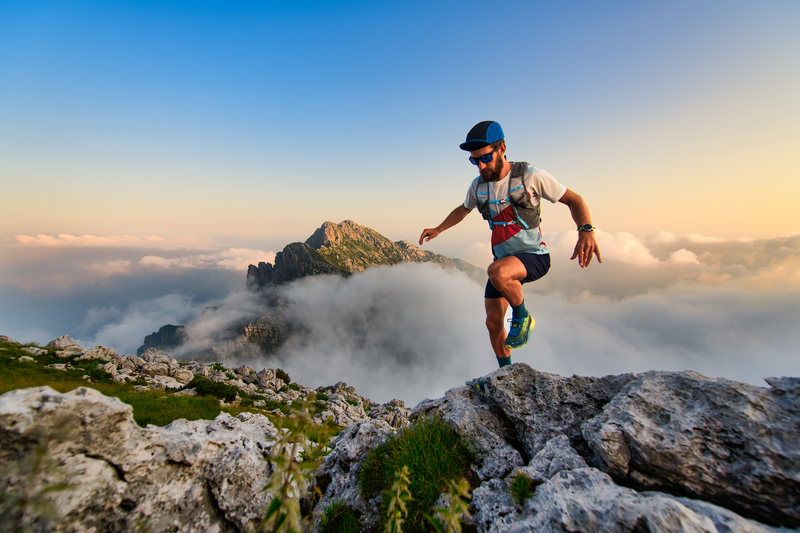
94% of researchers rate our articles as excellent or good
Learn more about the work of our research integrity team to safeguard the quality of each article we publish.
Find out more
ORIGINAL RESEARCH article
Front. Mar. Sci. , 20 July 2021
Sec. Coral Reef Research
Volume 8 - 2021 | https://doi.org/10.3389/fmars.2021.658708
The coral microbiome is one of the most complex microbial biospheres. However, the ecological processes shaping coral microbiome community assembly are not well understood. Here, we investigated the abundance, diversity, and community assembly mechanisms of coral-associated microbes from a highly diverse coral metacommunity in the South China Sea. Compared to seawater, the coral microbial metacommunity were defined by highly variable bacterial abundances among individual coral samples, high species evenness but not high species richness, high β-diversity, and a small core microbiome. We used variation partitioning analysis, neutral community model, and null model to disentangle the influences of different ecological processes in coral microbiome assembly. Measured physico-chemical parameters of the surrounding seawater and the spatial factor together explained very little of the variation in coral microbiome composition. Neutral processes only explained a minor component of the variation of coral microbial communities, suggesting a non-stochastic community assembly. Homogeneous and heterogeneous selection, but not dispersal, contributed greatly to the assembly of the coral microbiome. Such selection could be attributed to the within-host environments rather than the local environments. Our results demonstrated that dispersal limitation and host filtering contribute significantly to the assembly of discrete coral microbial regimes and expand the metacommunity diversity.
Coral reefs have some of the highest biodiversity and productivity among marine ecosystems, and offer valuable ecosystem services to other organisms, as well as to the human society (Knowlton, 2001; Costanza et al., 2014). The complex community of microorganisms that form an integral part of the coral holobiont, including dinoflagellates, fungi, bacteria, archaea and viruses, contribute to the persistence and resilience of corals (Rosenberg et al., 2007; Wegley et al., 2007). The collection of all microbes that inhabit in an environment or in a host are termed as an microbiome. Specifically, the combined community of bacteria and archaea associated with coral are collectively termed as the coral prokaryotic microbiome (microbiome for short hereafter) (Ainsworth et al., 2015). Microorganisms are fundamental drivers of biogeochemical cycling in the coral reef ecosystem and contribute significantly to both host health and ecosystem homeostasis (Silveira et al., 2017; Webster and Reusch, 2017).
The abundance, diversity, distribution, and function of coral-associated microbes have been extensively investigated, as well as their correlations with environmental variables (Hoegh-Guldberg et al., 2007; Ziegler et al., 2017), and their response to global changes such as global warming and ocean acidification (Webster et al., 2016; Zhou et al., 2017). The community structure of the coral microbiome can change with coral host species, coral life stage, host health state, and environmental variables (Sweet and Bulling, 2017; Pollock et al., 2018). As a host-associated microbiome, the variation across individual corals can be high, similar to the gut microbiomes of humans (Turnbaugh and Gordon, 2009). Hosts and their microbiomes are ecological systems and the multispecies assemblages are structured by multiple ecological processes such as ecological drift, dispersal, and the interaction among microbial members and between the microbes and their hosts (Miller et al., 2018). However, we still lack information on the relative contributions of species richness and evenness to the coral microbiome diversity. Moreover, the mechanisms of community assembly governing the structure of coral microbiomes remain unclear, especially for the relative contributions of deterministic and stochastic processes such as dispersal, selection, and ecological drift.
Although it has been demonstrated that microbial biodiversity is generally high in a number of environments, how and why high diversity are maintained and generated is generally unknown (Zhou and Ning, 2017). Deterministic and stochastic processes simultaneously influence the assembly of microbial communities (Chase, 2010; Ofiteru et al., 2010; Chase and Myers, 2011; Stegen et al., 2016). Based on niche theory, deterministic processes mainly consider that microbial communities are shaped by the abiotic environmental factors (such as temperature, salinity, pH, and light availability) and biotic factors (such as competition and predation) (Vellend, 2010). These factors can determine the occurrence and relative abundance of species. In contrast, stochastic processes (such as immigration, emigration, birth, death, and diversification) are also believed to play important roles in structuring the microbial community (Chen et al., 2017; Zhou and Ning, 2017). A central debate lies on relative importance of these processes in controlling community structure, succession, and biogeography (Martiny et al., 2006; Zhou et al., 2013, 2014; Vellend et al., 2014; Stegen et al., 2015).
Recently, the metacommunity concept (Leibold et al., 2004) has been incorporated into host-associated microbiome studies (Miller et al., 2018), as well as into the coral reef microbiome studies (Hernandez-Agreda et al., 2017; Cleary et al., 2019). The metacommunity theory highlights the scales of interaction beyond the level of the individual host (Miller et al., 2018). Therefore, a regional study of the assembly of coral microbiomes would provide new insight into coral microbial ecology. Corals are diverse and widely, but patchily, distributed in the sea, forming discrete regional metacommunity. In contrast to the host-associated coral microbial metacommunity, the free-living reef seawater microbial metacommunity appear to be more broadly connecting and homogenizing. Therefore, comparing the two types of microbial metacommunity, with respect to diversity level, composition and ecological processes that maintain the community structure, would help to explore the coral microbial community assembly mechanisms.
The South China Sea abuts the western border of the Coral Triangle, and harbors an extraordinary diversity of reef corals (Huang et al., 2015). In this study, we simultaneously investigated the microbial metacommunity of corals as well as the surrounding reef seawaters using high-throughput 16S rRNA gene amplicon sequencing. By comparing their diversity level, spatial and environmental influences on community structure, and the stochastic and deterministic processes governing the community, we attempt to shed light on the community assembly mechanisms of coral microbiomes and the relatedness between the ecological processes and the diversity pattern (see a conceptual framework shown in Figure 1). The sampled coral colonies (n = 57) represented 15 known coral families and 30 genera, forming a regional coral metacommunity. Multivariate analysis, variation partitioning analysis, neutral community model, and null model were used to answer the following questions: (1) What is the diversity level of the coral microbiome metacommunity within the offshore South China Sea? (2) What are the relative contributions of deterministic and stochastic processes, such as selection, dispersal, and drift, for the coral microbial metacommunity?
Figure 1. Conceptual framework of this study. The figure presents the research contents and goals of this study. Coral microbiome and seawater microbiome were simutaneously investigated and their diversity levels, community structures, influencing factors and community assembly mechanisms were compared. The major goal is to illustrate the roles of stochastic and deterministic processes involving in coral microbiome assembly. Larger circles indicate seawater microbial metacommunity and coral microbial metacommunity, smaller circles indicate seawater microbial community at each of the sampling locations or coral microbial community of each colony, and colared filled dots indicate microbes.
Coral and surrounding seawater samples were collected at 15 locations within the South China Sea, in August and September 2016 (Figure 2; Supplementary File 1). Small pieces (∼ 1 cm × 1 cm) of coral colonies with both tissue and skeleton were collected by SCUBA diving, immediately placed in plastic bags, and stored at −20∘C until analysis. Corals were taxonomically classified according to Corals of the World1. For seawater sampling, 1–2 L water was filtered on board through polycarbonate membrane with a diameter of 47 mm and a pore-size of 0.2 μm (Millipore, Billerica, MA, United States). The filter was frozen in liquid nitrogen on board and stored at −80∘C until DNA extraction. Temperature, depth that samples were collected, salinity, pH, and dissolved oxygen (DO) were measured in situ using a YSI profiler (YSI 6920, Yellow Spring Instruments, United States). A total of 72 samples, 15 seawater and 57 coral, were analyzed in this study.
Figure 2. Sampling sites of the coral reefs in South China Sea. Detailed information of the sampling sites are shown in the Supplementary Data.
Water samples for measuring nutrients (nitrate (NO3-N), nitrite (NO2-N), ammonium (NH4-N), phosphate (PO4-P), silicate (SiO3-Si)) were filtered through a polycarbonate membrane with diameter of 47 mm and pore-size of 0.45 μm (Millipore, Billerica, MA, United States) and then the water samples were stored at −20∘C. The nutrients were measured as previously described (Ling et al., 2014).
Genomic DNA from coral and water samples was extracted using the PowerSoil DNA Isolation Kit (QIAGEN, Hilden, Germany) by following the manufacturer’s protocol. A small piece (∼0.5 g) of coral colony with both tissue and skeleton was crushed by hammer and used to extract DNA. The filters for water samples were cut into pieces to extract DNA. The V4 region of the prokaryotic 16S rRNA gene was amplified using a barcoded universal primer pair 515F (5′-GTGCCAGCMGCCGCGGTAA-3′) and 806R (5′-GGACTACHVGGGTWTCTAAT-3′) (Caporaso et al., 2011). This primer pair targets both the bacterial and archaeal communities. The 50 μL PCR reaction contained 25 μL Phusion High-Fidelity PCR Master Mix (NEB, Ipswich, MA, United States), 1 μL of each primer (200 nM final concentration), 2 μL template DNA and 21 μL H2O. PCR cycling conditions were as follows: 95°C for 3 min, 30 cycles of 95°C for 45 s, 56°C for 45 s, 72°C for 45 s and final extension at 72°C for 10 min. The purified PCR products were then mixed equally and sequenced on the Illumina HiSeq 2500 platform at BGI (Shenzhen, China), generating 2 × 250 bp paired-end reads. The sequences generated in this study were deposited into the NCBI Short Reads Archive (SRA) database under accession number PRJNA629477.
Bacterial abundance of all samples were measured by quantifying the copy number of 16S rRNA gene using real-time PCR (qPCR). Primers EUB338 (5′-ACTCCTACGGGAGGCAGCAG-3′) and EUB518 (5′-ATTACCGCGGCTGCTGG-3′) (Fierer et al., 2005) were used in this study. To prepare qPCR standards, 16S rRNA sequences were amplified using the primers EUB338 and EUB518, the PCR products were then cloned using the TaKaRa pMD18-T vector kit (TaKaRa, Shiga, Japan). Plasmid DNA was extracted from positive clones and used as the template to amplify a fragment with the universal primers M13-47/RV-M. The PCR products were purified and ten-fold serially diluted to serve as qPCR standards. The qPCR reactions were performed in triplicate using a CFX96 Real-Time System (Bio-Rad Inc., United States). The thermal conditions were: initial denaturation at 95°C for 3 min, followed by 45 cycles of 95°C for 15 s, 55°C for 30 s and 72°C for 30 s. Each qPCR reaction (25 μL) consisted of 12.5 μL of TB GreenTM Premix Ex TaqTM II (Tli RNaseH Plus) RR820A (TaKaRa, Shiga, Japan), 2 μL of each primer, 0.5 μL DNA template and 8 μL ddH2O. The assay efficiency of the bacterial 16S rRNA genes was 98%, with the standard curve regression coefficient R2 = 0.997. As the abundance data followed the normal distribution, one-way ANOVA was used to test whether the 16S rRNA gene abundances among different coral families (those present in more than five samples) were overall significantly different.
The 16S rRNA gene sequences were processed by using LotuS (Hildebrand et al., 2014) and QIIME (Caporaso et al., 2010). First, we performed read quality control using LotuS. Briefly, quality control criteria for raw reads were: (1) average sequence quality >27; (2) sequence length >170 bp; (3) no ambiguous bases; (4) no mismatches within barcode and primer; (5) homopolymer <8 bp. Reads passed the quality control were then chimera-checked and clustered into Operational Taxonomic Units (OTUs) under a 97% identity cut-off by using UPARSE (Edgar, 2013) implemented in QIIME. Subsequently, taxonomic assignment for each OTU was carried out by using the RDP classifier implemented in QIIME against the SILVA database v132 as reference (Quast et al., 2013) with a bootstrap cut-off of 80%. All the samples were randomly subsampled to the smallest library size (42,110 reads) in order to standardize the uneven sequencing effort.
α-diversity indices, including observed OTU richness, Chao1, Shannon index, Pielou’s J index, Simpson index, and PD were calculated for each sample using the vegan 2.5-6 package (Oksanen et al., 2019) implemented in the R software (v3.5.12). These metrics indicate different aspects of diversity. Observed OTU richness and Chao1 indicate species richness, Pielou’s J indicates species evenness, and Shannon index and Simpson index indicate overall diversity level. PD indicates phylogenetic diversity, which has been defined as the minimum total length of all the phylogenetic branches of a given set of taxa. As the data did not follow normal distribution, Kruskal-Wallis test was conducted to determine whether the α-diversity indices and β-diversity indicator (pairwise Bray-Curtis distance, which is a distance normalized to 0–1, where 0 indicates the two samples share all the species and 1 indicates the two samples do not share any species) of microbial community differed significantly between coral and seawater samples. In order to eliminate the influence of uneven sampling efforts, all the coral samples (n = 57) as well as a fraction of randomly selected 15 coral samples (equal to seawater sample number) were both subjected to the Bray-Curtis distance calculating. The random selection was performed for 100 replicates. Pearson correlation analysis was used to assess the relationship between α-diversity indices and environmental variables. The 16S rRNA sequence abundance in coral samples was included in the Pearson correlation analysis to assess the relationship between diversity and abundance of coral microbiomes.
Non-metric multidimensional scaling analysis (NMDS) was carried out to demonstrate the β-diversity patterns of coral and seawater microbiomes with Bray-Curtis similarity matrices using the vegan package in R. Permutational multivariate analysis of variance (PERMANOVA) was used to evaluate the significance of grouping among samples with 999 iterations. Sample type (coral or seawater) was considered as a factor and tested in PERMANOVA. Coral family was also considered as a factor for coral samples and tested.
To explore the potential controlling factors for the prokaryotic community composition, we employed Mantel tests implemented in the R package vegan to assess the correlations between the community similarity and environmental factors.
Core OTUs in coral microbiomes have been defined using different percentage cut-offs, ranging from 30 to 100% (Hernandez-Agreda et al., 2017). In this study, we respectively identified the core OTUs in seawater and coral samples according to the criteria that only OTUs present in more than 80% of samples were considered as core OTUs (Hernandez-Agreda et al., 2016). The proportions of core OTUs, as well as proportions of reads in core OTUs, for individual samples were calculated, and Kruskal-Wallis test was used to assess the significance of difference between coral and water samples. All the coral samples (n = 57) as well as a fraction of randomly selected 15 coral samples (equal to seawater sample number) were both subjected to the core OTU analysis.
A heatmap was used to present the distribution pattern of the most dominant 36 genera (each occupied at least 0.5% of total reads of the whole dataset) among coral and seawater samples. The heatmap was generated using the ComplexHeatmap v1.20.0 package (Gu et al., 2016) implemented in the R software. Genera and samples were, respectively, clustered based on z-score transformed relative abundances of 16S rRNA sequences grouped into those genera.
We used variation partitioning analysis (VPA) to quantify the relative influences of environmental and spatial factors in shaping the microbial community composition (Peres-Neto et al., 2006). To explore the spatial predictor of prokaryotic community composition, the geographical distance matrix as Principal Coordinates of Neighbourhood Matrix (PCNM) was calculated between each pair of sampling sites based on their longitude and latitude coordinates (Hu et al., 2018). The explained variance (R2) of the VPA models was reported based on 999 permutation tests. Environmental factors involved in VPA included temperature, salinity, pH, dissolved oxygen, and multi-nutrient concentrations (NO3-N, NO2-N, NH4-N, PO4-P, SiO3-Si).
Neutral community model (NCM) was used to evaluate the contributions of the stochastic processes to the microbial community assembly, by predicting the relationship between the frequency of the OTUs and their relative abundance (Sloan et al., 2006; Hu et al., 2019). Like the neutral theory, the model also assumes the equivalent of birth, death and dispersal rates of species and ignores the difference between species and their response to the environmental factors, thus assuming species are “neutral” and their ecological processes are random (Burns et al., 2016). In general, more abundant taxa are expected to be more widespread, whereas rare taxa will be lost in particular communities because of ecological drift. In the model, the parameter m is the estimated immigration rate and the parameter R2 indicates the overall fit to the model (Sloan et al., 2006). Here, the immigration rate m refers to the probability that random loss of an individual in a community is replaced via dispersal from the metacommunity, rather than reproduction within the local community. Therefore, the m can be seen as the representation of dispersal limitation (Burns et al., 2016). The 95% confidence interval of the model was calculated based on 1000 bootstrap replicates. All the coral samples (n = 57) as well as a fraction of randomly selected 15 coral samples (equal to seawater sample number) were both subjected to NCM analysis. R code used for the model was according to Burns et al. (2016) and provided in the Supplementary File 2.
Null model analysis was carried out using the framework described by Stegen et al. (2013) and Stegen et al. (2015) to quantify the relative importance of the four ecological processes: selection, dispersal, diversification, and drift. The model assumes that phylogenetically related species share more similar ecological niches than phylogenetically distant species. The analysis was performed using the OTU tables from coral and seawater samples, and the null model expectation was generated with 1000 randomizations. Interpretation of the modeling results followed the description recently reviewed (Zhou and Ning, 2017). Briefly, null model-based phylogenetic and taxonomic β-diversity metrics, indicated by β-nearest taxon index (βNTI) and Bray-Curtis-based Raup-Crick (RCBray), were calculated (Stegen et al., 2013). To quantify the phylogenetic turnover between communities, β-mean-nearest taxa distance (βMNTD) was calculated which can quantify the phylogenetic distance between each species in a community and its closest relative in another community using the R function “comdistnt” in the package picante (v1.8.2). Then the βMNTD was recalculated for 1000 times after the species was randomly shuffled across the tips of the phylogeny. The βNTI was used to express the difference between observed βMNTD and the mean of the null distribution in s.d. units. |βNTI| > 2 stands for the dominance of selection, with βNTI > 2 and < −2 indicating the significant influence of heterogeneous selection and homogeneous selection, respectively. RCBray can further distinguish the processes of non-selection (|βNTI| < 2). Dispersal limitation is quantified as the fraction of pairwise comparisons with |βNTI| < 2 and RCBray > 0.95. The importance of homogenizing dispersal was quantified by the |βNTI| < 2 and RCBray < −0.95. |βNTI| < 2 and |RCBray| < 0.95 indicates the influence of “undominated” processes which mostly consist of weak selection, weak dispersal, diversification, and/or drift (Stegen et al., 2015; Zhou and Ning, 2017; Tripathi et al., 2018). The analysis was conducted in the R software according to Chase et al. (2011) and (Stegen et al., 2013). All the coral samples (n = 57) as well as a fraction of randomly selected 15 coral samples (equal to seawater sample number) were both subjected to Null model analysis. R code used for the model was provided in Supplementary File 2.
Relevant statistical analyses and plots were run in R statistical software (v3.5.1) by using the packages ape v5.3 (Paradis et al., 2004), ComplexHeatmap v1.20.0 (Gu et al., 2016), ggplot2 v3.2.1 (Ginestet, 2011), phyloseq v1.30.0 (McMurdie and Holmes, 2012), and vegan v2.5-6 (Oksanen et al., 2019). The custom R codes used here were shown in the Supplementary File 2.
Coral samples (n = 57) and ambient water samples (n = 15) were collected at 15 locations in the South China Sea (Figure 2; Supplementary File 1). One seawater sample was collected at each sampling site. Physico-chemical parameters of the water bodies, including temperature, salinity, pH, dissolved oxygen and multi-nutrient concentrations (NO3-N, NO2-N, NH4-N, PO4-P, SiO3-Si), were measured and the data are listed in Supplementary File 1. Overall, the sampling areas represented shallow (2–12 m), warm (29–30∘C), and oligotrophic reef environments that exhibited a high level of homogeneity with respect to environmental conditions.
The 57 corals samples were collected from 55 stony Scleractinea and two soft Alcyonacea coral colonies. These samples represented highly diverse coral taxa, including 15 coral families and 30 genera. Six of the coral families contained more than five samples: Acroporidae, Agariciidae, Euphylliidae, Fungiidae, Merulinidae, and Mussidae. Results of coral taxonomic classification for each sample are listed in Supplementary File 1.
We used real-time PCR to quantify the bacteria in both the coral tissue and seawater samples. The 16S rRNA gene copies were highly variable among coral samples, ranging from 4.3 × 106 to 1.0 × 1010 copies per gram of wet coral tissue (Figure 3A). In contrast, bacterial abundances in the seawater were much more consistent, ranging from 1.3 × 105 to 6.3 × 105 copies per ml (Figure 3A). We compared the relative bacterial abundance among the six coral families that were present in more than five samples. Fungiidae corals had the highest bacterial abundance, while Acroporidae corals had the lowest (Figure 3B). The coral family-specific bacterial abundance differed significantly among the six families (one-way ANOVA, P < 0.001). It is worth noting that the variation within a coral family was less than the variation across families, suggesting a host-related pattern of bacterial abundance distribution.
Figure 3. Bacterial 16S rRNA gene abundance in coral samples and surrounding seawater. (A) Box plot of coral and seawater samples. (B) Box plot of coral samples grouped by coral family. One-way ANOVA test shows the signifcantly different 16S rRNA gene abundances among coral families present in more than five samples.
Microbial communities of the coral holobiont and surrounding seawater were analyzed through 16S rRNA gene amplicon sequencing using the Illumina Miseq platform. In total, 6,387,026 clean reads were obtained from coral samples (n = 57, mean = 112,053) and 2,144,534 from water samples (n = 15, mean = 142,969), which together were clustered into 29,869 operational taxonomic units (OTUs) based on a cutoff of 97% sequence similarity. To assess the α- and β-diversity, all the samples were randomly subsampled to 42,110 reads to standardize the uneven sequencing effort.
Significant differences in α- and β-diversity were evident between the coral microbiome and the surrounding seawater microbiome. For α-diversity, although the coral and seawater microbial communities had similar levels of species richness (Chao1 Index, Figure 4A), coral samples had significantly higher levels of evenness (Pielou’s J Index, Kruskal-Wallis test P < 0.01, Figure 4B), therefore leading to higher levels of overall diversity (Shannon Index, Kruskal-Wallis test P < 0.01, Figure 4C). Variations of both species richness and species evenness across the coral samples were larger than those of seawater samples. Correlation analysis showed that only PO4-P was related to the variation of α-diversity among coral samples, while all other factors were not related to the α-diversity pattern of coral or seawater communities (Supplementary Figure 1).
Figure 4. α-diversity and β-diversity of seawater and coral-associated microbiomes. (A) Species richness represented by the Chao1 index. (B) Species evenness represented by the Pielou’s index. (C) Overall diversity represented by the Shannon index. (D) β-diversity represented by Bray-Curtis distance. Kruskal-Wallis test showed significant difference between coral and seawater samples on the α-diversity indices Pielou’s J (B) and Shannon (C), and the β-diversity indicator Bray-Curtis distance (D). Significance code: P < 0.01, ***.
Our data also demonstrated that the β-diversity among coral samples were significantly higher than that among water samples (Bray-Curtis distance, Kruskal-Wallis test P < 0.01, Figure 4D). This result indicated strong heterogeneity in coral microbial assemblage composition. In order to eliminate the effects of distinct sample numbers between coral (57 samples) and seawater (15 samples) subsets, we also randomly selected 15 coral samples for 100 times and compared the β-diversity of them to that of the 15 water samples. The result (Supplementary Figure 2) was highly similar to the comparison between the full 57 coral samples and 15 seawater samples (Figure 4D).
Coral and seawater microbial communities shared 8,614 OTUs, which constituted 31.19 and 79.27% of the total OTUs of coral samples and seawater samples, respectively (Figure 5A). In general, these shared OTUs represented abundant microbial taxa that accounted for 77.96 and 96.78% of total reads from all coral samples and all seawater samples, respectively (Figure 5B). To assess the core microbiome, 131 and 534 core OTUs were identified for coral and seawater samples, respectively (Figure 5C). These core OTUs made up 0.47 and 4.92% of total OTUs, accounting for 44.81 and 93.98% of total reads of coral and seawater samples, respectively (Figure 5D). Meanwhile, core OTUs made up 1.53–23.38% of OTUs (Figure 5E) and accounted for 1.86–89.91% of reads in individual coral samples (Figure 5F). For seawater samples, the percentages of the core OTUs was 9.71–37.38% in individual samples (Figure 5E), which accounted for 82.18–98.40% of the reads of each samples (Figure 5F). Such differences in contributions of core OTUs to individual samples were significant between coral and seawater communities (Kruskal-Wallis test P < 0.01, Figures 5E,F). Comparison between randomly selected 15 coral samples and the 15 seawater samples resulted in similar patterns (Supplementary Figure 3).
Figure 5. Shared OTUs and shared core OTUs between coral and seawater microbiomes. Shared OTUs (A), reads (B), core OTUs (C), and core reads (D) between coral and seawater microbiomes. Percentages of core OTUs (E) and core reads (F) in coral and seawater samples, respectively. Kruskal-Wallis test showed significant difference between coral and seawater samples in the proportions of core OTUs (E) and core reads (F). Significance code: P < 0.01, ***.
The community compositions of coral and seawater samples exhibited distinct patterns as demonstrated by the heatmap showing the relative abundances of 36 most abundant genera (Figure 6). Genera were grouped into clusters based on their relative abundances among samples. A few genera were present in both coral and seawater samples (Clusters 2 and 3, Figure 6), while other genera were enriched in either corals (Cluster 4) or in seawater (Cluster 1). Typical planktonic bacterial taxa, such as Prochlorococcus and Synechococcus, SAR11 clade of α-Proteobacteria, and OM60 clade of γ-Proteobacteria, were enriched in the seawater community. Taxa related to sponge symbionts (e.g., AqS1 and PAUC26f) and taxa within the Planctomycetes (e.g., Bythopirellula, Blastopirellula, Rhodopirellula, and the Pir4 lineage) were enriched in coral samples. The common taxa shared by corals and seawater were largely within γ-Proteobacteria, such as Vibrio, Alteromonas, Pseudomonas, and Pseudoalteromonas.
Figure 6. Heatmap showing the composition of the most abundant microbial genera in coral and seawater samples. Dendrograms show the clustering of samples and clustering of microbial genera, based on the relative abundances of the genera.
Non-metric multidimensional scaling analysis ordination showed that samples from corals and seawater formed distinct clusters in the ordination space (PERMANOVA P = 0.001, R2 = 0.179, Figure 7A). Additionally, NMDS ordination of the six most common families displayed a coral family-related pattern (PERMANOVA P = 0.001, R2 = 0.3, Figure 7B). In particular, corals in the family Fungiidae appeared to harbor significantly different microbial communities from the other families.
Figure 7. NMDS analyses based on Bray-Curtis similarity matrices that were calculated from relative abundances of 16S rRNA sequences clustered into OTUs. (A) NMDS for all the samples, and (B) NMDS for coral samples with at least five samples within a coral family. PERMANOVA test results were shown to evaluate the significance of grouping among samples. Sample type (coral or seawater) (A) and coral family (B) were considered as factors and tested.
Variation portioning analysis (VPA) was used to assess the relative importance of environmental and spatial factors in shaping coral and seawater microbial community composition. The combined explanatory power of spatial and environmental factors for the seawater microbiomes (adjusted R2, 35.5%) was higher than for the coral microbiomes (6.6%). This striking result indicated that a large amount of the variance (64.5% for seawater samples and 93.4% for coral samples) remained unexplained by the environmental or spatial variables. We used the Mantel test to assess the correlation between microbiome composition and environmental factors. Salinity, pH, and nitrate concentration of the seawater could explain the variation of coral microbiome composition, while only pH could explain that of seawater (Supplementary Figure 4). However, the Mantel correlation coefficient ρ was generally < 0.2, indicating a low explanatory power.
The neutral community model (NCM) was used to test the presence of stochasticity in community assembly (Figures 8A–C). The degree of model fitting for the seawater samples (R2 = 0.667) was higher than that for all coral samples (R2 = 0.428), as well as higher than that for a fraction of randomly picked 15 coral samples (R2 = 0.204 ± 0.099). This result indicated that stochastic processes play a less important role in shaping the prokaryotic community composition of the coral holobiont. The rate of immigration for seawater samples (m = 0.4) was much higher than all coral samples (m = 0.031) or 15 randomly picked coral samples (m = 0.049), indicating that coral microbial communities were highly limited by dispersal.
Figure 8. Processes of community assembly in coral and seawater microbial communities using NCM (A–C) and null model (D) analyses. In NCM (A–C), the solid blue lines indicate the best fit to the NCM, and the dashed blue lines represent 95% confidence intervals around the model prediction. m indicates the immigration rate of the prokaryotic taxa, and R2 indicates the fit to this model. All coral samples (n = 57) and a fraction of randomly selected coral samples (n = 15, equal to the number of seawater samples) were both subjected to NCM and null model analyses. The random selection was performed for 100 replicates. Result of one representative run of the 100 replicates was shown in panel (B). In panel (D), error bar shows the standard deviation among 100 replicates.
To further assess the importance of different community assembly processes (mainly selection and dispersal) in shaping microbiome structure, we also employed the newly developed null-model-based framework based on the βNTI and RCBray metrics. Among the five components of the framework shown in Figure 8D, “heterogeneous selection” and “homogeneous selection” are considered to be deterministic, “undominated” mainly consists of stochastic processes, while dispersal (“dispersal limitation” and “homogenizing dispersal”) can be deterministic or stochastic. Our results showed that the relative contributions of individual components were highly different between coral and seawater metacommunities (Figure 8D). For the whole coral metacommunity (sample number = 57), the proportion of dispersal limitation was the highest (33.15%), followed by the homogeneous selection (26.25%), heterogeneous selection (18.98%), and homogenizing dispersal (2.51%). In contrast, for the seawater metacommunity, dispersal limitation and heterogeneous selection occupied very low proportions (5.71 and 0.95%, respectively) while homogenizing dispersal (34.29%) and homogeneous selection (31.43%) occupied high proportions. The “undominated” fractions were 19.11% for coral samples and 27.62% for water samples. Modeling based on all 57 coral samples or on 15 randomly selected samples generated similar results (Figure 8D).
First, our results demonstrated that while the coral microbiome had a similar level of species richness compared to the surrounding seawater, it possessed a significantly higher level of species evenness, indicating that coral-associated microbial taxa are distributed more evenly than the “free-living” seawater taxa. However, different results in comparison between coral and seawater microbial diversity have been previously reported from the South China Sea. For instance, Chen et al. (2011) and Li et al. (2013) found that Shannon and Simpson indices were not significantly different between coral and seawater samples. These two studies sampled at a single location and analyzed a limited number of samples. In contrast, another study (Cai et al., 2018) that analyzed greater number of coral and seawater samples from a larger geographic area in the South China Sea presented an α-diversity level similar to that seen in the current study. The water environment conditions likely did not correlate with the diversity level of the coral microbiome, neither the bacterial abundance of coral colony, although the bacterial abundance appeared to correlate with the coral family. Further study would be necessary to explore the factors that influence the diversity and abundance of coral-associated bacteria, such as the within-coral trophic mode.
Second, the β-diversity of the coral microbial metacommunity was significantly higher than the seawater metacommunity. Throughout our sampling locations the seawater metacommunity exhibited a highly redundant composition, while little commonality was evident across the coral samples. Considering the coral species sampled in this study only represent a small fraction of those present at these sites, our observations suggest that a tremendous diversity of coral-associated microbiomes could exist. Previous studies found that over 60% of the OTUs identified in coral microbiomes are present in less than 10% of individual corals within a same species (Ainsworth et al., 2015; Hernandez-Agreda et al., 2016), supporting our finding that there is high variability in community composition among corals. High β-diversity has also been observed in the sponge metacommunity (Thomas et al., 2016), suggesting a common feature for host-associated microbiome in the sea.
Third, we observed that coral microbiome compositions differed not only from seawater but also between different coral host families, suggesting that host type is an important factor structuring the coral microbiomes. This is in agreement with a previous study, showing that host type, rather than location, drove the coral microbiome assemblage in the South China Sea (Cai et al., 2018). Recently, host traits such as coral colony size or coral phylogeny were also found to influence the microbiome diversity (Pollock et al., 2018). Our data also provides support to the widely observed correlations between host phylogenetic relationship and microbiome composition, a universal pattern known as phylosymbiosis that has previously been observed in the microbiomes of marine sponges (Thomas et al., 2016), plant roots (Yeoh et al., 2017), insects (Brooks et al., 2016), and mammal guts (Ley et al., 2008).
The core microbiome is composed of a suite of members which are broadly distributed across microbial communities from a particular type of habitat (Turnbaugh et al., 2009; Zaura et al., 2009; Shade and Handelsman, 2012). We observed that the core microbiome only accounted for a small fraction of individual coral samples. This is in agreement with the high β-diversity estimated from the coral microbial communities. A small core fraction, or the lack of congruence on microbial diversity, is likely a common feature across coral microbiomes. For example, Ainsworth et al. (2015) found that only 0.09% of all bacterial phylotypes were present in 90% of the sampled coral hosts of Acropora granulosa (Ainsworth et al., 2015). Moreover, they only detected seven bacterial taxa that were universal across three coral species, Acropora granulosa, Leptoseris spp. and Montipora capitata. In another study, only 27 universal microbial taxa were found across five locations and four coral species in the South China Sea (Cai et al., 2018). A survey on the microbial diversity of corals from a single species, Pachyseris speciosa, also revealed a very small ubiquitous core microbiome, but a highly variable community (Hernandez-Agreda et al., 2018). Similar trends were also observed in marine sponge (Thomas et al., 2016) and human gut microbiomes (Turnbaugh and Gordon, 2009), showing that very few common bacteria were shared by individuals. Such a small core suggested that coral microbial communities are more enriched in specialists and opportunists rather than generalists.
Via VPA, we observed that, combined, environmental and spatial factors only played a minor role in shaping the structure of coral-associated microbiomes. This may be in part due to the homogeneous physico-chemical conditions of the offshore reef environment where we sampled. Moreover, this result also suggests that there existed a large proportion of variation that could not be explained via VPA, meaning some other processes such as drift, dispersal limitation, species interaction, host-control, or other unmeasured environmental factors may have significant influence. Although VPA has been widely used in ecological studies to determine the relative importance of environmental selection and spatial effects on microbial community structure, it is limited in inferring the effects from ecological processes (Gilbert and Bennett, 2010; Smith and Lundholm, 2010). For example, the interaction among microbes can affect microbiome structure, which cannot be assessed in the VPA (Lima-Mendez et al., 2015; Wei et al., 2016). Moreover, VPA tends to underestimate the contribution by environmental variables (Gilbert and Bennett, 2010). However, host traits, such as host species and colony size, and the within-host environments such as coral trophic mode are more closely related with the coral-associated microbiome (Pollock et al., 2018), and such biotic environments are difficult to measure. These limitations could explain the low contribution of environmental factors in shaping the prokaryotic community structure observed in the current study.
Via NCM, we were able to estimate the contribution of processes such as dispersal and ecological drift in community assembly, which are difficult to observe but have important effects on the community structures (Tong et al., 2019). The model-fitting parameter R2 in the seawater samples (0.667) was higher than in the coral samples (0.428), suggesting that non-neutral processes may have a stronger effect on coral communities. Furthermore, according to the estimated m value, the dispersal of prokaryotes across coral colonies is likely highly limited (m = 0.031). For the planktonic microbiome, stochastic processes are often observed playing an important role in community assembly, such as in lakes (Roguet et al., 2015), rivers (Chen et al., 2019), and seawater (Mo et al., 2018). However, for the host-associated microbiome, the effects of neutral processes are complex. For example, during the course of zebrafish development, neutral processes played a decreasing role in intestinal microbiome assembly, with the R2 values being 0.81, 0.62, and 0.39 corresponding to larval, juvenile, and adult microbiomes, respectively (Burns et al., 2016). However, also representing a host-associated community, the skin microbiome exhibited a strong neutral processes-controlling feature (Tong et al., 2019). Interestingly, the coral hosts themselves likely refute the neutral theory of biodiversity (Dornelas et al., 2006). Together, these could explain the observed, less neutral pattern of microbial diversity in the coral holobiont.
Unlike the process-oriented neutral models, null models generate statistically expected patterns by deliberately excluding certain mechanisms of interest (Gotelli and Ulrich, 2012) and also have been widely used in microbial ecology studies (Zhou and Ning, 2017). Our null model analysis showed that the coral microbiome was governed by dispersal limitation, homogenous selection, and heterogeneous selection, while the seawater microbiome was mainly controlled by homogenizing dispersal and homogenous selection. This difference might be attributed to the distinct traits of the host-associated and planktonic environments. The broad connection among marine ecosystems would lead to a higher immigration rate and thus higher effect of homogenizing dispersal. As small, single-cell organisms, microbes are considered to be ubiquitous and not dispersal limited (Lindstrom and Ostman, 2011). In contrast, coral microbes inhabit rock-like compartments within the reefs, restricting their dispersal rate between individual corals. Meanwhile, selection was observed to be an important factor, including both homogeneous and heterogeneous selection. Generally, a host allows certain microbial taxa to colonize or persist (i.e., host filtering). It is widely accepted that host filtering is an important selection process (Robinson et al., 2010), which is broadly accepted to be deterministic (Zhou and Ning, 2017). Together, in such coral-associated systems, there are a large number of biotic factors that can shape community assembly, including host filtering and interaction between microbes and hosts, between microbes and symbiotic algae, and between different microbial groups (Levy and Borenstein, 2013; Goodrich et al., 2014). These biotic factors form within-host environments or niches that select certain microbes, which might occur via largely deterministic processes.
The coral microbiome metacommunity in the offshore South China Sea investigated here exhibited common features of host-associated microbial communities, which are high species evenness, high β-diversity, and a small core microbiome. Local environments of the coral hosts and spatial factors had little effect on coral microbiome assembly, while host traits and within-host environmental conditions played important roles in limiting the rate of immigration and filtering the species of coral-associated microbes. Our study highlights the roles of dispersal limitation and selection (host filtering) in shaping coral microbiome diversity and structure. As coral diversity is extremely great and their traits are temporally varying, host selection and dispersal limitation would lead to distinct microbial community among corals, reflected by high β-diversity and small core microbiome. However, traits of host-associated niches are difficult to physico-chemically delineate. Future investigations that link host traits and coral microbiomes would provide more in-depth insight to coral microbial ecology.
The datasets presented in this study can be found in online repositories. The names of the repository/repositories and accession number(s) can be found below: The sequences generated in this study were deposited into the NCBI Short Reads Archive (SRA) database under accession number PRJNA629477.
SH and LL conceived the research. JD organized the research cruises. YS, JZ, JD, and QY conducted the field sampling and the measurement of physico-chemical parameters. JZ and YS processed the samples for sequencing. JZ and AH performed sequence data processing and the statistical analyses. JZ, SH, and AH wrote the manuscript. All authors discussed and approved the final version of the manuscript.
This work was funded by Strategic Priority Research Program of the Chinese Academy of Sciences (Grant Number XDA13020301), National Natural Science Foundation of China (Grant Number 41576126), Key Special Project for Introduced Talents Team of Southern Marine Science and Engineering Guangdong Laboratory (Guangzhou) (Grant Number GML2019ZD0404), Natural Science Foundation of Guangdong Province (Grant Number 2017A030306020), Youth Innovation Promotion Association (Grant Number 2018377), and Rising Star Foundation of the South China Sea Institute of Oceanology (Grant Number NHXX2019ST0101).
The authors declare that the research was conducted in the absence of any commercial or financial relationships that could be construed as a potential conflict of interest.
The Supplementary Material for this article can be found online at: https://www.frontiersin.org/articles/10.3389/fmars.2021.658708/full#supplementary-material
Supplementary Figure 1 | Pearson correlations between microbial diversity indices and environmental factors. The 16S rRNA gene abundance was also included as a biotic factor to assess the relationship between bacterial abundance and diversity.
Supplementary Figure 2 | Bray-Curtis distance between coral samples (n = 15) and between seawater samples (n = 15). A 15 coral samples were randomly selected from the 57 collected, for 100 replicates. Result of one representative run out of the 100 replicates was shown. Kruskal-Wallis test showed significant difference between coral and seawater samples on the β-diversity (Bray-Curtis distance). Significance code: P < 0.01, ∗∗∗.
Supplementary Figure 3 | Percentages of core OTUs (A) and core reads (B) in coral (n = 15) and seawater samples (n = 15). A 15 coral samples were randomly selected from the 57 collected, for 100 replicates. Result of one representative run out of the 100 replicates was shown. Kruskal-Wallis test showed significant difference between coral samples and seawater samples on the proportions of core OTUs and core reads. Significance code: P < 0.01, ∗∗∗.
Supplementary Figure 4 | Correlations among environmental factors and between environmental factors and prokaryotic community. Spearman correlation implemented in the Mantel test was used to assess the relationship. The scale bars show the Mantel’s ρ values of −0.2, 0, 0.2, and 0.5. The Mantel’s P values are indicated by green (≥0.05) or orange (<0.05).
Supplementary File 1 | Information on the sampling sites and sampled coral colonies in the South China Sea (Excel file).
Supplementary File 2 | R codes for neutral model and null model analyses.
Ainsworth, T. D., Krause, L., Bridge, T., Torda, G., Raina, J. B., Zakrzewski, M., et al. (2015). The coral core microbiome identifies rare bacterial taxa as ubiquitous endosymbionts. ISME J. 9, 2261–2274. doi: 10.1038/ismej.2015.39
Brooks, A. W., Kohl, K. D., Brucker, R. M., Van Opstal, E. J., and Bordenstein, S. R. (2016). Phylosymbiosis: relationships and functional effects of microbial communities across host evolutionary history. PLoS Biol. 14:e2000225. doi: 10.1371/journal.pbio.2000225
Burns, A. R., Stephens, W. Z., Stagaman, K., Wong, S., Rawls, J. F., Guillemin, K., et al. (2016). Contribution of neutral processes to the assembly of gut microbial communities in the zebrafish over host development. ISME J. 10, 655–664. doi: 10.1038/ismej.2015.142
Cai, L., Tian, R. M., Zhou, G., Tong, H., Wong, Y. H., Zhang, W., et al. (2018). Exploring coral microbiome assemblages in the South China Sea. Sci. Rep. 8:2428.
Caporaso, J. G., Kuczynski, J., Stombaugh, J., Bittinger, K., Bushman, F. D., and Costello, E. K. (2010). QIIME allows analysis of high-throughput community sequencing data.pdf. Nat. Methods 7, 335–336.
Caporaso, J. G., Lauber, C. L., Walters, W. A., Berg-Lyons, D., Lozupone, C. A., Turnbaugh, P. J., et al. (2011). Global patterns of 16S rRNA diversity at a depth of millions of sequences per sample. Proc. Natl. Acad. Sci. U.S.A. 108, 4516–4522. doi: 10.1073/pnas.1000080107
Chase, J. M. (2010). Stochastic community assembly causes higher biodiversity in more productive environments. Science 328, 1388–1391. doi: 10.1126/science.1187820
Chase, J. M., Kraft, N. J. B., Smith, K. G., Vellend, M., and Inouye, B. D. (2011). Using null models to disentangle variation in community dissimilarity from variation in alpha-diversity. Ecosphere 2:art24. doi: 10.1890/es10-00117.1
Chase, J. M., and Myers, J. A. (2011). Disentangling the importance of ecological niches from stochastic processes across scales. Philos. T R Soc. B. 366, 2351–2363. doi: 10.1098/rstb.2011.0063
Chen, C. P., Tseng, C. H., Chen, C. A., and Tang, S. L. (2011). The dynamics of microbial partnerships in the coral Isopora palifera. ISME J. 5, 728–740. doi: 10.1038/ismej.2010.151
Chen, W. D., Pan, Y. B., Yu, L. Y., Yang, J., and Zhang, W. J. (2017). Patterns and processes in marine microeukaryotic community biogeography from xiamen coastal waters and intertidal sediments, Southeast China. Front. Microbiol. 8:1912.
Chen, W. D., Ren, K. X., Isabwe, A., Chen, H. H., Liu, M., and Yang, J. (2019). Stochastic processes shape microeukaryotic community assembly in a subtropical river across wet and dry seasons. Microbiome 7:138.
Cleary, D. F. R., Swierts, T., Coelho, F. J. R. C., Polonia, A. R. M., Huang, Y. S. M., Ferreira, M. R. S., et al. (2019). The sponge microbiome within the greater coral reef microbial metacommunity. Nat. Commun. 10:1644.
Costanza, R., De Groot, R., Sutton, P., Van Der Ploeg, S., Anderson, S. J., Kubiszewski, I., et al. (2014). Changes in the global value of ecosystem services. Global Environ. Change 26, 152–158.
Dornelas, M., Connolly, S. R., and Hughes, T. P. (2006). Coral reef diversity refutes the neutral theory of biodiversity. Nature 440, 80–82. doi: 10.1038/nature04534
Edgar, R. C. (2013). UPARSE: highly accurate OTU sequences from microbial amplicon reads. Nat. Methods 10, 996–998. doi: 10.1038/nmeth.2604
Fierer, N., Jackson, J. A., Vilgalys, R., and Jackson, R. B. (2005). Assessment of soil microbial community structure by use of taxon-specific quantitative PCR assays. Appl. Environ. Microbiol. 71, 4117–4120. doi: 10.1128/aem.71.7.4117-4120.2005
Gilbert, B., and Bennett, J. R. (2010). Partitioning variation in ecological communities: do the numbers add up? J. Appl. Ecol. 47, 1071–1082. doi: 10.1111/j.1365-2664.2010.01861.x
Ginestet, C. (2011). ggplot2: elegant graphics for data analysis. J. R. Statist. Soc. Series Statist. Soc. 174, 245–245. doi: 10.1111/j.1467-985x.2010.00676_9.x
Goodrich, J. K., Waters, J. L., Poole, A. C., Sutter, J. L., Koren, O., Blekhman, R., et al. (2014). Human genetics shape the gut microbiome. Cell 159, 789–799.
Gotelli, N. J., and Ulrich, W. (2012). Statistical challenges in null model analysis. Oikos 121, 171–180. doi: 10.1111/j.1600-0706.2011.20301.x
Gu, Z. G., Eils, R., and Schlesner, M. (2016). Complex heatmaps reveal patterns and correlations in multidimensional genomic data. Bioinformatics 32, 2847–2849. doi: 10.1093/bioinformatics/btw313
Hernandez-Agreda, A., Gates, R. D., and Ainsworth, T. D. (2017). Defining the core microbiome in corals’ microbial soup. Trends Microbiol. 25, 125–140. doi: 10.1016/j.tim.2016.11.003
Hernandez-Agreda, A., Leggat, W., Bongaerts, P., and Ainsworth, T. D. (2016). The microbial signature provides insight into the mechanistic basis of coral success across reef habitats. Mbio 7:e00560-16.
Hernandez-Agreda, A., Leggat, W., Bongaerts, P., Herrera, C., and Ainsworth, T. D. (2018). Rethinking the coral microbiome: simplicity exists within a diverse microbial biosphere. Mbio 9, 1–14.
Hildebrand, F., Tadeo, R., Voigt, A. Y., Bork, P., and Raes, J. (2014). LotuS an efficient and user-friendly OTU processing pipeline.pdf. Microbiome 2:30. doi: 10.1186/2049-2618-2-30
Hoegh-Guldberg, O., Mumby, P. J., Hooten, A. J., Steneck, R. S., Greenfield, P., Gomez, E., et al. (2007). Coral reefs under rapid climate change and ocean acidification. Science 318, 1737–1742.
Hu, A. Y., Li, S., Zhang, L. P., Wang, H. J., Yang, J., Luo, Z. X., et al. (2018). Prokaryotic footprints in urban water ecosystems: a case study of urban landscape ponds in a coastal city, China. Environ. Pollut. 242, 1729–1739. doi: 10.1016/j.envpol.2018.07.097
Hu, A. Y., Wang, H. J., Cao, M. X., Rashid, A., Li, M. F., and Yu, C. P. (2019). Environmental filtering drives the assembly of habitat generalists and specialists in the coastal sand microbial communities of Southern China. Microorganisms 7:598. doi: 10.3390/microorganisms7120598
Huang, D. W., Licuanan, W. Y., Hoeksema, B. W., Chen, C. A., Ang, P. O., Huang, H., et al. (2015). Extraordinary diversity of reef corals in the South China Sea. Mari. Biodiversity 45, 157–168. doi: 10.1007/s12526-014-0236-1
Leibold, M. A., Holyoak, M., Mouquet, N., Amarasekare, P., Chase, J. M., Hoopes, M. F., et al. (2004). The metacommunity concept: a framework for multi-scale community ecology. Ecol. Lett. 7, 601–613. doi: 10.1111/j.1461-0248.2004.00608.x
Levy, R., and Borenstein, E. (2013). Metabolic modeling of species interaction in the human microbiome elucidates community-level assembly rules. Proc. Natl. Acad. Sci. U.S.A. 110, 12804–12809. doi: 10.1073/pnas.1300926110
Ley, R. E., Hamady, M., Lozupone, C., Turnbaugh, P. J., Ramey, R. R., Bircher, J. S., et al. (2008). Evolution of mammals and their gut microbes. Science 320, 1647–1651.
Li, J., Chen, Q., Zhang, S., Huang, H., Yang, J., Tian, X. P., et al. (2013). Highly heterogeneous bacterial communities associated with the South China Sea reef corals Porites lutea, Galaxea fascicularis and Acropora millepora. PLoS One 8:e71301. doi: 10.1371/journal.pone.0071301
Lima-Mendez, G., Faust, K., Henry, N., Decelle, J., Colin, S., Carcillo, F., et al. (2015). Ocean plankton. determinants of community structure in the global plankton interactome. Science 348:1262073.
Lindstrom, E. S., and Ostman, O. (2011). The importance of dispersal for bacterial community composition and functioning. PLoS One 6:e25883. doi: 10.1371/journal.pone.0025883
Ling, J., Wu, M. L., Chen, Y. F., Zhang, Y. Y., and Dong, J. D. (2014). Identification of spatial and temporal patterns of coastal waters in sanya bay, South China sea by chemometrics. J. Environ. Inform. 23, 37–43. doi: 10.3808/jei.201400255
Martiny, J. B., Bohannan, B. J., Brown, J. H., Colwell, R. K., Fuhrman, J. A., Green, J. L., et al. (2006). Microbial biogeography: putting microorganisms on the map. Nat. Rev. Microbiol. 4, 102–112. doi: 10.1038/nrmicro1341
McMurdie, P. J., and Holmes, S. (2012). Phyloseq: a bioconductor package for handling and analysis of high-throughput phylogenetic sequence data. Pacific. Symposium. Biocomput. 2012, 235–246.
Miller, E. T., Svanback, R., and Bohannan, B. J. M. (2018). Microbiomes as metacommunities: understanding host-associated microbes through metacommunity ecology. Trends Ecol. Evolu. 33, 926–935.
Mo, Y. Y., Zhang, W. J., Yang, J., Lin, Y. S., Yu, Z., and Lin, S. J. (2018). Biogeographic patterns of abundant and rare bacterioplankton in three subtropical bays resulting from selective and neutral processes. ISME J. 12, 2198–2210. doi: 10.1038/s41396-018-0153-6
Ofiteru, I. D., Lunn, M., Curtis, T. P., Wells, G. F., Criddle, C. S., Francis, C. A., et al. (2010). Combined niche and neutral effects in a microbial wastewater treatment community. Proc. Natl. Acad. Sci. U.S.A. 107, 15345–15350. doi: 10.1073/pnas.1000604107
Oksanen, J., Blanchet, F., Friendly, M., Kindt, R., Legendre, P., Mcglinn, D., et al. (2019). Vegan: Community Ecology Package 2.5-6. R Package Version.
Paradis, E., Claude, J., and Strimmer, K. (2004). APE: analyses of phylogenetics and evolution in R language. Bioinformatics 20, 289–290. doi: 10.1093/bioinformatics/btg412
Peres-Neto, P. R., Legendre, P., Dray, S., and Borcard, D. (2006). Variation partitioning of species data matrices: estimation and comparison of fractions. Ecology 87, 2614–2625. doi: 10.1890/0012-9658(2006)87[2614:vposdm]2.0.co;2
Pollock, F. J., Mcminds, R., Smith, S., Bourne, D. G., Willis, B. L., Medina, M., et al. (2018). Coral-associated bacteria demonstrate phylosymbiosis and cophylogeny. Nat. Commun. 9:4921.
Quast, C., Pruesse, E., Yilmaz, P., Gerken, J., Schweer, T., Yarza, P., et al. (2013). The SILVA ribosomal RNA gene database project: improved data processing and web-based tools. Nucleic Acids Res. 41, D590–D596.
Robinson, C. J., Bohannan, B. J., and Young, V. B. (2010). From structure to function: the ecology of host-associated microbial communities. Microbiol. Mol. Biol. Rev. 74, 453–476. doi: 10.1128/mmbr.00014-10
Roguet, A., Laigle, G. S., Therial, C., Bressy, A., Soulignac, F., Catherine, A., et al. (2015). Neutral community model explains the bacterial community assembly in freshwater lakes. FEMS Microbiol. Ecol. 91:fiv125. doi: 10.1093/femsec/fiv125
Rosenberg, E., Koren, O., Reshef, L., Efrony, R., and Zilber-Rosenberg, I. (2007). The role of microorganisms in coral health, disease and evolution. Nat. Rev. Microbiol. 5, 355–362. doi: 10.1038/nrmicro1635
Shade, A., and Handelsman, J. (2012). Beyond the venn diagram: the hunt for a core microbiome. Environ. Microbiol. 14, 4–12. doi: 10.1111/j.1462-2920.2011.02585.x
Silveira, C. B., Cavalcanti, G. S., Walter, J. M., Silva-Lima, A. W., Dinsdale, E. A., Bourne, D. G., et al. (2017). Microbial processes driving coral reef organic carbon flow. FEMS Microbiol. Rev. 41, 575–595. doi: 10.1093/femsre/fux018
Sloan, W. T., Lunn, M., Woodcock, S., Head, I. M., Nee, S., and Curtis, T. P. (2006). Quantifying the roles of immigration and chance in shaping prokaryote community structure. Environ. Microbiol. 8, 732–740. doi: 10.1111/j.1462-2920.2005.00956.x
Smith, T. W., and Lundholm, J. T. (2010). Variation partitioning as a tool to distinguish between niche and neutral processes. Ecography 33, 648–655. doi: 10.1111/j.1600-0587.2009.06105.x
Stegen, J. C., Fredrickson, J. K., Wilkins, M. J., Konopka, A. E., Nelson, W. C., Arntzen, E. V., et al. (2016). Groundwater-surface water mixing shifts ecological assembly processes and stimulates organic carbon turnover. Nat. Commun. 7:11237.
Stegen, J. C., Lin, X., Fredrickson, J. K., Chen, X., Kennedy, D. W., Murray, C. J., et al. (2013). Quantifying community assembly processes and identifying features that impose them. ISME J. 7, 2069–2079. doi: 10.1038/ismej.2013.93
Stegen, J. C., Lin, X., Fredrickson, J. K., and Konopka, A. E. (2015). Estimating and mapping ecological processes influencing microbial community assembly. Front. Microbiol. 6:370.
Sweet, M. J., and Bulling, M. T. (2017). On the importance of the microbiome and pathobiome in coral health and disease. Front. Mari. Sci. 4:00009.
Thomas, T., Moitinho-Silva, L., Lurgi, M., Bjork, J. R., Easson, C., Astudillo-Garcia, C., et al. (2016). Diversity, structure and convergent evolution of the global sponge microbiome. Nat. Commun. 7:11870.
Tong, X. Z., Leung, M. H. Y., Wilkins, D., Cheung, H. H. L., and Lee, P. K. H. (2019). Neutral processes drive seasonal assembly of the skin mycobiome. mSystems 4, e00004–e00019.
Tripathi, B. M., Stegen, J. C., Kim, M., Dong, K., Adams, J. M., and Lee, Y. K. (2018). Soil pH mediates the balance between stochastic and deterministic assembly of bacteria. ISME J. 12, 1072–1083. doi: 10.1038/s41396-018-0082-4
Turnbaugh, P. J., and Gordon, J. I. (2009). The core gut microbiome, energy balance and obesity. J. Physiol. London 587, 4153–4158. doi: 10.1113/jphysiol.2009.174136
Turnbaugh, P. J., Hamady, M., Yatsunenko, T., Cantarel, B. L., Duncan, A., Ley, R. E., et al. (2009). A core gut microbiome in obese and lean twins. Nature 457, 480–484. doi: 10.1038/nature07540
Vellend, M. (2010). Conceptual synthesis in community ecology. Quart. Rev. Biol. 85, 183–206. doi: 10.1086/652373
Vellend, M., Srivastava, D. S., Anderson, K. M., Brown, C. D., Jankowski, J. E., Kleynhans, E. J., et al. (2014). Assessing the relative importance of neutral stochasticity in ecological communities. Oikos 123, 1420–1430. doi: 10.1111/oik.01493
Webster, N. S., Negri, A. P., Botte, E. S., Laffy, P. W., Flores, F., Noonan, S., et al. (2016). Host-associated coral reef microbes respond to the cumulative pressures of ocean warming and ocean acidification. Sci. Rep. 6:19324.
Webster, N. S., and Reusch, T. B. H. (2017). Microbial contributions to the persistence of coral reefs. ISME J. 11, 2167–2174. doi: 10.1038/ismej.2017.66
Wegley, L., Edwards, R., Rodriguez-Brito, B., Liu, H., and Rohwer, F. (2007). Metagenomic analysis of the microbial community associated with the coral Porites astreoides. Environ. Microbiol. 9, 2707–2719. doi: 10.1111/j.1462-2920.2007.01383.x
Wei, G. S., Li, M. C., Li, F. G., Li, H., and Gao, Z. (2016). Distinct distribution patterns of prokaryotes between sediment and water in the Yellow River estuary. Appl. Microbiol. Biotechnol. 100, 9683–9697. doi: 10.1007/s00253-016-7802-3
Yeoh, Y. K., Dennis, P. G., Paungfoo-Lonhienne, C., Weber, L., Brackin, R., Ragan, M. A., et al. (2017). Evolutionary conservation of a core root microbiome across plant phyla along a tropical soil chronosequence. Nat. Commun. 8:215.
Zaura, E., Keijser, B. J., Huse, S. M., and Crielaard, W. (2009). Defining the healthy “core microbiome” of oral microbial communities. BMC microbiology 9:259. doi: 10.1186/1471-2180-9-259
Zhou, G. W., Cai, L., Yuan, T., Tian, R. M., Tong, H. Y., Zhang, W. P., et al. (2017). Microbiome dynamics in early life stages of the scleractinian coral Acropora gemmifera in response to elevated pCO(2). Environ. Microbiol. 19, 3342–3352. doi: 10.1111/1462-2920.13840
Zhou, J. Z., Deng, Y., Zhang, P., Xue, K., Liang, Y. T., Van Nostrand, J. D., et al. (2014). Stochasticity, succession, and environmental perturbations in a fluidic ecosystem. Proc. Natl. Acad. Sci. U.S.A. 111, E836–E845.
Zhou, J. Z., Liu, W. Z., Deng, Y., Jiang, Y. H., Xue, K., He, Z., et al. (2013). Stochastic assembly leads to alternative communities with distinct functions in a bioreactor microbial community. mBio 4:e00584-12.
Zhou, J. Z., and Ning, D. L. (2017). Stochastic community assembly: does it matter in microbial ecology? Microbiol. Mol. Biol. Rev. 81, e00002–e00017.
Keywords: microbial community assembly, coral microbiome, stochastic process, deterministic process, neutral community model, null model, dispersal, South China Sea
Citation: Zhang J, Hu A, Sun Y, Yang Q, Dong J, Long L and Huang S (2021) Dispersal Limitation Expands the Diversity of Coral Microbiome Metacommunity in the South China Sea. Front. Mar. Sci. 8:658708. doi: 10.3389/fmars.2021.658708
Received: 26 January 2021; Accepted: 28 June 2021;
Published: 20 July 2021.
Edited by:
Stefano Goffredo, University of Bologna, ItalyReviewed by:
Cristiane Cassiolato Pires Hardoim, São Paulo State University, BrazilCopyright © 2021 Zhang, Hu, Sun, Yang, Dong, Long and Huang. This is an open-access article distributed under the terms of the Creative Commons Attribution License (CC BY). The use, distribution or reproduction in other forums is permitted, provided the original author(s) and the copyright owner(s) are credited and that the original publication in this journal is cited, in accordance with accepted academic practice. No use, distribution or reproduction is permitted which does not comply with these terms.
*Correspondence: Sijun Huang, aHVhbmdzaWp1bkBzY3Npby5hYy5jbg==; Lijuan Long, bG9uZ2xqQHNjc2lvLmFjLmNu
Disclaimer: All claims expressed in this article are solely those of the authors and do not necessarily represent those of their affiliated organizations, or those of the publisher, the editors and the reviewers. Any product that may be evaluated in this article or claim that may be made by its manufacturer is not guaranteed or endorsed by the publisher.
Research integrity at Frontiers
Learn more about the work of our research integrity team to safeguard the quality of each article we publish.