- 1Institut Mediterrani d’Estudis Avançats (IMEDEA, CSIC-UIB), Esporles, Spain
- 2Department of Biology and Ecology of Fishes, Leibniz Institute of Freshwater Ecology and Inland Fisheries, Berlin, Germany
- 3Division of Integrative Fisheries Management, Faculty of Life Sciences, Humboldt-Universität zu Berlin, Berlin, Germany
Despite their potential to generate high-quality positioning data, the use of high-resolution acoustic telemetry systems (HRATS) has been neglected in coastal marine areas due to the limitations that these environments pose to the transmission of acoustic signals. In this work, we applied a HRATS and social network analysis (SNA) to study the social interactions of the pearly razorfish (Xyrichtys novacula), a small coastal wrasse, in a Mediterranean marine reserve. Our analysis was based on proximity measures estimated from high-resolution trajectories from 232 individuals tracked during 55 days within a marine protected area. Associations were defined as the proportion of 5-min intervals in which two individuals were observed within 1 m from each other, and social networks were generated for the overall tracking period and for each particular day. The obtained network parameters were contrasted against 1,000 null association models obtained by randomly redistributing individual trajectories within the study area. The obtained networks showed a harem-like social structure, with agonistic behavior between males and larger association indices between individuals of different sex. Occasionally, sporadic associations of large groups of females were observed conducting excursions along the study area. By providing a comprehensive view of the organizational structure of the pearly razorfish, our study demonstrates the potential of HRATS to efficiently produce high-throughput tracking data from large numbers of individuals and of proper null social model formulation to reconstruct the social networks in wild-living marine fish populations. The combination of HRATS and SNA represents a powerful tool to study key ecological processes regarding the social interactions of individuals, including social dynamics, collective movements, and the response to environmental perturbations.
Introduction
The complex social interactions that occur within populations and communities are a fundamental aspect in ecological research aimed at understanding the functioning of ecosystems and their resilience to external perturbations (Tylianakis et al., 2008; Ings et al., 2009). Social Network Analysis (SNA) is the predominant framework used to investigate the interactions between social animals (Wey et al., 2008; Krause et al., 2009; Jacoby and Freeman, 2016). SNA comprises a series of flexible tools that allow robust quantitative analyses to be conducted on social groups, considering them networks of nodes connected by social ties (Croft et al., 2008; Farine and Whitehead, 2015). The main aim of SNA is to analyze relevant behavioral features such as the social structure of populations (Papageorgiou et al., 2019), the individual behavioral variability (Mourier et al., 2019), the mating behavior (McDonald et al., 2013), and the transmission of information and disease (Couzin and Krause, 2003; Martínez-López et al., 2009; Stockmaier et al., 2021). Characterizing the causes that lead to the organization of animal societies provides a reference point to study social evolution in many different taxa (Grueter et al., 2020).
A crucial consideration when conducting a SNA resides in how interactions among individuals are defined and recorded because it conditions the social structure patterns that arise from the SNA (Marin and Wellman, 2011; Castles et al., 2014). Usually, SNA requires continuous monitoring of unequivocally identified individuals over long periods of time. Many traditional studies were based on observations of focal individuals or groups using visual tools, such as direct observations, video-cameras, and drones, to study the social structure of large-bodied vertebrates such as mammals (e.g., Connor et al., 2001; Cantor et al., 2012) and sharks (Mourier et al., 2012; Butcher et al., 2021). In recent years, the popularization of miniaturized GPS and other tracking devices has dramatically increased our ability to monitor the movements of terrestrial animals (Rutz et al., 2012; Kays et al., 2015), providing precise positioning data that allows interaction networks to be derived from the spatial proximity of individuals (Whitehead, 2008; Farine, 2015). Proximity-based networks are grounded on the obvious assumption that the co-occurrence of individuals in space and time is a prerequisite to form an interaction. However, proximity networks are not always a good indicator of the real interaction networks and can lead to different conclusions on the social structure of the studied organisms (Castles et al., 2014; Farine, 2015). Nevertheless, tracking devices have a great potential to gather information from a large number of individuals at the same time, especially if they are difficult to observe directly. Moreover, recent analysis tools, such as the utilization of random null models to infer the significance of the obtained network (Farine, 2017; Davis et al., 2018; Pasquaretta et al., 2021), provide analytical methods to obtain robust estimations of the observed social structures (Spiegel et al., 2016).
The application of proximity metrics for SNA in marine systems has been less used due to the limitation of tracking animals in aquatic environments at high temporal and spatial resolutions. GPS-based methods are not available underwater, posing significant challenges for assessing the high-resolution behavior of fishes in the wild (Krause et al., 2013). The most used technique to study the movement and behavior of aquatic animals, passive acoustic telemetry (Heupel et al., 2006; Hussey et al., 2015), has traditionally provided presence-absence data (i.e., detections of tagged animals by the acoustic receivers placed across the study area), and has been combined with SNA to study the connectivity between distant areas (Jacoby et al., 2012; Lédée et al., 2015) and to infer the association patterns in different fish species (Haulsee et al., 2016; Jacoby et al., 2016). In such analyses, the associations between individuals were inferred from the co-occurrence of individuals within the detection range of an acoustic receiver, either placed on a fixed position of the study area or attached to a focal individual (serving as a proximity sensor). The detection range of acoustic receivers is usually large (between 200 and 800 m) and greatly depends on the frequency of the biotransmitter. Consequently, each detection entails a considerable positional uncertainty that makes it difficult to assume an interaction when two individuals are detected by the same receiver as they might be several hundreds of meters apart from each other. Indeed, it has been seen that the network patterns that are obtained from passive acoustic telemetry are highly dependent on the acoustic range of the telemetry setup (Mourier et al., 2017). In addition, traditional passive acoustic telemetry techniques also present a limitation in terms of the number of individuals that can be monitored simultaneously due to the drastic reduction in the detection probability caused by the collisions between acoustic signals (Binder et al., 2016). These facts have limited the use of acoustic tracking and SNA to disentangle the architecture of marine societies.
With the emergence of high-resolution acoustic telemetry systems (HRATS), now it is possible to obtain trajectories of aquatic organisms with unprecedented detail (Niezgoda et al., 2002; Krause et al., 2013; Baktoft et al., 2015), allowing a better definition of the associations between individuals based on their spatial proximity. HRATS precisely locate individuals tagged with acoustic transmitters using hyperbolic multilateration algorithms (also known as reverse-GPS). To this end, acoustic signals must be detected by at least three different receivers in the array, which greatly limits the extension of the area that can be covered with a limited number of receivers. Moreover, new signal coding systems (e.g., the Binary-Phase Shift coding system, BPSK, Weiland et al., 2011) reduce the length of the emitted signals (<1 ms), decreasing the probability of signal collision to negligible levels. This allows for monitoring a representative fraction of a population (hundreds to thousands of individuals) at the same time, providing the opportunity to expand behavioral studies to the population level (Wey et al., 2008; Puga-Gonzalez et al., 2021). HRATS have been successfully used in freshwater environments, such as lakes (Baktoft et al., 2015) and rivers (Leander et al., 2020), and more recently, in marine environments (Aspillaga et al., 2021). By providing fine-scale positions of large numbers of tagged individuals, HRATS are a valuable tool to study the social structure of marine organisms based on proximity-based association networks, even with elusive small-sized species.
The objective of our study was to test the performance of HRATS and SNA characterizing the social structure of a free-living fish population in a marine environment, using the pearly razorfish (Xyrichtys novacula, Labridae) as a study species. The pearly razorfish is a small wrasse that inhabits coastal sandy bottoms in the Atlantic Ocean and the Mediterranean Sea and has a large socioeconomic value for local fisheries (Alós et al., 2016). It is a protogynous hermaphrodite with an evident sexual dimorphism, where males are larger than females and present a characteristic coloration pattern. It is a sedentary species with a small home range (ranging from 86 to 292 m2, Shen and Clark, 2016), living in polygynous social systems. Males establish and protect territories or harems that enclose the smaller territories of several females (Marconato et al., 1995; Shen and Clark, 2016). The harem-like territorial organization of this species is permanent and can be observed both inside and outside the spawning period, which occurs from July to September (Cardinale et al., 1998). Here, we applied the SNA approach on high-resolution trajectory data from a free-living pearly razorfish population to obtain a complete view of the social interactions and their temporal evolution.
Materials and Methods
High-Resolution Acoustic Telemetry System
The study was carried out in the Bay of Palma Marine Reserve (Mallorca, Balearic Islands, Spain, Figure 1). A small sand patch of 12.5 ha (600 m × 270 m), with depths ranging from 12 to 17 m and surrounded by a Posidonia oceanica seagrass meadow, was selected to install the acoustic telemetry array. This specific area was selected based on previous knowledge on the distribution and habitat preference of the species. Pearly razorfish individuals were tracked using the Juvenile Salmon Acoustic Telemetry System (JSATS, McMichael et al., 2010) manufactured by Lotek Wireless Inc. (Canada) and consisting of WHS-4250L receivers and L-AMT series transmitters (working frequency: 416.7 kHz). A total of 70 acoustic receivers were installed covering the abovementioned area using a between-receiver distance of around 50 m (Figure 1D). The array was installed in April 2019 and remained operational until October 2019, with the exceptions of the days from June 5–10, during which the receivers were temporally retrieved to download the data and perform maintenance tasks. The tracking period encompassed the spawning period of the pearly razorfish, which occurs between July and September (Cardinale et al., 1998). The performance of this HRATS system in the study area has been described by Aspillaga et al. (2021), demonstrating the potential of this system to generate trajectory data with a high spatial (few meters) and temporal (seconds) resolution while simultaneously monitoring hundreds of individuals without signal collision issues.
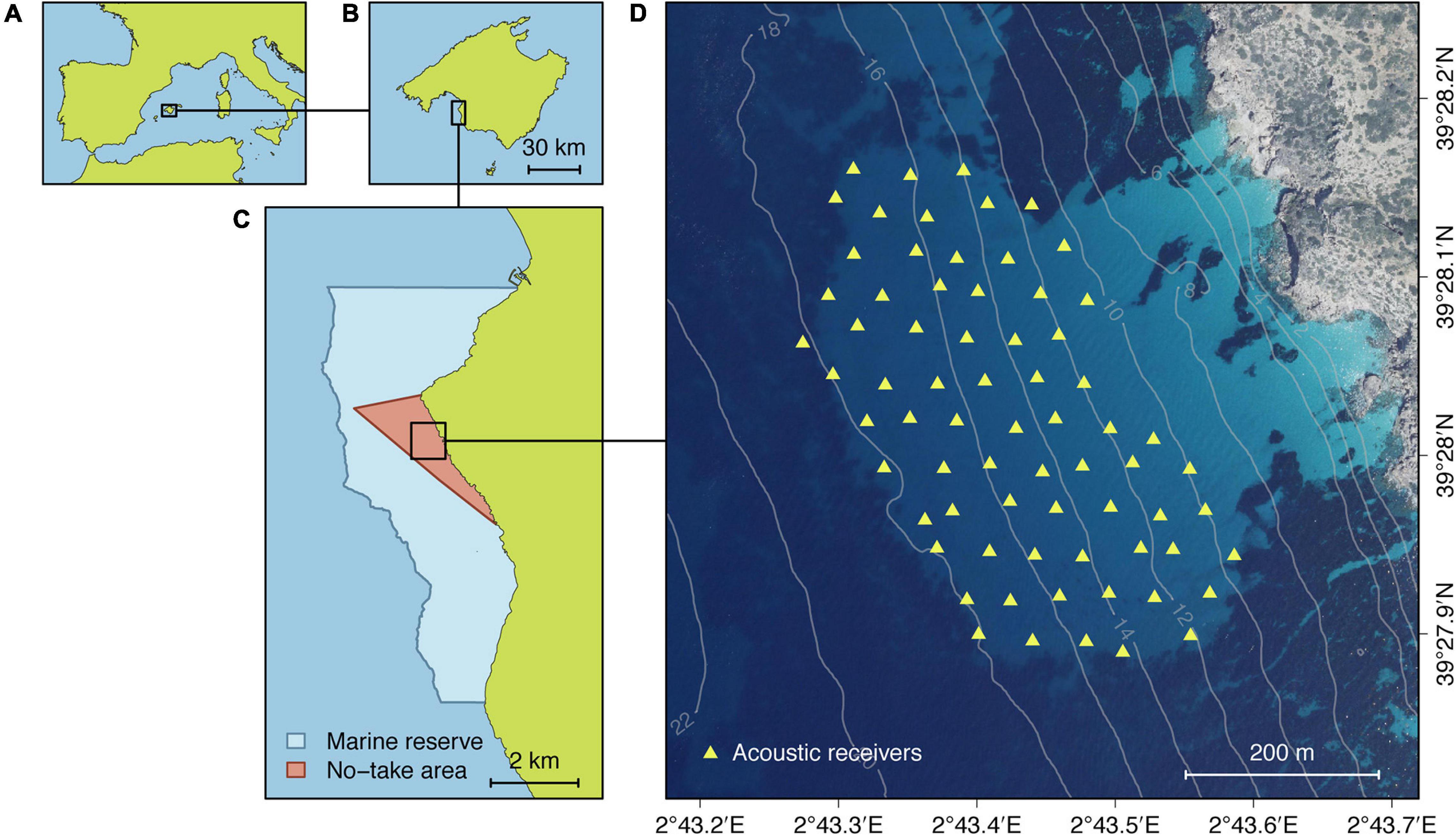
Figure 1. Location of the study site within the Bay of Palma Marine Reserve (Mallorca, Balearic Islands, Spain, A–C) and the acoustic telemetry array (WHS-4250L receivers) used to monitor the movements of pearly razorfish individuals (D). Light areas in panel D correspond to sandy bottoms and dark areas to Posidonia oceanica seagrass meadows.
Fish Sampling and Tagging
Pearly razorfish individuals were captured within the study area from a boat using hook-and-line gear and live shrimps as bait. Individuals were anesthetized by submersion in a 0.1 g⋅–1 solution of tricaine methanesulfonate (MS-222). A small incision was made in the ventral area to implant the L-AMT transmitter in the peritoneal cavity. The used transmitter model depended on the size of the tagged individual, with larger individuals being tagged with larger transmitters (weight-range: 0.32–3.5 g; emission period: 2, 5, and 10 s; battery life: 75–218 days). The incision was immediately closed with a non-absorbable suture and the fish was moved to a tank filled with clean seawater until complete recovery of normal behavior was observed. Finally, individuals were released at the same capture location. The total length (size) of individuals was measured with an accuracy of 1 mm and sex was visually determined based on the sexual dimorphism of the species. A total of 320 individuals (125 males and 195 females) were tagged on 14 different days in April (n = 49), May (n = 242), June (n = 19), and July 2019 (n = 10). The size ranges were 16.3–22.3 cm for males and 9.6–18.8 cm for females. The tagging protocol followed the guidelines provided by the Spanish Government (RD 53/2013) and was approved by the Committee on the Ethics of Animal Experimentation of the University of the Balearic Islands (Ref. CEEA 107/01/19). The Department of Environment, Agriculture and Fisheries of the Government of the Balearic Islands granted permission for fishing, operating, and releasing the animals in the Bay of Palma Marine Reserve.
Data Processing
Detection data from receivers was downloaded to the computer using the WHS Host software (Lotek Wireless Inc.). The positions of transmitters were estimated from the signals that were simultaneously detected by three or more receivers applying the hyperbolic multilateration algorithm implemented in the UMAP software (Lotek Wireless Inc.). All the estimated positions were then imported to the R computing environment (R Core Team, 2020), where all the data pre-processing and analyses were conducted.
The estimated raw positions were pre-processed using the same methodology proposed by Aspillaga et al. (2021). First, twin detections (i.e., positions with duplicated timestamps generated when the positioning algorithm converged to multiple solutions) and positions with a dilution of precision value (i.e., a positioning quality indicator provided by the UMAP software, Niezgoda et al., 2002) larger than 1 were removed from the dataset. Then, a trajectory filter and a continuous-time correlated random walk movement model (CTCRWMM, Johnson et al., 2008) were applied to remove system-induced outliers and generate regular trajectories at 1-min intervals. The trajectory filter removed positions generating unrealistic movements (Freitas et al., 2008), defined by turning angles of <15∘, step lengths of >15 m, or speeds of >2 m⋅s–1. The CTCRWMM was fitted using the crawl package for R (Johnson and London, 2018), assuming a positioning error of 3.3 m (similar to conventional terrestrial GPS devices), value that was extracted from the precision of positioning tests conducted in the same acoustic receiver array (Aspillaga et al., 2021).
To ensure the best representation of our razorfish population case study, days with at least 100 individual trajectories were subsampled from the complete dataset. From the resulting dataset, only data from individuals with a tracking period larger than 7 days were included in the SNA. The final dataset consisted of 232 individuals (79 males, size range: 16.3–22 cm; 153 females, size range: 9.6–17.3 cm) and 55 tracking days, taking place from May to July 2019, thus encompassing periods before and after the beginning of the spawning season. The applied subsampling removed data from the beginning and the end of the experiment, where fewer individuals were tracked each day, and from individuals for which few positions were obtained due to transmitter failures or post-tagging mortality.
Social Network Analysis
Associations between individuals were quantified in 5-min intervals based on the proximity between paired trajectories. During each interval, a positive interaction was considered when the minimum distance between two trajectories (calculated with the gDistance function from the rgeos package for R, Bivand and Rundel, 2020) was smaller than 1 m (i.e., we considered that two individuals interacted when they were at less than 1 m away from each other). This short distance was selected to take advantage of the high-resolution nature of the data since a sub-meter precision was reported for the trajectories obtained with this system after applying the filtering algorithm and the CTCRWMM (Aspillaga et al., 2021). Association weights were calculated as the ratio between the number of 5-min intervals in which an interaction was detected and the total number of intervals in which both individuals were observed. Given the highly resident nature of the pearly razorfish and the large amount of available data, unique associations within each day were considered spurious and therefore removed from the dataset. A general undirected network was generated from the adjacency matrix calculated for the entire tracking period. Moreover, daily networks were also generated from the adjacency matrices that were calculated for each separate day. The networks were created, analyzed, and visualized using the igraph package for R (Csardi and Nepusz, 2006).
To quantify and compare the rate and the intensity of the associations between individuals of the same and different sex, three sub-networks were generated dividing the general one depending on the sex of the interacting individuals: interactions between females (FF), interaction between males (MM), and interactions between males and females (MF). From the resulting subnetworks, edge-level (network-level) and node-level (individual-level) parameters were calculated. At the edge-level, the number and the mean weight of the observed associations were calculated. At the node-level, the binary degree (i.e., number of associations of an individual) and weighted degree (i.e., the sum of the weights of an individual’s associations) were calculated.
The significance of the edge- and node-level parameters was quantified by comparing them against random expectations calculated from null social network models. Null models were generated using a pre-network randomization approach, using permutations of the raw positioning data to estimate the association patterns that would be expected if individuals were randomly distributed through the study area. This type of pre-network randomizations has been found to be more robust than the more commonly used node-level permutations, and it has been shown that can reduce both type I and type II error rates (Farine, 2017; Puga-Gonzalez et al., 2021). In our case, each permutation consisted of shuffling the trajectory of each individual by changing its center (mean x and y coordinates) to a new location, which was randomly sampled within the 95% minimum convex polygon extracted from the centers of all the individual trajectories. In this way, the main characteristics of individual trajectories were maintained within each day, but the possible correlation between the space-use of the different individuals was broken. Then, general and daily networks were constructed using the same methodology as with the observed trajectories and the same parameters were calculated for each sex-specific sub-network (MM, FF, and MF). Finally, the significance (p-value) of each parameter was estimated by calculating the proportion of random iterations in which the mean value was larger or smaller (depending on the direction of the comparison) than the observed value. We used a threshold of p < 0.05 to consider that a parameter was significantly different from the random expectation.
Results
Data Summary
The analyzed dataset contained 7,930, 1-day long trajectories corresponding to a total of 232 razorfish individuals (153 females and 79 males) distributed throughout 55 tracking days (from May 11 to July 11, 2019), with an average of 144 ± 25 individuals (mean ± SD) tracked per day (82 ± 20 females and 62 ± 8 males). A total of 2.44⋅108 detections were obtained by the acoustic receiver array, from which 3.05⋅107 raw positions were estimated using hyperbolic multilateration. The total number of positions was expanded to 7.45⋅107 using the CTCRWMM. Trajectories had an average duration of 13.1 ± 2 h per day (12.7 ± 2.1 h for females and 13.6 ± 1.8 h for males), coinciding with the sunlight hours during the study period.
General Network
From the entire tracking period, a total of 960 dyads (i.e., associations between individuals) were identified (Figure 2), from which 52% corresponded to associations between females (FF), 14% to associations between males (MM), and 34% to associations between individuals of different sex (MF) (Figure 3A). Overall, associations presented low edge weight values; 29% of the dyads (n = 279) had weights below 1⋅10–3, representing sporadic encounters between individuals. The highest edge weight values were observed in MF associations, with a median and 95% inter-quantile range of 1.6⋅10–3 [3⋅10–4–2.6⋅10–1], followed by the edge weights of FF (3.2⋅10–3 [4⋅10–4–4.2⋅10–2]) and MM (1⋅10–3 [2⋅10–4–1.9⋅10–2]) associations (Figure 3B). Only 8% of individuals (n = 19, 15 females and 4 males) did not present any association. When compared against null models, the number of edges of all the sex-specific sub-networks were found to be significantly higher than the values expected if individuals were randomly distributed (p < 0.001) (Figure 4). Regarding the strength of the associations, we did not find significant differences from random networks in the mean weight of FF associations (p = 0.085) (Figure 4). By contrast, the average weight of MM and MF associations were significantly smaller and larger, respectively, than the average weights obtained from random networks (p < 0.001), indicating an active avoidance of males toward other males and an association preference between males and females.
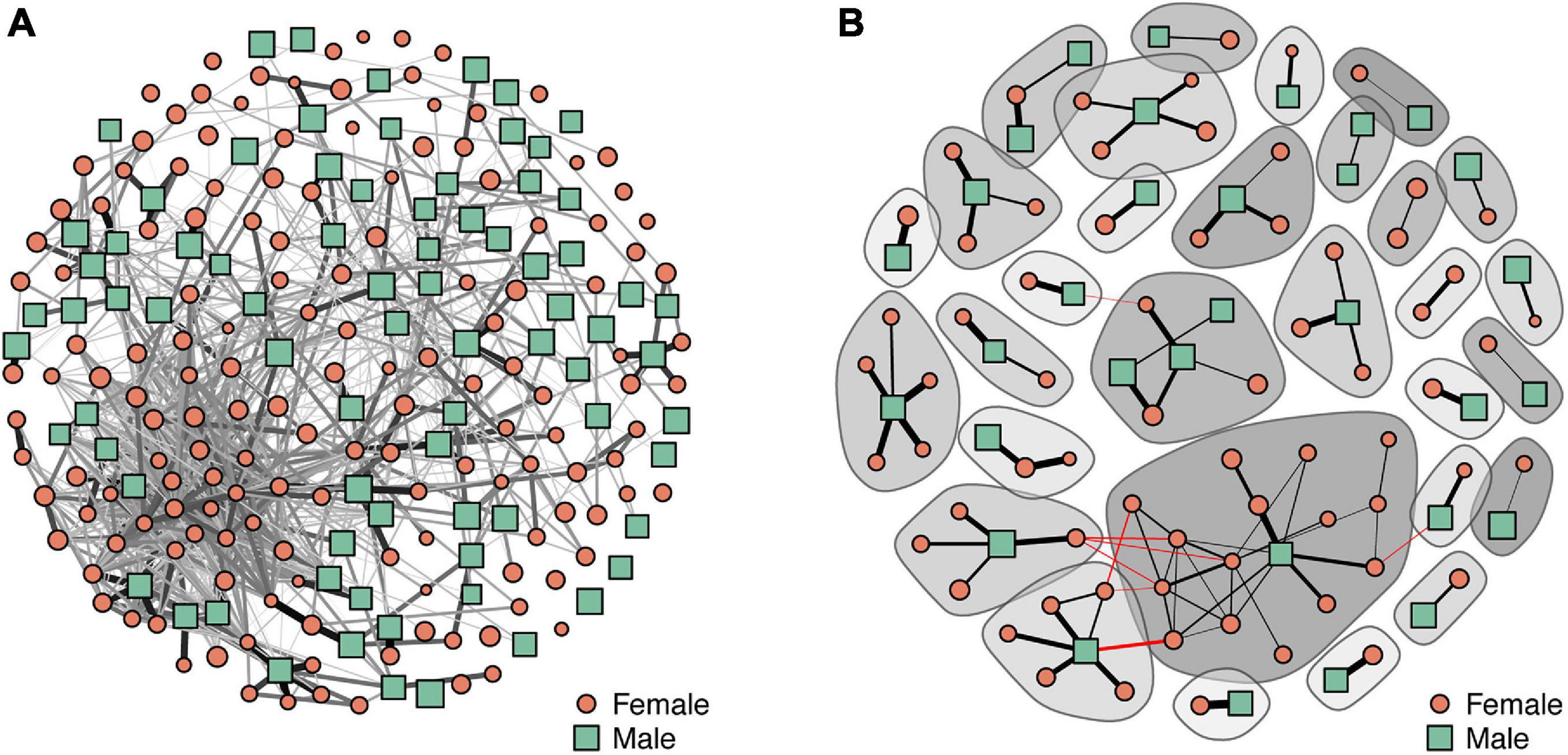
Figure 2. Social network of the pearly razorfish population for the entire tracking period. (A) Entire network for all the 232 razorfish individuals (960 edges). (B) A subset of the entire network showing the strongest 10% edges (edge weight > 0.03, 90 edges, 101 individuals). Node size is proportional to the body size of each individual. Darker and thicker edges represent higher edge weights. The shadowed contours in (B) indicate the different groups identified by the fast greedy modularity optimization algorithm for finding community structures (Clauset et al., 2004) and red edges associations between individuals classified in different groups.
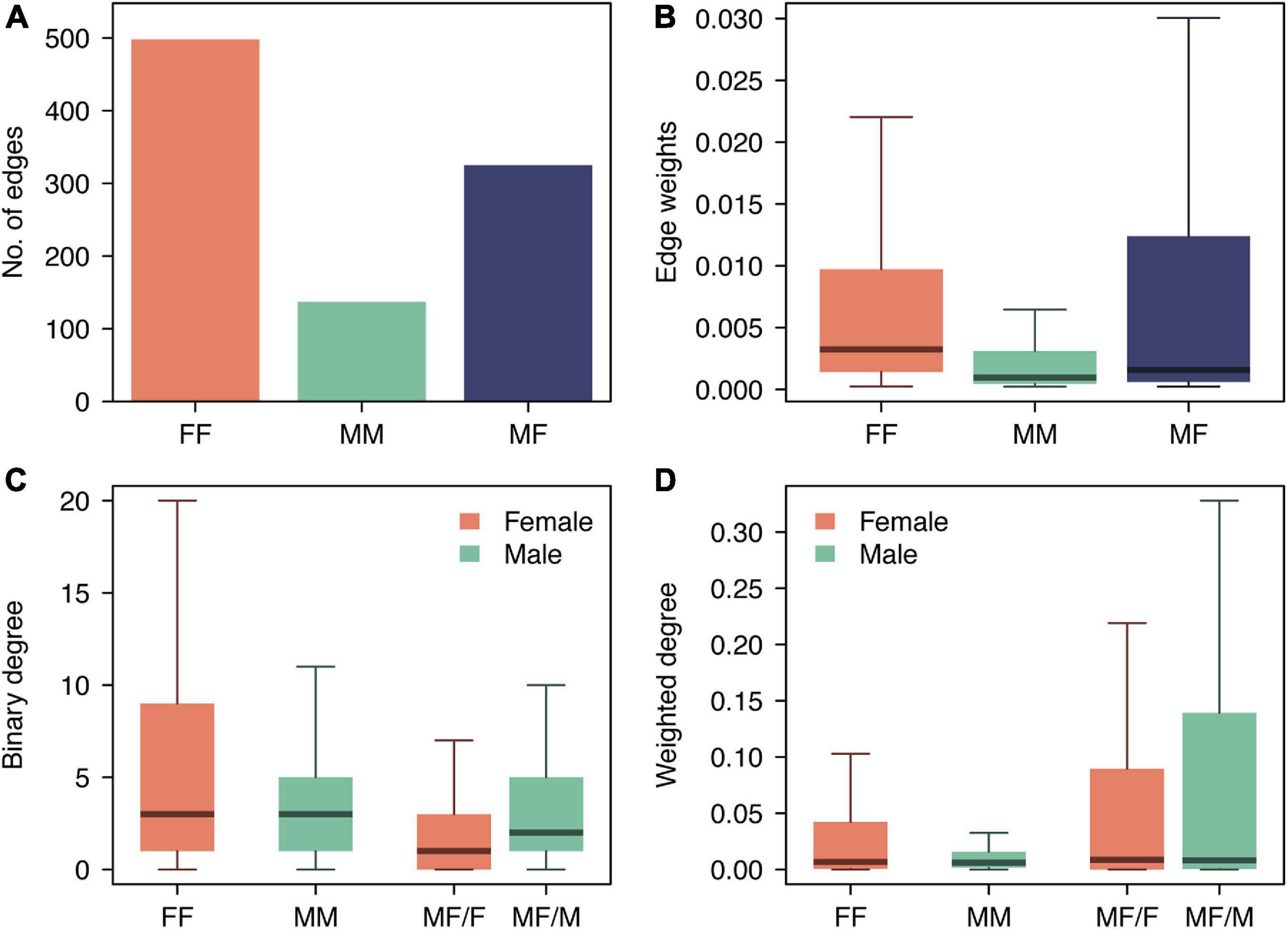
Figure 3. Comparison of edge-level (A,B) and node-level (C,D) network parameters among the sex-specific social networks of the pearly razorfish population. (A) the number of edges and (B) edge weight values in each subnetwork; (C) binary degree and (D) weighted degree or strength of individuals from different sex within each subnetwork (FF: female-female associations; MM: male-male associations; MF: male-female associations; MF/F: females in male-female associations; MF/M: males in male-female associations). Boxplots indicate the median and the interquartile range and whiskers the minimum and maximum values at 1.5 times the interquantile distance.
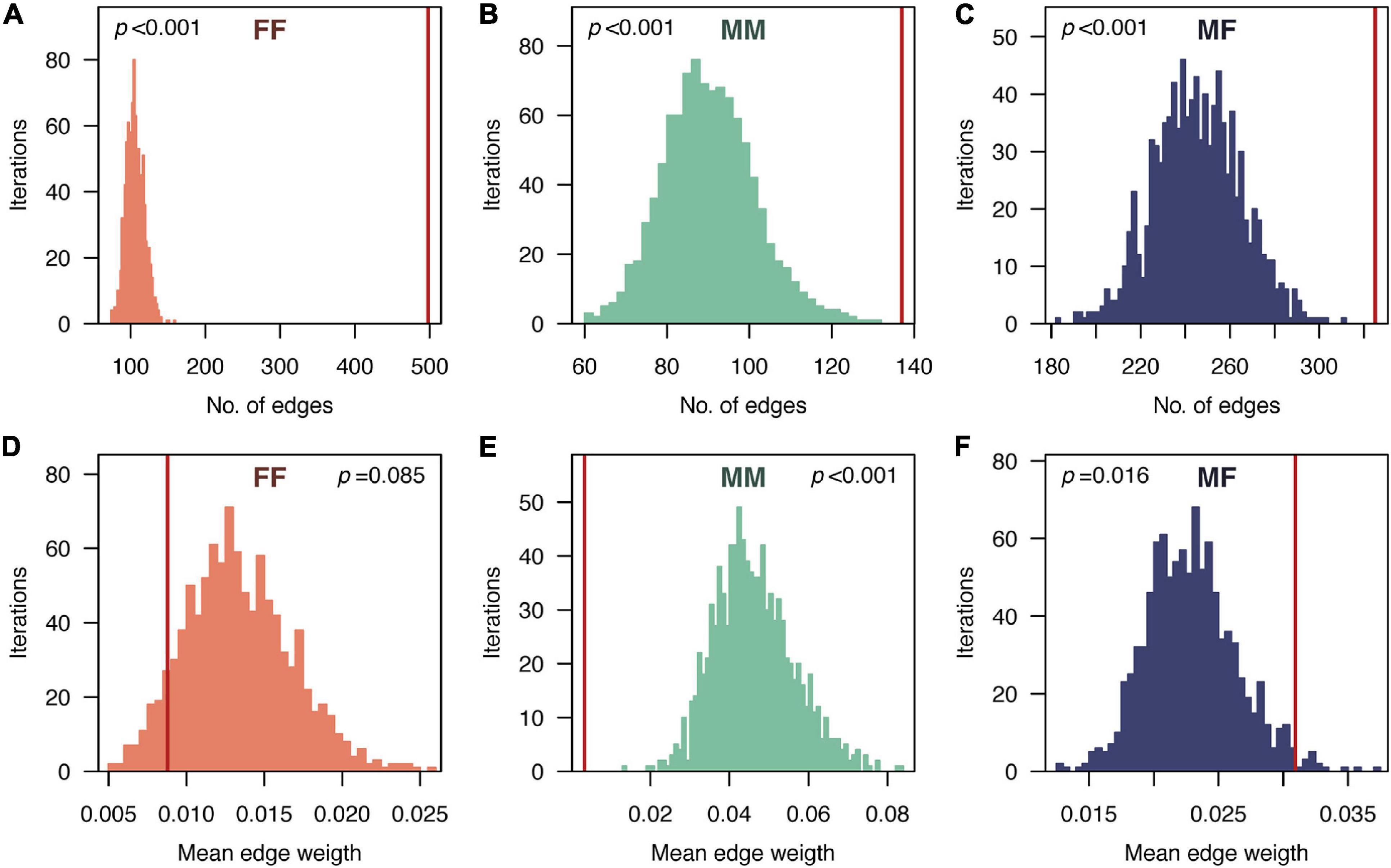
Figure 4. Comparison of edge-level social network parameters (number of edges, A–C and mean edge weights, D–F) observed in the sex-specific sub-networks of the pearly razorfish population and the expected values obtained from random networks (FF: female-female; MM: male-male; MF: male-female). Histograms show the distribution of the random expectations obtained from 1,000 random networks. The vertical red lines represent the empirically observed values. p-values were calculated as the proportion of randomized values that were higher or lower (depending on the direction of the comparison) than the observed value.
At the node (i.e., individual) level, females showed higher binary degree values when associating with other females, with a median and 95% interquantile range of 3 [0–35.4], than when associating with males (MF/F), presenting a median value of 1 [0–9.2] (Figure 3C). The weighted degree of both types of female associations presented similar values (FF associations: 8.6⋅10–3 [0–0.35]; MF/F associations: 6.7⋅10–3[0–0.45]) (Figure 3D). Each male interacted with a similar number of males (3 [1–10]) and females (2 [0–21]) (Figure 3C), but the strength of these interactions presented clear differences: the weighted degree of males interacting with other males (6.1⋅10–3 [0–0.06]) was much smaller than the weighted degree of males interacting with females (0.08 [0–0.81]) (Figure 3D). When compared against random expectations, the mean binary degree values in all the sex-specific networks were larger than the expected (p < 0.001) (Figure 5). The observed mean weighted degree was also significantly larger than the random expectations in all cases (p < 0.001), except for MM associations, in which the mean weighted degree was significantly smaller than the expected (p < 0.001).
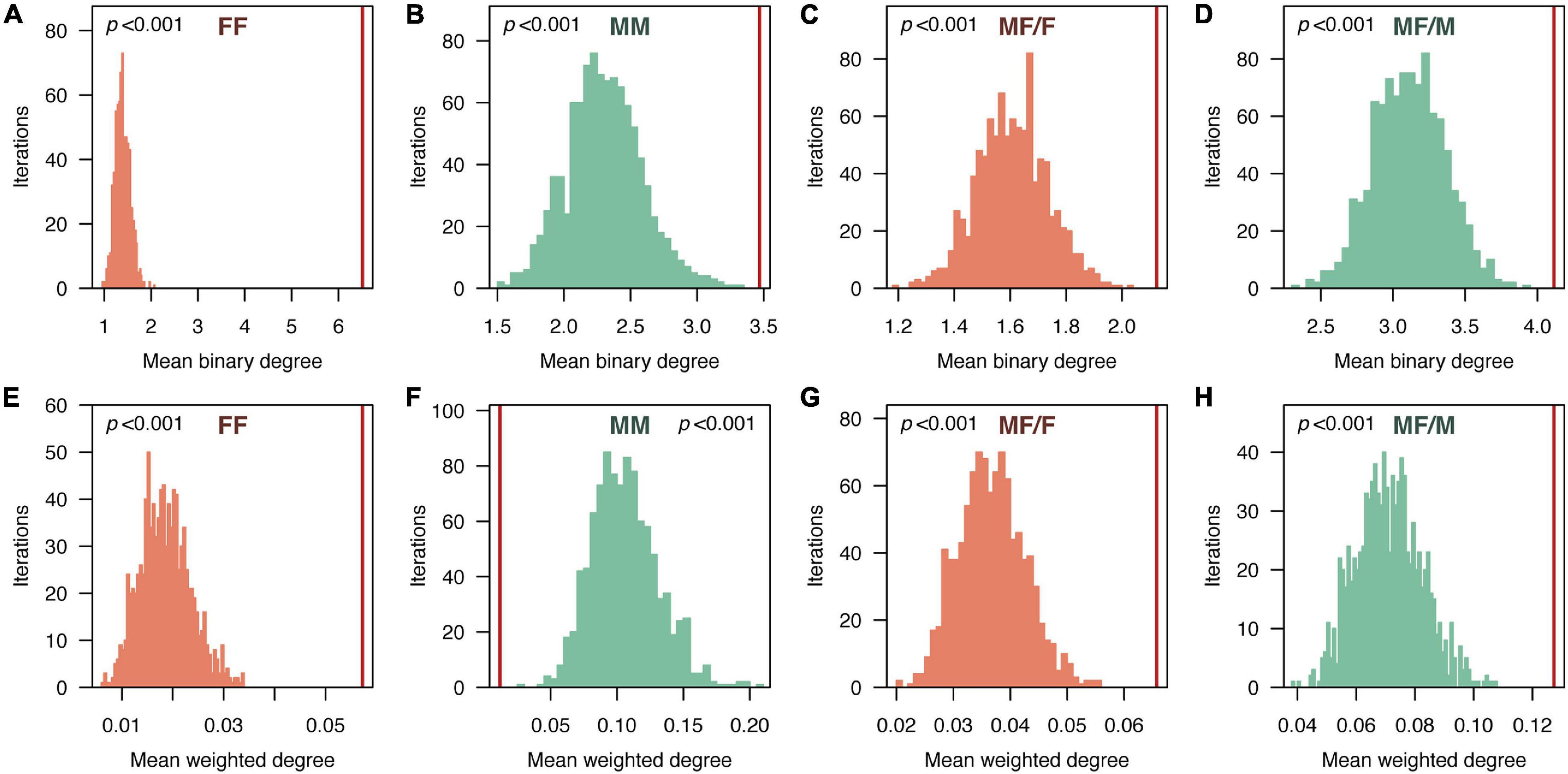
Figure 5. Comparison of node-level social network parameters (A–D: mean binary degree; E–H: mean weighed degree) observed in the sex-specific sub-networks of the pearly razorfish population and the expected values obtained from random networks (FF: female-female associations; MM: male-male associations; MF/F: females in male-female associations; MF/M: males in male-female associations). Histograms show the distribution of the random expectations obtained from 1,000 random networks. The vertical red lines represent the empirically observed values. p-values were calculated as the proportion of randomized values that were higher or lower (depending on the direction of the comparison) than the observed value.
Temporal Variability
Analyzing the associations of individuals on a daily basis provided a more detailed view of the patterns observed in the general network for the entire tracking period. Considerable differences were observed in some of the sex-specific sub-networks across the tracking period (Figure 6). For instance, the significantly higher binary and weighted degree values that could be observed in females within FF networks could not be found on all the days; 58.2% of the days (n = 32) did not present any significant departure from random expectations in terms of binary degree and 72.2% of the days (n = 40) in terms of weighted degree (Figures 6A,B). However, the daily association patterns between females indicated extreme departures from the random expectations in several days between May 27 and June 4, indicating sporadic but strong associations of groups of females on specific days, also observable in the whole social architecture of the population (Figure 2). Despite the binary degree of males interacting with other males being elevated in the general network, significantly lower values (65.5% of the days, n = 36) or no differences (34.5% of the days, n = 19) were observed in the daily networks (Figure 6C). By contrast, the weighted degree coincided with the observations in the general network, being significantly lower than the random expectations on every day in the tracking period (Figure 6D). Female and male associations in MF networks presented the same daily pattern, with significantly higher binary degrees on 59.9% of the days (n = 28) and no differences on 49.1% of the days (n = 28), while the weighted degree of interactions was higher on every single day (Figures 6E–H).
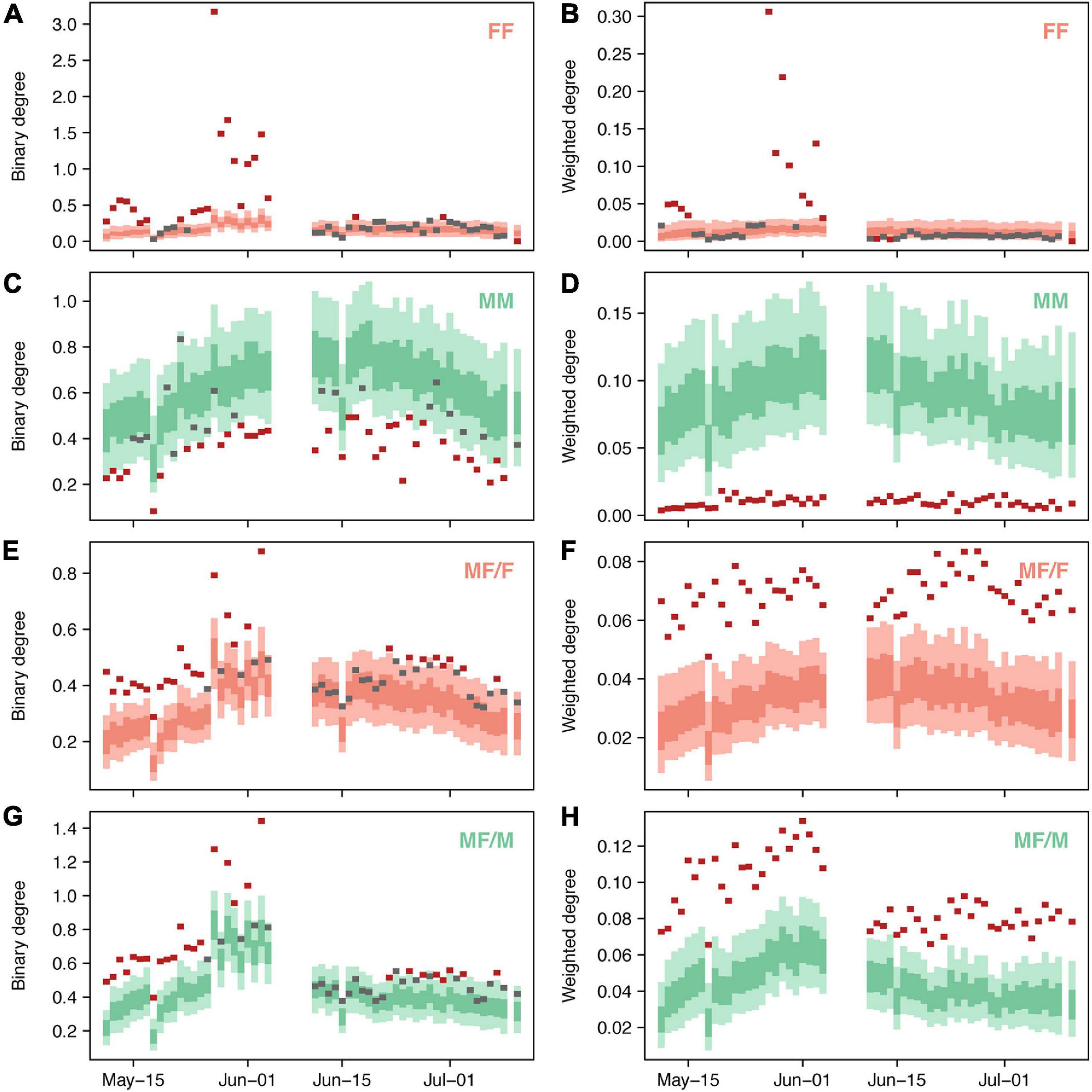
Figure 6. Temporal variation of node-level parameters (A, C, E, G: mean binary degree; B, D, F, H: mean weighted degree) in sex-specific networks (FF: female-female associations; MM: male-male associations; MF/F: females in male-female associations; MF/M: males in male-female associations). The dark and light-colored bars represent the 50% and 95% inter-quantile ranges, respectively, of the average parameters obtained from random networks. Superposed segments indicate the empirically observed average parameters for each day: in red, the ones representing significant deviations from random expectations (p < 0.05), and in gray, the ones lacking of significant differences (p > 0.05).
Discussion
Proximity networks are able to reproduce the complexity of social interactions that occur within animal populations and communities, improving our understanding of the function and resilience of ecosystems (Croft et al., 2008). With the proliferation of tracking and bio-logging devices, proximity metrics have gained popularity within the SNA framework (Webber and Vander Wal, 2019), despite that some discussion exists regarding the validity of proximity-based association indices as a proxy for directly measured interaction indices (Castles et al., 2014; Farine, 2015). The quantification of association indices based on direct encounters of individuals (measured here as < 1 m proximity dyads) has been neglected in aquatic systems due to the limitations that aquatic environments pose to the continuous positioning of large numbers of individuals (e.g., GPS does not work underwater). Here, we demonstrate the usefulness of HRATS and SNA to study the association patterns in wild fish populations. Our method, using an approach that can be extended to other marine species, has produced the most detailed view of a marine fish society in a species that, due to its morphology and habitat, is hard to observe directly (but some works have been done underwater, e.g., Marconato et al., 1995; Shen and Clark, 2016). With the HRATS, we were able to monitor an average of 144 individuals per day (with a total of 232 monitored individuals included in the analysis), obtain high-quality spatial data from where to infer the associations between individuals, and conduct a SNA at the population level. Our study demonstrates the high potential of combining HRATS and SNA to unveil the architectural patterns of resident marine species, opening the possibility of testing a wide range of ecological hypotheses on the effect of individual traits and ecological and environmental parameters on the social structures occurring in the wild.
By combining HRATS and SNA, our findings suggest that the pearly razorfish displays a harem-like territorial behavior, where males defend territories that enclose the home range of several females. The observed low strength of the associations between males, which was significantly lower than the strength expected if individuals were randomly distributed, demonstrates that males strongly avoid individuals of the same sex. However, the number of associations between males, regardless of their strength, was significantly larger than expected, indicating sporadic contacts between males with neighboring territories. Our results agree with previous descriptions of this species’ social organization based on repeated scuba diving observations on a limited number of individuals (Shen and Clark, 2016). These authors described that males divide the area into adjacent circular territories that do not overlap. They also documented occasional confrontations between males at the border of adjacent territories and against males that intruded neighboring territories, which might explain the obtained large numbers of associations with small strengths. The spatial avoidance behavior was not observed among females. Despite that the general network indicated a high number of associations with high strength between females, this pattern was not observed in most daily networks, where the number of associations and their strength did not significantly differ from the null models. The associations between males and females were, in every case, more frequent and stronger than random expectations, indicating strong and constant ties between individuals of different sex. When looking at the number of associations in MF sub-networks, we could observe that, on average, females were most often associated with only one male, while males were typically associated with two or more females, indicating the typical harem-like structure in which several females co-occur within the territory of a male. Marconato et al. (1995) and Shen and Clark (2016) described the harem-like social structure of this species, where males were observed encompassing the smaller sub-territories of two to six females. The male:female proportion of individuals included in the study (1:1.93) was similar to the ratio described for this species using underwater visual censuses (1:85, Espino et al., 2015), but higher than the ratio obtained by the same authors using trawls (1:4.9). This suggests that males could be overrepresented in our dataset, probably because they are more susceptible to be fished due to their territorial behavior. However, a thorough quantification of the population size would be required to know the exact representativeness of our study in terms of the proportion of the monitored population. Similar harem-like structures have been widely observed in marine species like parrotfishes (Mumby and Wabnitz, 2002), groupers (Zabala et al., 1997), and clownfishes (Warner, 2011). Thus, our work provides a novel method to delve into the study of the mechanisms and consequences of this social space-use behavior.
Our results also demonstrated punctual changes in the associating behavior of females. During a few days in May and June, groups of females tended to associate with each other more than during the rest of the days. In these events, high numbers of associations and high association strengths were observed between females, with groups of up to 20 females appearing strongly associated with each other. From a direct visualization of the trajectories of the females, extensive collective-like excursions were identified along the study area. Similar punctual aggregations have been found in marine species related to reproduction (e.g., Sadovy de Mitcheson and Colin, 2012; Aspillaga et al., 2016). However, the hypothesis of a spawning-related behavior is unlikely because the period in which this phenomenon was observed (May–June) was before the spawning season (July–September, Cardinale et al., 1998) and because of the lack of participation of male individuals. Indeed, we did not observe any changes in network structure patterns related to the start of the breeding season, which confirms that the establishment of harems is permanent and observable outside the spawning period (Cardinale et al., 1998). Nevertheless, the punctual collective-like movements could be representing mate choice exploratory trips pursued by females to assess quality among males in the area before settling for mating. Another possible hypothesis would be a commensalism behavior of females toward other species, such as the different ray species that also co-occur in the area during that period (pers. obs.), which males would not display due to their necessity of protecting their territories. Similar commensal relationships were also observed in the pearly razorfish and other razorfish species by Shen and Clark (2016), where groups of up to 15 individuals were attracted by the feeding activity of other sand-dwellers such as goatfishes and snake eels. However, the authors did not report any sexual specificity of such behaviors, their duration, or spatial extent. In all cases, the causes, mechanisms, and consequences of these extraordinary aggregation behavioral events in females of a territorial species deserve further attention.
Our approach combining HRATS and SNA brings out three major steps toward improving our understanding of the functioning of marine fish societies: it is based on high-resolution spatial data, considers a large number of monitored individuals, and provides raw data from which properly design null models for hypothesis testing. Regarding the ability of our system to produce high-resolution positional data, we were able to infer association patterns from extremely low spatial proximity values (<1 m). The JSATS used in this study makes it possible to track individuals with a spatial resolution of less than 1 m and a temporal resolution of a few seconds (Aspillaga et al., 2021). This is a great improvement over the conventional presence-absence acoustic tracking data, which due to its large positional uncertainty (200–800 m), may mask the real structure of the studied association networks. Instead, HRATS are able to produce high-throughput tracking data at high accuracy and resolution using hyperbolic multilateration algorithms. These systems, also known as reverse-GPS, allows transmitters to be simple and small, as most of the energy is located at the receivers, and represent one of the most promising tracking technologies to deepening insights into the most fundamental behavioral mechanisms of animals, like the allocentric representation of space (Toledo et al., 2020). Therefore, we believe that proximity metrics generated by HRATS will produce a revolution in our understanding of marine fish populations, at least for resident, home range-forming species.
A second major advance of using HRATS for SNA is that it opens the possibility of tracking a large fraction of a marine fish population simultaneously. Transmitters using the binary phase-shift keying (BPSK) coding systems allow for using thousands of transmitters emitting unique IDs on a single acoustic frequency (416 kHz in this study). This represents a significant advantage from the conventional tracking systems using the pulse position modulation (PPM) coding system, which limited the detectability of individuals co-occurring in the same area due to the effect of signal collisions. The number of monitored individuals is a crucial parameter to obtain a representative view of the social behavior of the population (Whitehead, 2008). Based on a simulation study, Silk et al. (2015) described that the social position of individuals measured in partial social networks (where some individuals were not identified) was strongly correlated with their position in the full social network, but this correlation became stronger as the proportion of identified individuals increased. In our case, we were able to track an unprecedented number of pearly razorfish individuals (n = 232) in a relatively small area (12.5 ha). In addition, the miniaturization of transmitters (the smallest L-AMT series transmitters weights 0.22 g) on HRATS allows tracking almost the entire range of fish sizes. Conventional tracking has always been limited to the large and adult fraction of the population (see discussion in Alós et al., 2011) due to the larger size of the transmitters, which has notably restricted our ability to study the movement of small-bodied individuals and across the ontogeny of marine species. For example, a previous study using a conventional acoustic telemetry system in a nearby area only monitored 12 pearly razorfish individuals with sizes ranging from 15.3 to 20.9 cm (Alós et al., 2012). Thus, HRATS systems emerge as a powerful tool to study the behavior and social interactions of small wild fish populations.
The third relevant point of our approach is that it generates valuable data for hypothesis testing using null models. Null models consist in generating randomized permutations of the data with the patterns that would be expected in the absence of the process of interest (Croft et al., 2011; Farine, 2017), and are especially important in the SNA framework due to the non-independence of the social data. The most commonly used and simple method to generate null models in SNA is the node-permutation, where the identity of the network nodes (i.e., the attributes of the individuals) are shuffled to break the link between the network and the trait of interest (Farine and Whitehead, 2015; Farine, 2017). More recently, conducting permutations on the raw observational data (i.e., pre-network permutations) has been proposed as a more flexible and robust alternative to network permutations because it allows for better control over the hypothesis that is being tested. Conducting pre-network permutations on animal trajectory data presents the difficulty of maintaining the autocorrelation structure of original individual tracks during the data stream randomization. For instance, Spiegel et al. (2016) generated random models of the social structure of a lizard population by randomly switching the date associated to each individual track, keeping the spatiotemporal structure of the trajectories but decoupling the possible synchronization between individuals. The pearly razorfish is a highly territorial species with a small home-range size (Alós et al., 2012; Shen and Clark, 2016). In our study, their movements were restricted to the study area, a sandy patch enclosed by a seagrass meadow. Based on these facts, we applied a pre-network permutation approach by randomly distributing the trajectories of individuals within the study area, keeping the spatial correlation of all the positions from the same individual but breaking any possible spatial pattern arisen from agonistic or affiliative behaviors between individuals. This randomization method allowed us to analyze the overall association structure of the pearly razorfish population, and more specifically, the strength of the associations between individuals of the same and different sex.
Conclusion
HRATS are a powerful tool to study the movements of resident fish populations and, in combination with SNA tools, can provide a complete description of their social structure. In the case of the pearly razorfish, this combination generated a unique dataset composed of more than 7,900 high-resolution daily trajectories from 232 individuals, providing the most detailed view of a marine population to date. The SNA suggested a harem-like social structure, with agonistic interactions between males and larger association indices between individuals of different sex. In addition, we were able to detect associations of large groups of females conducting synchronous movements along the study area that were not previously described in this species. The combination of HRATS and SNA opens a wide range of research questions that can be addressed for the first time with elusive or small-bodied species that would be difficult to study otherwise, such as the effect of the genetic and personality traits on the position of the individual in the network, and the effect of environmental or anthropic impacts (e.g., professional and recreational fisheries) in the overall structure of the network.
Data Availability Statement
The raw data supporting the conclusions of this article will be made available by the authors, without undue reservation.
Ethics Statement
The animal study was reviewed and approved by the Committee on the Ethics of Animal Experimentation of the University of the Balearic Islands (Ref. CEEA 107/01/19).
Author Contributions
EA and JA conceived the original study. RA provided the telemetry material. EA, JA, MM-B, and MB-S conducted the telemetry experiments. EA and JA led the data analysis and wrote the first manuscript. All authors made significant comments to the manuscript, and read and approved the final manuscript.
Funding
The telemetry system was financed by the German Federal Ministry of Education and Research (Grant No. #033W024A). EA was supported by a Margalida Comas Postdoctoral Grant from the Government of the Balearic Islands and the European Social Fund (Grant No. PD/023/2018). JA was supported by a Ramon y Cajal Grant (Grant No. RYC2018-024488-I) and received funding from the CLOCKS R&D Project (Grant No. PID2019-104940GA-I00) and the Intramural Research Project JSATS (Grant No. PIE 202030E002) funded by the Spanish Ministry of Science and Innovation and the Spanish National Research Council. The publication fee was supported by the CSIC Open Access Publication Support Initiative through its Unit of Information Resources for Research (URICI).
Conflict of Interest
The authors declare that the research was conducted in the absence of any commercial or financial relationships that could be construed as a potential conflict of interest.
Acknowledgments
We would like to thank David Gambin and the staff from Lotek Wireless Inc., for their technical assistance during this study. Special thanks to Sebastià Cabanellas, Guillermo Follana, Arancha Lana, and Christopher Monk for their support during the fieldwork. We are grateful to the Direcció General de Pesca i Medi Marí del Govern de las Illes Balears for the support and authorization of this work.
References
Alós, J., Cabanellas-Reboredo, M., and Lowerre-Barbieri, S. (2012). Diel behaviour and habitat utilisation by the pearly razorfish during the spawning season. Mar. Ecol. Prog. Ser. 460, 207–220. doi: 10.3354/meps09755
Alós, J., March, D., Palmer, M., Grau, A., and Morales-Nin, B. (2011). Spatial and temporal patterns in Serranus cabrilla habitat use in the NW Mediterranean revealed by acoustic telemetry. Mar. Ecol. Prog. Ser. 427, 173–186. doi: 10.3354/meps09042
Alós, J., Palmer, M., Rosselló, R., and Arlinghaus, R. (2016). Fast and behavior-selective exploitation of a marine fish targeted by anglers. Sci. Rep. 6:38093. doi: 10.1038/srep38093
Aspillaga, E., Arlinghaus, R., Martorell-Barceló, M., Follana-Berná, G., Lana, A., Campos-Candela, A., et al. (2021). Performance of a novel system for high-resolution tracking of marine fish societies. Anim. Biotelem. 9:1. doi: 10.1186/s40317-020-00224-w
Aspillaga, E., Bartumeus, F., Linares, C., Starr, R. M., López-Sanz, À, Díaz, D., et al. (2016). Ordinary and extraordinary movement behaviour of small resident fish within a mediterranean marine protected area. PLoS One 11:e0159813. doi: 10.1371/journal.pone.0159813
Baktoft, H., Zajicek, P., Klefoth, T., Svendsen, J. C., Jacobsen, L., Pedersen, M. W., et al. (2015). Performance assessment of two whole-lake acoustic positional telemetry systems - is reality mining of free-ranging aquatic animals technologically possible? PLoS One 10:e0126534. doi: 10.1371/journal.pone.0126534
Binder, T. R., Holbrook, C. M., Hayden, T. A., and Krueger, C. C. (2016). Spatial and temporal variation in positioning probability of acoustic telemetry arrays: fine-scale variability and complex interactions. Anim. Biotelem. 4:4. doi: 10.1186/s40317-016-0097-4
Bivand, R., and Rundel, C. (2020). Rgeos: Interface to Geometry Engine - Open Source (‘GEOS’). R package version 0.5-5. Available online at: https://cran.r-project.org/package=rgeos (accessed January 27, 2021).
Butcher, P. A., Colefax, A. P., Gorkin, R. A., Kajiura, S. M., López, N. A., Mourier, J., et al. (2021). The drone revolution of shark science: a review. Drones 5:8. doi: 10.3390/drones5010008
Cantor, M., Wedekin, L. L., Guimarães, P. R., Daura-Jorge, F. G., Rossi-Santos, M. R., and Simões-Lopes, P. C. (2012). Disentangling social networks from spatiotemporal dynamics: the temporal structure of a dolphin society. Anim. Behav. 84, 641–651. doi: 10.1016/j.anbehav.2012.06.019
Cardinale, M., Colloca, F., and Ardizzone, G. D. (1998). Growth and reproduction of Xyrichthys novacula (Pisces: Labridae) in the Mediterranean Sea. Sci. Mar. 62, 193–201. doi: 10.3989/scimar.1998.62n3193
Castles, M., Heinsohn, R., Marshall, H. H., Lee, A. E. G., Cowlishaw, G., and Carter, A. J. (2014). Social networks created with different techniques are not comparable. Anim. Behav. 96, 59–67. doi: 10.1016/j.anbehav.2014.07.023
Clauset, A., Newman, M. E. J., and Moore, C. (2004). Finding community structure in very large networks. Phys. Rev. E Stat. Nonlin. Soft. Matter Phys. 70:066111. doi: 10.1103/PhysRevE.70.066111
Connor, R. C., Heithaus, M. R., and Barre, L. M. (2001). Complex social structure, alliance stability and mating access in a bottlenose dolphin “super-alliance.”. Proc. R. Soc. B Biol. Sci. 268, 263–267. doi: 10.1098/rspb.2000.1357
Couzin, I. D., and Krause, J. (2003). Self-organization and collective behavior in vertebrates. Adv. Study Behav. 32, 1–75. doi: 10.1016/S0065-3454(03)01001-5
Croft, D. P., James, R., and Krause, J. (2008). Exploring Animal Social Networks. Princeton: Princeton University Press.
Croft, D. P., Madden, J. R., Franks, D. W., and James, R. (2011). Hypothesis testing in animal social networks. Trends Ecol. Evol. 26, 502–507. doi: 10.1016/j.tree.2011.05.012
Csardi, G., and Nepusz, T. (2006). The igraph software package for complex network research. InterJournal Complex Syst. 1695, 1–9. Available online at: https://igraph.org (accessed January 27, 2021).
Davis, G. H., Crofoot, M. C., and Farine, D. R. (2018). Estimating the robustness and uncertainty of animal social networks using different observational methods. Anim. Behav. 141, 29–44. doi: 10.1016/j.anbehav.2018.04.012
Espino, F., Triay-Portella, R., González, J. A., Haroun, R., and Tuya, F. (2015). Population structure of the pearly razorfish, Xyrichtys novacula (Actinopterygii: Labridae), in sand-seagrass mosaics: spatial variation according to habitat features and sampling techniques. Sci. Mar. 79, 179–188. doi: 10.3989/scimar.04219.05a
Farine, D. R. (2015). Proximity as a proxy for interactions: issues of scale in social network analysis. Anim. Behav. 104, e1–e5. doi: 10.1016/j.anbehav.2014.11.019
Farine, D. R. (2017). A guide to null models for animal social network analysis. Methods Ecol. Evol. 8, 1309–1320. doi: 10.1111/2041-210X.12772
Farine, D. R., and Whitehead, H. (2015). Constructing, conducting and interpreting animal social network analysis. J. Anim. Ecol. 84, 1144–1163. doi: 10.1111/1365-2656.12418
Freitas, C., Lydersen, C., Fedak, M. A., and Kovacs, K. M. (2008). A simple new algorithm to filter marine mammal Argos locations. Mar. Mammal Sci. 24, 315–325. doi: 10.1111/j.1748-7692.2007.00180.x
Grueter, C. C., Qi, X., Zinner, D., Bergman, T., Li, M., Xiang, Z., et al. (2020). Multilevel organisation of animal sociality. Trends Ecol. Evol. 35, 834–847. doi: 10.1016/j.tree.2020.05.003
Haulsee, D. E., Fox, D. A., Breece, M. W., Brown, L. M., Kneebone, J., Skomal, G. B., et al. (2016). Social network analysis reveals potential fission-fusion behavior in a shark. Sci. Rep. 6:34087. doi: 10.1038/srep34087
Heupel, M. R., Semmens, J. M., and Hobday, A. J. (2006). Automated acoustic tracking of aquatic animals: scales, design and deployment of listening station arrays. Mar. Freshw. Res. 57, 1–13. doi: 10.1071/MF05091
Hussey, N. E., Kessel, S. T., Aarestrup, K., Cooke, S. J., Cowley, P. D., Fisk, A. T., et al. (2015). Aquatic animal telemetry: a panoramic window into the underwater world. Science 348:1255642. doi: 10.1126/science.1255642
Ings, T. C., Montoya, J. M., Bascompte, J., Blüthgen, N., Brown, L., Dormann, C. F., et al. (2009). Ecological networks - beyond food webs. J. Anim. Ecol. 78, 253–269. doi: 10.1111/j.1365-2656.2008.01460.x
Jacoby, D. M. P. P., and Freeman, R. (2016). Emerging network-based tools in movement ecology. Trends Ecol. Evol. 31, 301–314. doi: 10.1016/j.tree.2016.01.011
Jacoby, D. M. P., Brooks, E. J., Croft, D. P., and Sims, D. W. (2012). Developing a deeper understanding of animal movements and spatial dynamics through novel application of network analyses. Methods Ecol. Evol. 3, 574–583. doi: 10.1111/j.2041-210X.2012.00187.x
Jacoby, D. M. P., Papastamatiou, Y. P., and Freeman, R. (2016). Inferring animal social networks and leadership: applications for passive monitoring arrays. J. R. Soc. Interface 13:20160676. doi: 10.1098/rsif.2016.0676
Johnson, D. S., and London, J. M. (2018). Crawl: An R Package for Fitting Continuous-Time Correlated Random Walk Models to Animal Movement Data. Geneva: Zenodo.
Johnson, D. S., London, J. M., Lea, M.-A., and Durban, J. W. (2008). Continuous-time correlated random walk model for animal telemetry data. Ecology 89, 1208–1215. doi: 10.1890/07-1032.1
Kays, R., Crofoot, M. C., Jetz, W., and Wikelski, M. (2015). Terrestrial animal tracking as an eye on life and planet. Science 348:aaa2478. doi: 10.1126/science.aaa2478
Krause, J., Krause, S., Arlinghaus, R., Psorakis, I., Roberts, S., and Rutz, C. (2013). Reality mining of animal social systems. Trends Ecol. Evol. 28, 541–551. doi: 10.1016/j.tree.2013.06.002
Krause, J., Lusseau, D., and James, R. (2009). Animal social networks: an introduction. Behav. Ecol. Sociobiol. 63, 967–973. doi: 10.1007/s00265-009-0747-0
Leander, J., Klaminder, J., Jonsson, M., Brodin, T., Leonardsson, K., and Hellström, G. (2020). The old and the new: evaluating performance of acoustic telemetry systems in tracking migrating Atlantic salmon (Salmo salar) smolt and European eel (Anguilla anguilla) around hydropower facilities. Can. J. Fish. Aquat. Sci. 77, 177–187. doi: 10.1139/cjfas-2019-0058
Lédée, E. J. I., Heupel, M. R., Tobin, A. J., Knip, D. M., and Simpfendorfer, C. A. (2015). A comparison between traditional kernel-based methods and network analysis: an example from two nearshore shark species. Anim. Behav. 103, 17–28. doi: 10.1016/j.anbehav.2015.01.039
Marconato, A., And, V. T., and Marin, G. (1995). The mating system of Xyrichthys novacula: sperm economy and fertilization success. J. Fish Biol. 47, 292–301. doi: 10.1111/j.1095-8649.1995.tb01896.x
Marin, A., and Wellman, B. (2011). “Social network analysis: an introduction,” in The SAGE Handbook of Social Network Analysis, eds J. Scott and P. J. Carrington (Thousand Oaks, CA: SAGE Publications, Inc), 25.
Martínez-López, B., Perez, A. M., and Sánchez-Vizcaíno, J. M. (2009). Social network analysis. review of general concepts and use in preventive veterinary medicine. Transbound. Emerg. Dis. 56, 109–120. doi: 10.1111/j.1865-1682.2009.01073.x
McDonald, G. C., James, R., Krause, J., and Pizzari, T. (2013). Sexual networks: measuring sexual selection in structured, polyandrous populations. Philos. Trans. R. Soc. B Biol. Sci. 368:20120356. doi: 10.1098/rstb.2012.0356
McMichael, G. A., Eppard, M. B., Carlson, T. J., Carter, J. A., Ebberts, B. D., Brown, R. S., et al. (2010). The juvenile salmon acoustic telemetry system: a new tool. Fisheries 35, 9–22. doi: 10.1577/1548-8446-35.1.9
Mourier, J., Bass, N. C., Guttridge, T. L., Day, J., and Brown, C. (2017). Does detection range matter for inferring social networks in a benthic shark using acoustic telemetry? R. Soc. Open Sci. 4:170485. doi: 10.1098/rsos.170485
Mourier, J., Lédée, E. J. I., and Jacoby, D. M. P. (2019). A multilayer perspective for inferring spatial and social functioning in animal movement networks. bioRxiv [Preprint]. doi: 10.1101/749085
Mourier, J., Vercelloni, J., and Planes, S. (2012). Evidence of social communities in a spatially structured network of a free-ranging shark species. Anim. Behav. 83, 389–401. doi: 10.1016/j.anbehav.2011.11.008
Mumby, P. J., and Wabnitz, C. C. C. (2002). Spatial patterns of aggression, territory size, and harem size in five sympatric Caribbean parrotfish species. Environ. Biol. Fishes 63, 265–279. doi: 10.1023/A:1014359403167
Niezgoda, G., Benfield, M., Sisak, M., and Anson, P. (2002). Tracking acoustic transmitters by code division multiple access (CDMA)-based telemetry. Hydrobiologia 483, 275–286. doi: 10.1023/A:1021368720967
Papageorgiou, D., Christensen, C., Gall, G. E. C., Klarevas-Irby, J. A., Nyaguthii, B., Couzin, I. D., et al. (2019). The multilevel society of a small-brained bird. Curr. Biol. 29, R1120–R1121. doi: 10.1016/j.cub.2019.09.072
Pasquaretta, C., Dubois, T., Gomez-Moracho, T., Delepoulle, V. P., Le Loc’h, G., Heeb, P., et al. (2021). Analysis of temporal patterns in animal movement networks. Methods Ecol. Evol. 12, 101–113. doi: 10.1111/2041-210X.13364
Puga-Gonzalez, I., Sueur, C., and Sosa, S. (2021). Null models for animal social network analysis and data collected via focal sampling: pre-network or node network permutation? Methods Ecol. Evol. 12, 22–32. doi: 10.1111/2041-210X.13400
R Core Team (2020). R: A Language and Environment for Statistical Computing. Vienna: R Foundation for Statistical Computing.
Rutz, C., Burns, Z. T., James, R., Ismar, S. M. H., Burt, J., Otis, B., et al. (2012). Automated mapping of social networks in wild birds. Curr. Biol. 22, R669–R671. doi: 10.1016/j.cub.2012.06.037
Sadovy de Mitcheson, Y., and Colin, P. L. (2012). Reef Fish Spawning Aggregations: Biology, Research and Management. New York, NY: Springer Netherlands.
Shen, D., and Clark, E. (2016). Territorial and reproductive behavior of the three Caribbean Razorfishes of the Genus Xyrichtys (Labridae) at bonaire. Int. J. Ichthyol. 22, 33–59.
Silk, M. J., Jackson, A. L., Croft, D. P., Colhoun, K., and Bearhop, S. (2015). The consequences of unidentifiable individuals for the analysis of an animal social network. Anim. Behav. 104, 1–11. doi: 10.1016/j.anbehav.2015.03.005
Spiegel, O., Leu, S. T., Sih, A., and Bull, C. M. (2016). Socially interacting or indifferent neighbours? Randomization of movement paths to tease apart social preference and spatial constraints. Methods Ecol. Evol. 7, 971–979. doi: 10.1111/2041-210X.12553
Stockmaier, S., Stroeymeyt, N., Shattuck, E. C., Hawley, D. M., Meyers, L. A., and Bolnick, D. I. (2021). Infectious diseases and social distancing in nature. Science 371:eabc8881. doi: 10.1126/science.abc8881
Toledo, S., Shohami, D., Schiffner, I., Lourie, E., Orchan, Y., Bartan, Y., et al. (2020). Cognitive map-based navigation in wild bats revealed by a new high-throughput tracking system. Science 369, 188–193. doi: 10.1126/science.aax6904
Tylianakis, J. M., Didham, R. K., Bascompte, J., and Wardle, D. A. (2008). Global change and species interactions in terrestrial ecosystems. Ecol. Lett. 11, 1351–1363. doi: 10.1111/j.1461-0248.2008.01250.x
Warner, R. R. (2011). Mating behavior hermaphroditism reef fishes in coral reef fishes. Am. Sci. 72, 128–136.
Webber, Q. M. R., and Vander Wal, E. (2019). Trends and perspectives on the use of animal social network analysis in behavioural ecology: a bibliometric approach. Anim. Behav. 149, 77–87. doi: 10.1016/j.anbehav.2019.01.010
Weiland, M. A., Deng, Z. D., Seim, T. A., LaMarche, B. L., Choi, E. Y., Fu, T., et al. (2011). A cabled acoustic telemetry system for detecting and tracking juvenile salmon: part 1. engineering design and instrumentation. Sensors 11, 5645–5660. doi: 10.3390/s110605645
Wey, T., Blumstein, D. T., Shen, W., and Jordán, F. (2008). Social network analysis of animal behaviour: a promising tool for the study of sociality. Anim. Behav. 75, 333–344. doi: 10.1016/j.anbehav.2007.06.020
Whitehead, H. (2008). Analyzing Animal Societies: Quantitative Methods for Vertebrate Social Analysis. Chicago, IL: University of Chicago Press.
Keywords: acoustic telemetry, movement ecology, high-resolution tracking, animal social networks, social organization, fish behavior
Citation: Aspillaga E, Arlinghaus R, Martorell-Barceló M, Barcelo-Serra M and Alós J (2021) High-Throughput Tracking of Social Networks in Marine Fish Populations. Front. Mar. Sci. 8:688010. doi: 10.3389/fmars.2021.688010
Received: 30 March 2021; Accepted: 02 June 2021;
Published: 23 June 2021.
Edited by:
David M. P. Jacoby, Zoological Society of London, United KingdomReviewed by:
Paula Pattrick, South African Environmental Observation Network, South AfricaDavid José Nachón, University of Santiago de Compostela, Spain
Copyright © 2021 Aspillaga, Arlinghaus, Martorell-Barceló, Barcelo-Serra and Alós. This is an open-access article distributed under the terms of the Creative Commons Attribution License (CC BY). The use, distribution or reproduction in other forums is permitted, provided the original author(s) and the copyright owner(s) are credited and that the original publication in this journal is cited, in accordance with accepted academic practice. No use, distribution or reproduction is permitted which does not comply with these terms.
*Correspondence: Eneko Aspillaga, YXNwaWxsYWdhQGltZWRlYS51aWItY3NpYy5lcw==