- Nature Coast Biological Station, Institute of Food and Agricultural Sciences, University of Florida, Gainesville, FL, United States
The ecology of estuaries is shaped significantly by the extent of freshwater discharge which regulates abiotic processes and influences overall biological productivity. The Suwannee River Estuary of Florida’s Big Bend Coastline has historically been a productive and diverse estuarine ecosystem supported by significant freshwater inputs from the Suwannee River. In recent years, significant changes in land use and climatic conditions have resulted in lower discharges from the Suwannee. Our objectives were to explore the impact of freshwater inputs from the Suwannee River on the estuarine forage fish and sportfish communities downstream. We built a trophic-dynamic food web model in Ecopath with Ecosim to simulate different levels of discharge and evaluate how changes in discharge (drought and floods) would influence the trophic structure of the food web. Using the fitted model, we applied a series of different short-term and long-term flow projections under different climatic scenarios to evaluate impacts on fish functional groups and sportfish biomass. Simulations suggested that ecological production was more influenced by drought conditions than flood conditions. In our short-term scenarios, the drought simulations produced biomass changes that were approximately twice as substantial as the flood scenarios. When making comparisons to other published EwE models, we generally observed smaller changes in biomass production. Although this model focused on the influence of bottom-up effects, we observed strong top-down control of snook (Centropomus undecimalis) on the system. Several functional groups were particularly sensitive to changes in snook abundance which included spotted seatrout (Cynoscion nebulosus), sand seatrout (C. arenarius), and other members of the family Sciaenidae. Because snook have recently colonized the estuary, likely as a result of warmer winter temperatures, this finding has implications for climate change and natural resource management.
Introduction
Estuarine ecosystems are shaped significantly by the frequency and magnitude of freshwater inputs which can have an impact at the organismal level and also lead to broad ecological effects (Paerl, 2006; Baptista et al., 2010; Piazza and La Peyre, 2011). The major factors that influence discharge from riverine systems are precipitation patterns and anthropogenic extraction. Many climate change models predict significant variability in future precipitation and evapotranspiration rates, which will likely have strong impacts on riverine flow (Walther et al., 2002; Milly et al., 2005; Trenberth, 2011). Evapotranspiration rates are expected to increase with increasing future air temperatures, resulting in lower discharge rates to the estuary (Kingston et al., 2009). Globally, many rivers have experienced significant freshwater withdrawals for energy and agricultural purposes (Poff et al., 1997). These alterations often decrease the frequency and intensity of high flow events and modify overall discharge variability that is characteristic of estuarine systems downstream (Alber, 2002). Understanding how changes in river discharge will affect estuarine ecosystems is critical to management of the animal species inhabiting estuaries, with implications for conservation of ecosystem services that estuaries provide.
One way in which changes in river discharge can impact estuaries is by affecting the concentrations of limiting nutrients that support essential ecosystem functioning. Eutrophication of nitrogen and phosphorus can cause rapid growth in phytoplankton communities, leading to an increase in primary production (Livingston, 2000). This increase in primary production can stimulate growth via “bottom-up effect” (White, 1978), in which primary consumers directly benefit by grazing, while secondary and tertiary consumers benefit from greater densities of prey. While upper trophic level species can benefit from an increase in ecological production, high levels of eutrophication have frequently demonstrated to have negative effects on these same taxa. Prominent examples are evident in Chesapeake Bay and the Mississippi River Delta, where hypereutrophic events have created “dead zones” from hypoxic conditions (Rabalais et al., 2002; Kemp et al., 2005; Diaz and Rosenberg, 2008). These hypoxic conditions often kill benthic flora and fauna with subsequent effects being observed throughout the ecosystem. This is often the result of bacteria respiring at high rates when dense populations decompose large amounts of algal biomass.
One of the first taxa within the fish community to exhibit a response to bottom-up effects would likely be forage fish. They are generally defined as small-bodied fishes that constitute large prey bases for predatory fish, birds, and marine mammals (Alder et al., 2008; Pikitch et al., 2012). These species tend to occupy low trophic levels and often feed upon planktonic organisms. They are also characterized by their ecological importance of transferring energy from low to high trophic levels. Most forage fish have stochastic population dynamics due to their quick response to changes in the environment and short life spans (Pikitch et al., 2012), making them an ideal study species when analyzing the impacts of bottom-up effects on fish communities. Additionally, the nutrients associated with river discharge may significantly affect larval fish production (Grimes and Kingsford, 1996) via increased phytoplankton and zooplankton production.
Due to the complexity of species interactions in estuarine ecosystems and the spatial-temporal time scales in which river flow affects ecological processes, it is often difficult to determine the effects of river flow empirically. Food web models allow exploration of processes that can influence trophic structure of ecosystems, and they allow for large-scale hypothesis testing in ecology, a discipline where traditional experimentation is often not practical (Hilborn and Mangel, 1997). Through a system of mathematical equations, they capture a wide array of ecological mechanisms such as trophic interactions, feedbacks, and time lags in biological production. Food web models have gained increasing attention in the field of fisheries management, where a shift from single-species management to ecosystem-based fisheries management is underway (Pikitch et al., 2004). Assessing the impact of global climate change or water withdrawals across large watersheds is quite difficult due to extensive sampling required across large spatial and temporal scales, and multiple confounding factors that can shade effects of discharge changes on food web components. By changing parameterized relationships between environmental input variables and biological communities, ecologists can explore hypotheses regarding the causal impact that changes in the environment have on local flora and fauna. Fortunately for ecological modeling in estuarine systems, many of the basic physiological processes of the resident organisms are at least roughly understood to make this research possible. For example, environmental tolerances of fish to factors such as salinity and dissolved oxygen are understood sufficiently to predict biomass changes following changes in discharge. Similarly, changes in estuarine nutrient concentrations resulting from changes in discharge can be used to predict changes in primary production, and cascading effects through the food web are therefore possible to explore.
One ecosystem modeling approach that has been widely applied in marine research is the Ecopath with Ecosim (EwE) software package (Christensen and Walters, 2004). EwE models have been developed for a range of aquatic ecosystems to address issues ranging from fisheries management, invasive species, climate change, eutrophication, ecological restoration, and environmental impact analysis (Walters, 2000; Martell et al., 2002; Chagaris et al., 2015, 2017; Colléter et al., 2015). EwE is particularly useful to study the ecosystem effects of freshwater flow in estuaries because it incorporates a basic nutrient uptake model with detailed trophic processes to assess impacts across the entire food web. With respect to estuarine ecosystems, EwE models have been developed to analyze the impact of river diversions on estuarine fish in the Gulf of Mexico (de Mutsert et al., 2012; de Mutsert et al., 2017) and other estuaries (Townsend, 2014; Ihde and Townsend, 2017; Sakamoto and Shirakihara, 2017; Smith et al., 2020). Thus, EwE is a common tool used to develop and test hypotheses relating to freshwater inputs and eutrophication.
The Suwannee River Estuary is a unique case study of an ecosystem experiencing the impacts of both climate and land use. The estuary is located along Florida’s Big Bend coastline, approximately 180km north of Tampa Bay. The system is supported by one of the largest undammed rivers in the eastern United States (Benke, 1990) and in recent decades, the frequency of drought conditions has increased dramatically (Seavey et al., 2011). From 1995 to 2008, drought conditions occurred on average about 4.23 months/year compared to 0.42 months/year from 1942 to 1995 (Seavey et al., 2011). Seavey et al. (2011) demonstrated that Suwannee River discharge has declined per amount of rainfall, suggesting some impacts of water withdrawals in the system. Since 1975, human population growth in the region has been considerable and the impacts of this population growth may be seen in the increase in irrigation intensive agricultural (see Marella et al., 2016). Regarding climatic impacts, changes in both precipitation and evapotranspiration have been projected for the area, which will likely impact discharge (Swain and Davis, 2016; Neupane et al., 2019). Swain and Davis (2016) estimated a ∼5% increase in evapotranspiration between the years 2039 and 2069, and Neupane et al. (2019) forecasted a 12% increase for the 2080s under a high emissions scenario. The latter authors also estimated a decrease of 13% in mean discharge for the Suwannee with a 25.1% decrease during summer when anthropogenic water demands are high.
These projected changes in discharge are very likely to impact the fish communities of the Suwannee River Estuary. Fish recruitment, diversity, and overall productivity are all tied to riverine discharge (Peebles, 2002; Sosa-López et al., 2007; Gillson, 2011). It is likely that these metrics of fish ecology would be negatively affected by reductions in discharge. Drought conditions have already been hypothesized to have negatively impacted local oyster (Crassostrea virginica) populations (Seavey et al., 2011). Oysters are not only a commercially important and culturally iconic fishery within the region, but they are ecosystem engineers that provide critical habitat for finfish, crustaceans, and other invertebrates (Grabowski and Peterson, 2007; Beck et al., 2011).
This study developed an ecosystem model to explore how different discharge scenarios and associated nutrient concentrations will impact the estuarine ecosystem. Our analysis focused on how forage fish and recreationally important fish species will be impacted through trophic interactions that are altered with changes in river discharge. We hypothesized that changes in discharge and nutrients within the estuary will have ecosystem-wide effects on fish communities. Drought conditions may lead to overall less production of biomass, while flood conditions may cause an increase in primary production that will positively impact fish biomass through bottom-up effects. The Suwannee River is an ideal study site for research on the impact of discharge on estuarine ecosystems because of its natural flow regime (Benke, 1990; Franklin et al., 1995). This allows scientists to understand the natural ecosystem dynamics that occur between riverine and estuarine systems as well as the general watershed.
Materials and Methods
Site Description and Data Availability
The Suwannee River Estuary is located in the Big Bend region of Florida’s Gulf Coast (29.08°N – 29.3°N; 83.01°W – 83.25°W) (Figure 1). The Suwannee River flows 396km unobstructed from the Okefenokee Swamp in southern Georgia to the Gulf of Mexico. From 2015 to 2018, mean annual discharge in the river was 7,954cfs (United States Geological Survey, 2020). This estuary is characterized by a relatively undeveloped shoreline and watershed with extensive seagrass beds and salt marsh habitat. Monthly mean water temperatures range from 14°C (January) to 30°C (August) with an average depth in the estuary of approximately 3.10 m (Frazer, 2018). Salinity levels vary substantially with peaks during winter (25.12psu, December) and a minimum during early spring (18.54psu, March). Most of the fish community is constituted of temperate/subtropical, estuarine species such as bay anchovies (Anchoa mitchilli), spot (Leiostomus xanthurus), spotted seatrout (Cynoscion nebulosus), and red drum (Sciaenops ocellatus). Marine transients (e.g., scombrids) are occasionally present in more saline portions of the estuary, while freshwater species such as gars (Lepisosteus spp.) and sunfish (Centrarchidae) are generally found near the mouth of the river and upstream. Species of snapper (Lutjanidae) and grouper (Serranidae) inhabit the estuary as juveniles but then move offshore as they become older. In recent years, warmer temperatures have allowed black and red mangrove (Avicennia germinans and Rhizophora mangle) populations to expand northward and now compete with marsh grass along shorelines. The warmer temperatures and increase in mangrove habitat have been implicated in the expansion of common snook (Centropomus undecimalis) into the region (Osland et al., 2013; Purtlebaugh et al., 2020). Snook are important predatory gamefish in Florida that utilize mangrove habitat extensively and prey upon and/or compete with other sportfish.
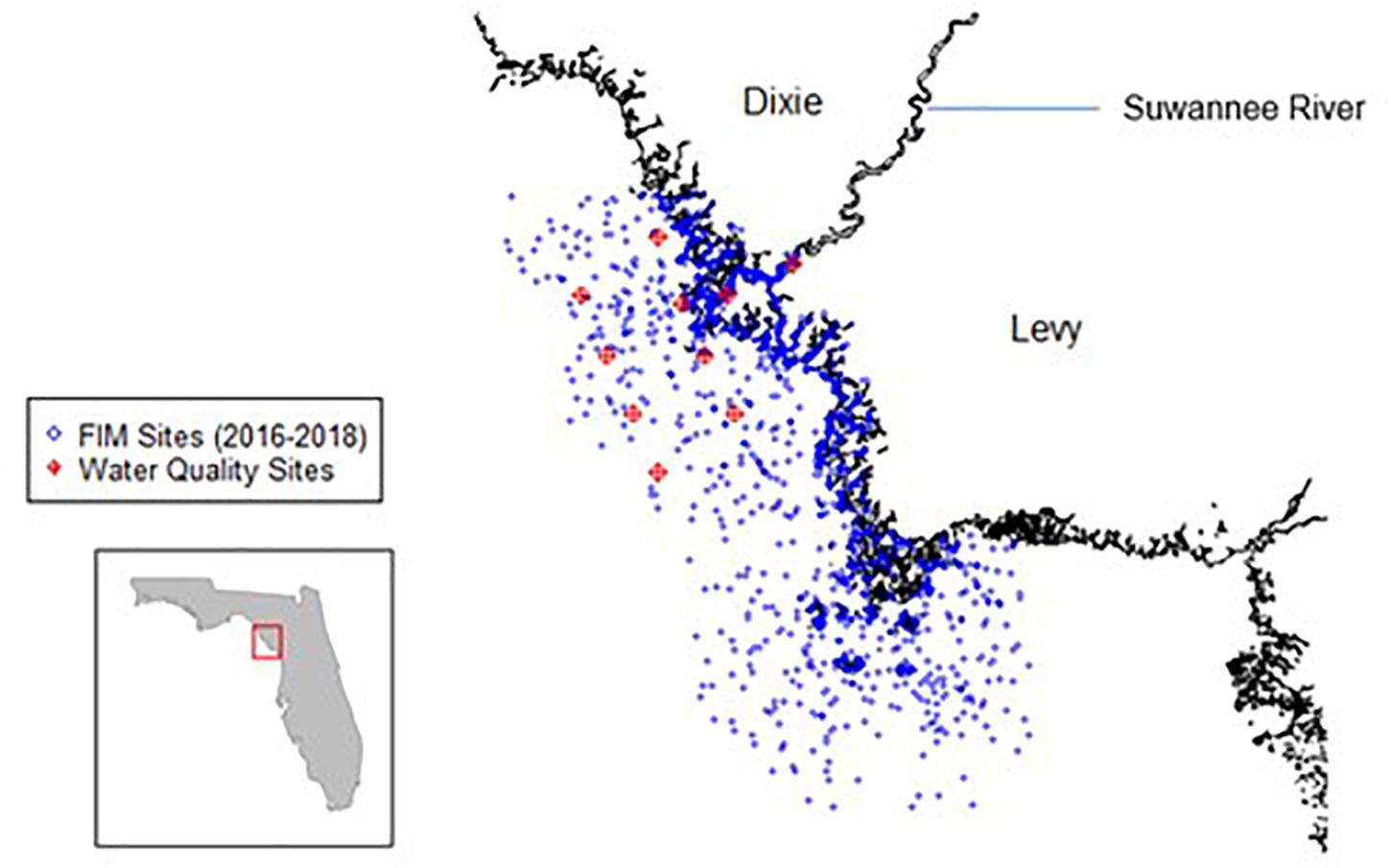
Figure 1. Map of the Suwannee River Estuary. FIM sampling locations from 2016 to 2018 are in blue, and Project COAST water quality sites are in red (Florida Fish and Wildlife Conservation Commission, 2021; Frazer, 2018).
Our model relied heavily on a long-term dataset on fish and macroinvertebrate abundances collected by the Florida Fish and Wildlife Conservation Commission’s (FWC) Fisheries Independent Monitoring (FIM) Program, which has operated a monitoring program in Cedar Key since 1996. The FIM program conducts monthly, multi-gear, stratified random sampling in the estuary. A 21.3 m seine with a mesh size of 3mm was utilized for collecting fish less than 150 mm standard length (SL) along and near the shoreline in waters generally less than 1.0 m in depth. A 183-m seine with a mesh size of 38-mm was deployed along shorelines for collecting larger bodied fish in depths less 2.5 m. Offshore seagrass habitat was sampled by means of a 6.1 m wide otter trawl. Approximately thirty small seine hauls were taken each month along with fifteen hauls from the otter trawl and large seine, respectively. Over the course of this 20+ year survey, approximately 300 different nekton species have been collected.
In addition to the fisheries independent data, water quality has been monitored monthly since 1997 by the Project COAST program at ten fixed sites distributed throughout the estuary, providing measurements of nitrogen and phosphorus concentrations, dissolved oxygen, and chlorophyll-a (Frazer, 2018). Discharge from the Suwannee River has been measured continuously since 1941 by the United States Geological Survey at the Wilcox Station, located approximately 40km upstream from the river mouth (United States Geological Survey, 2020). Seagrass density and coverage were measured in 2009 by the Big Bend Seagrass Aquatic Preserve (Florida Department of Environmental Protection, 2018). Lastly, recreational landings and effort information from this region have been collected since 1981 through National Oceanic and Atmospheric Association Marine Recreational Information Program (MRIP) creel surveys (National Oceanic and Atmospheric Administration, 2018).
Ecopath With Ecosim
The Suwannee River Estuary Model (SREM) was built using Ecopath with Ecosim in order to understand and predict how the system may respond to changes in freshwater flow. The Ecopath component is a static snapshot of the food web that links biomass pools through equations based on the production and consumption rates and describes the initial state for the time-dynamic component, Ecosim. Building a balanced Ecopath model first consists of identifying the functional groups of the model ecosystem. Functional groups are groups of species with similar characteristics and range from primary producers to high trophic level predators. The basic input requirements for Ecopath are biomass densities, consumption rates, mortality rates, and diet composition for each functional group. The functional groups must have these parameters in balance whereby the energy leaving a group cannot be greater than that which enters the group. Ecotrophic efficiency (EE) is a term unique to Ecopath defined as the “proportion of the production that is utilized in the system” (Christensen and Walters, 2004), and values less than 1.0 satisfy the condition of mass balance.
Ecosim is a time dynamic simulation module that is generally used to test the effects of implementing new harvest policies or changes in the environment. Ecosim uses a set of differential equations to model biomass fluxes over time. One of the main principles in Ecosim that is used to model consumption rates is foraging arena theory. This theory states that prey items must balance both their time and location in space to minimize risk to predation and maximize feeding opportunities (Walters and Martell, 2004; Ahrens et al., 2012). In EwE, the vulnerability parameters define the rate at which biomass pools move into and out of states of foraging where they are vulnerable to predation. High levels of vulnerability signify that if predator biomass were to increase, a high amount of predation would occur. Low vulnerabilities represent a scenario where predator biomass increased and there was not a significant change in predation mortality. We used forcing functions to influence rates of primary production and foraging arena parameters. In Ecosim, nutrients are apportioned either to biomass pools or free nutrients. These free nutrients are available for uptake by primary producers and balanced by inflow rates and outflow rates of nutrients for the system. Interaction rates of primary producers with free nutrients are modeled by means of a Michaelis-Menten response, whereby uptake asymptotically increases with increases in nutrient concentrations (Christensen et al., 2005).
The Suwannee River Estuary Model
Ecopath Parameterization
Of the approximately 300 species collected by the FIM program, those that occurred in more than one percent of hauls in all gear types of the FIM data or at least five percent of hauls in one gear type were selected for inclusion. A total of eighty-five different fish and invertebrate species met these criteria. Species were categorized into functional groups based on similar biological characteristics such as diet, habitat, and taxonomy using hierarchical clustering. In Ecopath, fifty-nine functional groups with thirty-seven fish groups (Table 1 and Supplementary Material) were created. Regarding fish groups, species of particular research interest and commercial importance were broken into age stanzas, which allowed for a more detailed life history analysis. These species included snook (0, 1 – 4, 4+ years old), spotted seatrout (Cynoscion nebulosus) (0, 1 – 2.5, 2.5+ years old), red drum (Sciaenops ocellatus) (0, 1 – 4, 4+ years old), and mullet (Mugil spp.) (0, 1+ years old). The sixteen invertebrate groups ranged from commercially valued blue crabs (Callinectes sapidus) and shrimp (Farfantepenaeus spp.) to benthic decapods, small crustaceans, infauna, and several zooplanktonic groups. Primary producers included macroalgae, seagrass, phytoplankton, and microphytobenthos.
Biomass densities (grams/meter2) for the fish groups were estimated from FIM samples collected during the Ecopath base year of 1997. The numerical densities (number/m2) at each site were first converted to biomass densities using length composition data of the catch along with length-weight relationships. Biomass density inputs were calculated by averaging across the three gear types. Due to differences in gear selectivity, catchability coefficients were assigned to functional groups for different gear types, with a baseline value assumed, initially, to be 0.50. Because some functional groups demonstrated little to no representation in certain gear types, these gears were excluded from the density calculation of those groups. For the adult snook age stanza, we did not use a biomass density from 1997, as the species was not observed in the estuary at that time. Instead, we input a value from 2007, which was the first year the species was consistently observed, although at minimal densities. Consumption and mortality parameters for finfish were obtained from fishbase, literature reviews, and stock assessments (Froese and Pauly, 2020). For functional groups with multiple species, the consumption and mortality rate inputs of the group were calculated by averaging over all species within the group.
Because we did not have estimates of biomass densities for invertebrate groups, we allowed the model to solve for biomass by inputting the consumption, mortality, and ecotrophic efficiency parameters. These parameter values were taken from an Ecopath model of the West Florida Shelf (Chagaris et al., 2015). Because biomass densities of detritus were also not measured directly in the estuary, these values were also estimated from another EwE model, which was built for the Tampa Bay Estuary (Chagaris and Mahmoudi, 2009). We estimated phytoplankton biomass from Project COAST data by using relationships between chlorophyll-a and depth developed by Morel and Berthon (1989). Seagrass biomass data were collected by the Big Bend Seagrass Aquatic Preserve.
Diet compositions of fish were derived using data collected from FIM as well as other studies throughout the Gulf of Mexico. The diets of the thirty-seven finfish functional groups were assessed from a variety of locations in the Gulf of Mexico but with the majority of samples taken from Tampa Bay and the West Florida Shelf. For the three age-stanzas of snook, site specific diet compositions were obtained for the Suwannee Estuary. The individual prey items of each predator were assigned to the aforementioned functional groups, which created a diet matrix that would be directly input into Ecopath. Diet compositions for invertebrate species were assembled from Ecopath models built for Tampa Bay and the West Florida Shelf (Chagaris and Mahmoudi, 2009; Chagaris et al., 2015).
Four fishing fleets were created which included private and charter recreational fleets in addition to directed recreational red drum and spotted seatrout fisheries. This was essential, as directed trips for seatrout and red drum were highly seasonal and did not follow the overall trend of recreational effort in the region. This allowed the model to make better predictions of seatrout and red drum catch as a function of directed effort. Initial recreational landings were estimated from the MRIP data for 1997 in Levy County, as well as Citrus County to the south and Dixie County to the north (Figure 1). The aggregate landings were divided by the estimated habitat area of this three-county region. Lastly, discards were estimated from MRIP and input into Ecopath, where a 10% mortality rate was assumed. This was informed by a meta-analysis by Bartholomew and Bohnsack (2005), which assessed discard mortality rates in a variety of recreational fisheries.
The model was then mass-balanced following the protocols developed by Link (2010) regarding prebalancing diagnostics. This approach included having biomass across trophic levels range between five to seven orders of magnitude, predator biomass less than prey biomass, and consumption rates less than production rates for functional groups. To achieve mass-balance, we began by making adjustments to the catchability coefficients of the FIM data (previously assumed to be 0.5) to address for any gear selectivity biases in our biomass estimates. In several cases, diet compositions were also adjusted to reduce predation mortality, as these data generally had a high degree of uncertainty.
Ecosim Parameterization
The Ecosim analysis sought to fit model predictions to time series of relative abundance for various model trophic groups. We began by assembling a reference time series of biomass and landings estimates, as well as fishing effort and environmental forcing time series. Annual indices of abundance for fish functional groups were estimated from the FIM data using delta generalized linear models (GLM) (Lo et al., 1992; Maunder and Punt, 2004). The use of GLM’s was necessary to standardize across gears and habitats, as some species were more adequately sampled in certain habitats, months, or with certain gears, as opposed to other species. In delta GLM’s, a model of log-normal values of biomass densities from positive sets was first fitted, followed by a binomial model for the probability of occurrence. The product of the least squared means for each year from the two models was taken as the index. The predictor variables in both the log normal and binomial models included bottom and shoreline habitat types, bottom vegetation, gear type, salinity, temperature, dissolved oxygen, month, year, and location. Models were then compared and selected based on lowest AIC value (Akaike, 1974). Time series of recreational landings from 1997 to 2018 were developed from the MRIP data for each functional group, including dead discards. The FIM relative abundance indices and MRIP landings time series were used as reference data during the Ecosim calibration procedure. To weight each time series, we first evaluated the average annual coefficient of variation (CV) from the index standardization process. Then we subsequently utilized the reciprocal of the CV as the weight for each time series.
Several different environmental forcing time series were used in Ecosim to explain ecological processes within the estuary. Monthly Suwannee River discharge and nutrient concentrations were used to explain variable primary production rates. For the snook population increase, a general environmental forcing function, described by an increase in minimum temperatures and increasing mangrove habitat, was used to fit the population data. The nutrient time series included total nitrogen and phosphorus concentrations (μg/L) averaged over all sites within each month from the Project COAST and Lakewatch data. Months without nutrient data were interpolated from discharge values of the Suwannee River. Discharge was also collected at monthly intervals from 1997 to 2018 from the USGS Wilcox Station. Fitting the model with nutrients and discharge was done by using the nutrient loading forcing function application of Ecosim and setting the base proportion of free nutrients to 0.6. The snook environmental forcing function was applied to the search rate parameter for snook and allowed for an increase in consumption and subsequent biomass growth as environmental conditions approached optimum conditions. Regarding fishing effort, times series of private and charter trips, as well as targeted trips for spotted seatrout and red drum, were assembled from the MRIP data to drive fishing mortality on harvested species.
The Ecosim model was then fitted to the reference time series of biomass and catch by estimating the vulnerability parameters of each functional group with the objective of minimizing the model’s sum of squares between predicted and observed values. Sixteen model configurations were tested to achieve the best fit (Table 2). We tested scenarios based on fitting the model with the historic forcing time series of discharge as well as with the nutrient concentration time series. Also, due to the variable nature of estuaries and freshwater discharge, we utilized primary production anomalies, which allowed for better prediction of bottom-up processes that drive ecosystem-wide changes in fish and invertebrate biomass. These primary production anomalies were applied to four photosynthetic functional groups. The anomalies were estimated by the model after estimating the vulnerability parameters. In addition to forcing the model with environmental parameters, we also tested scenarios based on forcing the catch of select taxa. This was done for functional groups that had particularly variable catch time series that did not correlate well with the overall effort estimates and included small sharks, Spanish mackerel (Scomberomorus maculatus), other sciaenids, other demersals, and Gulf flounder (Paralichthys albiguttata). Lastly, we tested various prey switching scenarios to further reduce the sum of squares. For each configuration, the model was fitted by repeating the vulnerability search seven times. On each search, the most sensitive parameters were identified and then estimated to reduce the total sum of squares (Christensen et al., 2005). Seven searches were conducted because at this number the sum of squares was no longer reduced. On each search, fifty-seven vulnerability parameters were estimated based on the availability of reference biomass and catch time series. The first three searches were conducted while placing a high weighting on the snook time series in order to force the model to fit to snook. The last four runs were done while removing the heavy weighting on snook. We originally upweighted this time series to capture the exponential increase in snook biomass, which has been observed in the estuary. This would ensure that this potential top-down driver was present in the system. High snook weights were created by multiplying the highest weight in the model by approximately three orders of magnitude. Snook are an important sportfish in the region and also a species of research interest.
Projecting Future Flow Scenarios
Two sets of future flow projections, short-term and long-term, were made to understand the impact of discharge on fish biomass. We explored the ecological impacts of the future discharge projections made by Neupane et al. (2019) on the Suwannee River Estuary using Ecopath with Ecosim. In addition to the long-term climate projections from Neupane et al. (2019), a series of short-term (three-year) flow scenarios was tested to determine the impact of anomalous flood and drought conditions.
Short-Term Synthetic Streamflow Simulations
The short-term projections were designed to simulate three consecutive years of wet, dry, and normal flow as well as three years of high and low variance in discharge (Figure 2). The construction of short-term flow scenarios began with a multiplicative decomposition of the monthly historic time series. By decomposing a time series, seasonal, trend, and random components are partitioned in order to isolate the analysis on a targeted pattern. Properties such as seasonality can be removed, which reveals the overall trend on the time series. This is critical when determining the impact of flow on fish biomass, as seasonality is a confounding variable for both discharge and densities of many fish species. For the random component of the time series, the mean and standard deviation were calculated. Following the decomposition, autoregressive integrated moving average (ARIMA) models were fitted to the trend and random components in order to obtain the autocorrelation structure for use in the synthetic projections. These models are frequently used in time series analysis to forecast stationary data. Wet and dry years were identified as years that were ± 1 standard deviation of the mean annual discharge. Monthly averages were determined for these wet, normal, and dry years and subsequently scaled to a mean of one for each flow type. Short term (three year) flow scenarios were created by simulating thirty time series for each flow type using the fitted ARIMA models. Random time series of mean monthly flow were simulated using the mean and standard deviation from the ARIMA model of each flow type. Subsequently, the random component was simulated using the mean and standard deviation from the random component. Lastly, the synthetic flow projections were created by recomposing the multiplicative time series. This was done by multiplying the trend by the seasonal component and random component. High and low variability projections were made by repeating the previous steps while also increasing and decreasing the standard deviation of the random component by 30%. Lastly, we converted the discharge levels to nutrient concentrations based on an estimated logarithmic relationship between discharge and total nutrients, where:
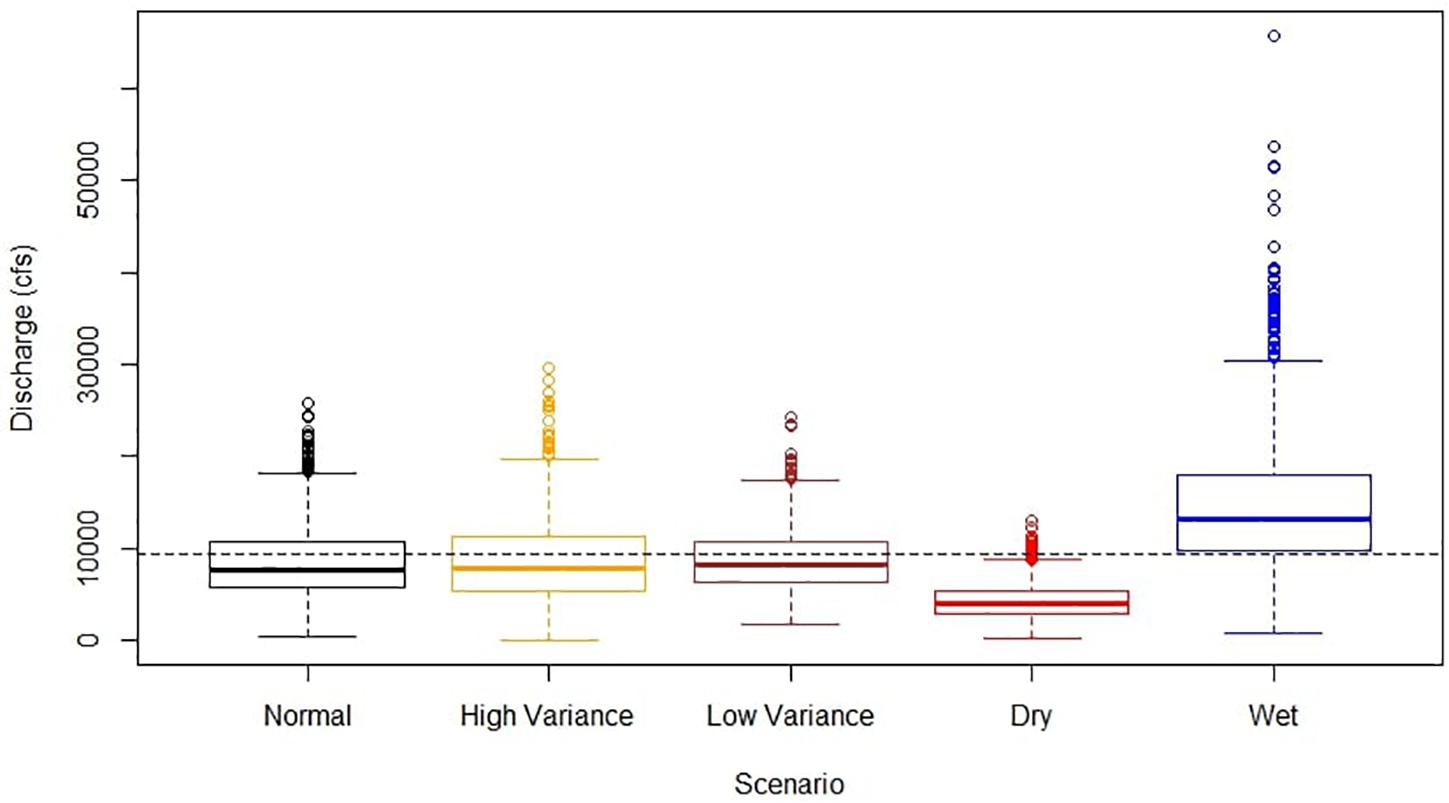
Figure 2. Projected monthly discharge from the short-term simulations. High and low variance scenarios were created by multiplying the random component of these simulations by 30%. Dry and wet scenarios were created by adding or subtracting mean discharge by one standard deviation.
This relationship was developed using the water quality data, which did not show a particularly high level of correlation between nutrients and discharge (R2 = 0.323).
Long-Term Climate Scenarios
Long-term climate projections were run to determine how changes in climate would impact discharge and subsequently bottom-up effects upon the estuarine ecosystem (Figure 3). We simulated the equilibrium response in fish biomass to freshwater discharge levels predicted under four different climate scenarios. These projections were informed by a previous study that evaluated climate change in watersheds of the Southeastern United States, one of which was the Suwannee watershed (Neupane et al., 2019). The authors forecasted a range of potential changes in Suwannee River discharge from a series of different climatic scenarios. These included projections for the 2050s and 2080s under low (RCP4) and high emission (RCP8) conditions (Table 3). In these scenarios, mean discharge ranged from 8,067 cfs to 9,454 cfs, while standard deviations varied between 4539 and 4978. Like the short-term projections, thirty different simulations were run, whereby discharge was multiplied by a random component that was assessed in the ARIMA model. The authors did not provide nutrient projections associated with discharge, and therefore the conversion applied in the short-term projections was also applied here.
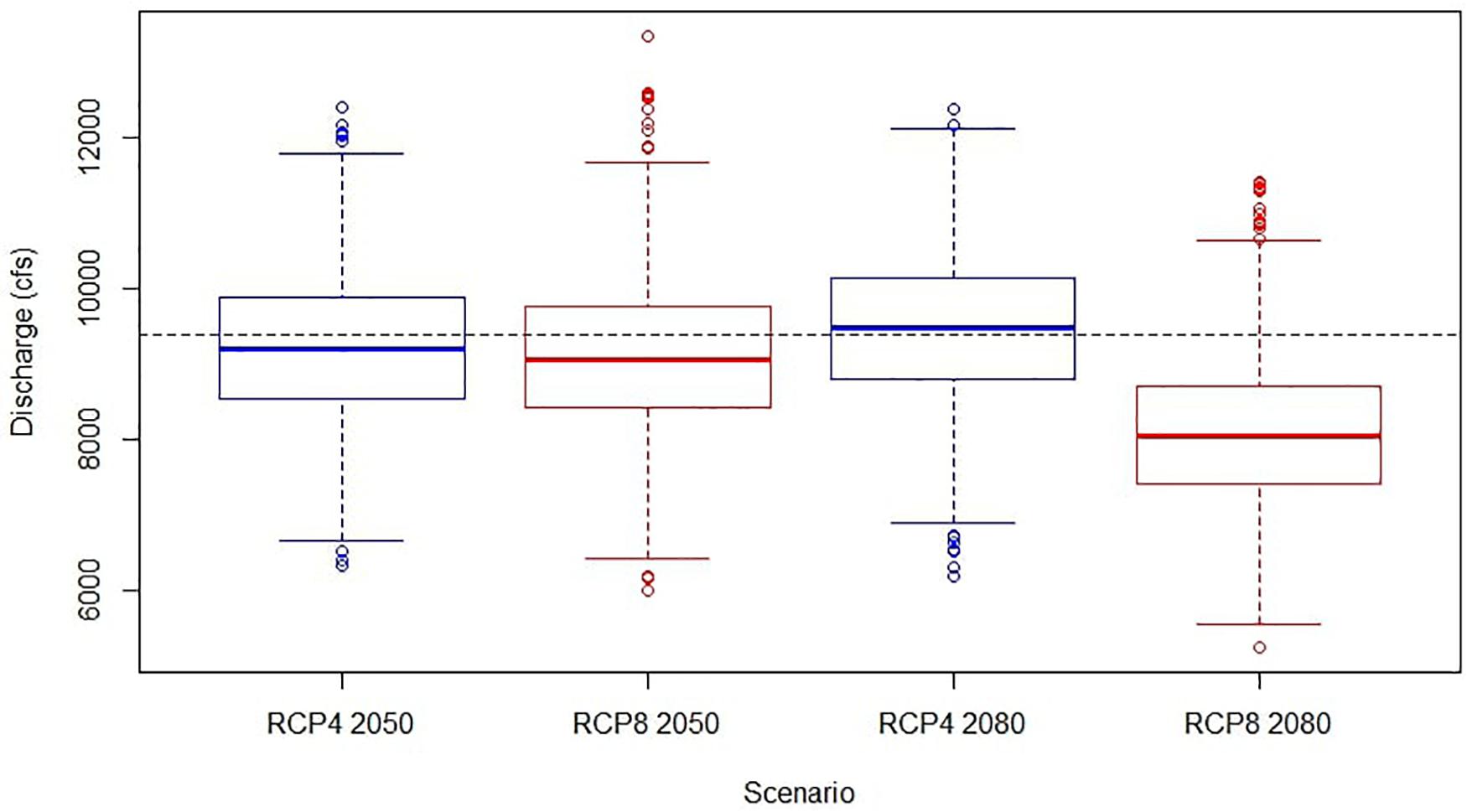
Figure 3. Mean annual discharge from the projected long-term simulations. Similar ranges of discharge are demonstrated for RCP 4/8 2050 and RCP 4 2080. Projections in RCP 8 2080 are noticeably lower, where a 13% decrease in mean discharge was observed from the current condition.
For all four climate scenarios, projections were run for fifty years, from the years 2019 to 2068. Because biomass of fish functional groups generally reached an equilibrium level after thirty years, the mean biomass of each group was taken for the last twenty years of each simulation. Relative changes in biomass were assessed with respect to a fifty-year status-quo projection under 2018 conditions of nutrient concentrations to determine the impact of nutrient enrichment on biomass. In the status quo projection, the snook environmental forcing function was held constant at a reduced level that limited further increases in snook biomass.
Results
Forage Fish Biomass and Transfer Efficiency
Our balanced Ecopath model showed expected levels of forage fish biomass, and the model explained most of the biomass for major forage groups. Fish functional groups with the highest biomass included adult mullet (4.79 g/m2), anchovies (Anchoa spp.) (1.43 g/m2), and pinfish (Lagodon rhomboides) (0.92 g/m2), while the lowest biomasses were observed in mojarra (Eucinostomus spp.) (0.12 g/m2), juvenile mullet (0.19 g/m2), and killifish (0.23 g/m2) (Table 1). Forage fish also tended to have the highest predation mortalities and ecotrophic efficiencies. These groups included pinfish, clupeids, killifish, and mojarra with ecotrophic efficiencies of 0.955, 0.985, 0.902, and 0.981, respectively (Table 1). Groups with low ecotrophic efficiencies and low percentages of biomass lost to predation included silversides and anchovies. These taxa demonstrated ecotrophic efficiencies of 0.343 and 0.367, respectively (Table 1).
We calculated total throughput in Ecopath, which is defined as the sum of all flows, including losses to predation, export, detritus, and respiration (Christensen et al., 2005). Species that lost the highest amount of biomass to predation included killifish and pinfish, which transferred 14 and 12% of their biomass to predators. Juvenile mullet and silversides lost low percentages of biomass to predation at only 1 and 2%, respectively (Figure 4). Adult mullet did not transfer any biomass to predators, as we assumed that all predation on mullet was on the juvenile stanza (Figure 4).
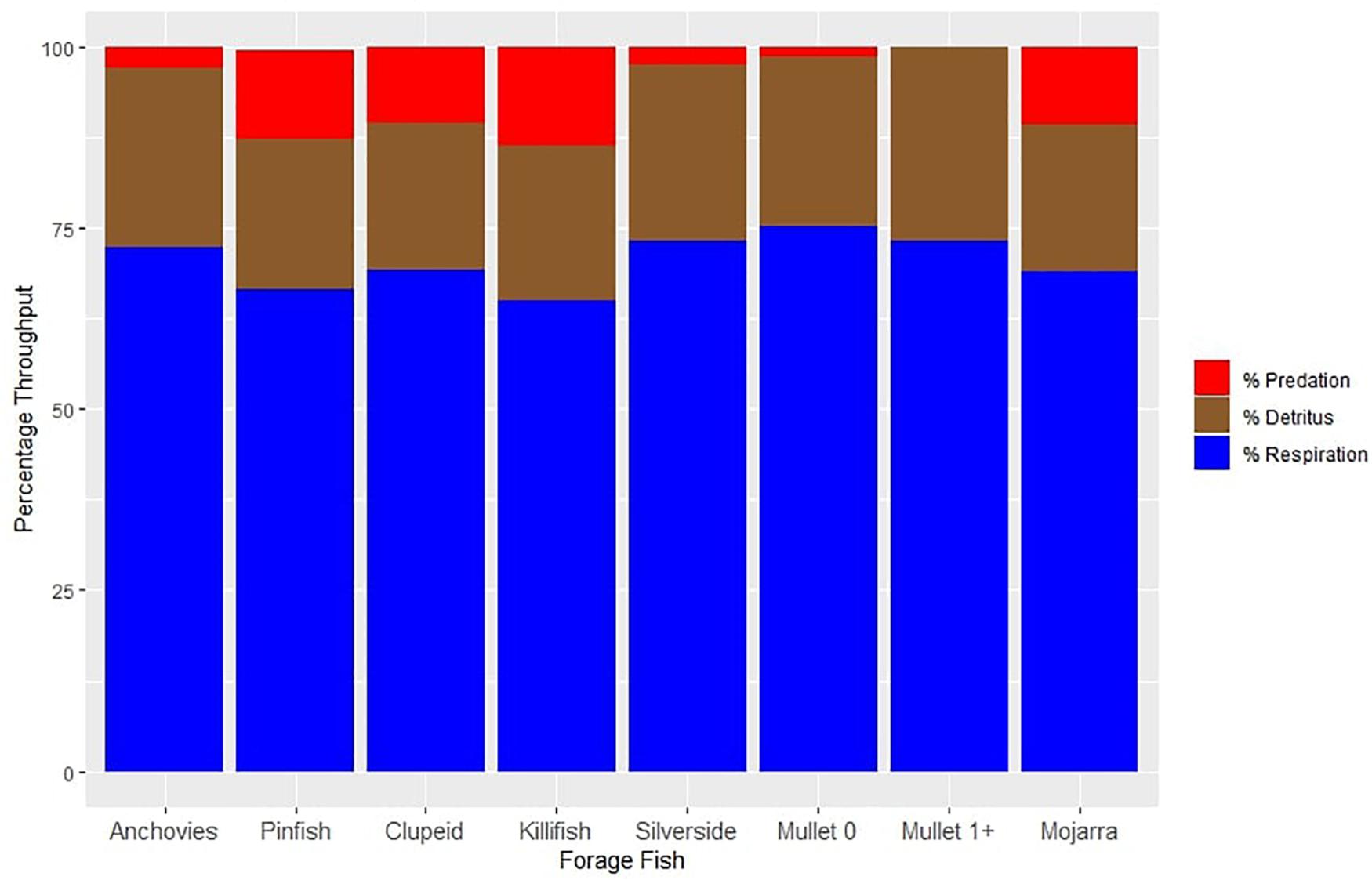
Figure 4. Percent throughput of forage fish taxa. This describes the different fates of biomass for each functional group. Biomass is either lost to predation, decomposes to detritus, or is metabolized through respiration. Values are presented as percentages of total biomass.
Ecosim Model Calibration
While each of the sixteen model runs may be considered as a valid hypothesis, Run 15 was considered the base run for use in the future flow simulations because it demonstrated the best fit based on the sum of squares (Table 2). We consistently observed that fitting the model with nutrients produced better model fits than using discharge as the main forcing function (Table 2 and Figure 5). The sum of squares (SS) values ranged from 1635 for Run 3 to an SS of 794 for Run 15. Regarding prey switching, we generally observed that a prey switching value of 1.0 set for all predators produced stronger model fits. A prey switching value of 1.0 allows predators to switch to more abundant prey items when their primary sources of prey become moderately scarce (Christensen et al., 2005). The latter scenario, Run 15, was considered the base run for use in the future flow simulations (Table 2).
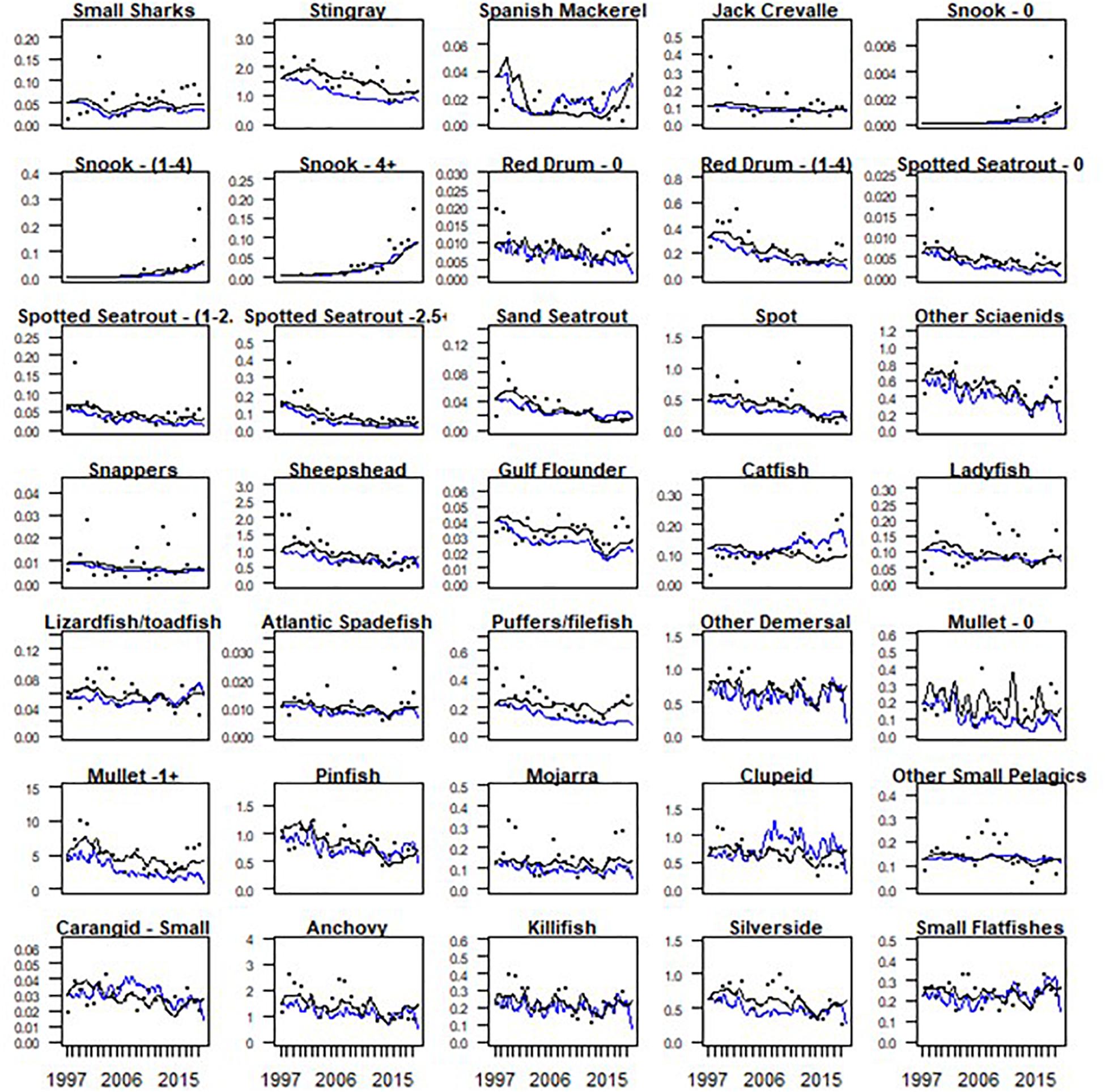
Figure 5. Model fits of all finfish. Black line represents model fits for the nutrient scenario. Blue line represents model fits for the discharge scenario.
Model fits varied for different functional groups and when utilizing different environmental forcing functions. We frequently observed that when primary production was forced by discharge, the model underpredicted fish biomass. This phenomenon was particularly relevant for stingrays (Mylobatiformes) and Gulf flounder, which had strong fits under the nutrient scenario, but relatively poor fits when forced with discharge (Figure 5). Strong fits were also observed for many forage fish under the nutrient scenario which included pinfish, killifish, anchovies, and adult mullet. Poor fits that were generally stable without and did not pick up on interannual variability included ladyfish (Elops saurus), snapper (Lutjanus griseus), and jack crevalle (Caranx hippos).
Short-Term Streamflow Projections
Similar overall trends were observed for both sportfish and forage fish in the short-term scenarios, with dry years resulting in lower biomass and wet years generally leading to higher overall biomass. However, forage fish exhibited larger percent changes than sportfish in all short-term scenarios (Figure 6). A common observation throughout the short and long-term scenarios was that drought conditions generally had larger impacts on biological productivity than flood conditions. Short-term dry scenarios consistently produced the largest percent changes in total biomass of forage fish and sportfish, averaging decreases of 17 and 14%, respectively (Table 3). The forage fish species with the greatest mean decline in biomass after three consecutive dry years included adult mullet (20%), pinfish (17%), and anchovies (16%), while the sportfish with the greatest declines were sand seatrout, mid-age stanza spotted seatrout and sheepshead (Figure 6). For recreational species, the largest percent decrease in any short-term simulation run was observed with sand seatrout in the dry scenario, whereby this group demonstrated a decrease of 26% (Figure 6). Of the forage species, adult mullet demonstrated the largest decrease of 26% in a dry scenario run as well (Figure 6). Although the wet and dry scenarios were both one standard deviation from normal discharge conditions, the biomass changes in the wet scenario were noticeably less. Biomass of both forage and sportfish increased only 9 and 7%, respectively in the wet scenario, which was approximately half of the change observed in the dry scenario (Table 3). For both sportfish and forage fish, the three most sensitive functional groups in the dry scenarios were also the most sensitive taxa in the wet scenarios. Of the sportfish taxa, the most substantial increase in any wet scenario run was a 15% change exhibited by sand seatrout (Figure 6). Certain functional groups exhibited patterns of resistance across scenarios as well. Jack crevalle and snappers demonstrated the most resistance of the sportfish species (−8 – +5%), while mojarra and juvenile mullet were consistently the most resistant forage fish in these short-term climate scenarios (−10 – +4%) (Figure 6).
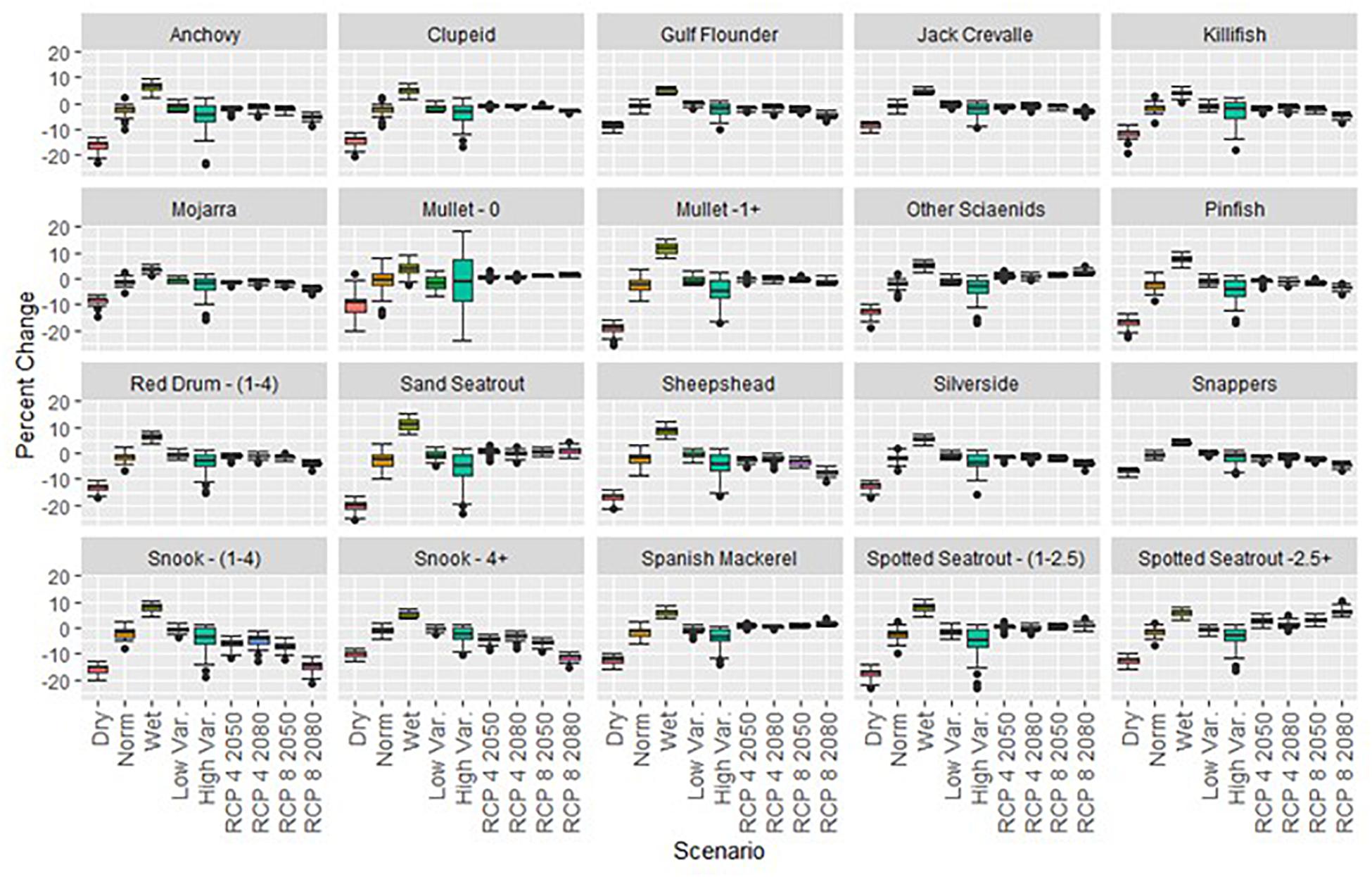
Figure 6. Changes in biomass densities for all fish groups. Functional groups tended to exhibit greater biomass changes in dry scenarios than flood conditions. Values are presented as percent changes from status quo conditions.
Although changes in mean discharge were not implemented in the high and low variance scenarios, fish biomass did decrease in both scenarios and to different extents. The high variance simulation demonstrated larger decreases in mean sportfish and forage fish biomass at 4 and 5%, respectively compared to the low variance conditions where biomass of these groups exhibited decreases of 0.65 and 0.83% (Table 3). This may indicate the greater sensitivity of ecological production to drought conditions as opposed to flood conditions. The most and least resistant groups of the high and low variance simulations were similar to the short-term climate scenarios. Also, the maximum declines observed in the high variance scenario were analogous to the declines of the dry scenario, which demonstrates that changes in both the mean and variability of discharge can have strong ecological impacts.
Long-Term Climate Scenarios
Like the short-term projections, biomass trends of forage fish and sportfish were similar across all long-term scenarios. A noticeable difference to the short-term projections was that recreationally caught fish demonstrated less resistance than forage fish (Table 3). The long-term scenarios generally demonstrated minimal decreases in biomass for both forage and sportfish, ranging from one to five percent (Table 3). The largest changes were exhibited in the high emissions scenarios, RCP 8 2050s and RCP 8 2080s (Table 3). In particular, the RCP 8 2080s conditions produced the largest decreases in biomass, where mean discharge was predicted to decrease most substantially (13% decrease). This is consistent with our hypothesis that an increase in the intensity of droughts would have greater negative impacts on fish biomass.
For individual function groups, the most substantial changes of any runs were generally observed in the RCP 8 2080s simulations. Of the thirty projections from this scenario, the largest increase was observed in the oldest spotted seatrout stanza (11%), while the largest decrease was represented by mid-age stanza snook (21%) (Figure 6). In the forage fish groups, the most substantial biomass change of any run in RCP 8 2080 was a 9% decrease observed for anchovies (Figure 6). In all long-term scenarios, we consistently observed that adult and mid-age stanza snook were the most sensitive groups to changes in discharge. For these groups, mean biomass changes ranged from a 3% decrease for adult snook in RCP 4 2080 to a 15% decrease for mid-age stanza snook in RCP 8 2080.
In general, long-term scenarios showed that a cluster of sportfish species consistently demonstrated decreases in biomass, while five groups exhibited increases. The groups that demonstrated decreases in biomass included both snook stanzas, sheepshead, snapper, Gulf flounder, red drum ages 1–4, and jack crevalle, while the groups that experienced population increases were both spotted seatrout stanzas, sand seatrout, Spanish mackerel, and other sciaenids (Figure 6). All biomass changes were relative to a status quo condition that assumed continued increases in snook biomass, which explains why snook exhibited relative population decreases even though they were forced to increase.
Equilibrium biomass for forage fish groups were generally stable across long-term scenarios. Anchovies and killifish were consistently the two most sensitive taxa to perturbances. The largest biomass changes of these groups were observed in the RCP 8 2080s scenario, whereby mean anchovy and killifish biomass both decreased 5% (Figure 6). Both mullet stanzas exhibited the most resistance of all the forage groups, as mean biomass of these groups changed less than 1.0% in three of the four scenarios (Figure 6).
When comparing the ratio of aggregated forage fish biomass relative to sportfish, this value was considerably lower in all long-term scenarios than in the short-term projections. For the short-term scenarios, the mean F/R ratio was 3.6, whereas in the long-term scenarios, the average value was 3.1. A decrease in this ratio depicts a decrease in the amount of available prey relative to predatory sportfish populations. The observed decrease in the ratio likely relates to the increase in snook biomass that continues beyond the three years of the short-term simulations. The F/R ratio was also greater in the short-term wet simulation than in the short-term dry scenario, where mean values were 3.7 and 3.5, respectively.
Discussion
Impact of Droughts and Floods
Our analysis indicated that drought conditions had a greater impact on ecological production than flood conditions. Droughts caused a decrease in mean biomass for both forage and sportfish, whereas flood conditions had more minor effects on biomass of both groups. While the wet and dry scenarios were constructed by proportional changes in discharge (changes of one standard deviation), the representative impacts on biomass were not proportional to these changes. Both sportfish and forage fish consistently demonstrated larger changes in the drought scenario than the wet scenario. A similar phenomenon was evident in the high variance projection, where the standard deviation in the random component was increased by 30%. Because there was no persistent change to the mean discharge, we would not expect to see a net change in biomass. On the contrary, both mean sportfish and forage fish biomass decreased by over 4% (Table 3). This change was greater than in simulations that implemented mild changes to mean discharge (RCP 4 2050s, RCP 4 2080s, RCP 8 2050s) and similar to the change experienced in the most extreme climatic scenario (RCP 8 2080s). This suggests the sensitivity of the model ecosystem to drought conditions and increased variability in discharge rates.
The limited increase in biomass predicted for the high flow scenarios may be a result of the underlying nutrient uptake equation for EwE, which is a Michaelis-Menten functional response (Michaelis and Menten, 1913). This relationship is relatively linear at low nutrient levels, but nutrient uptake approaches an asymptote at high concentrations. Because nutrient uptake changes more rapidly when approaching low nutrient concentrations than high concentrations, we would expect to observe greater biomass changes in drought conditions than flood conditions (Wallentinus, 1984). This expectation was evident in our projection simulations.
During floods with high nutrient and phytoplankton concentrations, it is possible that nutrients are replaced as a limiting factor by variables such as irradiance levels and CO2 concentrations. During algal blooms, competition for light can be a critical component that limits growth due to the effects of shading (Shigesada and Okubo, 1981; Huisman et al., 2004). Algal cells along the water’s surface may access abundant light resources but subsequently shade more benthic organisms. The effects of light limitation are also reflected in elevated chlorophyll-a concentrations in phytoplankton during low light conditions, which is likely a fitness strategy to increase photosynthetic potential (MacIntyre et al., 2002). In addition to the effects of light competition, there is also potential for CO2 limitation, particularly if nutrient and irradiance levels are high (Riebesell et al., 1993). This phenomenon may also be relevant for a subtropical estuary, such as the Suwannee Estuary, where high summer water temperatures are observed, thereby reducing the water’s ability to diffuse gaseous CO2.
While our model produced evidence for greater impacts of droughts than floods on ecological production, the literature demonstrates conflicting evidence of this phenomenon. An EwE model built for Saginaw Bay, Lake Huron was fitted with nutrient concentrations and produced similar results to our model (Kao et al., 2014). A common pattern for many functional groups was that biomass changed more substantially in low nutrient simulations than high nutrient simulations compared to a relative average nutrient projection (Kao et al., 2014). On the contrary, Althauser (2003) utilized EwE to analyze the relationship between river flow and estuarine productivity in a northern Gulf of Mexico estuary but did not observe limited effects of flooding on ecological production (2003). Flood events produced substantial increases in biomass for certain species, particularly for forage fish and zooplankton (Althauser, 2003). Also, there was not a consistent pattern of either floods or droughts having a greater impact on productivity. This may be explained by discharge being used to directly force primary production rates, as opposed to our study which converted discharge to nutrient concentrations in order to force primary production.
Similar studies in the northern Gulf of Mexico have also demonstrated the significance of drought conditions and overall flow on estuarine fish communities. Livingston et al. (1997) conducted a long-term study in Apalachicola Bay and found that periods of low flow were strongly associated with trophic reorganization within the estuary. During a two-year drought, herbivores, omnivores, and first order carnivores became very abundant while populations of upper trophic level carnivores decreased. These findings demonstrate similarities to an EwE analysis conducted by de Mutsert et al. (2012) where some functional groups were disproportionately impacted by changes in discharge from a river diversion project. In this study, mullet and anchovies demonstrated some of the largest biomass changes with changes in discharge, and their biomass was positively associated with discharge. Livingston et al. (1997) hypothesized that that trophic reorganization was caused by a decrease in nutrient enrichment and changes in turbidity and light penetration, while de Mutsert et al. (2012) focused the analysis on salinity tolerances. Although these two studies both demonstrated the significance of drought conditions and riverine flow on estuarine fish communities, the differences to our analysis were notable. While both studies observed significant changes in the structure of estuarine animal communities following changes in discharge, our analysis observed only slight variations between the responses of certain function groups. The reason for the difference between our findings and the aforementioned studies likely relates to how fish were allowed to respond to changes in salinity rather than their responses to bottom-up food web effects.
In the long-term climate scenarios for recreationally caught fish, certain clusters of species tracked together and responded in the same direction in the different projections. Seven species always demonstrated decreases in biomass, which included both snook stanzas, sheepshead, snapper, Gulf flounder, mid-age stanza red drum, and jack crevalle. On the contrary, five functional groups consistently exhibited increases in biomass, which were both spotted seatrout stanzas, sand seatrout, other sciaenids, and Spanish mackerel. The most likely reason that seatrout and other sciaenids were demonstrating increases in biomass was due more to changes in snook abundance than changes in the densities of their prey items, which generally exhibited small decreases in biomass. During the model fitting procedure, vulnerabilities of snook prey items were estimated to be high in order to produce the exponential increase in snook abundance that this population has demonstrated over the last decade (Purtlebaugh et al., 2020). Because seatrout and other sciaenids constituted large portions of snook diets, these groups were susceptible to top-down control by snook, such that decreases in snook result in increases in these species. Snook exhibited large decreases in biomass during drought conditions likely because of the high vulnerabilities of their prey items that were set during the fitting procedure. Although we used an environmental forcing function to project likely snook population increases, the observed decreases in snook abundance were relative to status quo discharge and nutrient conditions with the aforementioned snook environmental forcing function applied.
A relevant comparison can be made to Niiranen et al. (2013), where an EwE model was built for the Baltic Sea and analyzed the importance of both top-down and bottom-up effects on this marine food web. Evidence for an increase in biomass was common for functional groups, particularly forage fish groups, when nutrient loads were greater, but fishing pressure on cod (Gadus morhua callarias) also contributed significantly to population changes. In general, the model predicted a cod-dominated system when cod fishing mortality and nutrient enrichment were low. In the contradictory scenario, when cod fishing mortality and nutrient enrichment were high, the system was dominated by sprat (Sprattus sprattus) and exhibited a system influenced by eutrophication. A notable difference of this model to our work was that functional groups often demonstrated substantial biomass changes greater than 50%, while fish groups in our model generally displayed changes less than 20%. An important parallel to our model was that top-down and bottom-up effects can significantly impact an ecosystem, but our research suggested that snook may be exerting stronger top-down control on some species than the bottom-up effects of nutrient inputs.
In addition to predatory effects, snook may also demonstrate interspecific competition with other predators such as seatrout and Spanish mackerel (Naughton and Saloman, 1981; Blewett et al., 2006; Russell, 2005; Sagarese et al., 2016; Stevens et al., 2020). Like predatory impacts, competition would cause a decrease in the biomass of the competing species. Spanish mackerel may be more negatively impacted by competition with snook than seatrout because they have a greater diet overlap with snook. In our diet composition data, Spanish mackerel and snook were highly piscivorous, whereas seatrout demonstrated a more generalist diet that was constituted by forage fish and different invertebrate groups. Although the northern expansion of snook into this region has been noted, the ecological impacts of this expansion are still undetermined (Purtlebaugh et al., 2020). This model may provide insight to managers and fisheries scientists by revealing the strong predatory effects snook have on lower trophic levels as well as negative impacts on other predators through interspecific competition.
While the responses of functional groups varied with respect to changes in discharge, it is necessary to account for uncertainties in the model fitting procedure when interpreting these results. This is exemplified by biomass predictions for jack crevalle and snappers. Although these groups appeared not to be influenced by future environmental changes, during the model fitting procedure, poor fits were observed to the real time series data. Because the model did not originally capture the stochastic population dynamics of jack crevalle and snappers, modelers should not assume a strong predictive ability for these groups.
Research Recommendations
One major recommendation for further modeling developments is the incorporation of temperature and other climate responses (e.g., sea level rise, changes in habitat, species range expansions) as forcing functions on the system. Climate change projections were foundational to the discharge and nutrient simulations, but temperature will likely be the abiotic factor that is most directly driven by climate change and has the greatest ecological effect. Tropicalization and range expansion toward the poles are two commonly observed ecological phenomena associated with climate change (Nye et al., 2009; e Costa et al., 2014; Heck et al., 2015; Scheffel et al., 2018). Although the most prominent example of range expansion in the Suwannee estuary is the colonization of snook, there are also other examples of tropicalization throughout the Gulf. Fodrie et al. (2010) compared the composition of fish communities in the northern Gulf of Mexico from 2006 to 2007 to the 1970s and found numerous taxa from tropical latitudes that were observed between 2006 and 2007 but were historically absent. The region had also experienced an increase in sea surface temperatures of more than 3°C compared to the samples from the 1970s (Livingston, 1985). Although our model demonstrated moderate changes in biomass due to changes in nutrient concentrations, this model did not predict the inclusion of any new species into the system. Recommendations for future EwE research would be to include potential northward expanding species, linked to temperature forcing functions, and evaluate their impacts on the food web. In addition to tropicalization due to warmer temperatures, climate change also has implications for habitat types and associated animal communities. The transition of shorelines consisting of salt marsh (Spartina alternifora) to black mangrove (Avicennia germinans) has been documented in the estuary (Stevens et al., 2006). Changes in the densities of these habitat types will likely have impacts on the benthic invertebrate and nekton communities that inhabit them (Scheffel et al., 2018).
Another significant recommendation for future research would be to incorporate potential negative impacts of eutrophication and changes in discharge associated with precipitation patterns and water withdrawals on this system or similar estuaries. Many of Florida’s rivers and coastal estuaries are currently experiencing levels of eutrophication that are negatively impacting natural resources (Greening and Janicki, 2006; Turner et al., 2006; Thomas et al., 2007; Lapointe et al., 2015). This is a prominent concern in estuarine fisheries management, particularly in the Gulf of Mexico, where hypoxic events are often associated with fish kills and migration out of oxygen depleted waters (Rabalais et al., 2002). Benthic invertebrates can also be significantly impacted by these events, as many species are sessile and unable to migrate out of the system. These phenomena are generally not prevalent in the Suwannee Estuary due to its relatively undeveloped watershed and natural condition.
Our results suggested that increased frequency and intensity of low discharge via droughts and human water withdrawals would influence forage and sportfish biomass in the future. Understanding the impacts of both climate change and active water withdrawals will be critical for future predictions of impacts on this system, and best management practices could be implemented to at least partially mitigate these impacts. Future EwE models should also be designed to assess the effects of eutrophication and discharge effects on fish stocks.
Natural Resource Management
sOur results demonstrate that the impacts of droughts on ecological production have strong implications for natural resource management. Water management in Florida has particularly focused on minimum flow requirements as an important criterion for conservation (Doering et al., 2002; Mattson, 2002; Munson and Delfino, 2007). A major objective of this management has been to provide optimum environmental conditions for estuarine organisms downstream. There has likely been a larger focus on restoring historically lower salinity levels to estuaries, but this research may demonstrate the impact that drought conditions and nutrient concentrations can have on estuarine fish. This model can be used to inform water management districts on the impact that anomalously low flow events can have on ecological productivity.
Our study has implications for fisheries management with respect to varying harvest strategies during different environmental scenarios. Assuming fish production is at least partially driven by river outflow, fisheries managers may consider employing a feedback policy that varies harvests during different flow regimes. Concerns have been raised about fixed harvest rates that do not account for changing stock sizes or environmental conditions (Walters, 1986; Walters and Martell, 2004). Fixed harvest rates have the potential to further limit already depleted stocks by causing depensatory impacts that negatively affect fecundity and population growth. Future fishery management policies should consider effects of short-term and long-term changes in climate and water withdrawals that would directly influence sportfish populations.
Data Availability Statement
The original contributions presented in the study are included in the article/Supplementary Material, further inquiries can be directed to the corresponding author/s.
Author Contributions
DS and DC built the model and conducted the analysis. MA provided conceptual and strategic input on model development and simulations. All authors contributed to writing the manuscript.
Funding
Funding for this research was provided by fellowship donations from the Fish America Foundation to the Florida Forage Fish Coalition consisting of the International Game Fish Association, Pew Charitable Trusts, Wild Oceans, American Sportfishing Association, Florida Wildlife Federation, and the Angler Action Foundation. The views expressed herein were those of the author(s) and did not necessarily reflect the views of the Florida Forage Fish Coalition.
Conflict of Interest
The authors declare that the research was conducted in the absence of any commercial or financial relationships that could be construed as a potential conflict of interest.
Acknowledgments
We would like to acknowledge the FIM program of FWC for providing fish density data, the Project COAST and Lakewatch sampling programs for collecting nutrient concentration data, USGS for supplying Suwannee River discharge data, MRIP for providing fisheries landings data, and the Big Bend Seagrass Aquatic Preserve for collecting seagrass densities.
Supplementary Material
The Supplementary Material for this article can be found online at: https://www.frontiersin.org/articles/10.3389/fmars.2021.689950/full#supplementary-material
References
Ahrens, R. N., Walters, C. J., and Christensen, V. (2012). Foraging arena theory. Fish Fish. 13, 41–59. doi: 10.1111/j.1467-2979.2011.00432.x
Akaike, H. (1974). A new look at the statistical model identification. IEEE Trans. Automat. Control 19, 716–723. doi: 10.1109/tac.1974.1100705
Alber, M. (2002). A conceptual model of estuarine freshwater inflow management. Estuaries 25, 1246–1261. doi: 10.1007/bf02692222
Alder, J., Campbell, B., Karpouzi, V., Kaschner, K., and Pauly, D. (2008). Forage fish: from ecosystems to markets. Annu. Rev. Environ. Resour. 33, 153–166. doi: 10.1146/annurev.environ.33.020807.143204
Althauser, L. L. (2003). An Ecopath/Ecosim analysis of an estuarine food web: seasonal energy flow and response to river-flow related perturbations. Ph. D. Thesis. Baton Rouge: Louisiana State University.
Baptista, J., Martinho, F., Dolbeth, M., Viegas, I., Cabral, H., and Pardal, M. (2010). Effects of freshwater flow on the fish assemblage of the Mondego estuary (Portugal): comparison between drought and non-drought years. Mar. Freshw. Res. 61, 490–501. doi: 10.1071/mf09174
Bartholomew, A., and Bohnsack, J. A. (2005). A review of catch-and-release angling mortality with implications for no-take reserves. Rev. Fish Biol. Fish. 15, 129–154. doi: 10.1007/s11160-005-2175-1
Beck, M. W., Brumbaugh, R. D., Airoldi, L., Carranza, A., Coen, L. D., Crawford, C., et al. (2011). Oyster reefs at risk and recommendations for conservation, restoration, and management. Bioscience 61, 107–116. doi: 10.1525/bio.2011.61.2.5
Benke, A. C. (1990). A perspective on America’s vanishing streams. J. North Am. Benthol. Soc. 9, 77–88. doi: 10.2307/1467936
Blewett, D. A., Hensley, R. A., and Stevens, P. W. (2006). Feeding habits of common snook, Centropomus undecimalis, in Charlotte Harbor, Florida. Gulf Caribb. Res. 18, 1–14.
Chagaris, D., Binion-Rock, S., Bogdanoff, A., Dahl, K., Granneman, J., Harris, H., et al. (2017). An ecosystem-based approach to evaluating impacts and management of invasive lionfish. Fisheries 42, 421–431. doi: 10.1080/03632415.2017.1340273
Chagaris, D., and Mahmoudi, B. (2009). “Assessing the influence of bottom-up and top-down processes in Tampa Bay using Ecopath with Ecosim,” in Proceedings of the Tampa Bay Area Scientific Information Symposium, BASIS, Vol. 5, (St. Petersburg, FL: Tampa Bay Estuary Program), 263–274.
Chagaris, D. D., Mahmoudi, B., Walters, C. J., and Allen, M. S. (2015). Simulating the trophic impacts of fishery policy options on the West Florida Shelf using Ecopath with Ecosim. Mar. Coast. Fish. 7, 44–58. doi: 10.1080/19425120.2014.966216
Christensen, V., and Walters, C. J. (2004). Ecopath with Ecosim: methods, capabilities and limitations. Ecol. Model. 172, 109–139. doi: 10.1016/j.ecolmodel.2003.09.003
Christensen, V., Walters, C. J., and Pauly, D. (2005). Ecopath With Ecosim: A User’s Guide. Vancouver, BC: University of British Columbia, 154.
Colléter, M., Valls, A., Guitton, J., Gascuel, D., Pauly, D., and Christensen, V. (2015). Global overview of the applications of the Ecopath with Ecosim modeling approach using the EcoBase models repository. Ecol. Model. 302, 42–53. doi: 10.1016/j.ecolmodel.2015.01.025
de Mutsert, K., Cowan, J. H. Jr., and Walters, C. J. (2012). Using Ecopath with Ecosim to explore nekton community response to freshwater diversion into a Louisiana estuary. Mar. Coast. Fish. 4, 104–116. doi: 10.1080/19425120.2012.672366
de Mutsert, K., Lewis, K., Milroy, S., Buszowski, J., and Steenbeek, J. (2017). Using ecosystem modeling to evaluate trade-offs in coastal management: effects of large-scale river diversions on fish and fisheries. Ecol. Model. 360, 14–26. doi: 10.1016/j.ecolmodel.2017.06.029
Diaz, R. J., and Rosenberg, R. (2008). Spreading dead zones and consequences for marine ecosystems. Science 321, 926–929. doi: 10.1126/science.1156401
Doering, P. H., Chamberlain, R. H., and Haunert, D. E. (2002). Using submerged aquatic vegetation to establish minimum and maximum freshwater inflows to the Caloosahatchee Estuary, Florida. Estuaries 25, 1343–1354. doi: 10.1007/bf02692229
e Costa, B. H., Assis, J., Franco, G., Erzini, K., Henriques, M., Gonçalves, E. J., et al. (2014). Tropicalization of fish assemblages in temperate biogeographic transition zones. Mar. Ecol. Prog. Ser. 504, 241–252. doi: 10.3354/meps10749
Florida Department of Environmental Protection (2018). DEP Public Files. Tallahassee, FL: Florida Department of Environmental Protection.
Florida Fish and Wildlife Conservation Commission (2021). GIS & Mapping Data Downloads. Tallahassee, FL: Florida Fish and Wildlife Conservation Commission.
Fodrie, F. J., Heck, K. L. Jr., Powers, S. P., Graham, W. M., and Robinson, K. L. (2010). Climate-related, decadal-scale assemblage changes of seagrass-associated fishes in the northern Gulf of Mexico. Glob. Change Biol. 16, 48–59. doi: 10.1111/j.1365-2486.2009.01889.x
Franklin, M. A., Giese, G. L., and Mixon, P. R. (1995). Statistical Summaries of Surface-Water Hydrologic Data Collected in the Suwannee River Water Management District, Florida, 1906– 1993. Open-File Report 94-709. Tallahassee, FL: U.S. Geological Survey.
Froese, R., and Pauly, D. (2020). FishBase. World Wide Web Electronic Publication. Burlington, MA: ScienceOpen, Inc.
Gillson, J. (2011). Freshwater flow and fisheries production in estuarine and coastal systems: where a drop of rain is not lost. Rev. Fish. Sci. 19, 168–186. doi: 10.1080/10641262.2011.560690
Grabowski, J. H., and Peterson, C. H. (2007). Restoring oyster reefs to recover ecosystem services. Ecosyst. Eng. Plants Prot. 4, 281–298. doi: 10.1016/s1875-306x(07)80017-7
Greening, H., and Janicki, A. (2006). Toward reversal of eutrophic conditions in a subtropical estuary: Water quality and seagrass response to nitrogen loading reductions in Tampa Bay, Florida, USA. Environ. Manag. 38, 163–178. doi: 10.1007/s00267-005-0079-4
Grimes, C. B., and Kingsford, M. J. (1996). How do riverine plumes of different sizes influence fish larvae: do they enhance recruitment? Mar. Freshw. Res. 47, 191–208. doi: 10.1071/mf9960191
Heck, K. L. Jr., Fodrie, F. J., Madsen, S., Baillie, C. J., and Byron, D. A. (2015). Seagrass consumption by native and a tropically associated fish species: potential impacts of the tropicalization of the northern Gulf of Mexico. Mar. Ecol. Prog. Ser. 520, 165–173. doi: 10.3354/meps11104
Hilborn, R., and Mangel, M. (1997). The Ecological Detective: Confronting Models With Data, Vol. 28. Princeton, NJ: Princeton University Press.
Huisman, J., Sharples, J., Stroom, J. M., Visser, P. M., Kardinaal, W. E. A., Verspagen, J. M., et al. (2004). Changes in turbulent mixing shift competition for light between phytoplankton species. Ecology 85, 2960–2970. doi: 10.1890/03-0763
Ihde, T. F., and Townsend, H. M. (2017). Accounting for multiple stressors influencing living marine resources in a complex estuarine ecosystem using an Atlantis model. Ecol. Model. 365, 1–9. doi: 10.1016/j.ecolmodel.2017.09.010
Kao, Y. C., Adlerstein, S., and Rutherford, E. (2014). The relative impacts of nutrient loads and invasive species on a Great Lakes food web: an Ecopath with Ecosim analysis. J. Great Lakes Res. 40, 35–52. doi: 10.1016/j.jglr.2014.01.010
Kemp, W. M., Boynton, W. R., Adolf, J. E., Boesch, D. F., Boicourt, W. C., Brush, G., et al. (2005). Eutrophication of Chesapeake Bay: historical trends and ecological interactions. Mar. Ecol. Prog. Ser. 303, 1–29. doi: 10.3354/meps303001
Kingston, D. G., Todd, M. C., Taylor, R. G., Thompson, J. R., and Arnell, N. W. (2009). Uncertainty in the estimation of potential evapotranspiration under climate change. Geophys. Res. Lett. 36:L20403.
Lapointe, B. E., Herren, L. W., Debortoli, D. D., and Vogel, M. A. (2015). Evidence of sewage-driven eutrophication and harmful algal blooms in Florida’s Indian River Lagoon. Harmful Algae 43, 82–102. doi: 10.1016/j.hal.2015.01.004
Link, J. S. (2010). Adding rigor to ecological network models by evaluating a set of pre-balance diagnostics: a plea for PREBAL. Ecol. Model. 221, 1580–1591. doi: 10.1016/j.ecolmodel.2010.03.012
Livingston, R. J. (1985). “Organization of fishes in coastal seagrass system: the response to stress,” in Fish Community Ecology in Estuaries and Coastal Lagoons: Towards an Ecosystem Integration, ed. A. Yáñez-Arancibia (Mexico City: UNAM), 367–382.
Livingston, R. J. (2000). Eutrophication Processes in Coastal Systems: Origin and Succession of Plankton Blooms and Effects on Secondary Production in Gulf Coast Estuaries. Boca Raton, FL: CRC Press.
Livingston, R. J., Niu, X., Lewis, F. G. III, and Woodsum, G. C. (1997). Freshwater input to a gulf estuary: Long-term control of trophic organization. Ecol. Appl. 7, 277–299. doi: 10.1890/1051-0761(1997)007[0277:fitage]2.0.co;2
Lo, N. C. H., Jacobson, L. D., and Squire, J. L. (1992). Indices of relative abundance from fish spotter data based on delta-lognormal models. Can. J. Fish. Aquat. Sci. 49, 2515–2526. doi: 10.1139/f92-278
MacIntyre, H. L., Kana, T. M., Anning, T., and Geider, R. J. (2002). Photoacclimation of photosynthesis irradiance response curves and photosynthetic pigments in microalgae and cyanobacteria 1. J. Phycol. 38, 17–38. doi: 10.1046/j.1529-8817.2002.00094.x
Marella, R. L., Dixon, J. F., and Berry, D. R. (2016). Agricultural Irrigated Land-Use Inventory for the Counties in the Suwannee River Water Management District in Florida, 2015 (No. 2016-1111). Reston, VA: US Geological Survey.
Martell, S. J., Beattie, A. I., Walters, C. J., Nayar, T., and Briese, R. (2002). Simulating fisheries management strategies in the Strait of Georgia ecosystem using Ecopath and Ecosim. Fish. Centre Res. Rep. 10:16.
Mattson, R. A. (2002). A resource-based framework for establishing freshwater inflow requirements for the Suwannee River estuary. Estuaries 25, 1333–1342. doi: 10.1007/bf02692228
Maunder, M. N., and Punt, A. E. (2004). Standardizing catch and effort data: a review of recent approaches. Fish. Res. 70, 141–159. doi: 10.1016/j.fishres.2004.08.002
Milly, P. C., Dunne, K. A., and Vecchia, A. V. (2005). Global pattern of trends in streamflow and water availability in a changing climate. Nature 438:347. doi: 10.1038/nature04312
Morel, A., and Berthon, J. F. (1989). Surface pigments, algal biomass profiles, and potential production of the euphotic layer: relationships reinvestigated in view of remote-sensing applications. Limnol. Oceanogr. 34, 1545–1562. doi: 10.4319/lo.1989.34.8.1545
Munson, A. B., and Delfino, J. J. (2007). Minimum wet-season flows and levels in Southwest Florida Rivers 1. J. Am. Water Resour. Assoc. 43, 522–532. doi: 10.1111/j.1752-1688.2007.00040.x
National Oceanic and Atmospheric Administration (2018). Data from: Recreational Fisheries Statistics Queries. Washington, DC: National Oceanic and Atmospheric Administration.
Naughton, S. P., and Saloman, C. H. (1981). Stomach contents of juveniles of king mackerel (Scomberomorus cavalla) and Spanish mackerel (S. maculatus). Gulf Mexico Sci. 5:12.
Neupane, R. P., Ficklin, D. L., Knouft, J. H., Ehsani, N., and Cibin, R. (2019). Hydrologic responses to projected climate change in ecologically diverse watersheds of the Gulf Coast, United States. Int. J. Climatol. 39, 2227–2243. doi: 10.1002/joc.5947
Niiranen, S., Yletyinen, J., Tomczak, M. T., Blenckner, T., Hjerne, O., MacKenzie, B. R., et al. (2013). Combined effects of global climate change and regional ecosystem drivers on an exploited marine food web. Glob. Change Biol. 19, 3327–3342.
Nye, J. A., Link, J. S., Hare, J. A., and Overholtz, W. J. (2009). Changing spatial distribution of fish stocks in relation to climate and population size on the Northeast United States continental shelf. Mar. Ecol. Prog. Ser. 393, 111–129. doi: 10.3354/meps08220
Osland, M. J., Enwright, N., Day, R. H., and Doyle, T. W. (2013). Winter climate change and coastal wetland foundation species: salt marshes vs. mangrove forests in the southeastern United States. Glob. Change Biol. 19, 1482–1494. doi: 10.1111/gcb.12126
Paerl, H. W. (2006). Assessing and managing nutrient-enhanced eutrophication in estuarine and coastal waters: interactive effects of human and climatic perturbations. Ecol. Eng. 26, 40–54. doi: 10.1016/j.ecoleng.2005.09.006
Peebles, E. B. (2002). Temporal resolution of biological and physical influences on bay anchovy Anchoa mitchilli egg abundance near a river-plume frontal zone. Mar. Ecol. Prog. Ser. 237, 257–269. doi: 10.3354/meps237257
Piazza, B. P., and La Peyre, M. K. (2011). Nekton community response to a large-scale Mississippi River discharge: examining spatial and temporal response to river management. Estuar. Coast. Shelf Sci. 91, 379–387. doi: 10.1016/j.ecss.2010.11.001
Pikitch, E., Boersma, P. D., Boyd, I., Conover, D., Cury, P., Essington, T., et al. (2012). Little Fish, Big Impact: Managing a Crucial Link in Ocean Food Webs. Washington, DC: Lenfest Ocean Program.
Pikitch, E. K., Santora, C., Babcock, E. A., Bakun, A., Bonfil, R., Conover, D. O., et al. (2004). Ecosystem-based fishery management. Science 305, 346–347. doi: 10.1126/science.1098222
Poff, N. L., Allan, J. D., Bain, M. B., Karr, J. R., Prestegaard, K. L., Richter, B. D., et al. (1997). The natural flow regime. BioScience 47, 769–784.
Purtlebaugh, C. H., Martin, C. W., and Allen, M. S. (2020). Poleward expansion of common snook Centropomus undecimalis in the northeastern Gulf of Mexico and future research needs. PLoS One 15:e0234083. doi: 10.1371/journal.pone.0234083
Rabalais, N. N., Turner, R. E., and Wiseman, W. J. Jr. (2002). Gulf of Mexico hypoxia, aka “The dead zone”. Annu. Rev. Ecol. Syst. 33, 235–263. doi: 10.1146/annurev.ecolsys.33.010802.150513
Riebesell, U., Wolf-Gladrow, D. A., and Smetacek, V. (1993). Carbon dioxide limitation of marine phytoplankton growth rates. Nature 361, 249–251. doi: 10.1038/361249a0
Russell, M. (2005). Spotted Sea Trout (Cynoscion nebulosus) and Pinfish (Lagodon rhomboides) Dietary Analysis According to Habitat Type. Louisiana: Louisiana State University.
Sagarese, S. R., Nuttall, M. A., Geers, T. M., Lauretta, M. V., Walter, J. F. III, and Serafy, J. E. (2016). Quantifying the trophic importance of Gulf menhaden within the northern Gulf of Mexico ecosystem. Mar. Coast. Fish. 8, 23–45. doi: 10.1080/19425120.2015.1091412
Sakamoto, A., and Shirakihara, K. (2017). Ecosystem dynamics in Tokyo Bay with a focus on high trophic levels using Ecopath with Ecosim. J. Mar. Sci. Technol. 22, 1–10. doi: 10.1007/s00773-016-0388-8
Scheffel, W. A., Heck, K. L., and Johnson, M. W. (2018). Tropicalization of the northern Gulf of Mexico: impacts of salt marsh transition to black mangrove dominance on faunal communities. Estuar. Coasts 41, 1193–1205. doi: 10.1007/s12237-017-0334-y
Seavey, J. R., Pine, W. E. III, Frederick, P., Sturmer, L., and Berrigan, M. (2011). Decadal changes in oyster reefs in the Big Bend of Florida’s Gulf Coast. Ecosphere 2, 1–14. doi: 10.2307/j.ctvx0709s.5
Shigesada, N., and Okubo, A. (1981). Analysis of the self-shading effect on algal vertical distribution in natural waters. J. Math. Biol. 12, 311–326. doi: 10.1007/bf00276919
Smith, M., Chagaris, D., Paperno, R., and Markwith, S. (2020). Ecosystem structure and resilience of the Florida Bay Estuary: an original ecosystem model with implications for everglades restoration. Mar. Freshw. Res. 72, 563–583. doi: 10.1071/mf20125
Sosa-López, A., Mouillot, D., Ramos-Miranda, J., Flores-Hernandez, D., and Chi, T. D. (2007). Fish species richness decreases with salinity in tropical coastal lagoons. J. Biogeogr. 34, 52–61. doi: 10.1111/j.1365-2699.2006.01588.x
Stevens, P. W., Dutka-Gianelli, J., Nagid, E. J., Trotter, A. A., Johnson, K. G., Tuten, T., et al. (2020). Niche partitioning among snook (Pisces: Centropomidae) in rivers of southeastern Florida and implications for species range limits. Estuar. Coasts 43, 396–408. doi: 10.1007/s12237-019-00650-x
Stevens, P. W., Fox, S. L., and Montague, C. L. (2006). The interplay between mangroves and saltmarshes at the transition between temperate and subtropical climate in Florida. Wetlands Ecol. Manag. 14, 435–444. doi: 10.1007/s11273-006-0006-3
Swain, E., and Davis, J. H. (2016). Applying downscaled global climate model data to a groundwater model of the Suwannee River basin, Florida, USA. Am. J. Clim. Change 5, 526–557. doi: 10.4236/ajcc.2016.54037
Thomas, P., Rahman, M. S., Khan, I. A., and Kummer, J. A. (2007). Widespread endocrine disruption and reproductive impairment in an estuarine fish population exposed to seasonal hypoxia. Proc. R. Soc. B Biol. Sci. 274, 2693–2702. doi: 10.1098/rspb.2007.0921
Townsend, H. (2014). Comparing and coupling a water quality and a fisheries ecosystem model of the Chesapeake Bay for the exploratory assessment of resource management strategies. ICES J. Mar. Sci. 71, 703–712. doi: 10.1093/icesjms/fst060
Trenberth, K. E. (2011). Changes in precipitation with climate change. Clim. Res. 47, 123–138. doi: 10.3354/cr00953
Turner, R. E., Rabalais, N. N., Fry, B., Atilla, N., Milan, C. S., Lee, J. M., et al. (2006). Paleo-indicators and water quality change in the Charlotte Harbor Estuary (Florida). Limnol. Oceanogr. 51, 518–533. doi: 10.4319/lo.2006.51.1_part_2.0518
United States Geological Survey (2020). USGS 02323500 Suwannee River Near Wilcox, Fla. Reston, VA: United States Geological Survey.
Wallentinus, I. (1984). Comparisons of nutrient uptake rates for Baltic macroalgae with different thallus morphologies. Mar. Biol. 80, 215–225. doi: 10.1007/bf02180189
Walters, C. (2000). Impacts of dispersal, ecological interactions, and fishing effort dynamics on efficacy of marine protected areas: how large should protected areas be? Bull. Mar. Sci. 66, 745–757.
Walters, C. J. (1986). Adaptive Management of Renewable Resources. New York, NY: Macmillan Publishers Ltd.
Walters, C. J., and Martell, S. J. (2004). Fisheries Ecology and Management. Princeton, NJ: Princeton University Press.
Walther, G. R., Post, E., Convey, P., Menzel, A., Parmesan, C., Beebee, T. J., et al. (2002). Ecological responses to recent climate change. Nature 416:389.
Keywords: Ecopath with Ecosim, forage fish, ecosystem model, top-down, bottom-up, Gulf of Mexico
Citation: Sinnickson D, Chagaris D and Allen M (2021) Exploring Impacts of River Discharge on Forage Fish and Predators Using Ecopath With Ecosim. Front. Mar. Sci. 8:689950. doi: 10.3389/fmars.2021.689950
Received: 01 April 2021; Accepted: 19 May 2021;
Published: 10 June 2021.
Edited by:
Chiara Piroddi, Joint Research Centre, ItalyReviewed by:
Igor Celic, National Institute of Oceanography and Applied Geophysics, ItalyAndrea Belgrano, Swedish University of Agricultural Sciences, Sweden
Copyright © 2021 Sinnickson, Chagaris and Allen. This is an open-access article distributed under the terms of the Creative Commons Attribution License (CC BY). The use, distribution or reproduction in other forums is permitted, provided the original author(s) and the copyright owner(s) are credited and that the original publication in this journal is cited, in accordance with accepted academic practice. No use, distribution or reproduction is permitted which does not comply with these terms.
*Correspondence: Dylan Sinnickson, dsinnickson@ufl.edu