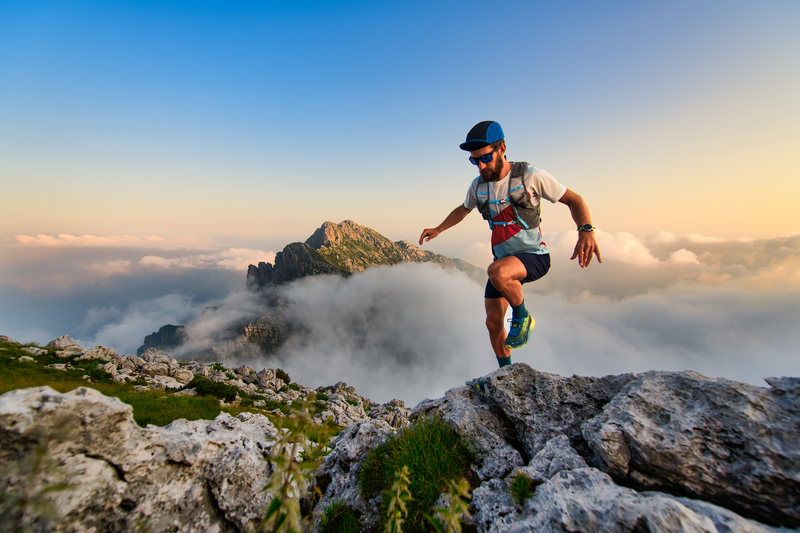
94% of researchers rate our articles as excellent or good
Learn more about the work of our research integrity team to safeguard the quality of each article we publish.
Find out more
ORIGINAL RESEARCH article
Front. Mar. Sci. , 26 April 2021
Sec. Marine Megafauna
Volume 8 - 2021 | https://doi.org/10.3389/fmars.2021.665337
Basking sharks (Cetorhinus maximus) were widely reported throughout New Zealand waters. Once commonly observed, and sometimes in large numbers, basking sharks are now infrequently reported. Basking shark observations are known to be highly variable across years, and their distribution and occurrence have been shown to be influenced by environmental predictors such as thermal fronts, chl-a concentration, and the abundance of prey (zooplankton). Little is known of basking sharks in the South Pacific and more information on distribution, habitat use, and migratory patterns is required to better understand the species’ regional ecology. Here, we used bootstrapped Habitat Suitability Models [HSM, ensembled from Boosted Regression Tree (BRT) and Random Forest (RF) models] to determine the drivers of basking shark distribution, predict habitat suitability and estimated uncertainty in the South Pacific for the first time. High−resolution environmental (1 km2 grid resolution) and biotic data, including inferred prey species, and all available basking shark records across New Zealand’s Exclusive Economic Zone (EEZ) were included in the ensemble HSMs. The most influential driver of modeled basking shark distribution was vertical flux of particulate organic matter at the seabed, which may indicate higher levels of primary production in the surface ocean and higher prey density in the mesopelagic zone and at the seafloor. The BRT and RF models had good predictive power (AUC and TSS > 0.7) and both models performed similarly with low variability in the model fit metrics. Areas of high basking shark habitat suitability included the east and west coasts of the South Island, Puysegur Ridge, and Auckland Island slope. The outputs produced here could be incorporated into future management framework for assessing threat and conservation needs (e.g., spatially explicit risk assessment) for this regionally protected species, as well as providing guidance for future research efforts (e.g., areas of interest for sampling).
The basking shark (Cetorhinus maximus) is a planktivorous coastal-pelagic species widely distributed in the temperate and tropical waters of the Atlantic and Pacific Oceans, and fringes of the Indian Ocean (southern Australia, Indonesia, South Africa) (Rigby et al., 2019). It is the second largest fish in the world after the whale shark (Rhincodon typus), reaching an maximum size greater than 10 m total length (Weigmann, 2016). Basking sharks are known for their slow surface swimming behavior but may also spend months at mesopelagic depths, and may dive to at least 1,264 m depth (Gore et al., 2008; Skomal et al., 2009; Dewar et al., 2018). The species also engages in long distance migrations and has been recorded crossing the Atlantic Ocean both from east to west and from north to south (Skomal et al., 2009; Braun et al., 2018; Dewar et al., 2018; Johnston et al., 2019). Recent genetic analysis suggests high gene flow and weak genetic structuring across the Atlantic and Pacific Oceans (Lieber et al., 2020). Despite their large size, basking sharks remain elusive and data-poor in the Pacific Ocean; habitat use and movement patterns in the South Pacific, and more specifically around New Zealand, are poorly understood.
New Zealand was once a hotspot for basking sharks in the South Pacific Ocean. Historically, the species was common in New Zealand coastal and offshore waters between 39°S and 51°S. Most records were from south of Cook Strait in cold, nutrient-rich waters along the Subtropical Front (Francis and Duffy, 2002). Individuals and large schools were most commonly reported during the spring and summer months along the east coast of the South Island and off Snares-Auckland Islands (Francis, 2017). Aerial surveys for Hector’s dolphins (Cephalorhynchus hectori) conducted around Bank’s Peninsula (east coast of the South Island) reported large groups of over 100 individuals in the early 1990s (Francis and Duffy, 2002). Subsequent aerial surveys for basking sharks and Hector’s dolphins have not seen any basking sharks. Only a few individuals are now reported annually, primarily as fisheries bycatch (Francis, 2017). Beyond New Zealand, basking sharks have been very infrequently observed across the South Pacific Ocean (Yatsu, 1995; Hernández et al., 2010).
Basking sharks are susceptible to exploitation from fishing due to their naturally low population sizes, presumed slow growth rates, and low reproductive rates (Francis, 2017). The species was subject to targeted fishing throughout its range and while most targeted fisheries ceased in the 2000s, basking sharks are still taken as bycatch by a number of fishing gear types (e.g., trawl, trammel net, set net). Elsewhere they are threatened by interactions with recreational vessels and commercial shipping due to the species’ time spent at the surface (Austin et al., 2019; Rigby et al., 2019). Population recovery has been low or negligible up to two decades after fishing ceased (Fowler et al., 2005). In 2002, basking sharks were listed in Appendix II of the Convention on International Trade in Endangered Species of Wild Fauna and Flora (CITES, 2002), and in 2005, were listed on Appendices I and II in the Convention of Migratory Species (CMS). In 2019, basking shark was assessed as globally Endangered by the International Union for Conservation of Nature (IUCN) Red List of Threatened Species (Rigby et al., 2019).
Basking sharks have been protected in New Zealand waters since 2010. There are no specific management measures in place for basking sharks, apart from mandatory reporting of captures and the return of captured individuals to the sea. There are very few fisheries independent data available and estimates of basking shark bycatch likely underestimate the total New Zealand catches because they do not account for captures in unobserved set net fisheries and inshore trawl fisheries (Francis, 2017). Patterns in unstandardized catch-per-unit-effort (CPUE) imply basking sharks were captured in relatively large numbers in the late 1980s and early 1990s, with peak bycatch occurring between 1988 and 1991 (Francis, 2017). Following this period, observed bycatch rates declined substantially. Off the east coast of the South Island raw CPUE peaked in 1991 at 81.9 sharks per 1,000 tows then fell to zero reported captures from 2005–2016 (Francis, 2017). In recent years, the species has occasionally been taken as bycatch in trawl and set net fisheries, with trawl bycatch typically occurring near or beyond the edge of the continental shelf (Francis and Smith, 2010; Francis, 2017). It is unclear if the recent decline in basking shark records in New Zealand is a result of a change to fishing practices that are less likely to encounter basking sharks, changes in regional availability of sharks, a real decline in abundance or a combination of these (Francis, 2017).
Distributions of basking shark are known to be highly variable between years, with up to 20 years between sightings reported from some Northern Hemisphere locations (Dewar et al., 2018). Their distribution and occurrence appears to be strongly linked to zooplankton/prey abundance at smaller spatial scales, but the drivers of broad scale distribution patterns are largely unknown (Sims, 2008). In the Northern Hemisphere, environmental predictors such as sea surface temperature (SST), thermal fronts, chl-a concentration, and the abundance of zooplankton seem to influence their distribution (Cotton et al., 2005; Austin et al., 2019). However, without sufficient information on the species’ distribution, habitat use, and migratory patterns, it is difficult to determine the cause of variability in abundance and distribution.
Correlative models that predict the occurrence of species in relation to environmental variables (termed habitat suitability models or species distribution models) have become an important part of resource management and conservation biology. Such models are capable of filling knowledge gaps on spatial and temporal distributions and predicting areas of suitable habitat for widely distributed species (Elith et al., 2006; Weber et al., 2017). By relating species’ sightings to environmental predictor variables, the abundance or probability of taxa presence at a given location can be estimated along with a characterization of the environmental drivers of species distributions. These models are becoming increasingly popular for use on marine species spanning large geographic and bathymetric ranges and have been employed for a range of cetaceans (Stephenson et al., 2020b), seabirds (Cleasby et al., 2020), and cartilaginous fishes, including basking sharks in the Northeast Atlantic (Austin et al., 2019).
Here, we combine functionally relevant, high−resolution environmental data (1 km2 grid resolution) with data on basking shark occurrences to predict basking shark habitat suitability across New Zealand’s Exclusive Economic Zone (EEZ). Unlike many habitat suitability models which include only environmental data and primary productivity, here we had the unique opportunity to also include high trophic level biotic data (zooplankton prey densities). The distribution of prey is often unavailable or overlooked, and at times, is a key predictor of species’ distributions (Dormann et al., 2018; Carroll et al., 2019). Understanding biotic interactions and their influence in driving species’ distributions is important for predicting into unsampled space because the trophic interactions that are at the core of species habitat use may be better captured (e.g., more accurate predictions due to climate change) (Araújo and Luoto, 2007). Regional differences in drivers of species’ distribution can also be present (Petatan-Ramirez et al., 2020). Thus, identifying factors that drive basking shark distribution across the New Zealand marine region is important for better understanding the species’ regional ecology and to direct and inform future research and spatially explicit conservation efforts for this protected species.
The study area extends over 4.2 million km2 of the South Pacific Ocean within the New Zealand EEZ (≈ 25–57°S; 162°E–172°W; Figure 1). New Zealand’s EEZ contains highly productive zones of mixing between warm, higher salinity, nutrient poor, northern waters, and cold, lower salinity, nutrient rich, southern waters. These productive zones support high biological diversity and a variety of customary, recreational, and commercial fisheries (Bradford-Grieve et al., 2006; Leathwick et al., 2006; Stephenson et al., 2018, 2020a). The New Zealand continental shelf environment is influenced by a combination of climatic features that produce a range of nearshore conditions, upwelling, tidal mixing, and upper-ocean mixing. Surface and thermocline waters of different origin are separated by three major fronts, the Subtropical Front, the Subantarctic Front, and the Polar Front, while the flow of bottom water is predominately carried by the Deep Western Boundary Current (Chiswell et al., 2015). New Zealand bathymetry is equally as complex, reaching abyssal depths beyond 10,000 m in the Kermadec Trench and includes over 800 distinct sea features (Rowden et al., 2005; Linley et al., 2017).
Figure 1. Map of the study region. New Zealand Exclusive Economic Zone (EEZ, black dashed line), bathymetry, and feature names used throughout the text modified from Stephenson et al. (2020a), and the location of basking shark records used in this study (black dots).
Basking shark records (n = 401) were collated from various sources including records from observed and self-reported captures in commercial fisheries, public sightings, media reports, museum records, fishery surveys, aerial surveys and beach cast specimens. Records variously included information on date, number of individuals, geographic co-ordinates and source (where available) and spanned a period of 131 years (1889–2020). The only directed sampling effort for New Zealand basking sharks were aerial surveys off the east coast of the South Island over the summer months (January to March) of 2010–2011. No sharks were observed during these surveys. The data were groomed to ensure accurate identification and only records that were confirmed or probable basking shark observations within the New Zealand EEZ were retained. Where geographic co-ordinates were missing an approximate position was assigned based upon the description of the location. This was necessary for all aerial survey records, media reports and public sightings. Because of difficulties in correcting for differences in sampling methods, all catch records were converted into presence records (Elith et al., 2011; Stephenson et al., 2018). To minimize the effect of spatial bias in the occurrence data, species records were aggregated spatially to a 1 km2 grid resolution (Aiello-Lammens et al., 2015; Stephenson et al., 2020b). Strandings and records without an approximate date reference (month) were removed. The final dataset included presence records of basking sharks at 369 unique sampling locations (Figure 1).
To characterize variability in the New Zealand marine environment, spatial environmental and biotic variables were collated at a 1 km2 grid resolution, with each spanning the breadth of the New Zealand EEZ (Table 1 and Supplementary Table 1, further details are available in Stephenson et al., 2020a). In addition to these variables, spatial estimates of various zooplankton densities (inferred prey for basking shark) (Pinkerton et al., 2020) were used as biological predictors in the models (Supplementary Table 1). Estimates of zooplankton densities did not cover the entire New Zealand EEZ (Supplementary Figures 1.9–1.15). Areas lacking this information will simply represent the modeled relationship between basking shark records and the environmental variables. A preliminary examination of currently available zooplankton density estimates reveals these are likely to cover core areas of basking shark distribution.
Table 1. Spatial environmental and biotic predictor variables included in the final models, collated for species distribution models from Stephenson et al. (2020a).
Of the available environmental and biotic variables, a subset was selected to be used in the models (Table 1) based on model tuning described in section “Predictor Variable Selection.” Although most of the chosen environmental variables were static (e.g., bathymetry, Bathy), several variables were dynamic in time, representing mean monthly statistics for the past 20 years (e.g., chlorophyll-a concentration, Chl-a, “temporal resolution” column in Table 1). The environmental data spans a much shorter timeframe than the basking shark observations (131 years), and thus, long-term trends in habitat suitability could not be examined. Prior to fitting of the habitat suitability models, values for each environmental and biotic variable were extracted for locations of basking shark records by overlaying the records onto each of the environmental and biotic variable layers using the “raster” package in R (Hijmans and van Etten, 2012). For dynamic environmental variables (mean monthly statistics), recorded dates of basking shark records were used to extract respective values from the month the record was made.
Habitat Suitability Models (HSMs) were used to analyze and spatially predict the distribution of basking shark habitat suitability (measured as habitat suitability index—HSI). Acknowledging that our environmental predictors are mean (or mean monthly) averages for the past 20 years, we explored models for two time periods: 1889–2020 (all data, n = 369) and as subset of basking shark occurrence which matched the time frame of our environmental predictors 2000–2020 (n = 123). The relationship between environmental variables, biotic variables and basking shark records was explored using ensemble predictions (Ensemble HSM) from Boosted Regression Tree (BRT) and Random Forest (RF) models. This approach limits dependence on a single model type or structural assumption and may result in a more robust characterization of the predicted spatial variation and uncertainties (Robert et al., 2016). To estimate basking shark distributions, BRT and RF models require locations of both presences (occurrence records) and absences. Here, true absences (i.e., sample locations where no basking sharks were recorded) were not available for opportunistic records such as public sightings, media reports, or museum records. True absences were also unavailable for the non-opportunistic sampling methods (i.e., trawl tows, observer records, scientific surveys), particularly from commercial records which are complicated with the inclusion of multiple gear types and fishing protocols (thus affecting catchability) and issues regarding a lack of reporting of basking shark interactions (Francis, 2017). Therefore, presence only modeling approaches using pseudo-absences (i.e., locations where basking sharks were not recorded within our study area) were necessary.
A two-dimensional kernel density estimate (KDE) was produced using all basking shark locations (presence data) using a cell size of 1 km2 and a default bandwidth (Supplementary Figure 2). Within the KDE, the 95% percentage volume contour (minimum area in which 95% of the KDE value is located) was selected (Calenge, 2006). The 95% KDE was used to create a probability grid from which pseudo-absences were sampled according to the probability of grid weights (that is, where KDE values were high, the chance of selecting an absence was high) (Georgian et al., 2019). Pseudo-absences were generated through random selection of points from within the probability grid except within a 1 km2-grid radius of the presence localities. By selecting pseudo-absences in this manner, the pseudo-absences were subject to the same sampling bias as the presence data. This method has been shown to increase the accuracy of presence only BRT and RF models (Elith et al., 2010; Cerasoli et al., 2017; Georgian et al., 2019). Following recommended best practice, the number of pseudo-absences selected by month were equivalent to the number of monthly presences (Barbet-Massin et al., 2012).
In most cases, the inclusion of many variables (e.g., >20 variables) in tree-based machine learning models (i.e., BRT and RF) is avoided because they only provide minimal improvement in predictive accuracy, and complicate interpretation of model outcomes (Leathwick et al., 2006). As the interpretation of drivers of distribution of basking shark was a key requirement, a reduction in the number of predictor variables was undertaken in order to produce a parsimonious model. A BRT model was initially fitted using all available environmental variables which was then subjected to a simplification process whereby environmental variables were removed from the models, one at a time, using the “simplify” function (Elith et al., 2006). This simplification process firstly assesses the relative contributions of each variable in terms of deviance explained, with the lowest contributing variables removed from the model. The model is then refitted with the remaining environmental variables. The change in deviance explained that resulted from removing the variable was then examined and the process repeated until the deviance explained decreased by >1% between removal of predictor variables. Despite having a relatively small influence on the model, Chl-a was retained as this predictor was found to be an important predictor of basking shark distribution elsewhere (Austin et al., 2019).
The final variables retained for modeling were Bathymetry, BPI broad, Chl-a, mixed layer depth (MLD), Turbidity, POCFlux, Slope, and SST (Table 1), as well as Copepoda, a known prey species for basking sharks (Grieve, 1966). Several environmental variables showed some co-linearity (Supplementary Figure 3) however, all levels of co-linearity were considered acceptable for tree-based machine learning methods (Pearson correlation < 0.75; Elith et al., 2010; Dormann et al., 2013). The “final” environmental variables selected through this approach were also used in RF models (Supplementary Table 1 and Supplementary Figures 1.1–1.8). Partial dependence plots were made for the BRT and RF models to evaluate the effect of each predictor on species’ distribution by plotting the effect of the predictor on the response (basking shark presence) after accounting for the average effects of all other model predictors (Elith et al., 2008).
BRT modeling combines many individual regression trees (models that relate a response to their predictors by recursive binary splits) and boosting (an adaptive method for combining many simple models to give improved predictive performance) to form a single ensemble model (Elith et al., 2008). Detailed descriptions of the BRT method are available in Ridgeway (2007) and Elith et al. (2008). All statistical analyses were undertaken in R (R Core Team, 2020) using the “Dismo” package (Hijmans et al., 2017). BRT models were fitted with a Bernoulli error distribution, a tree complexity of 2, a learning rate of 0.01 (with parameters selected so as to fit trees for each bootstrapped model), a bag fraction of 0.7 and random 10-fold cross evaluation following recommendations from Leathwick et al. (2006) and Elith et al. (2008). The BRT method has been widely used in ecological applications and has performed well in previous studies of fish, zooplankton, and cetacean distributions in New Zealand (Leathwick et al., 2006; Compton et al., 2013; Pinkerton et al., 2020; Stephenson et al., 2020b).
RF models fit an ensemble of regression (abundance data) or classification tree (presence/absence data) models describing the relationship between the distribution of an individual species and some set of environmental variables (Ellis et al., 2012). Following environmental and biotic predictor variable selection using the BRT model, RF models were fitted using the R package “randomForest” (Breiman, 2001) and were tuned using the train function in the R package “caret” (Kuhn et al., 2020). The train function selects optimal values for the complexity parameters mtry (the number of variables used in each tree node), maxnodes (the maximum number of terminal nodes in each trees), and ntree (the number of trees to grow).
BRT and RF models were bootstrapped 200 times. A random “training” sample with a sample size equal to the number of presence records was drawn with replacement. A random sample of pseudo-absences of equal number was drawn without replacement from the full set of available pseudo-absences stratified by month (to match the monthly environmental data) (Barbet-Massin et al., 2012) and the models were run using these presence-pseudo absence records. Presence records which were not randomly selected were combined with a random number of pseudo-absences and were set aside for independent assessment of model performance (referred herein as “evaluation” data). At each BRT and RF model iteration, geographic predictions were made using environmental predictor variables to a 1 km2 grid. Given that BRT and RF models used pseudo-absences, we refer to our outputs as “habitat suitability” (rather than the commonly used “probability of occurrence”) because we did not have information on “catchability” or “sightability” of basking sharks from the different sampling methods nor did we have estimates of species prevalence (Anderson et al., 2016; Georgian et al., 2019). HSI and a spatially explicit measure of uncertainty (measured as the standard deviation of the mean predicted HSI) were calculated for each grid cell using the 200 bootstrapped layers.
BRT and RF model performance were evaluated using AUC (area under the Receiver Operating Characteristic curve) and TSS (True Skill Statistic). AUC is an effective measure of model performance and a threshold-independent measure of accuracy, while the TSS is a threshold-dependent measure of accuracy, but is not sensitive to prevalence (Allouche et al., 2006; Komac et al., 2016). AUC scores range from 0 to 1, with a score of 0.5 indicating model performance is equal to random chance, a score > 0.7 indicating adequate performance, and a score > 0.80 indicating excellent performance (Hosmer et al., 2013). TSS, which takes into account Specificity (correct prediction of absence) and Sensitivity (correct prediction of presence) to provide an index ranging from –1 to +1, where +1 equals perfect agreement and –1 is no better than random, Allouche et al. (2006). A TSS value > 0.6 is considered useful (Allouche et al., 2006). Model fit metrics were calculated using both the “training” dataset and the “evaluation” dataset. The latter is considered a more robust and conservative method of evaluating goodness-of-fit of a model than using the same data with which the model was trained (Friedman et al., 2001).
We produced an ensemble model by taking weighted averages of the predictions from each model type, using methods adapted from Anderson et al. (2016, 2020) and Georgian et al. (2019). This adapted procedure derives a two-part weighting for each component of the ensemble model, taking equal contributions from the overall model performance (AUC value derived from the “evaluation”) and the uncertainty measure (SD) in each cell, as follows:
where AUCBRT and AUCRF are the model performance statistics; XBRT and XRF are the model predictions; SDBRT and SDRF are the bootstrap SDs; and XENS and SDENS are the weighted ensemble predictions and weighted SDs, respectively, from which maps of predicted species distribution and model uncertainty were produced.
Two measures of spatially explicit uncertainty were produced: an estimate of our spatial coverage of species occurrence (95% KDE) and the standard deviation of the predicted basking shark distribution (i.e., model uncertainty). The calculated spatial coverage of species occurrence was assumed to be indicative of basking shark distributions, and thus, is presumed to have more certain predictions of the species’ distribution of suitable habitat. Where predictions were projected outside the spatial coverage of species occurrence (i.e., where there are few or no sightings), it is assumed that the relationship between the environment and species’ records may be less robust and thus predictions outside this range contain some degree of uncertainty (e.g., similarly to the methods used in Stephenson et al., 2020b). Standard deviation (SD) of the mean predicted habitat suitability were estimated through the bootstrapping methods outlined in section “Bootstrapping the Models” and are provided as uncertainty estimates of basking shark distribution.
Ensemble model performance was assessed using AUC and TSS by comparing ensemble model predictions to all basking shark presence records and an equal number of randomly selected pseudo-absence data. To ensure that the random selection of pseudo-absence data did not provide misleading model performance metrics, this procedure was iterated 50 times and mean AUC and TSS score calculated for the ensemble model (Barbet-Massin et al., 2012). Ensemble partial dependence plots were created with an average of the BRT and RF partial dependence plots.
Model performance and outputs for the two time-series were found to be very similar and the outputs for the longer time series (1889–2020) are reported in the Results. The final model shown here is a temporally and spatially smooth prediction of basking shark HSI in New Zealand waters. By including all available data points, the model has retained a substantial amount of the New Zealand environment, including areas known to be historically important for basking sharks (e.g., east coast of the South Island). Inclusion of historical data across a long temporal span has been shown to achieve the highest model performance in SDMs, particularly when presence-only data is available (Lütolf et al., 2006). Model performance, partial dependence plot and predicted habitat suitability for the shorter time series (2000–2010) are found in the Supplementary Table 2 and Supplementary Figures 8, 9).
Of the records retained for use in the models, most basking shark records (72%, n = 265) occurred in the spring and summer months (September to February). Most (69%) records came from fishing interactions (trawl = 244, set net = 7, surface longline = 3), followed by public sightings (13%, n = 47), aerial surveys (11%, n = 41), research vessels (4%, n = 14), and alternative capture methods (e.g., harpoon) or unknown sources (4%, n = 13). Since 2000, most records (84%, n = 103) have been from fishing events, with one aerial record and 19 opportunistic sightings. The most recent coastal sighting and coastal fishing interaction (set net) occurred in March 2012 and August 2014, respectively, and the last known report of a school (≥3 individuals) was from April 2013 where seven individuals were captured in one trawling event. Estimated lengths were available for 169 records (42%); 32 records were <5 m (19%), 126 records were 5–10 m (74.5%), and 11 records were >10 m (6.5%) (Supplementary Figure 4). For observations where length was recorded, sex was available for 81 of these records (20% of all basking shark observations). Most sharks (89%, n = 72) were male and nine (11%) were female (Supplementary Figure 5).
AUC and TSS scores using evaluation data were very similar between models, with the RF model performing slightly better than the BRT model (AUC: 0.92 and 0.89; TSS: 0.72 and 0.69, respectively, Table 2). Both indices indicated the models were useful in predicting basking shark occurrence (>0.7). Measures of BRT and RF model performance scores had low variability (measured by the standard deviation of the mean), suggesting the models were performing consistently across bootstrap samples. Model fits between training data and evaluation data were similar, with model fits for the evaluation data slightly lower than the training data (as would be expected). The similarity of these fits provides some indication that the training data were not overfitted in the models.
Table 2. Mean cross-validated estimates of model performance for the bootstrapped boosted regression tree (BRT) and random forest (RF) models (time series 1889–2020).
The relative importance of each predictor and their influence on basking shark habitat suitability were consistent across BRT and RF models (Supplementary Figures 6, 7). The three most important variables in predicting basking shark habitat suitability were vertical flux (POCFlux, with 26.0% influence on the response), slope (Slope, 14.1%), and turbidity (Turbidity, 10.6%) (Figure 2). Bathymetry (Bathy, 9.7%) and BPI broad (BPI broad, 9.6%) were also moderately important variables. There was a strong positive relationship of predicted basking shark HSI with vertical flux, highest in areas where vertical flux was 20 mgC m–2 d–1 or greater than what would be expected for the given depth. High HSI was predicted in gently sloping and less complex seafloor topographies with moderate turbidity. Two depth strata had high HSI—nearshore depths and depths between 200 and 550 m. A less clear relationship was observed between HSI and SST and mixed layer depth (MLD), with low HSI occurring between temperatures of 12.5 and 15°C and in areas where the mixed layer depth was approximately 75 m. There was a weak relationship between HSI and copepod (Copepoda) densities, with low HSI occurring with low levels of copepod densities, a peak in HSI at moderate copepod densities (10–20 counts per 5 nautical miles), and a plateau in HSI values at the highest levels of copepod densities (>25 counts per 5 nautical miles). HSI was lowest at moderate levels of chl-a concentration (Chl-a) (0.5–1.0 mg m–3) and highest at high chl-a concentration (>1.2 mg m–3).
Figure 2. Partial dependence plots of the mean boosted regression tree (BRT) and random forest (RF) models for the nine variables (time series 1889–2020), showing the influence of each predictor variable on the response. Variables are ordered by influence as indicated in top left hand of plots. Shaded area represents standard deviation.
Areas of predicted high habitat suitability for basking sharks in New Zealand waters occurred along the continental slope, particularly along the 250 m contour along the North and South Islands, Mernoo Bank, Pukaki Rise, Puysegur Ridge, and around New Zealand’s offshore islands (Chatham Islands, Stewart Island, Bounty Islands, and Auckland Islands) (Figures 3, 4). Within the spatial coverage of species occurrence, areas of moderate uncertainty (SD > 0.2) included most offshore waters north of 40°S, the deeper depths (>500 m) of the Hokitika Canyon, northern Chatham Rise, coastal waters off east coast of the South Island (Canterbury Bight), Foveaux Strait and Puysegur Ridge (Figure 5). The northern North Island and features further from the continental shelf, including the eastern half of the Chatham Rise were outside of the estimated spatial coverage (95% KDE) of species occurrence. In addition, moderate—high uncertainty (SD > 0.2) was reported along deep sea features north of New Zealand, including the Kermadec Ridge and Trench, the Lau-Colville Ridge, and the Norfolk Ridge (Figure 5).
Figure 3. The predicted habitat suitability index (HSI) of basking shark in the New Zealand Exclusive Economic Zone (EEZ) from 1889 to 2020 modeled using the bootstrapped ensemble models for (A) west coast South Island; (B) east coast South Island; (C) south of South Island including Puysegur Ridge and Stewart Island; (D) Chatham Islands; and (E) Auckland Islands. Areas outside 95% kernel density estimate (KDE) probability grid indicating lower confidence that can be placed in the predicted probability occurrence are covered by crossed black lines. Note that the Chatham Islands (D) is outside the KDE probability grid estimate.
Figure 4. The predicted habitat suitability index (HSI) of basking shark in the New Zealand Exclusive Economic Zone (EEZ) from 1889 to 2020 modeled using the bootstrapped ensemble models. Areas outside 95% kernel density estimate (KDE) probability grid indicating lower confidence that can be placed in the predicted probability occurrence are covered by crossed black lines.
Figure 5. Standard deviation (SD) of the predicted habitat suitability index (HSI) of basking shark in the New Zealand Exclusive Economic Zone (EEZ) from 1889 to 2020 modeled using the bootstrapped ensemble models. Areas outside 95% kernel density estimate (KDE) probability grid indicating lower confidence that can be placed in the predicted probability occurrence are covered by crossed black lines.
This study has provided the first insight into habitat suitability for basking sharks in the Southwest Pacific. Our approach assessed habitat suitability by incorporating a combination of static and temporally dynamic environmental (n = 7), biotic (n = 1), and inferred prey (n = 1) predictors into ensembled HSI models. The BRT and RF models had good predictive power (AUC and TSS > 0.7) and both models performed similarly with low variability in the model fit metrics. The outputs produced here will be useful for fisheries risk assessment (e.g., spatially explicit risk assessment), as well as providing guidance for future research efforts (e.g., areas of interest for future sampling). However, caution should be considered given the relatively few species presence records and lack of true absence data.
Basking shark habitat suitability was largely influenced by variables representing ocean processes. The environmental predictors used in this work were comprehensive and many were dynamic (i.e., monthly means were available). Overall, areas with high levels of vertical flux of particulate organic matter at the seabed had high habitat suitability. This is likely indicative of higher levels of primary production in the surface ocean and higher prey density in the mesopelagic zone and at the seafloor and may be a suitable proxy when prey data is unavailable. The biotic predictive layers included here were found to have lower influence on habitat suitability compared to some of the environmental predictors. However, prey availability is highly patchy and temporally variable; thus, it is likely that a static variable reflecting prey abundance was unable to accurately represent the spatial distribution of prey. The inclusion of biotic predictors in the model is important in understanding species’ relationship with the marine environment in unobserved space and has been identified as a potential link in understanding effects of climate change. In the Northeast Atlantic, basking sharks are often observed in shallow, highly productive coastal waters during spring and summer months where they feed on zooplankton blooms (Sims, 2008). Historically, basking sharks were commonly observed in similar environments (e.g., east coast of the South Island) around New Zealand (Francis and Duffy, 2002). Although prey preference for New Zealand sharks is poorly understood, there is some relationship between New Zealand basking shark distribution and copepod abundance, as seen in the North Atlantic (Sims and Merrett, 1997).
The inclusion of dynamic (mean monthly) environmental variables here may allow the models to capture temporal change in patterns of basking shark distribution, including seasonal changes and interannual variability. Despite the availability of dynamic environmental predictors, true temporal changes in distribution are difficult to confirm due to the limited amount of biological data (basking shark observations) available. However, in our results, both inshore and offshore regions were highlighted as areas of high habitat suitability. This is particularly evident in the bimodal effect of the bathymetry predictor, where basking shark habitat suitability was observed to be highest in very shallow depths (<100 m), and again at depths between 200 and 500 m. This result is consistent with previous work where basking sharks have been shown to exhibit seasonal vertical space use in the Northeast Atlantic, with tagged individuals occupying shallow depths (<100 m) in the summer months and depths greater than 1,000 m in late winter/early spring (Doherty et al., 2019).
While bathymetry (and slope) were also found to be important predictors, their effect may be partially influenced by basking shark availability to fisheries (see below). Basking sharks have been shown to dive as deep as 1,264 m and have been regularly documented at depths of 600–1100 m (Francis and Duffy, 2002; Gore et al., 2008; Doherty et al., 2017). The species has also been shown to follow distinct water masses at depth, remaining at depths of 250 m or more for months without coming to the surface (Braun et al., 2018; Dewar et al., 2018). Basking sharks are known for complex diel vertical movements, which are thought to be influenced by shifts in prey availability and oceanography (Sims et al., 2005; Dewar et al., 2018). In well-stratified deep waters, basking sharks exhibit normal diel vertical movements (shallow depths at night, deeper depths during daylight), while sharks occupying inshore, inner-shelf areas near thermal fronts conduct reverse diel vertical movements (shallow depths during the day, deeper depths at night) (Sims et al., 2005). This may explain, at least in part, why more contemporary reports of basking sharks in deepwater fisheries have been made during daylight hours (when sharks are occurring at their preferred deeper depth range) but does not offer insight into the disappearance of inshore observations or why individuals are no longer seen at the surface in the region.
Water temperature had relatively minimal influence on basking shark distribution. Basking sharks appear to have a broad thermal range and are therefore relatively unrestricted by temperature (Sims et al., 2003). They are also known to cross tropical regions by submerging into deeper, colder water (Skomal et al., 2009), and one individual was encountered in tropical waters off Indonesia (Fahmi and White, 2015). While gradual changes in sea temperatures may have minimal effect on basking sharks, ocean heat waves and extreme processes associated with SSTs might be more relevant and are expected to become more prevalent with climate change. By 2100, climate change projections predict SST will increase by 2.5°C, which in turn is predicted to lead to a deepening in the surface mixed layer depth (by 15%), declines in primary production (4.5%) and particle flux (12%); the largest changes in macronutrients are predicted in eastern Chatham Rise and southern Sub-Antarctic waters (Law et al., 2018). Such changes may alter food availability (prey distribution and abundance) for basking sharks resulting in shifts in their distribution.
Predictors found to positively influence basking shark HSI could be further explored to better understand historic and future basking shark distribution. Predictors including chl-a concentration and vertical flux are often used as an index of phytoplankton abundance (primary production) and are strongly linked to primary consumers such as copepods. In recent decades, dramatic shifts in chl-a concentration have been reported in the South Pacific and the Southern Oceans (Del Castillo et al., 2019). Significant declines in chl-a concentration were observed in spring and summer months in the South Pacific from 1979 to 2000, while significant increases were linked to extreme summer marine heatwaves in the Southern Ocean between 2002 and 2018 (Gregg and Conkright, 2002; Montie et al., 2020). Highly variable oceanographic conditions around New Zealand are strongly correlated with climate phenomenon such as El Niño–Southern Oscillation (ENSO) (Bradford-Grieve et al., 2006). In the Northern Hemisphere, basking shark movement patterns have been linked to shifts in prey availability and oceanography (Sims et al., 2005; Gore et al., 2008; Dewar et al., 2018). One tagged individual was shown to remain in an area with putative upwelling and high abundance of phytoplankton in the Western Atlantic for up to a month (Gore et al., 2008). In the Northeast Atlantic, a northward shift in basking shark distribution in response to long-term zooplankton declines was found to correspond with declines in basking shark catch in Irish fisheries to the south from 1948 to 1975 (Sims and Reid, 2002). Similar models used in this project could be explored to predict basking shark distribution response to future climate change forecasting and events such as ENSOs [e.g., Earth System Models, Anderson et al. (2020)].
Areas of high basking shark habitat suitability included the east and west coasts of the South Island, Puysegur Ridge, and the Auckland Island slope. Some areas of Chatham Rise, specifically around Mernoo Bank (including Mernoo Saddle) and off the southern slope of Pitt Island (Chatham Islands), were also identified as areas of high habitat suitability. Much of Chatham Rise, however, was outside the spatial coverage of species occurrence and thus habitat suitability predictions hold a higher degree of uncertainty. Chatham Rise is a known hotspot for chondrichthyan diversity in New Zealand waters (Wetherbee, 2000), but interestingly, basking sharks have very rarely been reported from the area. Chatham Rise, as well as Puysegur Ridge, have relatively low densities of copepods (see Supplementary Figure 1.9) and may not be optimal feeding grounds for basking sharks. However, in international waters east and north-east of Chatham Rise, 15 juvenile basking sharks (180–310 cm total length) were reported by Japanese drift net vessels operating at shallow depths (10 m) (Yatsu, 1995). This report suggests juvenile sharks may inhabit epipelagic waters in the open ocean (Francis, 2017).
Given the long temporal span of the data, model predictions may be more representative of past, rather than current habitat suitability, particularly some inshore parts of the predicted distribution. Basking sharks are occasionally recorded from northern New Zealand and were reported to be regular visitors to the Hauraki Gulf during spring in the late nineteenth century (Cheeseman, 1891). The predictions presented here are smoothed over time, as there is a mismatch between the availability of basking shark records (131 years) and environmental data (approximately 20 years). It is possible that the models have highlighted seasonal patterns of distribution by indicating both inshore and offshore regions as areas of high habitat suitability with the presence of bimodality in the bathymetry HSI. However, these patterns could also be indicative of a shift to deeper, offshore habitats in recent decades.
There were several areas where the spatially explicit uncertainty (measured as the SD) was relatively high, indicating the relationship between basking sharks and the environment was more uncertain. Our understanding of basking shark use of the pelagic habitat remains relatively unknown, largely due to the spatial bias in observations (e.g., lack of open ocean pelagic research surveys). In areas with high uncertainty, such as Cook Strait, the northern Chatham Rise, and Foveaux Strait, few basking shark sightings where available and uncertainty might be linked to low sample size. Uncertainties regarding the most northern predictions of habitat suitability (north of 40°S) may, in part, be explained instead by a lack of information on copepod density north of 40°S (Pinkerton et al., 2020), but is more likely associated with a lack of basking shark data. Despite a lack of surface sightings around northern New Zealand, there is some evidence of basking shark captures in deepwater trawl fisheries off central Hawke’s Bay. Basking sharks may be present along the upper slope around northern New Zealand but there is no fisheries-independent data (research or public sightings) to indicate their presence. In the Kermadec Islands where there is minimal human presence, there is one unconfirmed stranding of a basking shark from Raoul Island (Morton, 1957).
The estimate of spatial coverage of species occurrence (top 95% of the KDE of basking shark occurrences) provides a representation of the likely geographic (and in turn environmental) space occupied by basking sharks within New Zealand waters. Predicted distribution outside of this area, should be treated with caution as this represents prediction into largely unsampled space. In this study, the environmental threshold reflects the distribution of presences only—and thus retains any spatial biases associated with these datasets. In particular, the spatial distribution of presences is related to the distribution of fishing effort and human population centers (for opportunistic sightings) and may not be an accurate representation of hotspots. However, using the top 95% of the KDE of basking shark occurrences provides a more conservative estimate of this species’ spatial distribution, which can be useful in determining when modeled predictions are occurring outside of sampled environmental space. This measure provides a meaningful threshold with which to classify broad areas as “uncertain.”
The lack of basking shark records in New Zealand waters during recent years highlights the need to better understand their overall abundance, distribution, movements, and habitat use in the South Pacific Ocean as well as changes to the sighting effort. Currently data collection on the species in the Southern Hemisphere is heavily reliant on interactions with fisheries, especially trawl fisheries (Francis and Smith, 2010; Hernández et al., 2010; Lucifora et al., 2015; Francis, 2017). Because of this, our knowledge of New Zealand basking shark distribution is essentially limited to areas of relatively high historic and current trawl fishing effort (Clarke et al., 2017; Baird and Wood, 2018). Most basking shark interactions occur during the spring-summer months, corresponding to when fishing vessels target commercially important species, such as spawning aggregations of arrow squid (Nototodarus sloanii) (Hurst et al., 2012). As a protected species, it is mandatory to report basking shark interactions with fisheries. However, there is uncertainty in the levels of reporting, and observer coverage is relatively low in some fisheries (e.g., inshore fisheries), particularly around northern New Zealand, so that presence records are likely underestimated (Francis, 2017). Understanding habitat use will assist in assessing risk to fishing activities and could be incorporated into management frameworks such as the spatially explicit risk assessment that New Zealand has in place for other protected species (Large et al., 2019).
Identifying areas of high habitat suitability could also assist in decision making processes for future research efforts. Previous research has identified the need to tag free-swimming basking sharks to better understand species movement, habitat use, and interactions with fisheries (Francis, 2017). This will require the ability to find individuals at the surface, and at an accessible location. Meeting these requirements has prevented any efforts of tagging New Zealand basking sharks to date. In the Atlantic Ocean, basking sharks have been successfully tagged off Cape Cod, Massachusetts and the west coast of Scotland and Isle of Man (Skomal et al., 2009; Doherty et al., 2017; Hawkes et al., 2020) and in the Pacific, off San Diego and Monterey Bay, California (Dewar et al., 2018). By identifying areas of high habitat suitability, research efforts can be directed to specific areas of interest to increase the tagging success. For example, the Auckland Islands has been identified as an area of high habitat suitability for basking sharks where basking sharks were historically sighted at the surface (Parrott, 1958). This area is also a known hotspot for other large filter-feeding vertebrates that feed along the Subtropical Front (STF), a continuous feature within the Southern Tropical Convergence at latitudes 39°–42°S, characterized by elevated primary productivity (Murphy et al., 2001; Rayment et al., 2015; Mackay et al., 2020). Southern Ocean oceanographic fronts have been identified as important foraging areas for a range of marine predators (Bost et al., 2009) and may also be important for basking sharks.
Differences in habitat suitability among sexes or size classes, a common observation among sharks, were not examined at this time due to the relatively small sample size of basking sharks across the region and low availability of size and sex data for most records. This information is becoming more readily available through fisheries observer data collection and should be explored further in the future. New Zealand has a considerable number of records of small (<5 m) sharks, accounting for nearly 20% of records where length has been recorded. Some of the most recent reports of basking sharks in New Zealand waters have been juvenile individuals, including a 3 m female captured east of the Auckland Islands in January 2018 and a 3.3 m male captured off the west coast South Island in August 2020. Both individuals were released alive. Continued collection of biological data on basking sharks is essential for understanding differences in habitat use across life history stages, particularly for juvenile basking shark as they are globally rare and their habitat preference is unknown.
More data on at-sea distribution of basking sharks is required to understand habitat use, threat overlap, and population status throughout the New Zealand and South Pacific region. The total South Pacific basking shark population size is unlikely to be high; in the Northeast Atlantic, basking shark numbers likely do not exceed 10 000 individuals (Lieber et al., 2020). With little population differentiation across global regions, it is plausible that basking sharks observed in New Zealand waters engage in large scale intra- and inter-oceanic migrations (Lieber et al., 2020). This may make species’ detection more difficult in the vast marine space of New Zealand’s EEZ. In the past, aerial surveys have been successful in detecting basking sharks distributed over relatively large spatial scales in New Zealand, and elsewhere for estimating regional population sizes (Francis and Duffy, 2002; Westgate et al., 2014). However, surveys conducted in 2010–2011 off Banks Peninsula failed to locate any basking sharks (Chapman and Duffy, 2011). These surveys have not been repeated since. Basking sharks may travel or feed in subsurface habitat, and therefore go undetected in aerial surveys. Alternative means of tracking these animals, such as autonomous underwater vehicles (AUV) (Hawkes et al., 2020) offer great potential for the future.
The raw data supporting the conclusions of this article will be made available by the authors, without undue reservation, to any qualified researcher.
CD and FS conceived the project. BF, FS, MF, CD, and MP collected the data. BF and FS analyzed the data and wrote the draft manuscript. GP created the maps. All authors discussed the results, contributed to the final manuscript, and approved the submitted version.
This project was funded by the Department of Conservation, Wellington, New Zealand (project CSP POP2020-03).
The authors declare that the research was conducted in the absence of any commercial or financial relationships that could be construed as a potential conflict of interest.
Thanks to Jade Maggs (NIWA) and Fisheries New Zealand (FNZ) RDM for data extractions. Thank you to Ben Sharp (FNZ) for feedback on the methods. Satellite data are used courtesy of NASA (MODIS, SeaWiFS ocean color) and NOAA (AVHRR, sea-surface temperature). Mixed-layer depth data are used courtesy of Oregon State University “ocean primary productivity” project.
The Supplementary Material for this article can be found online at: https://www.frontiersin.org/articles/10.3389/fmars.2021.665337/full#supplementary-material
Aiello-Lammens, M. E., Boria, R. A., Radosavljevic, A., Vilela, B., and Anderson, R. P. (2015). spThin: an R package for spatial thinning of species occurrence records for use in ecological niche models. Ecography 38, 541–545. doi: 10.1111/ecog.01132
Allouche, O., Tsoar, A., and Kadmon, R. (2006). Assessing the accuracy of species distribution models: prevalence, kappa and the true skill statistic (TSS). J. Appl. Ecol. 43, 1223–1232. doi: 10.1111/j.1365-2664.2006.01214.x
Anderson, A., Stephenson, F., and Behrens, E. (2020). Updated Habitat Suitability Modelling for Protected Corals in New Zealand Waters. NIWA Report Prepared for Department of Conservation (DOC) NIWA CLIENT REPORT No: 2020174WN). Kilbirnie: National Institute of Water & Atmospheric Research Ltd.
Anderson, O. F., Guinotte, J. M., Rowden, A. A., Tracey, D. M., Mackay, K. A., and Clark, M. R. (2016). Habitat suitability models for predicting the occurrence of vulnerable marine ecosystems in the seas around New Zealand. Deep Sea Res. I Oceanogr. Res. Pap. 115, 265–292. doi: 10.1016/j.dsr.2016.07.006
Araújo, M. B., and Luoto, M. (2007). The importance of biotic interactions for modelling species distributions under climate change. Glob. Ecol. Biogeogr. 16, 743–753. doi: 10.1111/j.1466-8238.2007.00359.x
Austin, R. A., Hawkes, L. A., Doherty, P. D., Henderson, S. M., Inger, R., Johnson, L., et al. (2019). Predicting habitat suitability for basking sharks (Cetorhinus maximus) in UK waters using ensemble ecological niche modelling. J. Sea Res. 153:101767. doi: 10.1016/j.seares.2019.101767
Baird, S. J., and Wood, B. A. (2018). Extent of Bottom Contact by New Zealand Commercial Trawl Fishing for Deepwater Tier 1 and Tier 2 Target Fishstocks, 1989–90 to 2015–16. New Zealand Aquatic Environment and Biodiversity Report No. 193. Wellington: Ministry for Primary Industries.
Barbet-Massin, M., Jiguet, F., Albert, C. H., and Thuiller, W. (2012). Selecting pseudo-absences for species distribution models: how, where and how many? Methods Ecol. Evol. 3, 327–338. doi: 10.1111/j.2041-210X.2011.00172.x
Behrenfeld, M. J., and Falkowski, P. G. (1997). Photosynthetic rates derived from satellite-based chlorophyll concentration. Limnol. Oceanogr. 42, 1–20. doi: 10.4319/lo.1997.42.1.0001
Bost, C. A., Cotté, C., Bailleul, F., Cherel, Y., Charrassin, J. B., Guinet, C., et al. (2009). The importance of oceanographic fronts to marine birds and mammals of the southern oceans. J. Mar. Syst. 78, 363–376. doi: 10.1016/j.jmarsys.2008.11.022
Bradford-Grieve, J., Probert, K., Lewis, K., Sutton, P., Zeldis, J., and Orpin, A. (2006). “New Zealand shelf region,” in The Sea, Vol 14: The Global Coastal Ocean: Interdisciplinary Regional Studies and Syntheses, eds A. Robinson and H. Brink (Cambridge, MA: Harvard University Press).
Braun, C. D., Skomal, G. B., and Thorrold, S. R. (2018). Integrating archival tag data and a high-resolution oceanographic model to estimate basking shark (Cetorhinus maximus) movements in the Western Atlantic. Front. Mar. Sci. 5:25. doi: 10.3389/fmars.2018.00025
Cael, B., Bisson, K., and Follett, C. L. (2018). Can rates of ocean primary production and biological carbon export be related through their probability distributions? Glob. Biogeochem. Cycles 32, 954–970. doi: 10.1029/2017GB005797
Calenge, C. (2006). The package “adehabitat” for the R software: a tool for the analysis of space and habitat use by animals. Ecol. Model. 197, 516–519. doi: 10.1016/j.ecolmodel.2006.03.017
Carroll, G., Holsman, K. K., Brodie, S., Thorson, J. T., Hazen, E. L., Bograd, S. J., et al. (2019). A review of methods for quantifying spatial predator–prey overlap. Glob. Ecol. Biogeogr. 28, 1561–1577. doi: 10.1111/geb.12984
Cerasoli, F., Iannella, M., D’Alessandro, P., and Biondi, M. (2017). Comparing pseudo-absences generation techniques in Boosted Regression Trees models for conservation purposes: a case study on amphibians in a protected area. PLoS One 12:e0187589. doi: 10.1371/journal.pone.0187589
Chapman, D. D., and Duffy, C. A. J. (2011). Vanishing Giants: Where are New Zealand’s Basking Sharks?. Palmerston: National Geographic Society.
Cheeseman, T. F. (1891). Notice of the occurrence of the basking shark (Selache maxima, L.) in New Zealand. Trans. Proc. New Zeal. Inst. 23, 126–127.
Chiswell, S. M., Bostock, H. C., Sutton, P. J. H., and Williams, M. J. M. (2015). Physical oceanography of the deep seas around New Zealand: a review. New Zeal. J. Mar. Freshw. Res. 49, 286–317. doi: 10.1080/00288330.2014.992918
CITES (2002). Consideration of Proposals for Amendment of Appendices I and II. Proposal: inclusion of Basking Shark (Cetorhinus maximus) on Appendix II of CITES. Prop. 12.36.
Clarke, S. C., Smith, N., Lyon, W., and Francis, M. (2017). “Western and Central Pacific Fisheries Commission shark post-release mortality tagging studies,” in WCPFC Scientific Committee 13th regular session, (Kolonia: Western and Central Pacific Fisheries Commission).
Cleasby, I. R., Owen, E., Wilson, L., Wakefield, E. D., O’Connell, P., and Bolton, M. (2020). Identifying important at-sea areas for seabirds using species distribution models and hotspot mapping. Biol. Conserv. 241:108375. doi: 10.1016/j.biocon.2019.108375
Compton, T. J., Bowden, D. A., Roland Pitcher, C., Hewitt, J. E., and Ellis, N. (2013). Biophysical patterns in benthic assemblage composition across contrasting continental margins off New Zealand. J. Biogeogr. 40, 75–89. doi: 10.1111/j.1365-2699.2012.02761.x
Cotton, P. A., Sims, D. W., Fanshawe, S., and Chadwick, M. (2005). The effects of climate variability on zooplankton and basking shark (Cetorhinus maximus) relative abundance off southwest Britain. Fish. Oceanogr. 14, 151–155. doi: 10.1111/j.1365-2419.2005.00331.x
Del Castillo, C. E., Signorini, S. R., Karaköylü, E. M., and Rivero-Calle, S. (2019). Is the Southern Ocean getting greener? Geophys. Res. Lett. 46, 6034–6040. doi: 10.1029/2019GL083163
Dewar, H., Wilson, S. G., Hyde, J. R., Snodgrass, O. E., Leising, A., Lam, C. H., et al. (2018). Basking Shark (Cetorhinus maximus) movements in the Eastern North Pacific determined using satellite telemetry. Front. Mar. Sci. 5:163. doi: 10.3389/fmars.2018.00163
Doherty, P. D., Baxter, J. M., Gell, F. R., Godley, B. J., Graham, R. T., Hall, G., et al. (2017). Long-term satellite tracking reveals variable seasonal migration strategies of basking sharks in the north-east Atlantic. Nat. Sci. Rep. 7:42837. doi: 10.1038/srep42837
Doherty, P. D., Baxter, J. M., Godley, B. J., Graham, R. T., Hall, G., Hall, J., et al. (2019). Seasonal changes in basking shark vertical space use in the north-east Atlantic. Mar. Biol. 166:129. doi: 10.1007/s00227-019-3565-6
Dormann, C. F., Bobrowski, M., Dehling, D. M., Harris, D. J., Hartig, F., Lischke, H., et al. (2018). Biotic interactions in species distribution modelling: 10 questions to guide interpretation and avoid false conclusions. Glob. Ecol. Biogeogr. 27, 1004–1016. doi: 10.1111/geb.12759
Dormann, C. F., Elith, J., Bacher, S., Buchmann, C., Carl, G., Carré, G., et al. (2013). Collinearity: a review of methods to deal with it and a simulation study evaluating their performance. Ecography 36, 27–46. doi: 10.1111/j.1600-0587.2012.07348.x
Elith, J., Graham, C. H., Anderson, R. P., Dudík, M., Ferrier, S., Guisan, A., et al. (2006). Novel methods improve prediction of species’ distributions from occurrence data. Ecography 29, 129–151. doi: 10.1111/j.2006.0906-7590.04596.x
Elith, J., Kearney, M., and Phillips, S. (2010). The art of modelling range-shifting species. Methods Ecol. Evol. 1, 330–342. doi: 10.1111/j.2041-210X.2010.00036.x
Elith, J., Leathwick, J. R., and Hastie, T. (2008). A working guide to boosted regression trees. J. Anim. Ecol. 77, 802–813. doi: 10.1111/j.1365-2656.2008.01390.x
Elith, J., Phillips, S. J., Hastie, T., Dudík, M., Chee, Y. E., and Yates, C. J. (2011). A statistical explanation of MaxEnt for ecologists. Divers. Distribut. 17, 43–57.
Ellis, N., Smith, S. J., and Pitcher, C. R. (2012). Gradient forests: calculating importance gradients on physical predictors. Ecology 93, 156–168. doi: 10.1890/11-0252.1
Fahmi, and White, W. T. (2015). First record of the basking shark Cetorhinus maximus (Lamniformes: Cetorhinidae) in Indonesia. Mar. Biodivers. Rec. 8:e18. doi: 10.1017/S1755267214001365
Fowler, S. L., Cavanagh, R. D., Camhi, M., Burgess, G. H., Cailliet, G. M., Fordham, S. V., et al. (2005). Sharks, Rays and Chimaeras: The Status of Chondrichthyan Fishes. Gland: IUCN.
Francis, M. P. (2017). Review of Commercial Fishery Interactions and Population Information for New Zealand Basking Shark. NIWA Client Report. Kilbirnie: National Institute of Water & Atmospheric Research Ltd.
Francis, M. P., and Duffy, C. (2002). Distribution, seasonal abundance and bycatch of basking sharks (Cetorhinus maximus) in New Zealand, with observations on their winter habitat. Mar. Biol. 140, 831–842. doi: 10.1007/s00227-001-0744-y
Francis, M. P., and Smith, M. H. (2010). Basking shark (Cetorhinus maximus) bycatch in New Zealand Fisheries, 1994–95 to 2007–08. New Zealand Aquatic Environment and Biodiversity Report No. 49. Wellington: Ministry of Fisheries.
Friedman, J., Hastie, T., and Tibshirani, R. (2001). The Elements of Statistical Learning. New York, NY: Springer.
Georgian, S. E., Anderson, O. F., and Rowden, A. A. (2019). Ensemble habitat suitability modeling of vulnerable marine ecosystem indicator taxa to inform deep-sea fisheries management in the South Pacific Ocean. Fish. Res. 211, 256–274. doi: 10.1016/j.fishres.2018.11.020
Gore, M. A., Rowat, D., Hall, J., Gell, F. R., and Ormond, R. F. (2008). Transatlantic migration and deep mid-ocean diving by basking shark. Biol. Lett. 4, 395–398. doi: 10.1098/rsbl.2008.0147
Gregg, W. W., and Conkright, M. E. (2002), Decadal changes in global ocean chlorophyll. Geophys. Res. Lett. 29, 1730, doi: 10.1029/2002GL014689
Hawkes, L. A., Exeter, O., Henderson, S. M., Kerry, C., Kukulya, A., Rudd, J., et al. (2020). Autonomous underwater videography and tracking of basking sharks. Anim. Biotelemet. 8, 1–10. doi: 10.1186/s40317-020-00216-w
Hernández, S., Vögler, R., Bustamante, C., and Lamilla, J. (2010). Review of the occurrence and distribution of the basking shark (Cetorhinus maximus) in Chilean waters. Mar. Biodivers. Rec. 3:E67. doi: 10.1017/s1755267210000540
Hijmans, R. J., Phillips, S., Leathwick, J., and Elith, J. (2017). dismo: Species Distribution Modeling R Package Version 1.1-4.
Hijmans, R. J., and van Etten, J. (2012). raster: Geographic Analysis and Modeling with Raster Data. R Package Version 2.0-12.
Hosmer, D. W. Jr., Lemeshow, S., and Sturdivant, R. X. (2013). Applied Logistic Regression. Hoboken, NJ: John Wiley & Sons.
Hurst, R. J., Ballara, S. L., MacGibbon, D., and Triantafillos, L. (2012). Fishery Characterisation and Standardised CPUE Analyses for Arrow Squid (Nototodarus gouldi and N. sloanii), 1989-90 to 2007-08, and Potential Management Approaches for Southern Fisheries. New Zealand Fisheries Assessment Report No. 47. Wellington: Ministry for Primary Industries.
Johnston, E. M., Mayo, P. A., Mensink, P. J., Savetsky, E., and Houghton, J. D. R. (2019). Serendipitous re-sighting of a basking shark Cetorhinus maximus reveals inter-annual connectivity between American and European coastal hotspots. J. Fish Biol. 95, 1530–1534. doi: 10.1111/jfb.14163
Komac, B., Esteban, P., Trapero, L., and Caritg, R. (2016). Modelization of the current and future habitat suitability of Rhododendron ferrugineum using potential snow accumulation. PLoS One 11:e0147324. doi: 10.1371/journal.pone.0147324
Kuhn, M., Wing, J., Weston, S., Williams, A., Keefer, C., Engelhardt, A., et al. (2020). Package ‘caret’. Vienna: The R Journal.
Large, K., Roberts, J., Francis, M., and Webber, D. N. (2019). Spatial assessment of fisheries risk for New Zealand Sea Lions at the Auckland Islands. New Zealand Aquatic Environment and Biodiversity Report No. 224. Wellington: Ministry for Primary Industries.
Law, C. S., Rickard, G. J., Mikaloff-Fletcher, S. E., Pinkerton, M. H., Behrens, E., Chiswell, S. M., et al. (2018). Climate change projections for the surface ocean around New Zealand. New Zeal. J. Mar. Freshw. Res. 52, 309–335. doi: 10.1080/00288330.2017.1390772
Leathwick, J., Elith, J., Francis, M., Hastie, T., and Taylor, P. (2006). Variation in demersal fish species richness in the oceans surrounding New Zealand: an analysis using boosted regression trees. Mar. Ecol. Prog. Ser. 321, 267–281. doi: 10.3354/meps321267
Lieber, L., Hall, G., Hall, J., Berrow, S., Johnston, E., Gubili, C., et al. (2020). Spatio-temporal genetic tagging of a cosmopolitan planktivorous shark provides insight to gene flow, temporal variation and site-specific re-encounters. Sci. Rep. 10, 1–17. doi: 10.1038/s41598-020-58086-4
Linley, T. D., Stewart, A. L., McMillan, P. J., Clark, M. R., Gerringer, M. E., Drazen, J. C., et al. (2017). Bait attending fishes of the abyssal zone and hadal boundary: community structure, functional groups and species distribution in the Kermadec, New Hebrides and Mariana trenches. Deep Sea Res. Ia Oceanogr. Res. Pap. 121, 38–53. doi: 10.1016/j.dsr.2016.12.009
Lucifora, L. O., Barbini, S. A., Di Giácomo, E. E., Waessle, J. A., and Figueroa, D. E. (2015). Estimating the geographic range of a threatened shark in a data-poor region: Cetorhinus maximus in the South Atlantic Ocean. Curr. Zool. 61, 811–826. doi: 10.1093/czoolo/61.5.811
Lütolf, M., Kienast, F., and Guisan, A. (2006). The ghost of past species occurrence: improving species distribution models for presence-only data. J. Appl. Ecol. 43, 802–815. doi: 10.1111/j.1365-2664.2006.01191.x
Lutz, M., Dunbar, R., and Caldeira, K. (2002). Regional variability in the vertical flux of particulate organic carbon in the ocean interior. Glob. Biogeochem. Cycles 16, 11–11. doi: 10.1029/2000GB001383
Mackay, A. I., Bailleul, F., Carroll, E. L., Andrews-Goff, V., Baker, C. S., Bannister, J., et al. (2020). Satellite derived offshore migratory movements of southern right whales (Eubalaena australis) from Australian and New Zealand wintering grounds. PLoS One 15:e0231577. doi: 10.1371/journal.pone.0231577
Mitchell, J. S., Mackay, K. A., Neil, H. L., Mackay, E. J., Pallentin, A., and Notman, P. (2012). Undersea New Zealand, 1:5,000,000. NIWA Chart, Miscellaneous Series No. 92. Kilbirnie: National Institute of Water & Atmospheric Research Ltd.
Montie, S., Thomsen, M. S., Rack, W. A., and Broady, P. A. (2020). Extreme summer marine heatwaves increase chlorophyll a in the Southern Ocean. Antarctic Sci. 32, 508–509. doi: 10.1017/S0954102020000401
Murphy, R. J., Pinkerton, M. H., Richardson, K. M., Bradford-Grieve, J., and Boyd, P. W. (2001). Phytoplankton distributions around New Zealand derived from SeaWiFS remotely-sensed ocean colour data. New Zeal. J. Mar. Freshw. Res. 35, 343–362. doi: 10.1080/00288330.2001.9517005
Parrott, A. W. (1958). Fishes from the Auckland and Campbell Islands. Domin. Museum Records 3, 109–119.
Petatan-Ramirez, D., Whitehead, D. A., Guerrero-Izquierdo, T., Ojeda-Ruiz, M. A., and Becerril-Garcia, E. E. (2020). Habitat suitability of Rhincodon typus in three localities of the Gulf of California: environmental drivers of seasonal aggregations. J. Fish. Biol. 97, 1177–1186. doi: 10.1111/jfb.14496
Pinkerton, M. (2016). Ocean Colour Satellite Observations of Phytoplankton in the New Zealand EEZ, 1997–2016. Prepared for the Ministry for the Environment. Wellington: NIWA.
Pinkerton, M. H., Décima, M., Kitchener, J. A., Takahashi, K. T., Robinson, K. V., Stewart, R., et al. (2020). Zooplankton in the Southern Ocean from the continuous plankton recorder: distributions and long-term change. Deep Sea Res. Part I Oceanogr. Res. Pap. 162:103303. doi: 10.1016/j.dsr.2020.103303
R Core Team (2020). R: A Language and Environment for Statistical Computing. Vienna: R Foundation for Statistical Computing.
Rayment, W., Dawson, S., and Webster, T. (2015). Breeding status affects fine-scale habitat selection of southern right whales on their wintering grounds. J. Biogeogr. 42, 463–474. doi: 10.1111/jbi.12443
Reynolds, R. W., Rayner, N. A., Smith, T. M., Stokes, D. C., and Wang, W. (2002). An improved in situ and satellite SST analysis for climate. J. Clim. 15, 1609–1625. doi: 10.1175/1520-0442(2002)015<1609:AIISAS>2.0.CO;2
Rigby, C. L., Barreto, R., Carlson, J., Fernando, D., Fordham, S., Francis, M. P., et al. (2019). Cetorhinus maximus (errata version published in 2020) [Online]. The IUCN Red List of Threatened Species 2019: e.T4292A166822294. Available online at: https://dx.doi.org/10.2305/IUCN.UK.2019-3.RLTS.T4292A166822294.en (accessed January 5, 2021).
Robert, K., Jones, D. O., Roberts, J. M., and Huvenne, V. A. (2016). Improving predictive mapping of deep-water habitats: considering multiple model outputs and ensemble techniques. Deep Sea Res. I Oceanogr. Res. Pap. 113, 80–89. doi: 10.1016/j.dsr.2016.04.008
Rowden, A. A., Clark, M. R., and Wright, I. C. (2005). Physical characterisation and a biologically focused classification of “seamounts” in the New Zealand region. New Zeal. J. Mar. Freshw. Res. 39, 1039–1059. doi: 10.1080/00288330.2005.9517374
Sims, D. W. (2008). Sieving a living: a review of the biology, ecology and conservation status of the plankton-feeding basking shark Cetorhinus maximus. Adv. Mar. Biol. 54, 171–220. doi: 10.1016/S0065-2881(08)00003-5
Sims, D. W., and Merrett, D. A. (1997). Determination of zooplankton characteristics in the presence of surface feeding basking sharks Cetorhinus maximus. Mar. Ecol. Prog. Ser. 158, 297–302. doi: 10.3354/meps158297
Sims, D. W., and Reid, P. C. (2002). Congruent trends in long-term zooplankton decline in the north-east Atlantic and basking shark (Cetorhinus maximus) fishery catches off west Ireland. Fish. Oceanogr. 11, 59–63. doi: 10.1046/j.1365-2419.2002.00189.x
Sims, D. W., Southall, E. J., Richardson, A. J., Reid, P. C., and Metcalfe, J. D. (2003). Seasonal movements and behaviour of basking sharks from archival tagging: no evidence of winter hibernation. Mar. Ecol. Prog. Ser. 248, 187–196. doi: 10.3354/meps248187
Sims, D. W., Southall, E. J., Tarling, G. A., and Metcalfe, J. D. (2005). Habitat-specific normal and reverse diel vertical migration in the plankton-feeding basking shark. J. Anim. Ecol. 74, 755–761. doi: 10.1111/j.1365-2656.2005.00971.x
Skomal, G. B., Zeeman, S. I., Chisholm, J. H., Summers, E. L., Walsh, H. J., McMahon, K. W., et al. (2009). Transequatorial migrations by basking sharks in the western Atlantic Ocean. Curr. Biol. 19, 1019–1022. doi: 10.1016/j.cub.2009.04.019
Stephenson, F., Bulmer, R., Leathwick, J., Brough, T., Clark, D., Greenfield, B., et al. (2020a). Development of a New Zealand Seafloor Community Classification (SCC). NIWA report prepared for Department of Conservation (DOC). Hamilton, ON: National Institute of Water & Atmospheric Research.
Stephenson, F., Goetz, K., Sharp, B. R., Mouton, T. L., Beets, F. L., Roberts, J., et al. (2020b). Modelling the spatial distribution of cetaceans in New Zealand waters. Divers. Distribut. 26, 495–516. doi: 10.1111/ddi.13035
Stephenson, F., Leathwick, J. R., Geange, S. W., Bulmer, R. H., Hewitt, J. E., Anderson, O. F., et al. (2018). Using Gradient Forests to summarize patterns in species turnover across large spatial scales and inform conservation planning. Divers. Distribut. 24, 1641–1656. doi: 10.1111/ddi.12787
Weber, M. M., Stevens, R. D., Diniz-Filho, J. A. F., and Grelle, C. E. V. (2017). Is there a correlation between abundance and environmental suitability derived from ecological niche modelling? A meta-analysis. Ecography 40, 817–828. doi: 10.5061/dryad.g2fd2
Weigmann, S. (2016). Annotated checklist of the living sharks, batoids and chimaeras (Chondrichthyes) of the world, with a focus on biogeographical diversity. J. Fish Biol. 88, 837–1037. doi: 10.1111/jfb.12874
Werdell, P. J. (2019). Inherent Optical Properties (IOPs) Algaorithm Theoretical Basis Document ID: EUM/RSP/REP/20/1160644. EUMETSAT.
Westgate, A. J., Koopman, H. N., Siders, Z. A., Wong, S. N. P., and Ronconi, R. A. (2014). Population density and abundance of basking sharks Cetorhinus maximus in the lower Bay of Fundy, Canada. Endang. Spec. Res. 23, 177–185. doi: 10.3354/esr00567
Wetherbee, B. M. (2000). Assemblage of deep-sea sharks on Chatham Rise, New Zealand. Fish. Bull. 98, 189–198.
Wright, D., Pendleton, M., Boulware, J., Walbridge, S., Gerlt, B., Eslinger, D., et al. (2012). ArcGIS Benthic Terrain Modeler (BTM), v. 3.0. Redlands, CA: Environmental Systems Research Institute.
Keywords: New Zealand, species distribution models, boosted regression tree models, threatened species, elasmobranch
Citation: Finucci B, Duffy CAJ, Brough T, Francis MP, Milardi M, Pinkerton MH, Petersen G and Stephenson F (2021) Drivers of Spatial Distributions of Basking Shark (Cetorhinus maximus) in the Southwest Pacific. Front. Mar. Sci. 8:665337. doi: 10.3389/fmars.2021.665337
Received: 07 February 2021; Accepted: 29 March 2021;
Published: 26 April 2021.
Edited by:
David W. Sims, Marine Biological Association of the United Kingdom, United KingdomReviewed by:
Alexandra McInturf, University of California, Davis, United StatesCopyright © 2021 Finucci, Duffy, Brough, Francis, Milardi, Pinkerton, Petersen and Stephenson. This is an open-access article distributed under the terms of the Creative Commons Attribution License (CC BY). The use, distribution or reproduction in other forums is permitted, provided the original author(s) and the copyright owner(s) are credited and that the original publication in this journal is cited, in accordance with accepted academic practice. No use, distribution or reproduction is permitted which does not comply with these terms.
*Correspondence: Brittany Finucci, YnJpdC5maW51Y2NpQG5pd2EuY28ubno=
Disclaimer: All claims expressed in this article are solely those of the authors and do not necessarily represent those of their affiliated organizations, or those of the publisher, the editors and the reviewers. Any product that may be evaluated in this article or claim that may be made by its manufacturer is not guaranteed or endorsed by the publisher.
Research integrity at Frontiers
Learn more about the work of our research integrity team to safeguard the quality of each article we publish.