- 1Centro de Investigação em Biodiversidade e Recursos Genéticos/Rede de Investigação em Biodiversidade e Biologia Evolutiva (CIBIO/InBIO), Universidade do Porto, Vairão, Portugal
- 2Laboratório Marítimo da Guia, Marine and Environmental Sciences Centre (MARE), Faculdade de Ciências, Universidade de Lisboa, Cascais, Portugal
- 3Instituto de Investigaciones Marinas, Consejo Superior de Investigaciones Científicas (IIM-CSIC), Vigo, Spain
- 4Marine Biological Association of the United Kingdom, Plymouth, United Kingdom
- 5Ocean and Earth Science, National Oceanography Centre Southampton, University of Southampton, Southampton, United Kingdom
- 6Centre for Biological Sciences, University of Southampton, Southampton, United Kingdom
In the pelagic environment diel vertical movements (DVM) are widespread across taxa, from zooplankton ascending from day-time depths into surface layers at night to avoid visual predators, to apex predators following prey movements to maximise foraging opportunities. The drivers of DVM in large predators such as pelagic sharks have only recently begun to be investigated in detail with the advent of sophisticated archival tags and high-resolution oceanographic datasets. In this study, we satellite tagged adult [>180 cm fork length, (FL)] blue sharks (Prionace glauca) in the North Atlantic Ocean to examine behavioural changes in response to the encountered environment, and therefore, to determine potential risks of capture using pelagic longline fisheries data. Although blue sharks recurrently use surface waters, cyclic diel behaviours were observed, with >95% of night-time spent above 250 m depth and variable day-time depth use. Hence, three different diel behaviours were identified during the tracking period: (i) regular normal DVM (nDVM) (dawn descent – dusk ascent, with over 90% of nighttime spent above 250 m, and between 5 and 50% of the day below this threshold); (ii) surface-oriented behaviour (occupation of surface waters both day and night), and (iii) deep depth-oriented nDVM [dawn descent – dusk ascent, with the majority (>50%) of daytime spent at depth]. Importantly, diel behaviours generally occurred in different ocean regions with nDVM frequently observed in high latitudes, associated with cold, highly productive waters (e.g., North Atlantic Current/Labrador Current convergence zone, West African upwelling area), while depth-oriented nDVM was observed in warm, oligotrophic areas. Thus, day-time occupation of shallow waters significantly increased with lower water temperature at depth (100 m), and with increasing concentration (and decreasing depth) of the chlorophyll a maximum. During nights of full moon blue sharks spent significantly more time in the depth range of longline hooks, while fishing effort and catches were also higher. We demonstrate that increased occupancy of surface layers driven by highly productive, cold waters and greater lunar illumination lead to higher capture risk. Understanding habitat-specific vulnerability to fishing in a commercially important pelagic shark species is essential for improving management and conservation measures.
Introduction
Apex pelagic predators, given their extensive movements and wide distributions, play an important role shaping the ecological structure and habitat use of oceanic communities (Sims, 2003; Campana, 2016; Boerder et al., 2019). Yet, over the past decades, populations of commercially important species have been declining due to overexploitation (Baum et al., 2003; Pacoureau et al., 2021). This has been partially fuelled by a lack of knowledge on the movement and habitat use patterns, and where and when fish interact with fishing vessels, which in turn hinder stock assessment models (Mejuto and García-Cortés, 2005; Queiroz et al., 2019). While original stock assessments assumed fish populations were evenly distributed at an ocean-basin scale, recent approaches that include a spatial structure have shown a largely improved estimation performance (Punt, 2019). However, most current stock assessments still account for catchability as a measure of spatial distribution (Punt, 2019), raising the need for movement-based stock assessments as opposed to fisheries-based (Baum et al., 2003; Dunn et al., 2019). Advances in satellite telemetry and the increasing availability of remotely sensed environmental data have, however, allowed the coupling of recorded behavioural data with aspects of the encountered environment (Whitford and Klimley, 2019; Francisco et al., 2020). This has permitted studies that describe habitat preferences of migratory marine vertebrates even in remote areas, such as the open ocean (Humphries et al., 2010; Guzman et al., 2018; Sequeira et al., 2018). In addition, understanding how environmental changes affect the movements and behaviour of pelagic fish has also enabled the identification of areas and periods of higher vulnerability to fisheries (Song et al., 2009; Queiroz et al., 2016, 2019; Hays et al., 2019). Taken together, this spatial and behavioural information is therefore essential to the development of more effective conservation measures (Hammerschlag et al., 2016; Sequeira et al., 2019; Hindell et al., 2020).
Besides wide-ranging distributions, pelagic predators such as sharks and tunas (Thunnus spp.) are also efficient divers, exploring a multitude of vertical habitats from the surface to the bathyal (Schaefer and Fuller, 2002; Wilson et al., 2005; Howey-Jordan et al., 2013). A particularly distinctive cyclic behaviour has been described for a wide range of pelagic species, from zooplankton to apex predators – diel vertical movements (DVM) (e.g., Weng et al., 2009; Coffey et al., 2017; Hafker et al., 2017). DVM in zooplankton is characterised by occupation of surface waters at nighttime and, as a phototaxic response, diving into deeper waters during daytime where light levels are lower (Lampert, 1989). These cyclic movements have been primarily associated with zooplankton avoidance of visual predators, which are then mimicked throughout the food chain to optimise prey encounter success (Hays, 2003). However, drivers of DVM for pelagic predators have generally been associated with thermoregulation and/or foraging (Pade et al., 2009; Campana et al., 2011; Queiroz et al., 2012; Andrzejaczek et al., 2019). For example, Atlantic bluefin tunas in the Gulf Stream reduced time spent in shallow warm waters and exhibited deeper DVMs possibly as a behavioural mechanism to maintain optimal body temperature (Teo et al., 2006). However, DVM in ectothermic fish may also represent a behavioural mechanism that balances the higher energy costs associated with night-time foraging activity in shallow, warm waters, with reduced activity in deep cold waters during daytime that lowers metabolic rate (Sims et al., 2006).
DVM associated with predator avoidance is particularly important for species at lower trophic levels, where day-time occupation of aphotic layers and night-time shoaling in the darkness decreases the predation success of visual predators, consequently increasing the chances of prey survival (Wirsing et al., 2011). However, for pelagic top predators, DVM has been mostly associated with foraging behaviour (Carey et al., 1990; Andrzejaczek et al., 2019). By following prey diel rhythms, predators increase the spatial and temporal overlap with prey distribution, increasing the success of prey encounter (Sims et al., 2005). Several species of marine megafauna are diel vertical migrators, and some of the most important components of their diet include mesopelagic fish and diel vertically migrating species, supporting the notion that DVM increases foraging success (Sims et al., 2005; Pade et al., 2009; Duffy et al., 2017; Braun et al., 2019a). Shifts in diel behavioural patterns have been linked to variations in the thermal profile of the water column and to the distribution and timing of peak productivity. For instance, both blue and salmon sharks have shown an increased surface occupancy in highly productive frontal regions, associated with cold surface temperatures and sharp water-column stratification (Queiroz et al., 2012; Coffey et al., 2017). Besides diel differences in vertical space-use, activity rates also follow a diel cycle, being generally higher at night than during the day (Andrews et al., 2009; Papastamatiou et al., 2018). For example, increased night-time activity of sharks was linked to high food abundance (Sims et al., 2006). Hence, this increased nocturnal activity has been associated with foraging on prey aggregations occurring in warm surface waters during this period (Sims et al., 2006; Papastamatiou et al., 2018).
Although changes in vertical behaviour are strongly linked to the physical and biological environment, they can also vary in response to the illuminated lunar fraction. Distributions of plankton on nights of full moon are generally deeper than on nights of new moon which manifests as a phototaxic response for predator avoidance (Tarling, 1999; Last et al., 2016). This behaviour is driven by a similar light-avoidance mechanism to the one observed for DVM, but here deepening occurs at night in response to moonlight. This behaviour cascades through trophic levels, from mesopelagic fish (Olivar et al., 2017) up to top predators (e.g., tunas and swordfish Xiphias gladius) where deeper movements on nights of full moon have been hypothesised to improve foraging success within prey aggregated at deeper depths (Musyl et al., 2003; Dewar et al., 2011; Abascal et al., 2015). Furthermore, behavioural variations linked to the lunar phase affect the capture risk of pelagic predators by commercial fisheries. For example, catches of commercial species such as yellowfin and blackfin tuna, and blue sharks were higher in nights of full moon (Curran, 2014; Orbesen et al., 2017), while catch rates for swordfish were highest during both new and full moon phases (Poisson et al., 2010; Lerner et al., 2013; Orbesen et al., 2017). The vertical behaviour of blue sharks has been the subject of a large number of studies (e.g., Carey et al., 1990; Campana et al., 2011; Queiroz et al., 2012; Braun et al., 2019b), but knowledge of the fine-scale behaviour of oceanic adult blue sharks and their vulnerability to fishing as a consequence is scarce. Blue sharks are one of the most exploited species by pelagic longline fisheries, with populations declining by ∼40% since the 1970s (Baum et al., 2003; Pacoureau et al., 2021) largely as a result of high mortality risk from fishing (Queiroz et al., 2016, 2019). Despite recently established fishing quotas for blue sharks in some regions, the uncertainty in current stock assessments make understanding shark fine-scale behaviour a priority for effective conservation management (Hammerschlag et al., 2016; Robinson et al., 2017; Boerder et al., 2019). To address these knowledge gaps, we used pop-off satellite-linked archival transmitters (PSATs) attached to adult blue sharks in the North Atlantic Ocean, to investigate potential coupling between open-ocean shark DVM with environmental fields and to identify both the spatial and temporal risks posed by longline fishing in the region.
Materials and Methods
Shark Tagging
A total of 22 adult blue sharks (Prionace glauca) were tagged in two general oceanic locations (in the mid-Atlantic and the north-western Atlantic regions) between June 2010 and August 2011. Sharks were captured on commercial baited surface longlines and brought alongside the vessel in the gear-hauling phase and tagged. Pop-off satellite-linked archival transmitter tags (PSATs; Mk-10 model, Wildlife Computers, Redmond, WA, United States) were rigged with a monofilament tether covered with silicone tubing and looped through a small hole made in the base of the first dorsal fin. Depth, external temperature, and light-level parameters were archived at 1 s intervals and stored as summary data over set intervals of 6 h (00:00, 06:00, 12:00, and 18:00). For each period, time-at-depth histograms (TAD; aggregated in eight depth bins, 50, 100, 150, 200, 250, 400, 600, >600 m), minimum and maximum depth and temperature, as well as profiles of water temperature at depth were available. All shark tagging procedures undertaken in this study were approved by institutional ethical review committees and completed by licenced, trained, and experienced personnel.
Track Processing
The movement of PSAT-tagged sharks was estimated using either satellite relayed data from each tag or from archival data after the tags were physically recovered. Positions of each shark between attachment and tag pop-up were reconstructed using software provided by the manufacturer (WC-GPE, global position estimator programme suite), where daily maximal rate-of-change in light intensity was used to estimate local time of midnight or midday for longitude calculations, and day-length estimation for determining latitude. Anomalous longitude estimates resulting from dive-induced shifts in the estimated timings of dawn and dusk from light curves were automatically discarded from the dataset using software provided by the manufacturer (WC-GPE); latitude estimates were subsequently iterated for the previously obtained longitudes. An integrated state-space model [unscented Kalman filter – UKFSST (Lam et al., 2008); using spatially complete NOAA Optimum Interpolation Quarter Degree Daily SST Analysis data] was then applied to correct the raw geolocation estimates and obtain the most probable track. A regular time-series of locations was then estimated using a continuous-time correlated random walk Kalman filter, CTCRW (Jonsen et al., 2005) performed in R [crawl package (Johnson et al., 2008)]. Subsequently, the CTCRW state-space model was applied to each individual track, producing a single position estimate per day. Argos positions were parameterised with the K error model parameters for longitude and latitude implemented in the crawl package (Jonsen et al., 2005). To obtain unbiased estimates of shark space use, gaps between consecutive dates in the raw tracking data were interpolated to one position per day. However, any tracks with gaps exceeding 20 days were split into segments prior to interpolation, thus avoiding the inclusion of unrepresentative location estimates (Queiroz et al., 2016, 2019).
Diel Diving Behaviour
To detect cyclical patterns in the behaviour of the sharks, maximum depths for each 6 h interval were analysed with a Lomb-Scargle (LS) periodogram (Lomb, 1976; Scargle, 1982), using a detection range between 10 and 30 h in R package lomb (Ruf, 2010; Azzurro et al., 2012). The maximum percentage of total data variance fitted by the corresponding periodicity was chosen as the peak value (Campbell et al., 2010; Azzurro et al., 2012).
To analyse diel differences in the behaviour of blue sharks, the time between 12:00 GMT and 18:00 GMT (6 h period) was considered daytime, while night-time was considered between 00:00 GMT and 06:00 GMT (6 h period). Time bins which encompassed sunset and sunrise times (between 06:00–12:00 GMT and 18:00–00:00 GMT, respectively; Supplementary Figure 1) were excluded from the analyses (Dewar et al., 2011; Abecassis et al., 2012). Following previous studies on the diel behaviour of blue sharks, three classes were defined based on individual TAD and maximum depth for each daytime and night-time period over 5-day periods (Campana et al., 2011; Queiroz et al., 2012). A 5-day period was classified as (i) depth-oriented nDVM if a shark spent more than 90% of the night-time above 250 m and 50% of the daytime below this depth; (ii) regular nDVM was characterised by over 90% of the night above 250 m, and between 5 and 50% of the day below the threshold. Finally, (iii) surface-oriented behaviour was characterised by over 90% of both day and night-time above 250 m. This depth threshold was used because it corresponds to the depth above which >95% of night-time occupancy for tracked blue sharks occurs (Supplementary Figure 1). Lastly, periods without clear day and night TAD patterns were classified as “other” and excluded from further analyses (corresponding to 4.0% of the total analysed periods). Subsequently, time-weighted depth and temperature were calculated for each 6 h day- and night-time bins using the function weighted.mean in R software, using the middle point of the bins defined for TAD data and the maximum depth/temperature. Pairwise Wilcoxon Rank Sum tests were used to statistically compare day and night-time weighted values, within each diel class. To further explore the behavioural thermoregulation hypothesis, a Pearson correlation was used to test the relationship between time-weighted temperatures during the day and the consecutive night. Moreover, individual dives were identified using the diveMove R package for high-resolution archival dive data available for three sharks (S3, S11, and S15), following Queiroz et al. (2017). For each dive, we assessed its duration and respective time-weighted temperatures. A Spearman correlation was used to compare the average time-weighted temperatures between day- and night-time dives.
Monthly mean modelled environmental data (0.25° × 0.25°) were acquired from Copernicus Marine Environment Monitoring Service (CMEMS) global ocean biogeochemistry non-assimilative hindcast (PISCES 1998–2011) for chlorophyll a (mg m–3) and global ocean physics reanalysis (GLORYS2V3 1993–2012) for temperature (°C) products for the North Atlantic Ocean. Environmental data was extracted from the surface to 1,750 m depth, and to account for the spatial error around real individual geolocations, data was averaged for 1.25° in latitude and 0.75° in longitude (using a 5 × 3 grid cell) around each position. The relationship between day-time occupation of shallow waters (TAD above 250 m depth) and environmental variables, (i) sea surface temperature (SST), (ii) temperature at depth 100 m, (iii) maximum concentration of chlorophyll a in the water column, and (iv) depth of the chlorophyll a maximum, was investigated using a general linear mixed model (GLMM) with binomial distribution and cauchit link function. Data exploration techniques were used to identify potential outliers and assess collinearity among independent variables. Hence, chlorophyll a concentrations above 1 mg m–3 were considered outliers and discarded from further analysis (corresponding to 2.56% of data); in addition, the depth of the chlorophyll a maximum showed a high collinearity with concentration – variance inflation factor (VIF) higher than three (Zuur et al., 2009) – and was removed from the model selection process. Individual sharks were considered an independent sampling unit and were included as random effects; however, individuals with less than 10 data points were discarded from the analyses [resulting in the exclusion of S12; (Zuur et al., 2009)]. General mixed models were constructed by backward selection of individual terms to allow for testing of biologically meaningful interactions. The selected terms were: (i) sea surface temperature (SST), (ii) temperature at depth 100 m, and (iii) maximum concentration of chlorophyll a in the water column. The model was trained with 75% of data (n = 456) and the optimal selection was based on Akaike Information Criterion (AIC). At each stage of the selection process, fitted models were compared to the null model. Consequently, the fitted model with the lowest AIC was chosen as an optimal structure, with which a GLMM was built using MASS R package. Normal quantile–quantile plots of deviance residuals were assessed for normality of residuals and fit, while homoscedasticity, model misspecification, and residual spatial autocorrelation were evaluated by inspecting plots of response residuals against fitted values and candidate explanatory variables, respectively. Spatial and temporal residual autocorrelation was further assessed by including respective covariate structures and comparing model performance. This resulted in the inclusion of an autocorrelation structure of order 1 corAR1 (nmle R package) in the model.
Lunar Phase and Hook Encounter Risk
Lunar phase (visible fraction of the disc, a continuous variable where 0 is dark moon and 1 corresponds to full moon) was extracted for each estimated shark geolocation. To account for the spatial error around individual geolocations, visible fraction of the disc data was averaged for 1.25° in latitude and 0.75° in longitude around each shark position. Data was obtained using the function moonAngle in the oce R package. To analyse shark night-time depth occupation in relation to the moon phase, the illuminated lunar fraction was divided into four 0.25 bins representative of the four lunar phases: (i) new moon, (ii) first and (iii) third quarter, and (iv) full moon.
Drifting pelagic longline fishing effort data at 0.01° × 0.01° grid resolution were obtained from Global Fishing Watch (GFW) and subsequently gridded using the same spatial resolution as the environmental data (0.25 × 0.25° grid cells) and between the years 2012 to 2016. The number of fishing days in each month within each grid cell was summed and then averaged across years (Queiroz et al., 2019). Geo-referenced catch data for blue shark were also obtained from logbooks of Spanish commercial longliners between 2013 and 2017. Similarly, monthly catch per unit of effort (CPUE) was summed within each 0.25 × 0.25° grid cells and averaged across years. GFW and CPUE analyses also considered the spatial error around individual geolocations. Despite the lack of fishing data for the same period sharks were tracked (2010–2011), fishing patterns and the associated capture risk are consistent across years (Kroodsma et al., 2018; Queiroz et al., 2019). Hence, by averaging fishing effort and CPUE across multiple years, interannual variation was considered when calculating the mean vulnerability of tracked sharks. Since longlines are deployed at night at depths between 100 and 300 m (Domingo et al., 2016), the TAD between 100 and 250 m was also calculated (TADhook) for each shark location. This percentage of time at hook depth was initially compared between nights of new and full moon; GFW fishing effort and CPUE were also analysed in relation to the lunar phase using an independent-sample Wilcoxon rank sum test.
Results
Between 2010 and 2011, a total of 15 blue sharks were successfully tracked in the North Atlantic for a total of 1,325 cumulative days (Figure 1 and Table 1). Sharks tagged in the mid-Atlantic either remained in the same general area for the tracking duration (between 90 and 120 days; S1, S4, S8, and S14) or moved southward (S2, S3, S7, and S15; these sharks were tracked between 78 and 120 days). Of these, S2, S7, and S15 moved south/south-east into the Cape Verde islands area, while S3 moved south-west into oligotrophic waters (Figure 1). The spatial distribution pattern for sharks tagged in the West Atlantic was more complex. Of the seven individuals tagged, four blue sharks (S5, S10, S11, and S12) remained in the overall area associated with the Gulf Stream (the North Atlantic Current/Labrador Current convergence zone) generally moving north along the edges of the frontal area, for periods ranging from seven to 89 days (Figure 1). Two sharks (S6 and S9) moved south into warmer waters (for 56 and 120 days, respectively) and one shark (S13) moved west into the shelf area off the American east coast, south of Nova Scotia. This shark was tracked for 180 days and was the only to have moved into cold surface waters (Supplementary Figure 2).
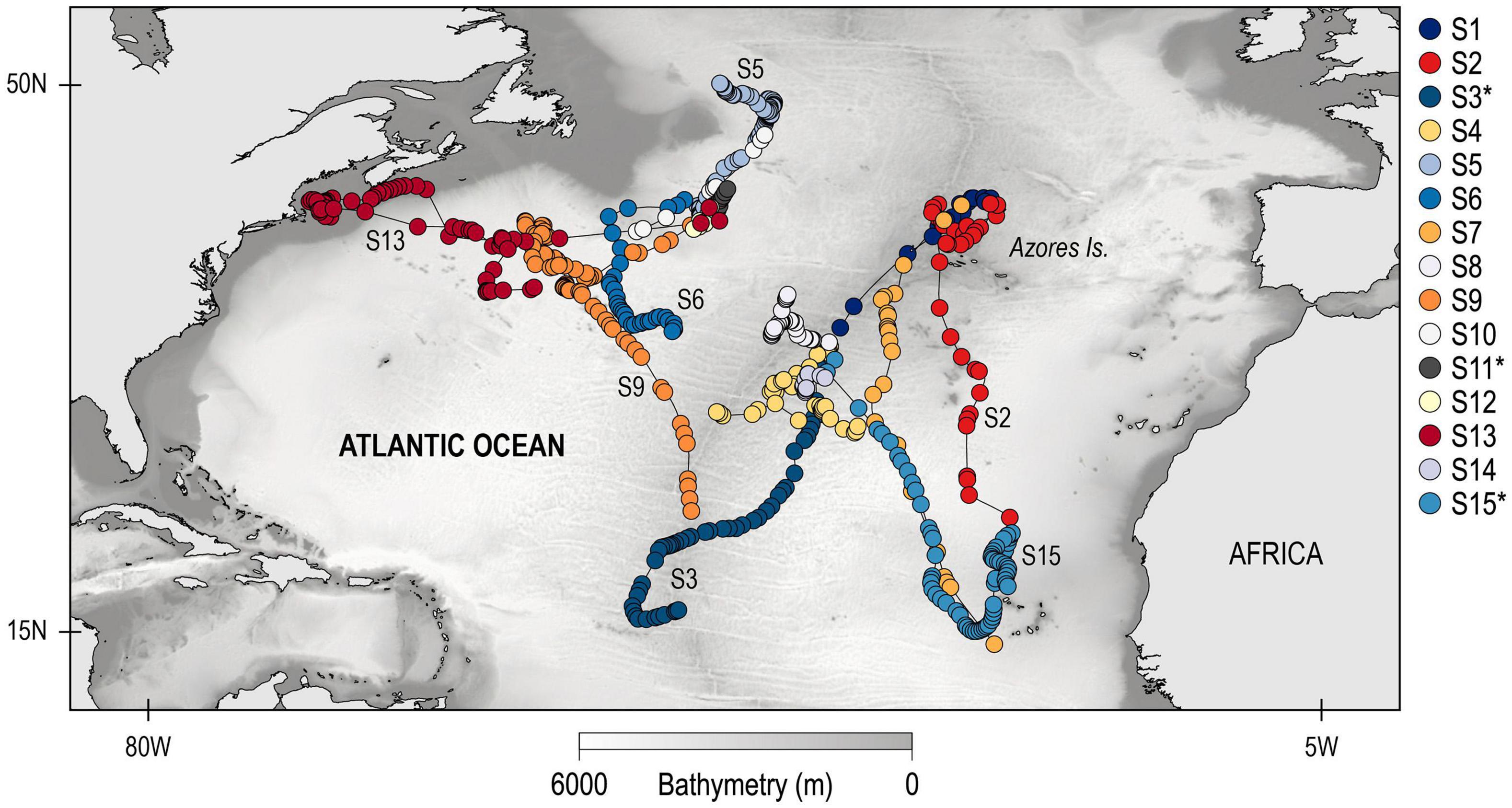
Figure 1. Individual geolocations for 15 blue sharks tracked in the North Atlantic Ocean between 2010 and 2011.
Diel Diving Behaviour
Periodograms of individual maximum dive depth for each 6 h bin showed a significant cyclic behaviour for 12 (out of 15) sharks, of which 11 individuals peaked significantly within the 23–25 h range, which was considered to represent a diel rhythm (Shepard et al., 2006; Campbell et al., 2010). The periodogram of S3 peaked significantly at 12 h, while the analysis was non-significant for sharks S8, S11, and S12 (Table 1).
Regular nDVM was the most observed behaviour (55.7% of the time; Figure 2A), followed by surface-oriented (22.8% of the time; Figure 2C) and finally, depth-oriented nDVM (17.5% of the time; Figure 2B). Overall, the time-weighted depth of blue sharks was significantly deeper during daytime and shallower during nighttime (pairwise Wilcoxon signed rank test, V = 27243, α = 0.05, p < 0.001, n = 236), with an average day-time depth of 175 ± 99 m and an average night-time depth of 59 ± 46 m. The same pattern was observed for each diel behaviour; while performing regular nDVM, the average day-time depth of tracked blue sharks was 146 ± 95 m and average night-time was 45 ± 38 m (median: 147 m and 33 m, respectively), whereas in depth-oriented nDVM behaviour, average day-time depth was 246 ± 74 m and night-time 92 ± 42 m (median: 244 m and 95 m, respectively). For surface-oriented behaviour, a shallower average depth was observed, with an average depth of 101 ± 49 m during the day and 19 ± 12 m during the night (median: 116 m and 21 m, respectively). Hence, the median day and night-time depth for each diel behaviour was different (Kruskal–Wallis rank sum test, daytime: chi-squared = 77.276, α = 0.05, p < 0.001, n = 236; night-time: chi-squared = 73.78, α = 0.05, p < 0.001, n = 236). Moreover, during the day, time-weighted water temperature was significantly colder (19.34 ± 3.16°C) than at night (23.26 ± 2.99°C; pairwise Wilcoxon signed rank test, V = 29306, α = 0.05, p < 0.001, n = 259). This general pattern was consistent across diel behaviours; during nDVM, average day-time temperature was 20.83 ± 3.10°C and average night-time temperature was 23.39 ± 3.33°C (paired Wilcoxon signed rank exact test, V = 3125, α = 0.05, p < 0.001, n = 88); while sharks were performing depth-oriented nDVM, the average temperature was 17.53 ± 2.66°C and 23.02 ± 2.50°C for day- and night-time, respectively (paired Wilcoxon signed rank exact test, V = 2065, α = 0.05, p < 0.001, n = 64). Finally, for surface-oriented behaviour, average day-time temperature was 18.85 ± 2.42°C and 23.28 ± 3.11°C at night (paired Wilcoxon signed rank exact test, V = 611, α = 0.05, p < 0.001, n = 35). However, time weighted temperatures during the day were positively correlated with the time-weighted temperatures experienced in consecutive nights (Pearson correlation = 0.49, t = 8.66, α = 0.05, p < 0.001, n = 244). Similarly, for a subset of archival data, average time-weighted temperatures during day-time dives were positively correlated with night-time dives (Spearman correlation = 0.18, S = 848744, α = 0.05, p < 0.05, n = 184).
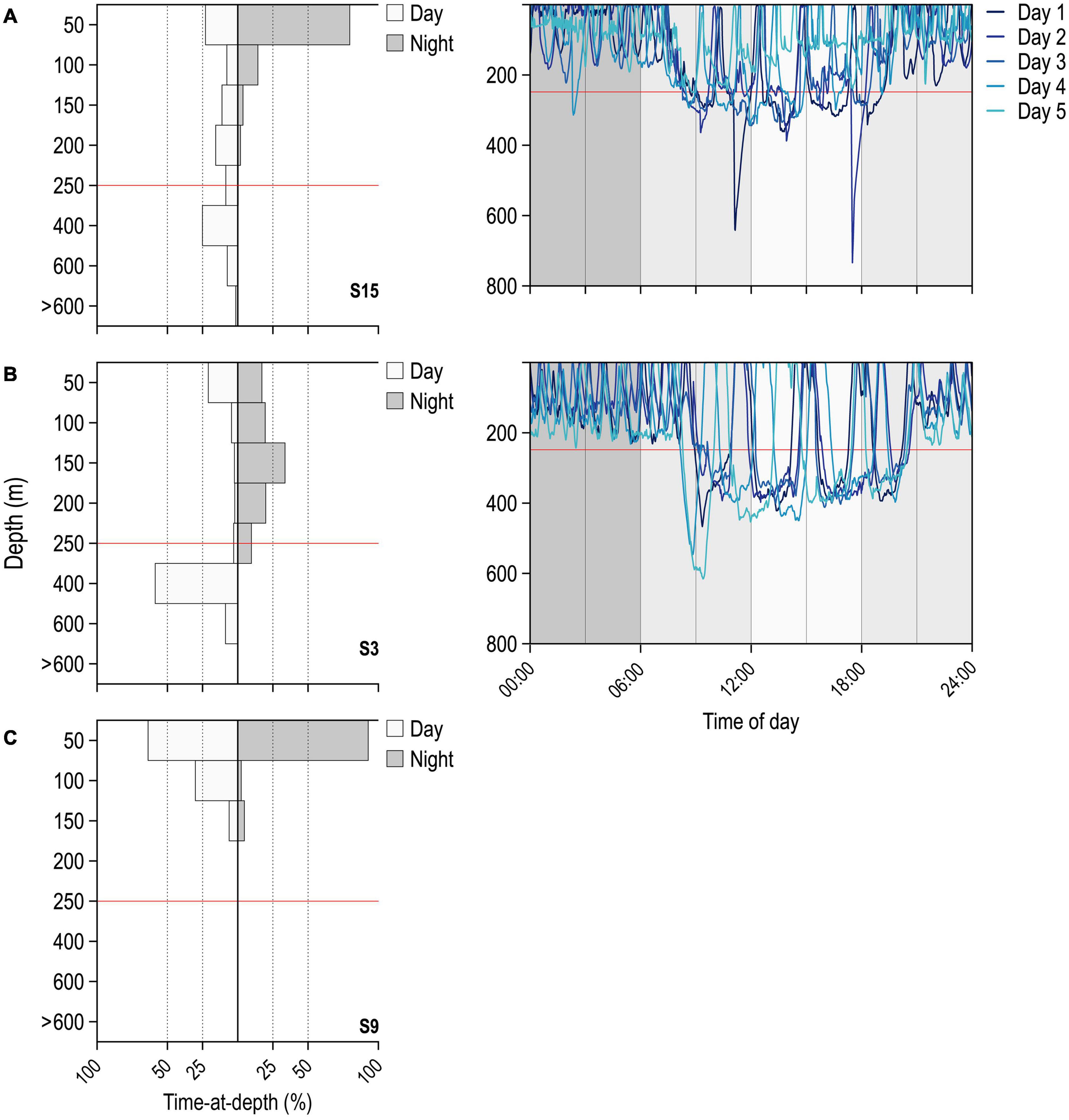
Figure 2. Diel behaviour of blue sharks. Left panel shows the percentage of time-at-depth for day- (white) and night-time (grey) for (A) nDVM (day-time occupancy above 250 m: 66.0%), (B) depth-oriented nDVM (32.7%), and (C) surface-oriented (100%) diel diving behaviours; Right panels show the correspondent 5-day archival depth use. Horizontal red lines denote the depth threshold considered to define each diel class (250 m depth).
Environmental-Linked Behavioural Changes
The vertical behaviour of the sharks changed throughout the tracking period in relation to both encountered temperature and chlorophyll a concentration in the water column, with regular nDVM (and surface-oriented) behaviour generally associated with colder, more productive waters (Figure 3). In contrast, depth-oriented diel behaviour was commonly observed in regions with less productive, warmer surface waters, but, strikingly, with deep chlorophyll a maxima layers (Figure 3). Similar patterns were evident from high-resolution archival data (Figure 4). Regular nDVM was generally characterised by shallower day-time dives, colder surface waters (23.55 ± 3.76°C) and higher chlorophyll a concentrations near the surface (Figures 4A,C), while depth-oriented nDVM was performed in regions of warmer (27.35 ± 0.80°C), oligotrophic surface waters, and lower, deeper maximum concentrations of chlorophyll a (Figures 4B,D). Differences in habitat use were also clear between the two diel behaviours over 24 h periods (Figure 5). During both normal diel patterns, sharks showed greater night-time occupation of waters above ca. 25°C (Figures 5A,B), however, during nighttime, a consistent occupation in lower temperatures (<17°C) was evident during depth-oriented nDVM (Figure 5D). Similarly, blue sharks maximised time at medium to high (>4.5 × 10–2 mg m–3) chlorophyll a concentrations during nighttime (Figures 5C,D), although time spent at these concentrations was greater during regular nDVM (Figure 5C). Importantly, in depth-oriented nDVM, a peak in the amount of time spent in layers of low chlorophyll a concentrations (ca.<1.4 × 10–2 mg m–3) was also observed during daytime (Figure 5D).
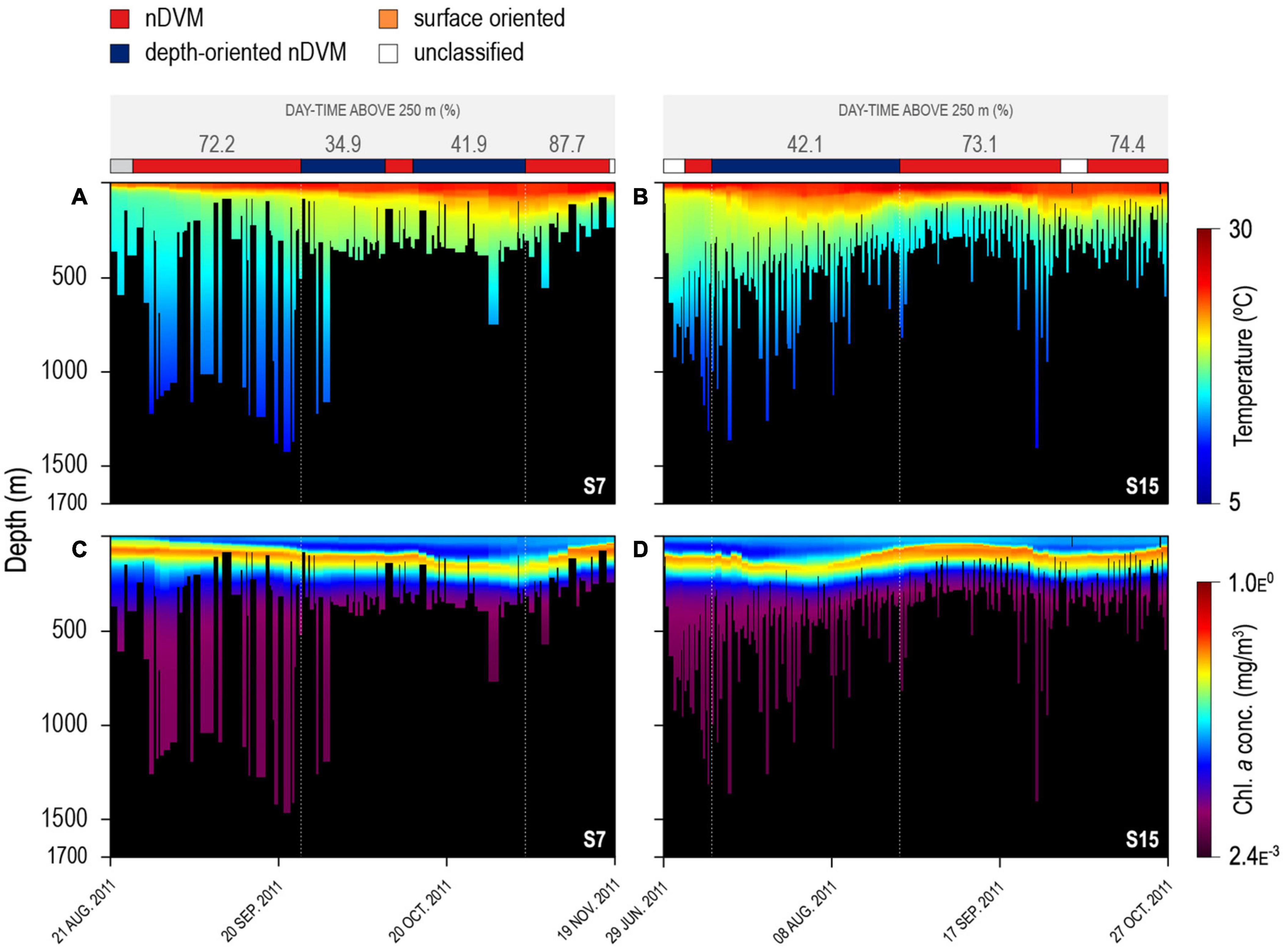
Figure 3. Maximum daily dive depths for two individual blue sharks, shark #7 on the left (A,C) and #15 on the right (B,D). Dive series are overlaid on water temperature (A,B) and chlorophyll a concentration (C,D). Coloured bar above represent the identified diel behaviour.
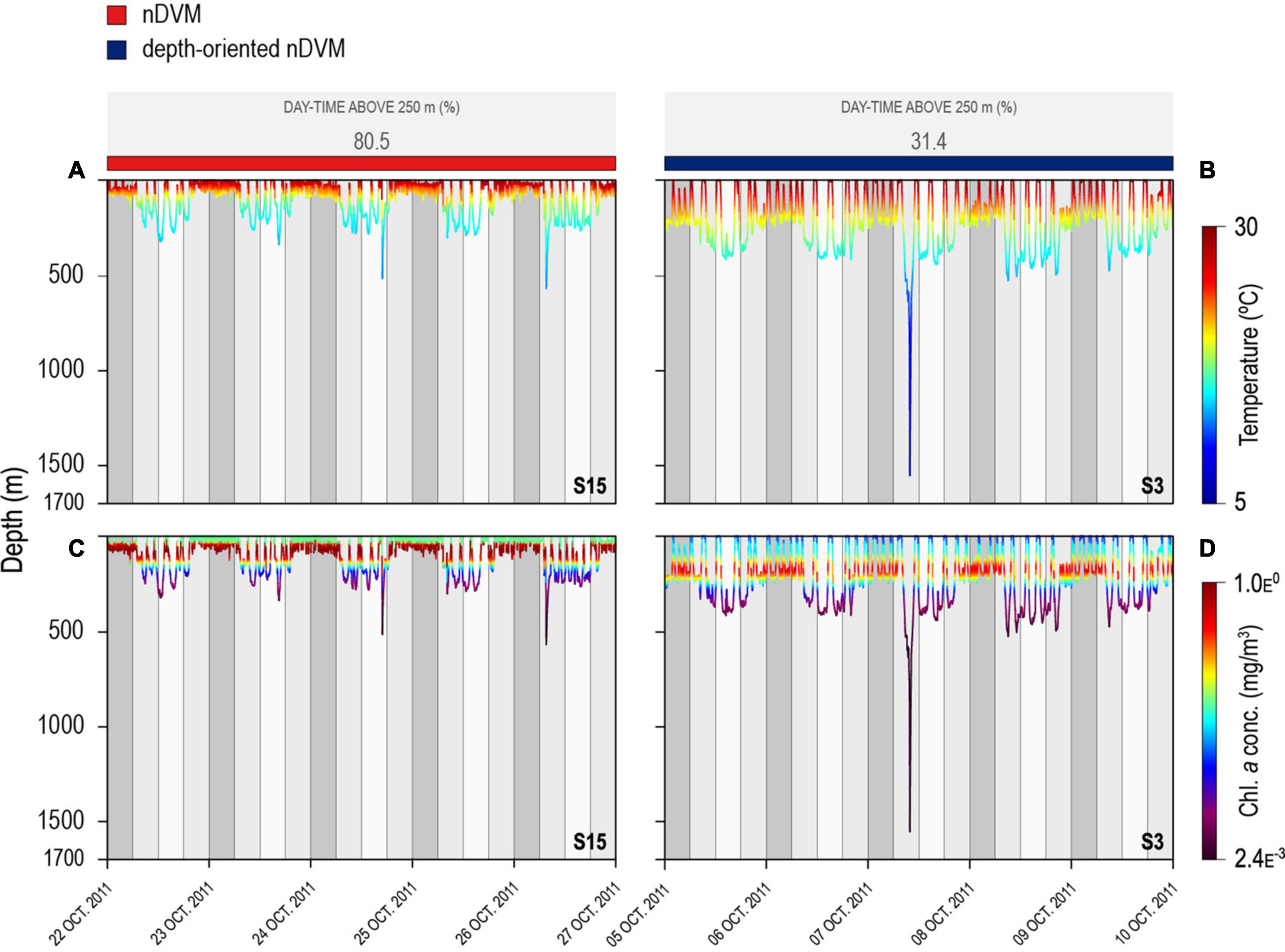
Figure 4. Archival 5-day period depth series for two individual blue sharks, during nDVM for shark #15 on the left (A,C) and depth-oriented nDVM for shark #3 on the right (B,D). Shark depth is overlaid with water temperature (A,B) and chlorophyll a concentration (C,D). Coloured bar above represent the identified diel behaviour.
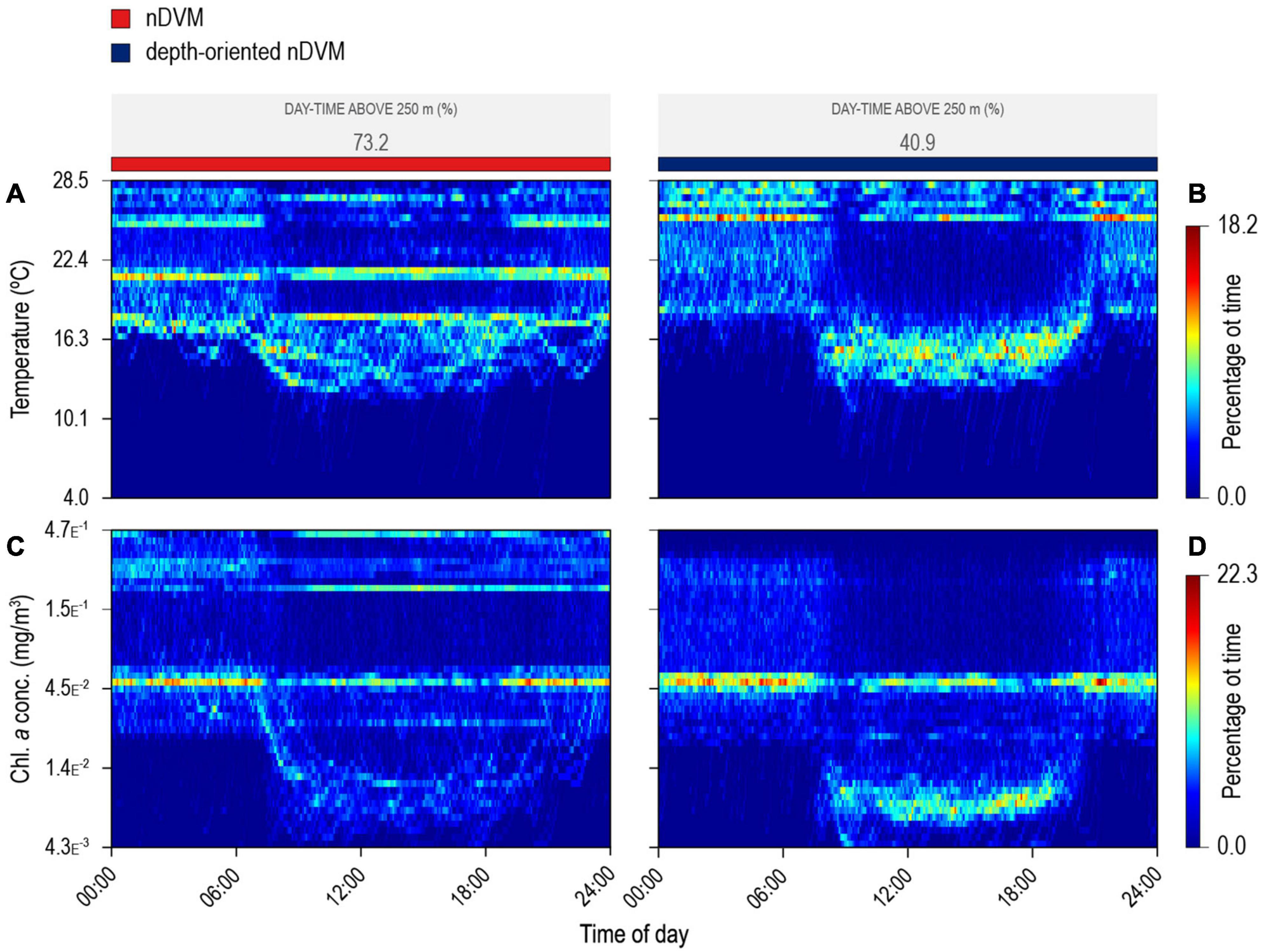
Figure 5. Blue shark habitat-use for a 24 h period, during nDVM on the left (A,C) and depth-oriented nDVM on the right (B,D). Time-at-temperature (A,B); and time-at-chlorophyll a (C,D). Coloured bar above represents the identified diel behaviour.
The GLMM analysis revealed that day-time occupancy in shallow water (i.e., TAD above 250 m) significantly increased with decreasing (i) water temperature at depth (100 m), and (ii) with increasing concentration of chlorophyll a (Table 2, Figure 6, and Supplementary Figure 3). The depth of the maximum concentration of chlorophyll a was inversely correlated with the concentration (Spearman’s rank correlation: rho = −0.92, S = 30395248, p-value < 0.001, n = 456); hence, the observed increased shark day-time occupancy in shallow water was also linked to the shoaling depth of the chlorophyll a maximum. Therefore, regular nDVM was predominantly observed associated with the Gulf Stream and the North Atlantic Current-Labrador Current convergence zone (NAC-LCCZ), but also in mid-Atlantic regions and in the proximity of archipelagos, such as the Azores and Cape Verde (Figure 7A). Surface-oriented behaviour was almost exclusively observed off the American east coast, in the vicinity of the Gulf Stream and in the mid-Atlantic (Supplementary Figure 4), whereas depth-oriented nDVM was generally observed during southward movements in mid-Atlantic regions (Figure 7B). Interestingly, almost no spatial overlap was observed between the two nDVM behaviours. This resulted in a latitudinal pattern, with surface-oriented and regular nDVM commonly observed in high latitudes of colder, productive waters (Figure 8). Regular nDVM was also frequent in low latitudes but only associated with cold, productive waters of frontal regions (in the western Africa upwelling region) (Figures 7A, 8). The depth-oriented nDVM more frequently observed in lower latitudes of warmer, deep chlorophyll a maxima and oligotrophic waters (Figure 8).
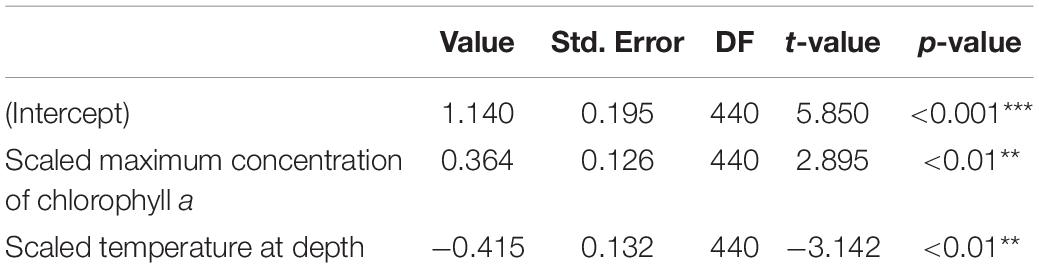
Table 2. Summary of the generalised linear mixed model (GLMM) comparing shark day-time occupancy in shallow water (i.e., TAD above 250 m) in response to water temperature at depth (100 m) and concentration of the chlorophyll a maximum (n = 456; p-value: ***<0.001; **<0.01).
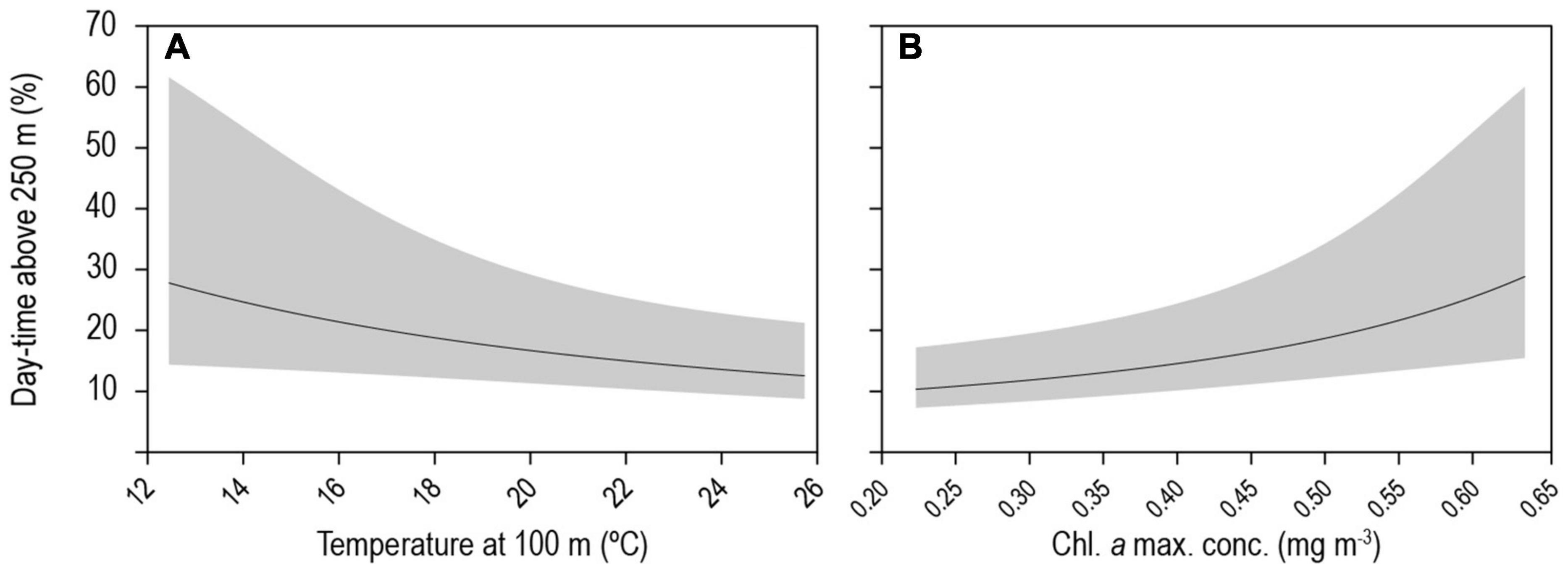
Figure 6. Generalised linear mixed model (GLMM) relationships between blue shark day-time occupancy above 250 m depth and environmental variables. GLMM predicted probabilities of depth-use along (A) temperature at depth (100 m) and (B) concentration of the chlorophyll a maximum. Continuous lines represent mean predicted values for the species and shaded areas represent the 95% confidence intervals.
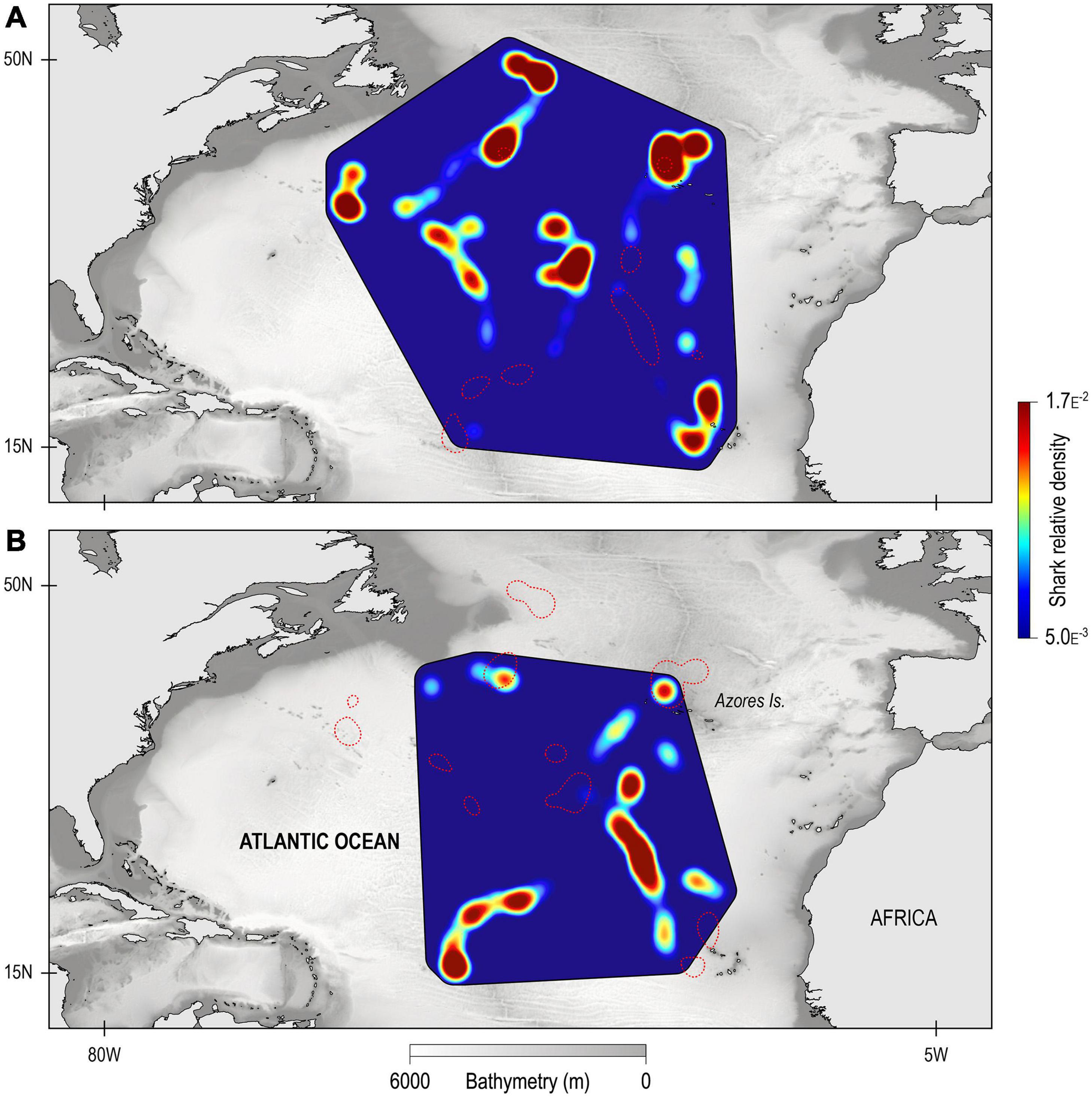
Figure 7. Kernel density estimates of space-use of tracked blue sharks, for (A) nDVM and (B) depth-oriented nDVM. The red dashed line denotes the 90th percentile for depth-oriented nDVM (top) and nDVM (bottom) shown to exemplify the mismatch in hotspot location of the different behaviours.
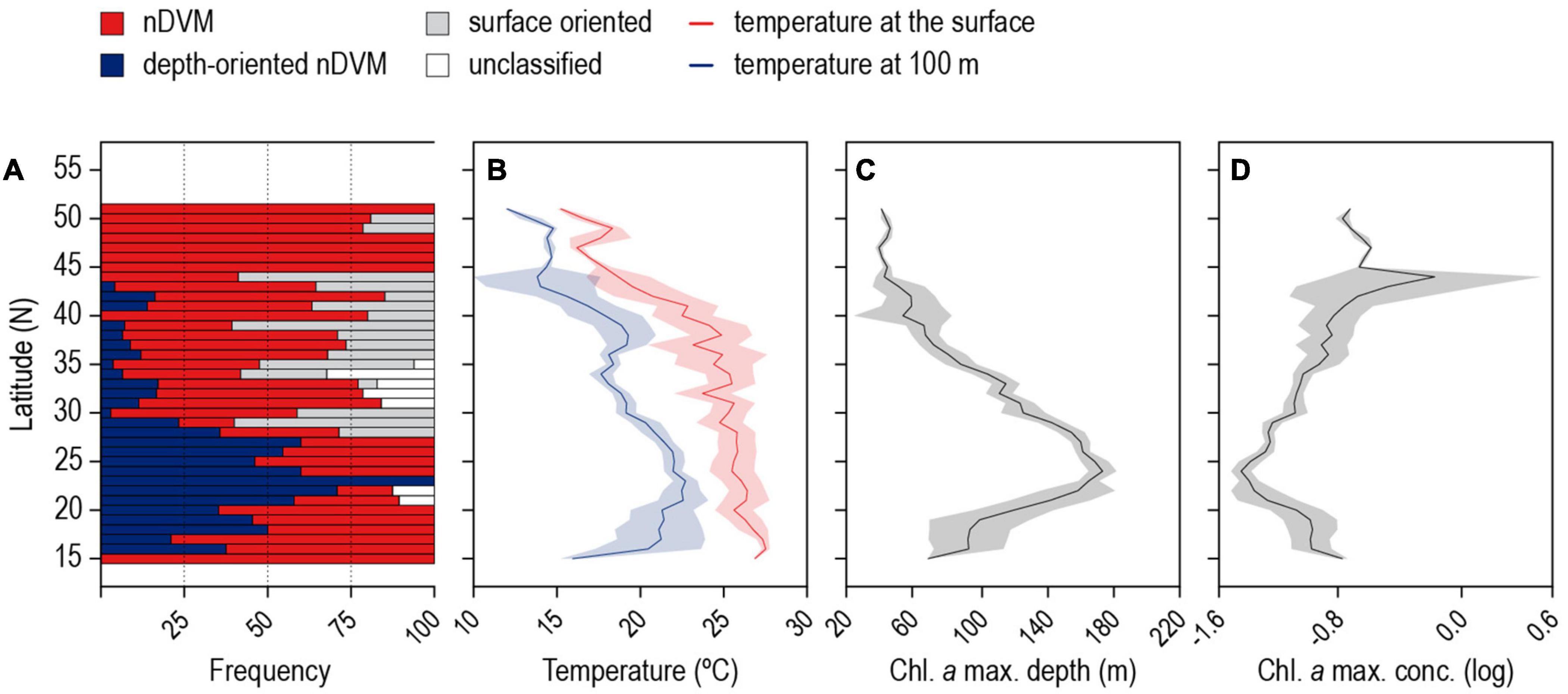
Figure 8. Latitudinal variation between 15 and 51°N for the (A) frequency of classified diel behaviours, (B) average temperature at the surface (red) and at 100 m depth (blue), (C) depth and (D) concentration of the chlorophyll a maximum in the water column.
Lunar Phase and Overlap With Surface Longlining Hooks
During nights of new moon, occupation of surface waters (less than 100 m; average: 81.9% ± 22.4) was higher than in nights of full moon (70.6% ± 25.5; Wilcoxon rank sum test, W = 15862, α = 0.05, p < 0.001, n = 424). However, TADhook was significantly greater on nights of full moon when compared to nights of new moon (15.55% ± 22.28 and 9.37% ± 18.06, respectively; Wilcoxon rank sum test, W = 25475, α = 0.05, p < 0.01, n = 424). In fact, along shark geolocations, both fishing effort (890 ± 611 fishing days) as well as CPUE were also highest on nights of full moon (278 ± 144 kg grid cell–1 set–1) when compared to nights of new moon (687 ± 443 days and 253 ± 192 kg grid cell–1 set–1, respectively; Wilcoxon rank sum test, fishing effort: W = 25475, α = 0.05, p < 0.01, n = 284; CPUE: W = 5777, α = 0.05, p < 0.01, n = 194).
Discussion
Blue sharks performed extensive movements covering a large area of the North Atlantic, with results showing that diel behaviours shifted in response to environmental changes. Specifically, sharks (i) spent more time at depth in less productive, warmer, and deep chlorophyll a maximum regions, and (ii) performed behavioural patterns linked with surface occupancy in colder, more productive waters. The latter was also associated with a higher spatio-temporal overlap with hook depths and thus, higher fishing risk in these areas, particularly on nights of full moon.
Horizontal Movements
Tracked sharks performed typical, extensive movements in the open ocean of the North Atlantic and also into shelf waters. Such movements are generally thought to be part of long-distance, seasonal migrations linked with foraging and/or reproduction (Queiroz et al., 2005; Nakano and Stevens, 2008; Stevens, 2010). During summer, blue sharks typically migrate north following the warming of surface waters at higher latitudes (Queiroz et al., 2010), exemplified by known movements into productive waters of the northern Gulf Stream and North Atlantic Current (Queiroz et al., 2019). Movements into shelf waters also occur, which allows sharks to forage on shelf-associated seasonal schools of small pelagic fish and tuna (Henderson et al., 2001; Southward et al., 2004). Hence, the summer diet of blue sharks in northern latitudes has been described to shift from being typically comprised of cephalopods to a preference for teleosts (Stevens, 1973).
Besides performing extensive horizontal movements, blue sharks we tracked also displayed high residency in specific regions, broadly associated with mesoscale oceanic features, such as thermal fronts and upwelling regions. For example, individuals tracked in the West Atlantic showed greater occupation of the Gulf Stream and the NAC-LCCZ, both frontal regions of high primary productivity and forage accumulation, where megafauna is known to aggregate (Campana et al., 2011; Scales et al., 2014; Braun et al., 2019b). Similarly, sharks tagged in the mid-Atlantic, near the Azores archipelago showed a longer residence within this area, which has been previously described as a preferred wintering ground for this species (Vandeperre et al., 2014). Three sharks displayed southward movements during the winter into the tropical Atlantic likely following warm SST isotherms toward southern waters (Queiroz et al., 2012). Female blue sharks S7 and S14 moved into the Eastern Tropical Atlantic (ETA), a highly productive area associated with the strong West African upwelling regime (Karstensen et al., 2008; Stramma et al., 2010; Gilly et al., 2013).
Diel Vertical Movements
The majority of tracked sharks in this study displayed a cyclical behaviour, with 11 out of 15 individuals showing a diel rhythm [peak within 23–25 h range; (Campbell et al., 2010)]. The vertical movements for one individual were cyclic at 12 h (semi-diel rhythm; S3) indicating circatidal movements (Shepard et al., 2006). However, no diel behaviour was detected (i.e., the periodogram was non-significant) for three individuals. These were either tracked for short periods of time (seven to 14 days, for S12 and S11, respectively) or were frequently recorded diving to extreme depths (S8). In this last case, the irregularity of deep dives along the tracking period could have reduced the chance of detecting cyclical patterns in the maximum depth, and thus a significant diel pattern. Nonetheless, using Lomb-Scargle periodograms we were able to identify cyclical rhythms for the majority of the tracked sharks, likely because it is robust to the presence of non-regular and zero-inflated data, both of which are usually considered to be typical disadvantages of satellite relayed telemetry data (Ruf, 2010; Schaffeld et al., 2016).
Interestingly, this study observed for the first time, to our knowledge, semi-diel movements for pelagic sharks in the open ocean. Instead, these have been often described for sharks associated with coastal and shelf areas, where the cyclical tidal transport of zooplankton influences the behaviour of predators in higher trophic levels (Shepard et al., 2006; Rodríguez-Cabello et al., 2016). Nonetheless, shark S3 was tracked moving exclusively in the open ocean, with frequent excursions into the bathyal (maximum dive depth of 1,704 m), possibly associated with deep-water foraging on organisms influenced by semi-diel patterns in deep-water currents. Indeed, semi-diel vertical migrations have also been observed in open-ocean deep-sea communities at over 1,000 m depth (Aguzzi et al., 2010, 2018), where tidal effects propagate throughout the water column from changes in strength and direction of deep-water currents (Uiblein et al., 2002; Trenkel et al., 2004; Lorance and Trenkel, 2006; Aguzzi et al., 2010) and by variations in temperature and salinity (Ratsimandresy et al., 2017). Furthermore, bathymetric features, such as deep-sea banks or ridges, create a displacement in the water column structure and on deep-water currents (Genin, 2004; Cotté and Simard, 2005), potentially favouring plankton advection from the bathyal to shallower depths, thereby acting as an upwelling area highly attractive for pelagic predators (Genin, 2004). Despite this, a semi-diel rhythm was only observed for one of the tracked individuals, with most sharks performing cyclic diel behaviour.
DVM can be observed at a global scale, from plankton to top predators (Longhurst and Harrison, 1989; Zhang and Dam, 1997), being possibly associated with thermoregulatory movements and/or as an optimisation of foraging (e.g., Sims et al., 2006; Last et al., 2016; Hafker et al., 2017). For example, by reducing day-time activity in deep, cold waters and foraging at night in shallow, warm waters, catsharks enhance energetic efficiency by conserving metabolic expenditure (Sims et al., 2006). Similarly, the blue sharks we tracked experienced significantly lower temperatures during the day than at night. This pattern was consistent for the three identified diel behaviours. Hence, we cannot exclude that blue sharks benefit from energy conservation by occupying colder waters during the day; however, our findings show no evidence that sharks re-surface at night to recover from heat loss, since day-time mean temperatures were positively correlated with the successive night (both using histogram or archival data) which does not support the behavioural thermoregulation hypothesis from the data we collected. If blue sharks were thermoregulating on a diel basis a negative relationship between daytime temperatures and consecutive nighttime temperatures is expected, where the colder the time-weighted temperatures experienced during the day were, the warmer the temperatures at night were expected to be (Thums et al., 2013); however, this was not the pattern we observed. It is possible that deep dives undertaken during the night would have been observed if sharks were diving to reduce internal temperature (Campana et al., 2011), however, during this period, the blue sharks we tracked remained largely in shallow waters above 250 m. Hence, our collective results indicate that the vertical patterns we recorded were most likely linked to prey aggregations and associated depth changes. The occupation of near-surface depths at night together with day-time mesopelagic dives were indicative of tracked blue sharks maximising the day/night spatial overlap with aggregations of diel vertically migrating prey, potentially increasing foraging success (Campana et al., 2011; Queiroz et al., 2012; Hammerschlag et al., 2016). Furthermore, regular nDVM and surface-oriented behaviour of sharks in this study were frequently observed associated with areas where prey typically aggregates near the surface, such as frontal regions, e.g., the NAC-LCCZ and the western Africa upwelling region (Sims et al., 2006; Campana et al., 2011). In these areas, sharks also maximised time in shallow layers with high chlorophyll a concentration, where prey densities are putatively higher (Ainley et al., 2005).
Vertical movements largely driven by tracking prey aggregations could also explain our observation of depth-oriented nDVM occurring in warm, oligotrophic surface waters in the mid-Atlantic region, where tracked blue sharks spent a larger proportion of the daytime targetting layers of maximum productivity at depth. The increased vertical overlap with layers of chlorophyll a maxima suggests blue sharks were targetting prey aggregations at depth (Bianchi et al., 2013), such as energetically profitable squid (Clarke et al., 1996; Galván-Magaña et al., 2013). Shifts in diel behaviours (for example, from regular nDVM to a distinctive depth-oriented nDVM) in response to increases in the abundance of deep-water prey, such as cephalopods or mesopelagic fish, were previously observed for blue sharks in the North Atlantic (Campana et al., 2011; Queiroz et al., 2012). Moreover, other top predators that feed on similar vertically migrating prey, such as tuna and swordfish, also displayed consistent cyclical tracking of the deep scattering layer (DSL), likely to maximise foraging success (Schaefer et al., 2009; Dewar et al., 2011; Sepulveda et al., 2018). Finally, our results demonstrate that the frequency of diel diving patterns showed a latitudinal gradient, with regular and depth-oriented nDVM generally observed in non-overlapping regions of the North Atlantic at higher latitudes, and higher day-time occupation of deep waters occurring toward lower latitudes. Taken together, our results suggest that the vertical movements of blue sharks were intricately linked to the water column profile, and consequently, to prey distribution patterns.
Lunar Phase and Overlap With Surface Longlining Hooks
During nights of new moon blue shark dives were generally restricted to shallow waters (between the surface and 100 m depth), while on nights of full moon, sharks displayed an increased occupation of depths between 100 and 250 m. Although several studies have related fish vertical movement shifts to the lunar phase (e.g., Bestley et al., 2009; Abascal et al., 2010; Eveson et al., 2018), few studies have described this behaviour for elasmobranchs (Graham et al., 2006; Shepard et al., 2006; Hammerschlag et al., 2016). For example, planktivorous basking sharks showed cyclical lunar activity, with an increased diving frequency fortnightly (Shepard et al., 2006), while the depth of whale sharks varied with lunar illumination, with shallower dives during snapper spawning events in full moon, likely to maximise foraging on fish eggs (Graham et al., 2006). Hence, occupation of deeper waters by blue sharks during nights of full moon may be driven by the phototaxic response of prey to higher luminosity near the surface (identical to the predator avoidance mechanisms triggering DVM) and, thus, prey species are found in deeper layers than on dark new moon nights (Lerner et al., 2013).
Catches of surface longliners targetting swordfish, tunas and sharks are generally higher in periods of increased lunar illumination (Podesta et al., 1993; Curran, 2014; Orbesen et al., 2017). In our study, an increased occupation of deeper depth layers during nights of full moon led to higher overlap with longline hooks [which are generally set 100–300 m depth (Domingo et al., 2016)], which is especially relevant since blue sharks are a commercially important species. We showed that fishing effort (i.e., number of fishing vessels per grid cell) and blue shark catches (CPUE from logbook catch data) were also higher during nights of full moon at the locations where blue sharks were tracked. Therefore, during this period blue sharks were more vulnerable to fishing activity, not only vertically (higher likelihood of hook encounter), but also geographically due to higher fishing pressure occurring in the region.
Conclusion
In summary, we found that the diel diving behaviours of blue sharks in relation to environmental fields and lunar illumination act to change the sharks’ vulnerability to fishing effort. Greater occupancy of cold, highly productive shallow waters was observed, where susceptibility to hook encounter was also high. Previous studies have linked an increased space-use of highly productive frontal areas by pelagic sharks (including blue sharks) to a higher exposure risk to fishing (Queiroz et al., 2016, 2019). However, despite the large decline of shark populations and the high extinction risk (Baum et al., 2003; Pacoureau et al., 2021), exploitation of the stocks of oceanic sharks has only recently been regulated in the North Atlantic (International Commission for the Conservation of Atlantic Tunas [ICCAT], 2020). Nonetheless, management measures of longline fisheries could be further improved by minimising shark mortality, for example, by implementing spatial management such as seasonal closures and marine protected areas (MPAs), enforcing adaptations to depth of hook deployment, and stronger selectivity of target species by fishing gear to reduce bycatch (Queiroz et al., 2016, 2019; Morgan et al., 2020; Pacoureau et al., 2021). Knowing where and when sharks are more vulnerable to fishing, such as we show here for adult blue sharks, will be important for the implementation of novel dynamic approaches for assessing the status of threatened oceanic sharks (Hays et al., 2019).
Data Availability Statement
The raw data supporting the conclusions of this article will be made available by the authors, without undue reservation.
Ethics Statement
The animal study was reviewed and approved by the Marine Biological Association of the United Kingdom (MBA) Animal Welfare Ethical Review Body (AWERB).
Author Contributions
NQ and DWS planned the data analysis. NQ led the data analysis with contributions from MV and DWS. MV led the manuscript writing with contributions from NQ, DWS, and all other authors. All authors approved the work for publication.
Funding
Funding was provided by the Save Our Seas Foundation (DWS and NQ), Fundação para a Ciência e a Tecnologia (FCT) under the grants PTDC/MAR/100345/2008 and COMPETE FCOMP-01-0124-FEDER-010580 and PTDC/BIA-COM/28855/2017 and COMPETE POCI-01–0145-FEDER-028855 (NQ and DWS), Natural Environment Research Council (NERC), United Kingdom (NE/R00997/X/1), European Research Council (ERC-AdG-2019 883583 OCEAN DEOXYFISH), NERC Oceans 2025 Strategic Programme (all to DWS), MARINFO–NORTE-01–0145-FEDER-000031 [funded by Norte Portugal Regional Operational Programme (NORTE2020), under the PORTUGAL 2020 Partnership Agreement, through the European Regional Development Fund (ERDF) to NQ], European Maritime and Fisheries Fund MAR-01.04.02-FEAMP-0006 (RR and NQ), and Xunta de Galicia, Spain, under the Isabel Barreto Programme 2009–2012 (GM). FCT supported NQ (CEECIND/02857/2018), MV (PTDC/BIA-COM/28855/2017), and GM (PTDC/MAR-BIO/4458/2012). DWS was supported by a Marine Biological Association Senior Research Fellowship.
Conflict of Interest
The authors declare that the research was conducted in the absence of any commercial or financial relationships that could be construed as a potential conflict of interest.
Acknowledgments
This research is part of the Global Shark Movement Project (globalsharkmovement.org).
Supplementary Material
The Supplementary Material for this article can be found online at: https://www.frontiersin.org/articles/10.3389/fmars.2021.688076/full#supplementary-material
Supplementary Figure 1 | Archival blue shark depth use (A) for a 24 h period, and (B) during night-time. Panel above represents the mean light-level for a 24 h period. Shaded red areas represent the twilight periods (time bins between 06:00–12:00 and 18:00–00:00) that were excluded from the analyses. Red line denotes the depth threshold used to classify differences in diel behaviour (i.e., 250 m depth). Notice in (B), that 97.5% of night-time occupancy occurred above this depth threshold.”
Supplementary Figure 2 | Tracks for 15 blue sharks overlaid on the average 2010/2011 sea surface temperature.
Supplementary Figure 3 | Pearson residuals from GLMM between blue shark day-time occupancy above 250 m depth and environmental variables. Pearson residuals along (A) fitted values; (B) concentration of the chlorophyll a maximum and (C) temperature at depth (100 m). (D) Quantile-quantile plot for the Pearson residuals.
Supplementary Figure 4 | Kernel density estimates of space-use of tracked blue sharks for the surface-oriented behaviour. The red dashed line represents the 90th percentile for depth-oriented nDVM shown to exemplify the mismatch in hotspot location of the different behaviours.
References
Abascal, F. J., Mejuto, J., Quintans, M., and Ramos-Cartelle, A. (2010). Horizontal and vertical movements of swordfish in the Southeast Pacific. ICES J. Mar. Sci. 67, 466–474. doi: 10.1093/icesjms/fsp252
Abascal, F. J., Mejuto, J., Quintans, M., García-Cortés, B., and Ramos-Cartelle, A. (2015). Tracking of the broadbill swordfish, Xiphias gladius, in the central and eastern North Atlantic. Fish. Res. 162, 20–28. doi: 10.1016/j.fishres.2014.09.011
Abecassis, M., Dewar, H., Hawn, D., and Polovina, J. (2012). Modeling swordfish daytime vertical habitat in the North Pacific Ocean from pop-up archival tags. Mar. Ecol. Prog. Ser. 452, 219–236. doi: 10.3354/meps09583
Aguzzi, J., Costa, C., Furushima, Y., Chiesa, J. J., Company, J. B., Menesatti, P., et al. (2010). Behavioral rhythms of hydrocarbon seep fauna in relation to internal tides. Mar. Ecol. Prog. Ser. 418, 47–56. doi: 10.3354/meps08835
Aguzzi, J., Fanelli, E., Ciuffardi, T., Schirone, A., De Leo, F. C., Doya, C., et al. (2018). Faunal activity rhythms influencing early community succession of an implanted whale carcass offshore Sagami Bay, Japan. Sci. Rep. 8:11163. doi: 10.1038/s41598-018-29431-5
Ainley, D. G., Spear, L. B., Tynan, C. T., Barth, J. A., Pierce, S. D., Glenn Ford, R., et al. (2005). Physical and biological variables affecting seabird distributions during the upwelling season of the northern California Current. Deep Sea Res. II Top. Stud. Oceanogr. 52, 123–143. doi: 10.1016/j.dsr2.2004.08.016
Andrews, K. S., Williams, G. D., Farrer, D., Tolimieri, N., Harvey, C. J., Bargmann, G., et al. (2009). Diel activity patterns of sixgill sharks, Hexanchus griseus: the ups and downs of an apex predator. Anim. Behav. 78, 525–536. doi: 10.1016/j.anbehav.2009.05.027
Andrzejaczek, S., Gleiss, A. C., Pattiaratchi, C. B., and Meekan, M. G. (2019). Patterns and drivers of vertical movements of the large fishes of the epipelagic. Rev. Fish Biol. Fish. 29, 335–354. doi: 10.1007/s11160-019-09555-1
Azzurro, E., Aguzzi, J., Maynou, F., José Chiesa, J., and Savini, D. (2012). Diel rhythms in shallow Mediterranean rocky-reef fishes: a chronobiological approach with the help of trained volunteers. J. Mar. Biol. Assoc. U.K. 93, 461–470. doi: 10.1017/s0025315412001166
Baum, J. K., Myers, R. A., Kehler, D. G., Worm, B., Harley, S. J., and Doherty, P. A. (2003). Collapse and conservation of shark populations in the Northwest Atlantic. Science 299, 389–392. doi: 10.1126/science.1079777
Bestley, S., Gunn, J. S., and Hindell, M. A. (2009). Plasticity in vertical behaviour of migrating juvenile southern bluefin tuna (Thunnus maccoyii) in relation to oceanography of the south Indian Ocean. Fish. Oceanogr. 18, 237–254. doi: 10.1111/j.1365-2419.2009.00509.x
Bianchi, D., Galbraith, E. D., Carozza, D. A., Mislan, K. A. S., and Stock, C. A. (2013). Intensification of open-ocean oxygen depletion by vertically migrating animals. Nat. Geosci. 6, 545–548. doi: 10.1038/ngeo1837
Boerder, K., Schiller, L., and Worm, B. (2019). Not all who wander are lost: improving spatial protection for large pelagic fishes. Mar. Policy 105, 80–90. doi: 10.1016/j.marpol.2019.04.013
Braun, C. D., Gaube, P., Afonso, P., Fontes, J., Skomal, G. B., and Thorrold, S. R. (2019a). Assimilating electronic tagging, oceanographic modelling, and fisheries data to estimate movements and connectivity of swordfish in the North Atlantic. ICES J. Mar. Sci. 76, 2305–2317.
Braun, C. D., Gaube, P., Sinclair-Taylor, T. H., Skomal, G. B., and Thorrold, S. R. (2019b). Mesoscale eddies release pelagic sharks from thermal constraints to foraging in the ocean twilight zone. Proc. Natl. Acad. Sci. U.S.A. 116, 17187–17192. doi: 10.1073/pnas.1903067116
Campana, S. E. (2016). Transboundary movements, unmonitored fishing mortality, and ineffective international fisheries management pose risks for pelagic sharks in the Northwest Atlantic. Can. J. Fish. Aquat. Sci. 73, 1599–1607. doi: 10.1139/cjfas-2015-0502
Campana, S. E., Dorey, A., Fowler, M., Joyce, W., Wang, Z., Wright, D., et al. (2011). Migration pathways, behavioural thermoregulation and overwintering grounds of blue sharks in the Northwest Atlantic. PLoS One 6:e16854. doi: 10.1371/journal.pone.0016854
Campbell, H. A., Sullivan, S., Read, M. A., Gordos, M. A., and Franklin, C. E. (2010). Ecological and physiological determinants of dive duration in the freshwater crocodile. Funct. Ecol. 24, 103–111. doi: 10.1111/j.1365-2435.2009.01599.x
Carey, F. G., Scharold, J. V., and Kalmijn, A. J. (1990). Movements of blue sharks (Prionace glauca) in depth and course. Mar. Biol. 106, 329–342. doi: 10.1007/bf01344309
Clarke, M. R., Clarke, D. C., Martins, H. R., and Silva, H. M. (1996). The diet of the blue shark (Prionace glauca L.) in Azorean waters.” Arquipélago. Life Mar. Sci. 14, 41–56.
Coffey, D. M., Carlisle, A. B., Hazen, E. L., and Block, B. A. (2017). Oceanographic drivers of the vertical distribution of a highly migratory, endothermic shark. Sci. Rep. 7:10434. doi: 10.1038/s41598-017-11059-6
Cotté, C., and Simard, Y. (2005). Formation of dense krill patches under tidal forcing at whale feeding hot spots in the St. Lawrence estuary. Mar. Ecol. Prog. Ser. 288, 199–210. doi: 10.3354/meps288199
Curran, D. S. (2014). “Shark catch in pelagic longline fisheries: a review of mitigation measures,” in Proceedings of the WCPFC Scientific Committee 10th Regular Session. WCPFC SC10-2014/EB-IP-11, Majuro, Republic of the Marshall Islands, (kolonia: WCPFC), 16.
Dewar, H., Prince, E. D., Musyl, M. K., Brill, R. W., Sepulveda, C., Luo, J., et al. (2011). Movements and behaviors of swordfish in the Atlantic and Pacific Oceans examined using pop-up satellite archival tags. Fish. Oceanogr. 20, 219–241. doi: 10.1111/j.1365-2419.2011.00581.x
Domingo, A., Forselledo, R., Miller, P., Jiménez, S., Mas, F., and Pons, M. (2016). General Description of Longline Fisheries. ICCAT Manual, 312. Madrid: ICCAT.
Duffy, L. M., Kuhnert, P. M., Pethybridge, H. R., Young, J. W., Olson, R. J., Logan, J. M., et al. (2017). Global trophic ecology of yellowfin, bigeye, and albacore tunas: understanding predation on micronekton communities at ocean-basin scales. Deep Sea Res. II Top. Stud. Oceanogr. 140, 55–73. doi: 10.1016/j.dsr2.2017.03.003
Dunn, D. C., Harrison, A. L., Curtice, C., DeLand, S., Donnelly, B., Fujioka, E., et al. (2019). The importance of migratory connectivity for global ocean policy. Proc. R. Soc. B Biol. Sci. 286:20191472. doi: 10.1098/rspb.2019.1472
Eveson, J. P., Patterson, T. A., Hartog, J. R., and Evans, K. (2018). Modelling surfacing behaviour of southern bluefin tuna in the great Australian bight. Deep Sea Res. II Top. Stud. Oceanogr. 157-158, 179–189. doi: 10.1016/j.dsr2.2018.03.007
Francisco, F. A., Nuhrenberg, P., and Jordan, A. (2020). High-resolution, non-invasive animal tracking and reconstruction of local environment in aquatic ecosystems. Mov. Ecol. 8:27. doi: 10.1186/s40462-020-00214-w
Galván-Magaña, F., Polo-Silva, C., Hernández-Aguilar, S. B., Sandoval-Londoño, A., Ochoa-Díaz, M. R., Aguilar-Castro, N., et al. (2013). Shark predation on cephalopods in the Mexican and Ecuadorian Pacific Ocean. Deep Sea Res. II Top. Stud. Oceanogr. 95, 52–62. doi: 10.1016/j.dsr2.2013.04.002
Genin, A. (2004). Bio-physical coupling in the formation of zooplankton and fish aggregations over abrupt topographies. J. Mar. Syst. 50, 3–20. doi: 10.1016/j.jmarsys.2003.10.008
Gilly, W. F., Beman, J. M., Litvin, S. Y., and Robison, B. H. (2013). Oceanographic and biological effects of shoaling of the oxygen minimum zone. Ann. Rev. Mar. Sci. 5, 393–420. doi: 10.1146/annurev-marine-120710-100849
Graham, R. T., Roberts, C. M., and Smart, J. C. (2006). Diving behaviour of whale sharks in relation to a predictable food pulse. J. R. Soc. Interface 3, 109–116. doi: 10.1098/rsif.2005.0082
Guzman, H. M., Gomez, C. G., Hearn, A., and Eckert, S. A. (2018). Longest recorded trans-Pacific migration of a whale shark (Rhincodon typus). Mar. Biodivers. Rec. 11:8. doi: 10.1186/s41200-018-0143-4
Hafker, N. S., Meyer, B., Last, K. S., Pond, D. W., Huppe, L., and Teschke, M. (2017). Circadian clock involvement in zooplankton diel vertical migration. Curr. Biol. 27, 2194–2201.e3. doi: 10.1016/j.cub.2017.06.025
Hammerschlag, N., Skubel, R. A., Calich, H., Nelson, E. R., Shiffman, D. S., Wester, J., et al. (2016). Nocturnal and crepuscular behavior in elasmobranchs: a review of movement, habitat use, foraging, and reproduction in the dark. Bull. Mar. Sci. 93, 355–374. doi: 10.5343/bms.2016.1046
Hays, G. C. (2003). A review of the adaptive significance and ecosystem consequences of zooplankton diel vertical migrations. Hydrobiologia 503, 163–170. doi: 10.1023/B:HYDR.0000008476.23617.b0
Hays, G. C., Bailey, H., Bograd, S. J., Bowen, W. D., Campagna, C., Carmichael, R. H., et al. (2019). Translating marine animal tracking data into conservation policy and management. Trends Ecol. Evol. 34, 459–473. doi: 10.1016/j.tree.2019.01.009
Henderson, A. C., Flannery, K., and Dunne, J. (2001). Observations on the biology and ecology of the blue shark in the North-east Atlantic. J. Fish Biol. 58, 1347–1358. doi: 10.1111/j.1095-8649.2001.tb02291.x
Hindell, M. A., Reisinger, R. R., Ropert-Coudert, Y., Hückstädt, L. A., Trathan, P. N., Bornemann, H., et al. (2020). Tracking of marine predators to protect Southern Ocean ecosystems. Nature 580, 87–92. doi: 10.1038/s41586-020-2126-y
Howey-Jordan, L. A., Brooks, E. J., Abercrombie, D. L., Jordan, L. K., Brooks, A., Williams, S., et al. (2013). Complex movements, philopatry and expanded depth range of a severely threatened pelagic shark, the oceanic whitetip (Carcharhinus longimanus) in the western North Atlantic. PLoS One 8:e56588. doi: 10.1371/journal.pone.0056588
Humphries, N. E., Queiroz, N., Dyer, J. R., Pade, N. G., Musyl, M. K., Schaefer, K. M., et al. (2010). Environmental context explains levy and brownian movement patterns of marine predators. Nature 465, 1066–1069. doi: 10.1038/nature09116
International Commission for the Conservation of Atlantic Tunas [ICCAT] (2020). International Commission for The Conservation of Atlantic Tunas Report for biennial period, 2018-19 PART II (2019). Madrid: ICCAT.
Johnson, D. S., London, J. M., Lea, M. A., and Durban, J. W. (2008). Continuous-time correlated random walk model for animal telemetry data. Ecology 89, 1208–1215. doi: 10.1890/07-1032.1
Jonsen, I. D., Flemming, J. M., and Myers, R. A. (2005). Robust state–space modeling of animal movement data. Ecology 86, 2874–2880. doi: 10.1890/04-1852
Karstensen, J., Stramma, L., and Visbeck, M. (2008). Oxygen minimum zones in the eastern tropical Atlantic and Pacific oceans. Prog. Oceanogr. 77, 331–350. doi: 10.1016/j.pocean.2007.05.009
Kroodsma, D. A., Mayorga, J., Hochberg, T., Miller, N. A., Boerder, K., Ferretti, F., et al. (2018). Tracking the global footprint of fisheries. Science 359, 904–908. doi: 10.1126/science.aao5646
Lam, C. H., Nielsen, A., and Sibert, J. R. (2008). Improving light and temperature based geolocation by unscented Kalman filtering. Fish. Res. 91, 15–25. doi: 10.1016/j.fishres.2007.11.002
Lampert, W. (1989). The adaptive significance of diel vertical migration of zooplankton. Funct. Ecol. 3, 21–27. doi: 10.2307/2389671
Last, K. S., Hobbs, L., Berge, J., Brierley, A. S., and Cottier, F. (2016). Moonlight drives ocean-scale mass vertical migration of zooplankton during the Arctic winter. Curr. Biol. 26, 244–251. doi: 10.1016/j.cub.2015.11.038
Lerner, J. D., Kerstetter, D. W., Prince, E. D., Talaue-McManus, L., Orbesen, E. S., Mariano, A., et al. (2013). Swordfish vertical distribution and habitat use in relation to diel and lunar cycles in the Western North Atlantic. Trans. Am. Fish. Soc. 142, 95–104. doi: 10.1080/00028487.2012.720629
Lomb, N. R. (1976). Least-squares frequency analysis of unequally spaced data. Astrophys. Space Sci. 39, 447–462. doi: 10.1007/bf00648343
Longhurst, A. R., and Harrison, W. G. (1989). The biological pump: profiles of plankton production and consumption in the upper ocean. Prog. Oceanogr. 22, 47–123. doi: 10.1016/0079-6611(89)90010-4
Lorance, P., and Trenkel, V. M. (2006). Variability in natural behaviour, and observed reactions to an ROV, by mid-slope fish species. J. Exp. Mar. Biol. Ecol. 332, 106–119. doi: 10.1016/j.jembe.2005.11.007
Mejuto, J., and García-Cortés, B. (2005). Reproductive and distribution parameters of the blue shark Prionace glauca, on the basis of on-board observations at sea in the Atlantic, Indian and Pacific Oceans. Collect. Vol. Sci. Pap. ICCAT 58, 974–1000.
Morgan, A., Calich, H., Sulikowski, J., Hammerschlag, N., and Yates, K. (2020). Evaluating spatial management options for tiger shark (Galeocerdo cuvier) conservation in US Atlantic Waters. ICES J. Mar. Sci. 77, 3095–3109. doi: 10.1093/icesjms/fsaa193
Musyl, M. K., Brill, R. W., Boggs, C. H., Curran, D. S., Kazama, T. K., and Seki, M. P. (2003). Vertical movements of bigeye tuna (Thunnus obesus) associated with islands, buoys, and seamounts near the main Hawaiian Islands from archival tagging data. Fish. Oceanogr. 12, 152–169. doi: 10.1046/j.1365-2419.2003.00229.x
Nakano, H., and Stevens, J. D. (2008). “The biology and ecology of the blue shark, Prionace glauca,” in Sharks of the Open Ocean: Biology, Fisheries and Conservation, eds M. D. Camhi, E. K. Pikitch, and E. A. Babcock (Oxford: Blackwell Scientific), 140–151.
Olivar, M., Pilar, P., Hulley, A., Castellón, A., Emelianov, M., López, C., et al. (2017). Mesopelagic fishes across the tropical and equatorial Atlantic: biogeographical and vertical patterns. Prog. Oceanogr. 151, 116–137. doi: 10.1016/j.pocean.2016.12.001
Orbesen, E. S., Snodgrass, D., Shideler, G. S., Brown, C. A., and Walter, J. F. (2017). Diurnal patterns in Gulf of Mexico epipelagic predator interactions with pelagic longline gear: implications for target species catch rates and bycatch mitigation. Bull. Mar. Sci. 93, 573–589. doi: 10.5343/bms.2016.1008
Pacoureau, N., Rigby, C. L., Kyne, P. M., Sherley, R. B., Winker, H., Carlson, J. K., et al. (2021). Half a century of global decline in oceanic sharks and rays. Nature 589, 567–571. doi: 10.1038/s41586-020-03173-9
Pade, N. G., Queiroz, N., Humphries, N. E., Witt, M. J., Jones, C. S., Noble, L. R., et al. (2009). First results from satellite-linked archival tagging of porbeagle shark, Lamna nasus: area fidelity, wider-scale movements and plasticity in diel depth changes. J. Exp. Mar. Biol. Ecol. 370, 64–74. doi: 10.1016/j.jembe.2008.12.002
Papastamatiou, Y. P., Watanabe, Y. Y., Demsar, U., Leos-Barajas, V., Bradley, D., Langrock, R., et al. (2018). Activity seascapes highlight central place foraging strategies in marine predators that never stop swimming. Mov. Ecol. 6:9. doi: 10.1186/s40462-018-0127-3
Podesta, G. P., Browder, J. A., and Hoey, J. J. (1993). Exploring the association between swordfish catch rates and thermal fronts on US longline grounds in the western North Atlantic. Cont. Shelf Res. 13, 253–277.
Poisson, F., Gaertner, J. C., Taquet, M., Durbec, J. P., and Bigelow, K. (2010). Effects of lunar cycle and fishing operations on longline-caught pelagic fish: fishing performance, capture time, and survival of fish. Fish. Bull. 108, 268–281.
Punt, A. E. (2019). Spatial stock assessment methods: a viewpoint on current issues and assumptions. Fish. Res. 213, 132–143. doi: 10.1016/j.fishres.2019.01.014
Queiroz, N., Humphries, N. E., Couto, A., Vedor, M., da Costa, I., Sequeira, A. M. M., et al. (2019). Global spatial risk assessment of sharks under the footprint of fisheries. Nature 572, 461–466. doi: 10.1038/s41586-019-1444-4
Queiroz, N., Humphries, N. E., Mucientes, G., Hammerschlag, N., Lima, F. P., Scales, K. L., et al. (2016). Ocean-wide tracking of pelagic sharks reveals extent of overlap with longline fishing hotspots. Proc. Natl. Acad. Sci. U.S.A. 113, 1582–1587. doi: 10.1073/pnas.1510090113
Queiroz, N., Humphries, N. E., Noble, L. R., Santos, A. M., and Sims, D. W. (2010). Short-term movements and diving behaviour of satellite-tracked blue sharks Prionace glauca in the northeastern Atlantic Ocean. Mar. Ecol. Prog. Ser. 406, 265–279. doi: 10.3354/meps08500
Queiroz, N., Humphries, N. E., Noble, L. R., Santos, A. M., and Sims, D. W. (2012). Spatial dynamics and expanded vertical niche of blue sharks in oceanographic fronts reveal habitat targets for conservation. PLoS One 7:e32374. doi: 10.1371/journal.pone.0032374
Queiroz, N., Lima, F. P., Maia, A., Ribeiro, P. A., Correia, J. P., and Santos, A. M. (2005). Movement of Blue Shark, Prionace glauca, in the North-East Atlantic based on mark - recapture data. J. Mar. Biol. Assoc. U.K. 85, 1107–1112. doi: 10.1017/s0025315405012154
Queiroz, N., Vila-Pouca, C., Couto, A., Southall, E. J., Mucientes, G., Humphries, N. E., et al. (2017). Convergent foraging tactics of marine predators with different feeding strategies across heterogeneous ocean environments. Front.Mar. Sci. 4:239. doi: 10.3389/fmars.2017.00239
Ratsimandresy, A., Hamoutene, D., Lang, C., MacSween, C., Marshall, K., Kenny, S., et al. (2017). Arctic Charr (Salvelinus Alpinus) Distribution in Seawater Cages in Relation to Environmental Conditions: Fisheries and Oceans Canada= Pêches et océans Canada. Canadian Technical Report of Fisheries and Aquatic Sciences. Fs97-6/3203E-PDF: vi + 20 p. St. John’s, NL: Fisheries and Oceans Canada.
Robinson, N. M., Nelson, W. A., Costello, M. J., Sutherland, J. E., and Lundquist, C. J. (2017). A systematic review of marine-based Species Distribution Models (SDMs) with recommendations for best practice. Front. Mar. Sci. 4:421. doi: 10.3389/fmars.2017.00421
Rodríguez-Cabello, C., González-Pola, C., and Sánchez, F. (2016). Migration and diving behavior of Centrophorus squamosus in the NE Atlantic. combining electronic tagging and Argo hydrography to infer deep ocean trajectories. Deep Sea Res. I Oceanogr. Res. Pap. 115, 48–62. doi: 10.1016/j.dsr.2016.05.009
Ruf, T. (2010). The lomb-scargle periodogram in biological rhythm research: analysis of incomplete and unequally spaced time-series. Biol. Rhythm Res. 30, 178–201. doi: 10.1076/brhm.30.2.178.1422
Scales, K. L., Miller, P. I., Hawkes, L. A., Ingram, S. N., Sims, D. W., Votier, S. C., et al. (2014). Review: on the front line: frontal zones as priority at-sea conservation areas for mobile marine vertebrates. J. Appl. Ecol. 51, 1575–1583. doi: 10.1111/1365-2664.12330
Scargle, J. D. (1982). Studies in astronomical time series analysis. II-statistical aspects of spectral analysis of unevenly spaced data. Astrophys. J. 263, 835–853.
Schaefer, K. M., and Fuller, D. W. (2002). Movements, behavior, and habitat selection of bigeye tuna (Thunnus obesus) in the eastern equatorial Pacific, ascertained through archival tags. Fish. Bull. 100, 765–788.
Schaefer, K. M., Fuller, D. W., and Block, B. A. (2009). “Vertical movements and habitat utilization of skipjack (Katsuwonus pelamis), yellowfin (Thunnus albacares), and bigeye (Thunnus obesus) tunas in the equatorial Eastern Pacific Ocean, ascertained through archival tag data,” in Tagging and Tracking of Marine Animals with Electronic Devices, eds J. L. Nielsen, H. Arrizabalaga, N. Fragoso, A. Hobday, M. Lutcavage, and J. Sibert (Dordrecht: Springer Netherlands), 121–144.
Schaffeld, T., Bräger, S., Gallus, A., Dähne, M., Krügel, K., Herrmann, A., et al. (2016). Diel and seasonal patterns in acoustic presence and foraging behaviour of free-ranging harbour porpoises. Mar. Ecol. Prog. Ser. 547, 257–272. doi: 10.3354/meps11627
Sepulveda, C. A., Aalbers, S. A., Heberer, C., Kohin, S., and Dewar, H. (2018). Movements and behaviors of swordfishXiphias gladiusin the United States Pacific leatherback conservation area. Fish. Oceanogr. 27, 381–394. doi: 10.1111/fog.12261
Sequeira, A. M. M., Hays, G. C., Sims, D. W., Eguíluz, V. M., Rodríguez, J. P., Heupel, M. R., et al. (2019). Overhauling ocean spatial planning to improve marine megafauna conservation. Front. Mar. Sci. 6:639. doi: 10.3389/fmars.2019.00639
Sequeira, A. M. M., Rodriguez, J. P., Eguiluz, V. M., Harcourt, R., Hindell, M., Sims, D. W., et al. (2018). Convergence of marine megafauna movement patterns in coastal and open oceans. Proc. Natl. Acad. Sci. U.S.A. 115, 3072–3077. doi: 10.1073/pnas.1716137115
Shepard, E. L. C., Ahmed, M. Z., Southall, E. J., Witt, M. J., Metcalfe, J. D., and Sims, D. W. (2006). Diel and tidal rhythms in diving behaviour of pelagic sharks identified by signal processing of archival tagging data. Mar. Ecol. Prog. Ser. 328, 205–213. doi: 10.3354/meps328205
Sims, D. W. (2003). Tractable models for testing theories about natural strategies: foraging behaviour and habitat selection of free-ranging sharks. J. Fish Biol. 63, 53–73. doi: 10.1111/j.1095-8649.2003.00207.x
Sims, D. W., Southall, E. J., Tarling, G. A., and Metcalfe, J. D. (2005). Habitat-specific normal and reverse diel vertical migration in the plankton-feeding basking shark. J. Anim. Ecol. 74, 755–761. doi: 10.1111/j.1365-2656.2005.00971.x
Sims, D. W., Wearmouth, V. J., Southall, E. J., Hill, J. M., Moore, P., Rawlinson, K., et al. (2006). Hunt warm, rest cool: bioenergetic strategy underlying diel vertical migration of a benthic shark. J. Anim. Ecol. 75, 176–190. doi: 10.1111/j.1365-2656.2005.01033.x
Song, L., Zhou, J., Zhou, Y., Nishida, T., Jiang, W., and Wang, J. (2009). Environmental preferences of bigeye tuna, Thunnus obesus, in the Indian Ocean: an application to a longline fishery. Environ. Biol. Fish. 85, 153–171. doi: 10.1007/s10641-009-9474-7
Southward, A. J., Langmead, O., Hardman-Mountford, N. J., Aiken, J., Boalch, G. T., Dando, P. R., et al. (2004). Long-term biological and environmental researches in the western English Channel. Adv. Mar. Biol 47, 1–105.
Stevens, J. D. (1973). Stomach contents of the blue shark (Prionace glauca L.) off south-west England. J. Mar. Biol. Assoc. U.K. 53, 357–361.
Stevens, J. D. (2010). “Epipelagic oceanic elasmobranchs,” in Sharks and Their Relatives II: Biodiversity, Adaptive Physiology and Conservation, eds J. C. Carrier, J. A. Musick, and M. R. Heithaus (Boca Raton, FL: CRC Press), 3–35.
Stramma, L., Schmidtko, S., Levin, L. A., and Johnson, G. C. (2010). Ocean oxygen minima expansions and their biological impacts. Deep Sea Res. I Oceanogr. Res. Pap. 57, 587–595. doi: 10.1016/j.dsr.2010.01.005
Tarling, G. (1999). The effect of lunar eclipse on the vertical migration behaviour of Meganyctiphanes norvegica (Crustacea: Euphausiacea) in the Ligurian Sea. J. Plankton Res. 21, 1475–1488. doi: 10.1093/plankt/21.8.1475
Teo, S. L. H., Boustany, A., Dewar, H., Stokesbury, M. J. W., Weng, K. C., Beemer, S., et al. (2006). Annual migrations, diving behavior, and thermal biology of Atlantic bluefin tuna, Thunnus thynnus, on their Gulf of Mexico breeding grounds. Mar. Biol. 151, 1–18. doi: 10.1007/s00227-006-0447-5
Thums, M., Meekan, M., Stevens, J., Wilson, S., and Polovina, J. (2013). Evidence for behavioural thermoregulation by the world’s largest fish. J. R. Soc. Interface 10:20120477. doi: 10.1098/rsif.2012.0477
Trenkel, V. M., Lorance, P., and Mahévas, S. (2004). Do visual transects provide true population density estimates for deepwater fish? ICES J. Mar. Sci. 61, 1050–1056.
Uiblein, F., Lorance, P., and Latrouite, D. (2002). Variation in locomotion behaviour in northern cutthroat eel (Synaphobranchus kaupi) on the Bay of Biscay continental slope. Deep Sea Res. I Oceanogr. Res. Pap. 49, 1689–1703. doi: 10.1016/s0967-0637(02)00065-1
Vandeperre, F., Aires-da-Silva, A., Fontes, J., Santos, M., Serrao Santos, R., and Afonso, P. (2014). Movements of blue sharks (Prionace glauca) across their life history. PLoS One 9:e103538. doi: 10.1371/journal.pone.0103538
Weng, K. C., Stokesbury, M. J., Boustany, A. M., Seitz, A. C., Teo, S. L., Miller, S. K., et al. (2009). Habitat and behaviour of yellowfin tuna Thunnus albacares in the Gulf of Mexico determined using pop-up satellite archival tags. J. Fish Biol. 74, 1434–1449. doi: 10.1111/j.1095-8649.2009.02209.x
Whitford, M., and Klimley, A. P. (2019). An overview of behavioral, physiological, and environmental sensors used in animal biotelemetry and biologging studies. Anim. Biotelem. 7:26. doi: 10.1186/s40317-019-0189-z
Wilson, S. G., Polovina, J. J., Stewart, B. S., and Meekan, M. G. (2005). Movements of whale sharks (Rhincodon typus) tagged at Ningaloo Reef, Western Australia. Mar. Biol. 148, 1157–1166. doi: 10.1007/s00227-005-0153-8
Wirsing, A. J., Heithaus, M. R., and Dill, L. (2011). Predator-induced modifications to diving behavior vary with foraging mode. Oikos 120, 1005–1012. doi: 10.1111/j.1600-0706.2010.18844.x
Zhang, X., and Dam, H. G. (1997). Downward export of carbon by diel migrant mesozooplankton in the central equatorial Pacific. Deep Sea Res. II Top. Stud. Oceanogr. 44, 2191–2202. doi: 10.1016/s0967-0645(97)00060-x
Keywords: diel vertical movement, longline fisheries, fishing risk, environment, lunar phase
Citation: Vedor M, Mucientes G, Hernández-Chan S, Rosa R, Humphries N, Sims DW and Queiroz N (2021) Oceanic Diel Vertical Movement Patterns of Blue Sharks Vary With Water Temperature and Productivity to Change Vulnerability to Fishing. Front. Mar. Sci. 8:688076. doi: 10.3389/fmars.2021.688076
Received: 30 March 2021; Accepted: 18 June 2021;
Published: 09 July 2021.
Edited by:
Mourier Johann, Institut de Recherche Pour le Développement (IRD), FranceReviewed by:
Daniel M. Coffey, Texas A&M University–Corpus Christi, United StatesYuuki Watanabe, National Institute of Polar Research, Japan
Copyright © 2021 Vedor, Mucientes, Hernández-Chan, Rosa, Humphries, Sims and Queiroz. This is an open-access article distributed under the terms of the Creative Commons Attribution License (CC BY). The use, distribution or reproduction in other forums is permitted, provided the original author(s) and the copyright owner(s) are credited and that the original publication in this journal is cited, in accordance with accepted academic practice. No use, distribution or reproduction is permitted which does not comply with these terms.
*Correspondence: Nuno Queiroz, nuno.queiroz@cibio.up.pt