et al., 2016; Danielson et al., 2017) and recovery from the forest-loss regime can take up to 20 years (Ferwerda et al., 2007; Paling et al., 2008; Imbert, 2018). Lastly, following an ecosystem collapse, no recovery is expected within the engineering design life (i.e., decades; Osland et al., 2020). These regimes provide a first model to assess failure and recovery of mangroves, but further research is required on the quantification of these regimes and their thresholds at different sites.
Monitoring of forest structure changes in the field, on the ground and by means of remote sensing, can support improved understanding of abovementioned regimes and their respective thresholds. A combination of observations of local forcing conditions in the field (e.g., waves) and larger scale forest damage from remote sensing can be particularly valuable for future persistence assessments. In addition, mangrove dynamics models combining hydrodynamic, morphodynamic and ecological processes need to be developed for mangroves, and can be validated with such observations, to reduce the uncertainty in mangrove persistence assessments.
Following a better understanding of the failure regimes of mangroves, and their recovery, mangrove persistence can also be related to an ecosystem state or resilience parameter, as discussed in section 6.1. Such a parameter could aid in identifying signals of stress (Lewis et al., 2016) and critical vulnerability thresholds depending on the present state of the mangroves (Ellison, 2012). Knowing these signals and thresholds is essential for an adaptive management approach. Accordingly, alternative engineering interventions should be developed that can be implemented when assessments show inadequate functionality or persistence, or when early-warning signals indicate a probability of exceedance of the mangrove thresholds. These engineering interventions may aim to prevent failure mechanisms (e.g., by reducing critical conditions to below-threshold values) or to aid the recovery process. Such an adaptive management approach that aims to increase mangrove resilience, limit failure mechanisms and increase post-storm recovery rates (green shading in Figure 6B) corresponds with the concepts of resilience engineering (Hollnagel et al., 2006).
6.3. Long-Term Mangrove Persistence: Adaptive Management
While hard-engineering infrastructure is mostly static, mangroves can adapt to environmental changes through their sediment trapping and regenerating capacity (Figure 1). For hard-engineering infrastructure, flood risk reduction projects typically consider timescales up to decades, but mangroves have persistent capacities to adapt to environmental changes on (much) longer timescales e.g., by keeping pace with sea-level rise. To utilize the long-term persistence of mangroves, adaptive management approaches require a long-term perspective. While the variability and uncertainty in mangrove conditions and dynamics require frequent assessments of their functionality and persistence (sections 6.1, 6.2), this long-term perspective for mangrove persistence needs to be integrated in planning, requiring an approach such as dynamic adaptation policy pathways (DAPP) (Figure 7; Haasnoot et al., 2013). DDAP describes how uncertainties can be included in adaptive management, by means of a long-term perspective, possible future interventions and a decision-support framework for when to take actions (e.g., when mangrove thresholds may be exceeded).
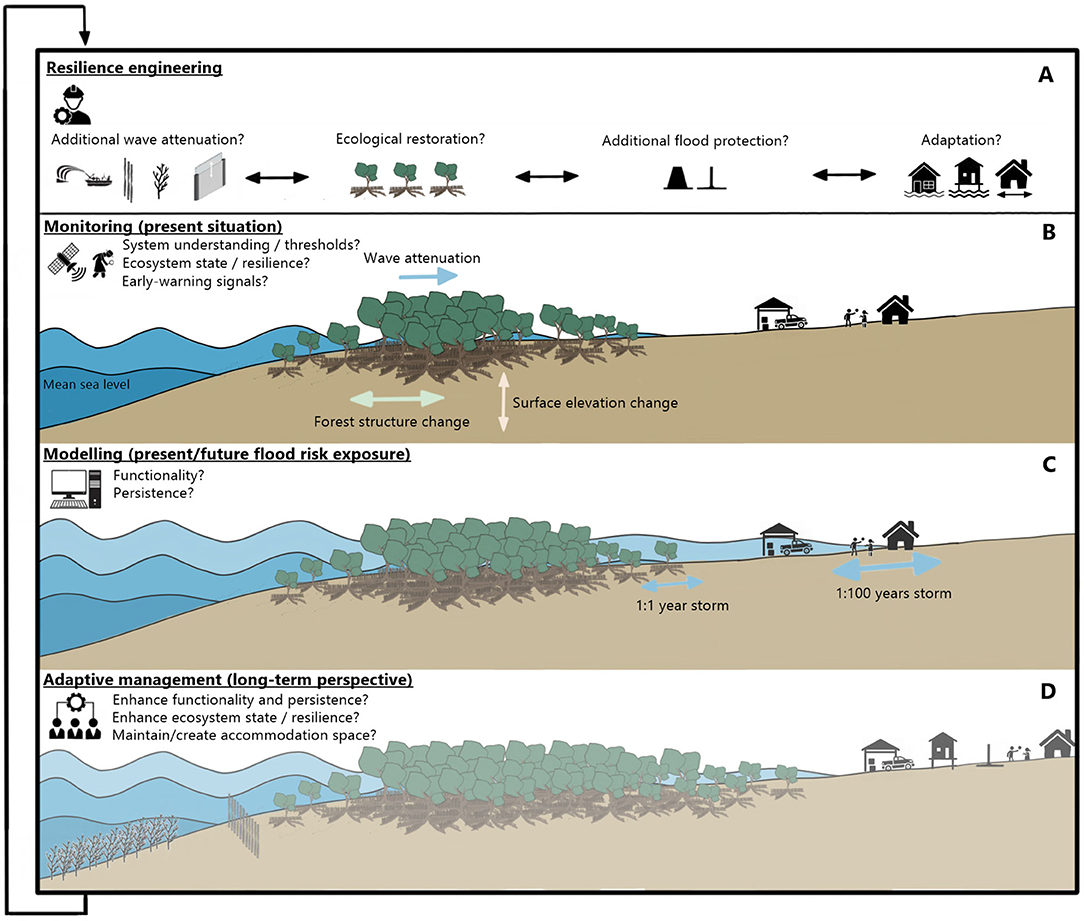
Figure 7. Schematic overview of an adaptive management approach with (A) (temporary) engineering interventions, in which humans enhance and adapt to the functionality and persistence of the mangroves. The mangroves are (B) monitored, (C) their functionality and persistence are assessed and predicted to determine the necessity of (temporary) engineering interventions within (D) a long-term perspective to reduce coastal flood risk with mangroves. The uncertainty of flooding events due to the variability in mangrove functionality and the uncertainty of monitoring and modelling tools is indicated with the blue arrows in (C).
The expansion of the considered timescales in flood risk projects incorporating mangroves, means that additional hydrodynamic processes (e.g., sea level rise), morphodynamic processes (e.g., the availability of sediment) and ecological processes (e.g., tree facilitation) should be internalized (Figure 4). For instance, storm intensity and frequency are expected to increase (Woodruff et al., 2013) and mangroves may become more frequently and more severely damaged. When mangroves cannot recover or seedling establishment fails, this may cause a retreating mangrove forest, reducing its functionality and persistence. Hybrid approaches, including engineering interventions, such as wave attenuation structures that either reduce tree damage or support seedling establishment, could be considered to ameliorate (changing) environmental conditions to below-threshold values, thereby enhancing mangrove functionality and persistence. Examples of such interventions could be permeable dams (Winterwerp et al., 2020), sediment nourishments (Baptist et al., 2019; de Vet et al., 2020), artificial reefs, rock fillets (Morris et al., 2020), or adjustable dams (Sadat-Noori et al., 2021) (Figure 7A).
Considering sea level rise scenarios (IPCC, 2019), tidal inundation depths and durations are expected to increase worldwide (although to different extents based on local tectonic setting and natural and human-induced impacts on vertical land motion). This relative (local) sea level rise will, amongst others, accommodate seedling delivery on the landward side of the mangroves, potentially inducing landward expansion or migration of the forest (Enwright et al., 2016). The preparation of landward accommodation space for future mangrove colonization, e.g., by rehabilitating present aquaculture ponds (van Bijsterveldt et al., 2020), could be considered to enhance mangrove functionality and persistence. In order to benefit from this adaptation capacity of mangroves, landward migration must be considered in present designs of additional flood protection infrastructure for hybrid solutions. Accordingly, dikes or seawalls may temporarily increase mangrove functionality, but they will reduce mangrove persistence by limiting inland accommodation space and potential for landward migration (Winterwerp et al., 2005, 2013).
Reducing coastal flood risk with mangroves at specific sites requires an adaptive management approach that aims at optimizing mangrove functionality in the short-term and accommodating mangrove persistence in the long term (Figure 7). Environmental conditions in which mangroves develop to their full potential in terms of functionality and persistence must be created, supported and/or maintained through an DAPP approach. Continuous monitoring of the mangroves' state and thresholds, as well as external stressors such as storms and sea level rise, is required in support of this approach (Figure 7B). Monitoring efforts also allow for continued improvement of model assessments and predictions of mangrove functionality and persistence (Figure 7C). When mangrove functionality or persistence are inadequate, or conditions have exceeded mangrove thresholds (or are close to doing so), management or engineering interventions may be considered, modelled and implemented (Figure 7A), keeping in mind a long-term perspective in support of mangrove persistence (Figure 7D). Ultimately, utilising and optimising the functionality and persistence of mangroves to reduce flood risk may also require humanity to accommodate and adapt to the flood risk reducing capacities that mangroves have to offer. The required support for such adaptation can only be achieved by strongly involving local communities and the many actors that benefit from mangroves' ecosystem services.
7. Conclusions
Coastal zones around the world face the continued challenge of supporting population growth while experiencing the consequences of climate change and increasing exposure to coastal flooding. Mangrove ecosystems can help in attenuating flood events (functionality) and can recover in, and adapt to, a changing climate (persistence). In combination with the provision of multiple other ecosystem services, mangroves are recognized as a suitable ecosystem to act as a NbS to reduce coastal flood risk. Many national agencies and international organizations are now advocating for the protection and restoration of mangroves, and their incorporation along with a gradient of engineering structures as hybrid solutions for coastal protection. If we are to implement mangroves to reduce coastal flood risk, then we need to understand, monitor and model the key processes, dynamics and thresholds that underpin mangrove functionality and persistence.
This review provides an overview of existing evidence, monitoring techniques and model approaches of the hydrodynamic, morphological and ecological processes relevant for mangrove functionality and persistence. Knowledge gaps in the processes and thresholds that determine wave attenuation and changes in surface elevation and forest structure in mangroves have been identified. Evidence of mangrove functionality and persistence in changing and extreme conditions is required to address these knowledge gaps and is key for the development of adaptive management strategies that can deal with this variability and uncertainty in mangrove dynamics.
In flood risk reduction projects with mangroves, the inherently site-specific processes, dynamics and thresholds that determine mangrove functionality and persistence need to be quantified and monitored. This knowledge and capability is critical for the successful maintenance of mangroves as a NbS over time horizons useful to coastal engineers, planners and decision makers, as well as the communities, infrastructure, economic activities and natural habitats they are protecting. This review shows the wide range of techniques that is required from different disciplines in order to monitor and model the processes relevant for mangroves to reduce coastal flood risk. When grouped around functionality and persistence, such monitoring techniques and models can provide the site-specific information required for the application of NbS guidelines, and can provide coastal managers and engineers with the evidence and tools required for adaptive management of mangroves to reduce coastal flood risk.
Author Contributions
EH, DF, and AS designed the research project. RG, EH, DW, and KW defined the scope of the review. RG performed the literature review and wrote the article with input from EH, DW, KW, DF, and AS. All authors reviewed and revised the manuscript. The article is approved by all authors.
Funding
This study was part of the Mangrove-RESCUE project, funded by the Dutch Research Council (NWO - Grant no. 15899). The NIWA Strategic Science Investment Fund project (contract FWCE2104 Estuary Sediment Dynamics and Evolution) supported AS contribution to this study.
Conflict of Interest
The authors declare that the research was conducted in the absence of any commercial or financial relationships that could be construed as a potential conflict of interest.
References
Adame, M. F., Neil, D., Wright, S. F., and Lovelock, C. E. (2010). Sedimentation within and among mangrove forests along a gradient of geomorphological settings. Estuarine Coastal Shelf Sci. 86, 21–30. doi: 10.1016/j.ecss.2009.10.013
Arkema, K. K., Guannel, G., Verutes, G., Wood, S. A., Guerry, A., Ruckelshaus, M., et al. (2013). Coastal habitats shield people and property from sea-level rise and storms. Nat. Clim. Change 3, 913–918. doi: 10.1038/nclimate1944
Asbridge, E. F., Lucas, R., Rogers, K., and Accad, A. (2018). The extent of mangrove change and potential for recovery following severe Tropical Cyclone Yasi, Hinchinbrook Island. Ecol. Evolut. 8, 10416–10434. doi: 10.1002/ece3.4485
Balke, T., Bouma, T. J., Herman, P. M., Horstman, E. M., Sudtongkong, C., and Webb, E. L. (2013a). Cross-shore gradients of physical disturbance in mangroves: implications for seedling establishment. Biogeosciences 10, 5411–5419. doi: 10.5194/bg-10-5411-2013
Balke, T., Swales, A., Lovelock, C. E., Herman, P. M., and Bouma, T. J. (2015). Limits to seaward expansion of mangroves: translating physical disturbance mechanisms into seedling survival gradients. J. Exper. Marine Biol. Ecol. 467, 16–25. doi: 10.1016/j.jembe.2015.02.015
Balke, T., Webb, E. L., van den Elzen, E., Galli, D., Herman, P. M., and Bouma, T. J. (2013b). Seedling establishment in a dynamic sedimentary environment: a conceptual framework using mangroves. J. Appl. Ecol. 50, 740–747. doi: 10.1111/1365-2664.12067
Baptist, M. J., Babovic, V., Uthurburu, J. R., Keijzer, M., Uittenbogaard, R. E., Mynett, A., et al. (2007). On inducing equations for vegetation resistance. J. Hydraulic Res. 45, 435–450. doi: 10.1080/00221686.2007.9521778
Baptist, M. J., Gerkema, T., van Prooijen, B. C., van Maren, D. S., van Regteren, M., Schulz, K., et al. (2019). Beneficial use of dredged sediment to enhance salt marsh development by applying a ‘Mud Motor'. Ecol. Eng. 127, 312–323. doi: 10.1016/j.ecoleng.2018.11.019
Bathmann, J., Peters, R., Naumov, D., Fischer, T., Berger, U., and Walther, M. (2020). The MANgrove–GroundwAter feedback model (MANGA)–Describing belowground competition based on first principles. Ecol. Model. 420:108973. doi: 10.1016/j.ecolmodel.2020.108973
Berger, U., and Hildenbrandt, H. (2000). A new approach to spatially explicit modelling of forest dynamics: Spacing, ageing and neighbourhood competition of mangrove trees. Ecol. Model. 132, 287–302. doi: 10.1016/S0304-3800(00)00298-2
Beselly, S. M., van der Wegen, M., Grueters, U., Reyns, J., Dijkstra, J., and Roelvink, D. (2021). Eleven years of mangrove–mudflat dynamics on the mud volcano-induced prograding delta in east Java, Indonesia: integrating UAV and satellite imagery. Remote Sens. 13, 1084. doi: 10.3390/rs13061084
Bhargava, R., Sarkar, D., and Friess, D. A. (2021). A cloud computing-based approach to mapping mangrove erosion and progradation: case studies from the Sundarbans and French Guiana. Estuarine Coastal Shelf Sci. 248:106798. doi: 10.1016/j.ecss.2020.106798
Bilkovic, D. M., Mitchell, M. M., La Peyre, M. K., and Toft, J. D. (2017). Living Shorelines. The Science and Management of Nature-Based Coastal Protection. Boca Raton, FL: Taylor and Francis.
Booij, N., Ris, R. C., and Holthuijsen, L. H. (1999). A third-generation wave model for coastal regions 1. Model description and validation. J. Geophys. Res. 104, 7649–7666. doi: 10.1029/98JC02622
Borsje, B. W., van Wesenbeeck, B. K., Dekker, F., Paalvast, P., Bouma, T. J., van Katwijk, M. M., et al. (2011). How ecological engineering can serve in coastal protection. Ecol. Eng. 37, 113–122. doi: 10.1016/j.ecoleng.2010.11.027
Bouma, T. J., van Belzen, J., Balke, T., Zhu, Z., Airoldi, L., Blight, A. J., et al. (2014). Identifying knowledge gaps hampering application of intertidal habitats in coastal protection: opportunities and steps to take. Coastal Eng. 87, 147–157. doi: 10.1016/j.coastaleng.2013.11.014
Bridges, T. S., Wagner, P. W., Burks-Copes, K. A., Bates, M. E., Collier, Z. A., Fischenich, C. J., et al. (2015). Use of Natural and Nature-Based Features (NNBF) for Coastal Resilience. Technical report, U.S. Army Corps of Engineers, Washington, DC.
Brinkman, R. M. (2006). Wave Attenuation in Mangrove Forests: An Investigation Through Field and Theoretical Studies. Ph.D. thesis, James Cook University.
Brückner, M. Z. M., Schwarz, C., and Dijk, W. M. V. (2019). Salt marsh establishment and eco-engineering effects in dynamic estuaries determined by species growth and mortality. J. Geophys. Res. 124, 2962–2986. doi: 10.1029/2019JF005092
Buffington, K. J., MacKenzie, R. A., Carr, J. A., Apwong, M., Krauss, K. W., and Thorne, K. M. (2021). Mangrove Species' Response to Sea-Level Rise Across Pohnpei, Federated States of Micronesia. Technical report, U.S. Geological Survey Open-File Report 2021–1002.
Bunting, P., Rosenqvist, A., Lucas, R. M., Rebelo, L.-M., Hilarides, L., Thomas, N., et al. (2018). The global mangrove watch–a new 2010 global baseline of mangrove extent. Remote Sens. 10:1669. doi: 10.3390/rs10101669
Cahoon, D. R. (2015). Estimating Relative Sea-Level Rise and Submergence Potential at a Coastal Wetland. Estuaries Coasts 38, 1077–1084. doi: 10.1007/s12237-014-9872-8
Cahoon, D. R., Hensel, P., Rybczyk, J., Mckee, K. L., Proffitt, C. E., and Perez, B. C. (2003). Mass tree mortality leads to mangrove peat collapse at bay islands, Honduras after Hurricane Mitch. J. Ecol. 91, 1093–1105. doi: 10.1046/j.1365-2745.2003.00841.x
Cahoon, D. R., Hensel, P. F., Spencer, T., Reed, D. J., McKee, K. L., and Saintilan, N. (2006). “Coastal wetland vulnerability to relative sea-level rise: wetland elevation trends and process controls,” in Ecological Studies, Vol. 190, Chapter 12, (Berlin; Heidelberg: Springer-Verlag), 271–292.
Cahoon, D. R., Lynch, J. C., Hensel, P., Boumans, R., Perez, B. C., Segura, B., et al. (2002a). High-precision measurements of wetland sediment elevation: I. recent improvements to the sedimentation-erosion table. J. Sedimentary Res. 72, 730–733. doi: 10.1306/020702720730
Cahoon, D. R., Lynch, J. C., Perez, B. C., Segura, B., Holland, R. D., Stelly, C., et al. (2002b). High-precision measurements of wetland sediment elevation: Ii. the rod surface elevation table. J. Sedimentary Res. 72, 734–739. doi: 10.1306/020702720734
Cahoon, D. R., and Turner, R. E. (1989). Accretion and canal impacts in a rapidly subsiding wetland ii. feldspar marker horizon technique. Estuaries 12, 260–268. doi: 10.2307/1351905
Carrasquilla-Henao, M., and Juanes, F. (2017). Mangroves enhance local fisheries catches: a global meta-analysis. Fish Fish. 18, 79–93. doi: 10.1111/faf.12168
Castañeda-Moya, E., Rivera-Moroy, V. H., Chambers, R. M., Zhao, X., Lamb-Wotton, L., Gorsky, A., et al. (2020). Hurricanes fertilize mangrove forests in the Gulf of Mexico (Florida Everglades, USA). Proc. Natl. Acad. Sci. U.S.A. 117, 4831–4841. doi: 10.1073/pnas.1908597117
Castañeda-Moya, E., Twilley, R. R., Rivera-Monroy, V. H., Zhang, K., Davis, S. E., and Ross, M. (2010). Sediment and nutrient deposition associated with hurricane wilma in mangroves of the Florida coastal everglades. Estuaries Coasts 33, 45–58. doi: 10.1007/s12237-009-9242-0
Chaussard, E., Amelung, F., Abidin, H., and Hong, S.-H. (2013). Sinking cities in indonesia: Alos palsar detects rapid subsidence due to groundwater and gas extraction. Remote Sens. Environ. 128, 150–161. doi: 10.1016/j.rse.2012.10.015
Chen, R., and Twilley, R. R. (1998). A gap dynamic model of mangrove forest development along gradients of soil salinity and nutrient resources. J. Ecol. 86, 37–51. doi: 10.1046/j.1365-2745.1998.00233.x
Cheong, S.-M., Silliman, B., Wong, P. P., van Wesenbeeck, B., Kim, C.-K., and Guannel, G. (2013). Coastal adaptation with ecological engineering. Nat. Clim. Change 3:787–791. doi: 10.1038/nclimate1854
Church, J. A., and White, N. J. (2011). Sea-Level Rise from the Late 19th to the Early 21st Century. Surveys Geophys. 32, 585–602. doi: 10.1007/s10712-011-9119-1
Cipollini, P., Calafat, F. M., Jevrejeva, S., Melet, A., and Prandi, P. (2017). Monitoring Sea Level in the coastal zone with satellite altimetry and tide gauges. Surveys Geophys. 38, 33–57. doi: 10.1007/s10712-016-9392-0
Clough, J., Park, R., and Fuller, R. (2010). SLAMM 6 Beta Technical Documentation. Technical Report May, Warren Pinnacle Consulting, Inc.
Danielson, T. M., Rivera-Monroy, V. H., Casta neda-Moya, E., Brice no, H., Travieso, R., Marx, B. D., et al. (2017). Assessment of Everglades mangrove forest resilience: implications for above-ground net primary productivity and carbon dynamics. Forest Ecol. Manag. 404, 115–125. doi: 10.1016/j.foreco.2017.08.009
de Vet, P. L., van Prooijen, B. C., Colosimo, I., Ysebaert, T., Herman, P. M., and Wang, Z. B. (2020). Sediment disposals in estuarine channels alter the eco-morphology of intertidal flats. J. Geophys. Res. 125:e2019JF005432. doi: 10.1029/2019JF005432
De Vriend, H. J., and Van Koningsveld, M. (2012). Building With Nature. Dordrecht: Thinking, Acting and Interacting Differently. Ecoshape, Building with Nature.
Downing, J. (2006). Twenty-five years with OBS sensors: the good, the bad, and the ugly. Continental Shelf Res. 26, 2299–2318. doi: 10.1016/j.csr.2006.07.018
Doyle, T. W., Krauss, K. W., and Wells, C. J. (2009). Landscape analysis and pattern of hurricane impact and circulation on mangrove forests of the everglades. Wetlands 29, 44–53. doi: 10.1672/07-233.1
Duke, N. C. (2001). Gap creation and regenerative processes driving diversity and structure of mangrove ecosystems. Wetlands Ecol. Manag. 9, 257–269. doi: 10.1023/A:1011121109886
Dutta, D., Das, P. K., Paul, S., Sharma, J. R., and Dadhwal, V. K. (2015). Assessment of ecological disturbance in the mangrove forest of Sundarbans caused by cyclones using MODIS. Nat. Hazards 79:775–790. doi: 10.1007/s11069-015-1872-x
Ecoshape (2020). Building with Nature Guideline. Available at: https://publicwiki.deltares.nl/display/BTG/Guideline (Accessed June 30, 2020).
Ellison, A. M., Felson, A. J., and Friess, D. A. (2020). Mangrove rehabilitation and restoration as experimental adaptive management. Front. Marine Sci. 7:327. doi: 10.3389/fmars.2020.00327
Ellison, J. C. (2012). Climate Change Vulnerability Assessment and Adaptation Planning for Mangrove Systems. Washington, DC: World Wildlife Fund (WWF).
Enwright, N. M., Griffith, K. T., and Osland, M. J. (2016). Barriers to and opportunities for landward migration of coastal wetlands with sea-level rise. Front. Ecol. Environ. 14, 307–316. doi: 10.1002/fee.1282
Ezcurra, P., Ezcurra, E., Garcillán, P. P., Costa, M. T., and Aburto-Oropeza, O. (2016). Coastal landforms and accumulation of mangrove peat increase carbon sequestration and storage. Proc. Natl. Acad. Sci. U.S.A. 113, 4404–4409. doi: 10.1073/pnas.1519774113
Feller, I. C., Dangremond, E. M., Devlin, D. J., Lovelock, C. E., Proffitt, E., Rodriguez, W., et al. (2015). Nutrient enrichment intensifies hurricane impact in scrub mangrove ecosystems in the Indian River Lagoon, Florida, USA. Ecology 96, 2960–2972. doi: 10.1890/14-1853.1
Ferwerda, J. G., Ketner, P., and McGuinness, K. A. (2007). Differences in regeneration between hurricane damaged and clear-cut mangrove stands 25 years after clearing. Hydro 591:35–45. doi: 10.1007/s10750-007-0782-7
Friess, D. A., Krauss, K. W., Horstman, E. M., Balke, T., Bouma, T. J., Galli, D., et al. (2012). Are all intertidal wetlands naturally created equal? Bottlenecks, thresholds and knowledge gaps to mangrove and saltmarsh ecosystems. Biol. Rev. 87, 346–366. doi: 10.1111/j.1469-185X.2011.00198.x
Furukawa, K., and Wolanski, E. (1996). Sedimentation in mangrove Forests. 1, 3–10. doi: 10.1023/A:1025973426404
Gedan, K. B., Kirwan, M. L., Wolanski, E., Barbier, E. B., and Silliman, B. R. (2011). The present and future role of coastal wetland vegetation in protecting shorelines: answering recent challenges to the paradigm. Climatic Change 106, 7–29. doi: 10.1007/s10584-010-0003-7
Gillis, L. G., Jones, C. G., Ziegler, A. D., van der Wal, D., Breckwoldt, A., and Bouma, T. J. (2017). Opportunities for protecting and restoring tropical coastal ecosystems by utilizing a physical connectivity approach. Front. Marine Sci. 4:374. doi: 10.3389/fmars.2017.00374
Giri, C., Ochieng, E., Tieszen, L. L., Zhu, Z., Singh, A., Loveland, T., et al. (2011). Status and distribution of mangrove forests of the world using earth observation satellite data. Glob. Ecol. Biogeogr. 20, 154–159. doi: 10.1111/j.1466-8238.2010.00584.x
Gladstone-Gallagher, R. V., Lohrer, A. M., Lundquist, C. J., and Pilditch, C. A. (2016). Effects of detrital subsidies on soft-sediment ecosystem function are transient and source-dependent. PLoS ONE 11:e0154790. doi: 10.1371/journal.pone.0154790
Goldberg, L., Lagomasino, D., Thomas, N., and Fatoyinbo, T. (2020). Global declines in human-driven mangrove loss. Glob. Change Biol. 26, 5844–5855. doi: 10.1111/gcb.15275
Gourgue, O., Belzen, J., Schwarz, C., Bouma, T. J., Koppel, J., and Temmerman, S. (2020). A convolution method to assess subgrid–scale interactions between flow and patchy vegetation in biogeomorphic models. J. Adv. Model. Earth Syst. 13:e2020MS002116. doi: 10.1029/2020MS002116
Green, M. O., and Coco, G. (2007). Sediment transport on an estuarine intertidal flat: Measurements and conceptual model of waves, rainfall and exchanges with a tidal creek. Estuarine Coastal Shelf Sci. 72, 553–569. doi: 10.1016/j.ecss.2006.11.006
Green, M. O., and Coco, G. (2014). Review of wave-driven sediment resuspension and transport in estuaries. Rev. Geophys. 52, 77–117. doi: 10.1002/2013RG000437
Grueters, U., Ibrahim, M. R., Satyanarayana, B., and Dahdouh-Guebas, F. (2019). Individual-based modeling of mangrove forest growth: MesoFON–Recent calibration and future direction. Estuarine Coastal Shelf Sci. 227:106302. doi: 10.1016/j.ecss.2019.106302
Grueters, U., Seltmann, T., Schmidt, H., Horn, H., Pranchai, A., Vovides, A. G., et al. (2014). The mangrove forest dynamics model mesoFON. Ecol. Model. 291, 28–41. doi: 10.1016/j.ecolmodel.2014.07.014
Haasnoot, M., Kwakkel, J. H., Walker, W. E., and ter Maat, J. (2013). Dynamic adaptive policy pathways: a method for crafting robust decisions for a deeply uncertain world. Glob. Environ. Change 23, 485–498. doi: 10.1016/j.gloenvcha.2012.12.006
Harris, R. J., Milbrandt, E. C., Everham, E. M., and Bovard, B. D. (2010). The effects of reduced tidal flushing on mangrove structure and function across a disturbance gradient. Estuaries Coasts 33, 1176–1185. doi: 10.1007/s12237-010-9293-2
Hochard, J. P., Hamilton, S., and Barbier, E. B. (2019). Mangroves shelter coastal economic activity from cyclones. Proc. Natl. Acad. Sci. U.S.A. 116, 12232–12237. doi: 10.1073/pnas.1820067116
Hoitink, A. J., and Hoekstra, P. (2005). Observations of suspended sediment from ADCP and OBS measurements in a mud-dominated environment. Coastal Eng. 52, 103–118. doi: 10.1016/j.coastaleng.2004.09.005
Hollmann, R., Merchant, C. J., Saunders, R., Downy, C., Buchwitz, M., Cazenave, A., et al. (2013). The ESA climate change initiative: satellite data records for essential climate variables. Bull. Am. Meteorol. Soc. 94, 1541–1552. doi: 10.1175/BAMS-D-11-00254.1
Hollnagel, E., Woods, D. D., and Leveson, N. (2006). Resilience Engineering: Concepts and Precepts. Farnham: Ashgate Publishing, Ltd.
Horstman, E. M., Bryan, K. R., and Mullarney, J. C. (2021). Drag variations, tidal asymmetry and tidal range changes in a mangrove creek system. Earth Surf. Process. Landf. 1–19. doi: 10.1002/esp.5124
Horstman, E. M., Dohmen-Janssen, C. M., Bouma, T. J., and Hulscher, S. J. M. H. (2015). Tidal-scale flow routing and sedimentation in mangrove forests: combining field data and numerical modelling. Geomorphology 228, 244–262. doi: 10.1016/j.geomorph.2014.08.011
Horstman, E. M., Dohmen-Janssen, C. M., Narra, P. M., van den Berg, N. J., Siemerink, M., and Hulscher, S. J. (2014). Wave attenuation in mangroves: a quantitative approach to field observations. Coastal Eng. 94, 47–62. doi: 10.1016/j.coastaleng.2014.08.005
Horstman, E. M., Lundquist, C. J., Bryan, K. R., Bulmer, R. H., Mullarney, J. C., and Stokes, D. J. (2018). “The Dynamics of Expanding Mangroves in New Zealand,” in Threats to Mangrove Forests. Coastal Research Library, Vol. 25, eds C. Makowski and C. Finkl (Cham: Springer). doi: 10.1007/978-3-319-73016-5_2
Howard, R. J., From, A. S., Krauss, K. W., Andres, K. D., Cormier, N., and Allain, L.. (2020). Soil surface elevation dynamics in a mangrove-to-marsh ecotone characterized by vegetation shifts. Hydrobiologia 847, 1087–1106. doi: 10.1007/s10750-019-04170-4
Hu, Z., Zhou, J., Wang, C., Wang, H., He, Z., Peng, Y., et al. (2020). A novel instrument for bed dynamics observation supports machine learning applications in mangrove biogeomorphic processes. Water Resou. Res. 56:e2020WR027257. doi: 10.1029/2020WR027257
Huxham, M., Kumara, M. P., Jayatissa, L. P., Krauss, K. W., Kairo, J., Langat, J., et al. (2010). Intra- and interspecific facilitation in mangroves may increase resilience to climate change threats. Phil. Trans. R. Soc. B Biol. Sci. 365, 2127–2135. doi: 10.1098/rstb.2010.0094
Imbert, D. (2018). Hurricane disturbance and forest dynamics in east Caribbean mangroves. Ecosphere 9:e02231. doi: 10.1002/ecs2.2231
IPCC (2019). “Summary for Policymakers, in IPCC Special Report on the Ocean and Cryoshpere in a Changing Climate, eds [H.-O. Pörtner, D.C. Roberts, V. Masson-Delmotte, P. Zhai, M.Tignor, E. Poloczanska, K. Mintenbech, M. Nicolai, A. Okem, J. Petzold, B. Rama, N. Weyer].
IUCN and WRI (2014). “A guide to the Restoration Opportunities Assessment Methodology (ROAM), in Assessing Forest Landscape Restoration Opportunities at the National or Sub-National Level. (Road-test edition) (Gland), 125.
Izaguirre, C., Méndez, F. J., Menéndez, M., and Losada, I. J. (2011). Global extreme wave height variability based on satellite data. Geophys. Res. Lett. 38, 1–6. doi: 10.1029/2011GL047302
Jones, A. R., Raja Segaran, R., Clarke, K. D., Waycott, M., Goh, W. S. H., and Gillanders, B. M. (2020). Estimating mangrove tree biomass and carbon content: a comparison of forest inventory techniques and drone imagery. Front. Marine Sci. 6:784. doi: 10.3389/fmars.2019.00784
Jones, C. G., Lawron, J. H., and Shachak, M. (1997). Positive and negative effects of organisms as physical ecosystem Engineers 78, 1946–1957. doi: 10.1890/0012-9658(1997)078[1946:PANEOO]2.0.CO;2
Jones, C. G., Lawton, J. H., Shachak, M., and Organisms, M. (1994). Organisms as ecosystem engineers. Oikos 69, 373–386. doi: 10.2307/3545850
Kauffman, J. B., and Cole, T. G. (2010). Micronesian mangrove forest structure and tree responses to a severe typhoon. Wetlands 30, 1077–1084. doi: 10.1007/s13157-010-0114-y
Kirezci, E., Young, I. R., Ranasinghe, R., Muis, S., Nicholls, R. J., Lincke, D., et al. (2020). Projections of global-scale extreme sea levels and resulting episodic coastal flooding over the 21st century. Sci. Rep. 10:11629. doi: 10.1038/s41598-020-67736-6
Koch, E. W., Barbier, E. B., Silliman, B. R., Reed, D. J., Perillo, G. M. E., Hacker, S. D., et al. (2009). Non-linearity in ecosystem services: temporal and spatial variability in coastal protection. Front. Ecol. Environ. 7, 29–37. doi: 10.1890/080126
Krauss, K. W., Allen, J. A., and Cahoon, D. R. (2003). Differential rates of vertical accretion and elevation change among aerial root types in Micronesian mangrove forests. Estuarine Coastal Shelf Sci. 56, 251–259. doi: 10.1016/S0272-7714(02)00184-1
Krauss K. W. Doyle T. W. Doyle T. J. Swarzenski C. M. From A. S. Day R. H. . (2009). Water level observations in mangrove swamps during two hurricanes in Florida. Wetlands 29, 142–149. doi: 10.1672/07-232.1
Krauss, K. W., Keeland, B. D., Allen, J. A., Ewel, K. C., and Johnson, D. J. (2007). Effects of season, rainfall, and hydrogeomorphic setting on mangrove tree growth in micronesia. Biotropica 39, 161–170. doi: 10.1111/j.1744-7429.2006.00259.x
Krauss, K. W., Mckee, K. L., Lovelock, C. E., Cahoon, D. R., Saintilan, N., Reef, R., et al. (2014). How mangrove forests adjust to rising sea level. New Phytol. 202, 19–34. doi: 10.1111/nph.12605
Krauss, K. W., and Osland, M. J. (2020). Tropical cyclones and the organization of mangrove forests: a review. Ann. Bot. 125, 213–234. doi: 10.1093/aob/mcz161
Kuenzer, C., Bluemel, A., Gebhardt, S., Quoc, T. V., and Dech, S. (2011). Remote sensing of mangrove ecosystems: a review. Remote Sens. 3, 878–928. doi: 10.3390/rs3050878
Kulp, S. A., and Strauss, B. H. (2019). New elevation data triple estimates of global vulnerability to sea-level rise and coastal flooding. Nat. Commun. 10:4844. doi: 10.1038/s41467-019-12808-z
Lang'at, J. K. S., Kairo, J. G., Mencuccini, M., Bouillon, S., Skov, M. W., Waldron, S., et al. (2014). Rapid losses of surface elevation following tree girdling and cutting in tropical mangroves. PLoS ONE 9:e107868. doi: 10.1371/journal.pone.0107868
Leong, R. C., Friess, D. A., Crase, B., Lee, W. K., and Webb, E. L. (2018). High-resolution pattern of mangrove species distribution is controlled by surface elevation. Estuarine Coastal Shelf Sci. 202, 185–192. doi: 10.1016/j.ecss.2017.12.015
Lewis, R. R., and Brown, B. (2014). Ecological Mangrove Rehabilitation. A field Manual For Practitioners. Seattle, WA: Mangrove Action Project.
Lewis, R. R., Milbrandt, E. C., Brown, B., Krauss, K. W., Rovai, A. S., Beever, J. W., et al. (2016). Stress in mangrove forests: early detection and preemptive rehabilitation are essential for future successful worldwide mangrove forest management. Marine Pollut. Bull. 109, 764–771. doi: 10.1016/j.marpolbul.2016.03.006
Li, S., Meng, X., Ge, Z., and Zhang, L. (2015). Evaluation of the threat from sea-level rise to the mangrove ecosystems in Tieshangang Bay, southern China. Ocean Coastal Manag. 109, 1–8. doi: 10.1016/j.ocecoaman.2015.02.006
Liénard, J., Lynn, K., Strigul, N., Norris, B. K., Gatziolis, D., Mullarney, J. C., et al. (2016). Efficient three-dimensional reconstruction of aquatic vegetation geometry: Estimating morphological parameters influencing hydrodynamic drag. Estuarine Coastal Shelf Sci. 178, 77–85. doi: 10.1016/j.ecss.2016.05.011
Long, J., Giri, C., Primavera, J., and Trivedi, M. (2016). Damage and recovery assessment of the Philippines' mangroves following Super Typhoon Haiyan. Marine Pollut. Bull. 109, 734–743. doi: 10.1016/j.marpolbul.2016.06.080
Lovelock, C. E., Adame, M. F., Bennion, V., Hayes, M., Reef, R., Santini, N., et al. (2015a). Sea level and turbidity controls on mangrove soil surface elevation change. Estuarine Coastal Shelf Sci. 153, 1–9. doi: 10.1016/j.ecss.2014.11.026
Lovelock, C. E., Bennion, V., Grinham, A., and Cahoon, D. R. (2011a). The role of surface and subsurface processes in keeping pace with sea level rise in intertidal wetlands of Moreton Bay, Queensland, Australia. Ecosystems 14, 745–757. doi: 10.1007/s10021-011-9443-9
Lovelock, C. E., Cahoon, D. R., Friess, D. A., Guntenspergen, G. R., Krauss, K. W., Reef, R., et al. (2015b). The vulnerability of Indo-Pacific mangrove forests to sea-level rise. Nature 526:559–563. doi: 10.1038/nature15538
Lovelock, C. E., Feller, I. C., Adame, M. F., Reef, R., Penrose, H. M., Wei, L., et al. (2011b). Intense storms and the delivery of materials that relieve nutrient limitations in mangroves of an arid zone estuary. Funct. Plant Biol. 38, 514–522. doi: 10.1071/FP11027
Lugo, A. E., and Snedaker, S. C. (1974). The ecology of mangroves. Ann. Rev. Ecol. Syst. 5, 39–64. doi: 10.1146/annurev.es.05.110174.000351
Lymburner, L., Bunting, P., Lucas, R., Scarth, P., Alam, I., Phillips, C., et al. (2020). Mapping the multi-decadal mangrove dynamics of the australian coastline. Remote Sens. Environ. 238:111185. doi: 10.1016/j.rse.2019.05.004
Macamo, C. C. F., Massuanganhe, E., Nicolau, D. K., Bandeira, S. O., and Adams, J. B. (2016). Mangrove's response to cyclone Eline, What is happening 14 years later. Aquat. Bot. 134, 10–17. doi: 10.1016/j.aquabot.2016.05.004
Mariotti, G., and Fagherazzi, S. (2013). Wind waves on a mudflat: the influence of fetch and depth on bed shear stresses. Continental Shelf Res. 60, S99–S110. doi: 10.1016/j.csr.2012.03.001
Marois, D. E., and Mitsch, W. J. (2015). Coastal protection from tsunamis and cyclones provided by mangrove wetlands – a review. Int. J. Biodivers. Sci. Ecosyst. Serv. Manag. 11, 71–83. doi: 10.1080/21513732.2014.997292
Mazda, Y., Magi, M., Ikeda, Y., Kurokawa, T., and Asano, T. (2006). Wave reduction in a mangrove forest dominated by Sonneratia sp. Wetlands Ecol. Manag. 14, 365–378. doi: 10.1007/s11273-005-5388-0
Mazda, Y., Magi, M., Kogo, M., and Hong, P. N. (1997a). Mangroves as a coastal protection from waves in the Tong King Delta, Vietnam. 1, 127–135. doi: 10.1023/A:1009928003700
Mazda, Y., Wolanski, E., King, B., Sase, A., Ohtsuka, D., and Magi, M. (1997b). Drag force due to vegetation in mangrove swamps. Mangroves Salt Marshes 1, 193–199. doi: 10.1023/A:1009949411068
McKee, K. L. (2011). Biophysical controls on accretion and elevation change in Caribbean mangrove ecosystems. Estuarine Coastal Shelf Sci. 91, 475–483. doi: 10.1016/j.ecss.2010.05.001
McKee, K. L., and Vervaeke, W. C. (2009). “Impacts of human disturbance on soil erosion potential and habitat stability of mangrove-dominated islands in the pelican cays and twin cays ranges, Belize,” in Smithsonian Contributions to the Marine Sciences 415–427.
Mendez, F. J., and Losada, I. J. (2004). An empirical model to estimate the propagation of random breaking and nonbreaking waves over vegetation fields. Coastal Eng. 51, 103–118. doi: 10.1016/j.coastaleng.2003.11.003
Menéndez, P., Losada, I. J., Torres-Ortega, S., Narayan, S., and Beck, M. W. (2020). The global flood protection benefits of mangroves. Sci. Rep. 10, 1–11. doi: 10.1038/s41598-020-61136-6
Menéndez, P., Losada, I. J., Torres-Ortega, S., Toimil, A., and Beck, M. W. (2019). Assessing the effects of using high-quality data and high-resolution models in valuing flood protection services of mangroves. PLoS ONE 14:e0220941. doi: 10.1371/journal.pone.0220941
Milbrandt, E. C., Greenawalt-Boswell, J. M., Sokoloff, P. D., and Bortone, S. A. (2006). Impact and response of southwest Florida mangroves to the 2004 hurricane season. Estuaries Coasts 29, 979–984. doi: 10.1007/BF02798659
Mogensen, L. A., and Rogers, K. (2018). Validation and comparison of a model of the effect of sea-level rise on coastal wetlands. Sci. Rep. 8, 1–14. doi: 10.1038/s41598-018-19695-2
Montgomery, J. M., Bryan, K. R., Horstman, E. M., and Mullarney, J. C. (2018). Attenuation of tides and surges by mangroves: contrasting case studies from New Zealand. Water 10:1119. doi: 10.3390/w10091119
Montgomery, J. M., Bryan, K. R., Mullarney, J. C., and Horstman, E. M. (2019). Attenuation of storm surges by coastal mangroves. Geophys. Res. Lett. 46, 2680–2689. doi: 10.1029/2018GL081636
Morison, J., Johnson, J., and Schaaf, S. (1950). The force exerted by surface waves on piles. J. Petroleum Technol. 2, 149–154. doi: 10.2118/950149-G
Morris, R. L., Boxshall, A., and Swearer, S. E. (2020). Climate-resilient coasts. Nat. Clim. Change 10, 482–485. doi: 10.1038/s41558-020-0742-z
Narayan, S., Beck, M. W., Reguero, B. G., Losada, I. J., Van Wesenbeeck, B. K., Pontee, N., et al. (2016). The effectiveness, costs and coastal protection benefits of natural and nature-based defences. PLoS ONE 11:e0154735. doi: 10.1371/journal.pone.0154735
Neumann, B., Vafeidis, A. T., Zimmermann, J., and Nicholls, R. J. (2015). Future coastal population growth and exposure to sea-level rise and coastal flooding-A global assessment. PLoS ONE 10:e0118571. doi: 10.1371/journal.pone.0118571
Nguyen, H. M., Bryan, K. R., and Pilditch, C. A. (2020). The effect of long-term aerial exposure on intertidal mudflat erodibility. Earth Surface Processes Landforms 45, 3623–3638. doi: 10.1002/esp.4990
Nguyen, H. M., Bryan, K. R., Pilditch, C. A., and Moon, V. G. (2019). Correction to: Influence of ambient temperature on erosion properties of exposed cohesive sediment from an intertidal mudflat. Geo Marine Lett. 39, 521. doi: 10.1007/s00367-019-00618-7
NOAA (2015). A Guide to Assessing Green Infrastructure Costs and Benefits for Flood Reduction. Technical report.
Norris, B. K., Mullarney, J. C., Bryan, K. R., and Henderson, S. M. (2017). The effect of pneumatophore density on turbulence: a field study in a Sonneratia-dominated mangrove forest, Vietnam. Continental Shelf Res. 147, 114–127. doi: 10.1016/j.csr.2017.06.002
Norris, B. K., Mullarney, J. C., Bryan, K. R., and Henderson, S. M. (2021). Relating millimeter-scale turbulence to meter-scale subtidal erosion and accretion across the fringe of a coastal mangrove forest. Earth Surface Processes Landforms 46, 573–592 doi: 10.1002/esp.5047
Opperman, J. J. (2014). A flood of benefits: Using Green infrastructure to reduce flood risks. Technical Report, Arlington, Virginia.
Osland, M. J., Feher, L. C., Anderson, G. H., Vervaeke, W. C., Krauss, K. W., Whelan, K. R., et al. (2020). A Tropical Cyclone-Induced Ecological Regime Shift: Mangrove Forest Conversion to Mudflat in Everglades National Park (Florida, USA). Wetlands. 40, 1445–1458. doi: 10.1007/s13157-020-01291-8
Paling, E. I., Kobryn, H. T., and Humphreys, G. (2008). Assessing the extent of mangrove change caused by Cyclone Vance in the eastern Exmouth Gulf, northwestern Australia. Estuarine Coastal Shelf Sci. 77, 603–613. doi: 10.1016/j.ecss.2007.10.019
Peters, R., Olagoke, A., and Berger, U. (2018). A new mechanistic theory of self-thinning: Adaptive behaviour of plants explains the shape and slope of self-thinning trajectories. Ecol. Model. 390, 1–9. doi: 10.1016/j.ecolmodel.2018.10.005
Peters, R., Vovides, A. G., Luna, S., Grüters, U., and Berger, U. (2014). Changes in allometric relations of mangrove trees due to resource availability-A new mechanistic modelling approach. Ecol. Model. 283, 53–61. doi: 10.1016/j.ecolmodel.2014.04.001
Peters, R., Walther, M., Lovelock, C., Jiang, J., and Berger, U. (2020). The interplay between vegetation and water in mangroves: new perspectives for mangrove stand modelling and ecological research. Wetlands Ecol. Manag. 28, 697–712. doi: 10.1007/s11273-020-09733-0
Pham, T. D., Yokoya, N., Bui, D. T., Yoshino, K., and Friess, D. A. (2019). Remote sensing approaches for monitoring mangrove species, structure, and biomass: opportunities and challenges. Remote Sens. 11, 1–24. doi: 10.3390/rs11030230
Phuoc, V. L. H., and Massel, S. R. (2006). Experiments on wave motion and suspended sediment concentration at Nang Hai, Can Gio mangrove forest, Southern Vietnam. Oceanologia 48, 23–40.
PIANC (2011). Working With Nature. PIANC Position Paper. Available online at: https://www.pianc.org/uploads/files/EnviCom/WwN/WwN-Position-Paper-English.pdf
Primavera, J. H., dela Cruz, M., Montilijao, C., Consunji, H., dela Paz, M., Rollon, R. N., et al. (2016). Preliminary assessment of post-Haiyan mangrove damage and short-term recovery in Eastern Samar, central Philippines. Marine Pollut. Bull. 109, 744–750. doi: 10.1016/j.marpolbul.2016.05.050
Proffitt, C. E., Milbrandt, E. C., and Travis, S. E. (2006). Red mangrove (Rhizophora mangle) reproduction and seedling colonization after Hurricane Charley: Comparisons of Charlotte Harbor and Tampa Bay. Estuaries Coasts 29, 972–978. doi: 10.1007/BF02798658
PTFCF (2018). Community-Based Mangrove Rehabilitation Training Manual. Makati City: Philippine Tropical Forest Conservation Foundation, Inc.
Quartel, S., Kroon, A., Augustinus, P. G. E. F., Van Santen, P., and Tri, N. H. (2007). Wave attenuation in coastal mangroves in the Red River Delta, Vietnam. J. Asian Earth Sci. 29, 576–584. doi: 10.1016/j.jseaes.2006.05.008
Radabaugh, K. R., Moyer, R. P., Chappel, A. R., Dontis, E. E., Russo, C. E., Joyse, K. M., et al. (2019). Mangrove damage, delayed mortality, and early recovery following hurricane irma at two landfall sites in Southwest Florida, USA. Estuaries Coasts. 43, 1104–1118. doi: 10.1007/s12237-019-00564-8
Roelvink, D., Reniers, A., van Dongeren, A., van Thiel de Vries, J., McCall, R., and Lescinski, J. (2009). Modelling storm impacts on beaches, dunes and barrier islands. Coastal Eng. 56, 1133–1152. doi: 10.1016/j.coastaleng.2009.08.006
Rogers, K., and Saintilan, N. (2008). Relationships between Surface Elevation and Groundwater in Mangrove Forests of Southeast Australia. J. Coastal Res. 24:63–69. doi: 10.2112/05-0519.1
Rogers, K., Saintilan, N., and Cahoon, D. R. (2005). Surface elevation dynamics in a regenerating mangrove forest at Homebush Bay, Australia. Wetlands Ecol. Manag. 13, 587–598. doi: 10.1007/s11273-004-0003-3
Rogers, K., Saintilan, N., and Woodroffe, C. D. (2014). Surface elevation change and vegetation distribution dynamics in a subtropical coastal wetland: Implications for coastal wetland response to climate change. Estuarine Coastal Shelf Sci. 149, 46–56. doi: 10.1016/j.ecss.2014.07.009
Ruf, C. S., Atlas, R., Chang, P. S., Clarizia, M. P., Garrison, J. L., Gleason, S., et al. (2016). New ocean winds satellite mission to probe hurricanes and tropical convection. Bull. Am. Meteorol. Soc. 97, 385–395. doi: 10.1175/BAMS-D-14-00218.1
Sadat-Noori, M., Rankin, C., Rayner, D., Heimhuber, V., Gaston, T., Drummond, C., et al. (2021). Coastal wetlands can be saved from sea level rise by recreating past tidal regimes. Sci. Rep. 11, 1–10. doi: 10.1038/s41598-021-80977-3
Saintilan, N., Khan, N. S., Ashe, E., Kelleway, J. J., Rogers, K., Woodroffe, C. D., et al. (2020). Thresholds of mangrove survival under rapid sea level rise. Science 368:1118–1121. doi: 10.1126/science.aba2656
Salmo, S. G., Lovelock, C. E., and Duke, N. C. (2013). Assessment of vegetation and soil conditions in restored mangroves interrupted by severe tropical typhoon 'Chan-hom' in the Philippines. Hydrobiologia 733:85–102. doi: 10.1007/s10750-013-1766-4
Schoonees, T., Gijón Mancheño, A., Scheres, B., Bouma, T. J., Silva, R., Schlurmann, T., et al. (2019). Hard structures for coastal protection, towards greener designs. Estuaries and Coasts. 42, 1709–1729. doi: 10.1007/s12237-019-00551-z
Schuerch, M., Spencer, T., Temmerman, S., Kirwan, M. L., Wolff, C., Lincke, D., et al. (2018). Future response of global coastal wetlands to sea-level rise. Nature 561, 231–234. doi: 10.1038/s41586-018-0476-5
Sherman, R. E., Fahey, T. J., Resources, N., Hall, F., and York, N. (2001). Hurricane Impacts on a mangrove forest in the dominican republic : damage patterns and early recovery Assoc. Trop. Biol. Conserv. 33, 393–408. doi: 10.1111/j.1744-7429.2001.tb00194.x
Shugart, H. (1984). A Theory of Forest Dynamics: The Ecological Implications of Forest Succession Models. New York, NY: Springer-Verlag.
Sidik, F., Neil, D., and Lovelock, C. E. (2016). Effect of high sedimentation rates on surface sediment dynamics and mangrove growth in the Porong River, Indonesia. Marine Pollut. Bull. 107, 355–363. doi: 10.1016/j.marpolbul.2016.02.048
Simard, M., Fatoyinbo, L., Smetanka, C., Rivera-Monroy, V. H., Casta neda-Moya, E., Thomas, N., et al. (2019). Mangrove canopy height globally related to precipitation, temperature and cyclone frequency. Nat. Geosci. 12, 40–45. doi: 10.1038/s41561-018-0279-1
Sippo, J. Z., Lovelock, C. E., Santos, I. R., Sanders, C. J., and Maher, D. T. (2018). Mangrove mortality in a changing climate: an overview. Estuarine Coastal Shelf Sci. 215, 241–249. doi: 10.1016/j.ecss.2018.10.011
Smith, T. J., Anderson, G. H., Balentine, K., Tiling, G., Ward, G. A., and Whelan, K. R. (2009). Cumulative impacts of hurricanes on Florida mangrove ecosystems: sediment deposition, storm surges and vegetation. Wetlands 29, 24–34. doi: 10.1672/08-40.1
Smith, T. J., Robblee, M. B., Wanless, H. R., and Doyle, T. W. (1994). Mangroves, hurricanes, and lightning strikes. BioScience 44, 256–262. doi: 10.2307/1312230
Smoak, J. M., Breithaupt, J. L., Smith, T. J., and Sanders, C. J. (2013). Sediment accretion and organic carbon burial relative to sea-level rise and storm events in two mangrove forests in Everglades National Park. Catena 104, 58–66. doi: 10.1016/j.catena.2012.10.009
Spalding, M., McIvor, A., Tonneijck, F. H., Tol, S., and van Eijk, P. (2014). Mangroves for Coastal Defence. Guidelines for Coastal Managers & Policy Makers. Published by Wetlands International and The Nature Conservancy, 42.
Spalding, M., and Parrett, C. L. (2019). Global patterns in mangrove recreation and tourism. Marine Policy 110:103540. doi: 10.1016/j.marpol.2019.103540
Steinke, T. D., and Ward, C. J. (1989). Some effects of the cyclones Domoina and Imboa on mangrove communities in the St Lucia Estuary. South Afr. J. Bot. 55, 340–348. doi: 10.1016/S0254-6299(16)31186-3
Stokes, D. J., and Harris, R. J. (2015). Sediment properties and surface erodibility following a large-scale mangrove (Avicennia marina) removal. Continental Shelf Res. 107:1–10. doi: 10.1016/j.csr.2015.07.011
Sutton-Grier, A. E., Wowk, K., and Bamford, H. (2015). Future of our coasts: the potential for natural and hybrid infrastructure to enhance the resilience of our coastal communities, economies and ecosystems. Environ. Sci. Policy 51, 137–148. doi: 10.1016/j.envsci.2015.04.006
Suzuki, T., Zijlema, M., Burger, B., Meijer, M. C., and Narayan, S. (2012). Wave dissipation by vegetation with layer schematization in SWAN. Coastal Eng. 59, 64–71. doi: 10.1016/j.coastaleng.2011.07.006
Swales, A., Bentley, S. J., Lovelock, C., and Bell, R. G. (2007). “Sediment processes and mangrove-habitat expansion on a rapidly-prograding muddy coast, New Zealand,” in Coastal Sediments '07, 1441–1454. doi: 10.1061/40926(239)111
Swales, A., Bentley, S. J., and Lovelock, C. E. (2015). Mangrove-forest evolution in a sediment-rich estuarine system: opportunists or agents of geomorphic change? Earth Surface Processes Landforms 40, 1672–1687. doi: 10.1002/esp.3759
Swales, A., Denys, P., Pickett, V. I., and Lovelock, C. E. (2016). Evaluating deep subsidence in a rapidly-accreting mangrove forest using GPS monitoring of surface-elevation benchmarks and sedimentary records. Marine Geol. 380, 205–218. doi: 10.1016/j.margeo.2016.04.015
Swales, A., and Lovelock, C. E. (2020). Comparison of sediment-plate methods to measure accretion rates in an estuarine mangrove forest (New Zealand). Estuarine Coastal Shelf Sci. 236:106642. doi: 10.1016/j.ecss.2020.106642
Swales, A., Reeve, G., Cahoon, D. R., and Lovelock, C. E. (2019). Landscape evolution of a fluvial sediment-rich avicennia marina mangrove forest: insights from seasonal and inter-annual surface-elevation dynamics. Ecosystems 22, 1232–1255. doi: 10.1007/s10021-018-0330-5
Taherkhani, M., Vitousek, S., Barnard, P. L., Frazer, N., Anderson, T. R., and Fletcher, C. H. (2020). Sea-level rise exponentially increases coastal flood frequency. Sci. Rep. 10, 1–17. doi: 10.1038/s41598-020-62188-4
Temmerman, S., Meire, P., Bouma, T. J., Herman, P. M. J., Ysebaert, T., and De Vriend, H. J. (2013). Ecosystem-based coastal defence in the face of global change. Nature 504, 79–83. doi: 10.1038/nature12859
Toffoli, A., and Bitner-Gregersen, E. M. (2017). “Types of ocean surface waves, wave classification,” in Encyclopedia of Maritime and Offshore Engineering, eds J. Carlton, P. Jukes and Y. S. Choo. doi: 10.1002/9781118476406.emoe077
UNEP (2014). Green Infrastructure Guide for Water Management: Ecosystem-Based Management Approaches for Water-Related Projects. Technical Report.
USACE (2015). Engineering With Nature. Working with Nature. ERDC Brochure. Available online at: https://ewn.el.erdc.dren.mil/pub/EWNFactSheet_Final.pdf
van Bijsterveldt, C. E., van Wesenbeeck, B. K., van der Wal, D., Afiati, N., Pribadi, R., Brown, B., et al. (2020). How to restore mangroves for greenbelt creation along eroding coasts with abandoned aquaculture ponds. Estuarine Coastal Shelf Sci. 235:106576. doi: 10.1016/j.ecss.2019.106576
Van Coppenolle, R., and Temmerman, S. (2020). Identifying global hotspots where coastal wetland conservation can contribute to nature-based mitigation of coastal flood risks. Glob. Planetary Change 187:103125. doi: 10.1016/j.gloplacha.2020.103125
van Eekelen, E., and Bouw, M. (2020). Building With Nature: Creating, Implementing and Upscaling Nature-Based Solutions. Rotterdam: nai010 Publishers.
Van Loon, A. F., Te Brake, B., Van Huijgevoort, M. H., and Dijksma, R. (2016). Hydrological classification, a practical tool for mangrove restoration. PLoS ONE 11:e0150302. doi: 10.1371/journal.pone.0150302
Van Maanen, B., Coco, G., and Bryan, K. R. (2015). On the ecogeomorphological feedbacks that control tidal channel network evolution in a sandy mangrove setting. Proc. R. Soc. A Math. Phys. Eng. Sci. 471;20150115. doi: 10.1098/rspa.2015.0115
Van Santen, P., Augustinus, P. G. E. F., Janssen-Stelder, B. M., Quartel, S., and Tri, N. H. (2007). Sedimentation in an estuarine mangrove system. J. Asian Earth Sci. 29:566–575. doi: 10.1016/j.jseaes.2006.05.011
van Wesenbeeck, B. K., de Boer, W., Narayan, S., van der Star, W. R. L., and de Vries, M. B. (2016). Coastal and riverine ecosystems as adaptive flood defenses under a changing climate. Mitigat. Adaptat. Strateg. Glob. Change 22, 1087–1094. doi: 10.1007/s11027-016-9714-z
Venditti, J. G., Church, M., Attard, M. E., and Haught, D. (2016). Use of ADCPs for suspended sediment transport monitoring: An empirical approach. Water Resou. Res. 52, 2715–2736. doi: 10.1002/2015WR017348
Vilas, M. P., Adams, M. P., Ball, M. C., Meynecke, J. O., Santini, N. S., Swales, A., et al. (2019). Night and day: Shrinking and swelling of stems of diverse mangrove species growing along environmental gradients. PLoS ONE 14:e0221950. doi: 10.1371/journal.pone.0221950
Vincenot, C. E., Carteni, F., Mazzoleni, S., Rietkerk, M., and Giannino, F. (2016). Spatial self-organization of vegetation subject to climatic stress–insights from a system dynamics–individual-based hybrid model. Front. Plant Sci. 7:636. doi: 10.3389/fpls.2016.00636
Vogt, J., Skóra, A., Feller, I. C., Piou, C., Coldren, G., and Berger, U. (2012). Investigating the role of impoundment and forest structure on the resistance and resilience of mangrove forests to hurricanes. Aquat. Bot. 97, 24–29. doi: 10.1016/j.aquabot.2011.10.006
Walcker, R., Laplanche, C., Herteman, M., Lambs, L., and Fromard, F. (2019). Damages caused by hurricane Irma in the human-degraded mangroves of Saint Martin (Caribbean). Sci. Rep. 9:18971. doi: 10.1038/s41598-019-55393-3
Wang, L., Jia, M., Yin, D., and Tian, J. (2019). A review of remote sensing for mangrove forests: 1956–2018. Remote Sens. Environ.. 231:111223. doi: 10.1016/j.rse.2019.111223
Watson, C. S., White, N. J., Church, J. A., King, M. A., Burgette, R. J., and Legresy, B. (2015). Unabated global mean sea-level rise over the satellite altimeter era. Nat. Clim. Change 5, 565–568. doi: 10.1038/nclimate2635
Webb, E. L., Friess, D. A., Krauss, K. W., Cahoon, D. R., Guntenspergen, G. R., and Phelps, J. (2013). A global standard for monitoring coastal wetland vulnerability to accelerated sea-level rise. Nat. Clim. Change 3, 458–465. doi: 10.1038/nclimate1756
Whelan, K. R. T. (2005). The Successional Dynamics of Lightning Initiated Canopy Gaps in the Mangrove Forests of Shark River, Everglades National Park, USA. Ph.D. thesis, Florida International University.
Willemsen, P. W., Horstman, E. M., Borsje, B. W., Friess, D. A., and Dohmen-Janssen, C. M. (2016). Sensitivity of the sediment trapping capacity of an estuarine mangrove forest. Geomorphology 273, 189–201. doi: 10.1016/j.geomorph.2016.07.038
Winterwerp, J. C., Albers, T., Anthony, E. J., Friess, D. A., Mancheño, A. G., Moseley, K., et al. (2020). Managing erosion of mangrove-mud coasts with permeable dams – lessons learned. Ecol. Eng. 158:106078. doi: 10.1016/j.ecoleng.2020.106078
Winterwerp, J. C., Borst, W. G., and de Vries, M. B. (2005). Pilot study on the erosion and rehabilitation of a mangrove mud coast. J. Coastal Res. 212, 223–230. doi: 10.2112/03-832A.1
Winterwerp, J. C., Erftemeijer, P. L., Suryadiputra, N., Van Eijk, P., and Zhang, L. (2013). Defining eco-morphodynamic requirements for rehabilitating eroding mangrove-mud coasts. Wetlands 33, 515–526. doi: 10.1007/s13157-013-0409-x
Woodroffe, C. (1992). Mangrove Sediments and Geomorphology. Trop. Mangrove Ecosyst. 41, 7–41. doi: 10.1029/CE041p0007
Woodroffe, C. D., Rogers, K., McKee, K. L., Lovelock, C. E., Mendelssohn, I. A., and Saintilan, N. (2016). Mangrove sedimentation and response to relative sea-level rise. Ann. Rev. Marine Sci. 8, 243–266. doi: 10.1146/annurev-marine-122414-034025
Woodruff, J. D., Irish, J. L., and Camargo, S. J. (2013). Coastal flooding by tropical cyclones and sea-level rise. Nature 504, 44–52. doi: 10.1038/nature12855
World Bank (2016). Managing Coasts with Natural Solutions: Guidelines for Measuring and Valuing the Coastal Protection Services of Mangroves and Coral Reefs. Technical Report, Wealth Accounting and the Valuation of Ecosystem Services Partnership (WAVES), Washington, DC.
World Bank (2017). Implementing Nature Based Flood Protection. Technical Report, World Bank, Washington, DC.
World Bank (2019). Forces of Nature: Assessment And Economic Valuation Of Coastal Protection Services Provided by Mangroves in Jamaica. Technical Report.
Worthington, T. A., zu Ermgassen, P. S., Friess, D. A., Krauss, K. W., Lovelock, C. E., Thorley, J., et al. (2020). A global biophysical typology of mangroves and its relevance for ecosystem structure and deforestation. Sci. Rep. 10, 1–11. doi: 10.1038/s41598-020-71194-5
Young, I., and Donelan, M. (2018). On the determination of global ocean wind and wave climate from satellite observations. Remote Sens. Environ. 215, 228–241. doi: 10.1016/j.rse.2018.06.006
Young, I. R., and Ribal, A. (2019). Multiplatform evaluation of global trends in wind speed and wave height. Science 364, 548–552. doi: 10.1126/science.aav9527
Yuvaraj, E., Dharanirajan, K., Jayakumar, S., and Saravanan (2014). Geomorphic settings of mangrove ecosystem in South Andaman Island: a geospatial approach. J. Earth Syst. Sci. 123, 1819–1830. doi: 10.1007/s12040-014-0503-3
Zhang, K., Liu, H., Li, Y., Xu, H., Shen, J., Rhome, J., et al. (2012). The role of mangroves in attenuating storm surges. Estuarine Coastal Shelf Sci. 102–103, 11–23. doi: 10.1016/j.ecss.2012.02.021
Zhang, K., Simard, M., Ross, M., Rivera-Monroy, V. H., Houle, P., Ruiz, P., et al. (2008). Airborne laser scanning quantification of disturbances from hurricanes and lightning strikes to mangrove forests in everglades national park, USA. Sensors 8, 2262–2292. doi: 10.3390/s8042262
Zhang, K., Thapa, B., Ross, M., and Gann, D. (2016). Remote sensing of seasonal changes and disturbances in mangrove forest: a case study from South Florida. Ecosphere 7:e01366. doi: 10.1002/ecs2.1366
List of Symbols
Keywords: nature-based solutions, functionality, persistence, monitoring, remote sensing, design guidelines, adaptive management, resilience engineering
Citation: Gijsman R, Horstman EM, van der Wal D, Friess DA, Swales A and Wijnberg KM (2021) Nature-Based Engineering: A Review on Reducing Coastal Flood Risk With Mangroves. Front. Mar. Sci. 8:702412. doi: 10.3389/fmars.2021.702412
Received: 29 April 2021; Accepted: 08 June 2021;
Published: 08 July 2021.
Edited by:
Stijn Temmerman, University of Antwerp, BelgiumReviewed by:
Lida Teneva, Independent Researcher, Sacramento, United StatesBarbara Zanuttigh, University of Bologna, Italy
Copyright © 2021 Gijsman, Horstman, van der Wal, Friess, Swales and Wijnberg. This is an open-access article distributed under the terms of the Creative Commons Attribution License (CC BY). The use, distribution or reproduction in other forums is permitted, provided the original author(s) and the copyright owner(s) are credited and that the original publication in this journal is cited, in accordance with accepted academic practice. No use, distribution or reproduction is permitted which does not comply with these terms.
*Correspondence: Rik Gijsman, ci5naWpzbWFuJiN4MDAwNDA7dXR3ZW50ZS5ubA==