- 1MARE-Marine and Environmental Sciences Centre, DBA-Departamento de Biologia Animal, Faculdade de Ciências, Universidade de Lisboa, Lisboa, Portugal
- 2IDL - Instituto Dom Luiz, Faculdade de Ciências, Universidade de Lisboa, Lisboa, Portugal
- 3INRAE, Centre Nouvelle-Aquitaine Bordeaux, UR EABX (Ecosystèmes Aquatiques et Changements Globaux), Bordeaux, France
Understanding food webs environmental condition is a challenging task since evaluations are limited by data on key ecosystem elements, by the availability of indicators that incorporate relevant guilds and by the difficulty in establishing cause-effect relations between pressures and health status, as multiple overlapping pressures can affect taxonomic elements differently. The present work aims to investigate food webs assessment under the Marine Strategy Framework Directive (MSFD), revealing gaps and future research needs in the North Eastern Atlantic. To understand reporting patterns, information on the criteria employed and the resulting assessment trends of Descriptor 4—Food webs were surveyed from the MSFD reports. A multivariate analysis was applied to food webs assessment status and spatially overlapping anthropogenic pressures to understand if the assessment was detecting pressures, considering fish elements. Results revealed that reporting strategies varied between Member States. High reporting effort was exhibited by the United Kingdom in opposition to Ireland or France. Reporting of other groups other than fish and plankton was limited to the United Kingdom due to the availability of monitoring programs and data. The analysis applied to criteria considering fish elements reinforced that reporting strategies and trends differed between countries, although some similarities were found for the Bay of Biscay and Iberian coast and the Celtic Seas. Food webs assessment trends for fish were variable in Spain and were stable or increased in Portugal and the United Kingdom. Anthropogenic drivers significantly influencing food web trends for fish elements were fishing, and climate anomalies in the southern Bay of Biscay and Iberian coast, while eutrophication and chemical contamination had effects on trends in the Celtic Sea and the North Sea. Results allowed to establish a relation between anthropogenic effects and food web patterns, however, these were limited since food webs assessment is incongruent in terms of criteria used and data is still limited at relevant scales. This study reinforced the necessity to increase Member States harmonization and calibration to improve our understanding of food webs environmental status.
Introduction
Healthy marine systems depend on monitoring plans and management measures that consider competing societal interests such as the sustainability and productivity of the systems, human well-being, and the development of human activities (European Commission, 2008, 2020; Korpinen et al., 2021). Several policies and legal instruments have been developed for that purpose and, in that scope, the Marine Strategy Framework Directive (MSFD), published in 2008 and revised in 2017, aims to implement an Ecosystem Based Approach (EBA) to the assessment and development of management measures in the European marine systems (European Commission, 2017a,b). The directive is structured in 11 ambitious descriptors that are in different stages of development. Among the descriptors that target biodiversity, Descriptor 4—Food webs (D4) aims to assess the status of the food chains, i.e., the network of predator-prey interactions between coexisting species and populations. This descriptor represents one of the most complex and unknown aspects of marine ecosystems, since the identification of simple indicators able to assess the status of the system with dynamic species interactions and the identification of underlying responses to pressures is challenging (Shephard et al., 2015; Otto et al., 2018). The assessment of the food-web descriptor includes criteria classified as primary—D4C1 Trophic guild species diversity and D4C2 Abundance across trophic, and secondary—D4C3 Trophic guild size distribution and D4C4 Trophic guild productivity (European Commission, 2017a). Nonetheless, the indicators and the methodology adopted may be different between Member States (MSs), which, are conditioned by existing data from national monitoring programs. Legislative updates have improved reporting consistency, considering the criteria selected and the spatial scale of the assessment (European Commission, 2017a,b). However, in the 2018 assessment, more than 60% of the coastal food webs were considered “not assessed,” while the shelf ecosystems were either “not assessed” or “unknown” for 90% of the cases (European Commission, 2020). The reasons behind low reporting and/or misreporting were the lack of appropriate metrics, the inexistence of appropriate datasets (that need to address an extensive number of ecosystem components), and the lack of knowledge on direct cause-effect relationships in Europe’s seas (European Commission, 2020).
There has been an attempt to develop fully operational indicators that can integrate trophic structure and functions, together with their interactions. But the lack of comparable data between taxonomic groups has made such integration difficult (Rombouts et al., 2013; Tam et al., 2017; Machado et al., 2019; Ministério do Mar, 2020). According to Tam et al. (2017), food web indexes should be sensitive to the magnitude and direction of response to underlying attribute/pressure, have a basis in theory, be specific, be responsive at an appropriate time scale, and be cost-effective to monitor or to update (Shin et al., 2010; Rombouts et al., 2013; Otto et al., 2018). The choice of a specific set of food web indicators can imply that some aspects of marine food webs are valued more than others. Therefore, a well-balanced selection process for indicators is required to encompass all currently known properties of marine food webs (Tam et al., 2017). As a result, indicators considering ecosystem components such as fish and Phyto/zooplankton elements have been further implemented due to long-term stock assessment programs, implemented by the European Commission (EC) through the Common Fisheries Policy (CFP) and the Continuous Plankton Recorder monitoring program in the Celtic Seas (CS) and Greater North Sea (GNS) (European Commission, 2020; Machado et al., 2021). Even though these indexes enable the evaluation of trophic guilds within ecosystem elements, they do not address the connectivity amidst ecosystem elements, hindering the assessment. As a result, a set of descriptors is commonly a recommended practice (Tam et al., 2017) and further emphasis on spatial and temporal resolutions should be further added (Machado et al., 2021). When trying to disentangle if ecosystem status is directly linked to pressures, further difficulties arise, since the environment is exposed to existing multiple pressures, and food web indicators lack the establishment of a clear and direct relation with anthropogenic pressures (pressure-status relationship) (Henriques et al., 2008; Shin et al., 2010; Crise et al., 2015; Probst and Stelzenmüller, 2015; Preciado et al., 2019). As a consequence, some authors have considered them as surveillance indicators, due to their limited interpretation of direct effects (ICES, 2015). On the other hand, results of food web surveillance can provide signals and indications on what multiple or combinations of pressure may be behind alterations. As a result, relevant indicators are those identifying emergent properties of food webs, which can address cumulative impacts, integrated dynamics, and responses to pressures, detect indirect and unintended consequences (Lynam et al., 2017; Tam et al., 2017). These are also often used in the context of evaluating trade-offs in management and mitigate impacts on food webs. In the last evaluation, indicators employed were considered short to show emergent proprieties that reflect the myriad of overlapping human pressures on food webs. Indices used incorporate a section of the system (fish elements) and mostly detect pressures driven by fisheries. In many instances, food webs assessments were incomplete, associated with high uncertainty, or are simply impossible due to a lack of suitable data. To overcome these obstacles, modeling approaches have been considered promising (Coll et al., 2016), but they also lack the appropriate data sets, what hinders their implementation. Solutions such as the use of long-term data series and cross-regional cooperation have been pointed out to further facilitate improved and consistent assessments (European Commission, 2020).
This study aim is to systematize D4—Food web assessment methods and environmental status, reported by MS under the MSFD framework, to identify existing inconsistencies and knowledge gaps. The environmental status and trends estimated by MSs were further used to disentangle if cause-effect relations, exerted by anthropogenic pressures, are being detected at the sea basin level. To achieve this purpose, the methodological criteria adopted to assess food webs in the second MSFD reporting cycle and their resulting assessment trends were surveyed, analyzed and statistically compared across MSs to characterize reporting strategy and congruency across the North Eastern Atlantic MSs. In a second phase, a multivariate analysis was applied to food web criteria status, considering fish ecosystem elements trends, to understand if these are significantly influenced by spatially overlapping anthropogenic pressures occurring in the sea basin. Information of human activities was used as a proxy of anthropogenic pressures to obtain information about potential exposure of food webs to anthropogenic pressure. It was hypothesized that fish food web indicators are detecting effects of anthropogenic pressure and therefore, they are contributing toward the assessment of anthropogenic effects on food webs functioning and structure.
Materials and Methods
A literature review was applied to MSFD reports submitted in the Central Data Repository (CDR) of the European Environment Information and Observation Network (EIONET) Portal and the Marine Information System For Europe (WISE Marine) database (EEA, 2020, 2021) to survey results on the assessment of food webs criteria obtained within the scope of Descriptor 4—Food webs. The search included all reports submitted until 2020, concerning the 2nd assessment cycle (2012–2018) in the Northeast Atlantic basin. The survey included the subregions Macaronesia (MAC), Bay of Biscay and Iberian coast (BBIC), Celtic Seas (CS) and the United Kingdom (UK) part of the GNS (Greater North Sea), as defined in Article 4(2) of Directive 2008/56/EC (European Commission, 2008; Figure 1). The list of ecosystem elements (targets) and human-driven pressures considered are defined in the MSFD, for Descriptor 4—Food webs, in the Commission Decision 2017/845 and 2017/848 (European Commission, 2017a,b).
Data Collection
The MSFD national reports included in the present work were from Ireland (IR), UK, France (FR), Spain (SP), and Portugal (PT), including the reports from the Autonomous Regions of the latter two countries. The existing information on food-web criteria assessed by each MS was retrieved from all reports. This survey collected the following data from each report: MSFD subregion, MS, food web criteria, ecosystem elements (target), human pressures, indicator, spatial scale, temporal scale, resulting trends: significance (i.e., significant, non-significant), direction (i.e., increase, decrease) and status of the assessment (i.e., GES, below GES). All these aspects were listed and counted, to understand MSs reporting patterns for this descriptor. If results were only available graphically, an image processing method was used to extract results from mean and standard error (e.g., Image J, software GraphClick, etc.). For comprehensive and in-depth analysis, spatial stratification groups were also devised for each MS (e.g., divisions and sub-divisions), whenever this information was available. The assessments were grouped based on the spatial unit of analysis (MSFD subregions, MSs divisions, and sub-divisions) available in the reports and regional estimations were obtained for each geographical scale of assessment. This allowed exploring the local pooled effects by classifying divisions and sub-divisions within MSFD sub-regions, whenever possible. Statistical maps were plotted to show the spatial distribution for the pooled results.
Since the only ecosystem element common to all MSs was Fish, a subset of the first database was built only including fish elements. This new dataset included food web criteria targeting Fish elements that were reported more than once, by more than one MS, to enable statistical significance and comparison across assessments. The food webs criteria fulfilling these thresholds (considering the Fish group) are identified in Table 1. Geographically referenced data on relevant anthropogenic pressures occurring in the marine environment was retrieved for each spatial unit of assessment. The pressures considered were fisheries, climate anomalies, noise, and input of nutrients, as these are the source of the most prevailing effects exerted by human activities in the European seas (Halpern et al., 2008; Crise et al., 2015; Korpinen et al., 2021). The data collected, its source and the temporal range are showed in Table 2.
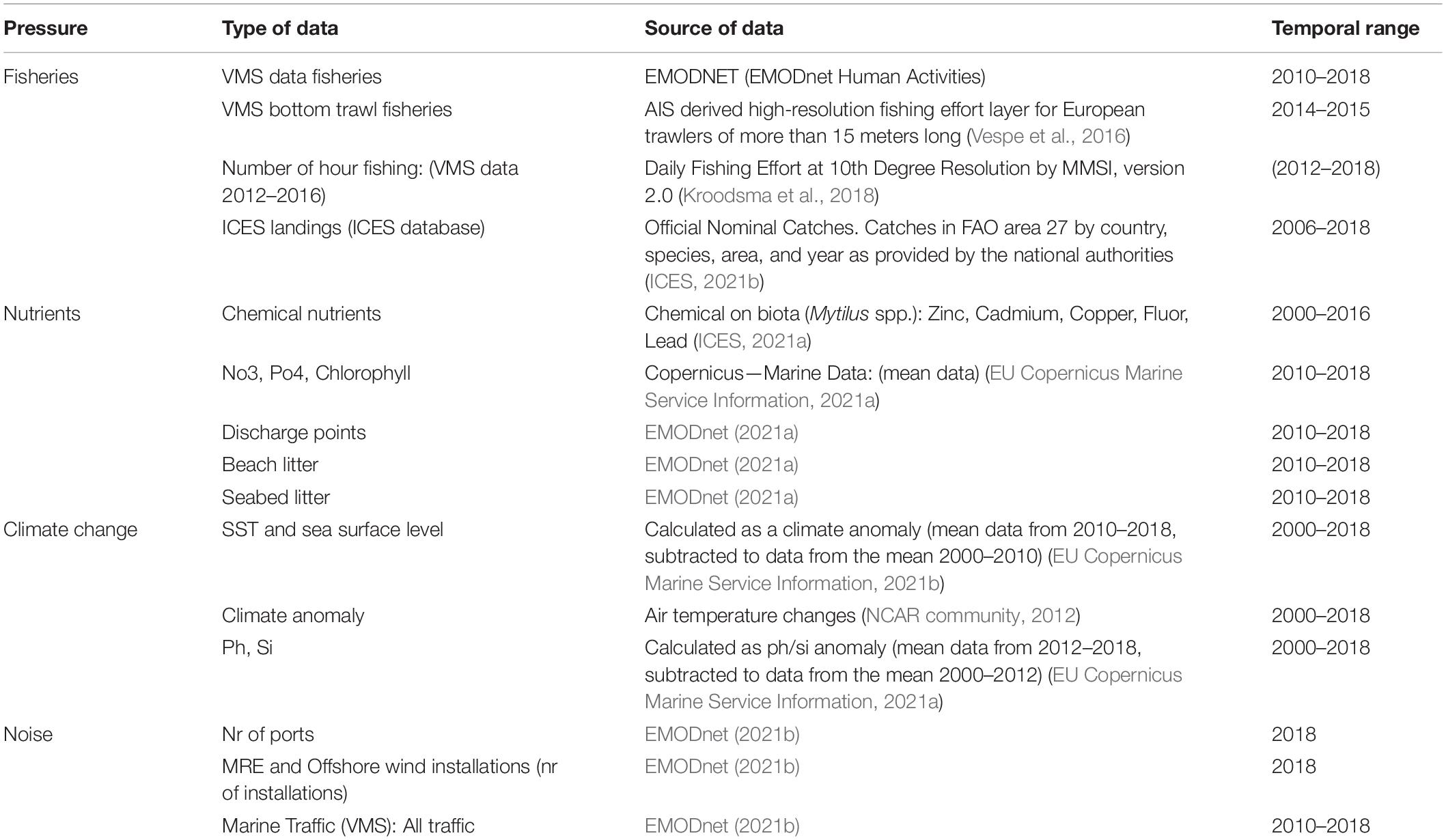
Table 2. Anthropogenic data retrieved for the study: type of pressure, data, source, and temporal scale of the dataset.
Data Analysis
To compare the food webs assessment made to answer MSFD requirements for each subregion, MS, ecosystem element (target) and criterion, the number of reported indicators and the corresponding assessment trend were estimated for each of these parameters. Assessment trends for each indicator were transformed into dummy variables: −1—Decreasing, 0—Stable, and 1—Increasing. A generalized linear model (GLM), using negative binomial distribution, was applied to the assessment trend, to understand if trend patterns were related to the categorical factors under study: subregion, MS, ecosystem element and criteria/indicator. Prior to the analysis, data were tested for normality (Shapiro-Wilk test) and homogeneity of variance (Cochran test) (Zar, 1999).
Since criteria addressing fish elements were common to all MSs, these criteria were selected for subsequent analysis. Multivariate analysis was used to find similarities between food web assessment trends across MSs units and to understand if anthropogenic pressures significantly explained variations observed. To delineate groups with distinct reporting strategy a hierarchical agglomerative clustering using arithmetic averages (CLUSTER) was used based on the Bray-Curtis similarity measure (Clarke, 1993) after the fourth-root transformation of reporting trends, according to Field et al. (1982). Afterward, a principal coordinates analysis (PCO) was performed on anthropogenic pressures normalized data using the Euclidean distance to determine if there is a spatial pattern of pressure variables that were associated with MSs food webs assessment [pressures data used: fisheries mean, ph, silica, seabed litter, beach litter, sea surface temperature anomaly, sea surface level, chlorophyll, phosphates (po4), nitrates (no3), port number, distance to port, bottom trawl (mean), marine traffic, number of wind turbines, the average number of wind turbines, CPUE, zinc, lead, cadmium, copper, and fluor across reported units (see data description in Table 2)] (Clarke and Warwick, 2001). A permutational multivariate analysis of variance (PERMANOVA) was used to test the hypothesis for significant differences among the cluster groups (defined by the Bray-Curtis measure on fourth root transformed data) (Anderson et al., 2008). The similarity percentages (SIMPER) routine was applied to identify which indicators contributed the most to the within-cluster similarity and the between-cluster dissimilarity (Clarke, 1993). Finally, the BEST (BIOENV) procedure was used to find the subset of pressure variables that significantly explained the clusters of food webs assessment trends determined in the cluster analysis, using the Spearman correlation (Clarke, 1993). All statistical analysis were developed in R software environment (R Core Team., 2019) and multivariate analyses were performed in PRIMER 6.0 software (Clarke and Gorley, 2006).
Results
A total number of 258 food web criteria were reported in the studied area. The number of criteria implemented per subregion, MS, ecosystem element and criteria/indicator type is shown in Supplementary Table 1. Food web criteria were more reported in the CS subregion (115), followed by GNS (75) (only including the UK section) and BBIC (65) subregions. The MS with the highest number of reported criteria was the United Kingdom (159), followed by PT (38) and SP (35). Ireland and France showed a very low effort for D4 implementation with 22 and 12 criteria, respectively. The most reported ecosystem elements were Fish (121), followed by Plankton (100) and Marine Mammals (25) (Figure 2A). Ecosystem elements targeted varied between MSs: the UK assessment included a wider number of ecosystem elements such as Fish, Plankton, Marine Mammals, and Marine birds), while other MSs only reported Fish and Plankton (IR) or just Fish (PT, FR, SP). Criteria reported varied between subregions and MS (Figures 2B,C). The most reported Criteria was the relative abundance of pairs (RAP), followed by maximum mean length (MML) and typical length (TyL)—all reported by the United Kingdom. Several criteria were only reported once across all studied MSs, i.e., Shannon diversity (kg), Shannon diversity (n), Species richness, Trophic diversity, Trophic diversity (vol), Trophic richness.
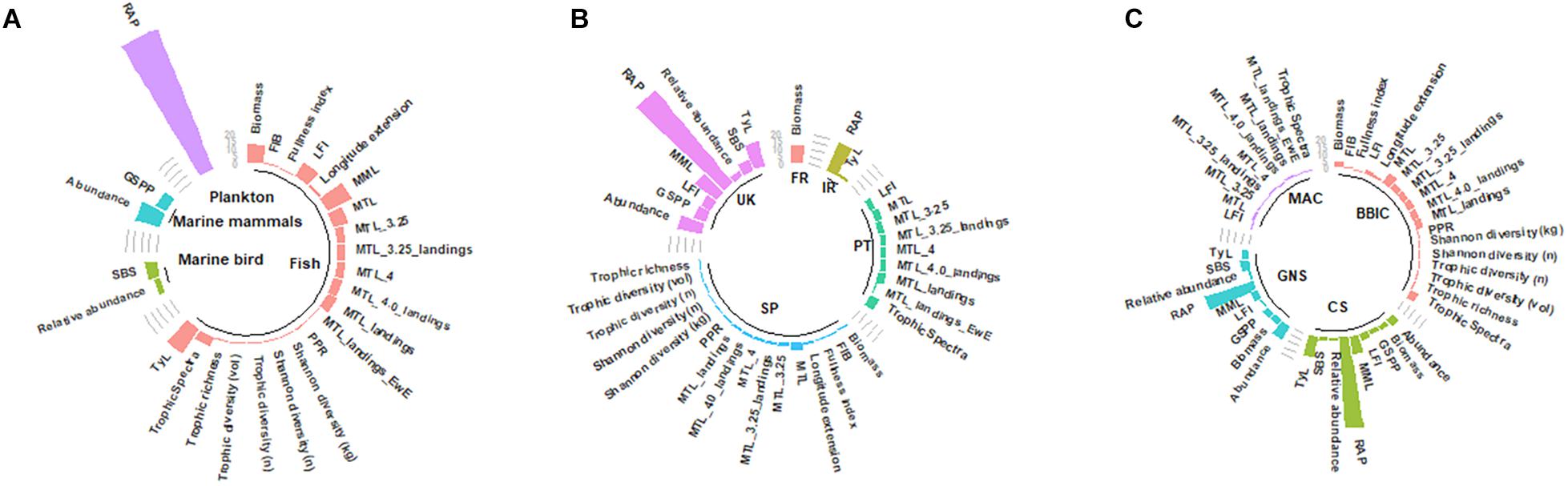
Figure 2. Number of reported criteria per ecosystem element (A), Member state (B) and subregion (C).
General linear models showed that factors influencing food webs average assessment trend were MSs, ecosystem elements and criteria, explaining 34.1% of existing deviance (p < 0.05; Table 3). MSs and ecosystem elements explained 3.7 and 3.8% of existing deviance, respectively. MSs average trends significantly decreased in FR (−0.46; SE = 0.16), while other MSs presented stable trends (Figure 3A). Significantly increasing trends were found for Marine mammal (0, 16; SE = 0.17), while a decrease was found for Marine birds (−0.42; SE = 0.19); however, these two ecosystem groups were only reported by the UK (Figure 3B). As for criteria, results showed that this factor the highest percent of existing deviance with 21.22%; Fishing in Balance (FiB), Primary Production Required (PPR) and Fullness index (FI) presented significantly higher average trends (with 1; SE = 0.00), while Trophic Diversity (n), Species richness (n), Trophic richness and Relative abundance presented the lowest significant trend (with −1; SE = 0.00) (Figure 3C).
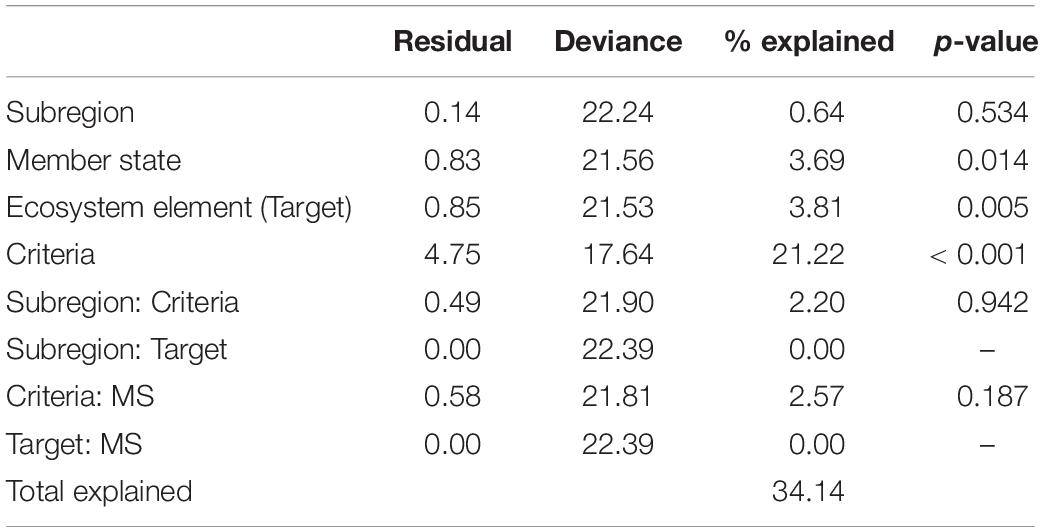
Table 3. Generalized linear models (GLMs) with gamma distribution applied to the average trend data for Descriptor 4—Food webs, per subregion, Member State, criteria, ecosystem element, and their corresponding interaction.
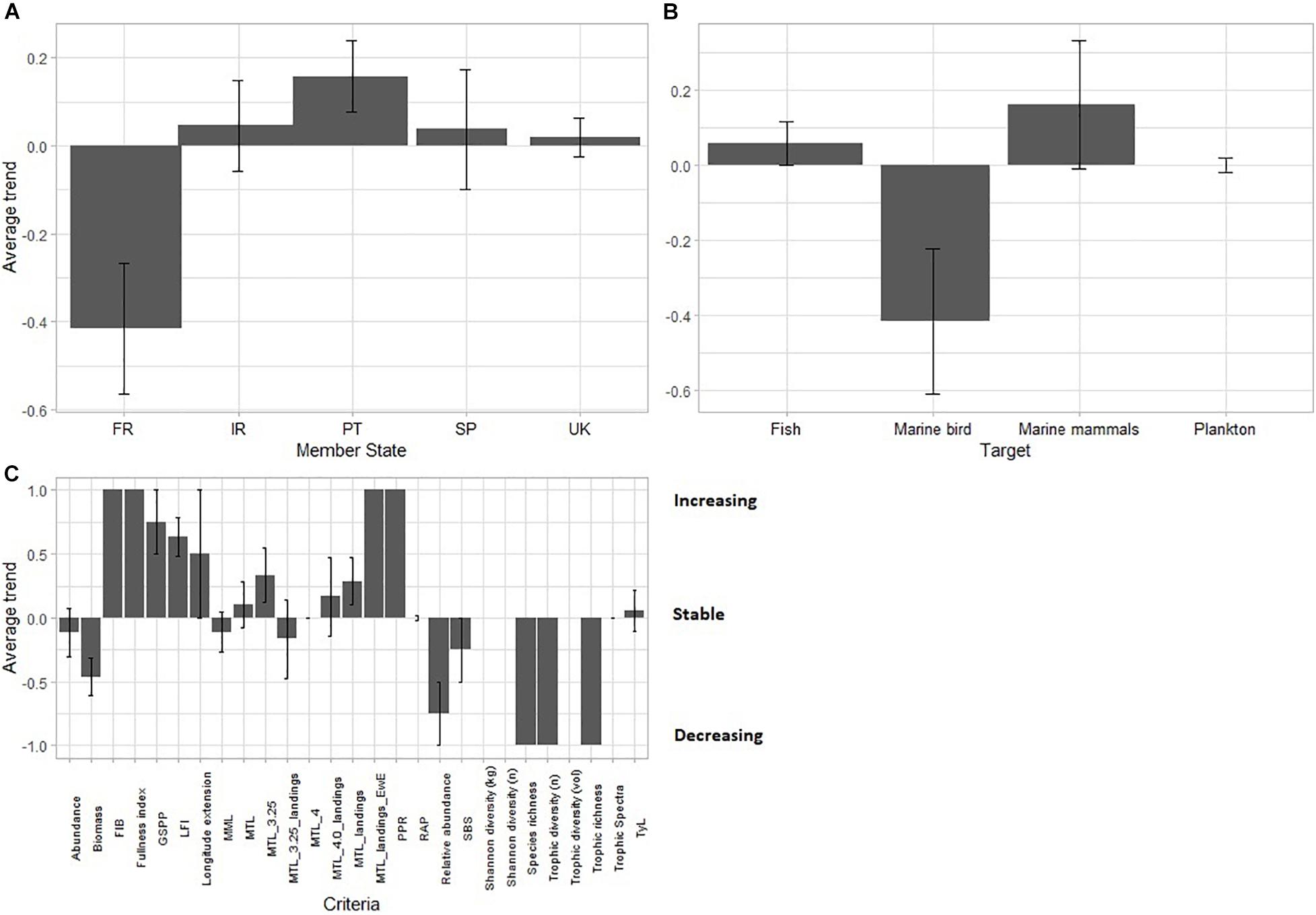
Figure 3. Average assessment trend for food web criteria per MS (A), ecosystem element (B) and criteria (C) resulting from the GLM analysis applied to Descriptor 4—Food webs data in the NE Atlantic.
When looking at food webs assessment targeting exclusively fish ecosystem elements, of the 115 criteria reported, 95 respected the established thresholds (were implemented more than once and were common to, at least, two MSs). These were reported mostly by BBIC (42), followed by the CS (33). As for MSs, UK was the country with the highest reporting number (42), followed by PT (30), while IR exhibited the lowest (2). The most reported criteria were TyL, MML, and Large Fish Indicator (LFI)/Mean Trophic Level (MTL) (with 19, 18, and 11 criteria, respectively) (Figure 4A). There was a division between subregions: MSs reporting for the GNS and CS (UK and IR) subregion used TyL, MML, and LFI fish criteria, while BBIC and MAC (SP and PT) used MTL, MTL with thresholds (using survey and landing data) and LFI (only in PT assessment) (Figure 4A).
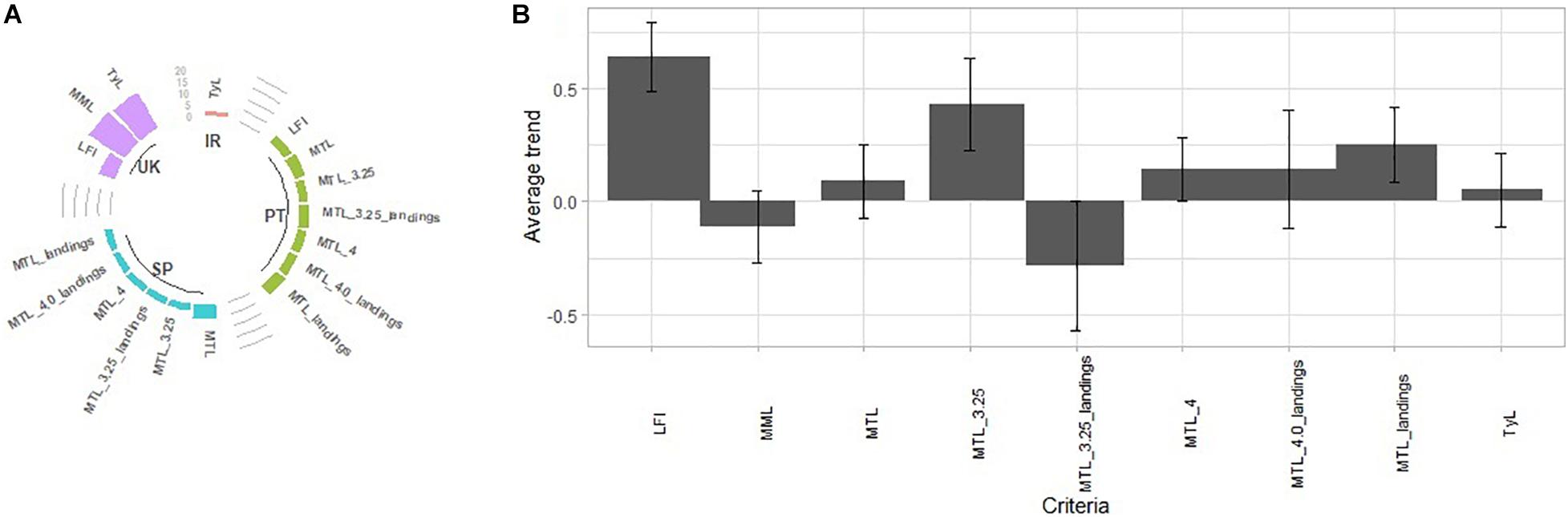
Figure 4. Number of fish criteria reported per Member State (A) and average assessment trend for fish food web indicators (B).
The average trend for fish criteria varied between 0 (SE = 0.00) in MAC and 0.2 (SE = 0.77) in GNS subregions. The MS with the highest average trend was IR, and PT, with 0.5 (SE = 0.71) and 0.2 (SE = 0.53), while the lowest trends were reported by SP and UK with a 0.1 average trend (SE = 0.62 and SE = 0.72, respectively). When analyzing criteria, results showed that the highest trends were obtained for LFI and MTL_3.25 (0.64; SE = 0.50 and 0.43; SE = 0.53, respectively), while the lowest trends were exhibited by MTL_3.25_landings and MML (−0.29; SE = 0.76 and −0.11; SE = 0.68, respectively) that exhibited decreasing patterns (Figure 4B).
In regard to the multivariate assessment, the cluster analysis revealed three groups (d, e, and g), three separate units (b, c and f) and one outlier (a) at the distance level of 54.9% (Figure 5A). When these clusters were superimposed with the spatial areas of assessment of each MS, a pattern could be observed (Figure 5B). The first group included eight UK assessment units (cluster d) and presented an average similarity of ∼86.99% within units. Two units also reported by the UK were separated from this cluster (cluster b and c, with 50–55% of similarity to cluster d). The second cluster included all PT units (including the Azores) and exhibited 93.24% of within-group similarity (cluster g). This cluster presented an average similarity of 61.69% to a single SP assessment unit (Cluster f). The third cluster included two SP assessment units (Cluster e) and presented 100% of within similarity (average trends were identical). The cluster plot showed that PT units had similarities to SP and UK reporting units (with 61.69 and 15.11% of similarity). The similarity between SP and PT was due to MTL reporting, and the similarity between PT and UK was due to LFI reporting, while the SP units presented no similarity (0%) with the UK ones (Figure 5A). In what concerns the outliers, a single SP assessment unit presented a dissimilarity of 97.5% from the overall units. Results showed that assessment for SP units was more heterogeneous, since it was separated in two cluster groups and one outlier, while PT and UK were more homogeneous (Figures 5A,B).
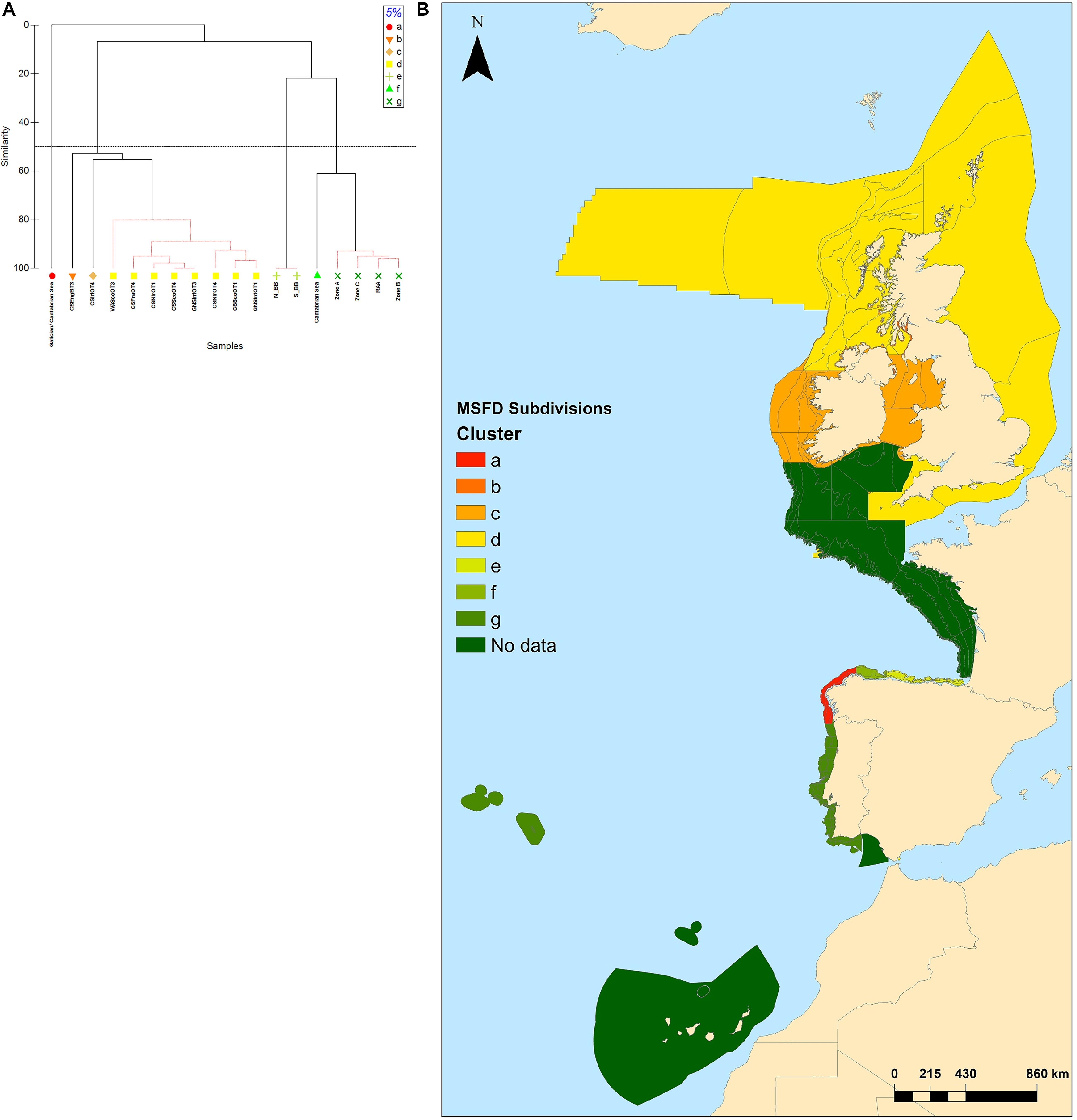
Figure 5. Dendrogram resulting from cluster analysis of Food webs transformed average trend, reported by Member States (A) and spatial distribution of the cluster assemblages (B). Five assemblages and three outliers were identified at the 57% level of similarity.
The PCO analysis for pressure variables showed a relevant pattern for sst, ssl, cadmium, mean fishing, Marine Traffic, and number of ports across Axis 1, which explained 28.1% of the variation. High values for these variables explained most of the PT and SP average trend results (clusters e, f, and g). Axis 2 explained 23% of the variation and was associated with a high amount of chemical nutrients (Lead, Copper, Zinc) and a high number of offshore wind turbines. This axis explained the UK units from the GNS and CS (cluster d and b) and SP (North Bay of Biscay units). Axis 3 explained 13% of variability, including variables such as no3, po4, port distance, and explained UK trend estimates (including clusters c and d). Axis 4 and 5, explained 10.1 and 9.1 21% of the variation, respectively. These had a high influence of CPUE and zinc, respectively. These two axes explained average trend patterns for partial units from the PT cluster (Zone B) and SP (North Bay of Biscay units) (Figure 6).
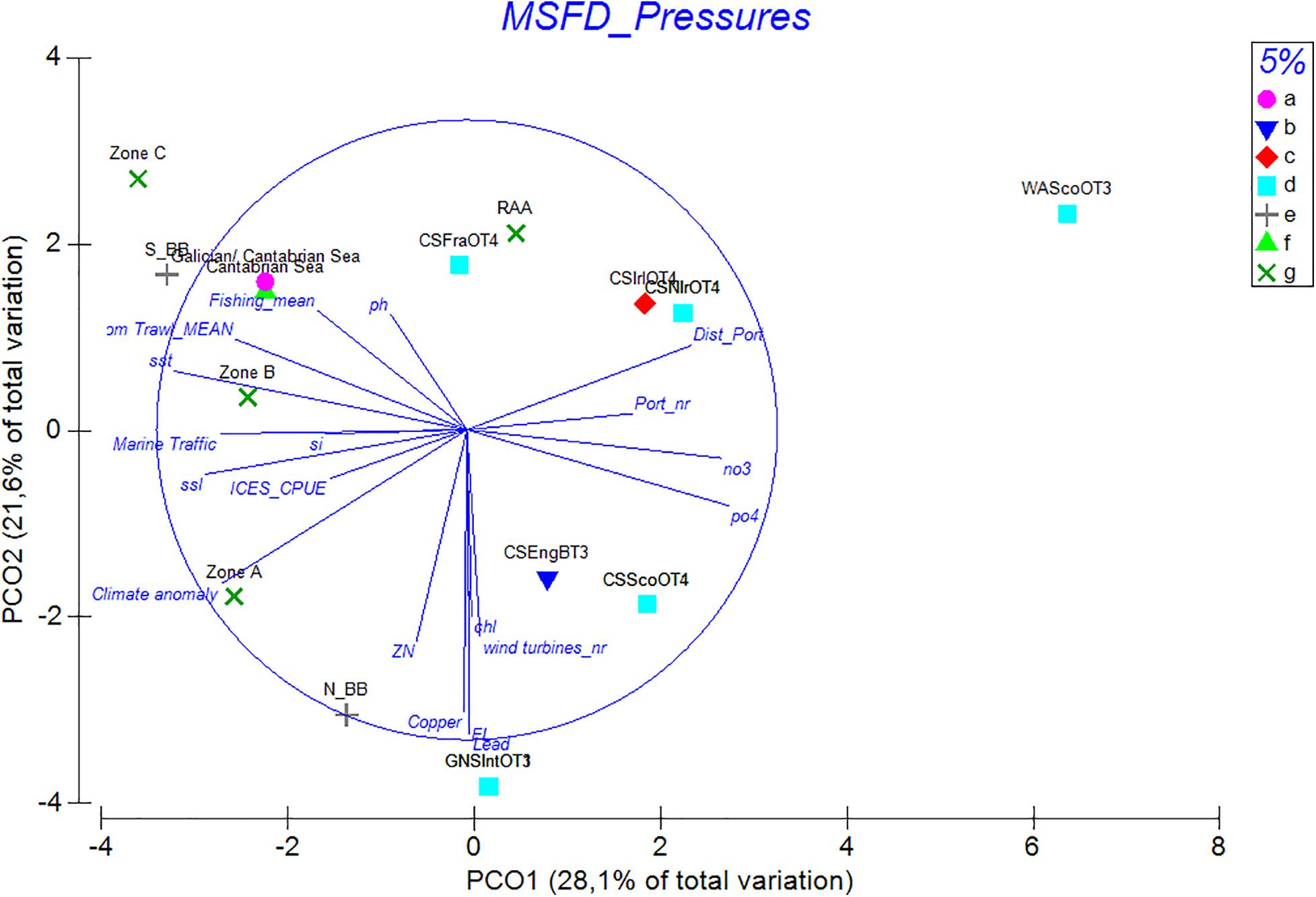
Figure 6. Principal coordinates analysis (PCO) for the anthropogenic pressures’ variables identified in Table 2. Clusters as obtained in the cluster analysis represented in Figure 4A.
PERMANOVA analysis showed significant differences between clusters (F = 86.26; P(perm) < 0.001) and the pair-wise analysis revealed significant differences among UK and SP units (d, e) and UK and PT units (d–g). Cluster e showed significantly higher trends (e = 1.00; SE = 0.22 average trend), while cluster a had significantly lower average trends (a = −1.00; SE = 0.30 average trend), evidencing heterogeneity in the SP reporting.
The SIMPER analysis showed that the within-group similarity ranged from 85.61 to 100% (groups d and e, respectively) and that the criteria that mostly contributed to this were MML, and TyL in group d, and MTL in group e. The between-groups dissimilarity varied between 39.31 and 100%, and the main discriminating species are listed in Supplementary Table 2.
The results of the BEST analysis, using anthropogenic data, revealed that the combination of sst, and mean bottom trawl provided the best match to explain the average reported trends. The correlation values were high (r = 0.592) and their influence was statistically significant (p < 0.01). When added to the combination, po4, Marine Traffic, and Zinc also presented a significant correlation with the average reported trends (r = 0.589).
Anthropogenic pressures identified above have been analyzed using descriptive (graphical) and statistical (correlation; spearman test) analysis to determine if they correlate with food web trends summaries for fish. The analyses showed that, in BBIC, an increase in sst and CPUE were associated with decreasing trends for food webs. While in the CS and GNS, high marine traffic and the number of offshore installations corresponded to food webs decreasing trends. However, none of these variables was significant.
Discussion
The new MSFD report was published in 2020, pointing out broad progresses in relation to the 1st MSFD report, published in 2012. Improvements were largely driven by the recently published legal documents that defined aspects such as criteria, ecosystem components, anthropogenic pressures and spatial scales for reporting (European Commission, 2017a,b), but were also motivated by MSs effort, that revealed lessons learned from the 1st cycle and increased reporting coherence (European Commission, 2020). However, food webs descriptors are still not properly assessed, are poorly coordinated within each subregion and are underreported due to a lack of data and consensus on the indicators or indexes that should be used. Food webs (D4) assessment is complex by nature as it needs to measure energy flow across guilds through simple parameters, that require detecting changes on energy transfer (ICES, 2015).
This work assessed D4 implementation and assessment results across the NE Atlantic basin, including four subregions and six MSs, to disentangle if the current assessment encompassed the ecological aspects of food webs at subregion scale (European Commission, 2017a) and if trends detected emergent properties from single or cumulative anthropogenic pressures.
Results revealed that reporting strategies varied between MSs since each country supplied a distinct level of information. Relevant knowledge gaps were identified for IR and FR, which reported two or one indicators, while MSs such as UK, SP, and PT developed fit-fo-purpose methods (ICES, 2015; Tam et al., 2017). As a result, reporting differences were found between MSs, ecosystem elements and criteria selected, showing a lack of congruency in D4 implementation. The UK was the only MS addressing other ecological elements than Fish and Plankton (i.e., marine mammals, marine birds) using specific data sets and indicators. This was due to the adoption of long-term monitoring programs and data availability. Also, the UK has closely followed OSPAR guidance in the implementation of indicators, what largely contributed to the choice of criteria (OSPAR., 2017; UK Marine Monitoring, Assessment Strategy, Uk Monitoring, and Assessment Reporting Group., 2019). That approach was not followed by other MSs in the CS, such as IR and FR.
In the BBIC subregion, SP and PT implemented food webs metrics indicated by ICES (2015) or Tam et al. (2017) and were coherent to some extent. On the other hand, the FR assessment reported raw information from stock assessment, not implementing food webs indicators and revealing lower reporting level and average trends. In the BBIC subregion, no higher-trophic (seabird and megafauna productivity) and lower-trophic (primary production) ecosystem elements were addressed. Indicators assessing such groups are needed to reflect processes viewed from the opposite ends of the food web (e.g., PPR, zooplankton index, seabird productivity index, etc.). For example, PPR is an integrative indicator that represents the amount of primary productivity to sustain a fishery and enables the comparison of energy requirements across different fisheries (Pauly and Christensen, 1995; Chassot et al., 2010; Tam et al., 2017), while seabird productivity is an indicator of ecosystems health, through food availability (forage fish), accumulation of contaminants and environmental pollutants, and physiologic stress caused by environmental change (Mallory et al., 2010).
According to the Directive, EU Member States can monitor as many guilds as deemed appropriate (with a minimum of three), but at least two non-fish guilds should be addressed to ensure that not only fish are monitored (European Commission, 2017a). Even though indicators based on fish abundance and biomass can inform on the structural properties of food webs, they provide only partial information about its functioning, failing to consider complex trophic interactions and whole-system energy flow (Rombouts et al., 2013). However, only the UK evaluated two non-fish guilds. The causes pointed out to explain this inequality are knowledge gaps in long term monitoring or the inexistence of minimum quality data that can support these assessments. Currently, the best data available comes from commercially exploited fish and shellfish stocks for which extensive monitoring programs exist and from phyto- and zooplankton communities obtained through the Continuous Plankton Recorder; but even these are limited to certain areas of the North Atlantic Ocean (European Commission, 2020). To overcome this issue, the EU has identified strategies such as the use of theoretical and empirical models to identify potential impacts and key properties that should be monitored and the necessity of harmonized monitoring programs to generate proper assessments for trophic levels (and marine regions) (European Commission, 2020).
The average trends established in the assessments were stable or improved in the UK, SP, PT and IR, and decreased in the FR assessment. The UK presented a significantly decreasing trend for Marine birds, that were considered below GES or at risk in the CS and GNS. Decreasing patterns are of concern and direct management actions could be either top-down control rules aimed at relieving fishing pressure on lower-trophic species or bottom-up policies directed to improve water quality or habitat, which may also include improved management at land-sea interfaces (Mallory et al., 2010; Tam et al., 2017). On the other hand, marine mammals exhibited the highest average trend, evidencing increasing populations in the UK and indicating a recovery of mammal’s populations. For the fish group, the average trend reported varied significantly between the type of metric employed, which resulted in high reporting heterogeneity.
When analyzing food webs assessment approaches employed, the multivariate analysis applied to fish criteria revealed three similar food webs assessment groups including UK units, PT units, and SP units. Some units from IR and SP were considered dissimilar from the main groups. Within group similarities were based on MS and showed that UK, IR, PT, and SP adopted distinctive reporting metrics and therefore resulting trends. Although the UK and IR used similar indicators (MML and TyL), IR data sets did not enable a comprehensive assessment such as the UK, because the survey time-series from Irish waters is comparatively shorter (Machado et al., 2019); what has resulted in a dissimilar assessment for IR (European Commission, 2020). To some extent, PT and SP used identical indicators (MTL and MTL with thresholds) increasing similarities. However, the PT assessment adopted indicators equally across all its spatial units, while the SP assessment employed indexes heterogeneously across its territory. The SP assessment reported research findings obtained by EU funded projects (EcArpha project), and peer-reviewed publications (Arroyo et al., 2019; Preciado et al., 2019). These studies included fit for purpose outputs concerning food webs assessment, but the metrics, and the temporal and spatial scales were dissimilar between them, what could explain part of the heterogeneity obtained in the SP assessments and the significant differences found between the SP clusters’.
When looking at anthropogenic pressures and how they overlap with food webs assessment trends, results for UK units in the CS basin were influenced by high input of organic nutrients (no3, po4) and port distance, while GNS units were influenced by primary production (chl), chemical nutrients (Lead, Zinc, Cadmium) and the number of offshore installations. The high input of nutrients in the UK-CS units evidenced eutrophication and higher primary productivity, which can increase bottom-up effects (Cury and Roy, 1989). In the NE Atlantic, eutrophication has been recorded in the southern parts of the North Sea and along the North western coast of France. Nevertheless, nutrient inputs from point sources have significantly decreased; although inputs from diffuse sources, i.e., losses from agricultural activities, are still too high (European Commission, 2020). In the GNS, chemical contamination has been decreasing due to regulations adopted, however, Cd levels are increasing in the Southern North Sea and need to be investigated. The assessment of chemical contaminants under the WFD showed that the worst scenarios can be been found in the Baltic and the GNS, with 55 and 51% of the area assessed below GES (European Commission, 2020). As for offshore installations (e.g., offshore renewable energies), long term impacts are still relatively unknown: offshore wind farms are a recently developed sector for which there are no long-term monitoring data. As a consequence, there is still a high level of uncertainty on the impacts of offshore wind parks on ecosystem structures and processes (Alexander et al., 2015). Noise affects especially marine mammals, but existing studies also show that habitat change, by adding artificial hard substrate in areas where mainly soft substrate occurs, can cause food webs shifts. Artificial reefs, such as offshore wind farms, are used by benthopelagic and benthic species as feeding grounds for prolonged periods (Mavraki et al., 2021).
In PT and SP units, anthropogenic pressures such as fishing (bottom trawl and mean fishing), seabed litter, sst, ssl, ph, and marine traffic explained food webs trends. Similar results were found by Korpinen et al. (2021) for this region, identifying global warming (increasing sst), fisheries and shipping (underwater noise) as the major challenges that need to be addressed when considering cumulative anthropogenic effects. This region is characterized by narrow shelf areas (Korpinen et al., 2021), were trawling activities occur more intensely (Eigaard et al., 2017). Studies on the ratio of the trawling footprint over the landings showed that the highest ratios occurred in the Iberian Portuguese area, reflecting the higher level of exploitation when compared with some of the Atlantic management areas where fishing effort has been reduced (Eigaard et al., 2017). Regarding marine traffic, this activity is widely distributed in all EU marine regions and its intensity is highest along shipping corridors and near ports. Underwater noise from commercial shipping is considered one of the most pervasive noise sources. Underwater distribution and noise effects occurring in Europe are still unknown, however, impacts have been observed on all trophic levels, from invertebrates to fish, marine mammals, and diving seabirds (Dekeling et al., 2014; Barnett, 2020; Farcas et al., 2020). Climate anomalies (i.e., sst, ssl, and ph) also explained food web average trends in the southern countries. Climate change effects on latitudes of species transition, such as the Iberian Peninsula, are expected to have wider degradation effects on food webs as the cold water species habitat may be contracted, and warmer species habitat may expand (Serrat et al., 2018), changing food webs structure and resilience (Lynam et al., 2017). Even minor temperature changes can have significant effects on the onset of the spring phytoplankton bloom, the relative abundance of zooplankton, and the abundance and distribution of commercial fish species (Alexander et al., 2015).
The resulting matrix showed significantly high correlation values and as a result, it was possible to establish links between trends of abundance and distribution of fish elements and human pressures, such as fishing and climate anomalies and marine traffic in the southern region of the BBIC or the input of nutrients and/or chemicals in the UK waters. These findings indicate a relationship between food webs pressure and state and highlight the most relevant anthropogenic disturbances across the marine areas under study. Nevertheless, the methodological approach applied in this work, presented limitations since the assessment data set was limited by MSs reporting (only enabling the comparison of fish elements), it did not account for spatial and temporal variability and anthropogenic pressures were difficult to unequivocally distinguish from environmental variability. Notwithstanding, this work provides relevant insights on aspects that are hindering the detection of impacts and need to be considered in future assessments such as (1) the metrics/indicators and ecosystem elements assessed should be harmonized across the same subregion, (2) the improvement of data mining and modeling for well monitored indicators (status and pressures) (Walmsley et al., 2017; Borja et al., 2019), (3) further development of monitoring networks, (4) improve the spatial coverage and resolution of the assessment, since not all MSs are adopting similar scales (Machado et al., 2019, 2020), (5) the methodologies and standards should account for the specificities of the region and detect region-specific sensitivity values (European Commission, 2020).
Future work on food webs should use data that are often automatically recorded (e.g., automatic identification system (AIS) for shipping, vessel monitoring system (VMS) for fisheries), stored in permit databases (e.g., marine construction, dredging, dumping, fish catches), or observed from satellites (e.g., sst, chl, ph, oil spills, etc.). The use of these data sets could improve the assessment of single or cumulative effects on food webs, which in the past have been limited by data availability (Borja et al., 2019; Korpinen et al., 2021). However, more work is still needed in food web index implementation and development, to include non-linear responses and synergistic and antagonistic effects of pressures on ecosystem elements (Korpinen and Andersen, 2016; Taherzadeh et al., 2019).
In general, although the EU has surpassed previous assessments, food web evaluation is still lacking an appropriate ecological dimension. The present study emphasized the need for EU MSs to further improve their coordination and calibration at sea basin level, concerning ecosystems elements, criteria, indicators, and the spatial and temporal scales used in food webs assessment and monitoring programmes. Only afterword’s it will be possible to determine coordinated objectives and targets and having effective measures tackling the right pressures. The analysis proposed here allows, as a first step, to define the highest pressures by which managers can steer toward food web targets in the studied basins. Insights to this discussion are both timely and relevant, especially as EU MSs are preparing their program of measures to fulfill the aims of the MSFD.
Conclusion
The present work highlights relevant aspects that need to be tackled in the assessment of food web in the context of the MSFD. The criteria and indicators selected need to be further calibrated, concerning the target element addressed and the metric employed, at subregion level. Although some advances have been made in this direction with the legal diploma 848/2017, the present work showed that food webs assessment is largely dependent on MS reporting. Only by using a harmonized set of indicators it will be possible to assess food webs status at an ecosystem level (at the subregion and sea basin level), to understand the effects of different human pressures, and to define effective management decisions. Importantly, the direct or indirect anthropogenic pressures were detected by trends assessed in the MSFD. The pressures of concern were fisheries, and climate change in the Iberian Peninsula, while eutrophication and chemical contamination affected CS and GNS surveyed regions. Overall, the MSFD assessment showed that human activities are not at an environmentally sustainable level and that pressures exert combined effects on food webs ecosystem components, i.e., fish. At the moment, the most urgent step would be to ensure a coherent assessment that foresees ecological relevant aspects of food webs and considers effects of relevant and on-going human pressures (Elliott et al., 2020), therefore promoting sea basin level calibration. A basis for this would be to continue to promote monitoring programs and data rich platforms that can support assessments (Korpinen et al., 2021).
Data Availability Statement
Publicly available datasets were analyzed in this study. This data can be found here: https://cdr.eionet.europa.eu/ and https:// water.europa.eu/marine/about/wise-marine.
Author Contributions
IM carried out data analysis and wrote the manuscript. JC and HC provided guidance on the analysis and provided edits for the manuscript. All authors contributed to the article and approved the submitted version.
Funding
This publication was financed by the Portuguese national funds through FCT–Fundação IP under project reference UIDB/04292/2020–granted to MARE, project reference UIDB/50019/2020–granted to IDL. This publication was also funded by the European Union’s Horizon 2020 Research and Innovation Programme under grant agreement N810139: Project Portugal Twinning for Innovation and Excellence in Marine Science and Earth Observation - PORTWIMS. IM has received funding support from the FCT–Fundação IP through a Ph.D. fellowship (PD/BD/135065/2017).
Conflict of Interest
The authors declare that the research was conducted in the absence of any commercial or financial relationships that could be construed as a potential conflict of interest.
Publisher’s Note
All claims expressed in this article are solely those of the authors and do not necessarily represent those of their affiliated organizations, or those of the publisher, the editors and the reviewers. Any product that may be evaluated in this article, or claim that may be made by its manufacturer, is not guaranteed or endorsed by the publisher.
Supplementary Material
The Supplementary Material for this article can be found online at: https://www.frontiersin.org/articles/10.3389/fmars.2021.699566/full#supplementary-material
References
Alexander, K. A., Kershaw, P., Cooper, P., Gilbert, A. J., Hall-Spencer, J. M., Heymans, J. J., et al. (2015). Challenges of achieving good environmental status in the Northeast Atlantic. Ecol. Soc. 20:49. doi: 10.5751/ES-07394-200149
Anderson, M., Gorley, R. N., and Clarke, R. K. (2008). Permanova+ for Primer: Guide to Software and Statisticl Methods. Plymouth: Primer-E Ltd.
Arroyo, N.-L., Safi, G., Vouriot, P., López-López, L., Niquil, N., Le Loc’h, F., et al. (2019). Towards coherent GES assessments at sub-regional level: signs of fisheries expansion processes in the Bay of Biscay using an OSPAR food web indicator, the mean trophic level. ICES J. Mar. Sci. 76, 1543–1553. doi: 10.1093/icesjms/fsz023
Barnett, K. (2020). Underwater Noise-The Neglected Threat to Marine Life. Hamburg: Coalition Clean Baltic.
Borja, A., Garmendia, J. M., Menchaca, I., Uriarte, A., and Sagarmínaga, Y. (2019). Yes, we can! large-scale integrative assessment of European regional seas, using open access databases. Front. Mar. Sci. 6:19. doi: 10.3389/fmars.2019.00019
Chassot, E., Bonhommeau, S., Dulvy, N. K., Mélin, F., Watson, R., Gascuel, D., et al. (2010). Global marine primary production constrains fisheries catches. Ecol. Lett. 13, 495–505. doi: 10.1111/j.1461-0248.2010.01443.x
Clarke, K. R. (1993). Non-parametric multivariate analyses of changes in community structure. Austr. J. Ecol. 18, 117–143. doi: 10.1111/j.1442-9993.1993.tb00438.x
Clarke, K. R., and Gorley, R. N. (2006). PRIMER V6: User Manual-Tutorial. Plymouth: Marine Laboratory.
Clarke, K. R., and Warwick, R. M. (2001). Change in Marine Communities: An Approach to Statistical Analysis and Interpretation, 2nd Edn. Plymouth: Primer-E Ltd.
Coll, M., Shannon, L., Kleisner, K., Juan-Jordá, M., Bundy, A., Akoglu, A., et al. (2016). Ecological indicators to capture the effects of fishing on biodiversity and conservation status of marine ecosystems. Ecol. Indic. 60, 947–962. doi: 10.1016/j.ecolind.2015.08.048
Crise, A., Kaberi, H., Ruiz, J., Zatsepin, A., Arashkevich, E., Giani, M., et al. (2015). A MSFD complementary approach for the assessment of pressures, knowledge and data gaps in Southern European Seas: the PERSEUS experience. Mar. Pollut. Bull. 95, 28–39. doi: 10.1016/j.marpolbul.2015.03.024
Cury, P., and Roy, C. (1989). Optimal environmental window and pelagic fish recruitment success in upwelling areas. Can. J. Fish. Aquat. Sci. 46, 670–680. doi: 10.1139/f89-086
Dekeling, R. P. A., Tasker, M. L., Van der Graaf, A. J., Ainslie, M. A., Andersson, M. H., André, M., et al. (2014). Monitoring Guidance for Underwater Noise in European Seas, Part I: Executive Summary. A guidance document within the Common Implementation Strategy for the Marine Strategy Framework Directive by MSFD Technical Subgroup on Underwater Noise. Report No. EUR 26557 EN. Luxembourg: Publications Office of the European Union.
EEA (2020). Eionet–Central Data Repository. Available online at: https://cdr.eionet.europa.eu/ (accessed April 2, 2021).
EEA (2021). WISE Marine. Marine Information System for Europe. Available online at: https://water.europa.eu/marine/about/wise-marine (accessed April 6, 2021).
Eigaard, O. R., Bastardie, F., Hintzen, N. T., Buhl-Mortensen, L., Buhl-Mortensen, P., Catarino, R., et al. (2017). The footprint of bottom trawling in European waters: distribution, intensity, and seabed integrity. ICES J. Mar. Sci. 74, 847–865. doi: 10.1093/icesjms/fsw194
Elliott, M., Borja, A., and Cormier, R. (2020). Activity-footprints, pressures-footprints and effects-footprints – walking the pathway to determining and managing human impacts in the sea. Mar. Pollut. Bull. 155:111201. doi: 10.1016/j.marpolbul.2020.111201
EMODnet (2021a). EMODnet Chemistry. Available online at: https://www.emodnet-chemistry.eu/ (accessed April 7, 2021)
EMODnet (2021b). EMODnet Human Activities. Available online at: https://www.emodnet-humanactivities.eu/ (accessed April 7, 2021)
EU Copernicus Marine Service Information (2021a). Global Ocean Biogeochemistry Hindcast. Available online at: https://resources.marine.copernicus.eu/?option=com_csw&view=details&product_id=GLOBAL_REANALYSIS_PHY_001_030 (accessed March 15, 2021).
EU Copernicus Marine Service Information (2021b). MULTIOBS_GLO_ PHY_TSUV_3D_MYNRT_015_012. Strasbourg: EU Copernicus Marine Service Information.
European Commission (2008). Directive 2008/56/EC of the European Parliament and of the Council of 17 June 2008 Establishing a Framework for Community Action in the Field of Marine Environmental Policy. Copenhagen: Marine Strategy Framework Directive.
European Commission (2017b). Commission Decision (EU) 2017/848 of 17 of May 2017 Laying Down Criteria and Methodological Standards on Good Environmental Status of Marine Waters and Specifications and Standardised Methods for Monitoring and Assessment, and Repealing Decision 2010/477/EU (Text with EEA relevance). Available online at: http://data.europa.eu/eli/dec/2017/848/oj/eng (accessed April 8, 2018)
European Commission (2017a). Commission Directive (EU) 2017/845 of 17 May 2017 amending Directive 2008/56/EC of the European Parliament and of the Council as Regards the Indicative Lists of Elements to be Taken into Account for the Preparation of Marine Strategies (Text with EEA relevance). Available online at: http://data.europa.eu/eli/dir/2017/845/oj/eng (accessed April 8, 2018)
European Commission (2020). Review of the Status of the Marine Environment in the European Union Towards Clean, Healthy and Productive Oceans and Seas Accompanying the Report from the Commission to the European Parliament and the Council on the Implementation of the Marine Strategy Framework Directive (Directive 2008/56/EC). Brussels: European Commission.
Farcas, A., Powell, C. F., Brookes, K. L., and Merchant, N. D. (2020). Validated shipping noise maps of the Northeast Atlantic. Sci. Total Environ. 735:139509. doi: 10.1016/j.scitotenv.2020.139509
Field, J., Clarke, K. R., and Warwick, R. M. (1982). A practical strategy for analysing multispecies distribution patterns. Mar. Ecol. Prog. Ser. 8, 37–52. doi: 10.3354/meps008037
Halpern, B. S., Walbridge, S., Selkoe, K. A., Kappel, C. V., Micheli, F., D’Agrosa, C., et al. (2008). A global map of human impact on marine ecosystems. Science 319:948. doi: 10.1126/science.1149345
Henriques, S., Pais, M. P., Costa, M. J., and Cabral, H. (2008). Efficacy of adapted estuarine fish-based multimetric indices as tools for evaluating ecological status of the marine environment. Mar. Pollut. Bull. 56, 1696–1713. doi: 10.1016/j.marpolbul.2008.07.008
ICES (2015). Report of the Workshop on Guidance for the Review of MSFD Decision Descriptor 4 – Foodwebs II (WKGMSFDD4-I). Denmark: International Credential Evaluation Service.
ICES (2021b). Official Nominal Catches 2006-2018. Denmark: International Credential Evaluation Service.
ICES (2021a). ICES Environmental database (DOME), Extractions 10-15 FEBRUARY 2021; Biota data for the OSPAR CEMP. Available online at: https://dome.ices.dk/views/ContaminantsBiota.aspx (accessed April 10, 2021).
Korpinen, S., and Andersen, J. H. (2016). A global review of cumulative pressure and impact assessments in marine environments. Front. Mar. Sci. 3:153. doi: 10.3389/fmars.2016.00153
Korpinen, S., Laamanen, L., Bergström, L., Nurmi, M., Andersen, J. H., Haapaniemi, J., et al. (2021). Combined effects of human pressures on Europe’s marine ecosystems. Ambio 50, 1325–1336. doi: 10.1007/s13280-020-01482-x
Kroodsma, D. A., Mayorga, J., Hochberg, T., Miller, N. A., Boerder, K., Ferretti, F., et al. (2018). Tracking the global footprint of fisheries. Science 359, 904–908. doi: 10.1126/science.aao5646
Lynam, C. P., Llope, M., Möllmann, C., Helaouët, P., Bayliss-Brown, G. A., and Stenseth, N. C. (2017). Interaction between top-down and bottom-up control in marine food webs. Proc. Natl. Acad. Sci. U.S.A. 114, 1952–1957. doi: 10.1073/pnas.1621037114
Machado, I., Costa, J. L., Leal, M. C., Pasquaud, S., and Cabral, H. (2019). Assessment level and time scales of biodiversity indicators in the scope of the marine strategy framework directive – a case study for the NE Atlantic. Ecol. Indic. 105, 242–253. doi: 10.1016/j.ecolind.2019.05.067
Machado, I., Moura, T., Figueiredo, I., Chaves, C., Costa, J. L., and Cabral, H. N. (2020). Effects of scale on the assessment of fish biodiversity in the marine strategy framework directive context. Ecol. Indic. 117:106546. doi: 10.1016/j.ecolind.2020.106546
Machado, I., Teixeira, C. M., Costa, J. L., and Cabral, H. (2021). Identifying assessment scales for food web criteria in the NE Atlantic: implications for the marine strategy framework directive. ICES J. Mar. Sci. 78, 246–263. doi: 10.1093/icesjms/fsaa217
Mallory, M. L., Robinson, S. A., Hebert, C. E., and Forbes, M. R. (2010). Seabirds as indicators of aquatic ecosystem conditions: a case for gathering multiple proxies of seabird health. Mar. Pollut. Bull. 60, 7–12. doi: 10.1016/j.marpolbul.2009.08.024
Mavraki, N., Degraer, S., and Vanaverbeke, J. (2021). Offshore wind farms and the attraction–production hypothesis: insights from a combination of stomach content and stable isotope analyses. Hydrobiologia 848, 1639–1657. doi: 10.1007/s10750-021-04553-6
Ministério do Mar (2020). Reavaliação do Estado Ambiental e Definição de Metas: Parte D, Subdivisão do Continente. Estratégia Marinha, Relatório do 2° ciclo. República Portuguesa: Ministério do Mar.
NCAR community (2012). Statistical Downscaling of the Community Climate System Model (CCSM) Monthly Temperature and Precipitation Projections. Available online at: https://gis.ucar.edu/data/climate (accessed January 21, 2021).
OSPAR. (2017). Fish and Food Webs. Intermediate Assessment 2017. Available online at: https://oap.ospar.org/en/ospar-assessments/intermediate-assessment-2017/biodiversity-status/fish-and-food-webs/ (accessed September 9).
Otto, S. A., Kadin, M., Casini, M., Torres, M. A., and Blenckner, T. (2018). A quantitative framework for selecting and validating food web indicators. Ecol. Indic. 84, 619–631. doi: 10.1016/j.ecolind.2017.05.045
Pauly, D., and Christensen, V. (1995). Primary production required to sustain global fisheries. Nature 374, 255–257. doi: 10.1038/374255a0
Preciado, I., Arroyo, N. L., González-Irusta, J. M., López-López, L., Punzón, A., Muñoz, I., et al. (2019). Small-scale spatial variations of trawling impact on food web structure. Ecol. Indic. 98, 442–452. doi: 10.1016/j.ecolind.2018.11.024
Probst, W. N., and Stelzenmüller, V. (2015). A benchmarking and assessment framework to operationalise ecological indicators based on time series analysis. Ecol. Indic. 55, 94–106. doi: 10.1016/j.ecolind.2015.02.035
R Core Team. (2019). R: A Language and Environment for Statistical Computing. Available online at: http://www.R-project.org/ (accessed March 14, 2019).
Rombouts, I., Beaugrand, G., Fizzala, X., Gaill, F., Greenstreet, S. P. R., Lamare, S., et al. (2013). Food web indicators under the marine strategy framework directive: from complexity to simplicity? Ecol. Indic. 29, 246–254. doi: 10.1016/j.ecolind.2012.12.021
Serrat, A., Muñoz, M., and Lloret, J. (2018). Condition and reproductive strategy of the Argentina sphyraena, a cold-water species in the Mediterranean sea. Environ. Biol. Fish. 101, 1083–1096. doi: 10.1007/s10641-018-0763-x
Shephard, S., van Hal, R., de Boois, I., Birchenough, S. N. R., Foden, J., O’Connor, J., et al. (2015). Making progress towards integration of existing sampling activities to establish joint monitoring programmes in support of the MSFD. Mar. Policy 59, 105–111. doi: 10.1016/j.marpol.2015.06.004
Shin, Y.-J., Shannon, L. J., Bundy, A., Coll, M., Aydin, K., Bez, N., et al. (2010). Using indicators for evaluating, comparing, and communicating the ecological status of exploited marine ecosystems. 2. setting the scene. ICES J. Mar. Sci. 67, 692–716. doi: 10.1093/icesjms/fsp294
Taherzadeh, N., Bengfort, M., and Wirtz, K. W. (2019). A trait-based framework for explaining non-additive effects of multiple stressors on plankton communities. Front. Mar. Sci. 6:351. doi: 10.3389/fmars.2019.00351
Tam, J. C., Link, J. S., Rossberg, A. G., Rogers, S. I., Levin, P. S., Rochet, M.-J., et al. (2017). Towards ecosystem-based management: identifying operational food-web indicators for marine ecosystems. ICES J. Mar. Sci. 74, 2040–2052. doi: 10.1093/icesjms/fsw230
UK Marine Monitoring, Assessment Strategy, Uk Monitoring, and Assessment Reporting Group. (2019). Marine Online Assessment Tool: Food Webs. Available online at: https://moat.cefas.co.uk/biodiversity-food-webs-and-marine-protected-areas/food-webs/ (accessed April 18, 2021).
Vespe, M., Natale, F., Gibin, M., Alessandrini, A., Mazzarella, F., Osio, G. C., et al. (2016). AIS Derived High Resolution Fishing Effort Layer for European Trawlers of More than 15 Meters Long 2014-2015. Available online at: http://data.europa.eu/89h/jrc-fad-ais1415 (accessed April 6, 2021)
Walmsley, S., Weiss, A., Claussen, U., and Connor, D. (2017). “Guidance for assessments under article 8 of the marine strategy framework directive,” in Proceedings of the Integration of assessment results, (Brussels: DG Environment).
Keywords: ecosystem-based assessment, trophic webs, anthropogenic pressures, good environmental status, marine monitoring
Citation: Machado I, Costa JL and Cabral H (2021) Response of Food-Webs Indicators to Human Pressures, in the Scope of the Marine Strategy Framework Directive. Front. Mar. Sci. 8:699566. doi: 10.3389/fmars.2021.699566
Received: 23 April 2021; Accepted: 24 August 2021;
Published: 05 October 2021.
Edited by:
Maria Bebianno, University of Algarve, PortugalReviewed by:
R. P. Prabath K. Jayasinghe, National Aquatic Resources Research and Development Agency, Sri LankaJosé M. Rico, University of Oviedo, Spain
Copyright © 2021 Machado, Costa and Cabral. This is an open-access article distributed under the terms of the Creative Commons Attribution License (CC BY). The use, distribution or reproduction in other forums is permitted, provided the original author(s) and the copyright owner(s) are credited and that the original publication in this journal is cited, in accordance with accepted academic practice. No use, distribution or reproduction is permitted which does not comply with these terms.
*Correspondence: Inês Machado, bWltYWNoYWRvQGZjLnVsLnB0