- 1AZTI, Marine Research, Basque Research and Technology Alliance, Pasaia, Spain
- 2Scottish Association for Marine Science, Scottish Marine Institute, Oban, United Kingdom
- 3French Research Institute for Exploitation of the Sea, Ifremer DYNECO PELAGOS, Plouzané, France
- 4Marine Institute, Galway, Ireland
- 5Environment Agency, Chief Scientist’s Group, Bristol, United Kingdom
- 6Plymouth Marine Laboratory, Plymouth, United Kingdom
- 7Laboratorio de Control de Calidad de los Recursos Pesqueros, Agencia de Gestión Agraria y Pesquera de Andalucía, Consejería de Agricultura, Ganadería, Pesca y Desarrollo Sostenible, Junta de Andalucía, Cartaya, Spain
- 8Phytoplankton Laboratory, Division of Oceanography and Marine Environment, Instituto Português do Mar e da Atmosfera, Lisbon, Portugal
- 9MARETEC – Marine, Environment and Technology Centre, LARSyS, Instituto Superior Técnico, Universidade de Lisboa, Lisbon, Portugal
- 10Centro Oceanográfico de A Coruña, Instituto Español de Oceanografía (IEO,CSIC), A Coruña, Spain
Across the European Atlantic Arc (Scotland, Ireland, England, France, Spain, and Portugal) the shellfish aquaculture industry is dominated by the production of mussels, followed by oysters and clams. A range of spatially and temporally variable harmful algal bloom species (HABs) impact the industry through their production of biotoxins that accumulate and concentrate in shellfish flesh, which negatively impact the health of consumers through consumption. Regulatory monitoring of harmful cells in the water column and toxin concentrations within shellfish flesh are currently the main means of warning of elevated toxin events in bivalves, with harvesting being suspended when toxicity is elevated above EU regulatory limits. However, while such an approach is generally successful in safeguarding human health, it does not provide the early warning that is needed to support business planning and harvesting by the aquaculture industry. To address this issue, a proliferation of web portals have been developed to make monitoring data widely accessible. These systems are now transitioning from “nowcasts” to operational Early Warning Systems (EWS) to better mitigate against HAB-generated harmful effects. To achieve this, EWS are incorporating a range of environmental data parameters and developing varied forecasting approaches. For example, EWS are increasingly utilizing satellite data and the results of oceanographic modeling to identify and predict the behavior of HABs. Modeling demonstrates that some HABs can be advected significant distances before impacting aquaculture sites. Traffic light indices are being developed to provide users with an easily interpreted assessment of HAB and biotoxin risk, and expert interpretation of these multiple data streams is being used to assess risk into the future. Proof-of-concept EWS are being developed to combine model information with in situ data, in some cases using machine learning-based approaches. This article: (1) reviews HAB and biotoxin issues relevant to shellfish aquaculture in the European Atlantic Arc (Scotland, Ireland, England, France, Spain, and Portugal; (2) evaluates the current status of HAB events and EWS in the region; and (3) evaluates the potential of further improving these EWS though multi-disciplinary approaches combining heterogeneous sources of information.
Introduction
Phytoplankton provide key ecosystem services to humans by providing food for marine life, oxygen and sequestering CO2 (Billett et al., 1983; Smetacek, 1999; Irigoien et al., 2004). However, some naturally occurring phytoplankton species can produce a range of marine biotoxins that accumulate in shellfish tissues through filter feeding (Vale et al., 2008; Wang, 2008). Thus, blooms of these harmful algal species (HABs) can negatively impact fisheries and aquaculture (Smayda, 1990; Berdalet et al., 2016; Sanseverino et al., 2016; FAO, 2018). The risk of intoxication causes significant economic losses for the aquaculture industry due to the temporary suspension of harvesting when toxin concentrations exceed the permissible regulatory limits as laid down in regulation EU853/2004 (Rodríguez-Rodríguez et al., 2011; Mardones et al., 2020; Martino et al., 2020). These temporary suspensions in harvesting cannot be prevented, but Early Warning Systems (EWS), such as bulletins issued to local harvesters to warn them of upcoming HAB events, aim to forecast their occurrence and reduce their socio-economic impact. Existing EWS have focused on some of the mechanisms/conditions that trigger the onset of blooms (e.g., nutrient availability) (Sverdrup, 1953), their transport (Farrell et al., 2012; Fehling et al., 2012; Whyte et al., 2014), and the conditions that favor the different functional groups of phytoplankton (Glibert et al., 2014; Wyatt, 2014). However, an EWS framework requires refinement to forecast the occurrence of harmful/toxic species affecting these regions. Predicting which species from a functional group will dominate, and where and when they will bloom presents major challenges (Huisman and Weissing, 1999).
The North-eastern Atlantic (NEA) region is heterogeneous in terms of which blooms are problematic and their associated triggering conditions. For example, upwelling conditions along the Spanish and Portuguese coasts can cause the rapid onset of HAB events that impact local shellfish harvesting sites (Díaz et al., 2016). Stratified (Raine, 2014), mixed (Gowen et al., 2012), and frontal water masses (Simpson et al., 1979) all exist within the Atlantic areas providing contrasting conditions for HAB development (Rathaille and Raine, 2011; Berdalet et al., 2017; Paterson et al., 2017). Early instances of EWS have focused mainly on detecting blooms, sometimes without considering whether the algae are actually producing toxins or how the bio-accumulation of toxins within shellfish tissues may result in harmful impacts at low cell densities (Hallegraeff, 2003; Davidson et al., 2011).
An improved ability to forecast HAB events would result in a significant benefit for the aquaculture industry. The potential to develop early warning systems has for a long time been a goal of HAB science, with early attempts to achieve this in European waters being discussed by Maguire et al. (2016). The EU shellfish hygiene directives require Atlantic Area countries to operate monitoring programs for the presence of harmful algae and shellfish biotoxins to ensure shellfish safety. In practice, monitoring methodology varies by country (Anderson, 1998) and is designed to protect human health rather than the economic viability of the aquaculture industry. However, in all cases these programs provide a “now-cast” upon which more sophisticated remote sensing, modeling or expert interpretation-based risk assessment or forecast systems can be built (Davidson et al., 2016).
Shellfish production is an important industry across the European Atlantic Arc, providing both healthy food and important economic support for remote family and small businesses in rural coastal areas (Munro and Wallace, 2018; Mardones et al., 2020). The distribution and effects of HABs in combination with other environmental and economic threats has reduced European shellfish production over the last two decades (Avdelas et al., 2021). HABs are sometimes associated with large-scale marine fish mortality events but are more frequently associated with various types of shellfish poisoning in humans (Sanseverino et al., 2016; Bresnan et al., 2021). The significant economic impact of shellfish biotoxins are reviewed by Mardones et al. (2020), with Martino et al. (2020) demonstrating that the harmful dinoflagellate Dinophysis and its toxins alone reduce shellfish production in Scotland by 15% per annum. Shellfish producers are not allowed to harvest and sell their product until it is deemed fit for human consumption. This can result in significant economic losses for the farmers (Rodríguez-Rodríguez et al., 2011; LeBihan et al., 2019; Theodorou et al., 2020; Karlson et al., 2021).
In order to address the challenges and potential benefits of HAB and biotoxin EWS we must first understand the shellfish aquaculture industry in this region. Scotland, England, Ireland, France, Portugal, and Spain are the main shellfish aquaculture producers (FAO, 2019) in the NEA regions (Figure 1). Pacific oysters (Crassostrea gigas) and blue mussels (Atlantic Mytilus edulis and Mediterranean Mytilus galloprovincialis) are the dominant shellfish aquaculture species in terms of production volume, although other species have high economic value such as native oysters (Ostrea edulis), and scallops (Aequipecten opercularis and Pecten maximus). As the latitude decreases other bivalve species start to gain greater importance such as Spisula spp. and Ensis spp. in Ireland or Ruditapes philippinarum in France. Clams and cockles dominate in southern countries of the NEA arc (Portugal and South Spain) with a high diversity of species (Ruditapes spp., Chamelea gallina, Donax trunculus, Callista chione, and Acanthocardia tuberculata).
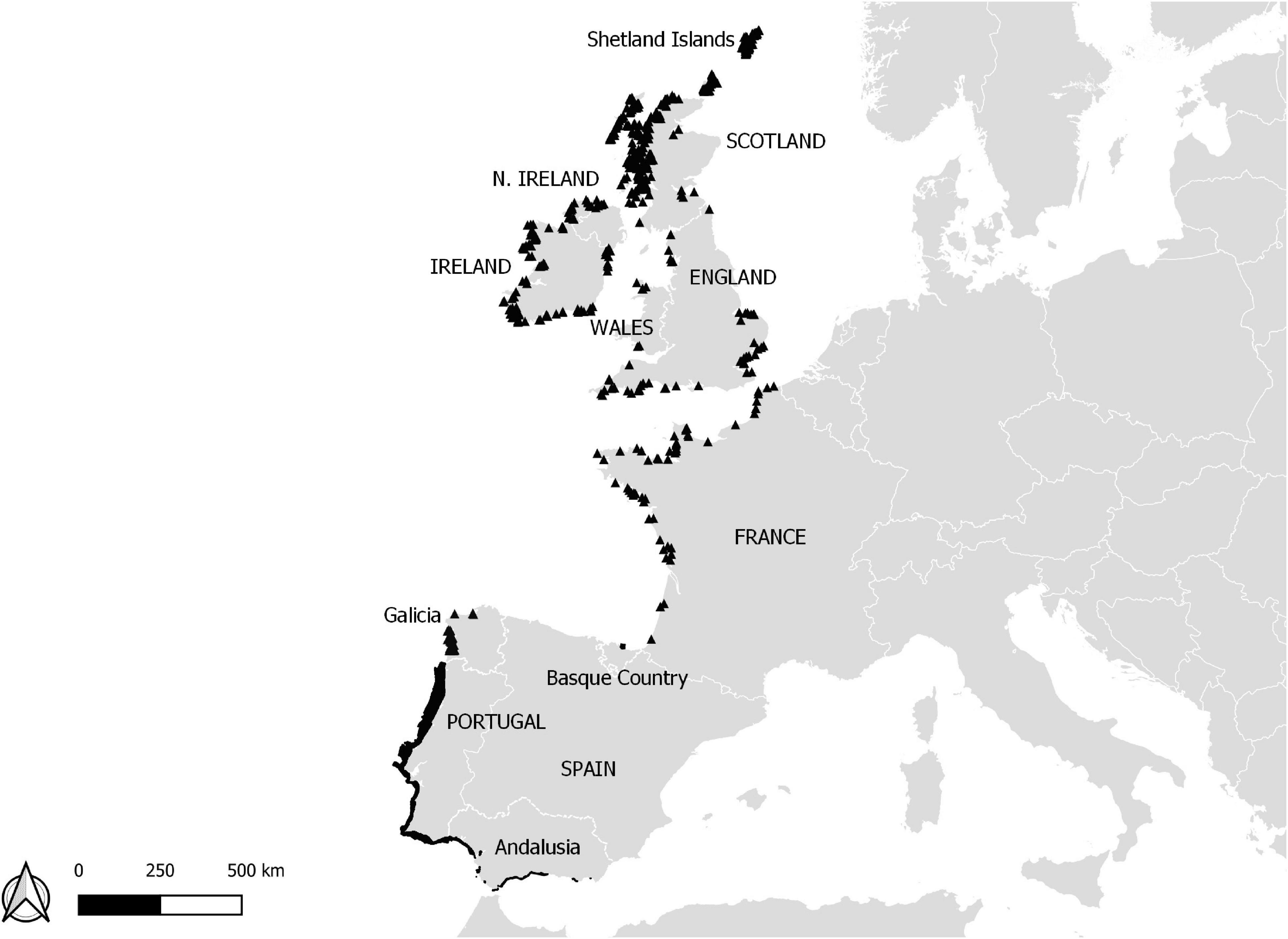
Figure 1. Spread of shellfish aquaculture production in Atlantic areas of Scotland, Ireland (North Ireland not included), England, France, Spain, and Portugal. The data providers are: Marine Institute in Ireland, Ifremer (from Euroshell project, 2013) in France and England the Scottish Government, Marine Environment and Technology Centre in Portugal, Junta de Andalucía in Andalusia, and AZTI in the Basque Country. Countries names are highlighted in capital letters, whereas regions are not.
Scottish production primarily occurs in the fjordic sea lochs that are typical of its west coast and islands and is dominated by mussels followed by Pacific oysters (Munro and Wallace, 2018). The value of the industry in 2017 was estimated at £12.4 million, with Atlantic mussels contributing £10.1m (8,232 tons) and Pacific oysters £2.0m. Production is regionally variable, and the northerly Shetland Islands accounted for ∼80% of the total. In England and Wales cultured shellfish species are primarily mussels and Pacific and native oysters (Hambrey and Evans, 2016; Defra, 2019). Besides these, wild species Queen scallop (Aequipecten opercularis) and Scallop (Pecten maximus) are harvested by dredging. Mussels and Pacific oysters dominate English production, and mussels in Wales (Ellis et al., 2015). In 2020 the Irish shellfish aquaculture industry was worth an estimated total of €51 million with an output of 24,000 tons. There is diverse variety of marine bivalve molluscan species produced from 97 production areas around the coast. mainly mussels, worth €11 million through both rope longline (10,300 tons) and bottom cultivation (4,400 tons) as well as Pacific and native oysters worth €37 million, with a smaller production of clam species (Spisula solida/Venerupis spp.). Some areas along the east and west coasts concentrate on dredging for razor clams (Ensis siliqua/magnus). Dredging of scallops occurs mainly on the southwest and west coasts, however, a large number dredged from the United Kingdom and French coastlines are landed in Ireland. In France, shellfish farming is mainly represented by Pacific oysters and mussels, although scallop and Manila clam Tapes philippinarum from the Atlantic coastline also contribute significantly to the overall production. On the Basque Coast (Spain), at the south-eastern part of the Bay of Biscay mussel farming is in its infancy. In contrast, Galicia is an area of intensive mussel (Mytilus galloprovincialis) aquaculture, approx. 250,000T per year. More than 90% of all mussels in Spain are produced in Galicia on 3,350 rafts operated by 2,300 families (estimates from 2012). Galician farmers harvest from natural beds and cultured bivalves (clams, cockles, scallops). The socio-economic importance of these activities in Galicia is very high. In Andalusia, shellfish are mainly harvested from the wild, principally clams, and cockles (Chamelea gallina, Donax trunculus, Callista chione, and Acanthocardia tuberculata). Aquaculture production focuses on mussels (Mytilus galloprovincialis), oysters (Crassostrea gigas and Crassostrea angulata), and some clam species (Ruditapes decussatus and Ruditapes philippinarum) and is undertaken in the marshes of large rivers and estuaries. Since 2000, mussel aquaculture has been undertaken in the open sea.
In this article, we first discuss the regional occurrence of toxin-producing HABs and their environmental drivers. We then consider early warning approaches to best mitigate HAB impacts for both producers and consumers of shellfish in the NEA countries, noting that the EWS must adapt to regional heterogeneity in the ecology of HABs and the structure of the aquaculture industry.
Monitoring of Marine Biotoxin Occurrence and Causative Organisms
Regulations to Avoid Human Poisoning
There are several EU food safety legislative requirements laid down for the production, harvesting and analysis for contaminants of live bivalve molluscs from classified production areas for human consumption. Specifically, EU regulations (853/2004, 854/2004, and 627/2019) specify the maximum permissible regulatory limits for the presence of marine biotoxins (Table 1). In compliance with the regulations, EU member states monitor for the presence and quantify the concentration of marine biotoxins in shellfish from classified production areas. These areas have specific sampling sites known as “representative monitoring points” (RMPs) which are routinely sampled all year round (weekly to monthly). The monitoring frequency may be increased: following the onset of, and throughout a toxic event; during identified high-risk periods; during the presence of causative toxigenic phytoplankton species in the water column; or during the occurrence of biotoxins in adjacent shellfish areas. When the regulatory marine biotoxin concentrations are exceeded in a particular shellfish species, this results in a mandatory closure for the harvesting of the affected shellfish species in the area.
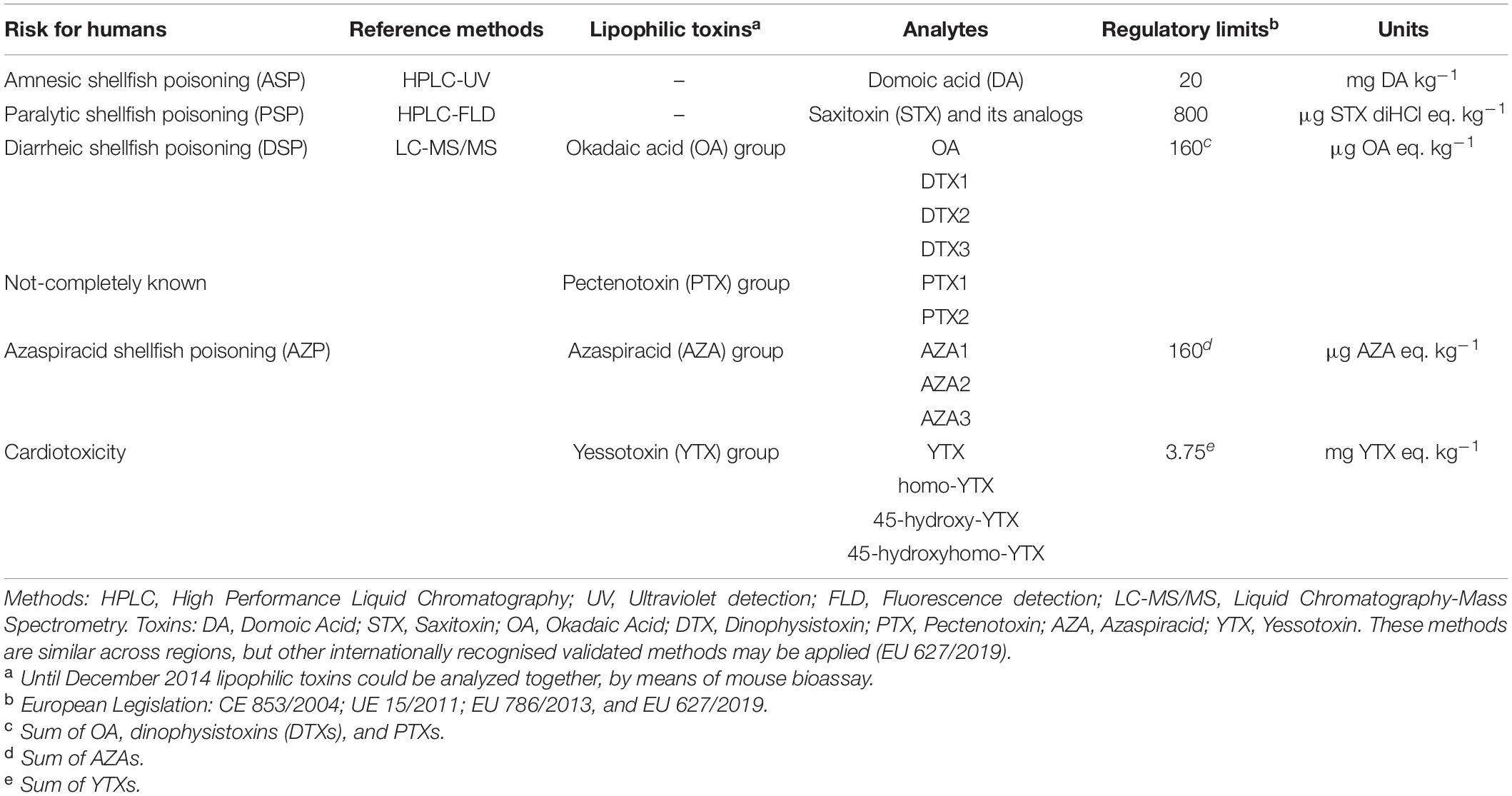
Table 1. Summary of toxins typically analyzed in Europe with the analytical methods and the regulatory limits (maximum quantities allowed in bivalve molluscs placed on the market).
According to Regulation (EC) No 854/2004 (Annex II, Chapter II, B, 7) and Articles 59 and 61 in EC 627/2019, plankton samples for regulatory monitoring purposes are to be representative of the water column and to provide information on the presence of toxic species as well as population trends. For shallow stations (depth <5 m), a water sample taken using a bucket, oceanographic bottle or a pole sampler is considered suitable for quantification purposes. Sampling should avoid the disturbance of the bottom sediment. In locations where depths are >5 m depth sampling should be integrated by means of a hose (Lindahl, 1986), which is considered more suitable for species that present heterogeneous vertical distribution (e.g., Raine, 2014). Net sampling (10–20 μm) can be used to take an integrated sample of the water column, pulling it from the bottom up to the surface. The methodology for hose and bottle sampling, and net hauls is described in the European Standard EN 15972:2011. For identification and quantification of toxin-producing phytoplankton, there is no specific method required by EU legislation, although an EU NRL best practice guide is due to be published soon1. Post collection phytoplankton are enumerated by microscopy, with inverted microscopy after sedimentation of fixed samples (Utermöhl, 1958) being the widely used standard. The Utermöhl procedure is described in the European Standard En 15204, 2006. Monitoring toxigenic phytoplankton potentially provides an indication and early insight of the potential subsequent onset of toxification of shellfish above regulatory levels (Davidson et al., 2016; Maguire et al., 2016; Botelho et al., 2019). The abundance of harmful phytoplankton generally follows a seasonal cycle with the greatest abundance in spring and summer, and to a lesser extent in autumn months (Coates et al., 2018).
Main Groups of Poisoning Syndromes and Associated Toxins
Recently, Bresnan et al., 2021 used the IOC-ICES-PICES Harmful Algal Event Database (HAEDAT) to describe the diversity of harmful algal events along the Atlantic margin of Europe from 1987 to 2018 (Figure 2). Closures of shellfish production areas are mainly due to the regular annual occurrence of Diarrhetic Shellfish Toxins (DSTs) with protracted closure periods typical over the summer months. Paralytic Shellfish Toxins (PSTs) and Amnesic Shellfish Toxins (ASTs) occur less frequently and usually result in shorter closure periods. The occurrence of Azaspiracid toxins (AZA’s), in the arc area, although observed from the Southwest along the West and through to the Northwest coast of Ireland, is not a regular occurrence in the region. The length of closure periods associated with AZAs is also extremely variable. Most events recorded were due to DSTs produced by species of the Dinophysis acuminata complex and Dinophysis acuta. Their seasonal patterns have been thoroughly described in European Atlantic waters (e.g., Reguera et al., 2012; Batifoulier et al., 2013; Fernández et al., 2019; Salas and Clarke, 2019). However, there is strong interannual variability in the timing and duration of shellfishery closures, which can respond to physical factors such as temperature, stratification and water circulation. Therefore, four main poisoning syndromes (DSP, AZ, ASP, and PSP) and group of associated toxins (DST, AZA, AST, and PST) have been identified as most problematic to the shellfish industry in the European Atlantic Arc countries (Figure 2). These are introduced in the following paragraphs in order from the greatest to least concern. Table 2 shows a more comprehensive summary of syndromes and associated causative species; for an exhaustive review see Bresnan et al. (2021).
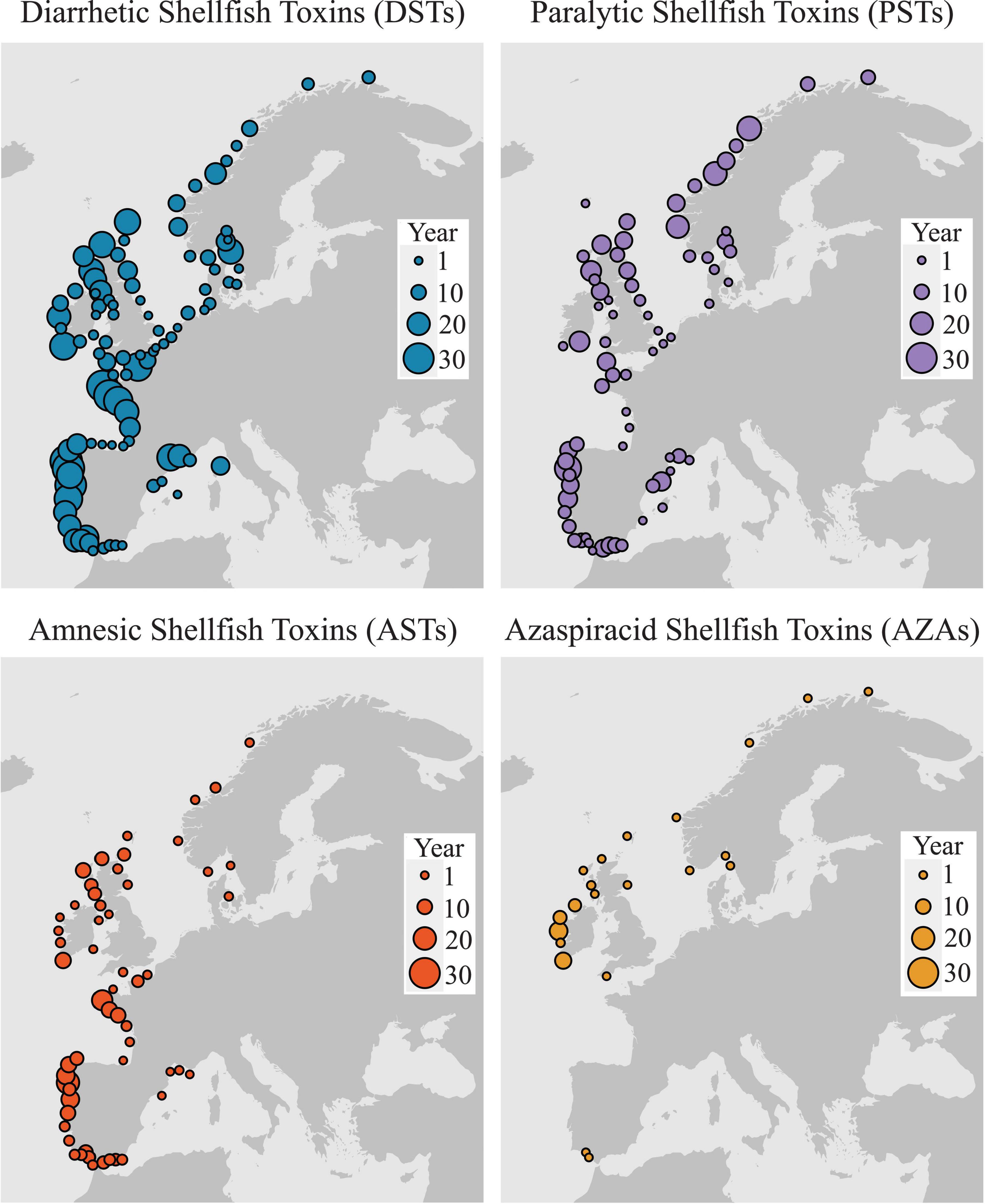
Figure 2. Number of years where events associated with harmful algae have been recorded in IOC-ICES-PICES HAEDAT database. The majority of events are management actions closing shellfish harvesting areas to protect human health.
DSP – Diarrhetic Shellfish Poisoning; DST – Diarrhetic Shellfish Toxins: Dinophysis spp. are common in European waters where cell densities are highest in summer and generally absent or below the detection threshold in the winter months (November to February). Blooms are thought to mainly develop in open waters, possibly at frontal regions and then be advected to coastal aquaculture sites (Delmas et al., 1993; Batifoulier et al., 2013; Whyte et al., 2014; Raine et al., 2016). In Scotland, early season blooms are typically related to D. acuminata, whereas occasional late summer blooms are related to D. acuta (Swan et al., 2018). Recently Paterson et al. (2017) demonstrated the potential for a frontal region to limit the transport of a D. acuta bloom, preventing it from significantly impacting aquaculture sites in Loch Fyne. In Ireland, D. acuminata starts to appear from late May, and usually peaks in late June–July. Similar to Scotland D. acuta starts to appear in Irish waters from June/July onward and tends to peak later in the summer during August (Salas and Clarke, 2019) and can result in prolonged closure periods for mussel harvesting. Additional events can occur in September/October with occasional winter closures as observed in 2012–2013 (Clarke, 2020). In France, Dinophysis spp. are also responsible for the highest number of closure periods, the main causative species being D. acuminata, Dinophysis sacculus, D. acuta, and Dinophysis caudata. Dinophysis blooms occur from April to October, but start later in the English Channel when compared- to the south in the Bay of Biscay. In Spanish Galician waters, D. acuminata occur every year, although there is strong interannual variability in the duration of the closures related to this species (Reguera et al., 2012; Moita et al., 2016). In the autumn transition, from upwelling to downwelling conditions at the end of summer, blooms of D. acuta appear in some years (Díaz et al., 2016, 2019; Ruiz-Villarreal et al., 2016). A recent review of 30 years data show that there has been no increase in frequency or intensity of D. acuta in Galician Rias (Díaz et al., 2016). Dinophysis ovum, D. sacculus, and Dinophysis fortii (with a preference for mild and warm temperatures) are also common in the south of Spain and Portugal. In Spanish Andalusian waters, the Dinophysis acuminata complex is present most of the year, but concentrations are seasonal with two or three peaks occurring in spring and autumn (Fernández et al., 2019). Dinophysis acuta reaches peak concentrations in summer and the beginning of autumn (Fernández et al., 2019). In Portugal, Dinophysis blooms are observed from April to October (spring to autumn) during the upwelling season and particularly during the autumn transition, associated with periods of thermohaline stratification between moderate pulses of upwelling or during downwelling events (Moita et al., 2006; Trainer et al., 2010; Reguera et al., 2012, 2014).
PSP – Paralytic Shellfish Poisoning; PST – Paralytic Shellfish Toxins: Several members of this genus Alexandrium contain the gene-specific to produce STX and its mere presence implies a risk (Swan and Davidson, 2012). The highly toxic PST producing species Alexandrium catenella (formerly identified as Gonyaulax excavata, Alexandrium fundyense, and/or Group I ribotype/N. American Alexandrium tamarense) occurs in Scottish waters and can result in shellfish flesh samples above regulatory threshold limits at low cell densities. However, indistinguishable (at least by light microscopy) non-toxic Alexandrium tamarense can co-occur (Touzet et al., 2010). Alexandrium minutum is the primary causative organism responsible for PSP in Irish shellfish and in the south west of England, although non-toxin-producing strains have been recorded in Ireland (Touzet et al., 2007; Clarke, 2020) and Scotland (Brown et al., 2010; Lewis et al., 2018). In 2020 in southwest Ireland, the highest ever PSP concentrations were observed in mussels and oysters, where unusually the PSP event lasted for several weeks. Along the French Atlantic coast, the main toxic species is also A. minutum with regular blooms in bays and semi-enclosed areas around French Brittany (e.g., Penze estuary, Bay of Brest, and Rance) resulting in closures (Santos et al., 2014; Chapelle, 2016). The Alexandrium occurrence, bloom and regulation in each bay is, however, driven by different biological processes such as water temperature, dilution rates, river inputs, and/or pathogens (Chambouvet et al., 2008; Chapelle et al., 2015; Sourisseau et al., 2017). The population of A. minutum is also heterogeneous along the coast with sub-populations having different physiologies and levels of toxicity (Le Gac et al., 2016). The species Alexandrium ostenfeldii has been identified in various locations in the region including Spain, France, United Kingdom, and Ireland (Bresnan et al., 2021). In addition to saxitoxins this species can produce spirolides (Davidson et al., 2015) and other toxins including gonyautoxins (Martens et al., 2017), but with spirolides being the dominant toxins in European and North Atlantic A. ostenfeldii populations (Sopanen et al., 2011).
Paralytic Shellfish Toxins are also associated with Gymnodinium catenatum (Visciano et al., 2016). This species is prevalent in Iberian waters (Galician and Andalusian waters in Spain, and Portugal) and poses a concern. However, it has not been identified in the Basque Country area (Muñiz et al., 2017). The timing of G. catenatum blooms is associated with relaxation of upwelling events from late summer and subsequent advection into coastal areas, (Bravo et al., 2010) which are more common on the Mediterranean side than on the Atlantic side of Andalusia. In the Gulf of Cadiz and the Iberian Peninsula, the dominant organisms are Gymnodinium catenatum and Alexandrium minutum (Vale et al., 2008), the former being almost exclusive in the Atlantic coasts of Spain and Portugal, from mid-summer to autumn.
ASP – Amnesic Shellfish Poisoning; AST – Amnesic Shellfish Toxins: The genus Pseudo-nitzschia contains species or strains capable of producing domoic acid (DA) (Landsberg, 2002; Moestrup et al., 2009). In Scotland, similar to Dinophysis, this genus is thought to be transported advectively by oceanographic currents (Fehling et al., 2012; Siemering et al., 2016). Pseudo-nitzschia is categorized within the low toxin producing Pseudo-nitzschia delicatissima group and the (usually) toxic Pseudo-nitzschia seriata group. Typically blooms of the P. delicatissima group start earlier in the year (March–April), with blooms of the P. seriata group occurring in July–August when most toxicity is expected (Fehling et al., 2006; Rowland-Pilgrim et al., 2019). Analysis of Pseudo-nitzschia blooms in Loch Creran (Argyll and Bute, Scotland) has suggested that these are related to periods of poor weather (low air pressure and rainfall preceding the blooms). In Ireland, Pseudo-nitzschia australis blooms, observed from March to May, from the southwest up along the west coast, are the common cause of harvesting closures (usually short 1–2 weeks) (Clarke, 2020). DA producing species P. seriata and Pseudo-nitzschia multiseries have also been detected in Irish waters. In France, blooms of Pseudo-nitzschia species, including P. australis, are regularly observed from the Bay of Biscay to the English Channel (Nezan et al., 2010; Thorel et al., 2017), from March to November. These blooms appear linked to the seasonal and interannual frequency (Husson et al., 2016) of physical and hydrological processes (i.e., river discharge, upwelling, vertical mixing). In Galicia, blooms of Pseudo-nitzschia have been reported for decades (Míguez, 1996; Velo-Suarez et al., 2008), but with only one report of human intoxification, involving two people, in 2004 (HAEDAT database). In the Basque Country, the detection of toxins in April 2017 coincided with a bloom of Pseudo-nitzschia spp., but the causative species could not be elucidated. During the upwelling season in Portugal, from spring to late summer, blooms of Pseudo-nitzschia spp. are recurrent (Palma et al., 2010) although harvesting closures are very few per year and of short term duration (1 month maximum). P. australis (Costa and Garrido, 2004) and P. multiseries (Godinho et al., 2018) have been reported associated with these events.
AZP – Azaspiracids Shellfish Poisoning; AZA – Azaspiracid Toxins: In Ireland, Azadinium spinosum is the causative species of Azaspiracids, which can occur all around the Irish coastline, but predominantly cause closures around the northwest, west and southwest coasts. Similar to DSP, significant and prolonged closure periods are caused by AZA events, and on occasion can carry over from 1 year to the next, as observed in 2005–2006, and in 2013–2014 (Clarke, 2020). Azadinium has also been observed in Scottish and southwest of England waters (Paterson, 2017; Dhanji-Rapkova et al., 2019). The genus has also been detected in France (Brittany) and Spain (Galicia) since early 2000s (Magdalena et al., 2003) with a recent event in south Spain (Tillmann et al., 2017). However, reports of human illness have been sporadic in the NEA region (Bresnan et al., 2021). Also occurring in Irish waters, producing different AZA compounds (not currently legislated for), is the closely related Amphidoma languida. In Portugal, the genus is routinely monitored but no blooms or associated impacts have occurred to date.
We now describe typical patterns of HAB shellfish closures using as examples a northern (Ireland) and southern region (Portugal) since other countries around them follow similar patterns:
In Ireland, it is not unusual to have site closures due to toxins above regulatory levels from all four main toxin groups ASTs, AZAs, DSTs and PSTs (Table 2). Most years shellfish production area closures are due to DST and AZA. However, the extent, the maximum concentrations observed, and the length of the closure varies considerably from year to year, and occasionally intoxication of shellfish occurs late in the year (Clarke, 2020). The number of closures due to AST and PST is variable, with some years having no closures at all due to concentrations below regulatory levels. When there is an AST or PST event, it is usually short-lived with closures in place for 1–2 weeks (Table 3).
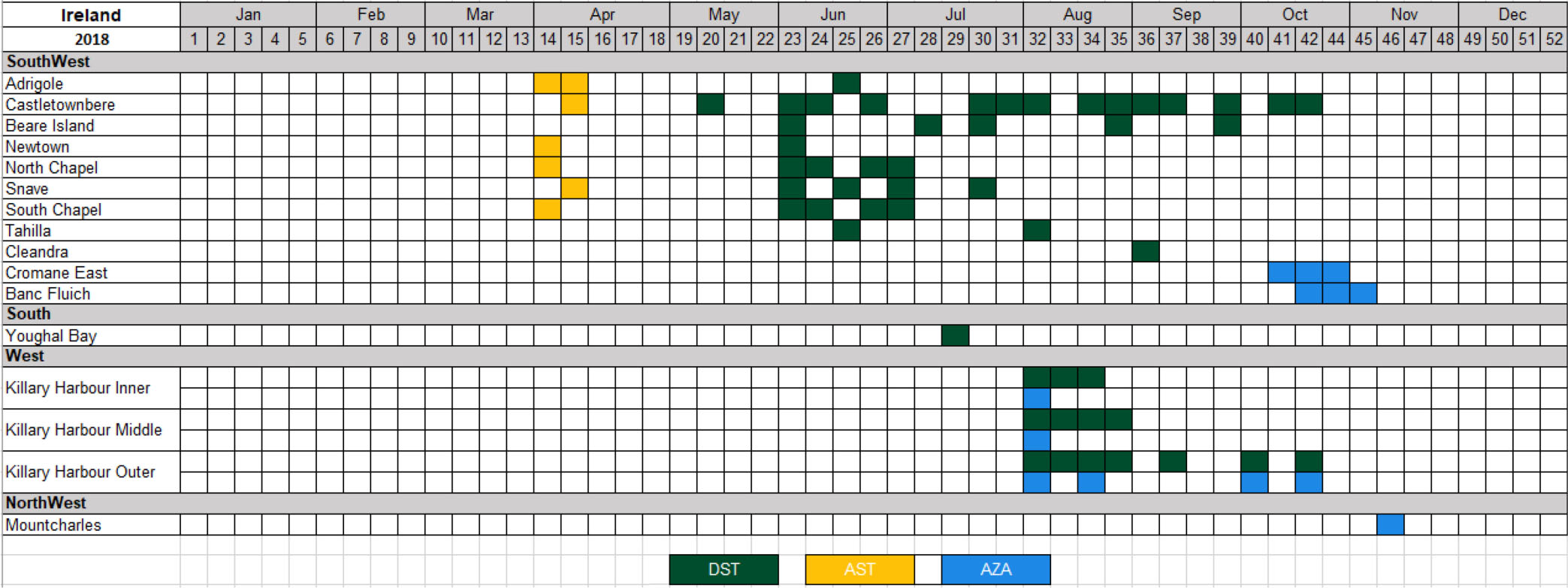
Table 3. Closure table of Irish production areas in 2018 due to toxins concentrations above regulatory levels.
In Portugal, events occur mainly from spring to autumn and are associated with upwelling (AST) or the subsequent stratification after wind relaxation (DST and PST) (Table 4). Closures can last 9 months from the beginning of the year. AST events are less frequent where the Pseudo-nitzschia genus is always present in Iberian waters. PST events usually occur between September and December/February associated with Gymnodinium catenatum blooms, at the end of the upwelling season (Moita et al., 2003; Pitcher et al., 2010) until January in northern Portuguese waters (Pazos et al., 2006). Pseudo-nitzschia species were regularly observed in water samples and during spring-summer upwelling episodes (Palma et al., 2010).
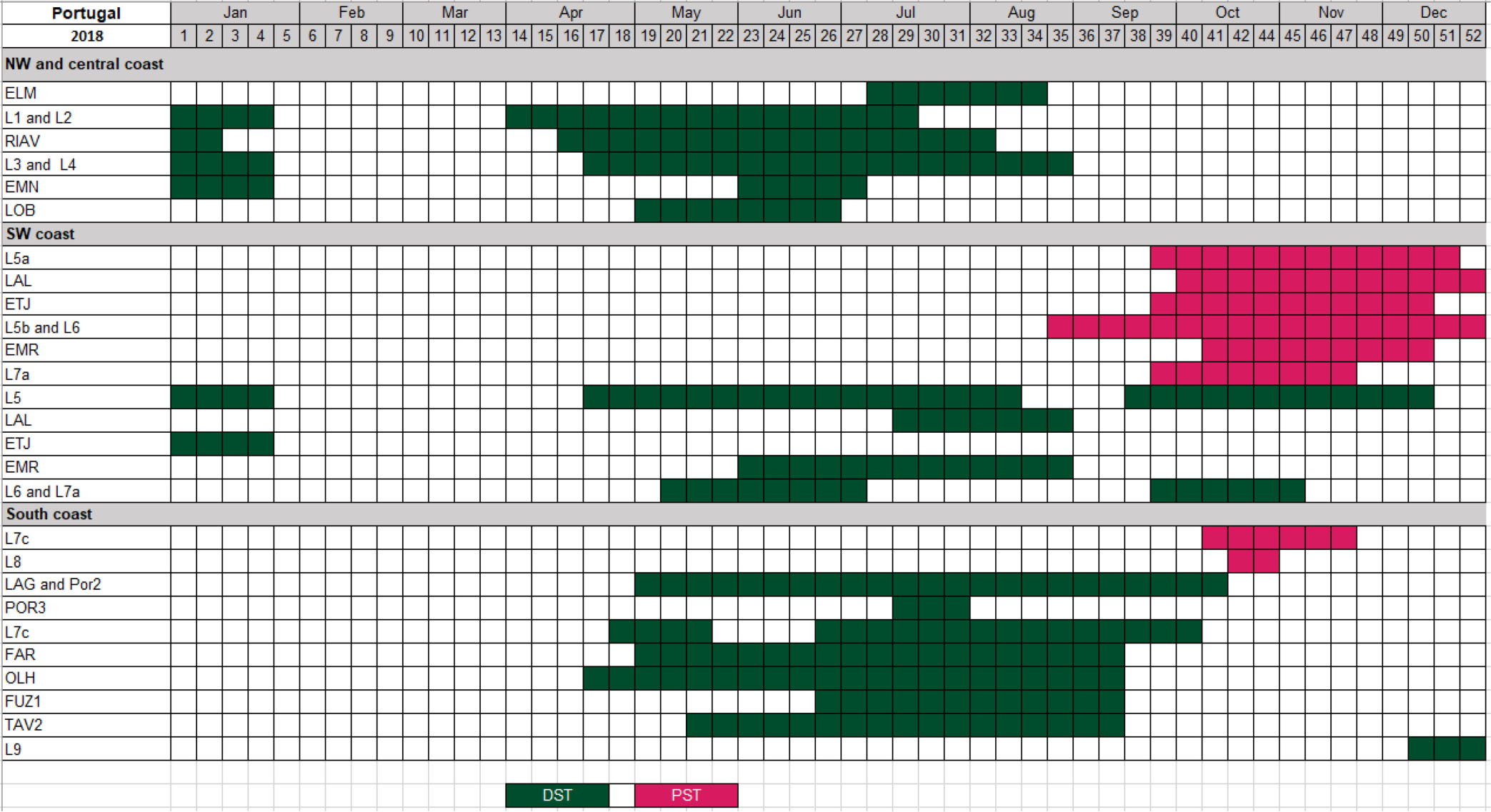
Table 4. An overview of the spatial and temporal distribution of ban-on-harvesting periods in Portugal during 2018.
The Historical Evolution of EWS
This section has shown that there is a heterogeneity of toxic events that can be harmful for human health and lead to shellfish area closures that have a direct, negative impact on the industry. Risk and uncertainty (Mousavi and Gigerenzer, 2014) represents a management challenge that impacts the industry throughout the year, not just when outbreaks occur. Therefore, the need for reducing this uncertainty is great, but shellfish farms are generally small businesses without the capacity to develop their own EWS. Therefore, most EWS developments have been initiated by the scientific community after noting the needs of the industry and regulatory authorities, and the scientific potential. For example, in Ireland, farming closures since the late 1980s especially in the southwest, have been a major concern for the continual development of the sector and drove the development of forecasting approaches in that location. Initially, as part of the ASIMUTH project, several institutes in Scotland, Ireland, France, Spain, and Portugal developed risk assessments, models and algorithms to assess the probability of occurrence of a HAB event and disseminated this information to stakeholders in “bulletins” (Davidson et al., 2016; Maguire et al., 2016). This was the precursor of the PRIMROSE project, which has improved these bulletins and added additional operational models (https://www.shellfish-safety.eu/).
Operational or pre-operational systems have previously been trialed in Scotland, France, Ireland, the Basque country, and the wider United Kingdom as described below:
In Scotland, the introduction of weekly HAB bulletins and assessments came after the trade association Seafood Shetland approached SAMS following a large, advected bloom of Dinophysis in 2013, that impacted all the active shellfish harvesting sites on the West coast of Shetland. The bloom, exacerbated by strong westerly winds, caused Dinophysis numbers in the affected sites to accumulate rapidly, raising toxin concentrations in the shellfish above actionable levels within 2–3 days of the bloom’s arrival (Whyte et al., 2014). This time span was shorter than the weekly frequency of the regulatory monitoring program and hence intoxicated shellfish reached market. As a result, seventy people reported illnesses associated with symptoms of diarrhetic shellfish poisoning. The approach to reporting HABs in Shetland has developed iteratively through dialogue between local stakeholders and scientists at SAMS. The Scottish bulletin has been well received by industry who continue to be regularly updated on its development through local stakeholder workshops.
By contrast, in France EWS development has mainly been driven by scientific interest, but, unfortunately, has failed to attract enough interest from the industry. As an example, a French oceanographic forecasting system (PREVIMER; Charria et al., 2014) was built 10 years ago, and although some scientific developments related to HABs were progressed within this system through several joint projects (EASYCO, ASIMUTH) there was little industry interest was noted in its use.
As in Scotland, the Irish bulletin seems to have attracted the interest of the industry. The bulletin is usually prepared on Monday–Tuesday each week and then uploaded to the public access website from where it is publicly accessible and available for download. The bulletin is weighted to those areas where impacts from HABs are greatest, primarily the aquaculture sites of the southwest and west. However, bulletins and the forecasts they contain are assembled and issued for the whole country. There is an increase in the use of the bulletin during the bloom season where detectable amounts of biotoxins are more likely to occur. Besides the weekly bulletin, the Marine Institute provides up-to-date information on its website, https://webapps.marine.ie/habs related to the concentration of phytoplankton and biotoxins for the inshore and offshore production areas.
The ShellEye project was funded for 4 years by the United Kingdom Biotechnology and Biological Sciences Research Council (BBSRC) and Natural Environment Research Council (NERC) to develop a water quality bulletin service specifically for the shellfish industry, using the latest satellite and modeling technologies. ShellEye was driven by industry demand with the bulletin service being trialed by a number of shellfish farmers and related stakeholders, focused on four pilot sites in England, Wales, and Scotland: St Austell Bay, Menai Straits, Morecambe Bay, and Loch Ryan. Feedback obtained from shellfish farmers demonstrated the potential for the service, confirming they were able to use the information in the day-to-day management of their farm, and were interested to continue receiving bulletins, e.g., “We enjoyed the bulletins hugely and find it amazing to be able to see so clearly. We actually harvested our first batch of oysters this week, so the emails were very useful.”
The Basque country is a special case since aquaculture activity is very recent and has been driven by a coordinated group of local industries, local institutions, and local scientists. Therefore, the need for an EWS has been, from the beginning, driven by industry, institutions and scientists together. As farming is offshore, the EWS is required to include, not only harmful events, but also information on extreme events (e.g., winds and waves).
Short-Term Forecast of Hab Occurrence and Toxin Presence in Shellfish
A development within modern alert systems is the use of a range of approaches to provide a forecast rather than a “now cast” of HAB and biotoxin conditions. These can be summarized into several major types (Davidson et al., 2016; Maguire et al., 2016; Mateus et al., 2019).
1. Type 1: Industry alert “bulletin” reports, which are compiled from datasets including data generated from biotoxin and/or phytoplankton monitoring programs, and include, where available, additional environmental variables. These parameters are often synthesized into graphical and map images for easy interpretation by stakeholders. The simplest bulletins are only warning systems reporting current findings, and do not necessarily function as early warning systems. However, through an “expert interpretation” to assess current and short-term future evolution of HAB and biotoxin risk, these bulletins can function as forecast systems for aquaculture businesses.
2. Type 2: Particle tracking based systems. These systems rely on Lagrangian dispersion modeling approaches. They aim to identify key production points of toxic phytoplankton and track their dispersal using oceanographic modeling. Lagrangian particle tracking models are a useful tool to predict the transport of HAB events once they have been observed. Particle tracking models, coupled to circulation models, are used in all NEA arc countries.
3. Type 3: Statistical models based on remote sensing. These models aim to improve the inference of presence from environmental conditions (e.g., sea surface temperature and surface chlorophyll) from satellite images and the statistical relationship with the presence of toxic phytoplankton. The approach aims to forecast the presence of toxic events under particular environmental conditions, even if the cause-effect relationships are not fully understood.
4. Type 4: Statistical (e.g., Generalized Additive Models, GAMs) and machine learning models (e.g., Bayesian networks) based on the fusion of multiple sources of data. These types of model analyze environmental conditions during historic toxic events to establish relationships between environmental factors and the development of toxic events.
5. Type 5: Mechanistic full-low trophic ecosystem models. These systems rely on the use of the mechanistic understanding of the relationships between environmental conditions and the presence of toxin-producing phytoplankton. As such, the relationships of environmental conditions with the proliferation of toxic phytoplankton, its dispersion and assimilation by bivalves is explicitly addressed. However, most of these models work at aggregated trophic levels and hence struggle to differentiate between harmful and benign phytoplankton. The degree of complexity of these systems varies, dependent on the complexity of the models they rely on.
Forecasting systems are rarely comprised of just one approach, but instead are a combination of shared information from different multiple approaches. For example, the boundary between type 4 and type 5 models may not be clear, since statistical modeling always uses some of the mechanistic knowledge available (e.g., variables to include), whereas mechanistic models use statistical inference of equations and parameters. The above approaches are currently incorporated in “bulletin” reports from each region utilizing the various datasets and data products available (Supplementary Table 1). Most of this information in Atlantic arc countries, especially HAB in situ data, had not been previously available publicly. However, this information is now becoming available through public bulletins, datasets, websites and media platforms providing access to monitoring data and data products in combination with forecasting models (Table 5). The current status of forecasting systems in Atlantic Arc countries is summarized below.
In Scotland, the alert system includes a “traffic light” based risk index and a number of other data products that summarize current and historical HAB and shellfish biotoxin risk for the country as a whole. In addition, a more detailed “expert interpretation” based risk assessment is provided for the Shetland Islands. Bulletins have been produced weekly since 2014 (type 1 system) and are available via www.HABreports.org in pdf format providing a summary of HAB and biotoxin concentrations in the current and previous 3 weeks. Additional information is provided on relevant environmental conditions allowing for expert interpretation-based risk assessment for the week ahead to be generated. This additional information includes mean wind direction in the current and three preceding weeks from local meteorological stations, forecasted sea surface currents from the Mercator Ocean model, sea surface temperature from JPL and Chlorophyll from Copernicus. HAB predictions based on the WeStCOMS mathematical model (type 2) are also integrated within the HABreports web site with the aim of predicting the likely impact on coastal aquaculture of any blooms that have been detected offshore by remote sensing (type 2 system). The effectiveness of the early warning of the Scottish system has been evaluated by Davidson et al. (2021) who found predictions to be 74% accurate.
The Scottish HABreports alert system also includes model based HAB predictions from the WeStCOMS mathematical model (type 2 system) with the aim of predicting the likely impact on coastal aquaculture of any blooms that have been detected offshore by remote sensing (type 2 system). The WeStCOMS mathematical model is a coupled meteorological/oceanographic model that includes meteorological forcing using the open-source Weather Research and Forecasting (WRF) model v. 3.5.1 (Skamarock and Klemp, 2008). This is a non-hydrostatic atmospheric model, nested within the NOAA National Centers for Environmental Prediction (NCEP) operational forecast model with 1° spatial resolution (Juang, 2000). The WRF model domain covers Scotland and its neighboring seas with a grid of 140 × 240 points. The finest resolution is around 2 km in the central part. The oceanographic model is a Finite-Volume Coastal Ocean Model (FVCOM) based hydrodynamic model. Model bathymetry was based on gridded data from SeaZone (2007) and refined in certain key areas using Admiralty charts and some multi-beam surveys. Vertical eddy viscosity and diffusivity were computed using the Mellor-Yamada 2.5 scheme (Mellor and Yamada, 1982). Horizontal diffusion was represented using a Smagorinsky (1963) eddy parameterization with a fixed coefficient (C = 0.2). The bottom boundary layer was parameterized with a logarithmic wall-layer law using a drag coefficient. The hydrodynamic model was integrated numerically using a time-split method with an external time step of 0.6 s. Boundary conditions: Water movement within hydrodynamic models is predominantly driven by tidal forcing applied at the “open” (water) boundaries. The tidal forcing was derived from the 1/30° degree implementation of the Oregon State University Tidal Prediction Software (OTIS) for the European shelf (Egbert and Erofeeva, 2002). At each of the domain’s open boundary nodes, the 11 primary tidal constituents were used to derive sea surface elevation time series. Open boundaries also included a 6-km-wide “sponge layer” to filter high-frequency numerical wave reflection noise using a Blumberg-Kantha implicit gravity wave radiation condition (Wu et al., 2011). The use of this model to operationally simulate HABs is explained more fully by Aleynik et al. (2016) and Davidson et al. (2021).
In England, since the completion of the ShellEye project outlined above, there is currently no operational forecasting of HABs undertaken for this region. However, the project demonstrated the potential for a water quality monitoring service for the aquaculture industry, using satellite ocean color products to provide early warning of the growth or decline of certain high-biomass HAB species in a weekly bulletin (Miller et al., in press). Of the marine biotoxin producing genera, only Pseudo-nitzschia spp. can form a dense enough bloom to affect ocean color; but not all blooms of this species release toxins meaning that careful interpretation of results is required.
The unstructured grid hydrodynamic model FVCOM is also run operationally for a domain covering the Southwest United Kingdom, producing 3-day predictive forecasts. The model is forced by an operational WRF for surface forcing and the lateral boundary data are obtained from the CMEMS AMM15 model. The river input is modeled from the WRF temperature and precipitation. The Lagrangian particle tracking model PyLAG (Uncles et al., 2020) is run offline on saved hourly outputs from FVCOM. This Lagrangian model uses a Milstein numerical scheme for advection and diffusion, with the diffusivities provided directly from the hydrodynamic model. There are no biological behaviors, and the virtual particles are modeled as buoyant, however, it is possible to set up with 3D advection and some simple behaviors (e.g., temperature dependence). Particles are seeded based on the HAB-risk product (Kurekin et al., 2014) in a 200-m radius around identified high risk areas. These are advected until the end of the forecast period and the results of the model are served as both a gridded particle density and probability field: this takes into consideration both the uncertainty from the identification algorithm and the drifting particles.
ShellEye also investigated single site statistical models of the environmental conditions that promote the release of algal toxins (Type 3 models). It was found that sea surface temperature (SST), solar radiation, wind speed, time-lagged rainfall and wind direction, were useful in predicting the onset of DSP toxins (Schmidt et al., 2018).
In Ireland, since 2013, the Marine Institute has produced a weekly HAB bulletin which is published and publicly available in PDF format through the in-house developed HABs database and website platform. The objective of the bulletin is to provide a short-term (3–5 days) predictive forecast on the likelihood of the occurrence of a HAB event around the Irish coastline and inshore areas. This prediction is based on current and historical data of HABs species and marine biotoxin occurrence from the national monitoring programs, incorporating observed trends and patterns with data input from satellite data and hydrodynamic models.
The bulletin provides information on the potential development and occurrence of toxins in shellfish and/or harmful phytoplankton species (type 1 system) in aquaculture areas, based on in situ monitoring and data generated over the preceding 3 weeks. In detail, the bulletin contains geographical maps of the cell densities of the major HAB phytoplankton species (Pseudo-nitzschia spp., Azadinium-type spp., Dinophysis spp., and Alexandrium spp.) and their associated causative biotoxin concentration in shellfish [Domoic Acid (AST), Azaspiracids (AZA), Okadaic Acid and Dinophysistoxins 1, -2 (DST), and Saxitoxin and related compounds (PST)]. The number and region of any closures of shellfish aquaculture production areas due to these toxin concentrations exceeding regulatory levels in shellfish are presented as doughnut charts with accompanying text comments as regards the observations based on the preceding 3 weeks of data. Other HAB species are also detailed, particularly the bloom-forming species types, commonly observed in Irish waters, including Karenia mikimotoi, Phaeocystis spp., and Noctiluca scintillans. Data and results are mapped and tabulated from historical trends of observed biotoxin concentrations per geographical region from the beginning of the current year to date and also for the current week and additionally the historical occurrence of toxicity per region over the last 10 years per toxin group. Furthermore, a list of the top five phytoplankton species per geographical region from the preceding to the current week is also displayed.
Satellite imagery detailing chlorophyll-a concentration and its anomaly (in comparison to the average of the past 60 days) and sea surface temperature (SST) are also displayed. The method used to calculate the chlorophyll-a anomaly uses the 60-day median chlorophyll-a concentration, not including the last 2 weeks to prevent a potential recent bloom skewing the median field (Stumpf et al., 2003 and Tomlinson et al., 2004). For SST, the information provided by the Irish Weather Buoy Network (IMOS) on the 10-year weekly average temperature is used and compared with the same week of the past 10 years, so the anomaly is based on the long-term weekly average and each measured sea surface temperature value has the appropriate weekly average value.
Over many years, hydrodynamic models for three Irish sites (Bantry Bay in the Southwest of Ireland, Killary Harbour and Cleggan at the West coast of Ireland) have been developed within the Marine Institute. These provide information on water flow, particle tracking and physical oceanographic features (type 2 system). For this, hydrodynamic forecasts from a Regional Ocean Modeling System (ROMS) for 3 days in Irish coastal waters is created. ROMS is an evolution of the S-Coordinate Rutgers University Model (SCRUM), as described by Song and Haidvogel (1994). The numerical aspects of ROMS have been described in detail by Shchepetkin and McWilliams (2005), where the ROMS AGRIF version developed in France (Debreu et al., 2012) is used. The downscale models of areas of particular interest (Bantry Bay, Killary Harbour, and Cleggan Bay) incorporate online particle tracking with virtual particles released at pre-defined transects at the start of each model run (Leadbetter et al., 2018). The operational model also produces an estimate of the ocean state as a 3–7 days forecast of the dominant regional physical processes that result in water exchange events between the bay and its adjacent shelf for these three areas. Upwelling and down-welling signals indicate potential for future toxic contamination of shellfish in certain bays in the south-west of Ireland. The skill of the model in simulating toxic HAB episodes up to 10 days in advance is demonstrated by Cusack et al. (2016). Similar to the preparation of the weekly Scottish bulletin the data summary graphics are produced using automated routines, which are then assessed by an expert evaluator. This evaluator provides expert opinion and analysis based on the datasets and all the parameters described above, and provides text to the weekly bulletin to provide a national forecast for the current week (3–5 days forecast), describing the cautionary predictive risk of a potential new HAB event occurring, or an existing event continuing/dissipating per toxin group or for bloom forming species (Dabrowski et al., 2016; Maguire et al., 2016; Leadbetter et al., 2018).
In France, Ifremer produces publicly available (type 1) weekly HAB bulletins based only on toxins and/or phytoplankton monitoring for all areas of the coast. Despite previous short-term forecast attempts (type 2 and 5), there is currently no operational forecasting included in bulletins, however, a new alert system remains in development as outlined below.
For the PST producing Alexandrium minutum, the retention time in the bays, water temperature, riverine nutrient input and resources competition were identified as the main abiotic drivers of blooms (Guallar et al., 2017). Subsequently, mechanistic approaches (type 5) based on these observations were evaluated (Sourisseau et al., 2017) but forecasting capacity has decreased over the years since the first intoxification event (2012), suggesting some evolution of the plankton communities.
Modeling studies (type 5) of the fish-killing dinoflagellate Karenia mikimotoi suggested that stratification (Gentien et al., 2007; Vanhoutte-Brunier et al., 2008) may not be the only driver of these blooms and that transport in the coastal current, as well as seeding from offshore blooms influence the incidence, consistent with modeling of this species in Scottish waters (Gillibrand et al., 2016; Sourisseau et al., 2016). A HAB forecasting exercise (simulating K. mikimotoi and also Pseudo-nitzschia spp., and Phaeocystis spp.) based on such mechanistic models (type 5) over a short time scale (∼ 4 days) was conducted during the PREVIMER project (Ménesguen et al., 2014) with mixed success prior to this operational model being terminated in 2017.
Currently, there is no specific hydrodynamic model in operation to forecast HABs advection in France. However, hydrodynamic condition forecasts at different frequencies (hourly to daily) are provided at several resolutions (from 4 km to 500 m) up to 4 days in advance. These 3D field solutions are used to forecast advections of exceptional events by using tools such as the Lagrangian particle tracking model Ichthyop (Lett et al., 2008).
Using type 4 approaches, a range of statistical relationships between HABs and environmental variables were obtained using different methodologies (Díaz et al., 2013; Hernandez Farinas et al., 2015; Husson et al., 2016; Guallar et al., 2017; Barraquand et al., 2018; Karasiewicz et al., 2018). These studies suggest that the whole planktonic community (species interactions and ecological niches) should be taken into account to fully understand HABs dynamic (Karasiewicz et al., 2018). In particular it is important to represent large functional groups (diatoms vs. dinoflagellates) which appeared to be linked with decadal variability of river inputs.
Work in different Spanish regions occurs autonomously and hence each is considered separately. For the Basque Country (North of Spain, Southeast Bay of Biscay waters), several systems are being developed: (i) a bulletin or report based on sampling (type 1 system); (ii) an operational hydrodynamic model based on the Regional Ocean Modeling System (type 2), with a mean horizontal resolution of 670 m and 32 vertical levels (Ferrer et al., 2007, 2009, 2013); and, (iii) a machine learning system (type 4) based on supervised classification and Bayesian networks (Fernandes et al., 2010, 2013, 2015).
The ROMS hydrodynamic model is used to obtain a 4-day forecast of current, temperature, and salinity fields in the south-eastern Bay of Biscay. ROMS has been used in several studies to model the water circulation in the study area (e.g., Ferrer et al., 2007, 2009, 2015; Ferrer and Caballero, 2011; Caballero et al., 2014; Laiz et al., 2014; Legorburu et al., 2015). The ROMS domain used in the EuskOOS modeling system covers the southeast Bay of Biscay, extending from 43.24 to 44°N and from 3.4 to 1.3°W, with a mean horizontal resolution of 670 m. Vertically, the water column is divided into 32 sigma-coordinate levels. These levels are more concentrated within the surface waters, where most of the variability occurs. The bathymetry was obtained from the European Marine Observation and Data network (Vasquez et al., 2015), smoothed to ensure stable and accurate simulations (Haidvogel et al., 2000). The atmospheric forcing used in ROMS is obtained from the Weather Research and Forecasting model (WRF) run by Euskalmet (Basque Meteorological Agency). The boundary conditions are obtained from the 3-D hourly data provided by the NEMO model for the Iberian-Biscay-Ireland region. The online 3-D Lagrangian particle tracking module existing in ROMS is activated and is generating forecasts of the 4-day evolution of several virtual particles released at fixed points and at 00:00 UTC in the ROMS domain at high temporal resolution (1 min). These virtual particles are initially located at the sea surface to forecast the drift of HABs from and to the pilot aquaculture farm (located in the Mendexa region). For the analysis of specific events in the past, the Sediment, Oil spill, and Fish Tracking model (SOFT) is used with the ROMS outputs and satellite-derived information.
A machine learning approach was used to find non-linear correlations (Symmetrical Uncertainty Score; SUS; Hall, 1999) and key non-correlated predictors (Correlated-based feature subset selection; CFS; Hall and Smith, 1997; Hall, 2000) between environmental conditions across the region and the presence of DSTs in shellfish in aquaculture sites. The presence of these toxins is often correlated with higher concentrations of phytoplankton cell density (blooms), but it is also observed that toxins in shellfish can occur at low cell densities (Table 6). The model has been validated using 10-fold cross-validation with an accuracy of 67% and only 3% of false positives. If we use the presence of D. acuminata as a predictor, the accuracy increments to 83%, without increasing the false positives. The relationships found by the model have been observed in other areas of the world (Zhu et al., 2017). Relationships between toxins, nutrients, and productivity are common (Bates et al., 1989; Hutchins and Bruland, 1998; Pan et al., 1998; Sunda et al., 2005; Anderson et al., 2008; Sun et al., 2011; Lelong et al., 2012; Tatters et al., 2012; Schnetzer et al., 2013; Sison-Mangus et al., 2014; McCabe et al., 2016; Drakulović et al., 2017; Grzebyk et al., 2017; Zhu et al., 2017). Surprisingly, temperature is not a selected predictor of toxin presence (Zhu et al., 2017; Smith et al., 2018), but it might be because there have not been significant changes in the temperature range in the region (Revilla et al., 2010). Negative correlations with silicates might be due to previous blooms of diatoms. The presence of toxins is correlated with nutrient concentrations outside of the aquaculture site. In the region, there is an important bathymetric gradient with a transition from a coastal current from West to East to a weaker oceanic current in the opposite direction. In the west, limited eddies that transport nutrient-rich waters to the open sea are common (Ferrer and Caballero, 2011). Therefore, these eddies facilitate water mixing and nutrient upwelling, supporting the proliferation of toxic phytoplankton species as well as their transport to other coastal areas (Figure 3). This transport can take between 1 and 4 weeks depending on oceanographic and meteorological conditions (Ferrer and Caballero, 2011). This type of phytoplankton species transport mechanism has been previously documented in northern areas of the Bay of Biscay (Batifoulier et al., 2013). Similarly, some Scottish harmful algal bloom genera/species are thought to develop offshore and be advected by winds and currents to the coast. An example of this was the exceptional Dinophysis bloom in the Shetland Islands in 2013 that resulted in an outbreak of shellfish poisoning (Whyte et al., 2014).
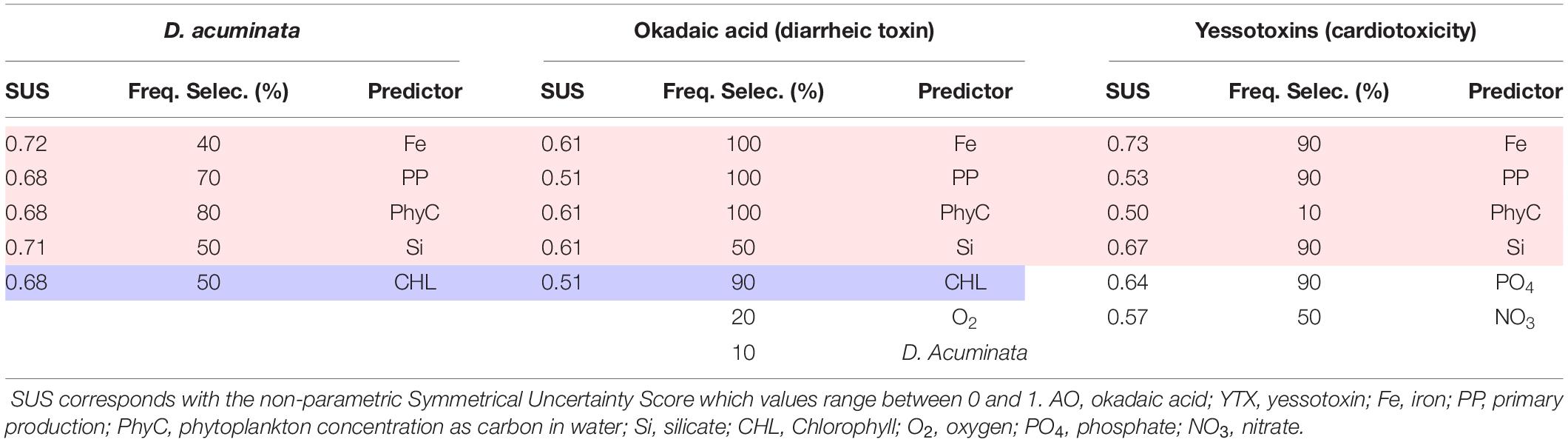
Table 6. Selection of potential predictors using cross-validation showing the percentage of times that each predictor has been selected (Freq. Selec.).
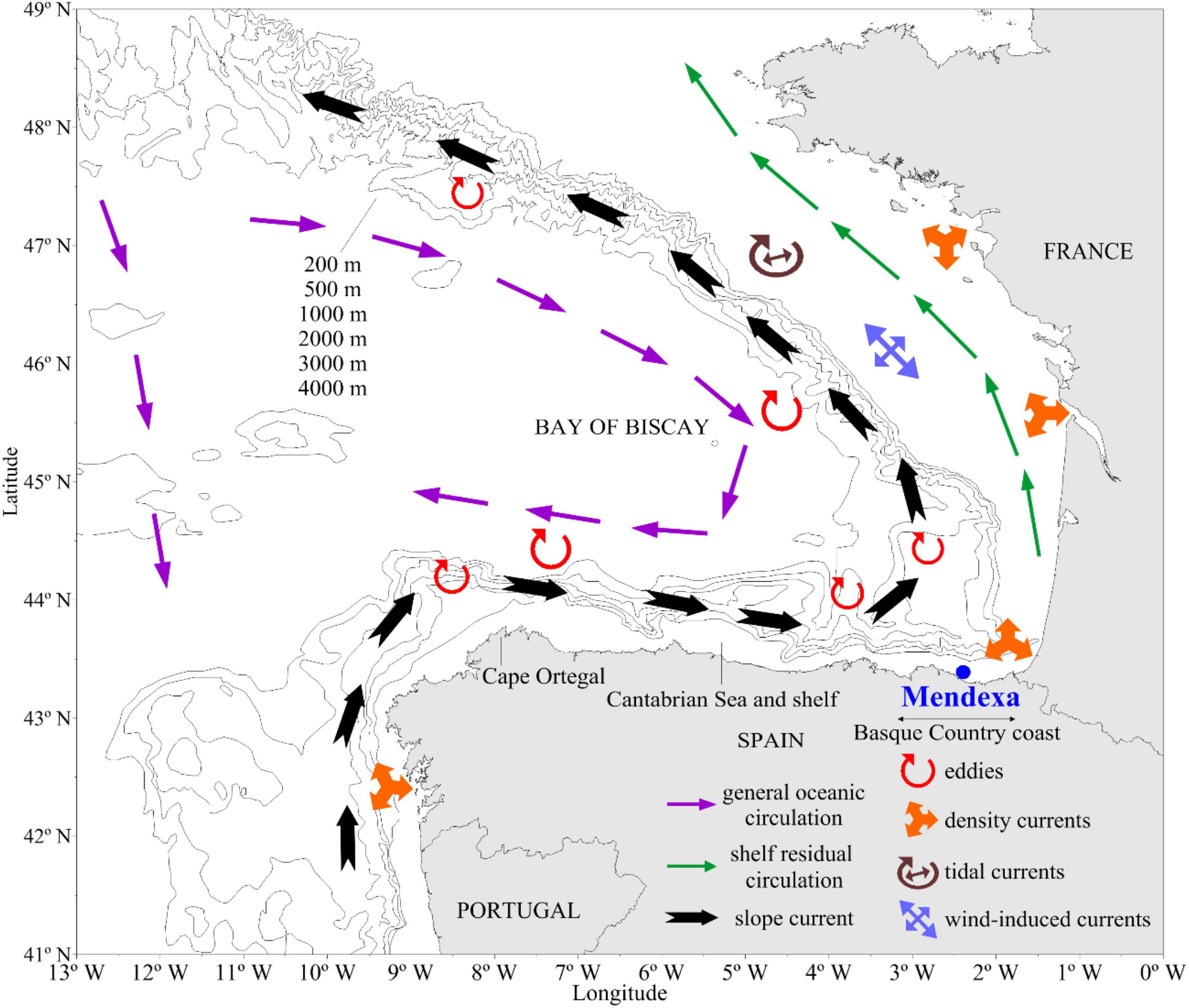
Figure 3. Map of main physical characteristics in the Bay of Biscay [adapted from Ferrer and Caballero (2011)].
Along the Spanish coast, the general tendency at the sea surface is a flow from west/northwest to east/southeast, this occurs at least 90% of the time (based on small buoys trajectories analysis). CTD data indicates that this flow is evident at 10–15 m where peaks of chlorophyll appear. Thus, most HABs are expected to come from the western Spanish coast (or offshore areas with eddies such as the Cape Ortegal longitude, 8°W, as predicted in the Bayesian network model) than from the French coast.
In Galicia, an oceanographic system (type 2) has been developed to forecast Dinophysis spp. presence (Ruiz-Villarreal et al., 2016). A HAB bulletin was designed to provide information on the current and potential future state of HABs and biotoxins in Galicia within a 3-day forecast. This early warning system supports decisions of the Galician regulatory monitoring system operated by INTECMAR as well as providing forecasts to the Galician aquaculture industry. The system integrates HAB information from monitoring programs with hydrodynamical models and both physical and biochemical data from in situ networks along with satellite imagery. It has been shown to be capable of making predictions of bloom transport, but not the initiation of a toxic event, especially for low biomass HAB species. Additionally, it has been shown that early warning of the risk of autumn toxic dinoflagellate blooms in the Galician Rías is feasible by combining Lagrangian particle tracking simulations with HAB data from Portuguese monitoring (Ruiz-Villarreal et al., 2016). The Lagrangian particle tracking model Ichthyop (Lett et al., 2008) is used for Galicia. Ichthyop runs offline forced by the hourly results of the Meteogalicia ROMS nowcast and forecast simulations2. In common with approaches elsewhere in the Atlantic Arc, the HAB cells are considered as passive particles that move with the flow and do not have “behavior” (Velo-Suárez et al., 2010; Dippner et al., 2011; Aoki et al., 2012; Ruiz-Villarreal et al., 2016). Approximately 1000 virtual particles are randomly released each day in the first 20 m of the water column at six locations/configurations: Inside the Galician Rías (Vigo, Pontevedra, Arousa, and Muros). Along the Portuguese shelf, 10,000 particles are released inside two polygons: (1) between 40.8024° N and 41.27° N and inshore the 200-m isobath; and (2) between 41.27° N and 41.87° N and inshore of the 200-m isobath. This allows forecasting of the transport from the northern Portuguese shelf to the Galician rías, where the harvesting areas are located.
In Andalusia, type 1 bulletins based on in situ monitoring are used. Upwelling is thought to be important to HAB development in this region (García et al., 2002; Navarro and Ruiz, 2006; Prieto et al., 2006, 2009; Navarro et al., 2012). In 2006, a neural network model was developed to forecast Dinophysis acuminata (Velo-Suárez and Gutiérrez-Estrada, 2007), but is not regularly used in bulletins. Recently a proof-of-concept based on satellite information has been developed (Caballero et al., 2020).
In Portugal, a prototype oceanographic system (type 2) is being updated to forecast HAB and shellfish closures areas. The system has been developed by IPMA and IST within the ASIMUTH project (Pinto et al., 2016; Silva et al., 2016). Originally, it operated for a test period of 1 year, producing weekly bulletins with an illustration of open and closed production areas, along with a forecast for potentially impacted areas, based on the ocean circulation and toxin concentrations. The updated version of the bulletin follows the same approach, with the forecast relying on a combination of field data and model results. The system relies on the hydrodynamic forecast, simulating tri-dimensional velocity fields and thermal distribution, coupled to a model of Lagrangian elements to simulate the passive transport of particles, without incorporating any biological processes. Results from the national weekly shellfish and toxin sampling program are the starting point of the forecast. The Lagrangian model is then triggered to simulate the transport of particles from those areas impacted by HABs to determine the pathways of dispersion/retention along the coast and assess the impact on neighboring areas. Model predictions with a 4-day forecast capacity rely on the PCOMS model (Mateus et al., 2012). The model setup consists of 50 vertical layers, with 43 cartesian-coordinate layers at the bottom, and seven sigma coordinate layers in the top 10 m. The model simulates the 3D thermohaline structure and velocity fields for the West Iberian coast and is systematically validated with tidal gauge data and sea surface temperature from remote sensing. The particle tracking model runs offline, relying on the previously computed hydrodynamic solutions. Particles move in two dimensions (x and y), according to local current fields pre-calculated by the hydrodynamic model. Production areas are defined as polygons along the Portuguese coast, corresponding to major bivalve production areas by the regulatory authority in Portugal (IPMA), also responsible for the national monitoring program. All polygons are populated with particles and their movement along the coast to adjacent polygons is monitored. The risks to each production area are then assessed based on the possibility of receiving particles from known contaminated areas. Two new pilot areas are being tested for local prediction, namely the Ria Formosa (in the south) and Ria de Aveiro (on the northwest coast). The Portuguese bulletin is planned to be made available in a web page format, updated twice a week (Wednesday and Friday). In addition to the modeled surface currents, wind and particle transport, it will also include monitoring results for HABs (and microbiology), oceanographic information, remote sensing data for SST and Chlorophyll-a. A warning trigger for neighboring monitoring laboratories (Galicia and Andalusia) is also planned to be included in the system.
Discussion
Effectiveness of Current Early Warning Systems
This study describes the range of toxin-producing phytoplankton, their associated toxins, the impact they can have on shellfish aquaculture in the Atlantic arc countries and the various EWS that are being developed to safeguard the industry and human health. It demonstrates that in most countries in the region, EWS are still in the early stages of development and testing. Some systems only have the capacity to provide end users with a warning based on current official control monitoring programs, while others, such as Ireland and the Shetland Islands in Scotland, are providing both risk assessments and forecasts. However, several proof-of-concept systems are under development and show promise as illustrated by the examples in section “Short-Term Forecast of HAB Occurrence and Toxin Presence in Shellfish” and those in the literature (Vilas et al., 2014; Torres Palenzuela et al., 2019; Caballero et al., 2020). Much of the current development of EWS is centered around in situ regulatory monitoring data of harmful species and their toxins at aquaculture sites. These data are designed to be used for operational decisions on whether a harvesting area should be closed to protect human health and are therefore not designed to provide the early warning needed to manage aquaculture business risk. Environmental data comes from different sources, ranging from programs that monitor the state of the ocean to numerical models of ocean physics and biology. Combining approaches, often involving huge volumes of data with very different spatial and temporal resolution into information systems to support end-users therefore poses significant technological challenges.
When it comes to ascertaining the best fit model approach the choice of model is driven by the species of concern and the topography of each region. For example, in Scotland, the type 2 particle tracking model using is based on an unstructured grid FVCOM system. This was chosen because of the complex fjordic nature of the Scottish coastline and hence the need to be able to track the advective movement of HAB events at high spatial resolution.
Particle tracking models (ROMS, FVCOM, and MOHID) are among the simplest models, since they consider only physical components and hence are suitable for many toxic HABs that are known to be driven by advection processes. However, these models cannot be applied to all toxigenic species and information is required to know where and when the HAB event starts to initiate the model. At present, this is achieved by a combination of remote sensing and coastal monitoring, but neither are ideal for optimal early warning. Coastal monitoring can provide limited early warning, where satellite methods appear to be able to distinguish and differentiate between only a few (high biomass) species, and cloud cover can limit their operability. Combining with the additional approach of machine learning, this can integrate all this model information together with other sources of data (e.g., in situ observations or other models from Copernicus services) and result in a probabilistic forecast with the information and data available. The example of the Basque probabilistic model presented above, highlights that with enough data integration over 80% accuracy can be reached with almost no false positives. However, extension of this proof-of-concept will require a level of data gathering in near real time, which is not yet realistic, given the difficulties to identify the target species (D. acuminata) species in situ.
Lagrangian models play a central role in many current HAB early warning systems, as they provide information on the oceanographic transport of potentially toxic blooms, once identified by in situ observation. While the most probable origin of the blooms can potentially be determined by inverse drift computation (Abascal et al., 2012), in a similar fashion to that used in other oceanographic disciplines (Breivik et al., 2012; Drévillon et al., 2013; Suneel et al., 2016; Chen, 2019) this is not currently operational. Hence, initiation of Lagrangian model predictions of advective bloom transport requires information on the location and size of a potentially harmful bloom before it has reached the shellfishery.
One cost-effective method of obtaining this information is through satellite ocean color imagery, which can provide coverage of large marine areas at a resolution of a few hundred meters. Unfortunately, cloud cover obscures data collection and hence is their primary limitation. Satellite monitoring offers the potential to identify an advective bloom before it reaches the coast. This approach focuses mainly on the detection of chlorophyll and hence is suitable for detecting large blooms of phytoplankton at the water surface (Spyrakos et al., 2011). Satellite-based approaches increasingly use machine learning methods for discriminating certain dense HABs from harmless blooms (Velo-Suárez and Gutiérrez-Estrada, 2007; Gokaraju et al., 2011; Xu et al., 2014; Guallar et al., 2016). However, large blooms of phytoplankton do not necessarily lead to toxic events, and others, such as the dinoflagellate Dinophysis spp., responsible for DSP, can cause harm at concentrations of only a few hundred or thousand cells per liter, far too low to be observable from space (Broullón et al., 2020). This is common to many shellfish toxin producing species of Alexandrium, Dinophysis and Azadinium genus which do not produce the main biomass component of a bloom that a satellite image can detect. Satellites can also provide useful information on sea surface temperature (SST) highlighting the onset and extension of an upwelling event, a process often linked to the triggering of a toxic bloom.
Model initiation would therefore benefit from remote sensing approaches capable of better discriminating harmful from benign species, and a more holistic approach to combine data from multiple sources, for example using machine learning. This study shows the potential of combining satellite, modeled, laboratory, and in situ data (e.g., Basque Country example). It also shows that an effective early warning system needs to consider a complex array of parameters including the presence or absence of toxins, the presence or absence of specific phytoplankton species, oceanographic dispersal models, satellite data models and biogeochemical and meteorological data or model forecasts. The use of such models could be extended to estimate the origin of blooms, not just to establish potential pathways of dispersion or retention.
New data gathering methodologies that are currently under development to enhance our ability to identify blooms offshore, including enhanced remote sensing approaches, autonomous observation platforms and data assimilation techniques will be key to enhancing the model skill.
Of course, the further ahead we look the less reliable these forecasts become. Currently the Irish and Scottish forecasts predict the occurrence of HABs up to a week ahead. Given that official control monitoring takes place weekly, this is usually sufficient to prevent intoxicated shellfish being harvested. Trend analysis of previous years can also provide some information on the seasonal occurrence of toxin-producing phytoplankton, but this is generally limited by significant inter-annual variation. As a comparison, advances in meteorological forecasting have been slow, increasing in duration by a day each decade (Alley et al., 2019), and now give a reasonably accurate forecast up to 2 weeks in advance. The marine ecosystem that controls the growth of toxic phytoplankton is every bit as complex. This creates a quandary: the aquaculture industry wants long term forecasts, though this will lead to more false positives, and forecasting a toxic event that does not happen will reduce industry confidence in the process. At the moment, model systems are still a long way from being able to produce long-term predictions for the industry and rely instead on expert opinion to draw together the various data streams needed to produce a meaningful forecast.
How Can the Aquaculture Industry Use HAB Early Warning Systems?
Early warning systems offers several potential benefits to the industry. In the case of a possible closure, they allow shellfish farmers to source product from alternative, healthy sites, an important factor in maintaining important market supply chains. It also gives industry the opportunity to implement mitigation strategies such as erecting physical barriers and increasing the frequency of end product testing (EPT), to verify the presence of toxins in their shellfish. Giving farmers the ability to make better management decisions over harvesting and planned husbandry work minimizes costly product recall and reduces human health incidents, both of which have long-lasting detrimental effects on consumer confidence.
Future Considerations for Early Warning Systems
Looking ahead, the integration of multiple observational platforms at the right scale combined with real-time processing capacity is required to unlock the potential of forecasting systems. The term big data was coined to capture this emerging trend (Hu et al., 2014). In addition to its sheer volume, big data exhibits other unique characteristics when compared with traditional data. The need for real-time storage, processing and visualization is crucial for an effective system beyond previous proofs-of-concept. This development calls for new system architectures for data acquisition, transmission, storage, and large-scale data processing mechanisms from computer science (LeCun et al., 2015). Big data techniques enhanced by machine learning methods can increase the value of such data and its applicability to societal, industrial and management challenges. Such methods have proven their potential in fisheries forecasting (Fernandes et al., 2010) and automatic classification of zooplankton samples (Fernandes et al., 2009). The Probabilistic Graphical Models (PGMs) paradigm (Pearl, 1988; Castillo et al., 1997), based on probability theory and graph theory (Buntine, 1991), is such a tool. Machine learning and statistical methods have a wide literature of model validation and performance estimation that is needed for end-user trust and interpretation of model forecasts (Fernandes et al., 2010; Witten et al., 2017). To take these forecasts further a multi-disciplinary approach joining domain experts and artificial intelligence experts is key (Grosjean et al., 2004; Fernandes et al., 2012, 2013; Trifonova et al., 2015; Uusitalo et al., 2016; Hernández-González et al., 2019; Taconet et al., 2019; Cruz et al., 2021).
Data consolidation is a major challenge in developing a machine learning based system. As noted above, data sources may include in situ regulatory monitoring, sensors, meteorology, models and satellite: often provided in different formats, undocumented or with missing information. EWS developments will accelerate and reach their full potential only with FAIR data: findability, accessibility, interoperability, and reusability (Wilkinson et al., 2016; Bax et al., 2019; Stall et al., 2019). These curated datasets incorporate training information and so provide an opportunity to improve the efficiency and strength of statistical models. This method is already employed for essential biodiversity variables (EBVs), to study global biodiversity (Kissling et al., 2018), and to collect essential ocean variables (EOVs, Miloslavich et al., 2018) needed to monitor the ocean. Each owner and provider of data related to HABs should also consider contributing their data to these frameworks, as international networks such as HAEDAT currently focus on harmful events (Figure 2), rather than the distribution of toxin-producing algal species.
Climate change will also bring increased toxin threats from species such as Gymnodinium catenatum and Azadinium spp. (Higman et al., 2013; Turner et al., 2015), and from other toxin-producing organisms currently present elsewhere in Europe and in similar environments worldwide (Davidson et al., 2015). Evidence on climate change impacts on HABs have been described by Edwards et al. (2006) who noted shifts in the distribution of HABs in the North-East Atlantic since the 1960s. Links between increasing sea surface temperatures and wind intensity have led to an increase in the potentially toxic diatom Pseudo-nitzschia in the NEA since the mid-1990s (Hinder et al., 2012). Climate change effect on the timing and severity of toxic events is still uncertain (Wells et al., 2019) with model predictions and in situ data not always coinciding (Dees et al., 2017; Gobler et al., 2017).
As we have seen, the shellfish aquaculture industry differs between and even within countries in the NEA: hydrography may be very different; toxic species may change as may the toxicity of a particular species; and there is a great deal of inter-annual and seasonal variation. This creates a challenge when developing models which must be adapted and fitted to these divergent conditions. Over the past decade EWS have been implemented with varying degrees of success in different countries. Some, such as those in Ireland and Scotland are fully functional while others are still in the developmental proof of concept and trial stage. This heterogeneity means the overall value of these EWS systems to industry, while, regionally important, is currently low but rapidly increasing as cross region cooperation and method sharing increases.
Author Contributions
JF-S, KD, MS, MR, WS, DC, MR-V, and LF contributed to the initial idea, data analysis, and the manuscript writing. PM, RF, AS, MiM, and JS contributed to the initial idea and manuscript writing. PA, LM, CW, McM, and PN contributed to the data analysis and manuscript writing. All authors contributed to the article and approved the submitted version.
Funding
Interreg Atlantic Area Programme Project PRIMROSE (Grant No. EAPA_182/2016, https://www.shellfish-safety.eu/). The national monitoring program of bivalve molluscs in Portugal is funded by project SNMB—MONITOR (Monitor-16.02.01- FEAMP-0043): Competitiveness and sustainable development for the shellfish sector of mainland Portugal co-financed by the Portuguese Government, Operational Program (OP) March 2020, Portugal 2020 and European Union through the European Structural Funds and Investment Funds (FEEI) and European Maritime and Fisheries Fund (EMFF), AS acknowledges funding from IPMA fellowship (IPMA-BCC-2016-35). This study was partially supported by the project EGRECOST CALIDAD – Control de Calidad de Aguas Cultivos Marinos (Departamento de Desarrollo Económico e Infraestructuras del Gobierno Vasco) and by the UKRI projects CAMPUS (Grant No. NE/R00675X/1) and Off-Aqua (Grant No. BB/S004246/1). This study was partially supported by the European H2020 project FutureMARES (Grant No. 869300).
Conflict of Interest
The authors declare that the research was conducted in the absence of any commercial or financial relationships that could be construed as a potential conflict of interest.
The reviewer EB declared a past co-authorship with the authors to the handling editor.
Acknowledgments
IOC-ICES-PICES HAEDAT maps courtesy of Pieter Provoost, IOC project office for IODE, Ostend, Belgium. The authors thank three reviewers for their constructive comments that have improved the manuscript. This is contribution number 1037 from the Marine Research Division (AZTI-BRTA).
Supplementary Material
The Supplementary Material for this article can be found online at: https://www.frontiersin.org/articles/10.3389/fmars.2021.666583/full#supplementary-material
Footnotes
- ^ Monitoring of Toxin-producing Phytoplankton in Bivalve Mollusc Harvesting Areas; Guide to Good Practice: Technical Application; and, EU Working Group on Toxin-producing Phytoplankton Monitoring in Bivalve Mollusc Harvesting Areas.
- ^ http://mandeo.meteogalicia.es/thredds/catalogos/ROMS/catalog.html
References
Abascal, A. J., Castanedo, S., Fernández, V., and Medina, R. (2012). Backtracking drifting objects using surface currents from high-frequency (HF) radar technology. Ocean Dyn. 62, 1073–1089. doi: 10.1007/s10236-012-0546-4
Aleynik, D., Dale, A. C., Porter, M., and Davidson, K. (2016). A high resolution hydrodynamic model system suitable for novel harmful algal bloom modelling in areas of complex coastline and topography. Harmful Algae 53, 102–117.
Alley, R. B., Emanuel, K. A., and Zhang, F. (2019). Advances in weather prediction. Science 363:342.
Anderson, C. R., Siegel, D. A., Brzezinski, M. A., and Guillocheau, N. (2008). Controls on temporal patterns in phytoplankton community structure in the Santa Barbara Channel, California. J. Geophys. Res. 113:C04038. doi: 10.1029/2007JC004321
Anderson, D. M. (1998). Physiology and bloom dynamics of toxic Alexandrium species, with emphasis on life cycle transitions. Nato Asi Ser. G Ecol. Sci. 41, 29–48.
Aoki, K., Onitsuka, G., Shimizu, M., Kuroda, H., Matsuyama, Y., Kimoto, K., et al. (2012). Factors controlling the spatio-temporal distribution of the 2009 Chattonella antiqua bloom in the Yatsushiro Sea, Japan. Estuar. Coast. Shelf Sci. 114, 148–155. doi: 10.1016/j.ecss.2012.08.028
Avdelas, L., Avdic−Mravlje, E., Borges Marques, A. C., Cano, S., Capelle, J. J., Carvalho, N., et al. (2021). The decline of mussel aquaculture in the European Union: causes, economic impacts and opportunities. Rev. Aquac. 13, 91–118. doi: 10.1111/raq.12465
Barraquand, F., Picoche, C., Maurer, D., Carassou, L., and Auby, I. (2018). Coastal phytoplankton community dynamics and coexistence driven by intragroup density-dependence, light and hydrodynamics. Oikos Nordic Ecol. Soc. 127, 1834–1852. doi: 10.1111/oik.05361
Bates, S. S., Bird, C. J., de Freitas, A. S. W., Foxall, R., Gilgan, M., Hanic, L. A., et al. (1989). Pennate diatom Nitzschia pungens as the primary source of momoic acid, a toxin in shellfish from Eastern Prince Edward Island, Canada. Can. J. Fish. Aquat. Sci. 46, 1203–1215. doi: 10.1139/f89-156
Batifoulier, F., Lazure, P., Velo-Suarez, L., Maurer, D., Bonneton, P., Charria, G., et al. (2013). Distribution of Dinophysis species in the Bay of Biscay and possible transport pathways to Arcachon Bay. J. Mar. Syst. 109, S273–S283. doi: 10.1016/j.jmarsys.2011.12.007
Bax, N. J., Miloslavich, P., Muller-Karger, F. E., Allain, V., Appeltans, W., Batten, S. D., et al. (2019). A response to scientific and societal needs for marine biological observations. Front. Mar. Sci. 6:395. doi: 10.3389/fmars.2019.00395
Berdalet, E., Fleming, L. E., Gowen, R., Davidson, K., Hess, P., Backer, L. C., et al. (2016). Marine harmful algal blooms, human health and wellbeing: challenges and opportunities in the 21st century. J. Mar. Biol. Assoc. 96, 61–91. doi: 10.1017/S0025315415001733
Berdalet, E., Montresor, M., Reguera, B., Roy, S., Yamazaki, H., Cembella, A., et al. (2017). Harmful algal blooms in fjords, coastal embayments, and stratified systems: recent progress and future research. Oceanography 30, 46–57. doi: 10.5670/oceanog.2017.109
Billett, D. S. M., Lampitt, R. S., and Mantoura, R. F. C. (1983). Seasonal sedimentation of phytoplankton to the deep-sea benthos. Nature 302, 520–522. doi: 10.1038/302520a0
Botelho, M. J., Vale, C., and Ferreira, J. G. (2019). Seasonal and multi-annual trends of bivalve toxicity by PSTs in Portuguese marine waters. Sci. Total Environ. 664, 1095–1106. doi: 10.1016/j.scitotenv.2019.01.314
Bravo, I., Fraga, S., Figueroa, R. I., Pazos, Y., Massanet, A., and Ramilo, I. (2010). Bloom dynamics and life cycle strategies of two toxic dinoflagellates in a coastal upwelling system (NW Iberian Peninsula). Deep Sea Res. Part II Top. Stud. Oceanogr. 57, 222–234. doi: 10.1016/j.dsr2.2009.09.004
Breivik, Ø, Bekkvik, T. C., Wettre, C., and Ommundsen, A. (2012). BAKTRAK: backtracking drifting objects using an iterative algorithm with a forward trajectory model. Ocean Dyn. 62, 239–252. doi: 10.1007/s10236-011-0496-2
Bresnan, E., Arévalo, F., Belin, C., Branco, M. A., Cembella, A. D., Clarke, D., et al. (2021). Diversity and regional distribution of harmful algal events along the Atlantic margin of Europe. Harmful Algae 102:101976. doi: 10.1016/j.hal.2021.101976
Broullón, E., López-Mozos, M., Reguera, B., Chouciño, P., Doval, M. D., Fernández-Castro, B., et al. (2020). Thin layers of phytoplankton and harmful algae events in a coastal upwelling system. Prog. Oceanogr. 189:102449. doi: 10.1016/j.pocean.2020.102449
Brown, L., Bresnan, E., Graham, J., Lacaze, J. P., Turrell, E., and Collins, C. (2010). Distribution, diversity and toxin composition of the genus Alexandrium (Dinophyceae) in Scottish waters. Eur. J. Phycol. 45, 375–393. doi: 10.1080/09670262.2010.495164
Buntine, W. (1991). “Theory refinement on Bayesian networks”, in Uncertainty Proceedings, Elsevier, 52–60. doi: 10.1016/B978-1-55860-203-8.50010-3
Caballero, A., Ferrer, L., Rubio, A., Charria, G., Taylor, B. H., and Grima, N. (2014). Monitoring of a quasi-stationary eddy in the Bay of Biscay by means of satellite, in situ and model results. Deep Sea Res. Part II 106, 23–37. doi: 10.1016/j.dsr2.2013.09.029
Caballero, I., Fernández, R., Escalante, O. M., Mamán, L., and Navarro, G. (2020). New capabilities of Sentinel-2A/B satellites combined with in situ data for monitoring small harmful algal blooms in complex coastal waters. Sci. Rep. 10, 1–14. doi: 10.1038/s41598-020-65600-1
Castillo, E., Gutierrez, J., and Hadi, A. (1997). Expert Systems and Probabilistic Network Models. New York, NY: Springer Verlag.
Chambouvet, A., Morin, P., Marie, D., and Guillou, L. (2008). Control of toxic marine dinoflagellate blooms by serial parasitic killers. Science 322, 1254–1257. doi: 10.1126/science.1164387
Chapelle, A. (2016). Modélisation du Phytoplancton Dans Les Écosystèmes Côtiers. Application À L’eutrophisation et Aux Proliférations D’algues Toxiques. Brest: Université de Bretagne Occidentale.
Chapelle, A., Le Gac, M. L. C., Siano, R., Quere, J., Caradec, F., Le Bec, C., et al. (2015). The Bay of Brest (France), a new risky site for toxic Alexandrium minutum blooms and PSP shellfish contamination. Harmful Algae News 51, 4–5.
Charria, G., Repecaud, M., Quemener, L., Menesguen, A., Rimmelin Maury, P., et al. (2014). PREVIMER: a contribution to in situ coastal observing systems. Mercator Ocean Q. Newslett. 49, 9–20.
Chen, H. (2019). Performance of a simple backtracking method for marine oil source searching in a 3D 101 ocean. Mar. Pollut. Bull. 142, 321–334. doi: 10.1016/j.marpolbul.2019.03.045
Clarke, D. (2020). “New insights and perspectives from 20 years of monitoring algal events in Irish coastal waters,” in Proceedings of the 11th Irish Shellfish Safety Workshop, Marine Environment and Health Series No. 41, eds D. Clarke and M. Gilmartin (Galway: Marine Institute).
Coates, L., Swan, S., Davidson, K., Turner, A., Maskrey, B., and Algoet, M. (2018). Annual Report on the Results of the Biotoxin and Phytoplankton Official Control Monitoring Programmes for Scotland - 2017. Lowestoft: Cefas.
Costa, P. R., and Garrido, S. (2004). Domoic acid accumulation in the sardine Sardina pilchardus and its relationship to Pseudo-nitzschia diatom ingestion. Mar. Ecol. Prog. Ser. 284, 261–268. doi: 10.3354/meps284261
Cruz, R. C., Reis Costa, P., Vinga, S., Krippahl, L., and Lopes, M. B. (2021). A Review of recent machine learning advances for forecasting harmful algal blooms and shellfish contamination. J. Mar. Sci. Eng. 9:283. doi: 10.3390/jmse9030283
Cusack, C., Dabrowski, T., Lyons, K., Berry, A., Westbrook, G., Salas, R., et al. (2016). Harmful algal bloom forecast system for SW Ireland. Part II: are operational oceanographic models useful in a HAB warning system. Harmful Algae 53, 86–101. doi: 10.1016/j.hal.2015.11.013
Dabrowski, T., Lyons, K., Nolan, G., Berry, A., Cusack, C., and Silke, J. (2016). Harmful algal bloom forecast system for SW Ireland. Part I: description and validation of an operational forecasting model. Harmful Algae 53, 64–76. doi: 10.1016/j.hal.2015.11.015
Davidson, K., Anderson, D. M., Mateus, M., Reguera, B., Silke, J., Sourisseau, M., et al. (2016). Forecasting the risk of harmful algal blooms: preface to the Asimuth special issue. Harmful Algae 53, 1–7. doi: 10.1016/j.hal.2015.11.005
Davidson, K., Baker, C., Higgins, C., Higman, W., Swan, S., Veszelovszki, A., et al. (2015). Potential threats posed by new or emerging marine biotoxins in UK waters and examination of detection methodologies used for their control: cyclic imines. Mar. Drugs 13, 7087–7112. doi: 10.3390/md13127057
Davidson, K., Tett, P., and Gowen, R. J. (2011). “Harmful algal blooms,” in Marine Pollution & Human Health, eds R. M. Harrison and R. E. Hester (Cambridge: RSC publishing), 95–127.
Davidson, K., Whyte, C., Aleynik, D., Kurekin, A., Gontarek, S., McNeill, S., et al. (2021). HABreports: online early warning of harmful algal and biotoxin risk for the Scottish shellfish and finfish aquaculture industries. Front. Mar. Sci. 8:631732. doi: 10.3389/fmars.2021.631732
Debreu, L., Marchesiello, P., Penven, P., and Cambon, G. (2012). Two-way nesting in split-explicit ocean models: algorithms, implementation and validation. Ocean Model. 49–50, 1–21. doi: 10.1016/j.ocemod.2012.03.003
Dees, P., Bresnan, E., Dale, A. C., Edwards, M., Johns, D., Mouat, B., et al. (2017). Harmful algal blooms in the Eastern North Atlantic ocean. Proc. Natl. Acad. Sci. U.S.A. 114, E9763–E9764. doi: 10.1073/pnas.1715499114
Defra (2019). MAGIC Interactive Mapping Tool: Geographic Information About the Natural Environment From Across Government. London: Defra.
Delmas, D., Herbland, A., and Maestrini, S. Y. (1993). “Do Dinophysis spp. come from the “open sea” along the French Atlantic coast?,” in Toxic Phytoplankton Blooms in the Sea, eds T. Smayda and Y. Shimizu (Amsterdam: Elsevier Science), 489–494.
Dhanji-Rapkova, M., O’Neill, A., Maskrey, B. H., Coates, L., Swan, S. C., Alves, M. T., et al. (2019). Variability and profiles of lipophilic toxins in bivalves from Great Britain during five and a half years of monitoring: azaspiracids and yessotoxins. Harmful Algae 87:101629. doi: 10.1016/j.hal.2019.101629
Díaz, P. A., Reguera, B., Moita, T., Bravo, I., Ruiz-Villarreal, M., and Fraga, S. (2019). Mesoscale dynamics and niche segregation of two Dinophysis species in Galician-Portuguese coastal waters. Toxins 11:37.
Díaz, P. A., Reguera, B., Ruiz-Villarreal, M., Pazos, Y., Velo-Suarez, L., Berger, H., et al. (2013). Climate variability and oceanographic settings associated with interannual variability in the initiation of Dinophysis acuminata blooms. Mar. Drugs 11, 2964–2981. doi: 10.3390/md11082964
Díaz, P. A., Ruiz-Villarreal, M., Pazos, Y., Moita, T., and Reguera, B. (2016). Climate variability and Dinophysis acuta blooms in an upwelling system. Harmful Algae 53, 145–159. doi: 10.1016/j.hal.2015.11.007
Dippner, J. W., Nguyen-Ngoc, L., Doan-Nhu, N., and Subramaniam, A. (2011). A model for the prediction of harmful algae blooms in the Vietnamese upwelling area. Harmful Algae 10, 606–611. doi: 10.1016/j.hal.2011.04.012
Drakulović, D., Gvozdenović, S., Joksimović, D., Mandić, M., and Pestorić, B. (2017). Toxic and potentially toxic phytoplankton in the mussel and fish farms in the transitional area of Montenegrin Coast (South-Eastern Adriatic Sea). Turkish J. Fish. Aqu. Sci. 17, 885–900. doi: 10.4194/1303-2712-v17_5_05
Drévillon, M., Greiner, E., Paradis, D., Payan, C., Lellouche, J.-M., Reffray, G., et al. (2013). A strategy for producing refined currents in the Equatorial Atlantic in the context of the search of the AF447 wreckage. Ocean Dyn. 63, 63–82. doi: 10.1007/s10236-012-0580-2
Edwards, M., Johns, D. G., Leterme, S. C., Svendsen, E., and Richardson, A. J. (2006). Regional climate change and harmful algal blooms in the northeast Atlantic. Limnol. Oceanogr. 51, 820–829. doi: 10.4319/lo.2006.51.2.0820
EFSA (2008). Opinion of the scienti fi c panel on contaminants in the food chain on a request from the European commission on marine biotoxins in shell fish–azaspiracids. EFSA J. 723, 1–52. doi: 10.2903/j.efsa.2009.907
Ellis, T., Gardiner, R., Gubbins, M., Reese, A., and Smith, D. (2015). Aquaculture Statistics for the UK, with a Focus on England and Wales: 2012. Lowestoft: Cefas, 18.
Egbert, G. D., and Erofeeva, S. Y. (2002). Efficient inverse modeling of barotropic ocean tides. J. Atmos. Oceanic Technol. 19, 183–204. doi: 10.1175/1520-0426(2002)019<0183:EIMOBO>2.0.CO;2
En 15204 (2006). Water Quality - Guidance Standard on the Enumeration of Phytoplankton Using Inverted Microscopy (Utermöhl technique). Brussels: European Committee for Standardization.
FAO (2018). The State of World Fisheries and Aquaculture 2018. Meeting the Sustainable Development Goals. Rome: Food and Agriculture Organization of the United Nations.
FAO (2019). FAO Yearbook. Fishery and Aquaculture Statistics 2017/FAO Annuaire. Statistiques des Pêches et De l’aquaculture 2017/FAO Anuario. Estadísticas de Pesca y Acuicultura 2017. Rome: Food and Agriculture Organization of the United Nations.
Farrell, H., Gentien, P., Fernand, L., Lunven, M., Reguera, B., González-Gil, S., et al. (2012). Scales characterising a high density thin layer of Dinophysis acuta Ehrenberg and its transport within a coastal jet. Harmful Algae 15, 36–46. doi: 10.1016/j.hal.2011.11.003
Fehling, J., Davidson, K., Bolch, C. J., and Tett, P. (2006). Seasonality of Pseudo-nitzschia spp. (Bacillariophyceae) in western Scottish waters. Mar. Ecol. Prog. Ser. 323, 91–105. doi: 10.3354/meps323091
Fehling, J., Davidson, K., Bolch, C. J., Brand, T., and Narayanaswamy, B. E. (2012). The relationship between phytoplankton distribution and water column characteristics in North West European Shelf Sea Waters. PLoS One 7:e34098. doi: 10.1371/journal.pone.0034098
Fernandes, J. A., Irigoien, X., Boyra, G., Lozano, J. A., and Inza, I. (2009). Optimizing the number of classes in automated zooplankton classification. J. Plankt. Res. 31, 19–29. doi: 10.1093/plankt/fbn098
Fernandes, J. A., Irigoien, X., Goikoetxea, N., Lozano, J. A., Inza, I., Pérez, A., et al. (2010). Fish recruitment prediction, using robust supervised classification methods. Ecol. Model. 221, 338–352. doi: 10.1016/j.ecolmodel.2009.09.020
Fernandes, J. A., Irigoien, X., Lozano, J. A., Inza, I., Goikoetxea, N., and Pérez, A. (2015). Evaluating machine-learning techniques for recruitment forecasting of seven North East Atlantic fish species. Ecol. Inform. 25, 35–42. doi: 10.1016/j.ecoinf.2014.11.004
Fernandes, J. A., Kauppila, P., Uusitalo, L., Fleming-Lehtinen, V., Kuikka, S., and Pitkänen, H. (2012). Evaluation of reaching the targets of the water framework directive in the Gulf of Finland. Environ. Sci. Technol. 46, 8220–8228. doi: 10.1021/es300126b
Fernandes, J. A., Lozano, J. A., Inza, I., Irigoien, X., Pérez, A., and Rodríguez, J. D. (2013). Supervised pre-processing approaches in multiple class variables classification for fish recruitment forecasting. Environ. Model. Softw. 40, 245–254. doi: 10.1016/j.envsoft.2012.10.001
Fernández, R., Mamán, L., Jaén, D., Fernández Fuentes, L., Ocaña, M. A., and Gordillo, M. M. (2019). Dinophysis species and diarrhetic shellfish toxins: 20 years of monitoring program in Andalusia, South of Spain. Toxins 11:189. doi: 10.3390/toxins11040189
Ferrer, L., and Caballero, A. (2011). Eddies in the Bay of Biscay: a numerical approximation. J. Mar. Syst. 87, 133–144. doi: 10.1016/j.jmarsys.2011.03.008
Ferrer, L., Fontán, A., Chust, G., Mader, J., González, M., Valencia, V., et al. (2009). Low-salinity plumes in the oceanic region of the Basque Country. Cont. Shelf Res. 29, 970–984.
Ferrer, L., González, M., Valencia, V., Mader, J., Fontán, A., Uriarte, A. D., et al. (2007). “Operational coastal systems in the Basque Country region: modelling and observations,” in Proceedings 17th International Offshore (Ocean) and Polar Eng. Conference, eds J. S. Chung, M. Kashiwagi, I J. Losada, and L.-K. Chien (Lisbon: ISOPE), 1736–1743.
Ferrer, L., Zaldua-Mendizabal, N., Del Campo, A., Franco, J., Mader, J., Cotano, U., et al. (2015). Operational protocol for the sighting and tracking of Portuguese man-of-war in the southeastern Bay of Biscay: observations and modelling. Cont. Shelf Res. 95, 39–53. doi: 10.1016/j.csr.2014.12.011
Ferrer, L., Zaldua-Mendizabal, N., Del Campo, A., Franco, J., Mader, J., Cotano, U., et al. (2013). Protocolo operacional para el avistamiento y seguimiento del cnidario Physalia physalis (carabela portuguesa) en el sureste del golfo de Bizkaia. Rev. Invest. Mar. 20, 88–102.
García, C. M., Prieto, L., Vargas, M., Echevarría, F., García-Lafuente, J., Ruiz, J., et al. (2002). Hydrodynamics and the spatial distribution of plankton and TEP in the Gulf of Cadiz (SW Iberian Peninsula). J. Plankt. Res. 24, 817–833. doi: 10.1093/plankt/24.8.817
Gentien, P., Lunven, M., Lazure, P., Youenou, A., and Crassous, M. P. (2007). Motility and Autotoxicity in Karenia Mikimotoi (Dinophyceae). Philos. Trans. R. Soc. B Biol. Sci. 362, 1937–1946. doi: 10.1098/rstb.2007.2079
Gillibrand, P. A., Siemering, B., Miller, P. I., and Davidson, K. (2016). Individual-based modelling of the development and transport of a Karenia mikimotoi bloom on the North-West European continental shelf. Harmful Algae 53, 118–134. doi: 10.1016/j.hal.2015.11.011
Glibert, P. M., Wilkerson, F. P., Dugdale, R. C., Parker, A. E., Alexander, J., Blaser, S., et al. (2014). Phytoplankton communities from San Francisco Bay Delta respond differently to oxidized and reduced nitrogen substrates—even under conditions that would otherwise suggest nitrogen sufficiency. Front. Mar. Sci. 1:17. doi: 10.3389/fmars.2014.00017
Gobler, C. J., Doherty, O. M., Hattenrath-Lehmann, T. K., Griffith, A. W., Kang, Y., and Litaker, R. W. (2017). Ocean warming since 1982 has expanded the niche of toxic algal blooms in the North Atlantic and North Pacific oceans. Proc. Natl. Acad. Sci. U.S.A. 114, 4975–4980. doi: 10.1073/pnas.1619575114
Godinho, L., Silva, A., Branco, M. A. C., Marques, A., and Costa, P. R. (2018). Evaluation of intracellular and extracellular domoic acid content in Pseudo-nitzschia multiseries cell cultures under different light regimes. Toxicon 155, 27–31. doi: 10.1016/j.toxicon.2018.10.003
Gokaraju, B., Durbha, S. S., King, R. L., and Younan, N. H. (2011). A machine learning based spatio-temporal data mining approach for detection of harmful algal blooms in the Gulf of Mexico. IEEE J. Select. Top. Appl. Earth Observ. Remote Sens. 4, 710–720. doi: 10.1109/JSTARS.2010.2103927
Gowen, R. J., Tett, P., Bresnan, E., Davidson, K., and McKinney, A. (2012). Anthropogenic nutrient enrichment and blooms of harmful phytoplankton. Oceanogr. Mar. Biol. 50, 65–126. doi: 10.1201/b12157-3
Grosjean, P., Picheral, M., Warembourg, C., and Gorsky, G. (2004). Enumeration, measurement, and identification of net zooplankton samples using the ZOOSCAN digital imaging system. ICES J. Mar. Sci. 61, 518–525. doi: 10.1016/j.icesjms.2004.03.012
Grzebyk, D., Audic, S., Lasserre, B., Abadie, E., de Vargas, C., and Bec, B. (2017). Insights into the harmful algal flora in northwestern Mediterranean coastal lagoons revealed by pyrosequencing metabarcodes of the 28S rRNA gene. Harmful Algae 68, 1–16. doi: 10.1016/j.hal.2017.06.003
Guallar, C., Bacher, C., and Chapelle, A. (2017). Global and local factors driving the phenology of Alexandrium minutum (Halim) blooms and its toxicity. Harmful Algae 67, 44–60. doi: 10.1016/j.hal.2017.05.005
Guallar, C., Delgado, M., Diogene, J., and Fernandez-Tejedor, M. (2016). Artificial neural network approach to population dynamics of harmful algal blooms in Alfacs Bay (NW Mediterranean): case studies of Karlodinium and Pseudo-nitzschia. Ecol. Model. 338, 37–50. doi: 10.1016/j.ecolmodel.2016.07.009
Haidvogel, D. B., Arango, H. G., Hedström, K., Beckmann, A., Malanotte-Rizzoli, P., and Shchepetkin, A. F. (2000). Model evaluation experiments in the North Atlantic Basin: simulations in nonlinear terrain-following coordinates. Dyn. Atmos. Oceans 32, 239–281. doi: 10.1016/S0377-0265(00)00049-X
Hall, M. A. (1999). Correlation-Based Feature Selection for Machine Learning. Dissertation thesis. Waikato: The University of Waikato.
Hall, M. A. (2000). “Correlation-based feature selection of discrete and numeric class machine learning,” in Proceedings of the Seventeenth International Conference on Machine Learning (Stanford, CA: Stanford University), 359–366.
Hall, M. A., and Smith, L. A. (1997). “Feature subset selection: a correlation based filter approach,” in Proceedings of the International Conference on Neural Information Processing and Intelligent Information Systems (Berlin: Springer), 855–858.
Hallegraeff, G. M. (2003). “Harmful algal blooms: a global overview,” in Manual on Harmful Marine Microalgae, eds G. Hallegraeff, D. Anderson, A. Cembella, and H. Enevoldsen (Paris: IOC Manuals and Guides UNESCO), 1–22.
Hambrey, J., and Evans, S. (2016). Aquaculture in England, Wales and Northern Ireland: An Analysis of the Economic Contribution and Value of the Major Sub-sectors and the Most Important Farmed Species. Report SR694. Edinburgh: Sea Fish Industry Authority, 162.
Hernandez Farinas, T., Bacher, C., Soudant, D., Belin, C., and Barille, L. (2015). Assessing phytoplankton realized niches using a French national phytoplankton monitoring network. Estuar. Coast. Shelf Sci. 159, 15–27. doi: 10.1016/j.ecss.2015.03.010
Hernández-González, J., Inza, I., Granado, I., Basurko, O. C., Fernandes, J. A., and Lozano, J. A. (2019). Aggregated outputs by linear models: an application on marine litter beaching prediction. Inform. Sci. 481, 381–393. doi: 10.1016/j.ins.2018.12.083
Higman, W., Turner, A., Baker, C., Higgins, C., Veszelovszki, A., and Davidson, K. (2013). Research to Support the Development of a Monitoring Programme for New or Emerging Marine Biotoxins in Shellfish in UK waters. London: Report to the Food Standards Agency, 437.
Hinder, S. L., Hays, G. C., Edwards, M., Roberts, E. C., Walne, A. W., and Gravenor, M. B. (2012). Changes in marine dinoflagellate and diatom abundance under climate change. Nat. Clim. Change 2, 271–275. doi: 10.1038/nclimate1388
Hu, H., Wen, Y., Chua, T. S., and Li, X. (2014). Toward scalable systems for big data analytics: a technology tutorial. IEEE Access 2, 652–687. doi: 10.1109/ACCESS.2014.2332453
Huisman, J., and Weissing, F. J. (1999). Biodiversity of plankton by species oscillations and chaos. Nature 402, 407–410. doi: 10.1038/46540
Husson, B., Hernández-Fariñas, T., Le Gendre, R., Schapira, M., and Chapelle, A. (2016). Two decades of Pseudo-nitzschia spp. blooms and king scallop (Pecten maximus) contamination by domoic acid along the French Atlantic and English channel coasts: seasonal dynamics, spatial heterogeneity and interannual variability. Harmful Algae 51, 26–39. doi: 10.1016/j.hal.2015.10.017
Hutchins, D. A., and Bruland, K. W. (1998). Iron-limited diatom growth and Si:N uptake ratios in a coastal upwelling regime. Nature 393, 561–564. doi: 10.1038/31203
Irigoien, X., Huisman, J., and Harris, R. P. (2004). Global biodiversity patterns of marine phytoplankton and zooplankton. Nature 429, 863–867. doi: 10.1038/nature02593
Juang, H. M. H. (2000). The NCEP mesoscale spectral model: a revised version of the nonhydrostatic regional spectral model. Mon. Weather Rev. 128, 2329–2362. doi: 10.1175/1520-0493(2000)128<2329:TNMSMA>2.0.CO;2
Karasiewicz, S., Breton, E., Lefebvre, A., Hernandez Farinas, T., and Lefebvre, S. (2018). Realized niche analysis of phytoplankton communities involving HAB: Phaeocystis spp. as a case study. Harmful Algae 72, 1–13. doi: 10.1016/j.hal.2017.12.005
Karlson, B., Andersen, P., Arneborg, L., Cembella, A., Eikrem, W., John, U., et al. (2021). Harmful algal blooms and their effects in coastal seas of Northern Europe. Harmful Algae 102:101989. doi: 10.1016/j.hal.2021.101989
Kissling, W. D., Ahumada, J. A., Bowser, A., Fernandez, M., Fernández, N., García, E. A., et al. (2018). Building essential biodiversity variables (EBV s) of species distribution and abundance at a global scale. Biol. Rev. 93, 600–625. doi: 10.1111/brv.12359
Kurekin, A. A., Miller, P. I., and Van der Woerd, H. J. (2014). Satellite discrimination of Karenia mikimotoi and Phaeocystis harmful algal blooms in European coastal waters: Merged classification of ocean colour data. Harmful Algae 31, 163–176. doi: 10.1016/j.hal.2013.11.003
Laiz, I., Ferrer, L., Plomaritis, T. A., and Charria, G. (2014). Effect of river runoff on sea level from in-situ measurements and numerical models in the Bay of Biscay. Deep Sea Res. Part II 106, 49–67. doi: 10.1016/j.dsr2.2013.12.013
Landsberg, J. H. (2002). The effects of harmful algal blooms on aquatic organisms. Rev. Fish. Sci. 10, 113–390. doi: 10.1080/20026491051695
Le Gac, M., Metegnier, G., Chomérat, N., Malestroit, P., Quéré, J., Bouchez, O., et al. (2016). Evolutionary processes and cellular functions underlying divergence in Alexandrium minutum. Mol. Ecol. 25, 5129–5143.
Leadbetter, A., Silke, J., and Cusack, C. (2018). Creating a Weekly Harmful Algal Bloom Bulletin. Galway: Marine Institute, 63.
LeBihan, V., Guillotreau, B., Morineau, B., and Pardo, S. (2019). “The impact of shellfish trade bans caused by Harmful Algal Blooms (HABs) on a french regional economy: an input-output approach,” in Proceedings of the Oceanext-Interdisciplinary Conference, Nantes.
LeCun, Y., Bengio, Y., and Hinton, G. (2015). Deep learning. Nature 521, 436–444. doi: 10.1038/nature14539
Legorburu, I., Ferrer, L., Galparsoro, I., and Larreta, J. (2015). Distribution of river-borne particulate Pb in the Basque continental shelf (Bay of Biscay). Environ. Earth Sci. 74, 4261–4279. doi: 10.1007/s12665-015-4495-3
Lelong, A., Hégaret, H., Soudant, P., and Bates, S. S. (2012). Pseudo-nitzschia (Bacillariophyceae) species, domoic acid and amnesic shellfish poisoning: revisiting previous paradigms. Phycologia 51, 168–216. doi: 10.2216/11-37.1
Lett, C., Verley, P., Mullon, C., Parada, C., Brochier, T., Penven, P., et al. (2008). A Lagrangian tool for modelling ichthyoplankton dynamics. Environ. Modell. Softw. 23, 1210–1214. doi: 10.1016/j.envsoft.2008.02.005
Lewis, A. M., Coates, L. N., Turner, A. D., Percy, L., and Lewis, J. (2018). A review of the global distribution of Alexandrium minutum (Dinophyceae) and comments on ecology and associated paralytic shellfish toxin profiles, with a focus on Northern Europe. J. Phycol. 54, 581–598. doi: 10.1111/jpy.12768
Lindahl, O. (1986). “A dividable hose for phytoplankton sampling,” in Proceedings of the International Council for the Exploration of the Sea Report of the Working Group on Exceptional Algal Blooms, Copenhagen.
Magdalena, A. B., Lehane, M., Krys, S., Fernández, M. L., Furey, A., and James, K. J. (2003). The first identification of azaspiracids in shellfish from France and Spain. Toxicon 42, 105–108. doi: 10.1016/S0041-0101(03)00105-3
Maguire, J., Cusack, C., Ruiz-Villarreal, M., Silke, J., McElligott, D., and Davidson, K. (2016). Applied simulations and integrated modelling for the understanding of toxic and harmful algal blooms (ASIMUTH): integrated HAB forecast systems for Europe’s Atlantic Arc. Harmful Algae 53, 160–166. doi: 10.1016/j.hal.2015.11.006
Mardones, J. I., Holland, D. S., Anderson, L., Le Bihan, V., Gianella, F., Clément, A., et al. (2020). Estimating and Mitigating the Economic Costs of Harmful Algal Blooms on Commercial and Recreational Shellfish Harvesters. Belgium: GlobalHAB, 66.
Martens, H., Tillmann, U., Harju, K., Dell’Aversano, C., Tartaglione, L., and Krock, B. (2017). Toxin variability estimations of 68 Alexandrium ostenfeldii (Dinophyceae) strains from The Netherlands reveal a novel abundant gymnodimine. Microorganisms 5:29. doi: 10.3390/microorganisms5020029
Martino, S., Gianella, F., and Davidson, K. (2020). An approach for evaluating the economic impacts of harmful algal blooms: the effects of blooms of toxic Dinophysis spp. on the productivity of Scottish shellfish farms. Harmful Algae 99:101912. doi: 10.1016/j.hal.2020.101912
Mateus, M., Fernandes, J., Revilla, M., Ferrer, L., Villarreal, M. R., Miller, P., et al. (2019). “Early warning systems for shellfish safety: the pivotal role of computational science,” in Proceedings of the International Conference on Computational Science (Cham: Springer), 361–375.
Mateus, M., Riflet, G., Chambel, P. C., Fernandes, L., Fernandes, R., Juliano, M., et al. (2012). An operational model for the West Iberian coast: products and services. Ocean Sci. 8, 713–732. doi: 10.5194/os-8-713-2012
McCabe, R. M., Hickey, B. M., Kudela, R. M., Lefebvre, K. A., Adams, N. G., Bill, B. D., et al. (2016). An unprecedented coastwide toxic algal bloom linked to anomalous ocean conditions. Geophys. Res. Lett. 43, 10366–10376. doi: 10.1002/2016GL070023
Mellor, G. L., and Yamada, T. (1982). Development of a turbulence closure model for geophysical fluid problems. Rev. Geophys. 20, 851–875. doi: 10.1029/RG020i004p00851
Ménesguen, A., Dussauze, M., Lecornu, F., Dumas, F., and Thouvenin, B. (2014). Operatonial modelling of nutrients and phytoplankton in the Bay of Biscay and english channel. Mercator Ocean Q. Newslett. 49, 87–93.
Míguez, A., Fernlindez, L., and Fraga, S. (1996). “First detection of domoic acid in Galicia (NW of Spain)”, in Harmful Toxic Algal Blooms, eds T. Yasumoto, Y. Oshima, and Y. Fukuyo, Paris, 143–145.
Miller, P. I., Kurekin, A., Evers-King, H., Lockett, J., Davidson, K.-M., and Calder-Potts, R., et al. (in press). Satellite monitoring and modelling for early warning of water quality risks to shellfish farms. Bull. Am. Meteorol. Soc.
Miloslavich, P., Bax, N. J., Simmons, S. E., Klein, E., Appeltans, W., Aburto−Oropeza, O., et al. (2018). Essential ocean variables for global sustained observations of biodiversity and ecosystem changes. Glob. Change Biol. 24, 2416–2433. doi: 10.1111/gcb.14108
Moestrup, Ø, Akselman, R., Cronberg, G., Elbraechter, M., Fraga, S., Halim, Y., et al. (2009). IOC-UNESCO Taxonomic Reference List of Harmful Micro Algae. Available online at: http://www.marinespecies.org/hab (accessed January 27, 2021).
Moita, M. T., Oliveira, P. B., Mendes, J., and Palma, A. (2003). Distribution of Chlorophyll a and Gymnodinium catenatum associated with coastal upwelling plumes off central Portugal. Acta Oecol. 24, 125–132. doi: 10.1016/S1146-609X(03)00011-0
Moita, M. T., Pazos, Y., Rocha, C., Nolasco, R., and Oliveira, P. B. (2016). Toward predicting Dinophysis blooms off NW Iberia: a decade of events. Harmful Algae 53, 17–32. doi: 10.1016/j.jbusres.2014.02.013
Moita, M. T., Sobrinho-Gonçalves, L., Oliveira, P. B., Palma, S., and Falcao, M. (2006). A bloom of Dinophysis acuta in a thin layer off north-west Portugal. Afr. J. Mar. Sci. 28, 265–269. doi: 10.2989/18142320609504160
Mousavi, S., and Gigerenzer, G. (2014). Risk, uncertainty, and heuristics. J. Bus. Res. 67, 1671–1678.
Muñiz, O., Revilla, M., Rodríguez, J. G., Laza-Martínez, A., Seoane, S., Franco, J., et al. (2017). Evaluation of phytoplankton quality and toxicity risk based on a long-term time series previous to the implementation of a bivalve farm (Basque coast as a case study). Reg. Stud. Mar. Sci. 10, 10–19. doi: 10.1016/j.rsma.2016.12.012
Munro, L. A., and Wallace, I. S. (2018). Scottish Shellfish Farm Production Survey 2017. Aberdeen: Marine Scotland Science.
Navarro, G., and Ruiz, J. (2006). Spatial and temporal variability of phytoplankton in the Gulf of Cadiz through remote sensing images. Deep Sea Res. Part II 53, 1241–1260. doi: 10.1016/j.dsr2.2006.04.014
Navarro, G., Caballero, I., Prieto, L., Vázquez, A., Flecha, S., Huertas, I. E., et al. (2012). Seasonal-to-interannual variability of Chlorophyll-a bloom timing associated with physical forcing in the Gulf of Cádiz. Adv. Space Res. 50, 1164–1172. doi: 10.1016/j.asr.2011.11.034
Nezan, E., Chomerat, N., Bilien, G., Boulben, S., Duval, A., and Ryckaert, M. (2010). Pseudo-nitzschia australis on French Atlantic coast-an unusual toxic bloom. Harmful Algae News 41, 1–2.
Palma, S., Mouriño, H., Silva, A., Barão, M. I., and Moita, M. T. (2010). Can Pseudo-nitzschia blooms be modeled by coastal upwelling in Lisbon Bay? Harmful Algae 9, 294–303. doi: 10.1016/j.hal.2009.11.006
Pan, Y., Bates, S. S., and Cembella, A. (1998). Environmental stress and domoic acid production by Pseudo-nitzschia: a physiological perspective. Nat. Toxins 6, 127–135. doi: 10.1002/(SICI)1522-7189(199805/08)6:3/43.0.CO
Paterson, R. F. (2017). Investigating the Distribution, Seasonal Dynamics and Toxicity of Azadinium spinosum in Scottish waters using qPCR. Dissertation thesis. Aberdeen: The University of Aberdeen.
Paterson, R. F., McNeill, S., Mitchell, E., Adams, T., Swan, S. C., Clarke, D., et al. (2017). Environmental control of harmful dinoflagellates and diatoms in a fjordic system. Harmful Algae 69, 1–17. doi: 10.1016/j.hal.2017.09.002
Pazos, Y., Moroño, A., Triñanes, J., Doval, M., Montero, P., and Vilarinho, M. G. (2006). “Early detection and intensive monitoring during an unusual toxic bloom of Gymnodinium catenatum advected into the Galician Rías (NW, Spain),” in Proceedings of the 12th International Conference on HABs, Copenhagen.
Pearl, J. (1988). Probabilistic Reasoning in Intelligence Systems: Networks of Plausible Inference. San Francisco, CA: Morgan Kaufmann Publishers Inc.
Pinto, L., Mateus, M., and Silva, A. (2016). Modeling the transport pathways of harmful algal blooms in the Iberian coast. Harmful Algae 53, 8–16. doi: 10.1016/j.hal.2015.12.001
Pitcher, G. C., Figueiras, F. G., Hickey, B. M., and Moita, M. T. (2010). The physical oceanography of upwelling systems and the development of harmful algal blooms. Prog. Oceanogr. 85, 5–32. doi: 10.1016/j.pocean.2010.02.002
Prieto, L., Navarro, G., Cózar, A., Echevarria, F., and García, C. M. (2006). Distribution of TEP in the euphotic and upper mesopelagic zones of the southern Iberian coasts. Deep Sea Res. Part II Top. Stud. Oceanogr. 53, 1314–1328. doi: 10.1016/j.dsr2.2006.03.009
Prieto, L., Navarro, G., Rodríguez-Gálvez, S., Huertas, I. E., Naranjo, J. M., and Ruiz, J. (2009). Oceanographic and meteorological forcing of the pelagic ecosystem on the Gulf of Cadiz shelf (SW Iberian Peninsula). Cont. Shelf Res. 29, 2122–2137. doi: 10.1016/j.csr.2009.08.007
Raine, R. (2014). A review of the biophysical interactions relevant to the promotion of HABs in stratified systems: the case study of Ireland. Deep Sea Res. Part II Top. Stud. Oceanogr. 101, 21–31. doi: 10.1016/j.dsr2.2013.06.021
Raine, R., Cosgrove, S., Fennell, S., Gregory, C., Bernett, M., Purdie, D., et al. (2016). “Origins of Dinophysis blooms which impact Irish aquaculture,” in Proceedings of the 17th International Conference of Harmful Algae, eds L. A. O. Proença and G. M. Hallegraeff (Florianópolis: International Society for the Study of Harmful Algae), 46–49.
Rathaille, A. N., and Raine, R. (2011). Seasonality in the excystment of Alexandrium minutum and Alexandrium tamarense in Irish coastal waters. Harmful Algae 10, 629–635. doi: 10.1016/j.hal.2011.04.015
Reguera, B., Riobó, P., Rodríguez, F., Díaz, P. A., Pizarro, G., Paz, B., et al. (2014). Dinophysis toxins: causative organisms, distribution and fate in shellfish. Mar. Drugs 12, 394–461. doi: 10.3390/md12010394
Reguera, B., Velo-Suárez, L., Raine, R., and Park, M. G. (2012). Harmful Dinophysis species: a review. Harmful Algae 14, 87–106. doi: 10.1016/j.hal.2011.10.016
Revilla, M., Borja, A., Fontán, A., Franco, J., Manuel, G., Valencia, V., et al. (2010). Phytoplankton biomass and temperature trends in offshore waters of the Basque country. Globec Int. Newslett. 10:12.
Rodríguez-Rodríguez, G., Villasante, S., and García-Negro, M. C. (2011). Are red tides affecting economically the commercialization of the Galician (NW Spain) mussel farming? Mar. Policy 35, 252–257. doi: 10.1016/j.marpol.2010.08.008
Rowland-Pilgrim, S., Swan, S. C., O’Neill, A., Johnson, S., Coates, L., Stubbs, P., et al. (2019). Variability of amnesic shellfish toxin and Pseudo-nitzschia occurrence in bivalve molluscs and water samples–analysis of ten years of the official control monitoring programme. Harmful Algae 87:101623. doi: 10.1016/j.hal.2019.101623
Ruiz-Villarreal, M., García-García, L. M., Cobas, M., Díaz, P. A., and Reguera, B. (2016). Modelling the hydrodynamic conditions associated with Dinophysis blooms in Galicia (NW Spain). Harmful Algae 53, 40–52. doi: 10.1016/j.hal.2015.12.003
Salas, R., and Clarke, D. (2019). Review of DSP toxicity in Ireland: long-term trend impacts, biodiversity and toxin profiles from a monitoring perspective. Toxins 11:61. doi: 10.3390/toxins11020061
Sanseverino, I., Conduto, D., Pozzoli, L., Dobricic, S., and Lettieri, T. (2016). Algal Bloom and its Economic Impact. Luxembourg: European Union.
Santos, M., Costa, P. R., Porteiro, F. M., and Moita, M. T. (2014). First report of a massive bloom of Alexandrium minutum (Dinophyceae) in middle North Atlantic: a coastal lagoon in S. Jorge Island, Azores. Toxicon 90, 265–268. doi: 10.1016/j.toxicon.2014.08.065
Schmidt, W., Evers-King, H. L., Campos, C. J. A., Jones, D. B., Miller, P. I., Davidson, K., et al. (2018). A generic approach for the development of short-term predictions of Escherichia coli and biotoxins in shellfish. Aquac. Environ. Interact. 10, 173–185. doi: 10.3354/aei00265
Schnetzer, A., Jones, B. H., Schaffner, R. A., Cetinic, I., Fitzpatrick, E., Miller, P. E., et al. (2013). Coastal upwelling linked to toxic Pseudo-nitzschia australis blooms in Los Angeles coastal waters, 2005–2007. J. Plankt. Res. 35, 1080–1092. doi: 10.1093/plankt/fbt051
Shchepetkin, A. F., and McWilliams, J. C. (2005). The regional oceanic modeling system (ROMS): a split-explicit, free-surface, topography-following-coordinate oceanic model. Ocean Model. 9, 347–404. doi: 10.1016/j.ocemod.2004.08.002
Siemering, B., Bresnan, E., Painter, S. C., Daniels, C. J., Inall, M., and Davidson, K. (2016). Phytoplankton distribution in relation to environmental drivers on the NorthWest European Shelf Sea. PLoS One 11:e0164482. doi: 10.1371/journal.pone.0164482
Silva, A., Pinto, L., Rodrigues, S. M., de Pablo, H., Santos, M., Moita, T., et al. (2016). A HAB warning system for shellfish harvesting in Portugal. Harmful Algae 53, 33–39. doi: 10.1016/j.hal.2015.11.017
Simpson, J. H., Edelsten, D. J., Edwards, A., Morris, N. C. G., and Tett, P. B. (1979). The Islay front: physical structure and phytoplankton distribution. Estuar. Coast. Mar. Sci. 9:713. doi: 10.1016/S0302-3524(79)80005-5
Sison-Mangus, M., Jiang, S., Tran, K., and Kudela, R. M. (2014). Host-specific adaptation governs the interaction of the marine diatom. Pseudo-nitzschia and their microbiota. ISME J. 8, 63–76. doi: 10.1038/ismej.2013.138
Skamarock, W. C., and Klemp, J. B. (2008). A time-split nonhydrostatic atmospheric model for weather research and forecasting applications. J. Comput. Phys. 227, 3465–3485. doi: 10.1016/j.jcp.2007.01.037
Smagorinsky, J. (1963). General circulation experiments with the primitive equations: I. The basic experiment. Mon. Weather Rev. 91, 99–164. doi: 10.1175/1520-0493(1963)091<0099:GCEWTP>2.3.CO;2
Smayda, T. J. (1990). “Novel and nuisance phytoplankton blooms in the sea: evidence for a global epidemic,” in Toxic Marine Phytoplankton, eds E. Granéli, B. Sundstrøm, L. Edler, and D. M. Anderson (Amsterdam: Elsevier), 29–40.
Smith, M. E., Robertson Lain, L., and Bernard, S. (2018). An optimized Chlorophyll a switching algorithm for MERIS and OLCI in phytoplankton-dominated waters. Remote Sens. Environ. 215, 217–227. doi: 10.1016/j.rse.2018.06.002
Song, Y. T., and Haidvogel, D. B. (1994). A semi-implicit ocean circulation model using a generalized topography-following coordinate system. J. Comp. Phys. 115, 228–244. doi: 10.1006/jcph.1994.1189
Sopanen, S., Setälä, O., Piiparinen, J., Erler, K., and Kremp, A. (2011). The toxic dinoflagellate Alexandrium ostenfeldii promotes incapacitation of the calanoid copepods Eurytemora affinis and Acartia bifilosa from the northern Baltic Sea. J. Plankt. Res. 33, 1564–1573. doi: 10.1093/plankt/fbr052
Sourisseau, M., Jegou, K., Lunven, M., Quere, J., Gohin, F., and Bryere, P. (2016). Distribution and dynamics of two species of Dynophiceae producing high biomass blooms over the French Atlantic Shelf. Harmful Algae 53, 53–63. doi: 10.1016/j.hal.2015.11.016
Sourisseau, M., Le Guennec, V., Le Gland, G., Plus, M., and Chapelle, A. (2017). Resource competition affects plankton community structure; evidence from trait-based modeling. Front. Mar. Sci. 4:52. doi: 10.3389/fmars.2017.00052
Spyrakos, E., Vilas, L. G., Palenzuela, J. M. T., and Barton, E. D. (2011). Remote sensing chlorophyll a of optically complex waters (rias Baixas, NW Spain): application of a regionally specific chlorophyll a algorithm for MERIS full resolution data during an upwelling cycle. Remote Sens. Environ. 115, 2471–2485. doi: 10.1016/j.rse.2011.05.008
Stall, S., Yarmey, L., Cutcher-Gershenfeld, J., Hanson, B., Lernhert, K., Nosek, B., et al. (2019). Make scientific data FAIR’. Nature 570, 27–29. doi: 10.1038/d41586-019-01720-7
Stumpf, R. P., Culver, M. E., Tester, P. A., Tomlinson, M., Kirkpatrick, G. J., Pederson, B. A., et al. (2003). Monitoring Karenia brevis blooms in the Gulf of Mexico using satellite ocean color imagery and other data. Harmful Algae 2, 147–160. doi: 10.1016/S1568-9883(02)00083-5
Sun, C.-C., Wang, Y.-S., Wu, M.-L., Dong, J.-D., Wang, Y.-T., Sun, F.-L., et al. (2011). Seasonal variation of water quality and phytoplankton response patterns in Daya Bay, China. Int. J. Environ. Res. Public Health 8, 2951–2966. doi: 10.3390/ijerph8072951
Sunda, W. G., Price, N. M., and Morel, F. M. M. (2005). “Trace metal ion buffers and their use in culture studies,” in Algal Culturing Techniques, ed. R. A. Anderson (Cambridge, MA: Elsevier Academic Press), 35–63.
Suneel, V., Ciappa, A., and Vethamony, P. (2016). Backtrack modeling to locate the origin of tar balls depositing along the west coast of India. Sci. Total Environ. 569–570, 31–39. doi: 10.1016/j.scitotenv.2016.06
Sverdrup, H. U. (1953). On conditions for the vernal blooming of phytoplankton. ICES J. Mar. Sci. 18, 287–295. doi: 10.1093/icesjms/18.3.287
Swan, S. C., Turner, A. D., Bresnan, E., Whyte, C., Paterson, R. F., McNeill, S., et al. (2018). Dinophysis acuta in Scottish Waters and its influence on diarrhetic shellfish toxin profiles. Toxins 10:399. doi: 10.3390/toxins10100399
Swan, S., and Davidson, K. (2012). Monitoring Programme for the Presence of Toxin Producing Plankton in Shellfish Production Areas in Scotland. Scotland: Food Standards Agency.
Taconet, M., Kroodsma, D., and Fernandes, J. A. (2019). Global Atlas of AIS-based fishing activity - Challenges and opportunities. Rome: FAO.
Tatters, A. O., Fu, F. X., and Hutchins, D. A. (2012). High CO2 and silicate limitation synergistically increase the toxicity of Pseudo-nitzschia fraudulenta. PLoS One 7:e32116. doi: 10.1371/journal.pone.0032116
Theodorou, J. A., Moutopoulos, D. K., and Tzovenis, I. (2020). Semi-quantitative risk assessment of Mediterranean mussel (Mytilus galloprovincialis L.) harvesting bans due to harmful algal bloom (HAB) incidents in Greece. Aquac. Econ. Manag. 24, 273–293. doi: 10.1080/13657305.2019.1708994
Thorel, M., Claquin, P., Schapira, M., Le Gendre, R., Riou, P., Goux, D., et al. (2017). Nutrient ratios influence variability in Pseudo-nitzschia species diversity and particulate domoic acid production in the Bay of Seine (France). Harmful Algae 68, 192–205. doi: 10.1016/j.hal.2017.07.005
Tillmann, U., Jaén, D., Fernández, L., Gottschling, M., Witt, M., Blanco, J., et al. (2017). Amphidoma languida (Amphidomatacea, Dinophyceae) with a novel azaspiracid toxin profile identified as the cause of molluscan contamination at the Atlantic coast of southern Spain. Harmful Algae 62, 113–126. doi: 10.1016/j.hal.2016.12.001
Tomlinson, M. C., Stumpf, R. P., Ransibrahmanakul, V., Truby, E. W., Kirkpatrick, G. J., Pederson, B. A., et al. (2004). Evaluation of the use of SeaWiFS imagery for detecting Karenia brevis harmful algal blooms in the eastern Gulf of Mexico. Remote Sens. Environ. 91, 293–303. doi: 10.1016/j.rse.2004.02.014
Torres Palenzuela, J. M., González Vilas, L., Bellas, F. M., Garet, E., and González-Fernández, Á, et al. (2019). Pseudo-nitzschia blooms in a coastal upwelling system: Remote sensing detection, toxicity and environmental variables. Water 11:1954. doi: 10.3390/w11091954
Touzet, N., Davidson, K., Pete, R., Flanagan, K., McCoy, G. R., Amzil, Z., et al. (2010). Co-occurrence of the West European (Gr. III) and North American (Gr. I) ribotypes of Alexandrium tamarense (Dinophyceae) in Shetland, Scotland. Protist 161, 370–384. doi: 10.1016/j.protis.2009.12.001
Touzet, N., Franco, J. M., and Raine, R. (2007). ‘Characterization of nontoxic and toxin-producing strains of Alexandrium minutum (Dinophyceae) in Irish coastal waters’. Appl. Environ. Microbiol. 73, 3333–3342. doi: 10.1128/AEM.02161-06
Trainer, V. L., Pitcher, G. C., Reguera, B., and Smayda, T. J. (2010). The distribution and impacts of harmful algal bloom species in eastern boundary upwelling systems. Prog. Oceanogr. 85, 33–52. doi: 10.1016/j.pocean.2010.02.003
Trifonova, N., Kenny, A., Maxwell, D., Duplisea, D., Fernandes, J., and Tucker, A. (2015). Spatio-temporal Bayesian network models with latent variables for revealing trophic dynamics and functional networks in fisheries ecology. Ecol. Inform. 30, 142–158. doi: 10.1016/j.ecoinf.2015.10.003
Turner, A., Higgins, C., Veszelovski, A., Payne, D., Davidson, K., Hungerford, J., et al. (2015). Monitoring of new or emerging marine biotoxins in UK waters: Brevetoxins. Mar. Drugs 13, 1224–1254. doi: 10.3390/md13031224
Uncles, R. G., Clark, J. R., Bedington, M., and Torres, R. (2020). “On sediment dispersal in the Whitsand Bay marine conservation zone: neighbour to a closed dredge-spoil disposal site,” in Marine Protected Areas: Evidence, Policy, and Practise, eds R. Clark and J. Humphreys (Amsterdam: Elsevier Inc.), 599–569.
Utermöhl, H. (1958). Zur Vervollkommnung der quantitativen Phytoplankton-Methodik. Mitt. int. Verein. Theor. Angew. Limnol. 9, 1–38.
Uusitalo, L., Fernandes, J. A., Bachiller, E., Tasala, S., and Lehtiniemi, M. (2016). Semi-automated classification method addressing marine strategy framework directive (MSFD) zooplankton indicators. Ecol. Indic. 71, 398–405. doi: 10.1016/j.ecolind.2016.05.036
Vale, P., Botelho, M. J., Rodrigues, S. M., Gomes, S. S., and Sampayo, M. A. M. (2008). Two decades of marine biotoxin monitoring in bivalves from Portugal (1986–2006): a review of exposure assessment. Harmful Algae 7, 11–25. doi: 10.1016/j.hal.2007.05.002
Vanhoutte-Brunier, A., Fernand, L., Ménesguen, A., Lyons, S., Gohin, F., and Philippe, C. (2008). Modelling the Karenia mikimotoi bloom that occurred in the western english channel during summer 2003. Ecol. Model. 210, 351–376. doi: 10.1016/j.ecolmodel.2007.08.025
Vasquez, M., Mata Chacón, D., Tempera, F., O’Keeffe, E., Galparsoro, I., Sanz Alonso, J. L., et al. (2015). Broad-scale mapping of seafloor habitats in the north-east Atlantic using existing environmental data. J. Sea Res. 100, 120–132. doi: 10.1016/j.seares.2014.09.011
Velo-Suárez, L., and Gutiérrez-Estrada, J. C. (2007). Artificial neural network approaches to one-step weekly prediction of Dinophysis acuminata blooms in Huelva (Western Andalucía, Spain). Harmful Algae 6, 361–371. doi: 10.1016/j.hal.2006.11.002
Velo-Suarez, L., Gonzalez-Gil, S., Gentien, P., Lunven, M., Bechemin, C., Fernand, L., et al. (2008). Thin layers of Pseudo-nitzschia spp. and the fate of Dinophysis acuminata during an upwelling–downwelling cycle in a Galician Ria. Limnol. Oceanogr. 53:1816. doi: 10.4319/lo.2008.53.5.1816
Velo-Suárez, L., Reguera, B., González-Gil, S., Lunven, M., Lazure, P., Nézan, E., et al. (2010). Application of a 3D Lagrangian model to explain the decline of a Dinophysis acuminata bloom in the Bay of Biscay. J. Mar. Syst. 83, 242–252. doi: 10.1016/j.jmarsys.2010.05.011
Vilas, L. G., Spyrakos, E., Palenzuela, J. M. T., and Pazos, Y. (2014). Support Vector Machine-based method for predicting Pseudo-nitzschia spp. blooms in coastal waters (Galician rias, NW Spain). Prog. Oceanogr. 124, 66–77. doi: 10.1016/j.pocean.2014.03.003
Visciano, P., Schirone, M., Berti, M., Milandri, A., Tofalo, R., and Suzzi, G. (2016). Marine biotoxins: occurrence, toxicity, regulatory limits and reference methods. Front. Microbiol. 7:1051. doi: 10.3389/fmicb.2016.01051
Wang, D. Z. (2008). Neurotoxins from marine dinoflagellates: a brief review. Mar. Drugs 6, 349–371. doi: 10.3390/md20080016
Wells, M. L., Karlson, B., Wulff, A., Kudela, R., Trick, C., Asnaghi, V., et al. (2019). Future HAB science: Directions and challenges in a changing climate. Harmful Algae 91:101632. doi: 10.1016/j.hal.2019.101632
Whyte, C., Swan, S., and Davidson, K. (2014). Changing wind patterns linked to unusually high Dinophysis blooms around the coast of the Shetland Islands, Scotland. Harmful Algae 39, 365–373. doi: 10.1016/j.hal.2014.09.006
Wilkinson, M. D., Dumontier, M., Aalbersberg, I. J., Appleton, G., Axton, M., Baak, A., et al. (2016). The FAIR guiding principles for scientific data management and stewardship. Sci. Data 3:160018. doi: 10.1038/sdata.2016.18
Witten, I. H., Frank, E., Hall, M., and Pal, C. J. (2017). Data Mining: Practical Machine Learning Tools and Techniques With Java Implementations, 4th Edn. Burlington, MA: Morgan Kaufmann Series in Data Management Systems.
Wu, L., Chen, C., Guo, P., Shi, M., Qi, J., and Ge, J. (2011). A FVCOM-based unstructured grid wave, current, sediment transport model, I. Model description and validation. J. Ocean Univ. China 10, 1–8. doi: 10.1007/s11802-011-1788-3
Wyatt, T. (2014). Margalef’s mandala and phytoplankton bloom strategies. Deep Sea Res. Part II Top. Stud. Oceanogr. 101, 32–49. doi: 10.1016/j.dsr2.2012.12.006
Xu, X., Pan, D., Mao, Z., and Tao, B. (2014). A new algorithm based on the background field for red tide monitoring in the East China Sea. Acta Oceanol. Sin. 33, 62–71. doi: 10.1007/s13131-014-0404-y
Keywords: modeling, machine learning, toxins, phytoplankton, food production, short-term, regulation, early warning systems
Citation: Fernandes-Salvador JA, Davidson K, Sourisseau M, Revilla M, Schmidt W, Clarke D, Miller PI, Arce P, Fernández R, Maman L, Silva A, Whyte C, Mateo M, Neira P, Mateus M, Ruiz-Villarreal M, Ferrer L and Silke J (2021) Current Status of Forecasting Toxic Harmful Algae for the North-East Atlantic Shellfish Aquaculture Industry. Front. Mar. Sci. 8:666583. doi: 10.3389/fmars.2021.666583
Received: 10 February 2021; Accepted: 10 May 2021;
Published: 10 June 2021.
Edited by:
Jose Luis Iriarte, Austral University of Chile, ChileReviewed by:
Bernd Krock, Alfred Wegener Institute, Helmholtz Centre for Polar and Marine Research (AWI), GermanyEileen Bresnan, Marine Scotland, United Kingdom
Grant Colborne Pitcher, Department of Agriculture, Forestry and Fisheries, South Africa
Copyright © 2021 Fernandes-Salvador, Davidson, Sourisseau, Revilla, Schmidt, Clarke, Miller, Arce, Fernández, Maman, Silva, Whyte, Mateo, Neira, Mateus, Ruiz-Villarreal, Ferrer and Silke. This is an open-access article distributed under the terms of the Creative Commons Attribution License (CC BY). The use, distribution or reproduction in other forums is permitted, provided the original author(s) and the copyright owner(s) are credited and that the original publication in this journal is cited, in accordance with accepted academic practice. No use, distribution or reproduction is permitted which does not comply with these terms.
*Correspondence: Jose A. Fernandes-Salvador, jfernandes@azti.es