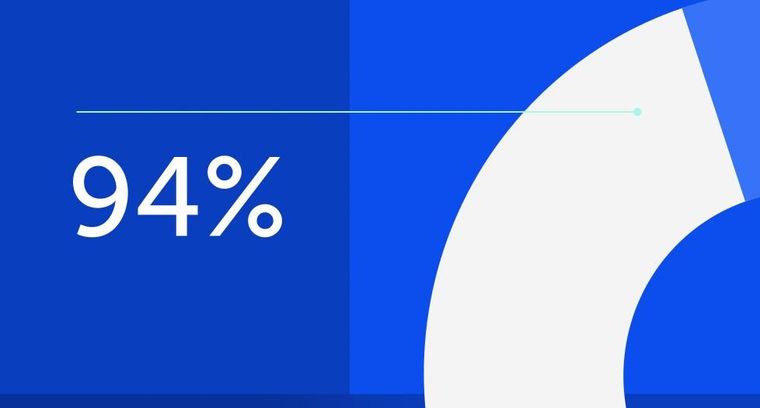
94% of researchers rate our articles as excellent or good
Learn more about the work of our research integrity team to safeguard the quality of each article we publish.
Find out more
ORIGINAL RESEARCH article
Front. Mar. Sci., 18 November 2021
Sec. Marine Ecosystem Ecology
Volume 8 - 2021 | https://doi.org/10.3389/fmars.2021.665474
This article is part of the Research TopicHabitat and Distribution Models of Marine and Estuarine Species: Advances for a Sustainable FutureView all 15 articles
Marine mammals have been proposed as ecosystem sentinels due to their conspicuous nature, wide ranging distribution, and capacity to respond to changes in ecosystem structure and functioning. In southern European Atlantic waters, their response to climate variability has been little explored, partly because of the inherent difficulty of investigating higher trophic levels and long lifespan animals. Here, we analyzed spatio-temporal patterns from 1994 to 2018 of one of the most abundant cetaceans in the area, the common dolphin (Delphinus delphis), in order to (1) explore changes in its abundance and distribution, and (2) identify the underlying drivers. For that, we estimated the density of the species and the center of gravity of its distribution in the Bay of Biscay (BoB) and tested the effect of three sets of potential drivers (climate indices, oceanographic conditions, and prey biomasses) with a Vector Autoregressive Spatio Temporal (VAST) model that accounts for changes in sampling effort resulting from the combination of multiple datasets. Our results showed that the common dolphin significantly increased in abundance in the BoB during the study period. These changes were best explained by climate indices such as the North Atlantic Oscillation (NAO) and by prey species biomass. Oceanographic variables such as chlorophyll a concentration and temperature were less useful or not related. In addition, we found high variability in the geographic center of gravity of the species within the study region, with shifts between the inner (southeast) and the outer (northwest) part of the BoB, although the majority of this variability could not be attributed to the drivers considered in the study. Overall, these findings indicate that considering temperature alone for projecting spatio-temporal patterns of highly mobile predators is insufficient in this region and suggest important influences from prey and climate indices that integrate multiple ecological influences. Further integration of existing observational datasets to understand the causes of past shifts will be important for making accurate projections into the future.
The global mean surface temperature has increased by approximately 1∘C from pre-industrial levels (IPCC, 2019), triggering shifts in the abundance, phenology and distribution of organisms worldwide (Parmesan and Yohe, 2003; Poloczanska et al., 2013). Marine ecosystems, despite having experienced a slower warming, show comparable or even greater shift rates and vulnerability than terrestrial systems (Burrows et al., 2011; Poloczanska et al., 2013; Pinsky et al., 2019), with seagrasses, corals, cephalopods and marine mammals exhibiting the most abrupt changes (Trisos et al., 2020).
Marine mammals, as wide ranging top predators, amplify trophic information across multiple spatiotemporal scales and can therefore act as sentinels of ecosystems’ responses to climate variability and change (Hazen et al., 2019). However, assessing climate change impacts in higher trophic levels and long lifespan animals such as marine mammals is challenging, as their relationships to climate may be non-linear and affected by time lags (Simmonds and Isaac, 2007; Barlow et al., 2021). In addition, identifying spatio-temporal trends in the context of climate change requires analyzing decadal or longer time series (Thorson et al., 2016), which are rarely available for marine mammal observation data.
Combining data from multiple sampling programs can help overcome this problem (Waggitt et al., 2020; Maureaud et al., 2021), but also increases the intrinsic variability related to observers’ skills, sampling design and protocols, which may result in confounding species range shifts with variations in the distribution and intensity of the sampling effort (Thorson et al., 2016). For that reason, separating the observation process from the true underlying spatial distribution is essential to accurately identify range shifts over time (Chust et al., 2014b) and to identify potential drivers (Erauskin-Extramiana et al., 2019b). Recently, a species distribution function (SDF) able to distinguish between sampling variation and true geographic variability has been developed (Thorson et al., 2016). Unlike conventional estimators such as the abundance-weighted average, the SDF is applied through a Vector Autoregressive Spatio Temporal (VAST) model that allows the estimation of species distribution over predicted locations rather than sampled locations, while also estimating a standard error that allows one to distinguish between sampling variation and significant variability (Thorson et al., 2016). Although model-based approaches had been used before to estimate shifts in the distribution of species, VAST typically involves estimating a Gaussian Markov random field (GMRF) representing latent variation in density that is constant over time (a “spatial” term), as well as a GMRF representing latent variation that changes among years (a “spatio-temporal” term), which is expected to improve predictions of species density and distribution compared with using only measured habitat variables (Thorson, 2019).
Until now, this estimator has been mainly applied to commercially important fish stocks (Godefroid et al., 2019; Perretti and Thorson, 2019; Xu et al., 2019), although the fragmented and methodologically variable nature of marine mammal observations suggest the method could be highly useful for analyzing the spatio-temporal patterns of marine megafauna too. Within that context, the Bay of Biscay (BoB hereafter), located in the Northeast Atlantic, off the coasts of France and Spain (Figure 1), represents an interesting study area since numerous marine mammal species (e.g., cetaceans) cohabit there, attracted by a highly diverse and abundant community of pelagic fish species (Astarloa et al., 2019; Louzao et al., 2019).
Figure 1. Spatial distribution of common dolphin sightings (displayed in segments of up to 10 km) over the BoB for the 1994–2018 period. Circle sizes are proportional to group size, while solid gray lines indicate the isobaths. Sightings in yellow represent the ferry data used to check model fit.
Such productivity and diversity, however, might be altered by climate change in the near future, as rising temperatures (0.26∘C per decade; Costoya et al., 2015) are expected to increase ocean stratification and reduce primary production and zooplankton biomass in the area (Chust et al., 2014a). In recent years, losses in fisheries production have already been reported (Free et al., 2019), together with changes in the composition, distribution, and phenology of fish species (Blanchard and Vandermeirsch, 2005; Chust et al., 2019; Baudron et al., 2020). Cetacean spatio-temporal variability, in contrast, has been mainly assessed by exploring changes in their relative abundance (Hemery et al., 2007; Castège et al., 2013; Authier et al., 2018), although both abundance and distribution are considered key criteria by the European Marine Strategy Framework Directive (MSFD; Directive 2008/56/EC) aiming to assess the environmental status of species and ecosystems in European Union waters.
Advancement of both MSFD criteria in this region is therefore necessary, especially when it is known that projections of climate change impacts on cetaceans at large spatial scales (e.g., global; MacLeod, 2009) do not always match with those at regional scales (Hazen et al., 2012). In the Northeast Atlantic, for example, warm-water cetaceans were predicted to expand poleward (MacLeod, 2009; Lambert et al., 2011, 2014), although the south-eastward shift detected for some Northeast Atlantic fish species in the BoB could indicate the opposite pattern in this particular area (Baudron et al., 2020). Indeed, some of the fish species (e.g., horse mackerel Trachurus trachurus, anchovy Engraulis encrasicolus, and sprat Sprattus sprattus) analyzed by Baudron et al. (2020) constitute an important food resource for many cetaceans in the BoB (Meynier et al., 2008;Spitz et al., 2018), which can heavily influence the spatial movements of their predators (Díaz López and Methion, 2019; Díaz López et al., 2019; Giralt Paradell et al., 2019).
The hypothesis that climate change may affect top predators through climate influences on their ectothermic prey has been often suggested (Robinson et al., 2005; Simmonds and Isaac, 2007; Evans and Waggitt, 2020). Most studies, however, examine environmental conditions (e.g., temperature) as proxies of prey distribution rather than studying prey data directly (Torres et al., 2008; Díaz López and Methion, 2019; Giralt Paradell et al., 2019) while others focus on exploring the effects of climate indices on the grounds that they act as an integrated measure of multiple variables (Hallett et al., 2004; Hemery et al., 2007). In the Northeast Atlantic, the North Atlantic Oscillation (NAO) is the dominant mode of climate variability, although additional climate indices such as the Atlantic Multidecadal Oscillation (AMO), the East Atlantic pattern (EA), or the South Biscay Climate (SBC) have been also found to exert strong influence, direct or indirectly, on both fish and cetacean species (Guisande et al., 2004; Hemery et al., 2007; Borja et al., 2008; Evans and Waggitt, 2020) through changes in ocean temperature and salinity, vertical mixing and circulation patterns (Drinkwater et al., 2003; Hurrell and Deser, 2009).
Given the multiple drivers potentially influencing cetacean spatio-temporal patterns, understanding the role of each of them is key for a better anticipating of future responses. For that reason, in this study we used a 25-year-long temporal series (1994–2018) to test the effect of prey biomasses, oceanographic conditions and climate indices on the abundance and distribution of the common dolphin (Delphinus delphis), one of the most abundant cetaceans inhabiting the BoB waters (Hammond et al., 2017). We used the VAST model (Thorson and Barnett, 2017) and the spatio-temporal species data compiled by Waggitt et al. (2020) to address two main research questions: (1) Has the abundance or the distribution of the common dolphin in the BoB experienced significant changes over the last two decades? (2) If so, are changes best explained by climatic, oceanographic, or prey variables? By answering these questions, this study intends to provide insights that will help understand past and future trends in the distribution and abundance of common dolphin in the BoB while contributing to the management for this species through the development of MSFD criteria in the context of climate change.
Cetacean data analyzed in this study, despite focusing on the BoB, belong to a large compilation made by Waggitt et al. (2020) that included observations collected on aerial and vessel (dedicated and opportunistic) surveys conducted in the Northeast Atlantic between 1980 and 2018. Although the data analyzed here (data providers in Supplementary Table 1) is a more updated version that includes higher-resolution tracklines (meaning that fewer data were omitted due to overlap with land-masses and more accurate measurements of distance traveled were obtained), the steps taken in the data processing and standardization stage were the same as in Waggitt et al. (2020), in which they (1) assessed differences in protocols by grouping data according to the (a) survey transect design (line transects, strip transects, and an intermediate method called ESAS, European Seabirds At Sea) and (b) the platform-type (vessel vs. aircraft) and (2) fitted detection functions using platform height and Beaufort sea-state as explanatory variables to estimate the proportion of animals missed by the observers (Marques and Buckland, 2004). They also assessed response bias (when animals react to the presence of the platform) through double-platform surveys that enabled the detection of animals before responsive movements. This correction was applicable to vessel surveys and is particularly relevant to common dolphins, which typically show a positive response to vessels (Cañadas et al., 2004). Finally, they calculated the effective strip half-width (ESW) which considers the decline in the detection probability as a function of distance and covariates and serves to estimate the area effectively covered (Area covered = ESW∗s∗L) when including the number of observation sides (s) and transect length (L). Full details can be found in Waggitt et al. (2020).
In order to match with the spatial resolution of the environmental data that we examined in later steps (see “Identification of Main Drivers” section), we divided larger transects into 10 km segments (García-Barón et al., 2019). Then, we examined the spatio-temporal coverage of surveys by summing the effort comprised in all segments per month and per year. In addition, we checked whether compiling data had led to a non-uniform distribution of sampling in space and time by exploring the annual latitudinal and longitudinal mean distributions and the corresponding linear regression trends.
Observations of common dolphin were analyzed by means of a spatio-temporal delta-generalized linear mixed model (delta-GLMM), referred to here as a VAST model (Thorson and Barnett, 2017) and available in R.1 This model is a flexible variant of the classical delta models that decompose density into two components (Stefánsson, 1996): (1) the probability of encountering the species at a given location and time; and (2) the expected density of the species when encountered. This two-part approach, also known as a hurdle model, helps combat statistical problems with zero-inflation and overdispersion in the original data (Martin et al., 2005) and is therefore suitable for use with cetacean survey data that usually show patchy distributions (Waggitt et al., 2020).
Another feature of the VAST model is that it decomposes spatio-temporal patterns in available point-count data into multiple additive components:
1. A temporal main effect (“intercepts”) representing changes in median abundance over time;
2. A spatial main effect (“spatial component”) representing the average spatial distribution during the modeled interval;
3. An interaction of space and time (“spatio-temporal component”) representing variation in distribution among years;
4. Density covariates, representing the impact of environmental conditions on expected density;
5. Catchability (a.k.a. detectability) covariates, representing the impact of environmental and/or sampling conditions on expected sampling data, but which do not reflect variation in population density and hence are “partialled out” prior to predicting densities.
Each of these components can be included in each of two linear predictors, and these two linear predictors are then transformed via inverse-link functions to predict the value of a response variable (in this case, dolphin samples). Spatial and spatio-temporal components are estimated as a Gaussian Markov random field (GMRF) and treated as a random effect. To improve computational speed, the value of these GMRFs is predicted at a fixed set of “knots” that defines a mesh of triangles that covers the entire modeled spatial domain. The value of the GMRF at any location within this domain is then predicted from the value of three knots surrounding that location. We use the stochastic partial different equation (SPDE) approximation to calculate the probability of GMRFs (Lindgren et al., 2011), and the projection from knots to locations is accomplished using bilinear interpolation as computed using R-INLA (Lindgren, 2012). The value of fixed effects are estimated using maximum likelihood techniques while integrating across the probability of random effects (Kristensen et al., 2016), and standard errors are calculated using a generalization of the delta method (Tierney et al., 1989). For further details, please see the VAST user manual.2
In our case, we treated year as a fixed effect (default VAST setting), such that there is no shrinkage in overall abundance across years. We modeled spatial and spatio-temporal variation as random effects to help account for multidimensional factors that are not included directly in the model but that can affect the density and distribution of the modeled species (Carroll et al., 2019). In particular, we estimated first-order autocorrelation among years in the spatio-temporal component, such that predicted hotspots in density decay slowly over time; this treatment allows spatio-temporal patterns to be predicted (with associated uncertainty) even in locations with sporadic sampling.
Detectability covariates were not considered here, because Beaufort sea-state and platform height were included in Waggitt et al. (2020). Density covariates were also omitted for our initial investigation of trends (but see “Identification of Main Drivers” section). As a response variable, the density of common dolphin was analyzed, after truncating the highest 5% to control outliers (Buckland et al., 2001). The spatio-temporal model was fitted assuming a lognormal error distribution and a Poisson-linked delta model such that the sum of both linear predictors is predicted log-density; this structure, was selected based on the lowest Akaike Information Criterion (AIC) (Sakamoto et al., 1986). Model parameters, as well as spatio-temporal components, were estimated using 200 knots (Supplementary Figure 1) based on previous studies that applied this same resolution in bigger areas (Carroll et al., 2019; Thorson, 2019), while confirming that results are qualitatively similar when increasing the number of knots (Supplementary Table 2). Species density was predicted at each knot by multiplying the predicted probability of occurrence by the predicted density. Density estimates for each knot were then interpolated to a standard grid of 0.1∘ spatial resolution (latitudinal range: 43∘–49∘N; longitudinal range: 1∘–10∘W) to match with the spatial resolution of the environmental data (see “Identification of Main Drivers” section) and multiplied by the area of the grid cell to create annual surfaces of common dolphin abundances across the BoB.
The annual abundances of common dolphin predicted for the study area were then analyzed by means of a linear regression to identify significant temporal trends and compared by means of a correlation test with an observed abundance index to check model fit. The observed abundance index was based on the encounter rate (individuals/km) of common dolphin estimated from monthly at-sea observations taken by a team of experienced observers in a constant effort-based systematic sampling scheme, i.e., the Pride of Bilbao ferry (Louzao et al., 2015; Robbins et al., 2020). This survey consistently crosses the BoB using the same route every year (Figure 1), and although it was also used as input for the baseline model, it only forms the 8% of the whole data set. Thus, we believe it can be used to compare the observed (ferry) and predicted (VAST) abundance indices and to determine whether the model predictions have been biased by differences in the effort.
An additional analysis with predicted abundances was also conducted to identify areas in which significant spatio-temporal changes occurred over the study period. For that, predicted abundances per grid cell were analyzed as a function of year by means of a linear regression. The slope and the p-value obtained in each cell, as indicators of change rate and its significance, were then plotted over the standard grid covering the study area.
Shifts in distribution were summarized by calculating the centroid of the distribution for a given year (termed center of gravity, CoG) after having predicted the density associated with every knot and year in the previous step. By means of the SDF estimator implemented in the VAST model, the CoG was calculated for the BoB population domain and standardized by the total abundance predicted for the study area, so that our analysis focused on changes in distribution after controlling for changes in total abundance (Thorson et al., 2016). Shifts in CoG were displayed in terms of “Eastings” and “Northings,” meaning km from the most western point of the study area and km from the Equator, respectively. Significant trends were identified using a linear regression against year.
To understand spatio-temporal patterns, three main groups of drivers were analyzed (Table 1), classified into local and regional covariates according to their spatio-temporal structure (a local covariate varies across space while a regional covariate is a univariate time series representing the covariate over the entire study area; Thorson, 2019):
Table 1. Summary of the local oceanographic, regional climatic and regional prey variables used in this study accompanied by a little description and the source from which they were obtained.
(1) Local oceanographic conditions integrated at 100 m depth, specifically temperature and chlorophyll a concentration (Chl-a), based on their direct relationship with climate change and their importance for predicting top predators distribution (Hazen et al., 2012; García-Barón et al., 2020).
(2) Regional climate indices, specifically North Atlantic Oscillation (NAO), East Atlantic Pattern (EA), and Atlantic Multidecadal Oscillation (AMO) climate indices (details in Table 1), due to their ability to extract the leading pattern in weather and climate variability over the North Atlantic and their relationship to cetacean and prey populations (Simmonds and Isaac, 2007; Borja et al., 2008; Evans et al., 2010; Evans and Waggitt, 2020).
(3) Regional biomasses of potential prey species, based on the assumption that climate change will affect cetaceans distribution through changes in their prey (Robinson et al., 2005; Simmonds and Isaac, 2007; Evans and Waggitt, 2020).
Temperature and Chl-a values were sourced from the Iberian Biscay Irish Ocean Reanalysis Model available at the Marine Environmental Monitoring Systems,3 providing values at a 0.08° spatial resolution, a 1-month temporal resolution and at 22 discrete depth intervals ranging from surface to 100 m depth. To test their effect on the annual estimates predicted by the baseline spatio-temporal model, the annual mean of both temperature and Chl-a was estimated integrating the data available in the first 100 m of the water column and then resampled with the raster package (Hijmans et al., 2017) at 0.1° (∼10 km) resolution (Waggitt et al., 2020). The three climate indices were downloaded from the National Oceanic and Atmospheric Administration (NOAA) at a monthly scale and averaged to obtain annual values,4 while the biomass of prey species was acquired from the International Council for The Exploration of Seas (ICES) website at annual scale.5 We selected prey species based on their relative importance in the common dolphin’s diet in the BoB (Meynier et al., 2008; Santos et al., 2013) as well as data availability and suitability because not every potential prey species (e.g., sprat, myctophids) was available for the spatio-temporal scale defined in this study. European anchovy (Engraulis encrasicolus) was the only prey species whose biomass had been estimated exclusively for the BoB. Horse mackerel (Trachurus trachurus) estimates were for the Northeast Atlantic, Atlantic mackerel (Scomber scombrus) and blue whiting (Micromesistius poutassou) for the Northeast Atlantic and adjacent waters and sardine (Sardina pilchardus) estimates for the Cantabrian-Atlantic Iberian waters (for information on the extent of stocks see Table 1). Although there is an assessment for the sardine stock of the BoB, data were only available from 2000 onward (ICES, 2019c), so we decided to use the biomass estimations from the Cantabrian sea and Atlantic Iberian waters instead after having checked that both indices were highly correlated (r = 0.87) and followed similar trends (Supplementary Figure 2). Finally, the biomasses of all species were summed and used as a proxy for total prey biomass available in the BoB.
For modeling purposes, local temperature and Chl-a variables were included as quadratic forms in the model to allow for non-linear responses (Perretti and Thorson, 2019). Regional climate indices were included as “spatially varying coefficients” as in Thorson (2019), which means that instead of estimating a single slope parameter presenting the effect of an oceanographic index on density, the model estimates a separate slope parameter for every modeled location (every knot). The biomass of each prey species, as well as the total biomass index, were first log transformed and then included as spatially varying coefficients since they were also available as a single regional time-series.
As a preliminary analysis, potential drivers were correlated with the abundance and CoG of common dolphin obtained in the previous baseline spatio-temporal model. Then, covariates-based modeling was performed in two different ways to identify the most parsimonious drivers and to uncover the relative contribution of covariates:
(1) Univariate spatio-temporal models were fitted for each variable using the same configuration as in the baseline spatio-temporal model. Univariate models were then compared with the baseline model by means of the AIC (Sakamoto et al., 1986). Only a decrease in the AIC > 2 in relation to the baseline spatio-temporal model was considered an improvement. When models differed by less than 2 units of AIC (ΔAIC ≤ 2), they were considered statistically equivalent (Arnold, 2010). The way in which covariates were related to the spatio-temporal patterns of common dolphin was also explored by plotting the functional relationships from the model parameters.
(2) Univariate models were fitted for each variable after setting the spatio-temporal variation (i.e., spatio-temporal random effects) to 0. This was done to remove the contribution of random effects and isolate the effect of the covariates since in VAST, random fields can also account for changes in distribution over time by capturing the residual spatial patterns that cannot be attributed to the fixed effect (Thorson et al., 2017). The abundances and CoG obtained from these models were then compared with those from the baseline spatio-temporal model to determine the amount of variation attributable to covariates.
A total of 1728 sightings of common dolphin collected in 21 different surveys were analyzed (Figure 1 and Supplementary Table 1). Those surveys mainly covered spring-summer months and showed a peak of maximum effort between the 2007 and 2012 period (Supplementary Figure 3). The mean latitude of sampling also varied and shifted significantly south over time (p = 0.001), while no significant change was observed in the mean longitude of sampling (Figure 2).
Figure 2. Sampling effort (number of segments of up to 10 km) as a function of year and longitude (A), and year and latitude (B). In both figures the size of the circle is proportional to the sampling effort; the black line indicates the mean value and the dotted line the linear temporal trend.
The common dolphin abundance estimated by the baseline spatio-temporal model showed a significant increase (p < 0.001) throughout the study period, accompanied by high variability (Figure 3 and Supplementary Table 3). This increase was most pronounced over the more recent years (2011–2017) and mainly occurred in the southeast corner of the BoB (Figure 4). These results agreed with the ferry data, which also showed an increasing trend and a significant correlation (r = 0.7, p = 0.003) with the predicted abundances (Supplementary Figures 4, 5).
Figure 3. Abundance of common dolphin in the BoB predicted by the baseline spatio-temporal model with standard deviation (shaded area), the linear trend, and its significance.
Figure 4. Spatio-temporal changes in the abundance of common dolphin (predicted by the baseline model) illustrated by means of the change rate (the slope of the linear regression). Hatched areas indicate those areas where change rate is not significant (p > 0.05).
The CoG also showed a high interannual variability, but no significant trend was found over time in either of the two axes (Figures 5A,B). In contrast, the correlation between eastings and northings showed as significant pattern (p = 0.005) in the direction of the shift, indicating that the distribution of common dolphins generally varied between the inner (southeast) and the outer part (northwest) of the BoB (Figure 5C).
Figure 5. The variation in the center of gravity (CoG) of common dolphin expressed in eastings (A) and northings axes (B), and as a function of both (C). Shaded area means the standard error, while the dashed line indicates the linear trend.
Neither the annual temperature nor the Chl-a concentration integrated at 100 m depth revealed a significant (p > 0.05) temporal trend across the full BoB (Supplementary Figure 6). The climate index AMO has remained in a positive phase since 1997, whereas NAO and EA indices have shown a higher variability with alternation between positive and negative phases (Supplementary Figure 7). Both anchovy and mackerel biomasses showed a significant (p ≤ 0.05) recovery after a period of low abundance, while sardine and horse mackerel underwent a severe decline (p ≤ 0.001). In contrast, blue whiting did not show any significant temporal trend (p = 0.2). The prey biomass index, on the other hand, exhibited a significant increase (p = 0.003), despite the large variability (Supplementary Figure 8).
The correlation between the potential drivers and the CoG (easting and northings) of common dolphin only showed weak relationships. In contrast, predicted abundance revealed several strong relationships (r > 0.5) with prey species, specifically mackerel and anchovy (positive correlation), and sardine and horse mackerel (negative correlation) (Figure 6). After prey species, only EA and NAO climate indices showed a moderate correlation with abundance (r∼0.40). Blue whiting was not significant (p > 0.05), while temperature, Chl-a, AMO and the prey biomass index showed weak relationships (r∼0.20) (Figure 6).
Figure 6. Pearson correlation among the common dolphin’s predicted abundance, CoG and potential drivers. Circle sizes are proportional to the correlation coefficient, which is indicated inside the circles. Non-significant correlations (p > 0.05) are shown without a circle.
For covariates-based models, the AIC score showed that the most substantial decrease was for the NAO index and regional prey species biomasses (especially anchovy and sardine). Local Chl-a concentration, as well as horse mackerel and mackerel, only contributed slightly, while remaining drivers (temperature, AMO, EA, blue whiting and prey species biomass index) were not relevant in terms of AIC (Table 2). Functional relationships of those important drivers revealed positive responses for NAO, anchovy, mackerel and negative for Chl-a, horse mackerel and sardine (Supplementary Figure 9).
Similarly, covariate-only models (with no random effects) showed that the NAO index and prey species biomasses were able to explain the increase in region-wide abundance of common dolphin (Figure 7). Chl-a concentration, despite having shown a decrease in AIC score (Table 2), did not contribute to explain the variability in the relative abundance (Figure 7), and neither did temperature, AMO index, or blue whiting (Supplementary Figure 10). EA and biomass indices did show a higher contribution in terms of variability, but they were not identified as important drivers according to AIC score (Supplementary Figure 10).
Figure 7. Abundance estimates predicted by the baseline spatio-temporal model (black line) and by the covariates-based model (with no random effects, colored line) so that the contribution made by each variable (A–F) can be visualized. Only drivers identified as relevant by AIC score are shown.
In the case of CoG, only Chl-a and temperature contributed to explain the observed variability but, even then, only in a very small proportion (Figure 8). In fact, the variation in the CoG explained by these variables only accounted for about 10–20 km, while the spatio-temporal model suggested variation of 100–300 km.
Figure 8. Center of gravity estimates predicted by the baseline spatio-temporal model (black line) and by the temperature and chlorophyll-based models (with no random effects, colored line), expressed in easting (A) and northings (B) axes.
The evaluation of the spatio-temporal patterns of common dolphin in the BoB agrees with the MSFD aiming to assess the abundance and distribution of species in European waters. Surveys providing information on species distribution and abundance in this region, however, have shown significant shifts in the spatial distribution of observations, which make necessary the application of methods such as VAST to account for uneven sampling effort.
The modeling of common dolphin sightings revealed a significant increase in abundance, which is in agreement with previous studies conducted in the BoB (Hemery et al., 2007; Authier et al., 2018; Saavedra et al., 2018) and in the wider Northeast Atlantic (Hammond et al., 2017; Evans and Waggitt, 2020) that also reported an increasing trend. In addition, data from ferry surveys, known to perform the same route every year, showed the same pattern and confirmed that the results were not biased by the detected latitudinal shift in effort.
In addition, the predicted abundance estimates were found to be quite coherent with those obtained in previous surveys conducted in summer 2012 in the BoB (Laran et al., 2017) and in summer 2016 in the Northeast Atlantic (ICES, 2020), in which 490,000 (95% CI: 340,000–720,000) small delphinids (common and striped dolphins) and 634,000 (95% CI: 353,000–1,140,000) common dolphins were estimated, respectively. Although it is not possible to make a direct comparison with our predictions, the ratios for common/striped dolphins and Northeast Atlantic/BoB estimated from Hammond et al. (2017) would lead to an approximate abundance of 360,000 (95% CI: 250,000–526,000) and 425,000 (95% CI: 237,000–764,000) individuals of common dolphin in the BoB for 2012–2016, respectively. These numbers were similar to our predictions in those years (359,000 ± 49,000 and 376,000 ± 71,500 individuals, respectively; Supplementary Table 2), and would indicate that, overall, abundance estimates from VAST were consistent with previous studies. This good agreement is remarkable, given the heterogeneity of the data used in this study that comprised 21 datasets, and emphasizes the importance of applying methods that are robust to shifts in sampling effort. In addition, the concordance between our results and those estimates made on summer also suggest that the spatio-temporal patterns obtained in this study should be interpreted as spring-summer trends, as this was the period of the year when most data were collected (Supplementary Figure 2B).
The increasing trend in abundance found in this study for the BoB, however, does not necessarily imply an overall population increase at the Northeast Atlantic level (i.e., species whole distribution range), and instead, could be due to the arrival of individuals from unsampled areas. That is why the results found in this study should be treated with caution and never be used to downplay the effects of incidental capture on common dolphin, especially when recent estimates suggest that the bycatch in the BoB is unsustainable for the population as a whole (ICES, 2020).
Local environmental variables, such as temperature and Chl-a used in this study, are often unable to capture complex associations between environment and ecological process due to time lags in species responses coupled with the non-linear intrinsic nature of population dynamics (Hallett et al., 2004).
This can be particularly true for Chl-a and cetaceans species that feed on zooplanktivorous fishes, since the abundance of the latter has been related to a period of zooplankton grazing and a phytoplankton decay (Díaz López et al., 2019). Under such circumstances, many researchers working with cetaceans often apply time-lagged Chl-a concentration for one and/or 2 months prior to the sighting month (Tobeña et al., 2016; Prieto et al., 2017; Pérez-Jorge et al., 2020; Barlow et al., 2021).
In this study, however, predictors were introduced at an annual scale to match the available temporal scales of both prey and climatic indices, which prevented its incorporation in a lagged phase and likely led to the low contribution of Chl-a in explaining the spatio-temporal patterns of common dolphin. Similarly, the lack of importance shown by temperature could be also a consequence of this annual resolution or could instead suggest that, within the core of the species range, temperature is not such an important variable to explain its abundance and distribution.
On the contrary, regional indices of climate, spanning several months and considering wider areas of influence, are less disturbed by local variability and very often outperform locally estimated environmental variables (Hallett et al., 2004). In addition, they usually hold information about several environmental factors (e.g., temperature, storms and precipitation, mixed layer depths or circulation patterns), which make them act as an integrated measure of meteo-oceanographic conditions that tend to explain more of the variability of the system than just, for example, ocean temperature (Hurrell and Deser, 2009; Thorson, 2019).
The results found in this study are a good example of this, as the NAO climate index was found to be the best predictor explaining the abundance of common dolphin according to AIC scores. Specifically, results showed a positive relationship between both, meaning that common dolphin abundance is enhanced during positive phases of NAO, which are characterized by colder and drier conditions over Mediterranean regions, central and southern Europe (e.g., BoB), and warmer and wetter conditions in northern Europe (Visbeck et al., 2001; Aravena et al., 2009; Hurrell and Deser, 2009).
Although the NAO index and similar climate indices have been previously related to the abundance of wide ranging predators in the BoB (Hemery et al., 2007; Louzao et al., 2015), responses are likely mediated through the influence of the climate indices on food resources rather than directly on higher trophic predators such as cetaceans (Drinkwater et al., 2003; Lusseau et al., 2004). Indeed, the NAO climatic index has been related to some biologically important phenomena, such as upwelling (Pérez et al., 2010), river run-off (Dupuis et al., 2006) and Ekman transport (Guisande et al., 2004), which are known to influence the recruitment of some of the main prey species (i.e., anchovy, sardine) of common dolphin (Guisande et al., 2004; Borja et al., 2008; Planque and Buffaz, 2008). We could therefore hypothesize a potential bottom-up process, in which NAO affects common dolphins through its influence on prey. In fact, bottom-up control has been suggested for the continental shelf food web of the BoB, where a highly diverse and abundant community of forage fishes regulates higher trophic levels (Lassalle et al., 2011).
Common dolphins are assumed to be opportunistic predators that feed on a wide variety of species, although a preference for energy-rich species, such as the anchovy, sardine, mackerel and horse mackerel investigated in this study, has been suggested (Meynier et al., 2008). Atlantic mackerel, however, is only present in large quantities during the first half of the year in the BoB, coinciding with its spawning period (Uriarte and Lucio, 2001), while Atlantic horse mackerel and the Iberian sardine are currently in serious decline (ICES, 2018, 2019b). European anchovy, in contrast, has been at a sustainable level since 2010, with an overall increasing trend that reached its maximum in 2019 (ICES, 2019a). The importance of prey species in common dolphin diet has been found to be related to their availability in terms of abundance (Santos et al., 2004; Meynier et al., 2008), which could explain the negative responses shown by species with low abundances (e.g., Iberian sardine and Atlantic horse mackerel) and the positive and larger contribution in terms of AIC made by those species with higher abundance (i.e., European anchovy). Blue whiting, on the other hand, did not seem to be relevant in explaining the variability of common dolphin over the study period, despite being more abundant than, for example, anchovy or mackerel. Evidence of blue whiting in the diet of the common dolphin was found in the BoB in the 1980s (Desportes, 1985), which could mean that it was important in the past but less so now, or that it is only important, given its poorer energetic condition (4.4 kJ g–1), in the absence of other remarkable prey species (Santos et al., 2013).
However, not all potential prey species were included and differences in the distribution of stocks may have also affected the results. In fact, only anchovy’s biomass had been estimated exclusively for the BoB. Remaining species biomasses were either estimated using adjacent areas (i.e., Iberian sardine) or distribution areas that extended considerably the observations range of common dolphin (i.e., blue whiting, mackerel and in a lesser extent horse mackerel), which could have contributed, for example, to the higher prominence of anchovy detected in this study.
The common dolphin is considered a warm-temperate species, and accordingly, its range is expected to expand in response to increasing water temperature (MacLeod, 2009). This northward expansion seems to be already happening, at least at the northern limit of the species range, as evidenced by a higher frequency of strandings and sightings in northern Britain and southern Scandinavia (MacLeod et al., 2005; Evans and Waggitt, 2020). The BoB, however, does not constitute a range edge within common dolphin’s distribution, which can explain why we did not find a northward shift in its CoG, but instead, switches between the inner (i.e., southeast) and the outer (i.e., northwest) part of the BoB. This pattern has also been detected when forecasting the future distribution of anchovy’s egg density in the BoB for spring (Erauskin-Extramiana et al., 2019a) and was associated to the contraction (southeast) and expansion (northwest) of anchovy population (Motos et al., 1996). A prey driven distribution was already suggested for albacore tuna in the area (Lezama-Ochoa et al., 2010), so we could hypothesize that the distributional shifts of common dolphins in the BoB are also driven by the distribution of their main prey. Similarly, the increase in common dolphin abundance detected in the southeast corner of the BoB could be also related to a higher prey availability. Indeed, other important prey species of the diet of common dolphin (e.g., horse mackerel, sprat) also shifted to the southeast of the BoB in the past 30 years (Baudron et al., 2020).
The prey variables considered in this study, however, could not explain much of the observed spatio-temporal variability of the CoG as a result of being introduced as a biomass index that changed across time but not across space, and hence, could not confirm or reject the hypothesized prey-driven distribution. Whether top predator abundance and distribution is driven by the environment or prey is a much debated question in ecology (Grinnell, 1917; Elton, 1927; Torres et al., 2008). However, acquiring co-occurring top predator and prey data in space and time to test these hypotheses is challenging. In this study, we have taken advantage of a large spatio-temporal compilation of top predator sightings, but in contrast, we have only been able to incorporate annual, non-spatial biomass indices of prey. Future work, therefore, should focus on improving prey data inputs to better understand their role in driving top predator distributional shifts in the BoB, a question that remains open. Climate indices, as for prey biomasses, were regional time-series rather than spatio-temporal datasets (i.e., changed across time but not across space), so their effect on the CoG is also difficult to understand. Local oceanographic variables did account for spatio-temporal changes, but even so, only explained a very small proportion of spatial shifts, which means that most of the distributional shifts occurred due to unidentified sources. This inability to attribute a source to distributional shifts was also found in previous studies with fishes (Thorson et al., 2017; Perretti and Thorson, 2019), and suggests that more effort must be made to understand when distributional shifts can be attributed to covariates in spatial random effects models (Hodges and Reich, 2010).
Climate change is believed to affect marine mammals through changes in their physical environment but also in their prey. However, many studies aimed at understanding climate impacts often employ environmental characteristics as proxies for prey distribution. In this study, we incorporated both environmental and prey variables estimated at local and regional scale and explored the relative importance of each of them in explaining the spatio-temporal variability in common dolphin data. Although we could not attribute much of the detected distributional shifts to the variables considered in this study, we could conclude that, in the BoB, climate indices and prey species biomasses can play an important role in driving the abundance patterns of the common dolphin.
Further research on climate change effects on common dolphin, however, should focus on comprising the whole distribution range of the species, given the increasingly feasible possibility for combining surveys across areas and regions provided by methods such as those used here. This way, we could address important knowledge gaps that have not been solved here, for example, if the increasing trend found in abundance is due to the arrival of new individuals or it is the result of an overall population growth. Answering to this question will undoubtedly help understand population dynamics and bycatch implications, but meanwhile, we reiterate our call for caution when interpreting the abundance patterns predicted in this study.
Any requests for survey data should be addressed to their owners. In future, some survey data may become open access. Please contact PE (cGV0ZXIuZXZhbnNAYmFuZ29yLmFjLnVr) for further details.
AA, MLo, and GC conceived and designed the study. AA applied the models and wrote the main text of the manuscript. JW collated and standardized survey data while MP and JT helped with the modeling approach. The remaining co-authors contributed survey data and revised the manuscript. All authors contributed to the article and approved the submitted version.
This research was funded by the Basque and the Spanish Government through CHALLENGES (CTM2013-47032-R) and EPELECO projects and LIFE-IP URBAN KLIMA 2050 project (Grant agreement no. LIFE18 IPC/ES/000001) which has received funding from European Union’s LIFE program. AA has benefited from a Basque Government scholarship (PRE_2016_1_0134) and MLo from Ramón y Cajal funding (Ministerio de Ciencia e Innovación, RYC-2012-09897).
The authors declare that the research was conducted in the absence of any commercial or financial relationships that could be construed as a potential conflict of interest.
All claims expressed in this article are solely those of the authors and do not necessarily represent those of their affiliated organizations, or those of the publisher, the editors and the reviewers. Any product that may be evaluated in this article, or claim that may be made by its manufacturer, is not guaranteed or endorsed by the publisher.
We thank the multiple organizations, institutions and surveys that provided data (ATLANCET, BIOMAN, CEMMA, CODA, ESAS, EVHOE, IBTS, IFAW, IWDG, JUVENA, KOSMOS, MARINELIFE, ORCA, PELACUS, PELGAS, SAMM, SCANS, SPEA, SWF, WDC; details in Supplementary Table 1), to the Bangor University, James Waggitt and Peter Evans for allowing a short visit, and to Rutgers University and the Pinsky Lab for hosting an academic exchange. We also thank Megan Ferguson and Leire Citores for their feedback and help as well as the editor and the two reviewers for their valuable suggestions and comments. This is contribution 1076 from AZTI Marine Research.
The Supplementary Material for this article can be found online at: https://www.frontiersin.org/articles/10.3389/fmars.2021.665474/full#supplementary-material
Aravena, G., Villate, F., Iriarte, A., Uriarte, I., and Ibáñez, B. (2009). Influence of the North Atlantic Oscillation (NAO) on climatic factors and estuarine water temperature on the Basque coast (Bay of Biscay): comparative analysis of three seasonal NAO indices. Continent. Shelf Res. 29, 750–758. doi: 10.1016/j.csr.2008.12.001
Arnold, T. W. (2010). Uninformative parameters and model selection using Akaike’s information criterion. J. Wildl. Manage. 74, 1175–1178. doi: 10.1111/j.1937-2817.2010.tb01236.x
Astarloa, A., Louzao, M., Boyra, G., Martinez, U., Rubio, A., Irigoien, X., et al. (2019). Identifying main interactions in marine predator–prey networks of the Bay of Biscay. ICES J. Mar. Sci. 76, 2247–2259. doi: 10.1093/icesjms/fsz140
Authier, M., Dorémus, G., Van Canneyt, O., Boubert, J.-J., Gautier, G., Doray, M., et al. (2018). Exploring change in the relative abundance of marine megafauna in the Bay of Biscay, 2004–2016. Prog. Oceanogr. 166, 159–167. doi: 10.1016/j.pocean.2017.09.014
Barlow, D. R., Klinck, H., Ponirakis, D., Garvey, C., and Torres, L. G. (2021). Temporal and spatial lags between wind, coastal upwelling, and blue whale occurrence. Sci. Rep. 11:6915. doi: 10.1038/s41598-021-86403-y
Baudron, A. R., Brunel, T., Blanchet, M. A., Hidalgo, M., Chust, G., Brown, E. J., et al. (2020). Changing fish distributions challenge the effective management of European fisheries. Ecography 43, 494–505. doi: 10.1111/ecog.04864
Blanchard, F., and Vandermeirsch, F. (2005). Warming and exponential abundance increase of the subtropical fish Capros aper in the Bay of Biscay (1973–2002). C. R. Biol. 328, 505–509. doi: 10.1016/j.crvi.2004.12.006
Borja, A., Fontán, A., Sáenz, J. O. N., and Valencia, V. (2008). Climate, oceanography, and recruitment: the case of the Bay of Biscay anchovy (Engraulis encrasicolus). Fish. Oceanogr. 17, 477–493. doi: 10.1111/j.1365-2419.2008.00494.x
Buckland, S. T., Anderson, D., Burnham, K., Laake, J., Thomas, L., and Borchers, D. (2001). Introduction to Distance Sampling: Estimating Abundance of Biological Populations. Oxford: Oxford university press.
Burrows, M. T., Schoeman, D. S., Buckley, L. B., Moore, P., Poloczanska, E. S., Brander, K. M., et al. (2011). The pace of shifting climate in marine and terrestrial ecosystems. Science 334, 652–655. doi: 10.1126/science.1210288
Cañadas, A., Desportes, G., and Borchers, D. (2004). The estimation of the detection function and g (0) for short-beaked common dolphins (Delphinus delphis), using double-platform data collected during the NASS-95 Faroese survey. J. Cetacean Res. Manag. 6, 191–198.
Carroll, G., Holsman, K. K., Brodie, S., Thorson, J. T., Hazen, E. L., Bograd, S. J., et al. (2019). A review of methods for quantifying spatial predator–prey overlap. Glob. Ecol. Biogeogr. 28, 1561–1577. doi: 10.1111/geb.12984
Castège, I., Soulier, L., Hémery, G., Mouchès, C., Lalanne, Y., Dewez, A., et al. (2013). Exploring cetacean stranding pattern in light of variation in at-sea encounter rate and fishing activity: lessons from time surveys in the south Bay of Biscay (East-Atlantic; France). J. Mar. Syst. 109-110, S284–S292. doi: 10.1016/j.jmarsys.2012.04.007
Chust, G., Castellani, C., Licandro, P., Ibaibarriaga, L., Sagarminaga, Y., and Irigoien, X. (2014b). Are Calanus spp. shifting poleward in the North Atlantic? A habitat modelling approach. ICES J. Mar. Sci. 71, 241–253. doi: 10.1093/icesjms/fst147
Chust, G., Allen, J. I., Bopp, L., Schrum, C., Holt, J., Tsiaras, K., et al. (2014a). Biomass changes and trophic amplification of plankton in a warmer ocean. Glob. Chang Biol. 20, 2124–2139. doi: 10.1111/gcb.12562
Chust, G., Goikoetxea, N., Ibaibarriaga, L., Sagarminaga, Y., Arregui, I., Fontán, A., et al. (2019). Earlier migration and distribution changes of albacore in the Northeast Atlantic. Fish. Oceanogr. 28, 505–516. doi: 10.1111/fog.12427
Costoya, X., Decastro, M., Gómez-Gesteira, M., and Santos, F. (2015). Changes in sea surface temperature seasonality in the Bay of Biscay over the last decades (1982–2014). J. Mar. Syst. 150, 91–101. doi: 10.1016/j.jmarsys.2015.06.002
Desportes, G. (1985). La nutrition des Odontocètes en Atlantique Nord-Est(côtes Françaises-îles Feroë). Berlin: Springer.
Díaz López, B., and Methion, S. (2019). Habitat drivers of endangered rorqual whales in a highly impacted upwelling region. Ecol. Indic. 103, 610–616. doi: 10.1016/j.ecolind.2019.04.038
Díaz López, B., Methion, S., and Giralt Paradell, O. (2019). Living on the edge: overlap between a marine predator’s habitat use and fisheries in the Northeast Atlantic waters (NW Spain). Prog. Oceanogr. 175, 115–123. doi: 10.1016/j.pocean.2019.04.004
Drinkwater, K. F., Belgrano, A., Borja, A., Conversi, A., Edwards, M., Greene, C. H., et al. (2003). The response of marine ecosystems to climate variability associated with the North Atlantic Oscillation. Geophys. Monogr. Ser. 134, 211–234. doi: 10.1029/134GM10
Dupuis, H., Michel, D., and Sottolichio, A. (2006). Wave climate evolution in the Bay of Biscay over two decades. J. Mar. Syst. 63, 105–114. doi: 10.1016/j.jmarsys.2006.05.009
Erauskin-Extramiana, M., Arrizabalaga, H., Cabré, A., Coelho, R., Rosa, D., Ibaibarriaga, L., et al. (2019b). Are shifts in species distribution triggered by climate change? A swordfish case study. Deep Sea Res. II Top. Stud. Oceanogr. 175:104666. doi: 10.1016/j.dsr2.2019.104666
Erauskin-Extramiana, M., Alvarez, P., Arrizabalaga, H., Ibaibarriaga, L., Uriarte, A., Cotano, U., et al. (2019a). Historical trends and future distribution of anchovy spawning in the Bay of Biscay. Deep Sea Res. II Top. Stud. Oceanogr. 159, 169–182. doi: 10.1016/j.dsr2.2018.07.007
Evans, P., and Waggitt, J. (2020). Impacts of climate change on marine mammals, relevant to the coastal and marine environment around the UK. MCCIP Sci. Rev. 2020, 421–455.
Evans, P. G. H., Pierce, G. J., and Panigada, S. (2010). Climate change and marine mammals. J. Mar. Biol. Assoc. U.K. 90, 1483–1487. doi: 10.1017/S0025315410001815
Free, C. M., Thorson, J. T., Pinsky, M. L., Oken, K. L., Wiedenmann, J., and Jensen, O. P. (2019). Impacts of historical warming on marine fisheries production. Science 363, 979–983. doi: 10.1126/science.aau1758
García-Barón, I., Authier, M., Caballero, A., Vázquez, J. A., Santos, M. B., Murcia, J. L., et al. (2019). Modelling the spatial abundance of a migratory predator: a call for transboundary marine protected areas. Divers. Distrib. 25, 346–360. doi: 10.1111/ddi.12877
García-Barón, I., Santos, M. B., Saavedra, C., Astarloa, A., Valeiras, J., García Barcelona, S., et al. (2020). Essential ocean variables and high value biodiversity areas: targets for the conservation of marine megafauna. Ecol. Indic. 117:106504. doi: 10.1016/j.ecolind.2020.106504
Giralt Paradell, O., Díaz López, B., and Methion, S. (2019). Modelling common dolphin (Delphinus delphis) coastal distribution and habitat use: insights for conservation. Ocean Coast. Manag. 179:104836. doi: 10.1016/j.ocecoaman.2019.104836
Godefroid, M., Boldt, J. L., Thorson, J. T., Forrest, R., Gauthier, S., Flostrand, L., et al. (2019). Spatio-temporal models provide new insights on the biotic and abiotic drivers shaping Pacific Herring (Clupea pallasi) distribution. Prog. Oceanogr. 178:102198. doi: 10.1016/j.pocean.2019.102198
Grinnell, J. (1917). The niche-relationships of the California thrasher. Auk 34, 427–433. doi: 10.2307/4072271
Guisande, C., Vergara, A., Riveiro, I., and Cabanas, J. (2004). Climate change and abundance of the Atlantic-Iberian sardine (Sardina pilchardus). Fish. Oceanogr. 13, 91–101. doi: 10.1046/j.1365-2419.2003.00276.x
Hallett, T., Coulson, T., Pilkington, J., Clutton-Brock, T., Pemberton, J., and Grenfell, B. (2004). Why large-scale climate indices seem to predict ecological processes better than local weather. Nature 430, 71–75. doi: 10.1038/nature02708
Hammond, P., Lacey, C., Gilles, A., Viquerat, S., Boerjesson, P., Herr, H., et al. (2017). Estimates of Cetacean Abundance in European Atlantic Waters in Summer 2016 from the SCANS-III Aerial and Shipboard Surveys. Yerseke: Wageningen Marine Research.
Hazen, E. L., Abrahms, B., Brodie, S., Carroll, G., Jacox, M. G., Savoca, M. S., et al. (2019). Marine top predators as climate and ecosystem sentinels. Front. Ecol. Environ. 17, 565–574. doi: 10.1002/fee.2125
Hazen, E. L., Jorgensen, S., Rykaczewski, R. R., Bograd, S. J., Foley, D. G., Jonsen, I. D., et al. (2012). Predicted habitat shifts of Pacific top predators in a changing climate. Nat. Clim. Change 3, 234–238. doi: 10.1038/nclimate1686
Hemery, G., D’amico, F., Castege, I., Dupont, B., D’elbee, J., Lalanne, Y., et al. (2007). Detecting the impact of oceano-climatic changes on marine ecosystems using a multivariate index: the case of the Bay of Biscay (North Atlantic-European Ocean). Glob. Change Biol. 14, 1–12. doi: 10.1111/j.1365-2486.2007.01471.x
Hijmans, R. J., Van Etten, J., Cheng, J., Mattiuzzi, M., Sumner, M., Greenberg, J. A., et al. (2017). Package ‘raster’. R package.
Hodges, J. S., and Reich, B. J. (2010). Adding spatially-correlated errors can mess up the fixed effect you love. Am. Statist. 64, 325–334. doi: 10.1198/tast.2010.10052
Hurrell, J. W., and Deser, C. (2009). North Atlantic climate variability: the role of the North Atlantic Oscillation. J. Mar. Syst. 78, 28–41. doi: 10.1016/j.jmarsys.2008.11.026
ICES (2018). Sardine (Sardina pilchardus) in Divisions 8.c and 9.a (Cantabrian Sea and Atlantic Iberian Waters) Advice Sheet. Report of the ICES Advisory Commitee, 2018. Washington, DC: ICES.
ICES (2019c). Sardine (Sardina pilchardus) in Divisions 8.a-b and 8.d (Bay of Biscay) Advice Sheet. Report of the ICES Advisory Commitee, 2019. Washington, DC: ICES.
ICES (2019b). Horse Mackerel (Trachurus trachurus) in Subarea 8 and Divisions 2.a,4.a,5.b,6.a,7.a-c,e-k (the Northeast Atlantic) Advice Sheet. Report of the ICES Advisory Commitee, 2019. Washington, DC: ICES.
ICES (2019a). Anchovy (Engraulis encrasicolus) in Subarea 8 (Bay of Biscay) Advice Sheet. Report of the ICES Advisory Commitee, 2019. Washington, DC: ICES.
ICES (2020). Workshop on Fisheries Emergency Measures to minimize BYCatch of Short-Beaked Common Dolphins in the Bay of Biscay and Harbour Porpoise in the Baltic Sea (WKEMBYC). Washington, DC: ICES.
IPCC (2019). “Technical summary,” in PCC Special Report on the Ocean and Cryosphere in a Changing Climate, eds H.-O. PöRtner, V. Masson-Delmotte, P. Zhai, E. Poloczanska, K. Mintenbeck, M. Tignor, et al. (Geneva: IPCC).
Kristensen, K., Nielsen, A., Berg, C. W., Skaug, H., and Bell, B. (2016). TMB: automatic differentiation and Laplace approximation. arXiv [Preprint]. arXiv:1509.00660
Lambert, E., MacLeod, C. D., Hall, K., Brereton, T., Dunn, T. E., Wall, D., et al. (2011). Quantifying likely cetacean range shifts in response to global climatic change: implications for conservation strategies in a changing world. Endang. Spec. Res. 15, 205–222. doi: 10.3354/esr00376
Lambert, E., Pierce, G. J., Hall, K., Brereton, T., Dunn, T. E., Wall, D., et al. (2014). Cetacean range and climate in the eastern North Atlantic: future predictions and implications for conservation. Glob. Chang. Biol. 20, 1782–1793. doi: 10.1111/gcb.12560
Laran, S., Authier, M., Blanck, A., Doremus, G., Falchetto, H., Monestiez, P., et al. (2017). Seasonal distribution and abundance of cetaceans within French waters- Part II: The Bay of Biscay and the English Channel. Deep Sea Res. II Top. Stud. Oceanogr. 141, 31–40. doi: 10.1016/j.dsr2.2016.12.012
Lassalle, G., Lobry, J., Le Loc’h, F., Bustamante, P., Certain, G., Delmas, D., et al. (2011). Lower trophic levels and detrital biomass control the Bay of Biscay continental shelf food web: implications for ecosystem management. Prog. Oceanogr. 91, 561–575. doi: 10.1016/j.pocean.2011.09.002
Lezama-Ochoa, A., Boyra, G., Goñi, N., Arrizabalaga, H., and Bertrand, A. (2010). Investigating relationships between albacore tuna (Thunnus alalunga) CPUE and prey distribution in the Bay of Biscay. Prog. Oceanogr. 86, 105–114. doi: 10.1016/j.pocean.2010.04.006
Lindgren, F., Rue, H., and Lindström, J. (2011). An explicit link between Gaussian fields and Gaussian Markov random fields: the stochastic partial differential equation approach. J. R. Stat. Soc. Ser. B Stat. Methodol. 73, 423–498. doi: 10.1111/j.1467-9868.2011.00777.x
Louzao, M., Afán, I., Santos, M., and Brereton, T. (2015). The role of climate and food availability on driving decadal abundance patterns of highly migratory pelagic predators in the Bay of Biscay. Front. Ecol. Evol. 3:90. doi: 10.3389/fevo.2015.00090
Louzao, M., García-Barón, I., Rubio, A., Martínez, U., Vázquez, J. A., Murcia, J. L., et al. (2019). Understanding the 3D environment of pelagic predators from multidisciplinary oceanographic surveys to advance ecosystem-based monitoring. Mar. Ecol. Prog. Ser. 617-618, 199–219. doi: 10.3354/meps12838
Lusseau, D., Williams, R., Wilson, B., Grellier, K., Barton, T. R., Hammond, P. S., et al. (2004). Parallel influence of climate on the behaviour of Pacific killer whales and Atlantic bottlenose dolphins. Ecol. Lett. 7, 1068–1076. doi: 10.1111/j.1461-0248.2004.00669.x
MacLeod, C. D. (2009). Global climate change, range changes and potential implications for the conservation of marine cetaceans: a review and synthesis. Endang. Spec. Res. 7, 125–136. doi: 10.3354/esr00197
MacLeod, C. D., Bannon, S. M., Pierce, G. J., Schweder, C., Learmonth, J. A., Herman, J. S., et al. (2005). Climate change and the cetacean community of north-west Scotland. Biol. Conserv. 124, 477–483. doi: 10.1016/j.biocon.2005.02.004
Marques, F., and Buckland, S. (2004). Covariate models for the detection function. Adv. Distance Sampl. 8, 31–47.
Martin, T. G., Wintle, B. A., Rhodes, J. R., Kuhnert, P. M., Field, S. A., Low-Choy, S. J., et al. (2005). Zero tolerance ecology: improving ecological inference by modelling the source of zero observations. Ecol. Lett. 8, 1235–1246. doi: 10.1111/j.1461-0248.2005.00826.x
Maureaud, A. A., Frelat, R., Pecuchet, L., Shackell, N., Merigot, B., Pinsky, M. L., et al. (2021). Are we ready to track climate-driven shifts in marine species across international boundaries? - A global survey of scientific bottom trawl data. Glob. Chang Biol. 27, 220–236. doi: 10.1111/gcb.15404
Meynier, L., Pusineri, C., Spitz, J., Santos, M. B., Pierce, G. J., and Ridoux, V. (2008). Intraspecific dietary variation in the short-beaked common dolphin Delphinus delphis in the Bay of Biscay: importance of fat fish. Mar. Ecol. Prog. Ser. 354, 277–287. doi: 10.3354/meps07246
Motos, L., Uriarte, A., and Valencia, V. (1996). The spawning environment of the Bay of Biscay anchovy (Engraulis encrasicolus L.). Sci. Mar. 60, 117–140.
Parmesan, C., and Yohe, G. (2003). A globally coherent fingerprint of climate change impacts across natural systems. Nature 421, 37–42. doi: 10.1038/nature01286
Pérez, F. F., Padín, X. A., Pazos, Y., Gilcoto, M., Cabanas, M., Pardo, P. C., et al. (2010). Plankton response to weakening of the Iberian coastal upwelling. Glob. Change Biol. 16, 1258–1267. doi: 10.1111/j.1365-2486.2009.02125.x
Pérez-Jorge, S., Tobeña, M., Prieto, R., Vandeperre, F., Calmettes, B., Lehodey, P., et al. (2020). Environmental drivers of large-scale movements of baleen whales in the mid-North Atlantic Ocean. Divers. Distrib. 26, 683–698. doi: 10.1111/ddi.13038
Perretti, C. T., and Thorson, J. T. (2019). Spatio-temporal dynamics of summer flounder (Paralichthys dentatus) on the Northeast US shelf. Fish. Res. 215, 62–68. doi: 10.1016/j.fishres.2019.03.006
Pinsky, M. L., Eikeset, A. M., Mccauley, D. J., Payne, J. L., and Sunday, J. M. (2019). Greater vulnerability to warming of marine versus terrestrial ectotherms. Nature 569, 108–111. doi: 10.1038/s41586-019-1132-4
Planque, B., and Buffaz, L. (2008). Quantile regression models for fish recruitment–environment relationships: four case studies. Mar. Ecol. Prog. Ser. 357, 213–223. doi: 10.3354/meps07274
Poloczanska, E. S., Brown, C. J., Sydeman, W. J., Kiessling, W., Schoeman, D. S., Moore, P. J., et al. (2013). Global imprint of climate change on marine life. Nat. Clim. Change 3:919. doi: 10.1038/nclimate1958
Prieto, R., Tobeña, M., and Silva, M. A. (2017). Habitat preferences of baleen whales in a mid-latitude habitat. Deep Sea Res. Part II Top. Stud. Oceanogr. 141, 155–167. doi: 10.1016/j.dsr2.2016.07.015
Robbins, J. R., Babey, L., and Embling, C. B. (2020). Citizen science in the marine environment: estimating common dolphin densities in the north-east Atlantic. PeerJ 8:e8335. doi: 10.7717/peerj.8335
Robinson, R. A., Learmonth, J. A., Hutson, A. M., MacLeod, C. D., Sparks, T. H., Leech, D. I., et al. (2005). Climate Change and Migratory Species. Thetford: British Trust for Ornithology The Nunnery.
Saavedra, C., Gerrodette, T., Louzao, M., Valeiras, J., García, S., Cerviño, S., et al. (2018). Assessing the environmental status of the short-beaked common dolphin (Delphinus delphis) in North-western Spanish waters using abundance trends and safe removal limits. Prog. Oceanogr. 166, 66–75. doi: 10.1016/j.pocean.2017.08.006
Sakamoto, Y., Ishiguro, M., and Kitagawa, G. (1986). Akaike Information Criterion Statistics. Dordrecht: D. Reidel.
Santos, M., Pierce, G., López, A., Martínez, J., Fernández, M., Ieno, E., et al. (2004). Variability in the diet of common dolphins (Delphinus delphis) in Galician waters 1991–2003 and relationship with prey abundance. ICES CM 9, 91–103.
Santos, M. B., German, I., Correia, D., Read, F. L., Cedeira, J. M., Caldas, M., et al. (2013). Long-term variation in common dolphin diet in relation to prey abundance. Mar. Ecol. Prog. Ser. 481, 249–268. doi: 10.3354/meps10233
Simmonds, M. P., and Isaac, S. J. (2007). The impacts of climate change on marine mammals: early signs of significant problems. Oryx 41, 19–26. doi: 10.1017/S0030605307001524
Spitz, J., Ridoux, V., Trites, A. W., Laran, S., and Authier, M. (2018). Prey consumption by cetaceans reveals the importance of energy-rich food webs in the Bay of Biscay. Prog. Oceanogr. 166, 148–158. doi: 10.1016/j.pocean.2017.09.013
Stefánsson, G. (1996). Analysis of groundfish survey abundance data: combining the GLM and delta approaches. ICES J. Mar. Sci. 53, 577–588. doi: 10.1006/jmsc.1996.0079
Thorson, J. T. (2019). Measuring the impact of oceanographic indices on species distribution shifts: the spatially varying effect of cold-pool extent in the eastern Bering Sea. Limnol. Oceanogr. 64, 2632–2645. doi: 10.1002/lno.11238
Thorson, J. T., and Barnett, L. A. K. (2017). Comparing estimates of abundance trends and distribution shifts using single- and multispecies models of fishes and biogenic habitat. ICES J. Mar. Sci. 74, 1311–1321. doi: 10.1093/icesjms/fsw193
Thorson, J. T., Ianelli, J. N., and Kotwicki, S. (2017). The relative influence of temperature and size-structure on fish distribution shifts: a case-study on Walleye pollock in the Bering Sea. Fish Fish. 18, 1073–1084. doi: 10.1111/faf.12225
Thorson, J. T., Pinsky, M. L., Ward, E. J., and Gimenez, O. (2016). Model-based inference for estimating shifts in species distribution, area occupied and centre of gravity. Methods Ecol. Evol. 7, 990–1002. doi: 10.1111/2041-210X.12567
Tierney, L., Kass, R. E., and Kadane, J. B. (1989). Fully exponential Laplace approximations to expectations and variances of nonpositive functions. J. Am. Stat. Assoc. 84, 710–716. doi: 10.2307/2289652
Tobeña, M., Prieto, R., Machete, M., and Silva, M. A. (2016). Modeling the potential distribution and richness of cetaceans in the azores from fisheries observer program data. Front. Mar. Sci. 3:202. doi: 10.3389/fmars.2016.00202
Torres, L. G., Read, A. J., and Halpin, P. (2008). Fine-scale habitat modeling of a top marine predator: do prey data improve predictive capacity. Ecol. Appl. 18, 1702–1717. doi: 10.1890/07-1455.1
Trisos, C. H., Merow, C., and Pigot, A. L. (2020). The projected timing of abrupt ecological disruption from climate change. Nature 580, 496–501. doi: 10.1038/s41586-020-2189-9
Uriarte, A., and Lucio, P. (2001). Migration of adult mackerel along the Atlantic European shelf edge from a tagging experiment in the south of the Bay of Biscay in 1994. Fish. Res. 50, 129–139. doi: 10.1016/S0165-7836(00)00246-0
Visbeck, M. H., Hurrell, J. W., Polvani, L., and Cullen, H. M. (2001). The North Atlantic Oscillation: past, present, and future. Proc. Natl. Acad. Sci. U.S.A. 98, 12876–12877. doi: 10.1073/pnas.231391598
Waggitt, J. J., Evans, P. G. H., Andrade, J., Banks, A. N., Boisseau, O., Bolton, M., et al. (2020). Distribution maps of cetacean and seabird populations in the North-East Atlantic. J. Appl. Ecol. 57, 253–269. doi: 10.1111/1365-2664.13525
Keywords: common dolphin, center of gravity, climate indices, predator-prey, environmental variability, time series, Bay of Biscay, VAST
Citation: Astarloa A, Louzao M, Andrade J, Babey L, Berrow S, Boisseau O, Brereton T, Dorémus G, Evans PGH, Hodgins NK, Lewis M, Martinez-Cedeira J, Pinsky ML, Ridoux V, Saavedra C, Santos MB, Thorson JT, Waggitt JJ, Wall D and Chust G (2021) The Role of Climate, Oceanography, and Prey in Driving Decadal Spatio-Temporal Patterns of a Highly Mobile Top Predator. Front. Mar. Sci. 8:665474. doi: 10.3389/fmars.2021.665474
Received: 08 February 2021; Accepted: 17 September 2021;
Published: 18 November 2021.
Edited by:
Mary C. Fabrizio, William & Mary’s Virginia Institute of Marine Science, United StatesReviewed by:
Bruno Díaz López, Bottlenose Dolphin Research Institute (BDRI), SpainCopyright © 2021 Astarloa, Louzao, Andrade, Babey, Berrow, Boisseau, Brereton, Dorémus, Evans, Hodgins, Lewis, Martinez-Cedeira, Pinsky, Ridoux, Saavedra, Santos, Thorson, Waggitt, Wall and Chust. This is an open-access article distributed under the terms of the Creative Commons Attribution License (CC BY). The use, distribution or reproduction in other forums is permitted, provided the original author(s) and the copyright owner(s) are credited and that the original publication in this journal is cited, in accordance with accepted academic practice. No use, distribution or reproduction is permitted which does not comply with these terms.
*Correspondence: Amaia Astarloa, YWFzdGFybG9hQGF6dGkuZXM=
Disclaimer: All claims expressed in this article are solely those of the authors and do not necessarily represent those of their affiliated organizations, or those of the publisher, the editors and the reviewers. Any product that may be evaluated in this article or claim that may be made by its manufacturer is not guaranteed or endorsed by the publisher.
Research integrity at Frontiers
Learn more about the work of our research integrity team to safeguard the quality of each article we publish.