- 1Department of Biological Sciences, California State University, Long Beach, Long Beach, CA, United States
- 2United States Fish and Wildlife Service, Fremont, CA, United States
- 3Marine Science Institute, University of California, Santa Barbara, Santa Barbara, CA, United States
- 4Bren School of Environmental Science and Management, University of California, Santa Barbara, Santa Barbara, CA, United States
- 5Environmental Market Solutions Lab, University of California, Santa Barbara, Santa Barbara, CA, United States
- 6Department of Marine Biology, Texas A&M University at Galveston, Galveston, TX, United States
- 7Department of Organismic and Evolutionary Biology, Harvard University, Cambridge, MA, United States
- 8Guy Harvey Research Institute, Nova Southeastern University, Dania Beach, FL, United States
- 9Monterey Bay Aquarium, Monterey, CA, United States
- 10Aquatic Research Consultants, San Pedro, CA, United States
- 11Departamento de Oceanografía Biológica, Centro de Investigación Científica y de Educación Superior de Ensenada (CICESE), Ensenada, Mexico
Many terrestrial and aquatic taxa are known to form periodic aggregations, whether across life history or solely during specific life stages, that are generally governed by the availability and distribution of resources. Associations between individuals during such aggregation events are considered random and not driven by social attraction or underlying community structure. White sharks (Carcharodon carcharias) have been described as a species that exhibits resource-driven aggregative behaviors across ontogenetic stages and juvenile white sharks are known to form aggregations at specific nursery sites where individuals may remain for extended periods of time in the presence of other individuals. We hypothesized juvenile white sharks form distinct communities during these critical early phases of ontogeny and discuss how a tendency to co-occur across life stages may be seeded by the formation of these communities in early ontogeny. We present results from a series of social network analyses of 86 juvenile white sharks derived from 6 years of passive acoustic telemetry data in southern California, demonstrating the likelihood of association of tagged juvenile white sharks is greater when sharks are of similar size-classes. Individuals in observed networks exhibited behaviors that best approximated fission-fusion dynamics with spatiotemporally unstable group membership. These results provide evidence of possible non-resource driven co-occurrence and community structure in juvenile white sharks during early life stages.
Introduction
Sociality, or the propensity of individuals to form social groups (Merriam-Webster., 2021), is a trait exhibited across taxa and varies between loose temporary aggregations to life-long associations (Alexander, 1974; Sabol et al., 2020). Social interactions have been shown to reduce predation risk and increase foraging efficiency, navigational capability, and reproductive opportunities (Farine et al., 2015; Berdahl et al., 2018; Campbell et al., 2018; Diaz-Aguirre et al., 2019). Thus, animal social groups usually form when the associated benefits of group behavior outweigh the costs, while the influence of such associated costs upon the decision to engage in social behaviors is driven by extrinsic (e.g., prey availability) and intrinsic (e.g., competition) variability (Krause and Ruxton, 2002; Silk et al., 2014). Animal social groups are thus characterized by motivated cohesion and can be distinguished from aggregative behaviors, which are temporary assemblages of individuals in response to non-social forcing factors, such as seasonal resource availability (Jacoby et al., 2011; Meese and Lowe, 2019; Grueter et al., 2020).
White sharks (Carcharodon carcharias) are circumglobally distributed in both temperate and tropical waters (Compagno, 2002). In the sub-adult to adult ontogenetic stages, they are known to occur as solitary individuals but are also found in seasonal aggregations at feeding grounds such as pinniped haul outs (Bruce et al., 2006; Robbins, 2007; Domeier and Nasby-Lucas, 2008; Jorgensen et al., 2010; Duffy et al., 2012; Kock et al., 2013, 2018; Kanive et al., 2021). To date, the extent to which socially motivated factors play a role in co-occurrences of individual white sharks remains unresolved, with only two published studies on the subject arriving at different conclusions (Findlay et al., 2016; Schilds et al., 2019). Specifically, Findlay et al. (2016) reported that associations between white sharks in their study were random, although they exhibited weak structuring by sex and body size, whereas Schilds et al. (2019) reported non-random, sex-dependent associations with temporal variability. These studies drew upon observations of sub-adult and adult sharks co-occurring under baited and chummed (burleyed) conditions, at locations proximal to pinniped haul-outs, with very different time thresholds constituting co-occurrence. Juvenile white sharks use nearshore and beach habitat, where they have been observed to exhibit high degrees of residency and aggregation site fidelity (Weng et al., 2007; Bruce and Bradford, 2008; Werry et al., 2012; Dicken and Booth, 2013; Harasti et al., 2017; Oñate-González et al., 2017; Curtis et al., 2018; Tamburin et al., 2019; White et al., 2019; Spaet J. L. Y. et al., 2020; Anderson et al., 2021a,b). Such site fidelity is common among elasmobranchs in early ontogeny, as these habitats typically provide opportunities for optimal growth and foraging, and reduced predation risk (Simpfendorfer and Milward, 1993; Heupel et al., 2007; Chapman et al., 2009). Preliminary high-spatial-resolution movement data derived from hyperbolic positioning via a high density acoustic array off Carpinteria, California, indicate individual juvenile white sharks form aggregations (5–30 individuals of multiple size-classes) and use overlapping, spatially restricted areas (∼8 km2) continuously, for periods of weeks to months (Spurgeon et al., unpublished data; Anderson et al., 2021b).
It is unknown whether co-occurrence of individual sharks is random, driven by resource availability and/or environmental factors, or is a function of socially mediated behaviors. Co-occurrence may be a function of overlap in home ranges and exploited niches, or may be due to preferred associations between individuals of phenotypic characteristics. In network theory, this propensity for individuals with similar traits to co-occur is termed assortativity (Newman, 2002; Noldus and Van Mieghem, 2015). Co-occurrence of individual sharks may afford the opportunity for the transfer of information and the development of association preferences. Thus, seasonal resource-driven aggregations may seed the development of social groups and assortative interactions (Jacoby et al., 2011).
In this study, we applied a network analysis to passive acoustic telemetry data gathered from tagged juvenile white sharks [ < 150–350 cm Total Length (TL)] in southern California over a 6-year period (2014–2019). This approach was designed to gather insight as to whether tagged shark co-occurrence at acoustically monitored sites was random, an artifact of resource-driven aggregation behavior, or may be driven by social preferences. We aimed to examine (1) whether apparent structure could be identified within generated networks, (2) the extent to which tagged sharks formed associations with other tagged individuals, (3) the extent of association stability across time, and (4) to characterize assortativity in association preferences.
Materials and Methods
Tag Models and Tagging Procedures
A total of ninety sharks, of which detection data from eighty-six individuals used in the study were accessed via one of three ways (incidental catch, targeted catch, dart tagging), and were outfitted with either Vemco V16 or V13 coded acoustic transmitters (Vemco | Innovasea, Nova Scotia, Canada; transmitter family V13-1x-069k, V13-2x-069k, V16-4x-069k, V16-5x-069k. V16-6x-069k) (Supplementary Table 1).
Incidental Catch
Commercial gillnet fishers, working in collaboration with CSULB and CICESE researchers, brought incidentally captured juvenile white sharks to the nearest port in a large fish tote (1.2 × 1.2 × 1.2 m) with flowing seawater. Incidentally-caught sharks were caught offshore (>3 nm) outside of State waters. Researchers physically assessed, measured, and surgically implanted a plasma sterilized V16 transmitter into the abdominal cavity of the shark through a small incision (5 cm). The wound was closed with 2–3 interrupted sutures, before the animal was released approximately 2 km offshore. All individuals were visually monitored during release and behavior was recorded. A previous study demonstrated post-release survival rates of juvenile white sharks retrieved live from gillnets to be in the order of 93% (Lyons et al., 2013), while a more recent analysis indicated that minimizing handling and on-deck holding times may in turn minimize non-lethal post-release negative effects (Raoult et al., 2019). Approximate elapsed times from researchers taking possession of the shark to offshore release were < 30 min.
Targeted Catch
Juvenile white sharks were caught in shallow inshore waters either via a purse-seine, or via targeted quick-extraction with a gillnet. Sharks were transferred to a custom-made staging tank and ventilated, before being sexed, sized, and outfitted with a surgically implanted V16 transmitter as described above. Approximate handling times in all instances were < 20 min.
Dart Tagging
Juvenile white sharks swimming close to, or at the surface (at least 2 m visibility) were approached by either a small boat or personal watercraft from behind, with the aim of minimizing stress and flight response from the targeted animal and tagged with either a V13 or V16 acoustic tag using a 3 m modified pole-spear fitted with a tag applicator. Tags were inserted into the dorsal musculature at the base of the first dorsal fin using a titanium dart tethered to the tag. When possible, targeted sharks were visually checked for the presence of existing tags, and sex of individual was determined using a pole-mounted dip camera. Tagging effort via this method was largely directed at locations where sharks were known to seasonally aggregate. Shark size (TL cm) was estimated by comparison to an object of known size (the watercraft) from orthogonal aerial drone footage. Comparative methods such these have been shown to produce valid estimates of size (Sequeira et al., 2016; May et al., 2019). All tagged sharks were assigned a size-class based upon their measured or estimated size at time of tagging (Table 1). For sharks detected in more than one calendar year, a growth rate of 25 cm per year was assumed (Cailliet et al., 1985).
Spatial and Temporal Detection Analysis
Acoustic detection data were acquired from a wide-spread acoustic receiver array of up to 75 Vemco VR2 and VR2W receivers, deployed between Estero Bay (35.448, −120.952) and San Diego [32.876, −117.260 (Figure 1)], but also included offshore island monitoring sites at Santa Catalina Island (33.389, −118.359). From north to south, these included locations proximal to the following locations/landmarks: Estero Bay, Morro Bay, Pismo Beach, Santa Barbara, Ventura, Santa Catalina Island, Santa Monica Bay, Long Beach—Huntington Beach, Newport Beach—Laguna Beach, Dana Point—San Clemente, Oceanside, and San Diego (Figure 1). Receivers were largely installed and maintained by CSULB, but also included receivers of collaborators: University of California San Diego (UCSD), the Southern California Acoustic Telemetry Tracking Network (SCATTN), and the Ensenada Center for Scientific Research and Higher Education (CICESE). Acoustic receivers were deployed in shallow (∼ 3–40 m depth) coastal waters within 1,000 m of the shoreline (50% within 400 m), across a range of environments, including calm protected habitats (e.g., embayments, harbors), exposed high energy sand substrata (e.g., beach habitat), rocky reef, and kelp forest habitats. Receiver detection range (nominal range 150–700 m) varied by site, habitat type, and transmitter power output (Heupel et al., 2006; Kessel et al., 2014; Huveneers et al., 2016). For example, range testing performed in waters off Santa Catalina Island and Long Beach Harbor revealed average detection ranges of 150 m to ∼ 200 m (V13 and V9 low power transmitters, respectively; Wolfe and Lowe, 2015; Clevenstine and Lowe, 2021), while mean detection ranges of high power output V16 transmitters in waters off San Clemente and Santa Barbara are estimated to be in the region of 500–700 m (Stirling et al., unpublished data). Thus, a nominal detection range of 500 m across the entire array was considered. To account for the possibility of false detections, raw receiver data were filtered to include only individuals that were detected two or more times per day (Simpfendorfer et al., 2015). Detection data were visually inspected to check for the possibility of double-tagged animals. Where double-tagged animals were identified, all data pertaining to the 2nd tag were removed from the analyses.
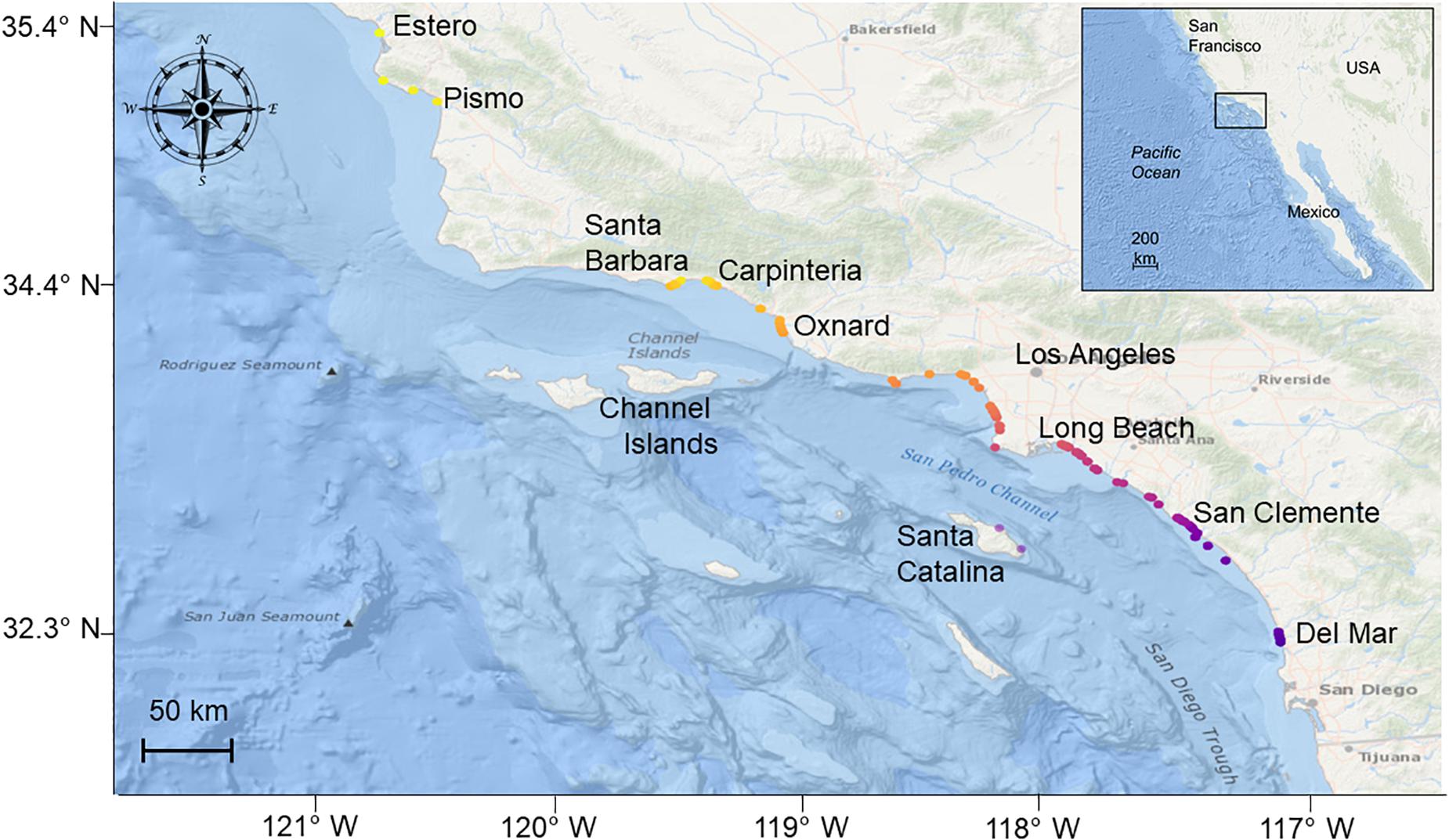
Figure 1. Study area (inset) and locations of all receivers used in the study (2014–2019). Receiver locations are colored according to latitude.
Social Network Construction and Association Definition
A gambit-of-the-group approach (Cairns and Schwager, 1987; Franks et al., 2010) was used to build proximity-based social networks using individual tagged sharks as nodes and strength of association as edges, calculated via the simple ratio index (SRI) using the R packages spatsoc (version 0.1.14; Robitaille et al., 2019) and igraph (version 1.2.5; Csardi and Nepusz, 2006). The SRI score’s an individual’s strength of association between 0 and 1, with 0 indicating no co-occurrence while a score close to 1 indicates a high level of co-occurrence (Aplin et al., 2013). SRI was chosen over other indices (e.g., half-weight index) as the properties and nature of acoustic detection data render them unlikely to violate the assumptions required for SRI: recorded associations are accurate, the probability of identification is independent of whether an individual is associated or not (Stehfest et al., 2013; Lilly et al., 2020). To comply with formatting requirements, detections were rounded to the nearest hour (i.e., hourly presence) and any subsequent detections of the same animal at the same receiver within that hour removed. Animal detections and associated relocations were grouped according to a temporal threshold of 1-h bins (time group), before being spatially grouped according to a threshold of 1 km radius (group). Thus, animals were considered to co-occur in space and time if they were detected at the same receiver within an hour of each other, or at separate receivers within an hour of each other, provided the two receivers were ≤ 1 km from each other. A 1 km spatial threshold was chosen as this allowed for individuals to be considered as being within the same group in instances where they were detected on separate receivers that had overlapping detection ranges, although creating such a spatial buffer introduces the possibility that sharks may be grouped together, or regarded as associating, when in-fact they could be up to 2 km from each other (assuming a 500 m detection range).
Data were then subset by Julian year (2014–2019) before generating networks and associated graphs. Network communities were identified through weighted eigenvector community detection (Newman, 2006), and community modularity (Q, the extent to which communities within a network are distinct from each other) was calculated using the R package igraph. Homophilic propensity, the tendency of individuals to associate with others with similar traits, was examined by calculating assortment coefficients based on estimated shark size. Strength of associations were calculated as edge lists using a temporal based nearest neighbor approach, whereby the nearest neighbor to each individual within each time group was calculated, incorporating a distance threshold of 1 km radius.
Pre-network permutation tests based on mean values from 10,000 randomized networks generated in the R package spatsoc were used to examine whether the observed overall network structure (all years combined) differed from structure of randomized networks. Coefficients of variation (CV’s) of SRI indices between vertices from observed networks were compared to those from the randomized networks using two-sample t-tests.
To further confirm the non-random nature of the observed structure, we used pre-network permutation tests (10,000) for each constructed network in each individual year of the study. CV’s of SRI indices from observed and random networks were compared to examine observed and expected relationships (Bejder et al., 1998; Farine and Whitehead, 2015; Farine et al., 2015), whereby tagged sharks were assumed to exhibit preferred co-occurrences where coefficients of variation from observed networks were greater than 97.5% of coefficients of variations from randomized (permuted) networks (Findlay et al., 2016). It was not possible to replicate this same process for the overall (all years combined) network as it was not possible to account for phenotypic changes in those individuals that were as identified as nodes in networks across more than 1 year.
Association Preference Examination
We used multiple linear regression to examine structural predictors of association strength (strength) in observed networks, with separate models run for each annual network. Predictor variables included estimated shark size-class (size-class), number of detections (detections), detection period (days), and number of acoustic receiver stations visited (statvis). The inclusion of size-class allows for identification of homophilic association preferences, while the inclusion of the number of detections, detection period, and the number of stations visited allows for identification of possible structure inherent to the data. The sex of the shark was not included as a predictor variable due to the number of animals in the study with unconfirmed sex (Supplementary Table 1). The most parsimonious model for each year was identified from a global model via AICc values using the R package glmulti (Calcagno and de Mazancourt, 2010), and confirmed by individually comparing the final model to the next two most parsimonious model iterations. Final models were also compared against null models (strength ∼ 1) to examine significance of predictors used (Supplementary Table 1). The global model was written as strength ∼ size class + statvis + detections + days + detections ∗ days.
A multiple regression quadratic assignment procedure with double-semi-partitioning (MRQAP-DSP; Farine, 2013) was used to further quantify the influence of specific structural factors (i.e., size-class) included in multiple regression models in each year. To examine whether association strength differed for sharks grouped in the same size-class, a binary matrix was constructed that encoded size-class and tested for correlation with a binary version of the SRI matrix using Mantel tests (999 permutations) following the method described by Farine and Whitehead (2015), using the R package vegan (Oksanen et al., 2011). This process was repeated for each yearly dataset (2014–2019).
Results
A total of 86 tagged juvenile white sharks were detected on at least 1 day across the study period (2014–2019) and included in the overall analyses. Of these, 29 (34%) were outfitted with internal tags, while 57 (66%) were tagged externally. Total number of days detected on acoustic receivers and sum detection period varied by shark, ranging from 1 to 424 days detected [66 ± 75 (mean ± SD)], across a sum period of 1–1,216 (265 ± 277) days (Figure 2). A total of 26 sharks were detected in 2 or more calendar years (range 2–4 years, 2.42 ± 0.58). The number of returning tagged sharks showed an increasing trend across the study (Figure 2) and was positively correlated with the cumulative number of sharks tagged (Pearson’s product-moment correlation; r = 0.82, p = 0.048). Only externally tagged sharks were observed to represent nodes in networks in more than 1 year (Supplementary Table 2). Tagged juvenile white sharks exhibited both temporal and spatial overlap in detection patterns, suggesting possible association preferences (Figure 2).
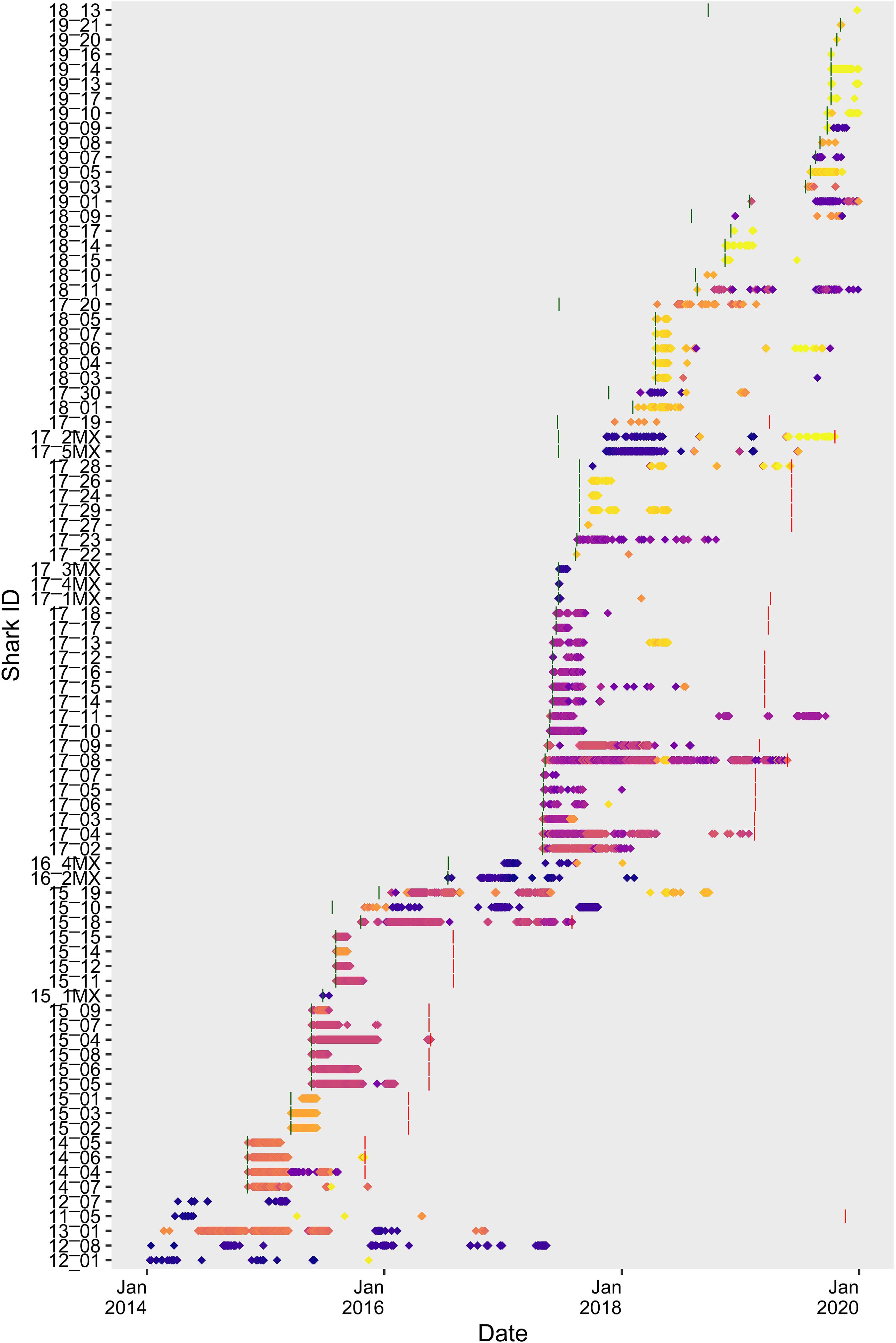
Figure 2. Time series plot showing presence/absence of tagged juvenile white sharks at monitored locations. Points are colored according to individual receiver station latitude demonstrate co-occurrence. Latitudes corresponding to point color are shown in Figure 1. Green and red vertical lines show tagging date and tag-battery life, respectively. Sharks with bars missing were either tagged prior to 2014, or tag-battery life extends beyond 2019.
Social Organization and Association Preference
Overall observed network structure (Figure 3) was compared against a null network generated from 10,000 pre-network permutations based upon the CV’s of individuals, accounting for years in which individual sharks were present in a network. Observed network strength values (mean = 0.36 ± 0.41) were significantly greater than expected values (0.27 ± 0.36; Welch Two Sample t-test; t = −2.513, p = 0.012), indicating there was apparent structure (i.e., association preferences) in juvenile white shark seasonal aggregations. Additionally, CV’s of observed association strength indices vs. randomized networks for each individual year indicated that, with the exception of 2016, observed network structure was significantly stronger than expected from a randomly structured network (Supplementary Figure 1). Thus, co-occurrence of tagged juvenile white sharks at acoustically monitored locations was considered non-random. Community modularity for all detections across all years was high (O̧ = 0.63), which reflects that although communities appear to be connected, they were spatiotemporally distinct with limited individual connections to other communities. Fifty-five of the 86 sharks (64%) included in analyses formed associations with another shark. Thirty-nine of the 86 sharks (45%) were a part of a significant network (Supplementary Table 2), with nine sharks (10%) identified as being part of a significant network in more than 1 year. The number of associations formed by individuals within a single year ranged from 1 to 10 (2.21 ± 1.65). The total number of associations per shark varied both within and between years throughout the study period but showed no discernable trend across the study period (Supplementary Table 1). Mean number of associations per shark was highest in 2015, which is reflected by the associated mean SRI value of 0.7. Lowest mean SRI values were observed in 2016 and 2019 (0.03 and 0.05, respectively), suggesting tagged sharks had low likelihoods of spatiotemporal overlap within acoustically monitored locations in those years (Table 2).
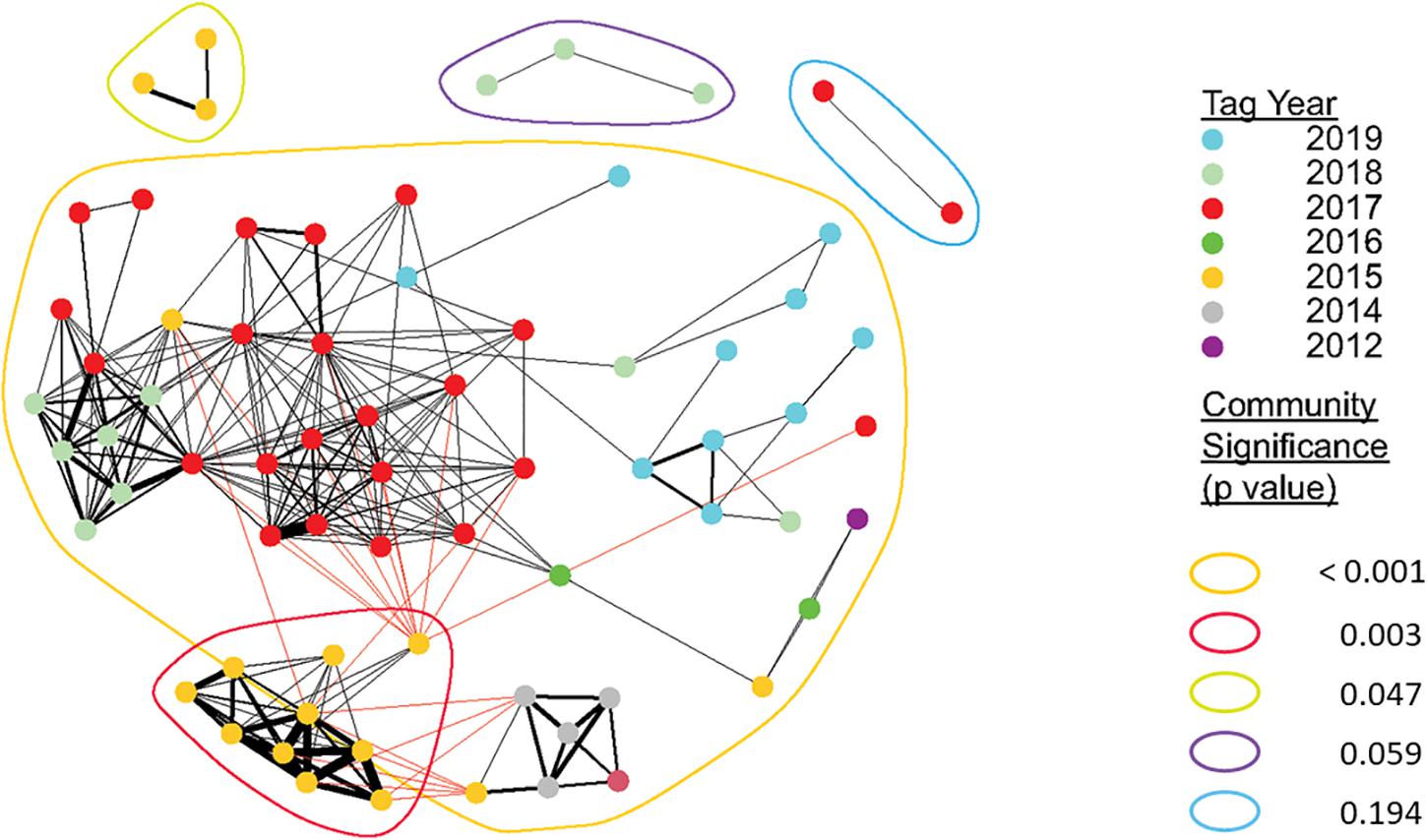
Figure 3. Sociogram showing associations of all tagged juvenile white sharks across all years. Nodes represent individual sharks, node color indicates the year in which they were detected or formed strongest associations (in the case of sharks detected over more than year). Edge thickness indicates the relative number of connections (associations) between nodes. Colors encircling multiple nodes represent identified distinct communities. Detected sharks that did not form dyadic connections to at least one other tagged shark (n = 31) are not shown. Community modularity for all detections across all years was high (Q = 0.63), which reflects that although communities appear to be connected, they were spatiotemporally distinct with limited individual connections to other communities.
The number of associations between sharks was not correlated with individual year (Pearson’s product-moment correlation; r = −0.240, p = 0.105) or with the total number of available (i.e., detectable) tagged sharks (Pearson’s product-moment correlation; r = −0.214, p = 0.148). Node degree, the number of connections an individual has with other sharks, was not found to correlate with the date of first detection, thus there was no relationship between how early in a calendar year a shark was tagged and the number of associations that shark had with other tagged sharks. The exception to this was in 2017, where a positive correlation was observed (Pearson’s product-moment correlation; r = −0.393, p = 0.018), which was likely a function of six sharks with both the highest degree and SRI values being tagged within 1 month of each other (two on the same day, three within 3 days, four within 8 days, five within 22 days). With the exception of 2015, returning sharks were not observed to renew individual associations from the previous year. However, the exception in 2015 is a function of sharks aggregating and forming association preferences in 2014 continuing to do so through the change of year into 2015.
Network analyses based on weighted eigenvector community detection demonstrated that for all years combined (Figure 3), as well as each individual year, some aggregating sharks formed distinct, significant communities (Table 2). In years where spatiotemporally separate aggregations formed, relatively high community modularity was observed, as indicated by high calculated O̧-values (Table 2, Figure 4, and Supplementary Video 1). Similarly, assortment coefficients were observed to be highest in years with higher O̧-values (Table 2). Of the 39 tagged animals that were members of significant communities, 18 were detected in two or more spatially distinct locations (considered separate aggregations) across 2014 (n = 4), 2015 [n = 8 (three sharks from the significant network in 2014)], 2017 (n = 3), 2018 (n = 1), and 2019 (n = 2) (Supplementary Table 2). Sixteen sharks were identified as being members of significant communities across two or more years. Fourteen were sharks identified in communities in two separate years. Two sharks (Shark IDs 15_19 and 17_08) were part of significant communities in three separate years (2016, 2017, 2018 and 2017, 2018, 2019, respectively).
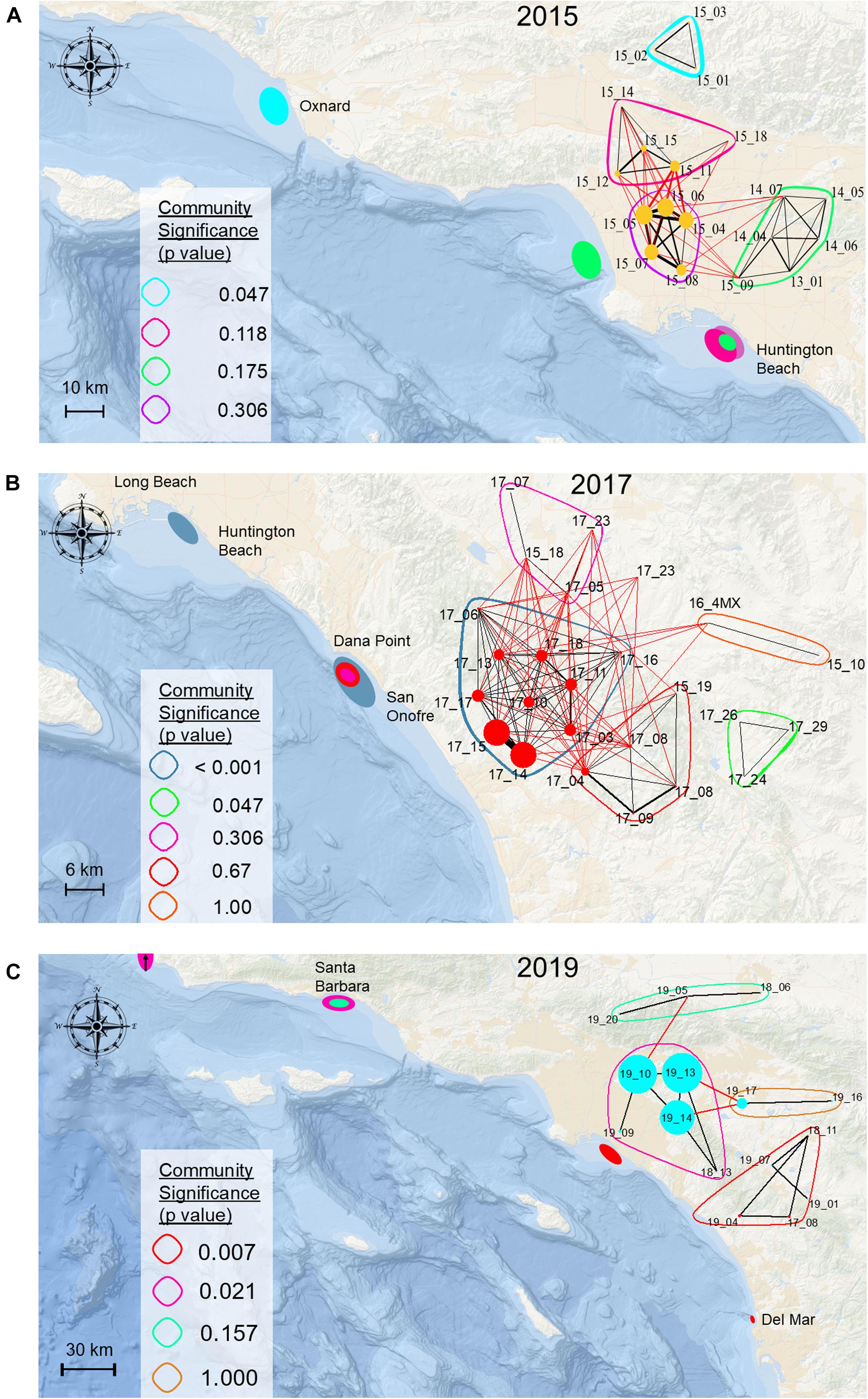
Figure 4. Maps depicting community modularity in observed networks for 2015 (A), 2017 (B), and 2019 (C). Community composition and corresponding discrete spatial locations of specific network communities are reflected in coloration of bands surrounding network nodes, and oval shaped markers within map coastal locations.
Observed Social Structure and Size Class
General linear models were run for each year in the dataset to examine potential predictors of association strength. For all years, the best fitting model was strength ∼ size class + detections∗days (Figure 5 and Supplementary Table 3). The interaction term of detections∗days was significant in all years for which it was included, except for 2018, where the interaction was not significant and these terms were removed, and the model re-run (Carey, 2013). Model correlation coefficients ranged from 0.19 to 0.83 (0.52 ± 0.23). The interaction term and response variable (association strength) were found to be strongly collinear across all models where included and is reflected by high associated variance inflation factors (Figure 5). Shark size-class was included in all final models, and was found to be a significant predictor of association for 3 of 6 final models [2017, 2018, and 2019 (Figure 5)]. The results from MRQAP-DSP regression indicated that shark size-class was a significant predictor of co-occurrence in 3 of 6 yearly datasets examined (2015, 2017, 2019; Table 3). Tagged shark co-occurrence was found to be significantly correlated with shark size-class in those same years [Mantel tests: (2015) r = 0.332, p = 0.001; (2017) r = 0.08, p = 0.04; (2019) r = 0.144, p = 0.014; Table 4].
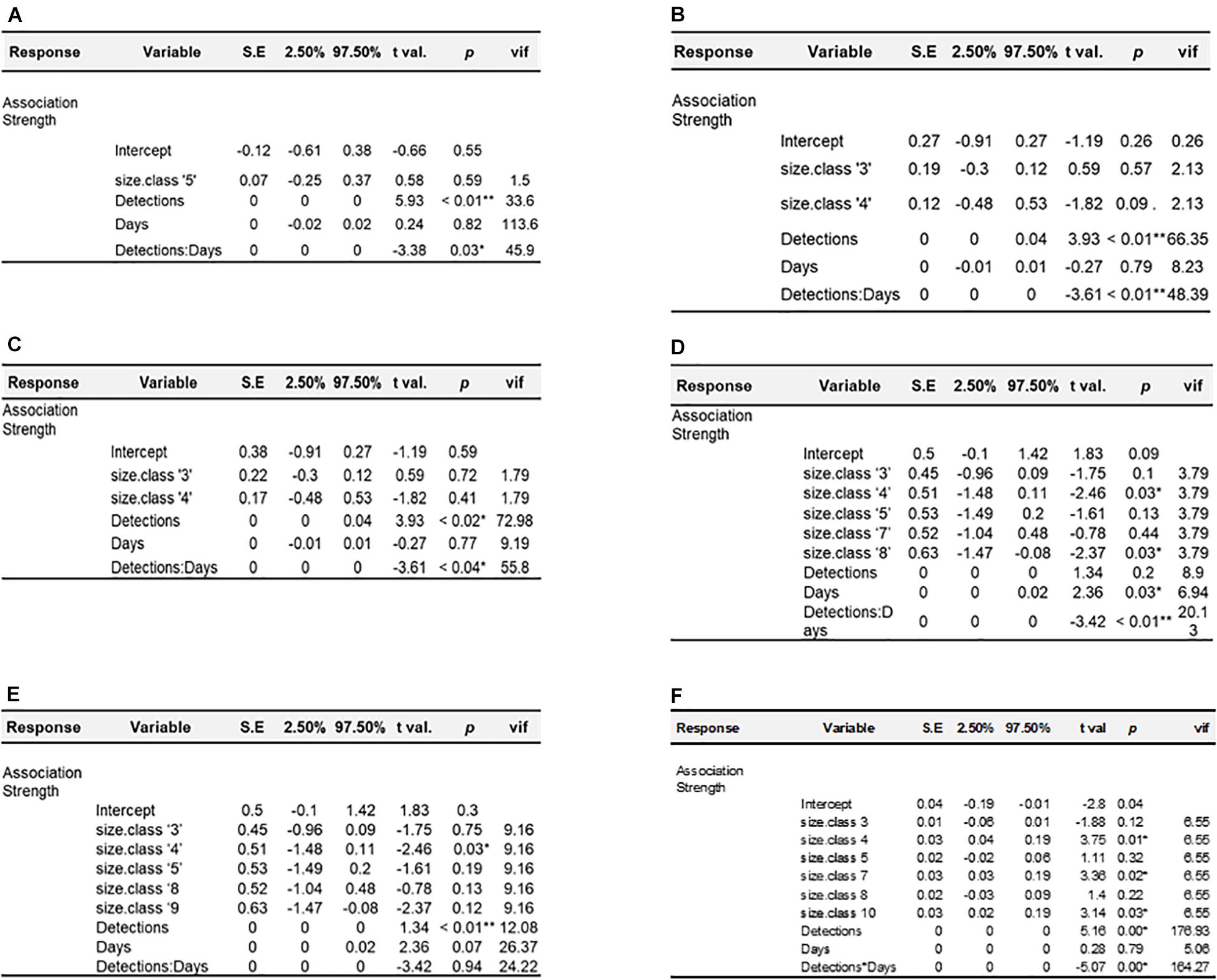
Figure 5. Results of multiple linear regression analyses. Each panel shows model results for the corresponding year; (A) 2014, (B) 2015, (C) 2016, (D) 2017, (E) 2018, (F) 2019.
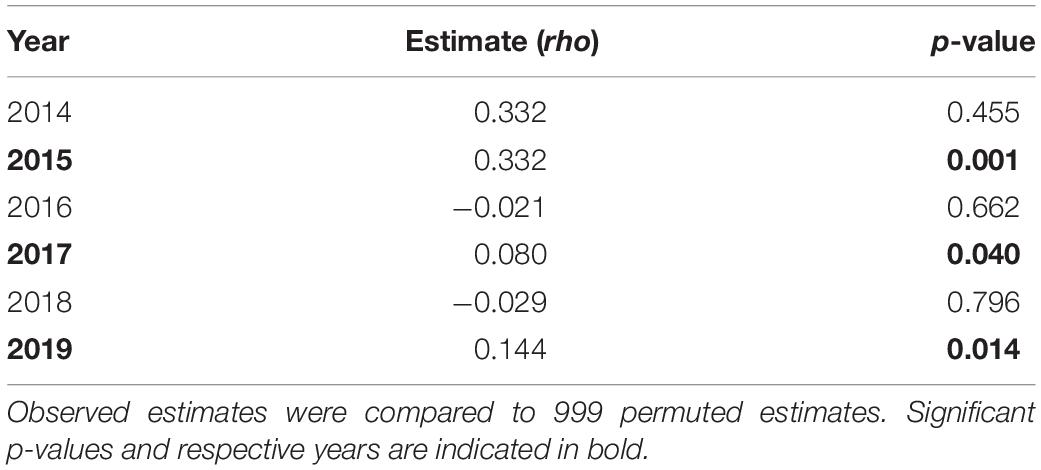
Table 4. Results of Mantel tests for correlation between yearly simple ratio index (SRI) matrices and shark size-class.
Discussion
Juvenile white sharks in southern California are known to seasonally aggregate in annually variable, spatially discrete nearshore locations (Lyons et al., 2013; White et al., 2019; Anderson et al., 2021a). The drivers behind these aggregation “hot spot” patterns, and the selection of specific habitat locations over an abundance of comparable available habitat remain unclear, and are not consistently explained by environmental correlates (Spaet J. et al., 2020; Anderson et al., 2021a). While the study included detection data from tagged juvenile white sharks at locations across southern California, a majority of annual detections and co-occurrences of tagged sharks occurred at seasonal aggregation hot spots, as indicated in Figures 2, 4. It must be acknowledge that a majority of tagged sharks included in the study (65%) were tagged externally, and the majority of these sharks were tagged at known aggregation locations (see Supplementary Table 1 for tagging locations). Thus, to some extent, detection patterns and observed network structures may be a function of tagging methodologies used. However, where this is the case, the patterns in the detection data also reflect that these sharks had already formed aggregations and may have also already formed preferential associations with individual conspecifics present within the aggregation. For instance, individuals caught offshore by commercial fishers were less likely to be found within nearshore aggregations. Individuals that spatiotemporally overlap each other are more likely to be associated with each other, due to inherently greater probability of co-occurrence. Pre-network (data stream) permutations can be used to examine whether a metric of interest (in this case observed association strength) differs to that which might occur at random. Our comparison of observed and permuted association strengths demonstrated that except for 2016 (a notably data deficient year) observed association preferences differed significantly to expected values, indicating apparent co-occurrence of individuals in space and time is non-random (Supplementary Figure 1). Observed relative association strengths in animal social networks may be a function of resource availability or site fidelity (Lusseau et al., 2006; Armansin et al., 2016). Thus, we incorporated methods that aimed to discern whether observed network structure could be explained by social factors, or were likely driven by other forcing (e.g., environmental conditions and/or resource availability).
Assortativity and Community Structure
Animals that exhibit homophilic association preferences may do so with respect to phenotypic characteristics such as species, sex, size, and kinship (Mourier and Planes, 2021). As animal size was the only phenotypic characteristic we were able to consistently gather, we were restricted to using size-class as a metric of assortment. Although the resulting assortativity coefficients were variable, our analyses suggest the likelihood of association of tagged juvenile white sharks is greater where sharks are of similar size-classes. A comparable relationship was described in a study of juvenile white sharks in South Africa (Findlay et al., 2016). The low variance explained by our multiple regression and MRQAP analyses indicates community structure was also driven by other factors we were unable to quantify, which could include spatiotemporal overlap due to environmental factors and/or resource availability, as well as sampling effects. However, black tip reef sharks (Carcharhinus melanopterus) have been documented to exhibit preferential associations to conspecifics of similar size and sex regardless of prey availability (Mourier et al., 2012), which lends support to apparent preferential association toward conspecifics of similar size in the current study.
Shark Co-occurrence and Group Dynamics
Individuals in observed networks exhibit behaviors that best approximate fission-fusion dynamics (formation and dissolution of groups over time) with spatiotemporally unstable group membership. Fission-fusion dynamics are common in animal groups across taxa, including elasmobranchs (Haulsee et al., 2016; Perryman et al., 2019; Papastamatiou et al., 2020), and are regarded as being advantageous in the exploitation of heterogeneous environments (Ramos-Fernández and Morales, 2014; Silk et al., 2014; Farine et al., 2015). Dyadic relationships seen between individual juvenile white sharks in our study are not represented by predictable or stable co-occurrence (association) as might be expected by animals that exhibit central-place foraging behavioral characteristics. Rather, the dyadic patterns observed in our study suggest individuals co-occur (fusion) punctuated by variable spatiotemporal intervals (fission). Such behaviors may be a function of exploitation of shared resources (e.g., food). A recent study of Australasian gannets (Morus serrator) determined that social associations in the study population were context dependent, and were most prevalent during foraging (constituting local enhancement). These associations were also most prevalent in regions where resources were clustered (Jones et al., 2020). Southern California juvenile white shark aggregation hot spots have been largely associated with shallow, sandy beach habitat, although across southern California, such habitat constitutes < 30% of total available shore type (Anderson et al., 2021a). Although we were not able to quantify resource homogeneity at aggregation hot spots, hot spot locations themselves were annually spatially variable. If spatial locations of aggregation hot spots are resource driven, we can expect there to be a comparatively higher degree of resource clustering at hot spot locations with respect to ostensibly similar neighboring habitat. Juvenile white sharks in the study were observed to co-occur at different receivers both within the same spatiotemporal aggregation, as well as separate, spatially discrete locations and aggregations within the same year (Supplementary Video 1). Although this behavior, which linked nodes between network communities, could simply indicate individuals at similar ontogenetic stages were responding in similar ways to the same environmental cues, it may also be attributable to following behaviors and association borne from local enhancement, where individuals are attracted to actively foraging conspecifics (Poysa, 1992). Thus, the interplay of environmental drivers and context-specific social behaviors may govern co-occurrence and apparent association of individual juvenile white sharks in the study.
Although 10% of tagged sharks were identified at the same locations across more than 1 year, there was no evidence of stability in structure across multiple years, as returning sharks did not form repeated associations at monitored locations in subsequent years. Juvenile white sharks aggregating at nearshore locations, including those in this study, have been demonstrated to exhibit overlapping, restricted area use (Lyons et al., 2013; Anderson et al., 2021a). An individual’s tendency toward association may be indicated by the propensity for spatiotemporal overlap and successive return to the same locations (Klimley and Holloway, 1999; Lilly et al., 2020), a trait exhibited in juvenile white sharks (Bruce et al., 2019; Spaet J. L. Y. et al., 2020; Anderson et al., 2021a). Observations of juvenile white shark interactions derived from drone surveys undertaken during the study period (Rex et al., unpublished data) indicate that individuals may often be within 10–20 m of each other while in these loose aggregations, and do not appear to be schooling in the manner seen in other aggregative shark species [e.g., scalloped hammerhead sharks (Sphyrna lewini), black tip reef sharks (Carcharhinus melanopterus)]. In addition, observations of these interactions suggest the presence of dominance hierarchies and conspecific aggression. Although tagged sharks generally exhibited low frequency of associations with other tagged conspecifics (Supplementary Table 1), the strength of those associations, as indicated by SRI indices, were relatively strong, with the exception of sharks in 2016 and 2019, which were both years with low mean and total associations (Table 1 and Supplementary Table 1). Individuals within heterogeneous environments likely do not experience equal benefits from local enhancement and information derived from social behaviors and co-occurrence (Jones et al., 2020). Thus, fission may not be a singular synchronous event, but rather an ongoing social construct governed by intrinsic plasticity. Aggregation dynamics in juvenile white sharks may therefore be governed by the interplay of resource quality, environmental heterogeneity, and the continuous evaluation of the cost-benefit relationship of sociality. This can be likened to the hypothesis of the ecological loop that affects dispersal, proposed by Bowler and Benton (2005), whereby the size of a population (in this case at an aggregation site), which is mediated by the environment (which varies in space and time), governs interactions between individuals for resources.
Although sociality has been examined and described in a number of elasmobranch species (Sims et al., 2000; Jacoby et al., 2010, 2011, 2016; Guttridge et al., 2013; Wilson et al., 2014, 2015; Armansin et al., 2016; Mourier et al., 2019; Perryman et al., 2019; Schilds et al., 2019; Mourier and Planes, 2021; Papastamatiou et al., 2020), the extent to which co-occurrence in white sharks as a function of social forcing factors is unknown. The difficulty of interpreting the true level of association between animals is a function of the data collection method, and the spatiotemporal thresholds used. For example, Schilds et al. (2019) used photographic identification of individuals present at a baited location within the same day as a means of identifying co-occurrence, thus arrival of connected nodes at the monitored location could potentially be hours apart. In the present study, shark presence and associations at monitored aggregation locations may be under-estimates as they only reflect tagged sharks within spatially discrete locations and time thresholds (1 h), which may better represent natural co-occurrence. However, the potential for temporally co-occurring individuals to be up to 2 km apart from each other in some instances, due to the nature of acoustic telemetered data, may also represent over-estimates of spatio-temporal co-occurrence.
While the primary drivers behind co-occurrence in shark species may be extrinsic factors such as resource availability and use, additional adaptive benefits of social interactions beyond direct biological benefits (e.g., increased opportunity for reproduction) have been identified and described (e.g., Jacoby et al., 2010; Mourier et al., 2012, 2019; Armansin et al., 2016; Jacoby and Freeman, 2016; Mourier and Planes, 2021; Papastamatiou et al., 2020). Papastamatiou et al. (2020) concluded that for sharks exhibiting central place foraging life history characteristics, information transfer during social foraging increased foraging efficiency and may lead to temporally stable social groups across years. Other shark species that exhibit more solitary life histories but aggregate seasonally at feeding sites may also exhibit preferential associations based on phenotypic characteristics (Findlay et al., 2016; Haulsee et al., 2016).
For naïve individuals, information transfer facilitates exploitation of patchy resources already identified by others (Aplin et al., 2013). The specific drivers governing where and when juvenile white sharks aggregate in southern California have yet to be defined but are thought to include water temperature and resource availability (White et al., 2019; Anderson et al., 2021a), and decisions by individual sharks to remain within a specific aggregation location may therefore reflect resource quality. Thus, perceived habitat quality derived from cues generated by both con and hetero-specifics, as well as transfer of information (e.g., following of “experienced” individuals by naïve individuals) within aggregation locations may lead to increased likelihood of association at a given location.
Conclusion
Although individual presence at aggregation sites may be driven by environmental cues and resource availability, the tendency of individuals to remain within aggregations may be the result of conscious behaviors, potentially driven by sociality, which may stem from naïve individuals acquiring information and experiencing local enhancement. To better understand the dynamics of associations between individuals, far higher resolution data is required. This could be achieved through the use of high-density acoustic arrays across much smaller spatial scales, specifically centered at aggregation hot spots [e.g., VPS (Vemco Positioning System) arrays], where the movements of individuals in three-dimensional space throughout an array can be achieved via trilateration of acoustic tag transmissions or by using proximity-based tags. Such studies would provide a more objective means of quantifying co-occurrence, fusion, and fission between individuals and groups, and elucidate the potential social dynamics that may govern space use and niche exploitation at juvenile white shark aggregation locations.
Data Availability Statement
The raw data supporting the conclusions of this article will be made available by the authors, without undue reservation.
Ethics Statement
The animal study was reviewed and approved by the CSULB Institutional Animal Care and Use Committee.
Author Contributions
JA, AC, EB, and CL conceived the study. CL, EB, EM, CFW, RL, CW, PR, JM, KL, JA, AC, EG-R, and OS-N tagged the sharks and collected the data. CFW, EB, EM, BS, JA, PR, and JM organized the data. JA analyzed the data, created the main figures, and drafted the manuscript. All authors contributed equally to manuscript revisions.
Funding
Funding for tagging and acoustic monitoring was provided by Monterey Bay Aquarium and AltaSea. Funding for data maintenance and analysis was provided by State of California. In-kind support for tagging and monitoring was provided by Los Angeles and Orange County Lifeguards, Cities of Huntington Beach, Seal Beach, Ventura, Santa Barbara, Newport Beach, Laguna, Long Beach, and San Clemente.
Conflict of Interest
CW was the Principal of the company Aquatic Research Consultants.
The remaining authors declare that the research was conducted in the absence of any commercial or financial relationships that could be construed as a potential conflict of interest.
Publisher’s Note
All claims expressed in this article are solely those of the authors and do not necessarily represent those of their affiliated organizations, or those of the publisher, the editors and the reviewers. Any product that may be evaluated in this article, or claim that may be made by its manufacturer, is not guaranteed or endorsed by the publisher.
Supplementary Material
The Supplementary Material for this article can be found online at: https://www.frontiersin.org/articles/10.3389/fmars.2021.688505/full#supplementary-material
References
Alexander, R. D. (1974). The evolution of social behavior. Annu. Rev. Ecol. Syst. 5, 325–383. doi: 10.1146/annurev.es.05.110174.001545
Anderson, J. M., Burns, E. S., Meese, E. N., Farrugia, T. J., Stirling, B. S., White, C. F., et al. (2021a). Interannual nearshore habitat use of young of the year white sharks off Southern California. Front. Mar. Sci. 8:645142. doi: 10.3389/fmars.2021.645142
Anderson, J. M., Spurgeon, E. A., Stirling, B. S., May, J. I., Rex, P. T., Hyla, B., et al. (2021b). High resolution acoustic telemetry reveals swim speeds and inferred field metabolic rates in juvenile white sharks (Carcharadon carcharias). Commun. Biol.
Aplin, L. M., Farine, D. R., Morand-Ferron, J., Cole, E. F., Cockburn, A., and Sheldon, B. C. (2013). Individual personalities predict social behaviour in wild networks of great tits (Parus major). Ecol. Lett. 16, 1365–1372. doi: 10.1111/ele.12181
Armansin, N. C., Lee, K. A., Huveneers, C., and Harcourt, R. G. (2016). Integrating social network analysis and fine-scale positioning to characterize the associations of a benthic shark. Anim. Behav. 115, 245–258. doi: 10.1016/j.anbehav.2016.02.014
Bejder, L., Fletcher, D., and Brager, S. (1998). A method for testing association patterns of social animals. Anim. Behav. 56, 719–725. doi: 10.1006/anbe.1998.0802
Berdahl, A. M., Kao, A. B., Biro, D., Flack, A., Westley, P. A. H., Codling, E. A., et al. (2018). Collective animal navigation and migratory culture: from theoretical models to empirical evidence. Philos. Trans. R. Soc. Lond. B Biol. Sci. 373:20170009. doi: 10.1098/rstb.2017.0009
Bruce, B. D., and Bradford, R. W. (2008). Spatial dynamics and habitat preferences of juvenile white sharks – identifying critical habitat and options for monitoring recruitment. Hobart, TAS: CSIRO Marine and Atmospheric Research.
Bruce, B. D., Harasti, D., Lee, K., Gallen, C., and Bradford, R. (2019). Broad-scale movements of juvenile white sharks Carcharodon carcharias in eastern Australia from acoustic and satellite telemetry. Mar. Ecol. Prog. Ser. 619, 1–5. doi: 10.3354/meps12969
Bruce, B. D., Stevens, J. D., and Malcolm, H. (2006). Movements and swimming behaviour of white sharks (Carcharodon carcharias) in Australian waters. Mar. Biol. 150, 161–172. doi: 10.1007/s00227-006-0325-1
Cailliet, G. M., Natanson, L. J., Weldon, B. A., and Ebert, D. (1985). Preliminary studies on the age and growth of the white shark Carcharodon carcharias, using vertebral bands. Bull. South. Calif. Acad. Sci. Mem. 9, 49–60.
Cairns, S. J., and Schwager, S. J. (1987). A comparison of association indices. Anim. Behav. 35, 1454–1469. doi: 10.1016/S0003-3472(87)80018-0
Calcagno, V., and de Mazancourt, C. (2010). glmulti: An R Package for easy automated model selection with (Generalized) Linear Models. J. Stat. Software 34:63083.
Campbell, L. A. D., Tkaczynski, P. J., Lehmann, J., Mouna, M., and Majolo, B. (2018). Social thermoregulation as a potential mechanism linking sociality and fitness: Barbary macaques with more social partners form larger huddles. Sci. Rep. 8:6074. doi: 10.1038/s41598-018-24373-4
Carey, G. (2013). Quantitative Methods in Neuroscience. Boulder, CO: University of Colorado, Boulder.
Chapman, D. D., Babcock, E. A., Gruber, S. H., Dibattista, J. D., Franks, B. R., Kessel, S. A., et al. (2009). Long-term natal site-fidelity by immature lemon sharks (Negaprion brevirostris) at a subtropical island. Mol. Ecol. 18, 3500–3507. doi: 10.1111/j.1365-294X.2009.04289.x
Clevenstine, A. J., and Lowe, C. G. (2021). Aggregation site fidelity and movement patterns of the protected marine predator giant sea bass (Stereolepis gigas). Environ. Biol. Fishes 104, 401–417. doi: 10.1007/s10641-021-01077-9
Compagno, L. (2002). Sharks of the world: an annotated and illustrated catalogue of shark species known to date. Bullhead, mackerel andcarpet sharks (Heterodontiformes, Lamniformes and Orectolobiformes), Vol. 2. Rome: Food and Agriculture Organization of the United Nations.
Csardi, G., and Nepusz, T. (2006). The igraph software package for complex network research. InterJournal Complex Syst. 1695, 1–9.
Curtis, T. H., Metzger, G., Fischer, C., McBride, B., McCallister, M., Winn, L. J., et al. (2018). First insights into the movements of young-of-the-year white sharks (Carcharodon carcharias) in the western North Atlantic Ocean. Sci. Rep. 8:10794. doi: 10.1038/s41598-018-29180-5
Diaz-Aguirre, F., Parra, G. J., Passadore, C., and Möller, L. (2019). Genetic relatedness delineates the social structure of southern Australian bottlenose dolphins. Behav. Ecol. 30, 948–959. doi: 10.1093/beheco/arz033
Dicken, M. L., and Booth, A. J. (2013). Surveys of white sharks (Carcharodon carcharias) off bathing beaches in Algoa Bay, South Africa. Mar. Freshw. Res. 64:530. doi: 10.1071/MF12336
Domeier, M., and Nasby-Lucas, N. (2008). Migration patterns of white sharks Carcharodon carcharias tagged at Guadalupe Island, Mexico, and identification of an eastern Pacific shared offshore foraging area. Mar. Ecol. Prog. Ser. 370, 221–237. doi: 10.3354/meps07628
Duffy, C., Francis, M. P., and Bonfil, R. (2012). “Regional population connectivity, oceanic habitat, and return migration revealed by sat-ellite tagging of white sharks, Carcharodon carcharias, at NewZealand aggregation sites,” in Global perspectiveson the biology and life history of the white shark, ed. M. L. Domeier (Boca Raton, FL: CRC Press), 310–318.
Farine, D. R. (2013). Animal social network inference and permutations for ecologists in R using asnipe. Methods Ecol. Evol. 4, 1187–1194. doi: 10.1111/2041-210X.12121
Farine, D. R., Firth, J. A., Aplin, L. M., Crates, R. A., Culina, A., Garroway, C. J., et al. (2015). The role of social and ecological processes in structuring animal populations: A case study from automated tracking of wild birds. R. Soc. Open Sci. 2:150057. doi: 10.1098/rsos.150057
Farine, D. R., and Whitehead, H. (2015). Constructing, conducting and interpreting animal social network analysis. J. Anim. Ecol. 84, 1144–1163. doi: 10.1111/1365-2656.12418
Findlay, R., Gennari, E., Cantor, M., and Tittensor, D. P. (2016). How solitary are white sharks: social interactions or just spatial proximity? Behav. Ecol. Sociobiol. 70, 1735–1744. doi: 10.1007/s00265-016-2179-y
Franks, D. W., Ruxton, G. D., and James, R. (2010). Sampling animal association networks with the gambit of the group. Behav. Ecol. Sociobiol. 64, 493–503. doi: 10.1007/s00265-009-0865-8
Grueter, C. C., Qi, X., Zinner, D., Bergman, T., Li, M., Xiang, Z., et al. (2020). Multilevel organisation of animal sociality. Trends Ecol. Evol. 35:24. doi: 10.1016/j.tree.2020.05.003
Guttridge, T. L., van Dijk, S., Stamhuis, E. J., Krause, J., Gruber, S. H., and Brown, C. (2013). Social learning in juvenile lemon sharks, Negaprion brevirostris. Anim. Cogn. 16, 55–64. doi: 10.1007/s10071-012-0550-6
Harasti, D., Lee, K., Bruce, B., Gallen, C., and Bradford, R. (2017). Juvenile white sharks Carcharodon carcharias use estuarine environments in south-eastern Australia. Mar. Biol. 164, 1–14. doi: 10.1007/s00227-017-3087-z
Haulsee, D. E., Fox, D. A., Breece, M. W., Brown, L. M., Kneebone, J., Skomal, G. B., et al. (2016). Social network analysis reveals potential fission-fusion behavior in a shark. Sci. Rep. 6, 1–9. doi: 10.1038/srep34087
Heupel, M. R., Carlson, J. K., and Simpfendorfer, C. A. (2007). Shark nursery areas: Concepts, definition, characterization and assumptions. Mar. Ecol. Prog. Ser. 337, 287–297. doi: 10.3354/meps337287
Heupel, M. R., Semmens, J. M., and Hobday, A. J. (2006). Automated acoustic tracking of aquatic animals: scales, design and deployment of listening station arrays. Mar. Freshw. Res. 57:91. doi: 10.1071/MF05091
Huveneers, C., Simpfendorfer, C. A., Kim, S., Semmens, J. M., Hobday, A. J., Pederson, H., et al. (2016). The influence of environmental parameters on the performance and detection range of acoustic receivers. Methods Ecol. Evol. 7, 825–835. doi: 10.1111/2041-210X.12520
Jacoby, D. M. P., Busawon, D. S., and Sims, D. W. (2010). Sex and social networking: the influence of male presence on social structure of female shark groups. Behav. Ecol. 21, 808–818. doi: 10.1093/beheco/arq061
Jacoby, D. M. P., Croft, D. P., and Sims, D. W. (2011). Social behaviour in sharks and rays: analysis, patterns and implications for conservation. Fish Fish. 13, 399–417. doi: 10.1111/j.1467-2979.2011.00436.x
Jacoby, D. M. P., and Freeman, R. (2016). Emerging network-based tools in movement ecology. Trends Ecol. Evol. 31, 301–314. doi: 10.1016/j.tree.2016.01.011
Jacoby, D. M. P., Papastamatiou, Y. P., and Freeman, R. (2016). Inferring animal social networks and leadership: Applications for passive monitoring arrays. J. R. Soc. Interface 13:20160676. doi: 10.1098/rsif.2016.0676
Jones, T. B., Green, J. A., Patrick, S. C., Evans, J. C., Wells, M. R., Rodr Iguez-Malag, M. A., et al. (2020). Consistent sociality but flexible social associations across temporal and spatial foraging contexts in a colonial breeder. Ecol. Lett. 23, 1085–1096. doi: 10.1111/ele.13507
Jorgensen, S. J., Chapple, T. K., Anderson, S., Hoyos, M., Reeb, C., and Block, B. A. (2010). “Connectivity among White Shark Coastal aggregation areas in the Northeastern pacific,” in Global Perspectives on the Biology and Life History of the White Shark, ed. M. L. Domeier (Boca Raton, FL: CRC Press), 159–168.
Kanive, P. E., Rotella, J. J., Chapple, T. K., Anderson, S. D., White, T. D., Block, B. A., et al. (2021). Estimates of regional annual abundance and population growth rates of white sharks off central California. Biol. Conserv. 257:109104. doi: 10.1016/j.biocon.2021.109104
Kessel, S. T., Cooke, S. J., Heupel, M. R., Hussey, N. E., Simpfendorfer, C. A., Vagle, S., et al. (2014). A review of detection range testing in aquatic passive acoustic telemetry studies. Rev. Fish. Biol. Fish. 24, 199–218. doi: 10.1007/s11160-013-9328-4
Klimley, A. P., and Holloway, C. F. (1999). School fidelity and homing synchronicity of yellowfin tuna, Thunnus albacares. Mar. Biol. 133, 307–317. doi: 10.1007/s002270050469
Kock, A., O’Riain, M. J., Mauff, K., Meÿer, M., Kotze, D., and Griffiths, C. (2013). Residency, habitat use and sexual segregation of white sharks, carcharodon carcharias in False Bay, South Africa. PLoS One 8:e55048. doi: 10.1371/journal.pone.0055048
Kock, A. A., Photopoulou, T., Durbach, I., Mauff, K., Meÿer, M., Kotze, D., et al. (2018). Summer at the beach: Spatio-temporal patterns of white shark occurrence along the inshore areas of False Bay, South Africa. Mov. Ecol. 6, 1–13. doi: 10.1186/s40462-018-0125-5
Lilly, J., McLean, M. F., Dadswell, M. J., Wirgin, I., Comolli, P., and Stokesbury, M. J. W. (2020). Use of social network analysis to examine preferential co-occurrences in Atlantic Sturgeon Acipenser oxyrinchus oxyrinchus Mitchill, 1815. Anim. Biotelemetry 8:14. doi: 10.1186/s40317-020-00201-3
Lusseau, D., Wilson, B., Hammond, P. S., Grellier, K., Durban, J. W., Parsons, K. M., et al. (2006). Quantifying the influence of sociality on population structure in bottlenose dolphins. J. Anim. Ecol. 75, 14–24. doi: 10.1111/j.1365-2656.2005.01013.x
Lyons, K., Jarvis, E. T., Jorgensen, S. J., Weng, K., O’sullivan, J., Winkler, C., et al. (2013). The degree and result of gillnet fishery interactions with juvenile white sharks in southern California assessed by fishery-independent and-dependent methods. Fish. Res. 147, 370–380. doi: 10.1016/j.fishres.2013.07.009
May, C., Meyer, L., Whitmarsh, S., and Huveneers, C. (2019). Eyes on the size: Accuracy of visual length estimates of white sharks, Carcharodon carcharias. R. Soc. Open Sci. 6:190456. doi: 10.1098/rsos.190456
Merriam-Webster. (2021). Sociality. Available Online at: https://www.merriam-webster.com/dictionary/sociality [Accessed March 3, 2021]
Meese, E. N., and Lowe, C. G. (2019). Finding a resting place: How environmental conditions influence the habitat selection of resting batoids. Bull. South. California Acad. Sci. 118:87. doi: 10.3160/0038-3872-118.2.87
Mourier, J., Lédée, E. J. I., and Jacoby, D. M. P. (2019). A multilayer perspective for inferring spatial and social functioning in animal movement networks. bioRxiv 2019:749085. doi: 10.1101/749085
Mourier, J., and Planes, S. (2021). Kinship does not predict the structure of a shark social network. Behav. Ecol. 32, 211–222. doi: 10.1093/beheco/araa119
Mourier, J., Vercelloni, J., and Planes, S. (2012). Evidence of social communities in a spatially structured network of a free-ranging shark species. Anim. Behav. 83, 389–401. doi: 10.1016/j.anbehav.2011.11.008
Newman, M. E. J. (2002). Assortative mixing in networks. Phys. Rev. Lett. 89:208701. doi: 10.1103/PhysRevLett.89.208701
Newman, M. E. J. (2006). Finding community structure in networks using the eigenvectors of matrices. Phys. Rev. E 74:036104. doi: 10.1103/PhysRevE.74.036104
Noldus, R., and Van Mieghem, P. (2015). Assortativity in complex networks. J. Complex Networks 3, 507–542. doi: 10.1093/comnet/cnv005
Oksanen, J., Blanchet, F. G., Friendly, M., Kindt, R., Legendre, P., McGlinn, D., et al. (2011). vegan: Community Ecology Package. Available online at: https://CRAN.R-project.org/package=vegan
Oñate-González, E. C., Sosa-Nishizaki, O., Herzka, S. Z., Lowe, C. G., Lyons, K., Santana-Morales, O., et al. (2017). Importance of bahia sebastian vizcaino as a nursery area for white sharks (Carcharodon carcharias) in the Northeastern Pacific: A fishery dependent analysis. Fish. Res. 188, 125–137. doi: 10.1016/j.fishres.2016.12.014
Papastamatiou, Y. P., Bodey, T. W., Caselle, J. E., Bradley, D., Freeman, R., Friedlander, A. M., et al. (2020). Multiyear social stability and social information use in reef sharks with diel fission-fusion dynamics: Shark sociality and information use. Proc. R. Soc. B Biol. Sci. 287:9. doi: 10.1098/rspb.2020.1063
Perryman, R. J. Y., Venables, S. K., Tapilatu, R. F., Marshall, A. D., Brown, C., and Franks, D. W. (2019). Social preferences and network structure in a population of reef manta rays. Behav. Ecol. Sociobiol. 73, 1–18. doi: 10.1007/s00265-019-2720-x
Poysa, H. (1992). Group foraging in patchy environments: the importance of coarse- level local enhancement. Ornis Scand. 23, 159–166. doi: 10.2307/3676444
Ramos-Fernández, G., and Morales, J. M. (2014). Unraveling fission-fusion dynamics: how subgroup properties and dyadic interactions influence individual decisions. Behav. Ecol. Sociobiol. 68, 1225–1235. doi: 10.1007/s00265-014-1733-8
Raoult, V., Williamson, J. E., Smith, T. M., and Gaston, T. F. (2019). Effects of on-deck holding conditions and air exposure on post-release behaviours of sharks revealed by a remote operated vehicle. J. Exp. Mar. Bio. Ecol. 511, 10–18. doi: 10.1016/j.jembe.2018.11.003
Robbins, R. (2007). Environmental variables affecting the sexual segregation of great white sharks Carcharodon carcharias at the Neptune Islands South Australia. J. Fish Biol. 70, 1350–1364. doi: 10.1111/j.1095-8649.2007.01414.x
Robitaille, A. L., Webber, Q. M. R., and Vander Wal, E. (2019). Conducting social network analysis with animal telemetry data: Applications and methods using spatsoc. Methods Ecol. Evol. 10, 1203–1211. doi: 10.1111/2041-210X.13215
Sabol, A. C., Lambert, C. T., Keane, B., Solomon, N. G., and Dantzer, B. (2020). How does individual variation in sociality influence fitness in prairie voles? Anim. Behav. 163, 39–49. doi: 10.1016/j.anbehav.2020.02.009
Schilds, A., Mourier, J., Huveneers, C., Nazimi, L., Fox, A., and Leu, S. T. (2019). Evidence for non-random co-occurrences in a white shark aggregation. Behav. Ecol. Sociobiol. 73, 1–12. doi: 10.1007/s00265-019-2745-1
Sequeira, A. M. M., Thums, M., Brooks, K., and Meekan, M. G. (2016). Error and bias in size estimates of whale sharks: Implications for understanding demography. R. Soc. Open Sci. 3:150668. doi: 10.1098/rsos.150668
Silk, M. J., Croft, D. P., Tregenza, T., and Bearhop, S. (2014). The importance of fission-fusion social group dynamics in birds. Ibis (Lond). 156, 701–715. doi: 10.1111/ibi.12191
Simpfendorfer, C. A., Huveneers, C., Steckenreuter, A., Tattersall, K., Hoenner, X., Harcourt, R., et al. (2015). Ghosts in the data: False detections in VEMCO pulse position modulation acoustic telemetry monitoring equipment. Anim. Biotelemetry 3:55. doi: 10.1186/s40317-015-0094-z
Simpfendorfer, C. A., and Milward, N. E. (1993). Utilisation of a tropical bay as a nursery area by sharks of the families Carcharhinidae and Sphyrnidae. Environ. Biol. Fishes 37, 337–345. doi: 10.1007/bf00005200
Sims, D. W., Southall, E. J., Quayle, V. A., and Fox, A. M. (2000). Annual social behaviour of basking sharks associated with coastal front areas. Proc. R. Soc. B Biol. Sci. 267, 1897–1904. doi: 10.1098/rspb.2000.1227
Spaet, J. L. Y., Patterson, T. A., Bradford, R. W., and Butcher, P. A. (2020). Spatiotemporal distribution patterns of immature Australasian white sharks (Carcharodon carcharias). Sci. Rep. 10, 1–13. doi: 10.1038/s41598-020-66876-z
Spaet, J., Manica, A., Brand, C., Gallen, C., and Butcher, P. (2020). Environmental conditions are poor predictors of immature white shark Carcharodon carcharias occurrences on coastal beaches of Eastern Australia. Mar. Ecol. Prog. Ser. 653, 167–179. doi: 10.3354/meps13488
Stehfest, K. M., Patterson, T. A., Dagorn, L., Holland, K. N., Itano, D., and Semmens, J. M. (2013). Network analysis of acoustic tracking data reveals the structure and stability of fish aggregations in the ocean. Anim. Behav. 85, 839–848. doi: 10.1016/j.anbehav.2013.02.003
Tamburin, E., Hoyos-Padilla, M., Sánchez-González, A., Hernández-Herrera, A., Elorriaga-Verplancken, F. R., and Galván-Magaña, F. (2019). New nursery area for white sharks (Carcharodon carcharias) in the Eastern Pacific Ocean. Turkish J. Fish. Aquat. Sci. 20, 325–329. doi: 10.4194/1303-2712-v20_4_08
Weng, K. C., O’Sullivan, J. B., Lowe, C. G., Dewar, H., Winkler, C., and Block, B. A. (2007). Movements, behavior and habitat preferences of juvenile white sharks Carcharodon carcharias in the Eastern Pacific. Mar. Ecol. Prog. Ser. 338, 211–224. doi: 10.3354/meps338211
Werry, J., Bruce, B., Sumpton, W., Reid, D., and Mayer, D. (2012). ““Beach Areas Used by Juvenile White Shark, Carcharodon carcharias,” in Eastern Australia,” in Global Perspectives on the Biology and Life History of the White Shark, ed. M. L. Domeier (Boca Raton, FL: CRC Press), 271–286.
White, C. F., Lyons, K., Jorgensen, S. J., O’Sullivan, J., Winkler, C., Weng, K. C., et al. (2019). Quantifying habitat selection and variability in habitat suitability for juvenile white sharks. PLoS One 14:e0214642. doi: 10.1371/journal.pone.0214642
Wilson, A. D. M., Brownscombe, J. W., Krause, J., Krause, S., Gutowsky, L. F. G., Brooks, E. J., et al. (2015). Integrating network analysis, sensor tags, and observation to understand shark ecology and behavior. Behav. Ecol. 26, 1577–1586. doi: 10.1093/beheco/arv115
Wilson, A. D. M., Croft, D. P., and Krause, J. (2014). Social networks in elasmobranchs and teleost fishes. Fish Fish. 15, 676–689. doi: 10.1111/faf.12046
Keywords: social structure, acoustic telemetry, network analysis, sociality, juvenile white shark
Citation: Anderson JM, Clevenstine AJ, Stirling BS, Burns ES, Meese EN, White CF, Logan RK, O’Sullivan J, Rex PT, May J (2021), Lyons K, Winkler C, García-Rodríguez E, Sosa-Nishizaki O and Lowe CG (2021) Non-random Co-occurrence of Juvenile White Sharks (Carcharodon carcharias) at Seasonal Aggregation Sites in Southern California. Front. Mar. Sci. 8:688505. doi: 10.3389/fmars.2021.688505
Received: 30 March 2021; Accepted: 19 July 2021;
Published: 14 September 2021.
Edited by:
David M. P. Jacoby, Institute of Zoology, Zoological Society of London, United KingdomReviewed by:
Sara Andreotti, Stellenbosch University, South AfricaCharlie Huveneers, Flinders University, Australia
Robert Perryman, Macquarie University, Australia
Copyright © 2021 Anderson, Clevenstine, Stirling, Burns, Meese, White, Logan, O’Sullivan, Rex, May, Lyons, Winkler, García-Rodríguez, Sosa-Nishizaki and Lowe. This is an open-access article distributed under the terms of the Creative Commons Attribution License (CC BY). The use, distribution or reproduction in other forums is permitted, provided the original author(s) and the copyright owner(s) are credited and that the original publication in this journal is cited, in accordance with accepted academic practice. No use, distribution or reproduction is permitted which does not comply with these terms.
*Correspondence: James M. Anderson, james.anderson@csulb.edu