- 1Center for Marine Studies, Federal University of Paraná, Pontal do Paraná, Brazil
- 2MARETEC/LARSyS, Instituto Superior Técnico, Universidade de Lisboa, Lisbon, Portugal
- 3Institute of Oceanography, Federal University of Rio Grande, Rio Grande, Brazil
- 4Department of Earth and Environmental Physics, Physics Institute, Federal University of Bahia, Salvador, Brazil
- 5Oceanographic Modeling and Observation Network (REMO), Salvador, Brazil
- 6Department of Meteorology, Institute of Geosciences, Federal University of Rio de Janeiro, Rio de Janeiro, Brazil
- 7Laboratory of Computational Methods in Engineering, COPPE, Federal University of Rio de Janeiro, Rio de Janeiro, Brazil
- 8Marine Environmental Monitoring Laboratory, Federal University of Pará, Belém, Brazil
- 9Company of Agricultural Research and Rural Extension of Santa Catarina (EPAGRI), Florianópolis, Brazil
- 10Faculty and Graduate School in Oceanography (FAOC/PPG-OCN), Rio de Janeiro State University, Rio de Janeiro, Brazil
- 11Brazilian Ocean Acidification Network (BrOA), Rio Grande, Brazil
- 12National Institute for Space Research (INPE), General Coordination of Space and Atmospheric Sciences, Centre for Weather Prediction and Climate Studies (CPTEC), Cachoeira Paulista, Brazil
- 13Oceanographic Institute, University of São Paulo, São Paulo, Brazil
- 14Directorate-General for Technical and Nuclear Development (DGDNTM) of the Brazilian Navy, Rio de Janeiro, Brazil
- 15Subsea Technology Lab, COPPE, Federal University of Rio de Janeiro, Rio de Janeiro, Brazil
- 16Laboratory of Computational Methods and Offshore Systems (LAMCSO), COPPE, Federal University of Rio de Janeiro, Rio de Janeiro, Brazil
- 17Physical Oceanography Laboratory, LOF/COPPE, Federal University of Rio de Janeiro, Rio de Janeiro, Brazil
- 18Atlantic International Research Centre, Lisbon, Portugal
- 19CoLAB +ATLANTIC, Peniche, Portugal
- 20Department of Oceanography, Institute of Geosciences, Federal University of Bahia, Salvador, Brazil
- 21Envex Engineering and Consulting, Curitiba, Brazil
- 22Brazilian Navy Hydrographic Center (CHM), Rio de Janeiro, Brazil
- 23PETROBRAS Research Centre (CENPES), Rio de Janeiro, Brazil
- 24Prooceano, Rio de Janeiro, Brazil
- 25LAMTec, University of the Azores, Ponta Delgada, Portugal
- 26Ministry of Science, Technology and Innovation (MCTI), Brasília, Brazil
- 27Sasakawa Global Ocean Institute, World Maritime University, Malmö, Sweden
Coastal ocean observing and modeling systems (coastal observatories), connected with regional and global ocean systems, improve the quality of information and forecasts for effective management of safe and sustainable maritime activities. The public availability of systematic and long-term information of the ocean is an engine for the Blue Economy, boosting economic growth, employment, and innovation. An overview of some Brazilian initiatives is presented in this paper, involving universities, private companies, federal and state agencies, covering institutions from south to north of Brazil. Although these initiatives focus mainly on ocean physics, integrated efforts can extend the scope to include biogeochemistry and marine biodiversity, helping to address interdisciplinary problems. Existing initiatives can be connected, and new ones fostered, to fill in the gaps of temporal and spatial coverage of ocean monitoring in the vast oceanic area under Brazilian jurisdiction (nationally referred to as the Blue Amazon, in reference to the similar richness of the Amazon Forest). The alignment among national and regional initiatives, as well as with international programs, can be promoted if coordinated by a national-level organization, maximizing the return of public investment and socioeconomic benefits. In light of international examples, possible future institutional arrangements are discussed, leveraging from existing national public policies and international cooperation that Brazil is taking part. The United Nations Decade of Ocean Science for Sustainable Development is a timely opportunity to encourage an institutional arrangement to support and articulate an integrated network of coastal observatories in Brazil.
Introduction
The economic activities of ocean-based industries are expanding rapidly, with a great potential for boosting economic growth, employment, and innovation (OECD, 2016; Stuchtey et al., 2020; Virdin et al., 2021). However, overfishing, pollution, and ocean acidification are important constraints to the development of the ocean economy, deteriorating the biodiversity and the health of the oceans (IPBES, 2019). The Blue Economy concept comprises a range of economic sectors and related policies to seek the sustainable use of the ocean, promoting economic growth and social inclusion (World Bank and United Nations Department of Economic and Social Affairs, 2017). To reverse the decline in ocean health and support a sustainable Blue Economy, the United Nations (UN) proclaimed a Decade of Ocean Science for Sustainable Development1, to be held from 2021 to 2030. The Ocean Decade will be a decisive contribution to the implementation of the Sustainable Development Goals (SDGs)2 of the UN 2030 Agenda, including SDG 14 devoted to conserving and sustainably managing ocean and marine resources (Ryabinin et al., 2019). Sustained ocean observations and forecasts play an essential role in this context (Rayner et al., 2019). A series of Essential Climate Variables (ECV) can characterize the local variability of physical and biogeochemical properties of coastal waters and provide relevant information to calibrate/validate or to be integrated into coastal models (GCOS, 2003). Thereafter, the scientific community can use the data to develop models to understand and predict the state of ecosystems and the impact of natural phenomena and/or anthropogenic interference in the coastal environment. As the series of ECV become longer, inferences can be made about the long-term climatic effects.
The Global Ocean Observing System (GOOS)3, a program under the Intergovernmental Oceanographic Commission (IOC) of the UNESCO, aims to deliver essential information for operational services, climate, and ocean health, linking local, national, and global initiatives monitoring the physical, chemical, and biological properties from the open ocean to the coast (IOC, 1998, 2019). The GOOS 2030 Strategy is based on the visionary Framework for Ocean Observing (Lindstrom et al., 2012). Integrated observing systems are implemented by GOOS Regional Alliances (GRAs), with a focus on coastal areas and regional seas, satisfying local requirements. GRAs enable regional cooperation, although there is great variability among the GRAs in terms of governance, scope, maturity of activity, and attitudes to data sharing (Tanhua et al., 2019).
In order to improve the quality of information and forecasts, coastal ocean observing and modeling systems (coastal observatories) need to be connected with regional and global systems, as coastal dynamics are driven by a range of physical processes from different temporal and spatial scales. Forecasts from operational models allow us to anticipate extreme events (e.g., coastal flooding) and predict the trajectory of pollutants released in the ocean and coastal seas (e.g., oil spills). A downscaling approach from global and regional models is normally followed to improve forecasts at coastal scale (e.g., Mateus et al., 2012). Numerical models are also essential to fill the gaps of sparse observations, helping to unveil current patterns and future trends. However, models must be validated by satellite and in situ observations and can be improved by data assimilation techniques for better oceanic forecasting (Kourafalou et al., 2015a, b; Davidson et al., 2019). The public availability of systematic real-time information on the ocean basins, regional seas, and coastal systems is fundamental for sustainable economic growth. Moreover, the investment in public information services can have a high economic return (Proctor and Howarth, 2008).
In Europe, the Copernicus Marine Environment Monitoring Service (CMEMS)4 provides free access to satellite and in situ observations, as well as modeling forecasts and reanalysis of the global ocean and European regional seas, generated by a network of European entities. CMEMS gives the necessary support to the development of a broad range of services related to the Blue Economy sectors worldwide. The European Marine Observation and Data Network (EMODnet)5 is another European initiative comprising more than 150 organizations that through seven discipline-based portals provide access to standardized observations, data quality indicators, and processed data products facilitating access and use (Martín Míguez et al., 2019). EuroGOOS, the European component of the GOOS, counts with 44 members and 5 Regional Operational Oceanographic Systems to coordinate and support the development and joint service provision in European maritime regions. Recently, EuroGOOS6 and the European Marine Board set up the European Ocean Observing System (EOOS)7 to align and connect existing initiatives, seeking efficiency, value for money, and to fill gaps in the European observing capacity (EuroGOOS-European Marine Board, 2016). EOOS acts as a framework that brings together users, system implementers, and funders to set priorities and facilitate the exchange of best practices and capacity within Europe. In addition, several coastal observatories are maintained by leading scientific institutions and consortia in Europe. The Joint European Research Infrastructure Network for Coastal Observatories (JERICO8) assembled 39 of these institutions of different countries to create an operational pan-European network of coastal observatories capable of timely and continuously delivering high-quality data and information products for research, but also industrial and management purposes, driving technological development in the marine services sector (Sparnocchia et al., 2016).
In the United States (US), marine data produced by federal agencies (e.g., Centre for Operational Oceanographic Products and Services, National Buoy Centre, Office of Coast Survey) or supported by federal funds need to be made publicly accessible to maximize the impact and accountability of the federal research investment, helping moving science and economy forward (NOAA Research Council, 2015). In order to do so, a free and open source web application was developed to deal with a large number of data providers (Simons, 2020). Thus, integration of these systems maximizes investment and lower costs of a range of entities (federal and state agencies, universities, private companies). The Integrated Ocean Observing System (IOOS)9, the US component of GOOS, aims to combine and convey the existing data in an integrated and standardized manner to ensure maximum interoperability, linking observations to modeling via data management and communication, allowing the development of improved products, services, and decision-support tools to meet the needs for ocean information of users and stakeholders (IOOS, 2018). IOOS first started with federal funding for several regional programs. Currently, IOOS includes 17 federal agencies and 11 regional associations, responsible for implementing regional observing systems covering the entire US coast and Great Lakes. Additional partners come from industry, academia, state, local, tribal governments, and other federal and non-federal organizations (Snowden et al., 2019). The regional associations complement the federal system, for instance, by providing higher-resolution observations, information, and tailored products for regional needs, as well as data portals. A non-profit organization (IOOS Association)10 is responsible for coordinating activities among the regional associations and facilitates collaboration with the federal agencies. Stakeholder engagement is considered fundamental for the success of the regional associations’ activities to deliver useful information. A clear understanding of the needs of stakeholders, including managers, decision-makers, end-users, and communities, is essential to achieve sustained funding and maximize the socioeconomic benefit of products and services delivered by integrated ocean observing systems (Mackenzie et al., 2019).
Similarly, a GOOS Regional Alliance to the South and Tropical Atlantic (OCEATLAN)11 represents the efforts of institutions in Argentina, Brazil, and Uruguay engaged in the planning and implementation of an operational oceanographic system. The Brazilian component (GOOS Brazil) is an executive committee subordinated to the Subcommission for the Sectorial Plan for the Resources of the Sea (PSRM)12, coordinated by the Interministerial Commission for the Resources of the Sea (CIRM)13. The PSRM is a 4-year pluriannual plan, currently on its 10th edition (2020–2023), aiming at integrating the Territorial Sea, the Economic Exclusive Zone, and the Continental Shelf into the Brazilian domain, through research activities, oceanographic monitoring, and climate studies, as well as exploration and conservation of its natural resources. The oceanic area under Brazilian jurisdiction, branded as Blue Amazon, currently totalizes 4.4 million km2, approximately half of the Brazilian terrestrial area. The Blue Amazon may reach 5.7 million km2 due to the efforts of the Brazilian Continental Shelf Survey Plan (LEPLAC)14, a government program instituted in 1989 to elaborate the proposal for the extension of the continental shelf, in accordance with the Law of the Sea Convention.
GOOS Brazil is responsible for several monitoring programs with publicly available data15. For example, the Prediction and Research Moored Array in the Tropical Atlantic (PIRATA), under the coordination of the National Space Institute (INPE) and the Brazilian Navy for more than 20 years, represents a successful multinational cooperation between Brazil, France, and the US that has evolved out of the necessity to address important scientific questions and to improve predictions in the Tropical Atlantic (Bourlès et al., 2019; Foltz et al., 2019). PIRATA represents a reference observational array of upper ocean and atmospheric moored data over the Tropical Atlantic, with climate-calibrated time series with a sampling interval of 10 min, covering latitudes from 20S to 20N since 1997. The National Buoy Program (PNBoia) aims to provide real-time information of the ocean state. Under the responsibility of the Brazilian Navy, PNBoia manages both moored buoys and drifters, collecting meteo-oceanographic data remotely since 1998. Initially, PNBoia consisted of eight moored buoys. Due to a public-private partnership with PETROBRAS, the Brazilian oil and gas company, plans are to help the maintenance and eventually increase the number of buoys along the Brazilian shelf. Continuous operation of these buoys is a challenge due to vandalism, maintenance problems, or the acquisition of new sensors produced abroad. The Brazilian component of the Global Sea Level Observing System (GLOSS-Brazil) is a network of 13 tide gauges operated and maintained by the Brazilian Navy, aiming to monitor the sea level for navigation and safety reasons. Yet, the current structure of GOOS Brazil does not include coastal operational modeling systems and regional associations, as EuroGOOS or IOOS. Nevertheless, GOOS Brazil is being reformulated to be aligned with The GOOS 2030 Strategy (IOC, 2019).
This paper presents an overview of coastal observation and operational modeling systems in Brazil, as a demonstration of ongoing efforts and existing institutional and scientific capital that could be aligned and empowered to boost the national capacity to observe and predict the ocean. Although most of these systems focus mainly on ocean physics, integrated efforts can extend the scope to include biogeochemistry and marine biodiversity, helping to address interdisciplinary problems. The UN Decade of Ocean Science for Sustainable Development is a timely opportunity to foster this debate toward an institutional arrangement to support and articulate data management and accessibility along the Blue Amazon. Taking this forward, we discuss possible institutional arrangements leveraging existing national public policies and international cooperation that Brazil is taking part, by mean of ocean science diplomacy, the intersection between ocean science and foreign policy (Polejack, 2021).
Brazilian Coastal Observing and Modeling Systems
The Brazilian Coastal Monitoring System (SiMCosta)
In 2007, the Brazilian government created the Brazilian Network on Global Climate Change Research (Rede CLIMA)16, with the overall objective to investigate a series of climate-related issues. This network was also formed to give support and to propose actions for the federal government to take in dealing with climate variability, especially concerning the consequences of the rapid climate change scenario. During two consecutive years, Rede CLIMA established its virtual infrastructure by selecting scientists from different Brazilian institutions. The science board of Rede CLIMA was organized in 13 networks: Climate Modeling, Agriculture, Economics, Biodiversity, Environmental and Ecosystem Services, Energy, Hydric Resources, Regional Development, Cities, Coastal Zones, the Oceans, Natural Disasters, and Health (Arraut et al., 2012). Each network was established in a specific institution but composed of several scientists from distinct Brazilian regions. The headquarters of the Coastal Zone network is located at the Federal University of Rio Grande due to its long tradition in dealing with education and research on coastal and oceanic ecosystems.
In December 2011, the proposal entitled Brazilian Coastal Monitoring System (SiMCosta)17, elaborated by the Coastal Zone network, was approved by the Climate Fund of the Brazilian Ministry of Environment (MMA) and Ministry of Science, Technology, and Innovation (MCTI). Hence, SiMCosta was created to collect, process, and distribute high-quality metocean data. SiMCosta follows the principles of the Global Climate Observing System (GCOS) and special sensors are attached to the buoys to measure oceanic (surface temperature and salinity, chlorophyll-a fluorescence, turbidity, colored dissolved organic matter, pH, dissolved oxygen, current profile, and surface wave statistics), and atmospheric properties (air temperature, atmospheric pressure, wind vector, gust wind, dew point temperature, humidity, and solar radiation). For its current configuration, SiMCosta is composed of 12 sea level stations (tide gauges) and 12 moored coastal buoys delivering meteorological and oceanographic data online (Figure 1). Tide gauges are more evenly distributed along the Brazilian coast, while moored buoys are more concentrated in a few states.
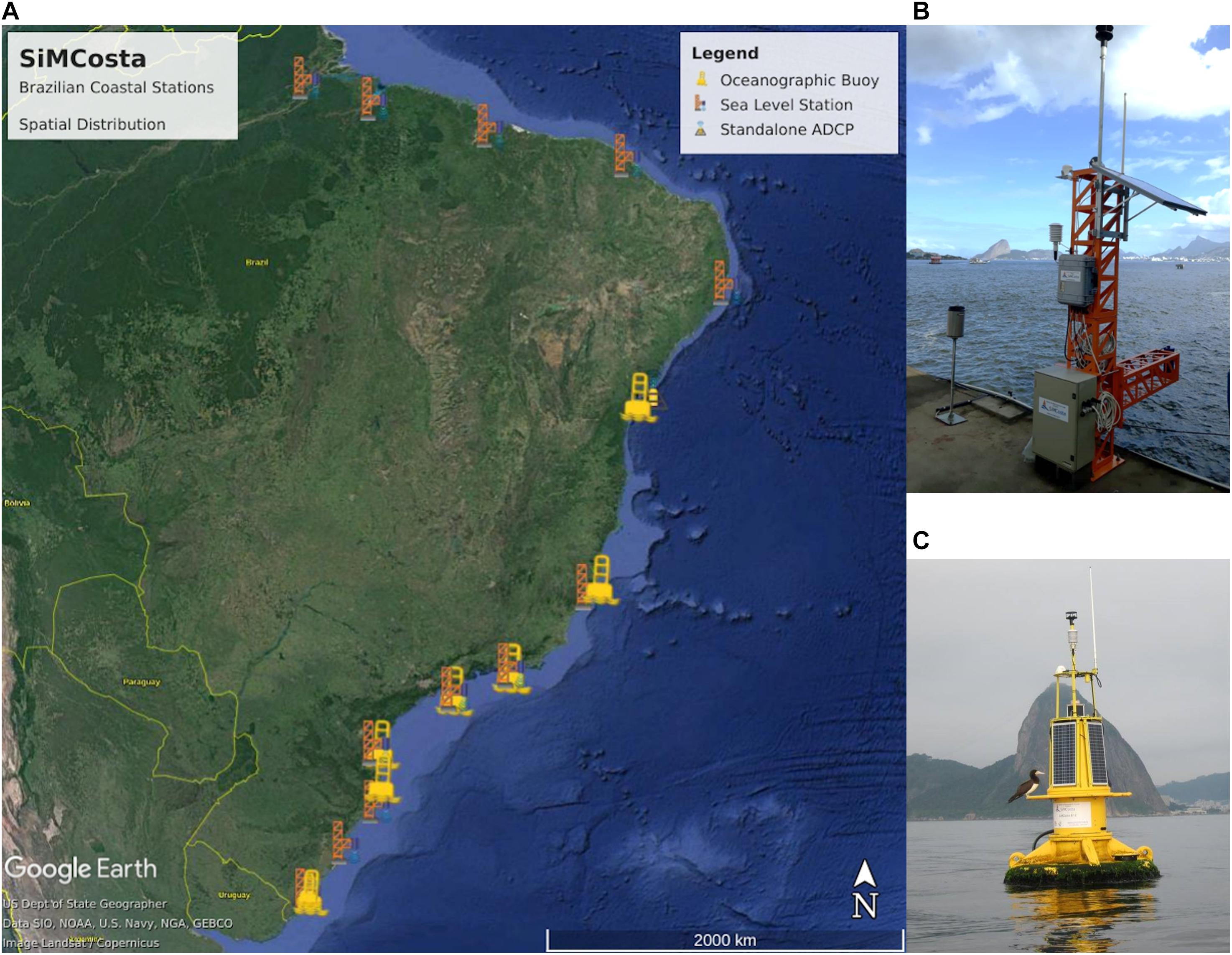
Figure 1. (A) The map spatial distribution of SiMCosta sea level stations and moored coastal buoys. (B) Example of SiMCosta sea level station and (C) moored coastal buoy. Data can be downloaded via www.simcosta.furg.br.
Sea level fluctuations are measured by a highly precise and stable microwave radar at 26 GHz with a resolution of 1 mm and an accuracy of ±2 mm. An effort is underway to link all SiMCosta sea level stations to the Geodetic Permanent Tide Gauge Network of the Brazilian Institute of Geography and Statistics (IBGE). The moored buoys are located in relatively shallow waters (depth < 25 m), where high-frequency (>1 h–1) data is necessary to characterize the high variability of short-scale oceanographic processes. One of the major efforts of SiMCosta’s team has been to develop improved data processing methods to allow the distribution of high-quality data. The SiMCosta data quality control system is heavily based on the experience acquired by the Quality Assurance/Quality Control of Real Time Oceanographic Data (QARTOD), from the US IOOS (U.S. Integrated Ocean Observing System, 2020). Users of SiMCosta metocean data can choose to download original and/or quality-controlled (flagged) data according to the SiMCosta quality control system. In summary, SiMCosta aims to improve the Brazilian coastal observing capacity by using advanced and integrated methodologies to collect, analyze, and distribute systematic time series of meteorological and oceanographic properties of several locations along the coast.
Another initiative of SiMCosta’s team is to offer ocean color products (i.e., chlorophyll-a concentration, total suspended solids concentration, turbidity, etc.) to the ocean community daily (Ávila et al., 2021; Silva and Garcia, 2021). The increased number of satellite sensors in recent years has considerably improved our spatial and temporal resolution for coastal studies. Satellite optical sensors allow a synoptic view of large coastal areas (Ciotti et al., 2010). The estimated product of satellite images will be locally validated using in situ radiometric and product measurements. In the long term, SiMCosta seeks to improve the capability of studies on climatic variability and change in collaboration with other national extreme events early warning systems (Khalid et al., 2020). By doing so, SiMCosta contributes with free and unrestricted access to high-quality coastal metocean data and ocean color products for the ocean community.
The Oceanographic Modeling and Observation Network (REMO)
The Oceanographic Modeling and Observation Network (REMO)18 focuses on the scientific and technological development of operational oceanography in Brazil, considering both numerical forecasts and hindcasts with data assimilation, and observational systems in the South Atlantic and regions along the Brazilian continental margin. REMO was created in 2007 by researchers from different institutions in Brazil. In 2011, full membership was granted in the Global Ocean Data Assimilation Experiment OceanView (GODAE OceanView), nowadays named OceanPredict19 (Bell et al., 2015). This membership allows REMO to participate in an international forum dedicated to the planning and development of operational oceanography in the global ocean and coastal areas.
The development of numerical ocean models with data assimilation is a largely desired goal for countries with an extensive oceanic area such as Brazil. The availability of such models improves safety of navigation, search and rescue operations, seabed exploitation, fisheries, nautical sports activities, among others. An ocean forecast system supported by REMO was implemented operationally in the Brazilian Navy Hydrographic Center (CHM)20 in January 2010, producing 5-day forecasts daily for an extensive area including latitudes 35.5oS to 7oN and longitudes between 20oW and the Brazilian coast (METAREA V). Long-term simulations of past conditions, or ocean hindcasts, on the other hand, provide a consistent dynamical interpolation of all the available data and have become an important aid for scientific research and environmental studies. REMO hindcasts have been widely used in recent years by the offshore oil industry operating in Brazilian waters. It is also important to address that the Brazilian Navy is the Governmental institution in Brazil entitled to provide numerical ocean modeling support and forecasts to any emergencies related to ocean oil spills, search and rescue operations, and other contingencies. The REMO modeling forecasts products are available to help the Brazilian Navy accomplish this task.
A suite of nested model configurations, mostly based on the Hybrid Coordinate Ocean Model – HYCOM (Bleck, 2002) has been developed and is continuously updated by REMO (Gabioux et al., 2013; Lima et al., 2013). The most recent configuration adopted within the basic REMO strategy has been to simulate the entire North, Equatorial, and South Atlantic with eddy-resolving 1/12o horizontal resolution, to generate lateral boundary conditions for a regional domain grid covering the METAREA-V with a horizontal resolution of 1/24o (Figure 2). Other regional grids are also in operation to simulate circulation on regional sites (Guanabara Bay and others). This strategy has been successful in dealing with the extremely intense computational demands associated with high-resolution ocean modeling, allowing to generate robust simulations of oceanographic phenomena at different time and spatial scales, such as: the large and mesoscale surface, subsurface, and deep currents; the mesoscale variability associated with baroclinic instabilities; the interaction between oceanic and shelf waters; the upper-ocean dynamics associated with atmospheric-forced interannual, seasonal, synoptic, and diurnal cycles; and the high-frequency oscillations, such as barotropic and baroclinic tides, and inertial-oscillations (e.g., Costa et al., 2017; Artana et al., 2019; Santana et al., 2020).
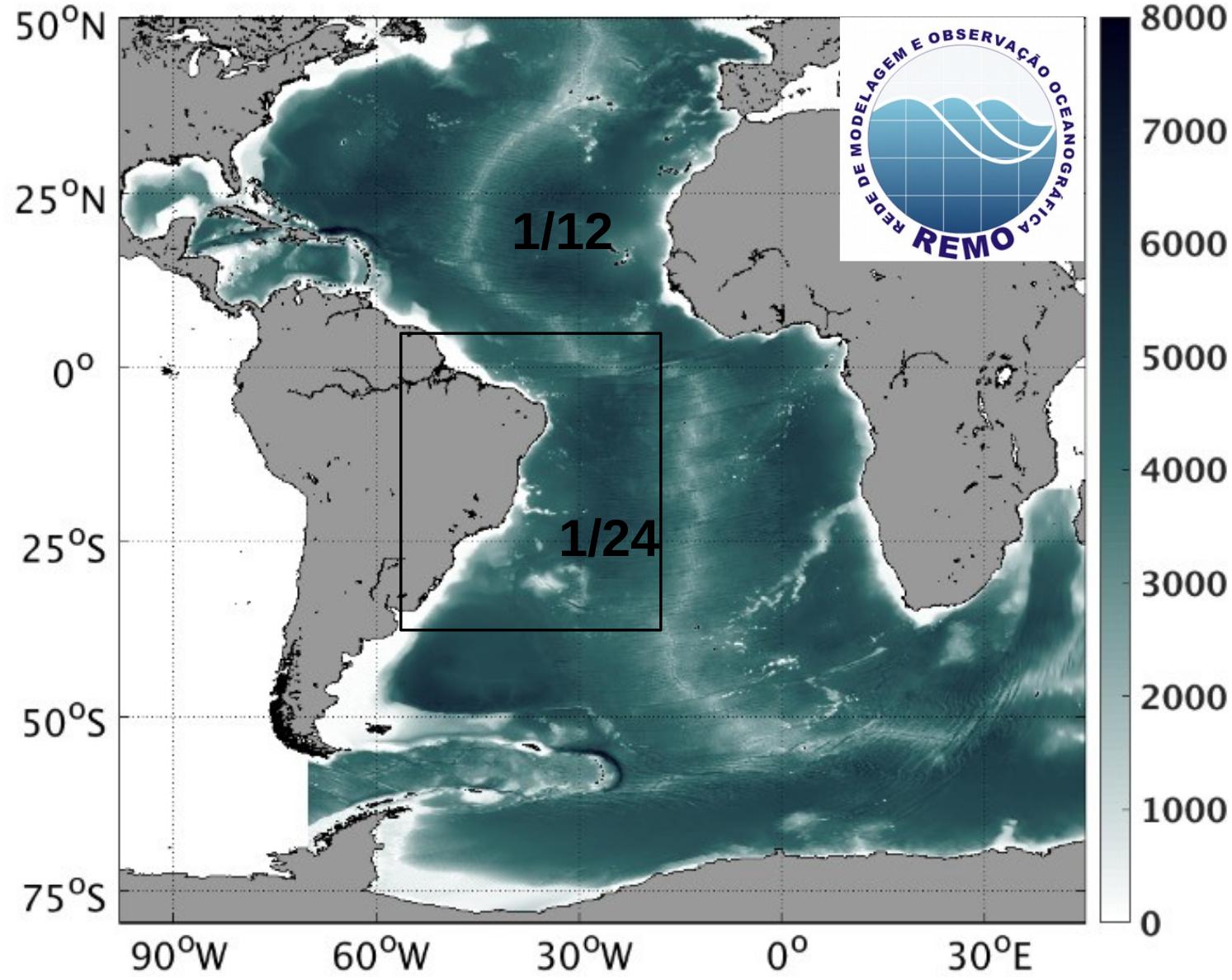
Figure 2. REMO models nesting configuration, operational at the CHM, showing the depth (m) of the Atlantic basin domain (HYCOM grid with 1/12o horizontal resolution), and the METAREA V domain (HYCOM grid with 1/24o horizontal resolution).
REMO benefits from the expertise of many research partners studying oceanographic processes associated with mesoscale dynamics in distinct Brazilian regions such as Cape São Tomé (Calado et al., 2010), Brazilian eastern shelf (Soutelino et al., 2013), and Equatorial Margin (Krelling et al., 2020a). Initiatives with feature oriented regional models were also implemented to study meanders and eddies of the Brazil Current (Calado et al., 2006, 2008) and North Brazil Undercurrent region (Krelling et al., 2020b).
Data assimilation schemes produce the so-called objective analysis by combining model outputs with in situ and remotely sensed observations, considering both the model and the observational errors. Data assimilation helps to improve model simulations by correcting possible bias and adjusting the mesoscale variability, introducing oceanic features, like meanders and eddies, at their observed time and location. REMO has adopted two complementary strategies concerning data assimilation. One of them is the Tendral Statistical Interpolation System – T-SIS (Halliwell et al., 2014), which has been mostly applied in hindcast simulations. T-SIS employs a version of the well-known Optimal Interpolation scheme, favoring computational efficiency, and was designed particularly to be implemented with HYCOM. At the same time, REMO was able to create the REMO Ocean Data Assimilation System (RODAS) based on the Ensemble Optimal Interpolation scheme – EnOI (Melo et al., 2013; Tanajura et al., 2014; Mignac et al., 2015; Carvalho et al., 2019; Tanajura et al., 2020), which has been implemented in the Navy operational forecasting system. A detailed description of RODAS is presented by Tanajura et al. (2020). Both schemes can assimilate vertical profiles of temperature (T) and salinity (S) from the ARGO system, XBTs, CTDs, gridded or along-track sea level anomaly data, and sea surface temperature fields. TSIS can also assimilate velocity information from moorings, ADCPs and drifters.
As discussed by Tanajura et al. (2020) on the description of the EnOI, the observational error covariance matrix depends on the observation type, and in general includes information about the instrumentation error (EI), the representativeness error (ER) due to unresolved scales, and an error associated with the relative “age” of each observation (EA). The latter is necessary in order to consider the relative importance between observations made a few days before the analysis time and observations at or closer to the analysis time. Therefore, the observation error variance EO2 of a single observation is given by EO2 = EI2 + ER2 + EA2. Model errors are also discussed in the article. It was shown that RODAS was able to substantially reduce the model errors with respect to the free run. The results of the assimilation run, in which all data were assimilated, could reduce SST errors by 50%, reduce vertical T and S errors by 47 and 46%, respectively, and improve SLA correlation by 50% with respect to the free run. The errors attained by RODAS are comparable with other OceanPredict systems.
Future developments within the scope of the REMO modeling activities are sought in different areas. Work is underway with the HYCOM + RODAS system to assimilate sea surface salinity and data from gliders, to better represent freshwater input from rivers and precipitation in coastal regions. Also, the system should be prepared to assimilate data that will be collected by the Sea Water and Ocean Topography (SWOT) satellite from 2022 on. From the methodological point of view, efforts are underway to construct a hybrid scheme by combining the EnOI with a Local Ensemble Transform Kalman Filter. Several initiatives are also being directed to improve the model configurations in general. Modeling is a continuously evolving field, and as new data, forcing fields, and sub-grid parametrizations become available they should be incorporated to improve model realism. Increasing horizontal and vertical resolution is foreseen, pending, however, on the availability of high-performance computing resources. It is expected that such combined initiatives should lead to improved hindcasts and more reliable forecasts.
On its ocean observational efforts, REMO is providing continuous support to the Brazilian Navy to maintain and operate the PNBoia buoys, being responsible for the acquisition of the buoy installed at Santos Basin and supporting technicians to maintain the other buoys. As the costs to operate buoys manufactured in other countries are quite high, due to factors such as floating currency exchange rates, difficulties to import spare parts, and other issues, REMO is also pursuing a Research and Development (R&D) initiative to fully develop and manufacture oceanic buoys in Brazil. This initiative is responsible for the development of the Brazilian Meteo-Oceanographic buoy (BMO-BR) with Brazilian universities and local technology companies. The BMO-BR has been tested and operated successfully on the Santos Basin shelf-break for one year. Recently, in December 2020, the BMO-BR was deployed by the Brazilian Navy as the first Brazilian deep water wave, meteorological and oceanographic buoy to operate on a 2200 m water depth, nearby offshore oil production installations at the Pre-Salt reservoirs in Santos Basin. The acquired metocean data will be used to evaluate REMO wave and oceanic models improving their forecasting skills.
The Santos Basin Ocean Observing System (Project Azul)
Each stage of the offshore oil and gas exploration and production (E&P) process presents a set of different activities that require a solid understanding of regional metocean conditions. This information can reduce uncertainties in designing offshore structures, assist in planning activities, reduce costs, and increase operations safety. Therefore, some research and development (R&D) projects are being carried out in Brazil based on the importance of defining a high quality and well-structured set of metocean data (in situ data and numerical model results) to plan and execute several E&P activities. These efforts are partly supported by the Brazilian National Agency of Petroleum, Natural Gas and Biofuels (ANP), which regulates the mandatory investment of 1% of oil companies’ revenues in R&D projects. The Santos Basin Ocean Observing System, also known as Project Azul, started in 2012 and was funded by Shell Brazil, a private oil and gas operator, in the context of the ANP R&D program. The project’s main goal was to develop an oceanic observation system for the Santos basin, the largest producer of oil and natural gas in Brazil, based on the acquisition of real-time oceanographic data and numerical modeling (Figure 3). Project Azul was developed by researchers and professionals from the Laboratory of Computational Methods in Engineering (LAMCE) of the Coordination of Postgraduate Engineering Programmes (COPPE) of the Federal University of Rio de Janeiro (UFRJ), and PROOCEANO, a Brazilian oceanographic technology company.
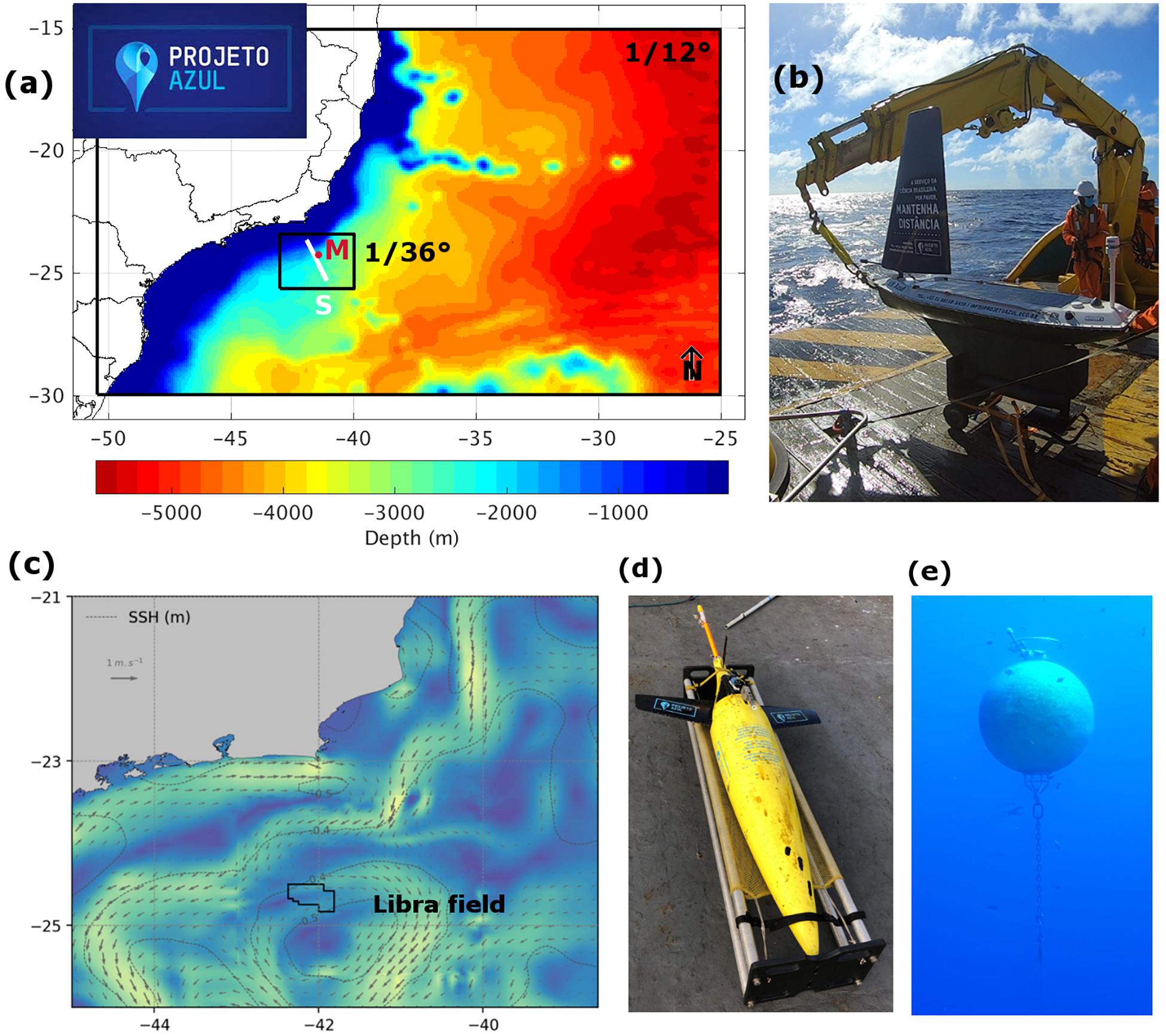
Figure 3. (a) Representation of Project Azul’s nested grids (black rectangles) and their bathymetry (color shading), in meters, the approximated seaglider trajectories (S, white line) and the positioning of the instrumented mooring line and sail buoy deployment (M, red dot). (b) Sailbuoy deployment. (c) Surface current and sea surface height forecast for the study area and the location of Libra field. (d) Sea glider and (e) the instrumented moored line used in Project’s phase 2.
The oceanographic data was obtained from a pioneering ocean observation system in the Santos basin, using surface drifters, Lagrangian floats, underwater gliders (used for the first time in Brazilian waters), and remote sensors (dos Santos et al., 2015). The purpose of this configuration was to maximize space coverage without extensive use of oceanographic vessels, reducing operational costs and risks, and minimizing emissions of carbon dioxide. Project Azul deployed 90 drifters, 36 floats, and 13 gliders missions in the first 3 years, with the real-time data made freely available to the scientific community through the project’s website21. The developments and results obtained throughout the project subsidized peer-reviewed articles, book chapters, doctoral theses, master’s dissertations, and undergraduate monographs. The extensive set of in situ oceanographic data enabled us to map in three-dimensions the Cabo Frio Eddy, an important mesoscale feature (Calado et al., 2010). The deployment of floats within different depths also allowed us to study the dynamics of subsurface currents, including the Intermediate Western Boundary Current (dos Santos et al., 2015). In situ data was assimilated into the Regional Ocean Modeling System (ROMS) (Shchepetkin and McWilliams, 2005; Haidvogel et al., 2008), through the primal incremental strong constraint 4D-variational data assimilation method (Moore et al., 2011), providing significant improvements in the numerical representation of the local ocean dynamics, including a better representation of Cabo Frio Eddy in surface and in-depth signatures (Fragoso et al., 2016). This represented a significant advance once the assimilation of remote sensing data does not guarantee the correct representation of a current system with high baroclinic instability.
Project Azul allowed significant innovations in terms of ocean observation system’s development, such as the higher spatial and temporal resolution of the ocean model, the most appropriate glider’s routes to better represent the variability and dynamic in the Santos basin, and the expansion of the system through the incorporation of new data acquisition sensors. The second phase of Project Azul (2017–2021) consolidated and improved the ocean observation system developed initially, with a particular focus on the Libra field, located in the northeast portion of the Santos basin pre-salt layer. This new phase included the use of autonomous surface vehicles and instrumented mooring lines, and the implementation of wave and data assimilative ocean models, both operational and with high spatial and temporal resolution (Mackenzie et al., 2019). The benefits brought by the project are not limited to oil and gas activities. The results generated will allow a better understanding of aspects of the ocean at a regional scale. Therefore, they can contribute to several studies, such as those associated with ocean climate and renewable energy.
Amazon Coastal Observatory (OCA)
The Amazon Coastal Zone covers approximately 1000 km of coastline (Dominguez, 2009) with several highly energetic environments of great global importance (Souza-Filho et al., 2009), such as the Amazon River estuary, which has 18% of the world’s fluvial discharge (Dai and Trenberth, 2002; Mikhailov, 2010) and the largest strip of continuous mangroves on the planet (Souza-Filho, 2005), cut by several estuaries and tidal channels. Due to the large size, high complexity, and importance of the Amazon Coastal Zone in the global context, the OCA22 was created to systemize and standardize the metocean data produced from a diversity of ecosystems, including the adjacent continental shelf, making it available and promoting multidisciplinary scientific development. The online platform allows viewing and requesting data for academic research.
The OCA’s database has been structured since 2009, with approximately 50 sampling stations available (Figure 4), comprising the OCA-Observational and OCA-Predict. The OCA-Observational provides past and real-time metocean data on rivers, tidal channels, estuaries, floodplains, and continental shelf. The data available on the online platform include currents (moorings and river cross-section), tides, waves, temperature, salinity, pH, Eh, conductivity, total dissolved solids, dissolved oxygen, nutrients, trace elements, chlorophyll, alkalinity, turbidity, suspended particulate matter, microplastic, bathymetric and sedimentological information, as well as meteorological parameters such as air temperature, humidity, atmospheric precipitation, and wind. The OCA-Predict provides tide predictions for various locations in the Amazon Coastal Zone. Meteoceanographic operational models are being developed to assist several socio-economic sectors in the region, including hydrodynamic models on a regional scale (entire Amazon Coastal Zone) and local scale (small estuaries and floodplains), foreseeing the upscaling for meso and large-scale operational models.
An initiative called “Oceanography in the Amazon” is composed by the OCA-Academic and OCA-News, aiming to spread out the knowledge, whether in academic, economic, or social terms, by translating numerical data into information for the entire community, supporting the safety and sustainability of the activities carried out in the Amazon coastal zone. The OCA-Academic gathers publications, theses, and dissertations. The OCA-News disseminate information from the academic world to all audiences with accessible language. Moreover, the OCA-Social develops citizen science projects, to integrate scientific and traditional knowledge, working on marine environmental monitoring issues. OCA works with a view to build new partnerships with ocean and coastal monitoring systems, being an initiative of local support for the training of researchers and information transfer. OCA has been supporting scientific development and publications such as tide propagation (Rollnic and Rosário, 2013; Prestes et al., 2017), discharge model (Borba and Rollnic, 2016; Prestes et al., 2020), Amazon Plume (Mascarenhas et al., 2016), salt intrusion in estuaries (Valentim et al., 2018), microplastic (Novaes et al., 2020), and meiofauna (Baia et al., 2021).
Santa Catarina Tide Gauges Network (SCTGN)
The first tide gauge of Santa Catarina state, one of the 27 federative units of Brazil, was the tide gauge of Imbituba, which in 1958 was defined as the Brazilian Altimetric Datum and is currently operated by the Brazilian Institute of Geography and Statistics (IBGE). The Santa Catarina coast has more than 450 km of extension, four important ports, and more than 50% of the population living in regions near the sea (Sebrae, 2013). Until recently, there was no other tide station available in the region, with just some isolated initiatives and short time series of tidal levels, normally related to academic works. In 2012, the Santa Catarina Agricultural Research and Outreach Company (EPAGRI) deployed its first automated tide gauge to meet the demands of a hydrodynamic modeling study at the Santa Catarina Island bay. This initiative was the first step toward the creation of the Santa Catarina Tide Gauges Network (SCTGN)23. Currently, the SCTGN has eleven tide gauges along the coast (Figure 5), composed of radar and piezoelectric level sensors, data loggers, and data transmission. The installation of new tide gauges over the years was made possible through financing from research projects, partnerships with ports, and EPAGRI’s resources. Furthermore, EPAGRI has a database infrastructure for receiving and storing the sea level time series (EPAGRI, 2020), with automated protocols for qualifying the data (Garbossa and Massignam, 2018). The tide gauges were adapted to measure the sea level every 5 min and water temperature at 15-min intervals. Besides, a research project focused on harmful algae tested the integration of a multiparametric probe to the tide gauge infrastructure to monitor physical-chemical parameters of the water near the shore.
The SCTGN has more than 5 million records stored in the database and more than 100 thousand records are acquired every day for tide and water temperature. This entire time series is freely available to the scientific community and public companies. However, increasing the visibility of this network and integrating other observation and modeling initiatives is mandatory to transform data into information. Recently, the tide gauge of Imbituba was adapted to perform measurements with 1-min intervals and redundancy to comply with the recommendations from the IOC for the sea level monitoring facility24. This tide gauge registered a meteotsunami event (Araujo et al., 2020), not previously recorded in the region, probably due to the lack of compatible monitoring. A recent partnership between EPAGRI and the Brazilian Sea Observatory (BSO, see below) allowed the validation of a numerical model for the Babitonga Bay, and is enabling the implementation of an operational modeling system for the entire Santa Catarina coast, which can support maritime activities, such as aquaculture and port operations. The way forward in coastal monitoring is to join efforts and knowledge from different research groups and institutions. Thus, SCTGN must establish partnerships with universities and initiatives, such as BSO, to convert data into useful information for society.
Brazilian Sea Observatory (BSO)
The Brazilian Sea Observatory (BSO)25 is a prototype of a coastal monitoring system, including an operational modeling system for the Brazilian coast and a WebGIS platform aiming to integrate forecast results, in situ measurements, satellite data, vessel positions, and an on-demand tool to predict the drift of oil spills (Figures 6, 7). BSO started to be developed in the frame of the User Uptake program from CMEMS. In order to deliver high-resolution forecasts of the Brazilian coast, an operational modeling system was developed based on a downscaling approach from the CMEMS forecast 1/12° global model, initially focusing on the south-eastern Brazilian shelf, including estuarine systems with important port activities and large environmental protection areas (e.g., Babitonga Bay and Paranaguá Estuarine Complex). The operational modeling system was implemented by the Centre for Marine Studies (CEM), from the Federal University of Paraná (UFPR), in collaboration with MARETEC, a research center of the Instituto Superior Técnico (IST – Universidade de Lisboa) from Portugal, through the application of the MOHID modeling system26, following a downscaling methodology similar to that previously described by Franz et al. (2016). The WebGIS platform was developed in partnership with the Brazilian company EnvEx Engineering and Consulting.
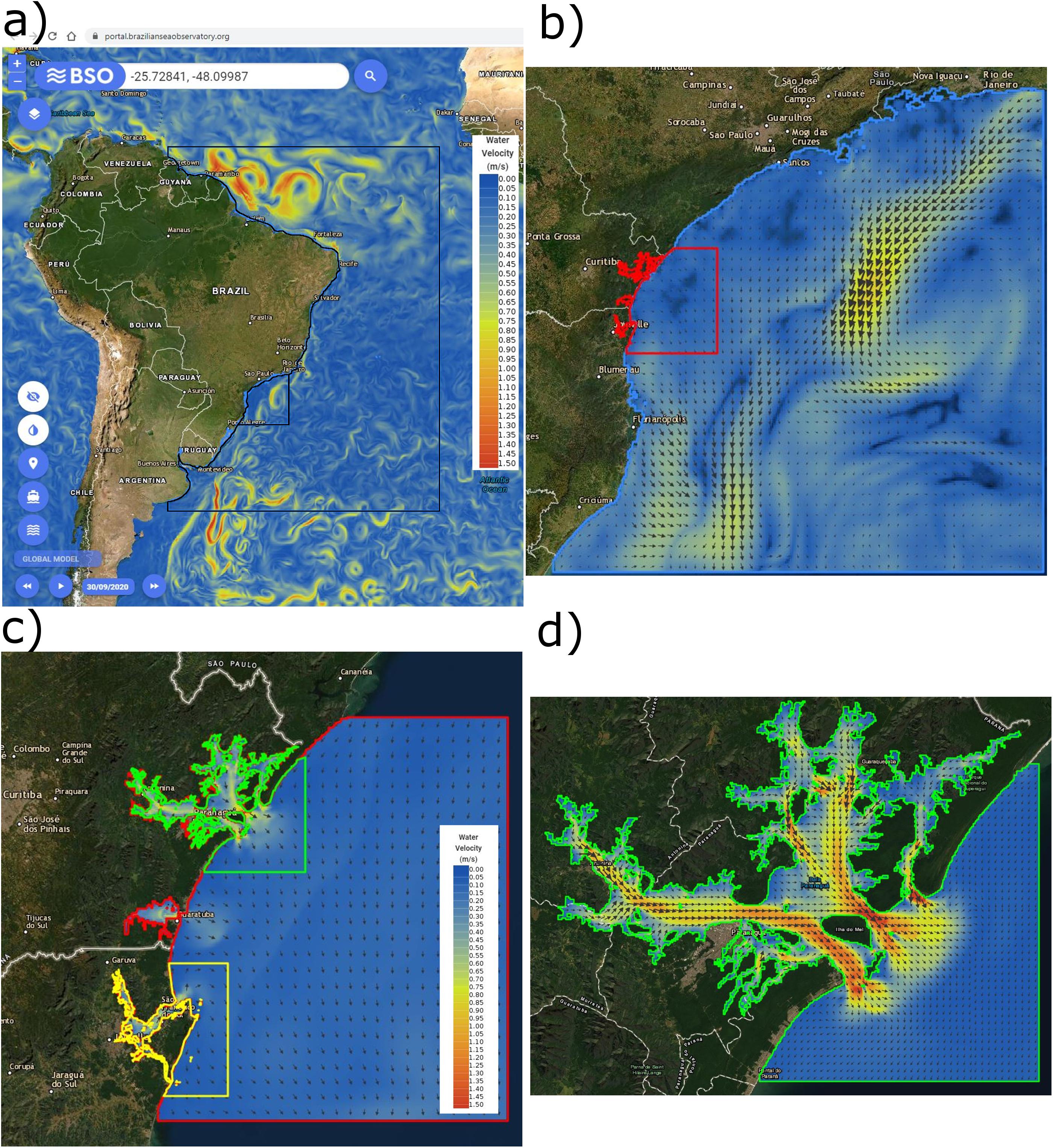
Figure 6. View of the WebGIS platform: forecasts from the CMEMS global model (a), south-eastern Brazilian shelf model (b), Santa Catarina and Paraná coastal model (c), Paranaguá Estuarine Complex model (d).
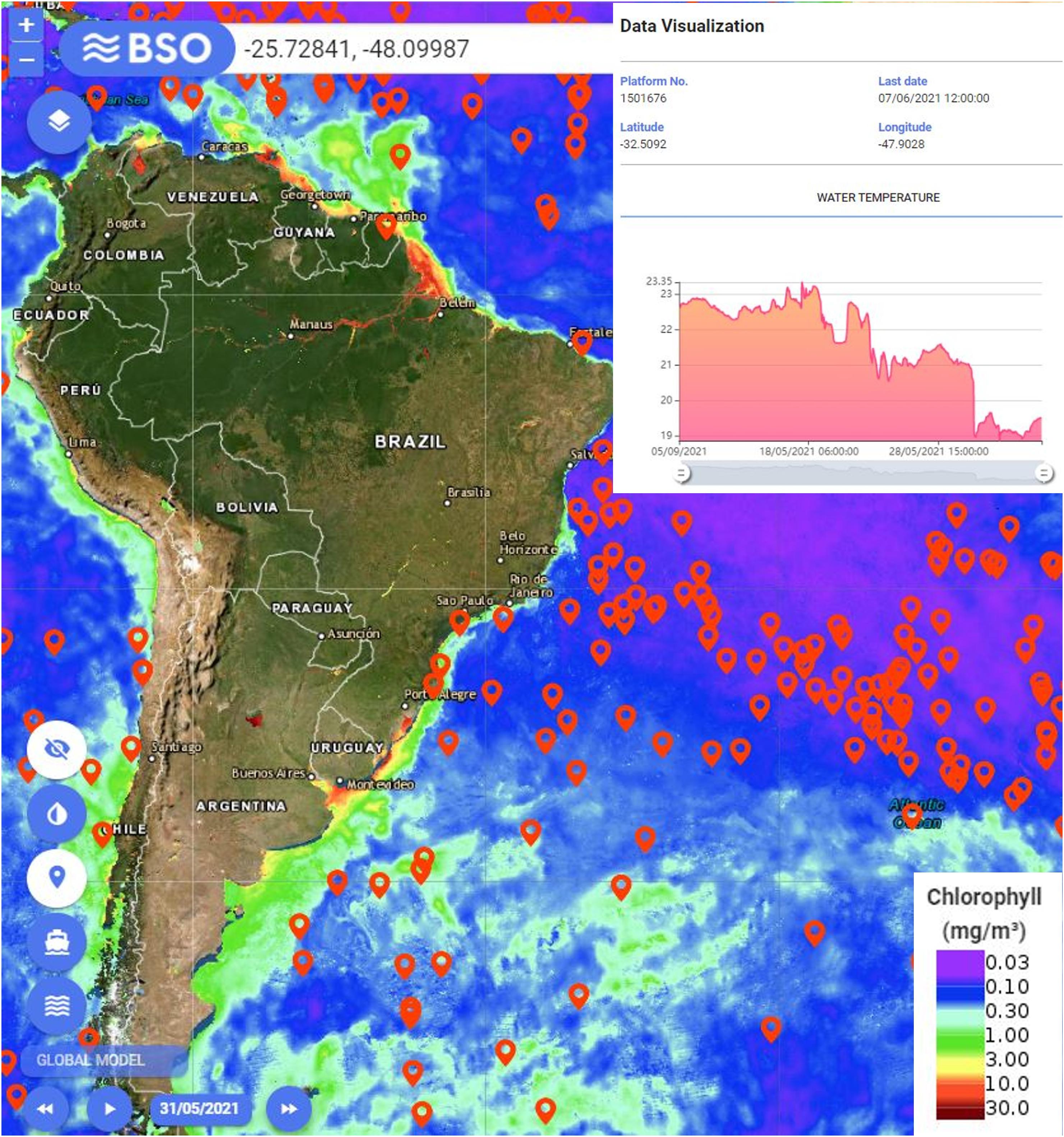
Figure 7. View of the WebGIS platform: satellite data of chlorophyll, drifting floats location and graph with in situ data.
A regional-scale model for the south-eastern Brazilian shelf is the first domain of the operational modeling system, with a horizontal grid resolution of approximately 3 km × 3 km and 50 vertical layers, with less than 1 m of thickness near the surface. The bathymetry of the regional model was defined based on the GEBCO27 online database. A coastal model for the Brazilian states of Santa Catarina and Paraná was nested to the regional model, with a horizontal grid resolution of 600 m × 600 m. The tidal level is considered in the oceanic boundary conditions of the coastal model through the tidal constituents from FES201428 (Finite Element Solution). The coastal model provides oceanic boundary conditions for the models of the Paranaguá Estuarine Complex and Babitonga Bay, with horizontal grid resolutions of 200 m × 200 m and 120 m × 120 m, respectively. The bathymetries for the coastal and estuarine models were defined based on nautical charts from the Brazilian Navy and data from local institutions and companies. Cartesian coordinates were used for the deeper layers and sigma coordinates for the layers closest to the surface, with few tens of centimeters of thickness near the surface. Atmospheric boundary conditions are obtained from the Global Forecast System (GFS29) with a horizontal resolution of 0.25°.
The lack of consistent flow data series is a great source of uncertainty to the accuracy of modeling results. The flow of 24 affluent rivers was considered through monthly averages calculated by using data from the National Water Resources Information System (SNIRH)30 or published papers and thesis. Although water quality parameters were initially considered to integrate the operational modeling system, this intent was postponed due to the shortage of data (e.g., nutrients) from rivers and coastal systems for model input and validation. Continuous monitoring programs are needed to decrease these uncertainties in the future, allowing to address water quality issues, such as oxygen depletion and phytoplankton growth. Validated watershed models can provide flows and water properties from affluent rivers to coastal systems. A coupling between watershed models and hydrodynamic models for the coastal zone can improve forecasts, as performed by Campuzano et al. (2016) for Western Iberia through the application of the MOHID modeling system.
The BSO modeling results were assessed with ARGO floats profiles, in situ measurements from CEM and SiMCosta, satellite observations, and tidal gauges data provided by Paranaguá Pilots Pilotage Services Ltda, EPAGRI and Brazilian Navy (BSO, 2019). The capacity of the forecasts in representing the effect of storm surges was evaluated in extreme events. Alerts of coastal floods are being released to coastal communities. The modeling system can provide information for different studies in the marine environment. Recently, the results of marine currents were used to support studies for the initial design of three submarine outfalls on the coast of Paraná, in compliance with environmental legislation, carried out by the company EnvEx for the Paraná Water Company (SANEPAR). The BSO modeling results are also currently supporting research related to microplastics dynamics and marine biology. The MOHID Lagrangian model was implemented to rapidly predict the drift of oil spills through an on-demand tool included in the WebGIS platform, based on the hydrodynamic forecasts from CMEMS for the entire Brazilian coast and BSO for the South-Eastern Brazilian shelf (Figure 6), including estuarine systems with high resolution. The influence of the wind drag is also considered based on the GFS results. An oil-spill detection tool based on satellite observations is planned to be included in the BSO to provide initial conditions automatically to the oil drift model. The on-demand simulation tool can be developed further to accomplish the specific needs of different stakeholders (e.g., to forecast the drift of algal blooms, marine litter, ship containers).
Brazilian Sea Observatory initiative wishes to promote a coastal cooperation network for the exchange of information and technology, being launched in a workshop to engage and raise the awareness of local, regional, and national stakeholders, not only maritime authorities but also the private sector, academic institutions, and community in general. In the future, the number of forecast results from operational models available in the WebGIS platform can be enhanced to encompass other important coastal systems, in partnership with local institutions and companies. The operational modeling system will be extended along the Santa Catarina State with a high-resolution coastal model to include the main shellfish aquaculture production zones in Brazil, in cooperation with EPAGRI. Furthermore, an operational model to the Amazon River and Pará River estuaries is being developed in collaboration with the Federal University of Pará (UFPA) and OCA. A partnership with REMO can enable gathering the forecasts from the CHM into the BSO WebGIS platform to predict the drift of oil spills and for the downscaling to BSO coastal models. The forecasts from BSO will be made available for downloading through the ERDDAP data server31, as performed by ocean observation systems and networks in the US (NOAA, IOOS), Europe (EMODNET), and other countries32.
Brazilian Network for Ocean Acidification (BrOA)
Ocean acidification refers to the decline in seawater pH, carbonate ion concentration, and the consequent decrease in calcium carbonate saturation state in seawater. These changes in seawater chemistry are caused by increasing atmospheric CO2 concentration from human emissions and subsequent absorption by the oceans of circa one-third of the excess carbon in the atmosphere (Doney et al., 2009; Jiang et al., 2019). The Brazilian Network for Ocean Acidification (BrOA)33 was created in 2012 by a group of scientists interested in the marine carbon cycle and the anthropogenic CO2 effects in seawater. The network nowadays includes researchers at all academic profiles from fourteen universities and research institutions in Brazil, whose main research topic is the observation of marine coastal and open ocean areas (Kerr et al., 2016), comprising the evaluation of the effects of ocean acidification in marine organisms through bioassays or in situ studies. BrOA members have, since 2012, published 13 peer-reviewed articles, although the topic ‘ocean acidification’ has been a subject of concern since 2000 among the Brazilian research community, with circa 150 published articles.
BrOA has identified the most ocean acidification sensitive ecosystems as the coral reefs and rhodolith beds along the eastern and north-eastern Brazilian shelf, as well as inner shelf areas under influence of megacities or riverine freshwater input (Kerr et al., 2016). Ocean acidification is exacerbated by the combination of anthropogenic CO2 uptake and increased organic matter degradation from human-driven eutrophication, especially at subsurface layers in coastal areas (Jiang et al., 2019; Cotovicz et al., 2020; Marotta et al., 2020). The extension and heterogeneity of the Brazilian coastline and Exclusive Economic Zone are also a challenge. Fixed station time-series such as those now included in SiMCosta and the maintenance of long-term ecological research (LTER/PELD) initiatives are strategic to a broader assessment of the acidification state and its impact on biodiversity in the country.
Brazilian Oil Research Group (BROIL)
Besides presenting a risk to those involved, accidents within any oil industry scope imply damages to the economy, tourism, fishing, and coastal communities. Oil spills also have alarming consequences for biodiversity and the ecosystem balance. It is precisely what happened in the middle of the second half of 2019 along the north-northeastern Brazilian coastline when crude oil slicks began to appear onshore. Approximately four months after the first appearances, nearly 1,000 beaches along more than 3,000 km of coastline had records of oil slicks. It became the most severe and extensive environmental disaster ever recorded in the history of Brazil, with significant environmental, economic, and social impacts. Unfortunately, we still do not know who, why, and where the origin of this spill occurred. The Brazilian Government, through the MCTI, the Brazilian Navy, and the National Council for Scientific and Technological Development (CNPq), launched a call for the setting up of research groups to improve the detection, monitoring, and mitigation of oil spills and fuel contamination at sea. Consequently, the Brazilian Oil Research Group (BROIL) was created, involving research institutions in Brazil and abroad, including the Federal Universities of Bahia, Pernambuco, and Rio de Janeiro along with INPE and Rio’s Pontifical Catholic University. Foreign partners include the Madeira Ocean Observatory (OOM-Portugal), the Institute of Research for Development – Laboratory for Studies in Geophysics and Spatial Oceanography (IRD/LEGOS-France) and the Institute of Coastal Research, Helmholtz-Zentrum Geesthacht (HZG-Germany).
Since observations of oil spills in the ocean correlate with oil tankers’ routes and the geographic location of oil/gas extraction blocks and offshore platforms, monitoring these areas is paramount to establishing contingency plans. Therefore, BROIL works upon three main pillars: (i) detection, through remote sensing techniques; (ii) control, through a set of the combined hydrographic ocean and oil weathering models; and (iii) remediation, through a set of biota oil-exposure case studies.
Detection
A series of open-source routines identifying potential oil spills based on two random forest classifiers were developed and tested with a Sentinel-1 SAR image dataset. The first random forest model is an ocean SAR image classifier. The labeling inputs were oil spills, biological films, rain cells, low wind regions, clean sea surface, ships, and terrain. The second was a SAR image oil detector called the Radar Image Oil Spill Seeker (RIOSS) (Conceição et al., 2021), which classified oil-like targets between Latitude 0° – 23°S and Longitude 49°W – 24°W, which corresponds to the affected area in 2019. We developed an optimized feature space to serve as input to such classification models, both in terms of variance and computational efficiency. It involved extensive research from image attribute definitions based on their correlations and classifier-based importance estimates. It included statistics, shape, fractal geometry, texture, and gradient-based attributes. Mixed adaptive thresholding was done to calculate some features studied, returning consistent dark spot segmentation results. We also related the selected features to the physical aspects of the imaged phenomena. This process helped us apply the attributes to a random forest, increasing the accuracy of our algorithm up to 90% and its ability to generate even more reliable results.
RIOSS works with three common steps in oil spill detection: image segmentation, feature extraction, and classification. Our method uses the block image separation technique, then applies mixed adaptive thresholding segmentation to identify possible dark targets in the SAR images. We performed the computation of a set of image features for each given block and gave them input to a random forest model tuned to classify its associated block. At first, the general classifier was trained to identify seven classes: oil spill, biological film, rain cells, low wind regions, sea, presence of ships, and terrain. This classifier can be helpful for the analysis of ships’ trajectory anomalies and be useful for other research groups like the Brazilian Sea Observatory (BSO). Then the oil detector was trained with two classes: oil spill and sea. More than 90% accuracy using only two classes was obtained, which is why we adopted this classifier as default for RIOSS. The features used to train both the classifiers and the detector’s random forest models were selected from five major categories: shape, complexity, statistical, gradient dependent, and textual features. To enhance our collection of images, we used Image augmentation, a technique often used in machine learning. It is a process of modifying dataset images into a set of variations (Shorten and Khoshgoftaar, 2019) before training or testing an algorithm so that learning is maximized in each sample. After the training step, the application of the classification method followed the RIOSS algorithm (Conceição et al., 2021). An input SAR image was divided into blocks by employing a clustering routine. The dark regions were segmented for each block, a feature set was calculated, and an oil spill probability was computed with the trained random forest model. At this stage, RIOSS is operational. The follow-up will be to add Sentinel-3 data to our remote sensing detection algorithms.
Control
A set of mathematical models will be implemented to simulate, predict and forecast the spill trajectory. They include the coupling of the Regional Ocean Modeling System - ROMS (Shchepetkin and McWilliams, 2003, 2005), forced with CMEMS reanalysis product outputs, and the Lagrangian model MEDSLIK-II (De Dominicis et al., 2013a, b). Both ROMS and MEDSLIK-II are open-source codes widely used in numerical ocean prediction, forecasting, and Lagrangian particle trajectory determination. MEDSLIK-II includes a proper representation of high-frequency currents and wind fields in the advective components of the Lagrangian trajectories and the Stokes drift velocities. It can be coupled with remote sensing data or numerical model outputs. This coupling (ROMS-MEDSLIK-II) allows integration of the results of ocean circulation modeling compatible with low latitude dynamics with a Lagrangian oil model. This step is currently under testing with different oil-type characteristics and numerical model outputs.
Remediation
Due to the magnitude of the 2019 oil spill in Brazil, entire marine natural populations were exposed to radiation, potentially causing consequences that range from fertility decrease to death. At this stage, we are using the ICRP reference organisms (i.e., flatfish, crab, and brown seaweed) to assess marine biota exposure due to uranium and thorium series radiation (Ulanovsky and Pröhl, 2006; Caffrey and Higley, 2013; ICRP, 2017). These three species, commonly observed in some areas along the northeastern coastline of Brazil, were selected as case studies. The oil spill scenario and the reference biota were simulated using the MCNP (Monte Carlo N-Particle) software (Pelowitz, 2005). Dose conversion coefficients for both uranium and thorium series were obtained as a function of oil activity concentration. The results obtained here can be used in an oil spill event and other worldwide recognized models. In addition, dose coefficients can be used strategically to assess the maximum exposure time for emergency oil control, removal, and mitigation.
Brazilian Earth System Model (BESM)
The development of the Brazilian Earth System Model (BESM) is an initiative of the Brazilian National Institute for Space Research (INPE) aimed at providing Brazil with the modeling capability ranging from extended weather (2–4 weeks) to seasonal climate (up to 6 months) to global climate change scenarios (decadal to centennial time scales). The BESM development, financed by the MCTI, the São Paulo Science and Technology Foundation (FAPESP), and the Coordination for the Improvement of Higher Education Personnel (CAPES), was based on the experience from INPE’s Centre for Weather Prediction and Climate Studies (CPTEC) global atmospheric modeling (BAM) and the use of state of the art component models for the global oceans (GFDL-MOM) and land model (SSiB/IBIS). The coupling strategy adopted was GFDL’s Flexible Modular System (FMS). The BESM model, initially described by Nobre et al. (2013), was eventually utilized for the Brazilian contribution to the CMIP5 project (Casagrande et al., 2016; Veiga et al., 2019; Capistrano et al., 2020). The current version of BESM3.0 couples BAM1.2 to MOM6 via FMS with a global ocean mesh of 1/4 × 1/4 degree lon-lat and 50 unevenly levels in the vertical. The model also incorporates SIS2 ice model and TOPAZ biogeochemistry model, both from GFDL. The in situ data from the PIRATA moorings corroborated observationally the coupled ocean-atmosphere simulations with the BESM model, suggesting the thermally indirect circulation (i.e., with enhanced rainfall over cooler sea surface temperatures) of the South Atlantic Convergence Zone (Nobre et al., 2012).
As a response to the aforementioned Call for Proposals in response to the 2019 massive oil spill in Brazil, INPE is leading a research consortium to develop an integrated satellite data, ocean modeling system to detect both ships and oil spills over the Brazilian ocean waters. For that end, a regional high resolution coupled ocean-atmosphere regional model over the Tropical-South Atlantic Ocean is being developed, with CPTEC’s Eta regional atmospheric model coupled to MOM6 regional model via FMS. The regional Eta-MOM6 shall use ocean and atmospheric lateral conditions from the BESM3.0 global coupled model, in order to address the need of higher resolution forecasting of ocean currents, temperature, and chlorophyll for oil spill detection and forecasting along the Brazilian coast.
Lastly, a Technical and Scientific Commission for the Advancement and Support to Monitoring activities and Neutralization of Impacts Arising from Marine Pollution by Oil and other Pollutants in the “Blue Amazon” (ComTecPolOleo) has been set up on October 2020, in order to coordinate all technical and scientific efforts being carried out in Brazil, after the 2019 oil spill. This Commission also serves as an advisory board to the Commander of the Navy in his capacity as National Maritime Authority, bearing in mind the importance of scientific knowledge in preparing for and mitigating marine pollution in Brazilian jurisdictional waters.
Future Perspectives
An integrated network of coastal observatories, connecting the existing initiatives described in this paper, as well as fostering new ones, could maximize the socio-economic impacts and benefits of public investments and fill the gaps in the Brazilian coastal ocean observing capacity, following the examples of Europe and the United States. A few steps are necessary for such integration to occur in Brazil, that would meet institutional standards, evidence, and responsiveness, for which it is proposed:
• The setting up of a new Brazilian Institute for the Sea (INMAR), a federally funded research institute to be publicly advertised soon and hopefully implemented over the next 2 years;
• The restructuring of the Brazilian node of GOOS, allowing for better interaction and interoperability;
• The modernization of the National Oceanographic Database, hosted by the Brazilian Navy Hydrographic Service;
• The integration between ocean physics observatories with those dedicated to marine biodiversity, as well as those focused on maritime safety, security and surveillance, under the banner of the Maritime Authority in Brazil;
• The exchange and integration of international ocean observatories through an ocean science diplomacy effort;
• Address the UN Decade of Ocean Science to raise public awareness and progress further in integrating and exchanging ocean observations, services and technologies for societal benefit;
• Engagement of stakeholders to catalyze the socioeconomic benefits of coastal observatories.
A proposal for a conceptual structure of a Brazilian Integrated Oceanographic System derived from our discussions is presented in Figure 8, integrating observing and modeling systems in different scales, such as the initiatives described herein, but also open to other initiatives linked to research projects and other actions that may not be covered in this paper. This integrated system should build up from the existing efforts carried out by the Brazilian node of GOOS that would compose the system’s governing body and hopefully have INMAR providing operational support to it. The third component of such a board should be the Regional Nodes of the system, allowing for a better engagement of stakeholders, including managers, decision-makers, and communities. Such an organization would facilitate the cooperation between federal and state-level agencies, the securement of funds and the provision of relevant services. This integrated system would ensure the sharing of infrastructure, knowledge, and data between observing and modeling systems, maximizing the socioeconomic benefit of products and services delivered and helping to achieve sustained funding. Alike to IOOS regional associations, the Regional Nodes would complement the federal system by providing higher-resolution observations and forecasts for regional needs, which would be publicly available through data portals and WebGIS platforms where information could be integrated and readily available for visualization. The Executive Committee for GOOS Brazil is supported by the Brazilian Hydrographic Service that plays a critical role in assisting with research platforms, shiptime, and therefore would greatly benefit from ocean observations and forecasts generated by Regional Nodes and coastal observatories for its activities, namely, search and rescue operations but also rapid response to environmental threats, such as marine pollution. The Brazilian Integrated Oceanographic System can deliver useful services to society and foster training, outreach, and capacity development, including technological innovation, enabling the development of the Blue Economy concept in Brazil. Profiting from the already established actions in education and outreach in the different observing capacities described in this paper, this integration would allow an optimization of these efforts, fostering technical capacity development as well as public engagement and citizen science to better supporting decision making. In this regard, INMAR will be central to connecting and supporting those initiatives.
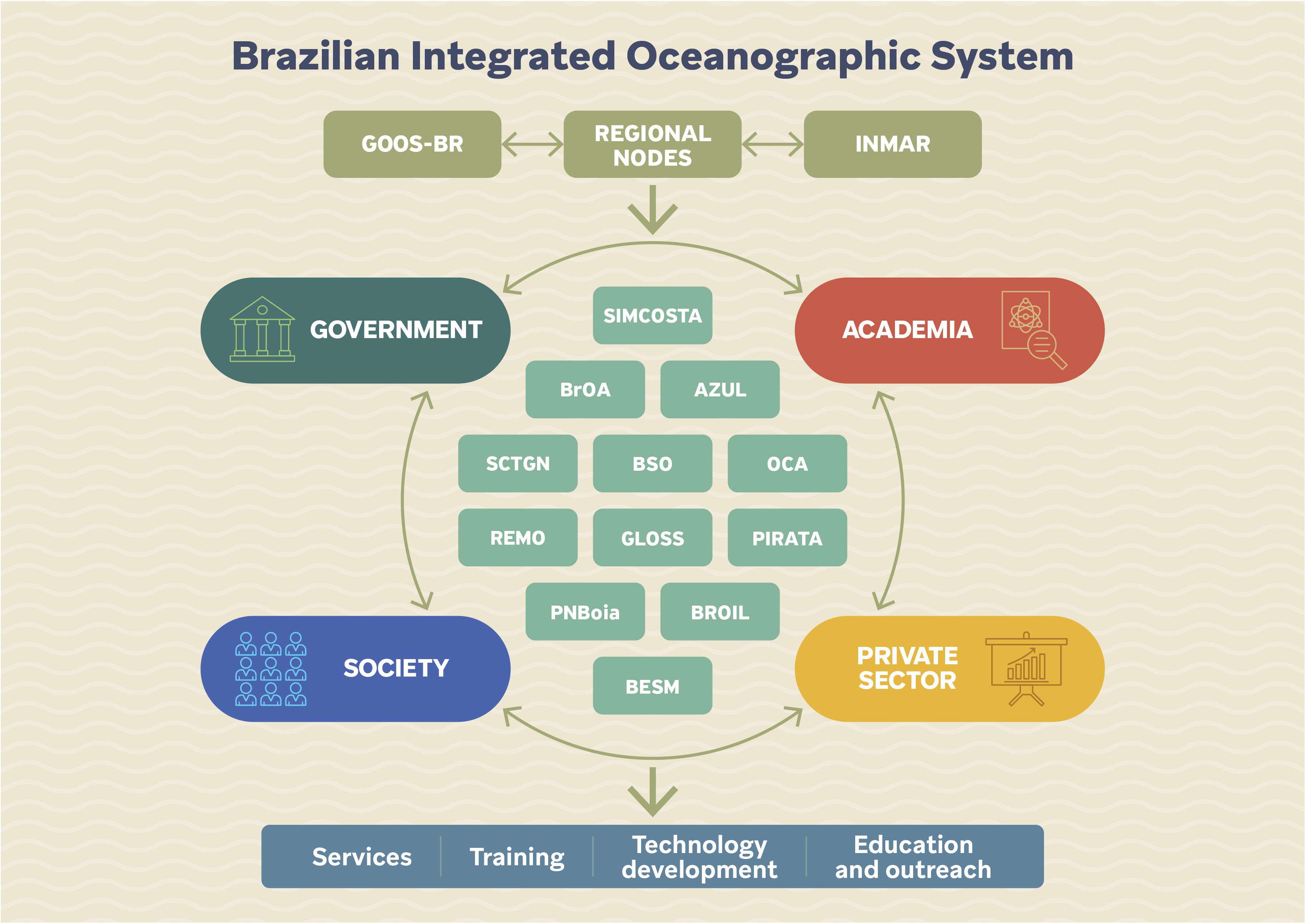
Figure 8. Proposal for a concept structure of a Brazilian Integrated Oceanographic System, integrating observing and modeling systems in different scales, such as the initiatives described herein, but also open to other initiatives linked to research projects and other actions not included in this paper.
The Role of the New Brazilian Institute for the Sea
Although this paper describes a few spontaneous interactions between initiatives, such as BSO with other initiatives such as REMO, OCA, and SCTGN, a coordinated structure that would also match with research infrastructure is greatly needed in Brazil. Historically speaking, back in 2013, a few initiatives were carried out for the setting up of a national institute for ocean research, under the regime of a federally funded research institute, led by MCTI and with a clear demonstration of support from the Brazilian Navy. The new institute would be part of a broader policy for the Brazilian Science, Technology and Innovation (STI) System with regard to Ocean Sciences but, unfortunately, the process has been hindered by bureaucratic difficulties.
More recently, MCTI launched a program called “Science at Sea” and readdressed plans for the setting up of a new National Institute for the Sea (INMAR, as it has been called) despite of budget restrictions facing the STI sector in recent years (Angelo, 2019). Officially, the process of establishing the new National Institute has already been deployed, facing some challenges ahead but representing an important step in integrating observations, research infrastructure and operational modeling systems across the country.
Some of the work that has been coordinated by ComTecPolOleo with regard to Marine Pollution by oil and other pollutants (such as plastics) will likely be absorbed by INMAR; but until then, some efforts and financial resources have been provided by the Brazilian Navy (Call CNPq/MCTI 06/2020) fostering the continuity and integration of efforts toward early warnings, detection and mitigation of marine pollution by oil at sea. However, this integration should not rely solely on project’s funding mechanisms, generally of a short lifespan, but rather in a national-level organization, facilitating the alignment among national and regional initiatives, securing federal resources, as well as integrating with international programs. Coastal observatories could then be continued and evolve to a sustained manner, providing greater return to society from invested resources.
Along with the institutional framework necessary to create a truly integrated coastal observatory network, several examples could be provided to support the concept that optimized human and financial resources are fundamental to ensure the maintenance of existing initiatives for long-term systematic monitoring and support the installation of new data collection platforms. An example is the project Costa Norte34, financially supported by Enauta O&G company under ANP R&D initiative. During the project time frame (2016–2020), a regional hydrodynamic modeling system based on the use of oceanographic data assimilation techniques was developed to represent the Brazilian equatorial margin coastal and oceanic dynamics (de Freitas Assad et al., 2020; Toste et al., 2020). The project developments could be integrated with other modeling and observation regional research initiatives, through the coordination of a national institute.
Investment in professional and academic training is also critical in some priority areas (e.g., maintenance of equipment and sensors, oceanographic data analysis, hydrodynamic modeling, and data assimilation). In addition, the optimization of shiptime and further costs related to oceanographic equipment purchase and maintenance, as well as the engagement of private companies, are elements that call up for the urgent setup of INMAR. The implementation of effective technology transfer mechanisms, properly qualified personnel and infrastructure for manufacture and maintenance of oceanographic equipment are mandatory to the establishment of a national ocean observing network along the Brazilian coast, as already identified by the Brazilian Ocean Sciences Capacity Building Programme (PPGMAR)35. In short, it is expected that INMAR would promote the necessary conditions to face the issues of funding, human resources, and technological capacities, promoting the development of the Blue Economy in Brazil, boosting economic growth, employment, and innovation, at the same time contributing to the sustainable use of oceans.
The Reformulation of GOOS Brazil
Another action in progress is the reformulation and upgrading of GOOS Brazil to be aligned with The GOOS 2030 Strategy (IOC, 2019). The Brazilian Navy, through the Directorate of Hydrography and Navigation (DHN), is the National Institution whose function is to promote and coordinate Brazil’s participation in the activities of the IOC related to Oceanic Services and Ocean Mapping (Federal Decree of 5 January 1994). Under the coordination of the Brazilian Navy, the GOOS Brazil Executive Committee will include representatives from five Ministries (Mines and Energy, Science, Technology and Innovation, Environment, Regional Development, and Education), as well as representatives of the scientific community involved with the implementation and maintenance of coastal observatories. The new structure might merge initiatives and already start to integrate at least in part those systems. The new organization of GOOS Brazil shall include specific groups dedicated to: (i) Ocean Gliders and Autonomous Underwater Vehicles, (ii) Drifters and ARGO Floats, (iii) Satellite Remote Sensing and Ocean Radar, (iv) Coastal Meteorological Stations, (v) National Sea Level Network, (vi) National Mooring Network, (vii) Hydrographic ships and Ships of Opportunity, and (viii) Animal tracking. In addition, GOOS Brazil will also include two important components, one dedicated to Quality Assurance and Control, and implementation of Best Practices, and the other responsible for organizing the Brazilian National Oceanographic Database.
The Modernization of the Brazilian National Oceanographic Database
The National Oceanographic Database (BNDO) is a repository center for hydrographic and oceanic data run by DHN and represents the Brazilian efforts toward the IOC/UNESCO “International Oceanographic Data and Information Exchange” (IODE) program. One of BNDO’s main activities is to gather, process and disseminate data collected by national and international institutions authorized by the national Decree n° 96.000/1988 to conduct research on the Brazilian continental shelf and jurisdictional waters. This Decree mandates that authorized institutions should provide, within 12 months after completion of data collection, all data and reports recorded in digital media (e.g., DVD) to BNDO.
Despite hosting a significant archive of oceanographic data pertaining to national and international research projects, those data sets are not promptly available online, imposing a lengthy and human resource-demanding process to get access by the interested user. Among these data there is a centennial and historical tidal data set, along with bathymetric surveys, used to construct nautical charts. However, bathymetric surveys are not publicly available, which would provide more data for improving the results of hydrodynamic models applied in coastal systems that rely strongly on bathymetry information. Furthermore, the nautical charts are not in a suitable format to be used directly by modelers, requiring an unnecessary effort to digitize the depth values, which need to be repeated by different research groups or companies. Thus, BNDO needs to be modernized to facilitate the transfer and accessibility of data in an integrated and standardized manner through web applications, maximizing the benefits of the federal research investment. Data collected by companies (e.g., from oil exploration and port activities) should also be provided to increase the availability of ocean observations. These companies, in turn, can benefit from the development of robust coastal observatories.
Ocean Observations in Support to Maritime Safety and Surveillance
The Brazilian Navy is also coordinating the implementation of the Monitoring System of the Blue Amazon (SisGAAz)36, which aims to monitor, continuously and in an integrated manner, the Brazilian Jurisdictional Waters and the international areas of Brazilian responsibility, to support search and rescue operations and rapid response in case of a threat, emergency, environmental disaster, conflict or criminal activity. A public consultation was elaborated in 2014, in which three consortia presented proposals with technological solutions for the SisGAAz implementation. However, in 2018, the acquisition process of SisGAAz was suspended, in part by the lack of financial resources37. Besides the high uncertainty in institutional and resource provision in Brazil (Gerhardinger et al., 2018), the lack of integration of efforts, knowledge and scientific infrastructure among institutions represent an additional barrier to advance in the proposal and implementation of public policies related to the ocean. The cooperation within institutions can avoid duplication of efforts and foster synergies to enable a complex system in Brazil such as SisGAAz, following the example of the European Maritime Safety Agency (EMSA)38, which plays an important role in increasing the safety and security of the EU maritime domain and contributes to the prevention and response to marine pollution. Among other functions, EMSA offers satellite-based Earth Observation services such as CleanSeaNet and the Copernicus Maritime Surveillance service, in collaboration with the European Space Agency (ESA), with a wide range of operational functions, such as oil spill and vessel detection, identification of polluters, maritime safety and security, and fisheries control. The collaboration with universities and research centers allowed the development of an automated early warning system for near-real-time modeling and prediction of the detected oil spills in Europe (Fernandes et al., 2017).
The Need to Incorporate the Monitoring of Marine Biodiversity in Brazil
The long-term monitoring of marine biodiversity in Brazil has been an important gap (Turra et al., 2013; Joly et al., 2018; Lacerda et al., 2018), but a growing number of initiatives are being recorded. National programs are being funded by a combination of national and subnational institutions (Brazilian Innovation Agency – FINEP, CNPq, and state Research Foundations – e.g., FAPESP), such as the Long-Term Ecological Research Program (PELD), National Biodiversity Research System Program (SisBiota), and National Institutes of Science, Technology and Innovation (INCT)39. Companies also contribute to the monitoring and conservation of emblematic marine species or habitats, both due to licensing obligations (although the data are not widely and easily shared) and socio-environmental programs. One of the most long-standing programs, Rede Biomar, is supported by PETROBRAS and includes humpback whales40, spinner dolphin41, albatross42, sea turtles43, goliath grouper44, and corals45 as target species or habitats. Right whale46, franciscana dolphin47, manatee48, pinnipeds49, manta ray50, and uçá crab51 are examples of other monitoring and conservation initiatives along the Brazilian coast.
Despite the recent efforts to widen the monitoring of the Brazilian marine biodiversity, syntheses of knowledge are still incipient. The Brazilian Synthesis Centre on Biodiversity and Ecosystem Services52 was created in 2019 by CNPq based on the PELD experience, with one project focused on the ocean. The ReefSyn – Brazilian Reefs Synthesis Group53 intends to (i) investigate the distribution of biodiversity components and reef ecosystem services along the Brazilian coast and its oceanic islands, (ii) understand the effects of climate change on the diversity and provision of ecosystem services, and (iii) contribute to current and future management strategies for Brazilian reefs, based on the organisms’ responses to climate change and the maintenance of reef functions. The Brazilian Platform on Biodiversity and Ecosystem Services (BPBES)54 is the national branch of the Intergovernmental Science-Policy Platform on Biodiversity and Ecosystem Services (IPBES) and is leading the Brazilian Assessment on Coastal and Marine Biodiversity and Ecosystem Services. The assessment aims to determine (i) the state of knowledge on biodiversity and ecosystem services, as well as trends and determinants of the transformation of Brazilian coastal and marine zones; (ii) the consequences of this transformation for human well-being associated with biodiversity and ecosystem services, taking into account the various knowledge and value systems; (iii) the necessary measures, and governance options, to mitigate degradation and to restore biodiversity and ecosystem services. The Brazilian Network for Monitoring Coastal Benthic Habitats (ReBentos)55 aims to detect the effects of regional and global environmental changes on benthic habitats, by creating time-series of data and syntheses on biodiversity along the Brazilian coast (Turra and Denadai, 2016), as the Protocols for Monitoring Benthic Coastal Habitats (Turra and Denadai, 2015), the Special Issue in the Brazilian Journal of Oceanography56 published in 2016 and the Springer Series on the Brazilian Marine Biodiversity (Lana and Bernardino, 2018; Sumida et al., 2020).
Information on Brazilian Biodiversity is shared through national data repositories linked to international ones, such as the Ocean Biodiversity Observation System (OBIS)57. The Information System of the Brazilian Biodiversity (SiBBr)58 is an online platform that integrates data on biodiversity and ecosystems from different sources in Brazil and abroad. The Environmental Information System of the Biota/FAPESP Program (SinBiota)59 was developed with the aim of integrating information generated by researchers linked to the Biota/FAPESP Program and relating them to a quality digital cartographic base, thus providing mechanisms for the dissemination of information on São Paulo’s biodiversity to the scientific community, decision makers, policy makers and educators. Besides these efforts, Brazil still needs a concerted approach to provide a holistic view of the trends in marine biodiversity to support policies and conservation strategies, as the National Action Plans for the Conservation of Endangered Species of the Chico Mendes Institute for Biodiversity Conservation (ICMBio), the National System of Coastal and Marine Protected Areas (Magris et al., 2020), and Marine Spatial Planning (Gerhardinger et al., 2020). The magnitude of these efforts illustrate the need to integrate oceanographic (mostly physical variables) with biological information, whose monitoring is also an important demand in the country, as evidenced by all the efforts aforementioned.
Ocean Science Diplomacy as a Tool for Interoperable Ocean Observations
As the ocean community advances in setting the minimal requirements to monitor the ocean state, as well as to produce better forecasts, the international debate becomes critical (Lindstrom et al., 2012). Therefore, the alignment and interoperability of ocean observatories do not only occur nationally, but it is the product of an international discussion. As a general rule, developing countries have less access to the required science and technology capacities to meet international standards and be effectively able to contribute to global observation networks. Efforts to overcome such challenges include international cooperation in ocean observations and effective mechanisms for the transfer of marine technologies and access to data, intending to benefit society in general (Salpin et al., 2018; de Young et al., 2019). Brazil has been an important player in the ocean science scenario in the Tropical and South Atlantic, boosting its international cooperation framework to meet its interests but also to address global ocean agendas through the application of scientific evidence to ocean management (Polejack and Barros-Platiau, 2020).
One such example is the Belém Statement on Atlantic Research and Innovation Cooperation60 signed in 2017 by Brazil, South Africa, and European Union. This Statement is an official international instrument that has gathered scientific expertise and became politically relevant, a case of science diplomacy in the Atlantic (Polejack et al., 2021). The Belém Statement provides a framework for the alignment of activities and budget, as well as an important platform for the exchange of scientists and capacity development, with the ambition to deliver information and services to society. On the issue of ocean observations, one of Belém’s core areas, the support to an All-Atlantic Ocean observing network (AtlantOS) will allow capacity building and technology transfer to improve monitoring and forecast (de Young et al., 2019). By providing an interconnected space for dialogue and exchange, the Belém Statement will aid countries to understand their different realities in implementing ocean observatories and learn from best practices. In addition, the Belém Statement is built upon existing initiatives such as the Atlantic International Research Centre (AIR Centre)61, a collaborative framework proposal by Portugal to address global challenges and local priorities in the Atlantic Ocean, including the interaction with research groups in Brazil, taking advantage of existing research centers and universities infrastructures. Finally, research networks and scientific results arising from the Belém Statement shall provide a critical framework deemed essential to achieve the high ambitions set up by the UN Decade of Ocean Science for Sustainable Development in the Atlantic (Claudet et al., 2019).
The Importance of the UN Decade of Ocean Science
The UN Decade of Ocean Science represents a unique opportunity to address joint efforts in favor of effective actions for the sustainable use of oceanic resources, promoting economic growth and social inclusion, at the same time as reversing the decline of ocean health. Better monitoring and forecasting capacities are key factors in this context, which will just be possible through a strong network of coastal observatories with massive participation of the academic community, public sector, companies, non-governmental entities, and local communities. It rests yet unclear on how this global ocean movement will concretely aid countries in establishing sustained coastal observatories required to meet international standards. However, some movements are emerging based on the implementation plan of the Ocean Decade, which considers different levels of actions: programs, projects, activities, and contributions. One example of an overarching program is CoastPredict62, which aims to promote “a predicted coastal ocean where society understands and can respond to changing ocean conditions,” and “best practices and standards for the global coastal ocean observing and prediction system, designed globally and implemented locally.” Recently, groups are emerging from the different Brazilian regions to support the achievement of the Ocean Decade goals after workshops promoted by the MCTI.
MCTI’s Science at Sea Program (MCTI, 2021), is expected to play an important role in the implementation of the UN Decade of Ocean Science in Brazil. This program aims to support and coordinate all relevant activities related to marine science at a national level with emphasis on six thematic areas: risk management; prevention and mitigation of environmental disasters at sea; marine biodiversity; coastal zones, transitional environments and continental shelf; ocean circulation, ocean-atmosphere interaction and climate variability; deep sea; and technology and infrastructure for marine research.
Engagement of Stakeholders
A clear understanding of stakeholders’ needs at a regional and local scale is fundamental to increase the use of ocean knowledge. The engagement of stakeholders (e.g., public managers, federal and state agencies, non-governmental entities, academia, industry, local communities) can be encouraged by the existence of associations formed by subnational entities, catalyzing the socioeconomic benefits of coastal observatories. The promotion of coastal observatories can generate comprehensive knowledge and understanding of the ocean along the Blue Amazon, providing qualified data, products, and services to support decision-making and address local socioeconomic needs. In addition, the promotion of public engagement and citizen science can raise awareness on the importance of long-term coastal observations for local communities’ livelihoods. Therefore, outreach and educational activities should be in the scope of the services provided by this integrated system. Brazil is coordinating the national efforts to benefit from the UN Decade implementation, led by the MCTI in the aforementioned “Science at Sea” program, but no concrete actions are still being tailored to address the alignment of the coastal observatories and secure budget in that regard. Thus, hopes are high that the Federal Government pursues the establishment of the INMAR as a way to create a “one-stop-shop” for ocean data and observing networks in the country. By providing the correct mechanism to allow better interaction among initiatives, such as the ones described in this paper, with a robust ocean infrastructure, Brazil would be taking a decisive step in coordinating the coastal observing efforts, so systems could become interoperable and data available for public use and open access. Understanding how different countries deal with similar issues will also be key and both the Belém Statement and the UN Ocean Decade could be beneficial for triggering national and subnational efforts in Brazil, but also serve as an opportunity to integrate Brazilian initiatives with those pertaining to international collaboration.
Data Availability Statement
The original contributions presented in the study are included in the article/supplementary materials, further inquiries can be directed to the corresponding author/s.
Author Contributions
GF provided the original outline, coordinated the writing, and assembled the final manuscript. CG (SiMCosta), JP, AMP, JL (REMO), LA, CB, RT (Project Azul), GF (BSO), LG (SCTGN), MR (OCA), LC (BrOA), CL (BROIL), PN (BESM), AT (Biodiversity and Ocean Decade) contributed different initiatives supported by all other authors. AT, SE, MC, JT-D, and AP contributed to the final overall outcome. All the authors contributed to the article and approved the submitted version.
Funding
BSO development was initially supported by the User Uptake program from the CMEMS and is currently funded by the Brazilian Navy and CNPq/MCTI call CNPq/MCTI 06/2020 – Research and Development for Coping with Oil Spills on the Brazilian Coast – Ciências do Mar Program, grant #440814/2020-0. SiMCosta is funded by MCTI and the MMA. Project AZUL was funded by Shell. The authors declare that Shell funded Project Azul, one of the initiatives described in this article, but the funder was not involved in the general preparation, writing, and submission of this article, because only a brief description is provided about the initiative not including any data or results. REMO was financially supported by PETROBRAS and ANP, and acknowledges the fellowship grant 446528/2014-5 provided by CNPq and the computational infrastructure provided by the MCTIC/FINEP/CT-Infra 01/2013 project 0761/13. LC acknowledges the Prociência/UERJ grant (2018/2021). The authors declare that PETROBRAS funded REMO, one of the initiatives described in this article, but the funder was not involved in the general preparation, writing, and submission of this article, because only a brief description is provided about the initiative not including any data or results. AP is supported by the Swedish Agency for Marine and Water Management – SWAM, and the German Ministry of Transport and Digital Infrastructure through the Land-to-Ocean Leadership Program and by MCTI. AT and MC would like to acknowledge CNPq for their productivity scholarship grants 310553/2019-9 and 310902/2018-5, respectively. BROIL is funded by the National Institute of Science and Technology – Petroleum Geophysics (INCT-GP) and MCTI/CNPq/CAPES/FAPS N° 16/2014 process 465517/2014-5, INCT PROGRAM (additive project entitled “Modeling, remote sensing, and preventive detection of oil/fuel accidents” by MCTI/CNPq/CAPES/FAPS 2019), and by the Brazilian Navy, CNPq, and MCTI call CNPq/MCTI 06/2020 – Research and Development for Coping with Oil Spills on the Brazilian Coast – Ciências do Mar Program, grant #440852/2020-0. MN would like to thank CAPES for the financial Support (Project Number 88881.145867/2017-01). FC was supported by the INTERREG Atlantic Area Project MyCOAST (EAPA 285/2016) co-funded by the ERDF (EU). This manuscript is built out of several separate contributions and was first idealized as an outcome of a roundtable performed in the workshop Coastal Observatories in Brazil and the World, an event promoted for the dissemination of the Brazilian Sea Observatory (BSO) initiative.
Conflict of Interest
HN is a managing partner of the company EnvEx Engineering. FS and JúP are managing partners and TM is employed by the company Prooceano. RM is employed by the company PETROBRAS.
The remaining authors declare that the research was conducted in the absence of any commercial or financial relationships that could be construed as a potential conflict of interest.
Publisher’s Note
All claims expressed in this article are solely those of the authors and do not necessarily represent those of their affiliated organizations, or those of the publisher, the editors and the reviewers. Any product that may be evaluated in this article, or claim that may be made by its manufacturer, is not guaranteed or endorsed by the publisher.
Acknowledgments
CG and EP acknowledge all the technical staff and students involved in the deployment of the SiMCosta buoys along the Brazilian coast. LG acknowledges all the institutions involved in supporting the implantation of the SC tide gauges along the SC coast. This manuscript is built out of several separate contributions and was first idealized as an outcome of a roundtable performed in the workshop Coastal Observatories in Brazil and the World, an event promoted for the dissemination of the Brazilian Sea Observatory (BSO) initiative.
Glossary
AIR Centre, Atlantic International: Research Centre; ANP, Brazilian National Agency of Petroleum, Natural Gas and Biofuels; BAM, INPE’s global atmospheric modeling; BESM, Brazilian Earth System Model; BMO-BR, Brazilian Meteo-Oceanographic buoy; BNDO, National Bank for Oceanographic Data; BPBES, Brazilian Platform on Biodiversity and Ecosystem Services; BrOA, Brazilian Network for Ocean Acidification Research; BROIL, Brazilian Oil research group; BSO, Brazilian Sea Observatory; CAPES, Coordination for the Improvement of Higher Education Personnel; CEM/UFPR, Centre for Marine Studies, Federal University of Paraná; CHM, Brazilian Navy Hydrography Centre; CIRM, Interministerial Commission for the Resources of the Sea; CMEMS, Copernicus Marine Environment Monitoring Service; CNPq, National Council for Scientific and Technological Development; ComTecPolOleo, Technical and Scientific Commission for the Advancement and Support to Monitoring activities and Neutralization of Impacts Arising from Marine Pollution by Oil and other Pollutants in the “Blue Amazon”; CPTEC, INPE’s Centre for Weather Prediction and Climate Studies; COPPE/UFRJ, Coordination of Postgraduate Engineering Programmes, Federal University of Rio de Janeiro; DHN, Directorate of Hydrography and Navigation; ECV, Essential Climate Variables; EMODNet, European Marine Observation and Data Network; EMSA, European Maritime Safety Agency; EnOI, Ensemble Optimal Interpolation scheme; EOOS, European Ocean Observing System; EPAGRI, Santa Catarina Agricultural Research and Outreach Company; ESA, European Space Agency; FAPESP, São Paulo Science and Technology Foundation; FINEP, Funding Agency for Studies and Projects - Brazilian Innovation Agency; GCOS, Global Climate Observing System; GEBCO, General Bathymetric Chart of the Oceans; GFS, Global Forecast System; GLOSS-Brazil, Brazilian component of the Global Sea Level Observing System; GODAE, Global Ocean Data Assimilation Experiment; GOOS, Global Ocean Observing System; GOOS Brazil, Brazilian GOOS node; HYCOM, Hybrid Coordinate Ocean Model; IBGE, Brazilian Institute of Geography and Statistics; ICMBio, Chico Mendes Institute for Biodiversity Conservation; INCT, National Institutes of Science, Technology and Innovation; INMAR, National Institute for the Sea; INPE, Brazilian National Institute for Space Research; IOC, Intergovernmental Oceanographic Commission; IODE, International Oceanographic Data and Information Exchange; IOOS, Integrated Ocean Observing System (US-GOOS); JERICO, Joint European Research Infrastructure Network for Coastal Observatories; LAMCE, Laboratory of Computational Methods in Engineering; LEPLAC, Brazilian Continental Shelf Survey Plan; MCTI, Ministry of Science, Technology, and Innovation; MMA, Ministry of Environment; OCA, Amazon Coastal Observatory; OCEATLAN, GOOS Regional Alliance to the South and Tropical Atlantic; PELD, Long-Term Ecological Research Program; PIRATA, Prediction and Research Moored Array in the Tropical Atlantic; PNBoia, National Buoy Program; PPGMAR, Brazilian Ocean Sciences Capacity Building Programme; PROOCEANO, Brazilian oceanographic technology company; PSRM, Sectorial Plan for the Resources of the Sea; QARTOD, Quality Assurance/Quality Control of Real Time Oceanographic Data; ReBentos, Brazilian Network for Monitoring Coastal Benthic Habitats; Rede CLIMA, Brazilian Network on Global Climate Change Research; REMO, Oceanographic Modeling and Observation Network; RIOSS, Radar Image Oil Spill Seeker; RODAS, REMO Ocean Data Assimilation System; ROMS, Regional Ocean Modeling System; SANEPAR, Paraná Water Company; SCTGN, Santa Catarina Tide Gauges Network; SDG, Sustainable Development Goals; SiBBr, Information System of the Brazilian Biodiversity; SiMCosta, Brazilian Coastal Monitoring System; Sisbiota, National Biodiversity Research System Program; SisGAAz, Monitoring System of the Blue Amazon; SNIRH, National Water Resources Information System; STI, Science, Technology and Innovation; SWOT, Sea Water and Ocean Topography Satellite; T-SIS, Tendral Statistical Interpolation System; UN, United Nations.
Footnotes
- ^ https://www.oceandecade.org/
- ^ https://sustainabledevelopment.un.org/
- ^ https://www.goosocean.org/
- ^ https://marine.copernicus.eu/
- ^ https://www.emodnet.eu/
- ^ http://eurogoos.eu/
- ^ http://www.eoos-ocean.eu/
- ^ https://www.jerico-ri.eu/
- ^ https://www.ioos.us/
- ^ http://www.ioosassociation.org/
- ^ http://oceatlan.org/
- ^ https://www.marinha.mil.br/secirm/psrm
- ^ https://www.marinha.mil.br/secirm/
- ^ https://www.marinha.mil.br/secirm/leplac
- ^ http://www.goosbrasil.org/
- ^ http://redeclima.ccst.inpe.br/
- ^ http://www.simcosta.furg.br/
- ^ www.rederemo.org
- ^ https://oceanpredict.org/
- ^ http://www.mar.mil.br/dhn/chm/meteo/prev/modelos/hycom-v.htm
- ^ http://www.projetoazul.eco.br/
- ^ oca.eco.br
- ^ https://ciram.epagri.sc.gov.br/litoral-online/
- ^ http://www.ioc-sealevelmonitoring.org/station.php?code=imbi
- ^ https://brazilianseaobservatory.org/
- ^ http://mohid.com/
- ^ https://www.gebco.net/
- ^ https://www.aviso.altimetry.fr/
- ^ https://www.ncdc.noaa.gov/
- ^ https://www.snirh.gov.br/
- ^ https://coastwatch.pfeg.noaa.gov/erddap/index.html
- ^ https://github.com/IrishMarineInstitute/awesome-erddap
- ^ www.broa.furg.br
- ^ www.projetocostanorte.eco.br
- ^ https://www.marinha.mil.br/secirm/psrm/ppgmar
- ^ https://www.ezute.org.br/en/sisgaaz-monitoring-system-of-the-blue-amazon/
- ^ https://www.marinha.mil.br/sites/default/files/relatorio-gestao/prog_e_proj_estrategicos_sisgaaz_arq6.pdf
- ^ http://www.emsa.europa.eu/
- ^ http://inct.cnpq.br/
- ^ http://www.baleiajubarte.org.br/projetoBaleiaJubarte/
- ^ http://golfinhorotador.org.br/
- ^ https://projetoalbatroz.org.br/sobre-o-projeto-albatroz/rede-biomar
- ^ http://www.projetotamar.org.br/
- ^ http://merosdobrasil.org/
- ^ http://coralvivo.org.br/
- ^ http://baleiafranca.org.br/
- ^ https://projetotoninhas.org.br/
- ^ http://www.vivaopeixeboimarinho.org/
- ^ https://www.pinipedesdosul.com.br/?
- ^ https://www.mantasdobrasil.org.br/
- ^ http://projetouca.org.br/
- ^ http://www.sinbiose.cnpq.br/web/sinbiose
- ^ http://www.sinbiose.cnpq.br/web/sinbiose/projetos-e-resultados
- ^ https://www.bpbes.net.br/
- ^ http://rebentos.org/index.php
- ^ https://www.scielo.br/j/bjoce/i/2016.v64nspe2/
- ^ https://obis.org/
- ^ https://www.sibbr.gov.br/
- ^ https://sinbiota.biota.org.br/
- ^ https://ec.europa.eu/research/iscp/pdf/belem_statement_2017_en.pdf
- ^ https://www.aircentre.org/
- ^ https://www.coastpredict.org/
References
Angelo, C. (2019). Brazil’s government freezes nearly half of its science spending. Nature 568, 155–156. doi: 10.1038/d41586-019-01079-9
Araujo, C. E. S., Boll, M. G., Garbossa, L. H. P., Vanz, A., and Candella, R. N. (2020). Atmospherically induced large amplitude sea-level oscillations on October 29, 2019 at Santa Catarina, Brazil. Nat. Hazards 106, 1–17. doi: 10.1007/s11069-020-04375-0
Arraut, E., Nobre, P., Nobre, C., and Scarpa, F. (2012). Brazilian Network on Global Climate Change Research (Rede CLIMA): structure, scientific advances and future prospects. Sustainab. Debate. 3, 241–256.
Artana, C., Provost, C., Lellouche, J.-M., Rio, M.-H., and Ferrari, N. (2019). The malvinas current at the confluence with the brazil current: inferences from 25 years of mercator ocean reanalysis. J. Geophys. Res. 124, 7178–7200. doi: 10.1029/2019JC015289
Ávila, R. A., Orozco, P. M. S., Andrade, M. M., and Möller, O. O. Jr. (2021). Temporal variability of suspended-solids concentration in the estuarine channel of patos lagoon, Southern Brazil. Water 13:646. doi: 10.3390/w13050646
Baia, E., Rollnic, M., and Venekey, V. (2021). Seasonality of pluviosity and saline intrusion drive meiofauna and nematodes on an Amazon freshwater-oligohaline beach. J. Sea Res. 170:102022. doi: 10.1016/j.seares.2021.102022
Bell, M. J., Schiller, A., Le Traon, P.-Y., Smith, N. R., Dombrowsky, E., and Wilmer-Becker, K. (2015). An introduction to GODAE oceanview. J. Oper. Oceanogr. 8, 2–11. doi: 10.1080/1755876X.2015.1022041
Bleck, R. (2002). An oceanic general circulation model framed in hybrid isopycnic-Cartesian coordinates. Ocean Model. 4, 55–88. doi: 10.1016/S1463-5003(01)00012-9
Borba, T. A. C., and Rollnic, M. (2016). Runoff quantification on Amazonian Estuary based on hydrodynamic model. J. Coastal Res. Spl. Issue 75, 43–47. doi: 10.2112/SI75-009.1
Bourlès, B., Araujo, M., McPhaden, M. J., Brandt, P., Foltz, G. R., Lumpkin, R., et al. (2019). PIRATA: A sustained observing system for tropical atlantic climate research and forecasting. Earth Space Sci. 6, 577–616. doi: 10.1029/2018EA000428
BSO. (2019). Final Report. Available Online at: https://brazilianseaobservatory.org/documents (Accessed February 08, 2021).
Caffrey, E. A., and Higley, K. A. (2013). Creation of a voxel phantom of the ICRP reference crab. J. Environ. Radioact. 120, 14–18. doi: 10.1016/j.jenvrad.2013.01.006
Calado, L., Da Silveira, I. C. A., Gangopadhyay, A., and De Castro, B. M. (2010). Eddy-induced upwelling off Cape São Tomé (22 S, Brazil). Continent. Shelf Res. 30, 1181–1188. doi: 10.1016/J.CSR.2010.03.007
Calado, L., Gangopadhyay, A., and Da Silveira, I. C. A. (2006). A parametric model for the Brazil Current meanders and eddies off southeastern Brazil. Geophys. Res. Lett. 33:2006GL026092. doi: 10.1029/2006GL026092
Calado, L., Gangopadhyay, A., and Da Silveira, I. C. A. (2008). Feature-oriented regional modeling and simulations (FORMS) for the western South Atlantic: Southeastern Brazil region. Ocean Model. 25, 48–64. doi: 10.1016/j.ocemod.2008.06.007
Campuzano, F., Brito, D., Juliano, M., Fernandes, R., de Pablo, H., and Neves, R. (2016). Coupling watersheds, estuaries and regional ocean through numerical modelling for Western Iberia: a novel methodology. Ocean Dyn. 66, 1745–1756. doi: 10.1007/s10236-016-1005-4
Capistrano, V. B., Nobre, P., Veiga, S. F., Tedeschi, R., Silva, J., Bottino, M., et al. (2020). Assessing the performance of climate change simulation results from BESM-OA2. 5 compared with a CMIP5 model ensemble. Geosci. Model Dev. 13, 2277–2296. doi: 10.5194/gmd-13-2277-2020
Carvalho, J. P. S., Costa, F. B., Mignac, D., and Tanajura, C. A. S. (2019). Assessing the extended-range predictability of the ocean model HYCOM with the REMO ocean data assimilation system (RODAS) in the South Atlantic. J. Oper. Oceanogr. 14, 13–23. doi: 10.1080/1755876X.2019.1606880
Casagrande, F., Nobre, P., de Souza, R. B., Marquez, A. L., Tourigny, E., Capistrano, V., et al. (2016). Arctic sea ice: Decadal simulations and future scenarios using BESM-OA. Atmospheric Climate Sci. 6:351. doi: 10.4236/acs.2016.62029
Ciotti, A., Garcia, C. A. E., and Jorge, D. F. (2010). Temporal and meridional variability of Satellite-estimates of surface chlorophyll concentration over the Brazilian continental shelf. Pan-Am. J. Aquat. Sci. 5, 236–253.
Claudet, J., Bopp, L., Cheung, W. W. L., Devillers, R., Escobar-Briones, E., Haugan, P., et al. (2019). A roadmap for using the UN decade of ocean science for sustainable development in support of science, policy, and action. One Earth 2, 34–42. doi: 10.1016/j.oneear.2019.10.012
Conceição, M. R., Mendonça, L. F., Lentini, C. A., Lima, A. T., Lopes, J. M., Vasconcelos, R. N., et al. (2021). SAR oil Spill detection system through random forest classifiers. Remote Sens. 13:2044. doi: 10.3390/rs13112044
Costa, V. S., Mill, G. N., Gabioux, M., Grossmann-Matheson, G. S., and Paiva, A. M. (2017). The recirculation of the intermediate western boundary current at the Tubarão Bight–Brazil. Deep Sea Res. Part I Oceanogr. Res. Papers 120:e001. doi: 10.1016/j.dsr.2016.12.001
Cotovicz, L. C., Vidal, L. O., de Rezende, C. E., Bernardes, M. C., Knoppers, B. A., Sobrinho, R. L., et al. (2020). Carbon dioxide sources and sinks in the delta of the Paraíba do Sul River (Southeastern Brazil) modulated by carbonate thermodynamics, gas exchange and ecosystem metabolism during estuarine mixing. Mar. Chem. 226:103869. doi: 10.1016/j.marchem.2020.103869
Dai, A., and Trenberth, K. E. (2002). Estimates of freshwater discharge from continents: Latitudinal and seasonal variations. J. Hydrometeorol. 3, 660–687. doi: 10.1175/1525-7541(2002)003<0660:eofdfc>2.0.co;2
Davidson, F., Alvera-Azcárate, A., Barth, A., Brassington, G. B., Chassignet, E. P., Clementi, E., et al. (2019). Synergies in operational oceanography: The intrinsic need for sustained ocean observations. Front. Mar. Sci. 6:450. doi: 10.3389/fmars.2019.00450
De Dominicis, M., Pinardi, N., Zodiatis, G., and Lardner, R. (2013a). MEDSLIK-II, a Lagrangian marine surface oil spill model for short-term forecasting–Part 1: Theory. Geosci. Model Dev. 6, 1851–1869. doi: 10.5194/gmd-6-1851-2013
De Dominicis, M., Pinardi, N., Zodiatis, G., and Archetti, R. (2013b). MEDSLIK-II, a Lagrangian marine surface oil spill model for short-term forecasting–Part 2: Numerical simulations and validations. Geosci. Model Dev. 6, 1871–1888. doi: 10.5194/gmd-6-1871-2013
de Freitas Assad, L. P., Toste, R., Böck, C. S., Nehme, D. M., Sancho, L., Soares, A. E., et al. (2020). Ocean climatology at Brazilian equatorial margin: a numerical approach. J. Comput. Sci. 44:101159. doi: 10.1016/j.jocs.2020.101159
de Young, B., Visbeck, M., Filho, M. C. A., Baringer, M. O., Black, C. A., Buch, E., et al. (2019). An integrated all-Atlantic ocean observing system in 2030. Front. Mar. Sci. 6:428. doi: 10.3389/fmars.2019.00428
Dominguez, J. M. (2009). “The coastal zone of Brazil,” in Geology and geomorphology of holocene coastal barriers of Brazil, eds S. R. Dilenburg and P. A. Hesp (Berlin: Springer-Verlag), 17–51. doi: 10.1007/978-3-540-44771-9_2
Doney, S. C., Fabry, V. J., Feely, R. A., and Kleypas, J. A. (2009). Ocean acidification: The other CO 2 problem. Ann. Ver. Mar. Sci. 1, 169–192. doi: 10.1146/annurev.marine.010908.163834
dos Santos, F. A., de Oliveira, A. L. S. C., Soares, F. L. M., Carvalho, G. V., São Tiago, P. M., Santos, N. G., et al. (2015). “Projeto Azul: operational oceanography in an active oil and gas area southeastern Brazil,” in Coastal Ocean Observing Systems, 1st Edn, eds Y. Liu, H. Kerkering, and R. H. Weisberg (London: Elsevier), 99–116.
EPAGRI. (2020). Banco de dados de variáveis ambientais de Santa Catarina. Available Online at: https://www.epagri.sc.gov.br/index.php/solucoes/publicacoes/documento (Accessed February 09, 20)
EuroGOOS-European Marine Board. (2016). Towards an end-to-end, Integrated and Sustained Ocean Observing System for Europe. Brussels: EuroGOOS European Marine Board.
Fernandes, R., Campuzano, F., Brito, D., Juliano, M., Braunschweig, F., and Neves, R. (2017). Automated system for near-real time prediction of oil spills from EU satellite-based detection service. Int. Oil Spill Confer. Proc. 2017, 1574–1593. doi: 10.7901/2169-3358-2017.1.1574
Foltz, G. R., Brandt, P., Richter, I., Rodriguez-Fonseca, B., Hernandez, F., Dengler, M., et al. (2019). The tropical Atlantic observing system. Front. Mar. Sci. 6:206. doi: 10.3389/fmars.2019.00206
Fragoso, M. R., Carvalho, G. V., Soares, F. L. M., Faller, D. G., Assad, L. P. F., Toste, R., et al. (2016). A 4D-variational ocean data assimilation application for Santos Basin, Brazil. Ocean Dyn. 66, 419–434. doi: 10.1007/s10236-016-0931-5
Franz, G. A. S., Leitão, P., Santos, A. D., Juliano, M., and Neves, R. (2016). From regional to local scale modelling on the south-eastern Brazilian shelf: case study of Paranaguá estuarine system. Braz. J. Oceanogr. 64, 277–294. doi: 10.1590/S1679-875920161195806403
Gabioux, M. G., da Costa, V. S., de Souza, J. M. A. C., de Oliveira, B. F., and de Moraes Paiva, A. (2013). Modeling the South Atlantic Ocean from medium to high resolution. Brazil. J. Geophys. 31:291. doi: 10.22564/rbgf.v31i2.291
Garbossa, L. H. P., Massignam, A. M. (2018). “Controle de qualificação de dados de monitoramento de maré de alta taxa,” in Anais do XI Encontro Nacional de Gerenciamento Costeiro (Encogerco), (Florianópolis: UFSC), 248–249.
GCOS. (2003). The Second Report on the Adequacy of the Global Observing System for Climate in Support of the UNFCCC. Report GCOS−82 (WMO/TD No.1143). Geneva, Switzerland: World Meteorological Organization.
Gerhardinger, L. C., Andrade, M. M., Correa, M. R., and Turra, A. (2020). Crafting a sustainability transition experiment for the brazilian blue economy. Mar. Policy 120:104157. doi: 10.1016/j.marpol.2020.104157
Gerhardinger, L. C., Gorris, P., Gonçalves, L. R., Herbst, D. F., Vila-Nova, D. A., De Carvalho, F. G., et al. (2018). Healing Brazil’s Blue Amazon: The role of knowledge networks in nurturing cross-scale transformations at the frontlines of ocean sustainability. Front. Mar. Sci. 4:395. doi: 10.3389/fmars.2017.00395
Haidvogel, D. B., Arango, H., Budgell, W. P., Cornuelle, B. D., Curchitser, E., Di Lorenzo, E., et al. (2008). Ocean forecasting in terrain-following coordinates: formulation and skill assessment of the Regional Ocean Modeling System. J. Comput. Phys. 227, 3595–3624. doi: 10.1016/j.jcp.2007.06.016
Halliwell, G. R. Jr., Srinivasan, A., Kourafalou, V., Yang, H., Willey, D., Le Hénaff, M., et al. (2014). Rigorous evaluation of a fraternal twin ocean OSSE system for the open Gulf of Mexico. J. Atmospheric Oceanic Technol. 31, 105–130. doi: 10.1175/JTECH-D-13-00011.1
ICRP. (2017). Dose coefficients for nonhuman biota environmentally exposed to radiation. Ann. ICRP 46, 1–136. doi: 10.1177/0146645317728022
IOC. (1998). Strategic Plan and Principles for the Global Ocean Observing System. IOC/INF-1091, Paris, 1998, GOOS Report No.41. Available Online at: https://www.goosocean.org/index.php?option=com_oe&task=viewDocumentRecord&docID=48 (Accessed June 11, 2021)
IOC. (2019). The Global Ocean Observing System 2030 Strategy. IOC, Paris, 2019, IOC Brochure 2019-5 (IOC/BRO/2019/5 rev.2), GOOS Report No.239. Available Online at: https://www.goosocean.org/index.php?option=com_oe&task=viewDocumentRecord&docID=24590 (Accessed February 09, 2021)
IOOS. (2018). U.S. IOOS Enterprise Strategic Plan (2018-2022). Available Online at: https://ioos.noaa.gov/about/governance-and-management/strategic-plan (Accessed February 09, 2021).
IPBES. (2019). Global assessment report on biodiversity and ecosystem services of the Intergovernmental Science-Policy Platform on Biodiversity and Ecosystem Services. Bonn, Germany: IPBES secretariat.
Jiang, L.-Q., Carter, B. R., Feely, R. A., Lauvset, S. K., and Olsen, A. (2019). Surface ocean pH and buffer capacity: past, present and future. Sci. Rep. 9:18624. doi: 10.1038/s41598-019-55039-4
Joly, C. A., Scarano, F. R., Turra, A., Bicudo, C. E. M., Young, C. E. F., Diniz-Filho, J. A. F., et al. (2018). “Biodiversidade em foco,” in Projeto de Ciência para o Brasil, 1ed Edn, eds J. L. Silva and J. G. Tundisi (Rio de Janeiro: Academia Brasileira de Ciências), 119–132.
Kerr, R., da Cunha, L. C., Kikuchi, R. K. P., Horta, P. A., Ito, R. G., Müller, M. N., et al. (2016). The Western South Atlantic Ocean in a high-CO2 World: current measurement capabilities and perspectives. Environ. Manage. 57, 740–752. doi: 10.1007/s00267-015-0630-x
Khalid, A., de Lima, A. D. S., Cassalho, F., Miesse, T., and Ferreira, C. (2020). Hydrodynamic and wave responses during storm surges on the Southern Brazilian coast: A real-time forecast system. Water 12:3397. doi: 10.3390/w12123397
Kourafalou, V. H., De Mey, P., Le Hénaff, M., Charria, G., Edwards, C. A., He, R., et al. (2015a). Coastal ocean forecasting: system integration and evaluation. J. Operat. Oceanogr. 8, s127–s146. doi: 10.1080/1755876X.2015.1022336
Kourafalou, V. H., De Mey, P., Staneva, J., Ayoub, N., Barth, A., Chao, Y., et al. (2015b). Coastal Ocean Forecasting: science foundation and user benefits. J. Operat. Oceanogr. 8, s147–s167. doi: 10.1080/1755876X.2015.1022348
Krelling, A. P., da Silveira, I. C., Polito, P. S., Gangopadhyay, A., Martins, R. P., Lima, J. A. M., et al. (2020a). A Newly Observed Quasi−stationary subsurface anticyclone of the North Brazil undercurrent at 4° S: The Potiguar Eddy. J. Geophys. Res. Oceans 125:e2020JC016268. doi: 10.1029/2020JC016268
Krelling, A. P., Gangopadhyay, A., da Silveira, I., and Vilela-Silva, F. (2020b). Development of a feature-oriented regional modelling system for the North Brazil Undercurrent region (1°–11° S) and its application to a process study on the genesis of the Potiguar Eddy. J. Operat. Oceanogr. 2020, 1–18. doi: 10.1080/1755876X.2020.1743049
Lacerda, L. D., Campos, E., Turra, A., Polejack, A., Muelbert, J., Mahiques, M. M., et al. (2018). “Ciências do Mar,” in Projeto de Ciência para o Brasil, 1ed Edn, eds J. L. Silva and J. G. Tundisi (Rio de Janeiro: Academia Brasileira de Ciências), 297–318.
Lana, P. C., and Bernardino, A. F. (2018). “Brazilian Estuaries: A Benthic Perspective,” in Brazilian Marine Biodiversity, ed. A. Turra (New York: Springer), 236.
Lima, J. A. M., Martins, R. P., Tanajura, C. A. S., Paiva, A. M., Cirano, M., Campos, E. J. D., et al. (2013). Design and implementation of the oceanographic modeling and observation network (REMO) for operational oceanography and ocean forecasting. Rev. Bras. Geofis. 31, 209–228.
Lindstrom, E., Gunn, J., Fischer, A., McCurdy, A., and Glover, L. K. (2012). A Framework for Ocean Observing. By the Task Team for an Integrated Framework for Sustained Ocean Observing. Paris, France: UNESCO.
Mackenzie, B., Celliers, L., Assad, L. P. F., Heymans, J., Rome, N., Thomas, J., et al. (2019). The role of stakeholders in creating societal value from coastal and ocean observations. Front. Mar. Sci. 6:137. doi: 10.3389/fmars.2019.00137
Magris, R. A., Costa, M. D., Ferreira, C. E., Vilar, C. C., Joyeux, J. C., Creed, J. C., et al. (2020). A blueprint for securing Brazil’s marine biodiversity and supporting the achievement of global conservation goals. Diver. Distr. 27, 198–215. doi: 10.1111/ddi.13183
Marotta, H., Peixoto, R. B., Fernandes, T., da, F., Oliveira, V. P., de Costa, R., et al. (2020). BIOMONITORAMENTO CONTÍNUO DE ÁGUAS DO PELD-BAÍA DE GUANABARA: O ENCONTRO DAS ESCALAS DE CURTO E LONGO PRAZOS NA VARIABILIDADE DAS ÁGUA TROPICAIS. Oecologia Aust. 24, 365–388. doi: 10.4257/oeco.2020.2402.10
Martín Míguez, B., Novellino, A., Vinci, M., Claus, S., Calewaert, J.-B., Vallius, H., et al. (2019). The european marine observation and data network (EMODnet): Visions and roles of the gateway to marine data in Europe. Front. Mar. Sci. 6:313. doi: 10.3389/fmars.2019.00313
Mascarenhas, A. C. C., Gomes, G. S., Lima, A. P. Y., Silva, H. K. N., Santana, L. S., Rosário, R. P., et al. (2016). Seasonal variations of the Amazon River Plume with focus on the Eastern Sector. J. Coastal Res. 75, 532–536. doi: 10.2112/SI75-107.1
Mateus, M., Riflet, G., Chambel, P., Fernandes, L., Fernandes, R., Juliano, M., et al. (2012). An operational model for the West Iberian coast: products and services. Ocean Sci. 8, 713–732. doi: 10.5194/os-8-713-2012
MCTI. (2021). Ordinance MCTI n. 4,719 of May 5, 2021. Available Online at: https://www.in.gov.br/web/dou/-/portaria-mcti-n-4.719-de-5-de-maio-de-2021-318198749
Melo, R. L., Freitas, A. C. N., Russo, L., Oliveira, J. F., Tanajura, C. A. S., and Alvarenga, J. B. R. (2013). Ocean forecasts in the southwestern Atlantic: Impact of different sources of sea surface height in data assimilation. Rev. Bras. Geofis. 31, 243–255. doi: 10.22564/rbgf.v31i2.292
Mignac, D., Tanajura, C. A. S., Santana, A., Lima, L. N., and Xie, J. (2015). Argo data assimilation into HYCOM with an EnOI method in the Atlantic Ocean. Ocean. Sci. 11, 195–213. doi: 10.5194/os-11-195-2015
Mikhailov, V. N. (2010). Water and sediment runoff at the Amazon River mouth. Water Resour. 37, 145–159. doi: 10.1134/S009780781002003X
Moore, A. M., Arango, H. G., Broquet, G., Powell, B. S., Weaver, A. T., and Zavala-Garay, J. (2011). The Regional Ocean Modeling System (ROMS) 4-dimensional variational data assimilation systems: Part I – System overview and formulation. Prog. Oceanogr. 91, 34–49. doi: 10.1016/j.pocean.2011.05.004
NOAA Research Council. (2015). NOAA Plan for Increasing Public Access to Research Results. Silver Spring, Maryland: NOAA Research Council.
Nobre, P., De Almeida, R. A., Malagutti, M., and Giarolla, E. (2012). Coupled ocean–atmosphere variations over the South Atlantic Ocean. J. Climate 25, 6349–6358. doi: 10.1175/JCLI-D-11-00444.1
Nobre, P., Siqueira, L. S., de Almeida, R. A., Malagutti, M., Giarolla, E., Castelão, G. P., et al. (2013). Climate simulation and change in the Brazilian climate model. J. Climate 26, 6716–6732.
Novaes, G. O., Monteiro, S. M., and Rollnic, M. (2020). Microplastics in the fluvio-estuarine beaches of Cotijuba Island, Pará River estuary (Brazil). J. Coastal Res. 95, 780–784. doi: 10.2112/SI95-152.1
Pelowitz, D. B. (2005). MCNPXTM User’s Manual, version 2.5.0. LA-CP-05e0369. Los Alamos National Laboratory.
Polejack, A. (2021). The importance of ocean science diplomacy for ocean affairs, global sustainability, and the UN decade of ocean science. Front. Mar. Sci. 8:664066. doi: 10.3389/fmars.2021.664066
Polejack, A., and Barros-Platiau, A. F. (2020). “A Ciência Oceânica como ferramenta de Cooperação e Diplomacia no Atlântico,” in Conservation of living resources in areas beyond national jurisdiction: BBNJ and Antarctica, eds A. F. Barros-Platiau and C. C. De Oliveira (Rio de Janeiro: Lumen Juris), 45–65.
Polejack, A., Gruber, S., and Wisz, M. S. (2021). Atlantic Ocean science diplomacy in action: the pole-to-pole All Atlantic Ocean Research Alliance. Hum. Soc. Sci. Commun. 8:52. doi: 10.1057/s41599-021-00729-6
Prestes, Y. O., Borba, T. A. C., Silva, A. C., and Rollnic, M. (2020). A discharge stationary model for the Pará-Amazon Estuarine system. J. Hydrol. Regional Stud. 28:100668. doi: 10.1016/j.ejrh.2020.100668
Prestes, Y. O., Silva, A. C., Rollnic, M., and Rosario, R. P. (2017). The M2 and M4 tides in the Pará river estuary. Tropical Oceanogr. 45, 26–37. doi: 10.5914/tropocean.v45i1.15198
Proctor, R., and Howarth, J. (2008). Coastal Observatories and operational oceanography: a European perspective. Mar. Technol. Soc. J. 42, 10–13. doi: 10.4031/002533208786842534
Rayner, R., Jolly, C., and Gouldman, C. C. (2019). Ocean observing and the blue economy. Front. Mar. Sci. 6:330. doi: 10.3389/fmars.2019.00330
Rollnic, M., and Rosário, R. P. (2013). Tide propagation in tidal courses of the Pará river estuary, Amazon Coast, Brazil. J. Coastal Res. Spl. 65, 1581–1586. doi: 10.2112/SI65-267.1
Ryabinin, V., Barbière, J., Haugan, P., Kullenberg, G., Smith, N., McLean, C., et al. (2019). The UN decade of ocean science for sustainable development. Front. Mar. Sci. 6:470. doi: 10.3389/fmars.2019.00470
Salpin, C., Onwuasoanya, V., Bourrel, M., and Swaddling, A. (2018). Marine scientific research in Pacific Small Island Developing States. Mar. Policy 95, 363–371. doi: 10.1016/j.marpol.2016.07.019
Santana, R., Costa, F. B., Mignac, D., Santana, A. N., Vidal, V. R. S., Zhu, J., et al. (2020). Model sensitivity experiments on data assimilation, downscaling and tides for the representation of the cape são tomé eddies. Ocean Dyn. 70, 77–94. doi: 10.1007/s10236-019-01307-w
Shchepetkin, A. F., and McWilliams, J. C. (2003). A method for computing horizontal pressure−gradient force in an oceanic model with a nonaligned vertical coordinate. J. Geophys. Res. Oceans 108, 35–41.
Shchepetkin, A. F., and McWilliams, J. C. (2005). The regional ocean modeling system: A split-explicit, free-surface, topography following coordinates ocean model. Ocean Model. 9, 347–404. doi: 10.1016/j.ocemod.2004.08.002
Shorten, C., and Khoshgoftaar, T. M. (2019). A survey on image data augmentation for deep learning. J. Big Data 6, 1–48.
Silva, G. S. D. M., and Garcia, C. A. E. (2021). Evaluation of ocean chlorophyll-a remote sensing algorithms using in situ fluorescence data in Southern Brazilian Coastal Waters. Ocean Coastal Res. 69:19. doi: 10.1590/2675-2824069.20-014gsdms
Simons, R. A. (2020). ERDDAP. Available Online at: https://coastwatch.pfeg.noaa.gov/erddap. Monterey, CA: NOAA/NMFS/SWFSC/ERD.
Snowden, J., Hernandez, D., Quintrell, J., Harper, A., Morrison, R., Morell, J., et al. (2019). The US integrated ocean observing system: governance milestones and lessons from two decades of growth. Front. Mari. Sci. 6:242. doi: 10.3389/fmars.2019.00242
Soutelino, R. G., Gangopadhyay, A., and Da Silveira, I. C. A. (2013). The roles of vertical shear and topography on the eddy formation near the site of origin of the Brazil Current. Continental Shelf Res. 70, 46–60. doi: 10.1016/J.CSR.2013.10.001
Souza-Filho, P. W., Lessa, G. C., Cohen, M. C., Costa, F. R., and Lara, R. J. (2009). “The subsiding macrotidal barrier estuarine system of the eastern Amazon coast, Northern Brazil,” in Geology and geomorphology of Holocene coastal barriers of Brazil, eds S. R. Dilenburg and P. A. Hesp (Berlin: Springer-Verlag), 347–375. doi: 10.1007/978-3-540-44771-9_11
Souza-Filho, P. W. M. (2005). Costa de manguezais de macromaré da Amazônia: cenários morfológicos, mapeamento e quantificação de áreas usando dados de sensores remotos. Revista Brasileira de Geofísica. 23, 427–435. doi: 10.1590/s0102-261x2005000400006
Sparnocchia, S., Nair, R., Petihakis, G., Aydoğdu, A., Dobricic, S., Farcy, P., et al. (2016). An interlinked coastal observatory network for Europe. J. Operat. Oceanogr. 9, s193–s201. doi: 10.1080/1755876X.2015.1114808
Stuchtey, M. R., Vincent, A., Merkl, A., Bucher, M., Haugan, P. M., Lubchenco, J., et al. (2020). Ocean Solutions That Benefit People, Nature and the Economy. Washington, DC: World Resources Institute.
Sumida, P. Y. G., Bernardino, A. G., and De Léo, F. C. (2020). Brazilian Deep-Sea Biodiversity. Cham: Springer, 261.
Tanajura, C. A. S., Mignac, D., Santana, A. N., Costa, F. B., Lima, L. N., Belayev, K. P., et al. (2020). Observing system experiments over the Atlantic Ocean with thE REMO ocean data assimilation system (RODAS) into HYCOM. Ocean Dyn. 70, 115–138. doi: 10.1007/s10236-019-01309-8
Tanajura, C. A. S., Santana, A., Mignac, D., Lima, L., Belyaev, K., and Ji-Ping, X. (2014). The REMO ocean data assimilation system into HYCOM (RODAS_H): General description and preliminary results. J. Atmos. Oceanic Sci. Lett. 7, 464–470. doi: 10.3878/j.issn.1674-2834.14.0011
Tanhua, T., McCurdy, A., Fischer, A., Appeltans, W., Bax, N., Currie, K., et al. (2019). What we have learned from the framework for ocean observing: Evolution of the global ocean observing system. Front. Mar. Sci. 6:471. doi: 10.3389/fmars.2019.00471
Toste, R., Soares, A. E., Nehme, D. M., Sancho, L., Böck, C. S., Assad, L. P., et al. (2020). 4DVAR data assimilation in the Brazilian equatorial margin-costa Norte Project. J. Coastal Res. 95, 1326–1332. doi: 10.2112/si95-255.1
Turra, A., Cróquer, A., Carranza, A., Mansilla, A., Areces, A. J., Werlinger, C., (2013). Global environmental changes: setting priorities for Latin American coastal habitats. Glob. Change Biol. 19, 1965–1969. doi: 10.1111/gcb.12186
Turra, A., and Denadai, M. R. (2015). Protocolos para o Monitoramento de Habitats Bentônicos Costeiros - Rede de Monitoramento de Habitats Bentônicos Costeiros - ReBentos. São Paulo: Instituto Oceanográfico da Universidade de São Paulo.
Turra, A., and Denadai, M. R. (2016). Linking biodiversity and global environmental changes in Brazilian coastal habitats. Brazil. J. Oceanogr. 64, 3–4. doi: 10.1590/S1679-87592016064sp2ed
U.S. Integrated Ocean Observing System. (2020). Manual for the Use of Real-Time Oceanographic Data Quality Control Flags, Version 1.2. Silver Spring, MD: National Oceanic and Atmospheric Administration, National Ocean Service, Integrated Ocean Observing System, 24. doi: 10.25923/w8y6
Ulanovsky, A., and Pröhl, G. A. (2006). Practical method for assessment of dose conversion coefficients for aquatic biota. Radiat. Environ. Biophys. 45, 203–214. doi: 10.1007/s00411-006-0061-4
Valentim, M. M., Monteiro, S. M., and Rollnic, M. (2018). The influence of seasonality on haline zones in an Amazonian Estuary. J. Coastal Res. Spl. 85, 76–80. doi: 10.2112/SI85-016.1
Veiga, S. F., Nobre, P., Giarolla, E., Capistrano, V., Baptista, M. Jr., Marquez, A. L., et al. (2019). The Brazilian Earth System Model Ocean–Atmosphere (BESM-OA) version 2.5: evaluation of its CMIP5 historical simulation. Geosci. Model Dev. 12, 1613–1642. doi: 10.5194/gmd-12-1613-2019
Virdin, J., Vegh, T., Jouffray, J.-B., Blasiak, R., Mason, S., Österblom, H., et al. (2021). The Ocean 100: Transnational corporations in the ocean economy. Sci. Adv. 7:eabc8041. doi: 10.1126/sciadv.abc8041
Keywords: coastal observatories, ocean observations, operational oceanography, Blue Amazon, blue economy, United Nations Decade of Ocean Science for Sustainable Development
Citation: Franz G, Garcia CAE, Pereira J, de Freitas Assad LP, Rollnic M, Garbossa LHP, da Cunha LC, Lentini CAD, Nobre P, Turra A, Trotte-Duhá JR, Cirano M, Estefen SF, Lima JAM, Paiva AM, Noernberg MA, Tanajura CAS, Moutinho JL, Campuzano F, Pereira ES, Lima AC, Mendonça LFF, Nocko H, Machado L, Alvarenga JBR, Martins RP, Böck CS, Toste R, Landau L, Miranda T, dos Santos F, Pellegrini J, Juliano M, Neves R and Polejack A (2021) Coastal Ocean Observing and Modeling Systems in Brazil: Initiatives and Future Perspectives. Front. Mar. Sci. 8:681619. doi: 10.3389/fmars.2021.681619
Received: 16 March 2021; Accepted: 08 July 2021;
Published: 10 August 2021.
Edited by:
Fei Chai, Second Institute of Oceanography, Ministry of Natural Resources, ChinaReviewed by:
Oscar Schofield, Rutgers, The State University of New Jersey, United StatesAvijit Gangopadhyay, University of Massachusetts Dartmouth, United States
Yuwu Jiang, Xiamen University, China
Tarron Lamont, Department of Environment, Forestry and Fisheries (DEFF), South Africa
Copyright © 2021 Franz, Garcia, Pereira, de Freitas Assad, Rollnic, Garbossa, da Cunha, Lentini, Nobre, Turra, Trotte-Duhá, Cirano, Estefen, Lima, Paiva, Noernberg, Tanajura, Moutinho, Campuzano, Pereira, Lima, Mendonça, Nocko, Machado, Alvarenga, Martins, Böck, Toste, Landau, Miranda, dos Santos, Pellegrini, Juliano, Neves and Polejack. This is an open-access article distributed under the terms of the Creative Commons Attribution License (CC BY). The use, distribution or reproduction in other forums is permitted, provided the original author(s) and the copyright owner(s) are credited and that the original publication in this journal is cited, in accordance with accepted academic practice. No use, distribution or reproduction is permitted which does not comply with these terms.
*Correspondence: Guilherme Franz, Z3VpbGhlcm1lLmZyYW56QHVmcHIuYnI=; Z3VpbGhlcm1lLmZyYW56QHRlY25pY28udWxpc2JvYS5wdA==