- 1School of Electrical Engineering and Computer Science, College of Engineering, Oregon State University, Corvallis, OR, United States
- 2Coastal Oregon Marine Experiment Station, Department of Fisheries and Wildlife, Oregon State University, Newport, OR, United States
- 3College of Earth, Ocean, and Atmospheric Sciences, Oregon State University, Corvallis, OR, United States
- 4National Oceanic and Atmospheric Administration, National Marine Fisheries Service, Alaska Fisheries Science Center, Hatfield Marine Science Center, Newport, OR, United States
Quantifying the spatial and temporal footprint of multiple environmental stressors on marine fisheries is imperative to understanding the effects of changing ocean conditions on living marine resources. Pacific Cod (Gadus macrocephalus), an important marine species in the Gulf of Alaska ecosystem, has declined dramatically in recent years, likely in response to extreme environmental variability in the Gulf of Alaska related to anomalous marine heatwave conditions in 2014–2016 and 2019. Here, we evaluate the effects of two potential environmental stressors, temperature variability and ocean acidification, on the growth of juvenile Pacific Cod in the Gulf of Alaska using a novel machine-learning framework called “stress-scapes,” which applies the fundamentals of dynamic seascape classification to both environmental and biological data. Stress-scapes apply a probabilistic self-organizing map (prSOM) machine learning algorithm and Hierarchical Agglomerative Clustering (HAC) analysis to produce distinct, dynamic patches of the ocean that share similar environmental variability and Pacific Cod growth characteristics, preserve the topology of the underlying data, and are robust to non-linear biological patterns. We then compare stress-scape output classes to Pacific Cod growth rates in the field using otolith increment analysis. Our work successfully resolved five dynamic stress-scapes in the coastal Gulf of Alaska ecosystem from 2010 to 2016. We utilized stress-scapes to compare conditions during the 2014–2016 marine heatwave to cooler years immediately prior and found that the stress-scapes captured distinct heatwave and non-heatwave classes, which highlighted high juvenile Pacific Cod growth and anomalous environmental conditions during heatwave conditions. Dominant stress-scapes underestimated juvenile Pacific Cod growth across all study years when compared to otolith-derived field growth rates, highlighting the potential for selective mortality or biological parameters currently missing in the stress-scape model as well as differences in potential growth predicted by the stress-scape and realized growth observed in the field. A sensitivity analysis of the stress-scape classification result shows that including growth rate data in stress-scape classification adjusts the training of the prSOM, enabling it to distinguish between regions where elevated sea surface temperature is negatively impacting growth rates. Classifications that rely solely on environmental data fail to distinguish these regions. With their incorporation of environmental and non-linear physiological variables across a wide spatio-temporal scale, stress-scapes show promise as an emerging methodology for evaluating the response of marine fisheries to changing ocean conditions in any dynamic marine system where sufficient data are available.
Introduction
Environmental variability and associated trends induced by climate change can create unique and potentially stressful conditions for marine fisheries that fluctuate through space and time. Increasing water temperatures and ocean acidification related to changing ocean conditions have the potential to negatively impact individual fish – through direct and indirect effects – which can potentially impact fisheries by way of fishable biomass, annual stock productivity, and spatial shifts in the population outside of the traditional fishing areas (Holsman et al., 2020; Laurel and Rogers, 2020). The Gulf of Alaska Pacific Cod (Gadus macrocephalus) fishery is one example of a commercial fishery that is highly susceptible to changing ocean conditions. In recent years, Pacific Cod abundance has declined dramatically in the Gulf of Alaska (GOA) ecosystem, leading to a fisheries disaster declaration in 2018 and a closure of the federal fishery in 2020 (Barbeaux et al., 2020). Quantifying the spatial and temporal footprint of modern environmental stressors on GOA Pacific Cod is crucial for ensuring the accuracy of plans and forecasts for this valuable fishery.
Decadal and long-term patterns influence the GOA’s thermal variability due to warm-phase shifts of climatic phenomena and background warming related to climate change. The GOA has also experienced two anomalous marine heatwave events in the past decade. Between late 2013 and 2016, a marine heatwave occurred in the GOA that exceeded the magnitude and duration of any other heatwave on record in the region. This heatwave event led to temperature anomalies greater than 2.5°C (Bond et al., 2015; Di Lorenzo and Mantua, 2016) and unprecedented shifts in the region’s biological communities, including increases in harmful algal blooms, reductions in fishery recruitment, and mass mortality of marine mammals and seabirds (Leising et al., 2015; Piatt et al., 2020; Suryan et al., 2021). In the summer of 2019, the GOA was impacted by another marine heatwave, leading to similarly extreme temperature anomalies (Amaya et al., 2020). The increasing frequency and intensity of marine heatwaves in the coming years are likely to coincide with challenges to marine fisheries’ long-term health (Oliver et al., 2018; Cornwall, 2019).
In addition to thermal variability, there are several sources of environmental variability that co-occur in the GOA and which have the potential to overlap, compound, or negate one another in ways that are still not fully understood (Bopp et al., 2013). Ocean acidification is one such potential stressor and refers to the gradual increase in hydrogen ions and consumption of carbonate ions in marine systems in response to elevated levels of anthropogenic carbon dioxide entering the ecosystem (e.g., Feely et al., 2004; Evans et al., 2014). The GOA, with the naturally lower concentrations of carbonate ions characteristic of high latitude marine habitats, is at higher risk for the effects of ocean acidification as additional losses of carbonate ions can lead to proportionally larger changes in seawater chemistry than would be expected in lower latitude systems (Fabry et al., 2009; Mathis et al., 2011). Additionally, the GOA and Kodiak Island regions are known to have higher seasonal variability in natural surface pCO2 levels (seasonal amplitudes of as much as 309.8 and 279.4 μatm, respectively) when compared to open ocean environments due to upwelling conditions (Chen and Hu, 2019). Some interactions between sea surface temperature and the carbonate system are known – for example, carbon dioxide becomes less soluble in water as water temperature increases – but the full effects are not yet known in the context of physiological stress to living marine resources (Mathis et al., 2015).
Recent declines in Pacific Cod stocks are likely connected to changing thermal conditions in the GOA. Pacific Cod is a stenothermic species with peak hatch success occurring in 4–5.5°C water (Laurel and Rogers, 2020) and optimal juvenile growth occurring below 13°C in nursery habitats (Hurst et al., 2010). It is likely that Pacific Cod, like other stenothermic species, exhibit stage-specific responses to warming, with potential for thermal bottlenecks between different life stages (Dahlke et al., 2020; Rogers et al., 2020). Early life stages may be particularly sensitive to changes in temperature due to higher metabolic rates in the embryonic, larval, and juvenile stages (e.g., Finn et al., 2002: Werner, 2002). Elevated temperatures in the GOA are associated with high larval mortality of Pacific Cod (Doyle and Mier, 2016) and a larger size at nursery entry. During the 2014–2016 and the 2019 marine heatwaves, it is hypothesized that a loss of suitable spawning habitat during heatwave conditions contributed to declines in Pacific Cod hatch success and consequent older life stages (Laurel and Rogers, 2020).
Pacific Cod also appear to be moderately impacted by ocean acidification in the GOA. Elevated levels of carbon dioxide in the water column during the first two weeks of the larval duration are associated with suppressed growth, although this trend has been shown to reverse as larvae grow (Hurst et al., 2019). By the juvenile stage, effects of elevated carbon dioxide levels on growth largely disappear (Hurst et al., 2012). It is hypothesized that fishes are better able to withstand the effects of ocean acidification than calcifying marine invertebrates due to their high metabolism and ability to regulate their inter/intracellular acid-base balance (e.g., Melzner et al., 2009). However, elevated levels of carbon dioxide could lead to several indirect impacts on fishes including behavioral changes (e.g., Williams et al., 2019); changes in the biochemical structure of prey (e.g., Cripps et al., 2016); and changes in the abundance of calcifying marine invertebrate prey (e.g., Lischka et al., 2011). Many of the direct and indirect impacts of ocean acidification on cod and other marine finfish species are unknown, and the modest impacts of ocean acidification observed in the laboratory may indeed be “significant” when compounded by other environmental stressors or realized over longer time scales. Here, we do not account for potential interaction between temperature and ocean acidification as further studies are needed to better understand the interactive effects of these two variables on Pacific Cod early life stages.
Quantifying and visualizing environmental conditions in space and time can be beneficial in understanding when and where the Pacific Cod fishery may be most influenced by changing ocean conditions in the GOA. By viewing the ocean as a dynamic mosaic of physical and biogeochemical seascapes, researchers, managers, and other end-users can efficiently communicate information about environmental conditions in the ocean through space and time. This approach is known as dynamic seascape classification (Kavanaugh et al., 2014). Recent advances have allowed for the dynamic classification of ocean regions based on multiple biophysical interactions observable by satellite remote sensing or marine ecosystem models (e.g., Kavanaugh et al., 2018). These dynamic classifications have been used to predict biogeochemical processes (Kavanaugh et al., 2014), phytoplankton structure and function (Kavanaugh et al., 2015; Montes et al., 2020), and individual species occurrences (Breece et al., 2016). The seascape ecology framework has potential utility for quantifying biological and human responses to known environmental stressors, including elevated temperature and ocean pCO2. By applying a dynamic seascape framework in marine resource management contexts, Klajbor (2020) established possible novel relationships between dynamic ocean management principles and socioeconomic vulnerability in fishing communities.
Large, biophysical time series like those used in a seascape ecology approach have been classified in space and time simultaneously using a probabilistic self-organizing map (prSOM) machine learning algorithm (Kavanaugh et al., 2014, 2016, 2018). The prSOM combines two statistical algorithms, K-Means and expectation-maximization, and a machine learning algorithm called the Self-Organizing Map (Kohonen, 1990; Richardson et al., 2003) to approximate the multivariate distribution of data as a mixture of Gaussians and uniform distributions (Anouar et al., 1998; Lebbah et al., 2015). The prSOM algorithm has utility in capturing biological metrics, such as Pacific Cod growth, in addition to environmental metrics because it is robust to non-linear relationships between variables and is topology-preserving. Also, the prSOM algorithm does not rely on data being identically distributed or independent (i.i.d). This feature is critical for classifying complex, potentially non-linear interactions like those found in data from spatio-temporal time series, which are not independent because the current year’s classification almost always depends on previous months, years, and nearby regions.
Here, we introduce “stress-scapes” as a novel machine learning framework to understand juvenile Pacific Cod’s response to potential environmental stressors across the GOA ecosystem. Juvenile Pacific Cod was selected for this project due to the availability of information and data related to the effects of temperature and ocean acidification on early life stages. While there remain many unknown biological responses to these stressors, both alone and in their potential interactive effects, we incorporate the most recent available data on this critical species for this proof-of-concept study. Stress-scapes incorporate remotely sensed sea surface temperature (SST) data, pCO2 data, and estimated juvenile Pacific Cod specific growth rate (SGR) into a prSOM algorithm to identify ocean conditions that may be stressful to juvenile Pacific Cod across a broad spatial and temporal scale. The juvenile Pacific Cod stress-scape presented in this study is not intended as a comprehensive reconstruction of Pacific Cod growth history in response to environmental variability in the GOA, but rather as a novel framework to visualize and classify potential environmental stress on the species across space and time. In this way, the stress-scape framework is distinct from an Individual-Based Model (IBM), which is a biophysical model that describes fish growth, recruitment, and mortality as dependent on environmental factors (Hinckley et al., 1996; Hermann and Moore, 2009; Koenigstein et al., 2016). IBMs are often coupled with Regional Ocean Modeling Systems (ROMS) and dispersal models to capture environmental conditions at depth and track the movement of fish in early life stages through their environment (e.g., Stockhausen et al., 2019). An IBM for Pacific Cod has already been developed for the GOA ecosystem (Hinckley et al., 2019).
In our case study, we develop weekly stress-scapes from 2010 to 2016, including marine heatwave conditions in 2014–2016, using existing juvenile Pacific Cod laboratory growth models and remotely sensed environmental data. These stress-scapes are then evaluated spatiotemporally to determine how well they capture changing conditions both seasonally and interannually, including during the marine heatwave event. We then evaluate the sensitivity of the stress-scape model to the Pacific Cod SGR input variable to determine the importance of this variable in influencing the model’s classification. Finally, we compare the stress-scape model output to otolith-derived growth data from juvenile Pacific Cod collected from Kodiak Island, AK to comment on the success of the model in capturing processes occurring in the field.
Materials and Methods
Environmental Data
Sea surface temperature and sea-surface carbonate chemistry data (pCO2) were collated for the GOA ecosystem between the years 2010–2016 (see Supplementary Material: Appendix A for spatial extent of environmental data). Environmental data were selected from publicly available modeled, in situ, and remotely sensed products. The data’s temporal range was selected to include dates before, during, and after the 2014–2016 marine heatwave event. Sea surface temperature data were subset from the National Oceanic and Atmospheric Administration (NOAA) Optimum Reynolds Interpolation SST V2 product provided by the OAR/ESRL PSL, Boulder, Colorado, United States (Reynolds et al., 2002). The product is developed using a combination of in situ and remotely sensed data, producing weekly means on a one-degree grid. Associated variance related to each point was also downloaded and used to calculate total error related to the stress-scape classification. Sea-surface carbonate chemistry data were subset from the NOAA National Center for Environmental Information CSIR-ML6 v2019a Global Sea-Surface pCO2 product. A smoothed mean monthly pCO2 product was selected from their ensemble mean product that is calculated using a combination of 6 machine learning techniques. The smoothed product differs from their raw product, as a 3 × 3 × 3 convolution is applied to the data through time, latitude, and longitude (Gregor et al., 2019). Mean monthly values ranged from 262.6 to 469.4 μatm in the study domain.
Only the environmental data from the known spatial and temporal ranges of Pacific Cod were included in the subsequent machine learning training and stress-scape visualization, ensuring that the selected data and resulting stress-scapes were relevant to juvenile Pacific Cod distribution. Age-0 juvenile Pacific Cod are distributed in shallow, nearshore habitats, typically <30 m depth during their first summer (Laurel et al., 2007, 2009). We used a bathymetric data subset from the General Bathymetric Chart of the Oceans 2020 Gridded Global Bathymetry product (GEBCO Bathymetric Compilation Group 2020, 2020) for 30 m and 100 m isobaths to compare habitat size across both depth distributions. Due to the small size of the habitat at <30 m depth, we selected 100 m depth as the cutoff for the juvenile habitat, acknowledging that this represents a broader habitat range than would be expected for a typical age-0 juvenile Pacific Cod during their first summer and subsequently may bias our interpretation toward cooler temperatures than would be experienced in situ (e.g., Laurel et al., 2012). We used a year-round temporal range for the juveniles in the nursery habitat to represent both the first summer in the nursery and overwintering in the nursery. The study’s spatial domain only includes the GOA, so any data that our filter included from the Bering Sea were excluded from the training of the prSOM model. Additionally, there were 5 points where the pCO2 or SST were not available (Supplementary Material: Appendix B); rather than attempt to interpolate the data where it was not possible due to missing data, the value of each pixel was set to NaN.
To account for seasonal variance the monthly z-scores were computed for each grid point across the GOA for each of the relevant variables:
where j is the variable index, i is the observation index and the represents an average value across the entire GOA through time. The spatio-temporal z-scores were used to train the prSOM to compute stress-scape classes across the GOA from 2010 to 2016 (Gregor et al., 2019); hence, the stress-scape classes represent regions that similarly deviate from the spatio-temporal mean.
Juvenile Pacific Cod Growth Data
Juvenile Pacific Cod temperature-dependent growth data were obtained from two existing experimental studies: Laurel et al. (2016) and Hurst et al. (2010). These studies were all conducted in the laboratory using age-0 juveniles collected from the GOA population. Similar growth data related to variable pCO2 are currently unavailable for age-0 juvenile Pacific Cod [although see Hurst et al. (2019) for earlier life stage responses]. As a proxy, we used the pCO2 growth response of age-0 juvenile Walleye Pollock (Gadus chalcogrammus) based on experimental work from Hurst et al. (2012). While response to ocean acidification is variable among marine finfish, Walleye Pollock are congeners of Pacific Cod and share a similar growth response at the age-0 juvenile stage (Laurel et al., 2016).
A weight-based growth model was used instead of a length-based growth model throughout this stress-scape analysis to better align with potential future bioenergetic applications of the stress-scape to fish bioenergetics. Therefore, a specific growth rate (SGR) model was used to approximate the percent change of fish wet weight over time. SGR were calculated from the formula:
where g is the instantaneous growth coefficient obtained from the equation:
where WW1 is the wet weight of the fish at the beginning of the experiment at time t1, and WW2 is the wet weight of the fish at the conclusion of the experiment at time t2.
A temperature-dependent growth model was developed using a general linear model with SGR as the independent variable and a quadratic temperature term as the dependent variable (Simple Linear Regression; R2adj = 0.636; P < 0.0001). Uncertainty in the model was evaluated using 95% confidence intervals and 95% prediction intervals.
Juvenile Pacific Cod growth response to ocean acidification was divided into two potential outcomes: growth under “low” pCO2 conditions (<1,000 μatm) and growth under “high” pCO2 conditions (>1,000 μatm). These two classifications represent growth at ambient to moderate pCO2 concentrations (“low”), and growth at high to very high pCO2 concentrations (“high”), because juvenile Pacific Cod growth only begins to change as pCO2 concentrations become very high (e.g., Walleye Pollock; Hurst et al., 2012). Growth under low pCO2 conditions was unchanged from the temperature-dependent growth curve and was represented by the equation:
Growth curves under high pCO2 conditions were calculated from the percent decrease in growth (−2.3%) observed in Hurst et al. (2012). Specific growth rates from the temperature-dependent growth curves were then depressed to reflect the percent change in growth that would be expected under elevated pCO2 conditions. Growth under high pCO2 conditions was represented by the equation:
Classification of Stress-Scapes
Stress-scapes were classified using remotely sensed observations of SST; partial pressure of CO2 (pCO2) as an indicator of the carbonate system; and the specific growth rate (SGR) of juvenile Pacific Cod. The prSOM approximates the multivariate distribution of SST, pCO2 and SGR through space and time. The prSOM was configured with 225 neurons in a 15 × 15 neuron map. Previous efforts in the North Pacific found that this configuration resulted in sufficiently large number of data points (e.g., >500) per node (Kavanaugh et al., 2014). The prSOM algorithm executed 50 epochs in order to give the prSOM enough time to converge. We applied Hierarchical Agglomerative Clustering (HAC) to the 225 neurons grouping them into five individual stress-scapes (e.g., Saraceno et al., 2006; Hales et al., 2012; Kavanaugh et al., 2018).
The SST, pCO2, and SGR maps of the GOA were interpolated using bilinear interpolation from 14 × 40 grid points to a 780 × 2340 image to classify stress-scape class boundaries in the remotely sensed data. The interpolated image was copied to the Graphics Processing Unit (GPU) memory and classified according to the trained neurons’ weight vectors and variances by identifying the stress-scape class most likely to generate each interpolated value in the GOA. Our unique prSOM implementation classified the GOA in parallel using the GPU. Parallel classification is an important distinction because many visualization methods rely on processing done on the Central Processing Unit (CPU), but the amount of data we needed to classify made classification on the CPU impractical. Any consumer-grade GPU released in the past decade can classify the entire GOA in a small number of clock cycles (∼1–100 clock cycles) by taking advantage of massively parallel processing. In contrast, even with a parallel CPU implementation, the number of clock cycles will range from 300,000 to 1.7 million clock cycles on most modern processors. There are 365 time slices, so the classification must be done 365 times; hence the speedup is substantial.
We selected 5 stress-scape classes from the HAC using the Gap Statistic (Tibshirani et al., 2001) which suggested 5 classes with 2 well separated classes and 3 less separated classes. Choosing an optimal number of stress-scapes is difficult as there is no single metric that can select an ideal number of clusters with certainty, and often, the statistically significant number of clusters does not agree with domain expert analysis. For our implementation, we compared results from three different methods of clustering evaluation: the elbow method, the silhouette method, and the Gap Statistic (see Supplementary Material: Appendix C). The elbow method works by estimating the amount of variance in the data explained by the clusters, an optimal number of clusters is chosen by identifying the point in the graph where an increase in the number of clusters does not provide additional explanation of the variance. The silhouette method works by estimating the quality of each cluster and then reporting the average quality over all the clusters for increasing number of clusters. An optimal number of clusters can be determined from the silhouette method by choosing a number K for which the quality of the clusters is greater than the quality of the K-1 and K + 1 clusters. The Gap Statistic works by computing the HAC algorithm for an increasing number of clusters. For each number of clusters, from 1 to 10, we computed the inter-cluster sum of square error, the average quality of the clusters, and the gap statistic using the clustGap() function in R.
In order to evaluate the effects of anomalous heatwave conditions on the stress-scape classification results, classification results from 2010 to 2013 were compared to classifications during a known anomaly, the marine heatwave event of 2014–2016, to more normal ocean conditions preceding the heatwave. In comparing the classification results, we identified a dominant stress-scape and examined the qualities of the dominant stress-scape using a decision tree and a table to records the frequency and the environmental characteristics of the stress-scapes. Further details describing our implementation of the prSOM are provided in Supplementary Material: Appendix C.
Sensitivity Analysis
We trained a second, bivariate prSOM to compare the results of the stress-scape to a classification that did not include juvenile Pacific Cod SGR. This bivariate classification allowed us to evaluate the sensitivity of the trivariate stress-scape framework to the additional information gained by including the juvenile Pacific Cod SGR variable in the training process. To account for the reduced variance in the bivariate prSOM, we used the Gap Statistic to estimate 4 classes in the bivariate prSOM. We applied Hierarchical Agglomerative clustering until the 225 neurons were merged into 4 distinct stress-scape classes. We focused our comparison efforts on the coastal regions near Kodiak Island.
Pacific Cod Field Growth
Otolith structural analysis of juvenile Pacific Cod collected from Kodiak Island nurseries is being completed as part of a related study (Thalmann et al., unpublished data). Therefore, after developing the lab-based model for juvenile Pacific Cod SGR, training the prSOM algorithm, and generating the mosaic of stress-scapes across the coastal GOA, we estimated otolith-based SGR from these field-collected juveniles to compare with our stress-scape classifications of growth observed in nearshore Kodiak Island nursery habitat. This comparison aimed not to provide a comprehensive analysis of otolith data but rather to identify a relationship between temporal patterns in the otolith-based growth rates and temporal patterns in the stress-scapes. Juvenile Pacific Cod were collected in annual summer beach seine surveys on Kodiak Island in 2010 and 2012–2016 through the NOAA Alaska Fisheries Science Center Fisheries Behavioral Ecology program. Samples were not available in 2011. Pacific Cod were collected from two shallow (<30 m) embayments along the northeastern coast of Kodiak Island during successive days in July each year. Fish were captured using a demersal beach seine (36 m bag with 5-mm mesh; 1 m × 2.25 m wings with 13-mm mesh), sorted to species, counted, and measured (total length, mm). Samples were then frozen (−20°C) and returned to Hatfield Marine Science Center in Newport, OR for post-processing. During post-processing, standard length (mm) and wet weight (g) were measured, and otoliths were removed for analysis (Table 1).
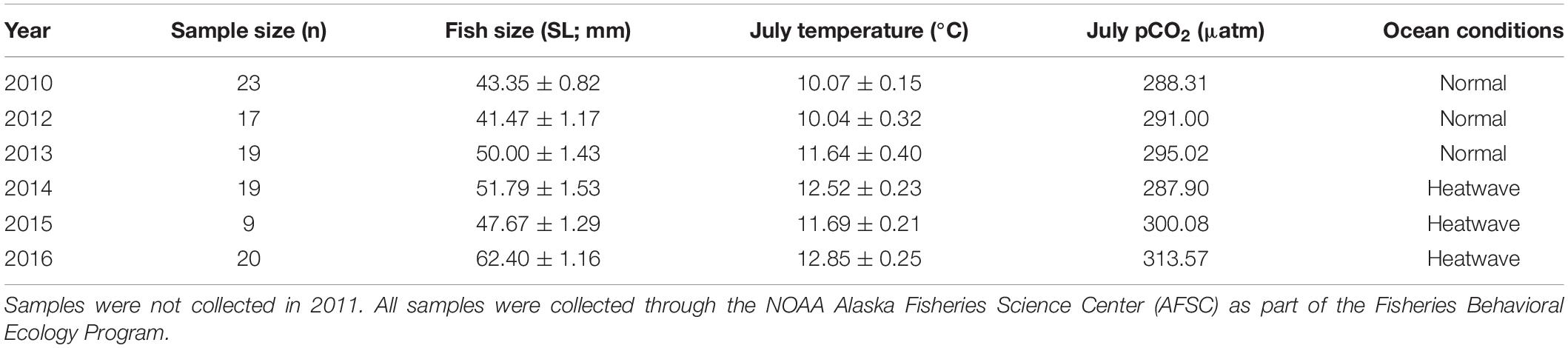
Table 1. Annual sample sizes, average fish size, mean July ocean temperature, mean July pCO2 levels, and ocean conditions for juvenile Pacific Cod captured annually in July 2010 and 2012–2016 from Kodiak Island, AK.
Otolith structural analysis was used to generate growth estimates for field-collected juveniles collected at Kodiak Island. Otoliths are calcium carbonate structures in the inner ear of teleost fishes that can be used to determine age and growth of an individual (Pannella, 1971; Campana and Thorrold, 2001). Otolith size and body size are highly correlated in Pacific Cod (DiMaria et al., 2010; Miller et al., 2016), and daily formation of otolith increments has been validated for Pacific Cod at 10°C up to 120 days post-hatch (Narimatsij et al., 2007). Sagittal otoliths were mounted on glass slides using thermoplastic resin and polished to expose the core in the transverse plane using Wetordry paper (800–2000 grit), Buehler lapping film (3–30 micron grit), and alumina slurry (0.3 micron). Polished otoliths were imaged at 100× and 400× magnification using a Leica DM1000 compound microscope and a Levenhuk M1000 digital camera. Final otolith radius and otolith radius from 8 and 16 days prior to capture were quantified using increment analysis. Daily increments for these two 8-day growth periods (representing three weeks of nursery residence at the same 8-day resolution as the stress-scape model) were counted and measured using ImagePro Premier software. Each otolith was interpreted three times with greater than 80% similarity in mean increment width between reads, and reads were averaged in order to compare otolith-generated estimates of growth to the stress-scape.
First, we estimated fish length and mass at 8-d and 16-d prior to capture based on otolith increment analysis, and then estimated growth in length and mass over these two 8-d periods for comparison with the 8-d prSOM output. Otolith radius measurements and fish standard length were log-transformed to meet parametric assumptions, and a log-linear model with otolith radius, year, and their interaction as independent variables and standard length as a dependent variable was developed (Multiple Linear Regression; R2adj. = 0.85; P < 0.0001; Supplementary Material: Appendix D). Using this relationship, we developed a proportional back-calculation model to estimate fish size 8- and 16-d prior to capture using the measured otolith radius from 8- and 16-d prior to capture (Francis, 1990; Campana and Jones, 1992). Length-based growth of juvenile Pacific Cod in mm per day was examined for each year and compared to a mm/d laboratory growth model obtained from the same studies (Hurst et al., 2010; Laurel et al., 2016) as the laboratory SGR model used in the stress-scape analysis.
To estimate juvenile wet weight 8- and 16-d prior to capture, we developed a second log-linear model with standard length and year as independent variables and wet weight as a dependent variable (Multiple Linear Regression; R2adj. = 0.96; P < 0.0001; Supplementary Material: Appendix D). Wet weight from 8- and 16-d prior to capture was then determined using a second proportional back-calculation model using estimated standard length from 8- and 16-d prior to capture. Juvenile Pacific Cod SGR in the field was calculated in the same manner as the laboratory SGR model described above from Hurst et al. (2010) and Laurel et al. (2016). We utilized weight-based SGR calculated from otoliths rather than length-based growth estimates for comparison to the stress-scape.
To compare otolith-generated SGR estimates from the 8- and 16- day periods prior to capture with the stress-scape, we determined the dominant stress-scape class for coastal Kodiak Island at an 8-day resolution in July for each year between 2010 and 2016. Mean SGR from the dominant stress-scape class was then compared to otolith-generated SGR for that year using a paired t-test. Otolith-generated growth estimates for each year were additionally compared to the temperature-dependent laboratory growth model using a paired t-test. We also compared otolith-derived growth rates in heatwave conditions in 2014–2016 to more normal conditions in 2010–2013 using a 1-way analysis of variance.
Results
Stress-Scape Analysis
Utilizing the prSOM and HAC, we resolved 5 unique stress-scapes across the GOA that quantified the spatio-temporal footprint of changing ocean conditions and the modeled specific growth rate (SGR) of juvenile Pacific Cod. Table 2 describes the means and standard deviations for the SST, pCO2, and SGR values of each of the resolved classes. Stress-scape classes were determined and distinguished semantically through a decision-tree of z-scores for each input variable, including the characteristics of each decision mode, which effectively describes how the classes were sorted (Figure 1). Stress-scape classes 2, 3, 4, and 5 (maroon, green, orange, and yellow) were distinguished by their respective growth rate input variables when they were decided by node 5. This highlights the non-linear relationship between SST and SGR. Class 1 (blue) was primarily distinguished by differences in SST and pCO2.
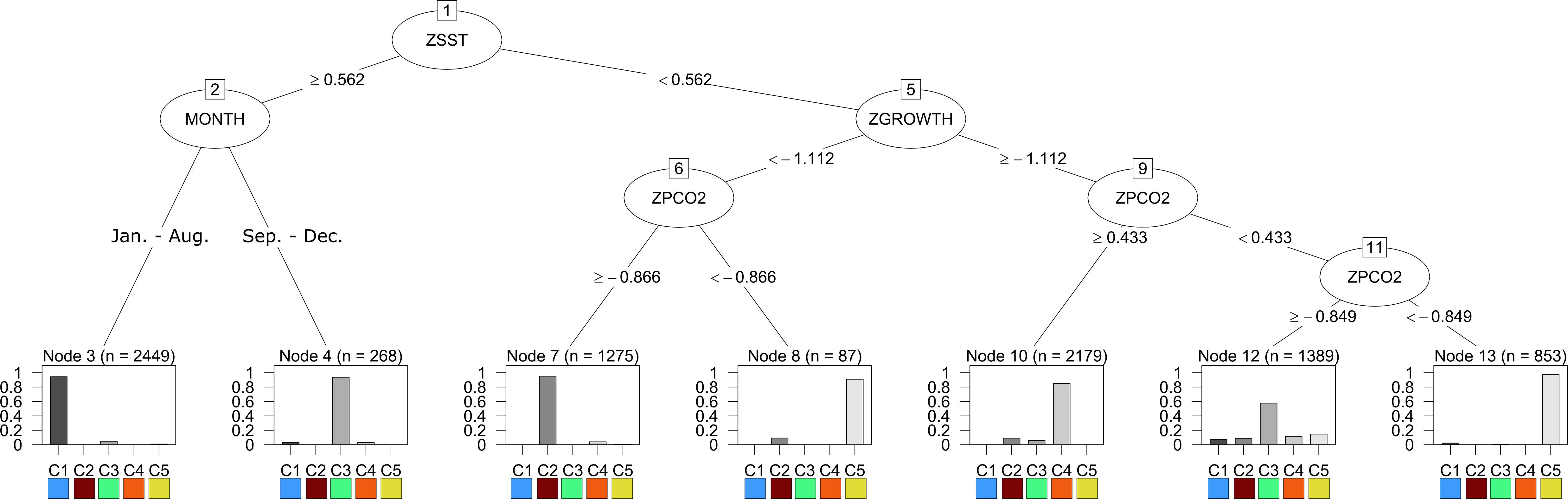
Figure 1. The results of the decision tree trained on the output of stress-scape classification using 5-stress-scapes and 4 variables: SST z-score (ZSST), juvenile Pacific Cod SGR z-score (ZGROWTH) measured in percent wet weight per day (%WW/day), pCO2 concentration (μatm) z-score (ZPCO2), and the month of the remotely sensed observation (MONTH). Each node of the decision tree represents a decision about the variable labeling the vertex and is labeled with a number. The root node represents a decision about the monthly z-score of SST. If the pixel’s SST is greater than.562 standard deviations above the mean SST value across the entire GOA from 2010 to 2016, the left branch is taken; otherwise, the right branch is taken. Note that by including SGR (ZGROWTH) as a classification variable we can make biologically relevant interpretations of the stress-scape classes that are classified by the right branch of node 1. The bar graph provides the percent of each class that is classified by each leaf node of the tree.
The stress-scape analysis identified distinct classes that corresponded to heatwave conditions in 2014–2016 compared to more normal ocean conditions in 2010–2013 for both coastal Kodiak Island (Figure 2) and the larger GOA coastal ecosystem (Figure 3). Animated versions of these figures with corresponding ribbon plots that show how the stress-scapes shift and progress through time can be found in Supplementary Material: Appendix E, Supplementary Videos 1, 2. At both the Kodiak and regional scales, seasonal and interannual patterns emerge in the incidence of each of the classes. Classes 2 and 5 contract over time, supplanted by expansions (in space and time) of classes 1 and 4. Class 2 is the low SST class that seems to be replaced by high pCO2 in class 4. Class 5, the pre-heatwave late spring-early summer class, is replaced by class 3, characterized by high SST, during heatwave years. Class 3 starts appearing earlier and persisting each year during the heatwave.
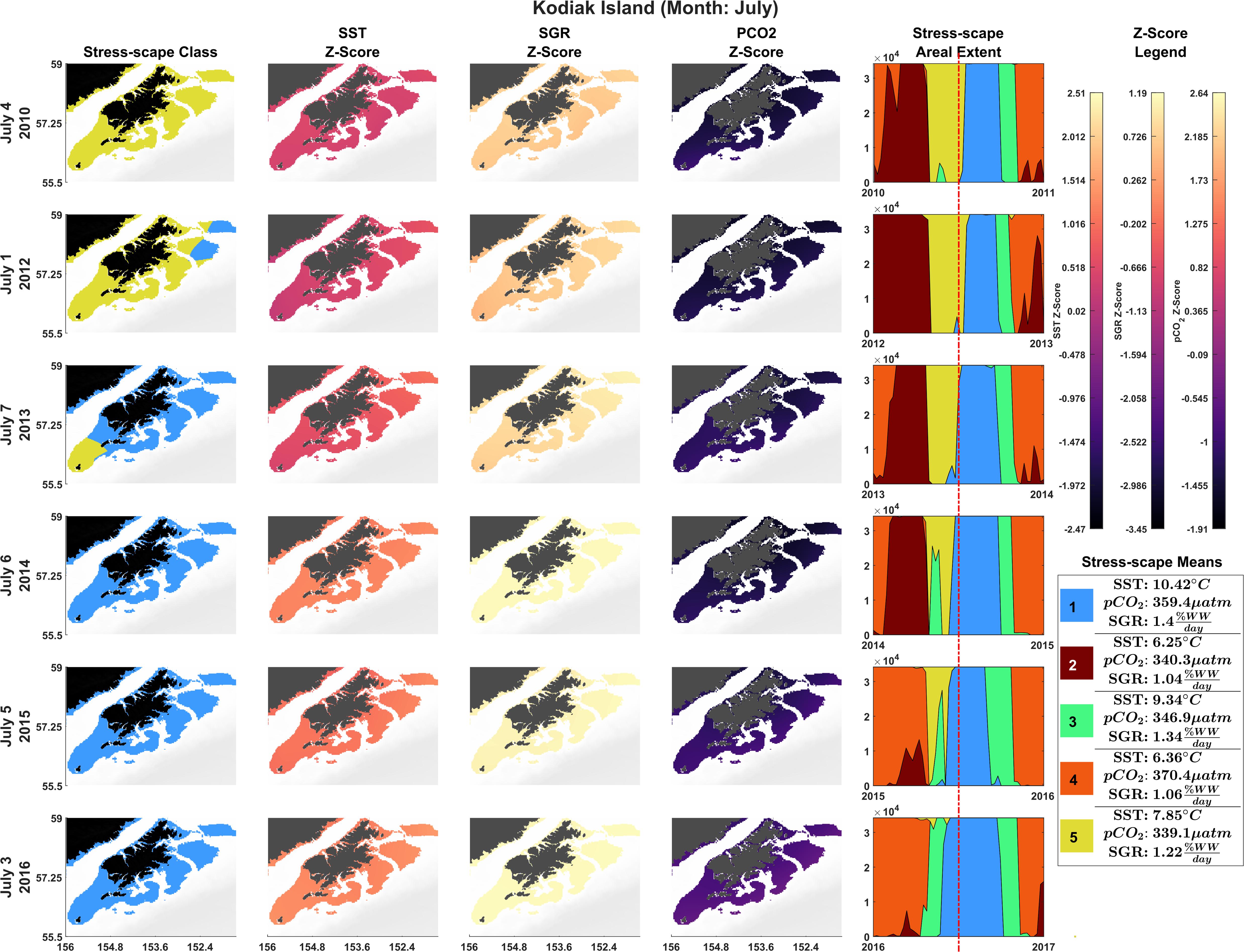
Figure 2. Dominant yearly stress-scape classes for coastal Kodiak Island during the first week of July 2010 and 2012–2016. Heatwave conditions are present in 2014–2016. Column 1 highlights the dominant stress-scape classes for coastal Kodiak Island. Column 2 is the z-score for SST; Column 3 is the z-score for SGR, and Column 4 is the z-score for pCO2. The red line marks the time shown in the figure across all years. Dark purple z-scores are below average and yellow z-scores are above average for that variable. An animated version of this figure can be found in Supplementary Material: Appendix E and Supplementary Video 1.
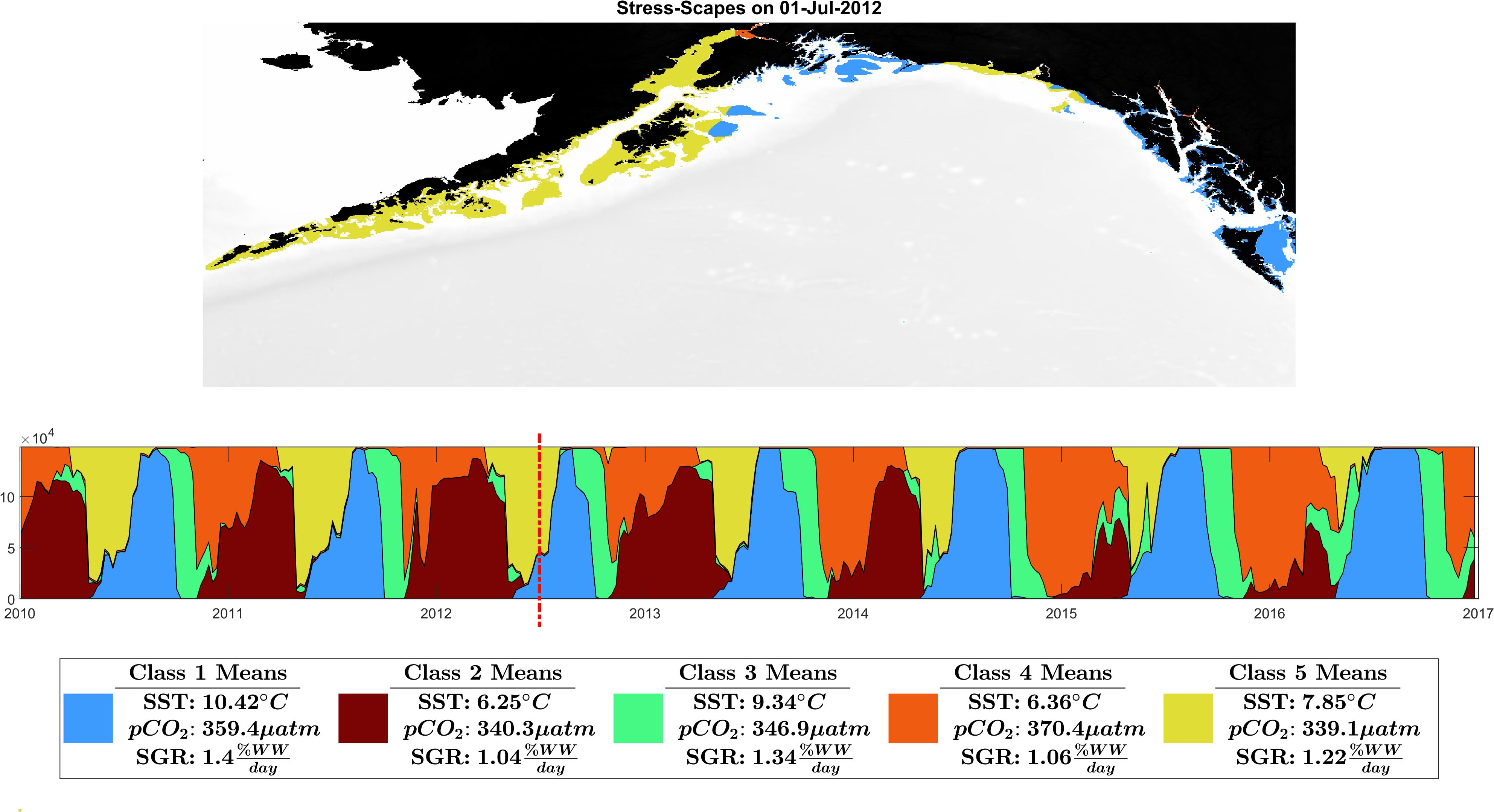
Figure 3. Ribbon plot showing total Stress-scape class area per time slice. Each column represents a single slice through time of the entire GOA. A stress-scape dominates in time if it persists along the x-axis. For example, in 2016, stress-scape class 1 was spatiotemporally dominant. From 2014 to 2016 stress-scape classes 1 and 4 dominate for a longer period than in 2010–2013. Classes 1 and 4 are coincident with the anomalous elevated growth rates seen in the otoliths data. A seasonal and interannual pattern emerges in the incidence of each of the classes: The spatiotemporal footprint of classes 2 and 5 contract over time, supplanted and are replaced by expansions of classes 1 and 4. Class 2, a low SST class, that seems to be replaced by high pCO2 class 4. Class 5, the pre-heatwave summer class, is replaced by the high SST class 3, which starts appearing earlier and persisting each year during the heatwave. This ribbon plot can be viewed as a video alongside a map of the GOA in Supplementary Material: Appendix E and Supplementary Videos 1, 2.
In July, stress-scape class 5 (yellow) dominated the areas around Kodiak Island in 2010 and 2012, whereas stress-scape class 3 (green) expands both around Kodiak and within the greater GOA area during heatwave conditions in 2014, 2015, and 2016. In 2013, stress-scape class 3 and stress-scape class 5 were both present throughout the coastal GOA. Stress-scape class 3 was characterized by above average temperatures (10.42 ± 2.48°C), higher pCO2, concentrations (359.4 ± 33.66 μatm), and high juvenile Pacific Cod SGR (1.40 ± 0.16% Wet weight/day). In contrast, stress-scape class 5 was characterized by near average temperatures (7.85 ± 2.25°C), lower pCO2 concentrations (339.1 ± 36.41 μatm), and near average SGR (1.22 ± 0.23% Wet weight/day).
Sensitivity Analysis
The bivariate prSOM, trained on SST and pCO2 but excluding SGR, resolved four stress-scape classes for the coastal GOA. Table 3 describes the means and standard deviations for the SST and pCO2 values of each of the resolved classes used for the sensitivity analysis. By excluding the SGR variable, the dominant stress-scape for coastal Kodiak Island was reclassified to a new stress-scape class 1 (blue), which identifies a mean SST of 10.42 ± 2.48°C and mean pCO2 concentration of 359.4 ± 33.66 μatm across all years of analysis. Stress-scape class 1 of the bivariate model corresponds to stress-scape class 3 of the trivariate-model. Figure 4 shows the dominant yearly classes for the bivariate prSOM sensitivity analysis near coastal Kodiak Island during the first week of July 2010 and 2012–2016 and should be evaluated using Table 3. Stress-scape class 1 is distinguished from the other three classes with its higher-than-average SST and near average pCO2 concentrations. The bivariate prSOM groups the two higher temperature classes of the trivariate dynamic stress-scape together because it lacks the SGR variable necessary for distinguishing between these classes. In addition, the bivariate prSOM is unable to distinguish between heatwave conditions and more normal ocean conditions in the GOA, with stress-scape class 1 dominant across all six years of the analysis.
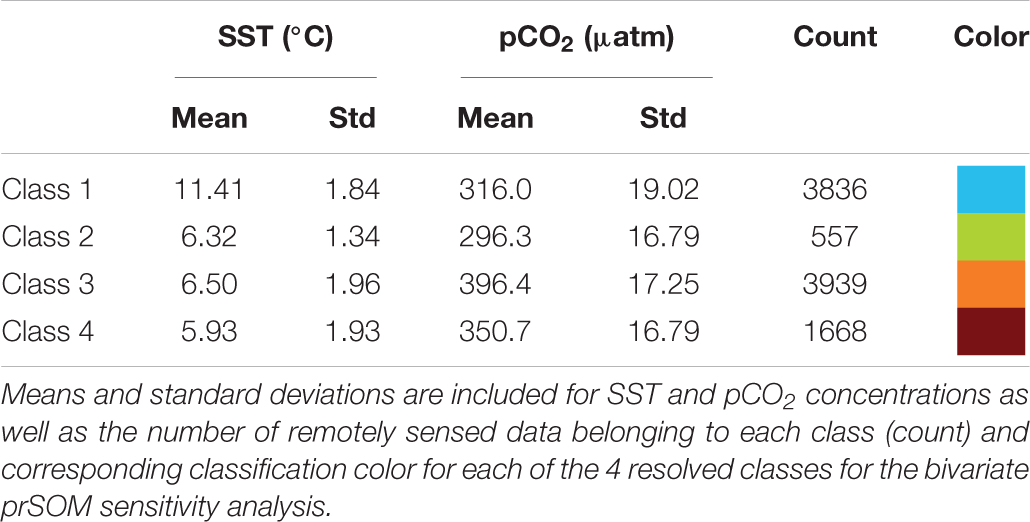
Table 3. Mean values for each of the 4 resolved stress-scapes that were classified to test the significance of the growth rate variable.
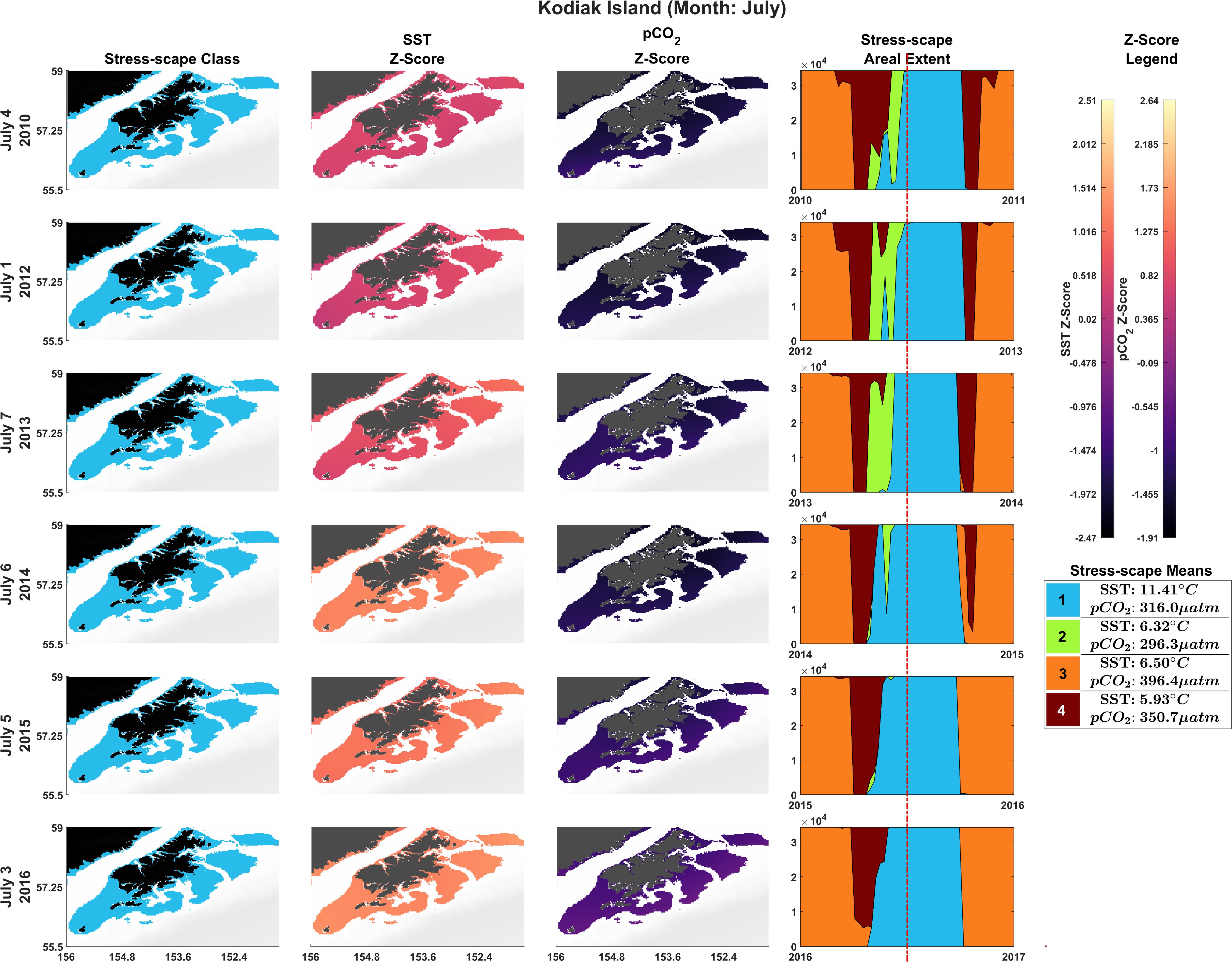
Figure 4. Dominant yearly classes for the bivariate prSOM sensitivity analysis near coastal Kodiak Island during the first week of July 2010 and 2012–2016. Normal ocean conditions are present in 2010–2013, and heatwave conditions are present in 2014–2016. Column 1 highlights the dominant stress-scape classes for coastal Kodiak Island. Column 2 is the z-score for SST, and Column 3 is the z-score for pCO2. The final column gives the areal extent of each stress-scape class. The red line indicates the time slice of the areal extent (see Supplementary Material: Appendix E and Supplementary Videos 1, 2).
Juvenile Nursery Growth
The dynamic stress-scape underestimated the observed juvenile Pacific Cod SGR in its classification schema for coastal Kodiak Island by 39–187% across all six years of the analysis (Figure 5). Mean July SGR from the dominant stress-scape for coastal Kodiak Island differed significantly from otolith-derived SGR for both the last 8 days of growth and the penultimate 8 days of growth (paired t-test; last 8 days: t5 = 6.72; P = 0.0011; penultimate 8 days: t5 = 6.94; P = 0.0009). Otolith-derived SGR were between 10 and 105% higher than the laboratory growth model across all six years for both the last 8 days of growth and the penultimate 8 days of growth (paired t-test; last 8 days: t5 = 4.69; P = 0.0053; penultimate 8 days: t5 = 5.39; P = 0.0029). In contrast, otolith-derived mm/d growth were between 13% and 79% lower than the laboratory growth model for both the last 8 days of growth and the penultimate 8 days of growth (paired t-test; last 8 days: t5 = 6.54; P = 0.0012; penultimate 8 days: t5 = 5.71; P = 0.0023). Across all years, field estimated growth rates were similar between heatwave conditions in 2014–2016 compared to more normal ocean conditions in 2010–2013 (1-way ANOVA; F1,102 = 0.75; P = 0.39), highlighting the difference in potential SGR predicted by the prSOM and the realized SGR observed in the field during heatwave conditions.
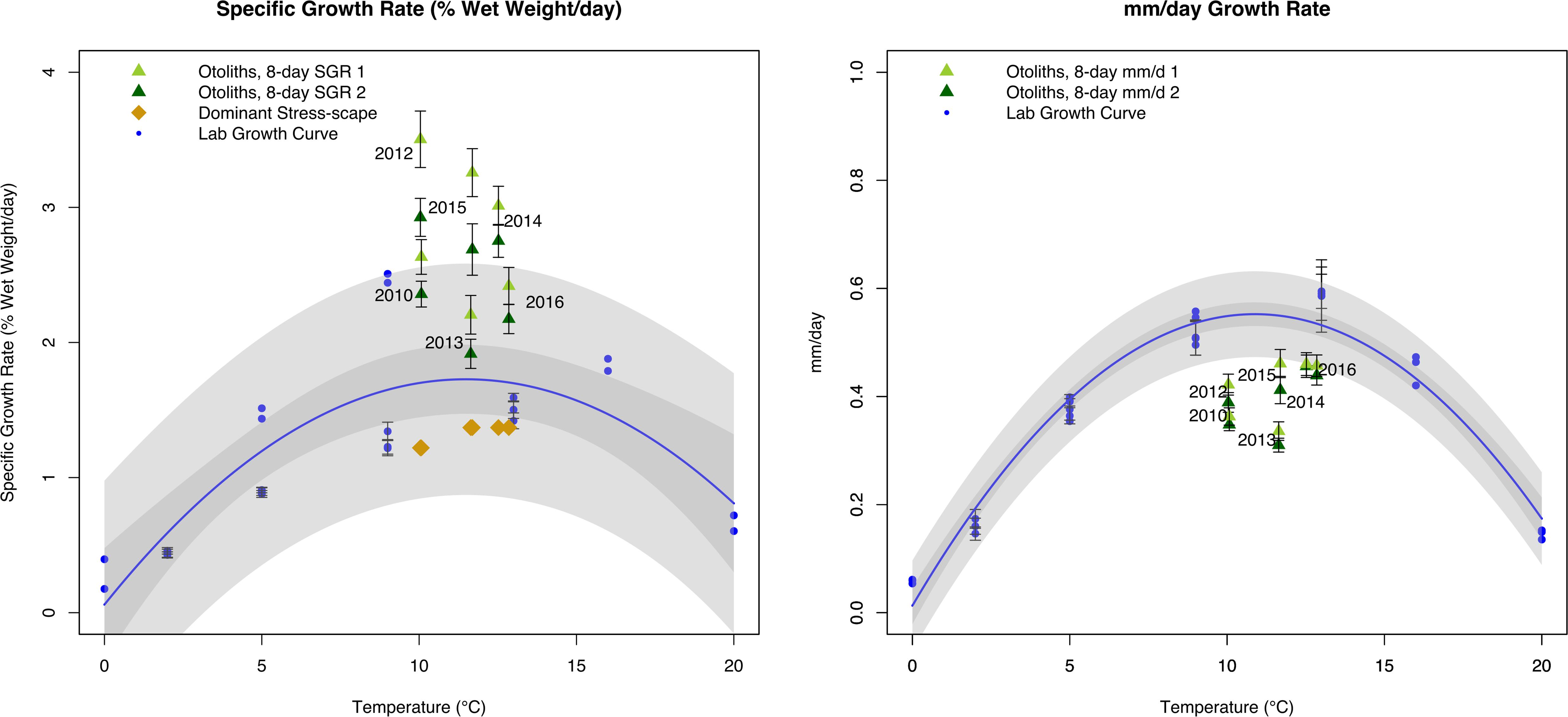
Figure 5. Comparison of juvenile Pacific Cod SGR (left panel;% wet weight/day) and mm/day growth rate (right panel) from individuals collected from Kodiak Island, AK in July 2010 and 2012–2016. Final 8 days of otolith growth prior to capture are represented by dark green triangles, and penultimate 8 days of otolith growth are represented by light green triangles. Laboratory models are represented by the blue growth curve and blue circles. Specific growth rate from the dominant stress-scapes in July near Kodiak Island are represented by gold diamonds. Error bars represent standard error. Gray polygons represent confidence and prediction intervals of the laboratory growth model.
Discussion
The application of seascape ecology methods to the evaluation of potential environmental stressors across a wide spatio-temporal scale on juvenile GOA Pacific Cod represents a new approach to visualize and synthesize the effects of extreme environmental variability on marine fisheries. Five dynamic stress-scapes were resolved from 2010 to 2016 that correspond to unique environmental conditions and growth responses of juvenile Pacific Cod. Stress-scape identity and spatial extent changed as a function of time, capturing unique conditions and responses during the 2014–2016 marine heatwave compared to the previous cooler conditions. Stress-scapes captured non-linear biological responses to the environment: when SGR was included as a variable (in addition to SST and pCO2) there was a single stress-scape that dominated during heatwave years that did not dominate prior to heatwave years. This dominant stress-scape corresponds to observed elevated growth rates from the otolith data from Kodiak Island. Even though SGR is a function of SST, including fish growth as a variable in the stress-scape captures patterns of growth that are comparable to those derived from otoliths in situ.
Output from the stress-scape visualization indicated that juvenile Pacific Cod experienced moderately higher potential growth during heatwave years compared to more normal environmental conditions. Accelerated growth is a common bioenergetic response of teleost fishes to warmer ocean conditions, with increased temperatures contributing to increased metabolism and faster growth as long as caloric and oxygen requirements are adequately met, until an upper limit above which growth will begin to decline (e.g., Beauchamp, 2009; Thalmann et al., 2020). These results suggest that warmer temperatures during the juvenile life stage led to faster growth than would be expected during normal ocean conditions. However, in contrast with these observed potential growth rates, Pacific Cod abundance was anomalously low in the GOA during marine heatwave conditions. Pacific Cod, like many stenothermic fish species, are likely to experience life stage-specific thermal bottlenecks, especially in the embryonic and adult spawner stages where the fish may be particularly vulnerable to environmental variability (Dahlke et al., 2020). It has been shown that Pacific Cod hatch success is strongly correlated with temperature, with hatch success most optimal at temperatures between 3 and 6°C (Laurel and Rogers, 2020). During the 2014–2016 marine heatwave, optimal spawning habitat was restricted to shallower regions and earlier times of the year, and this decline in suitable spawning habitat may have led to widespread reductions in reproductive output. Those individuals that survived the thermal bottleneck at the embryonic life stage may have experienced higher growth rates during the larval and juvenile life stages in warmer conditions, especially if prey was available and “matched” in time and space (Laurel et al., 2021). Additionally, juvenile Pacific Cod collected in Kodiak nurseries were two to four months old by July and the product of selective mortality. In poor survival years, such as 2015, slower growing or smaller fish can experience higher rates of mortality, leaving only the faster growing fish in the population (Litvak and Leggett, 1992; Sogard, 1997). Thus, the results from the stress-scape visualization likely do not capture the full impact of the 2014–2016 marine heatwave on Pacific Cod simply because it captures only a snapshot of the entire Pacific Cod life history, but the approach highlights the spatial and temporal extent of anomalous and potentially stressful conditions. With the addition of information on prey quantity and quality and pCO2 and temperature data throughout the water column, a more comprehensive exploration of the effects of the heatwave throughout the life history of Pacific Cod, and potentially other species, would be possible.
Otolith-derived SGR rates for field-collected juvenile Pacific Cod were between 39 and 187% higher than those predicted by the dominant stress-scape class for coastal Kodiak Island across most years of the analysis. This pattern highlights a potential underestimation of the stress-scape machine learning algorithm in predicting juvenile Pacific Cod growth in the field. The most parsimonious explanation for this result is that the temperature data used in the model did not accurately reflect shallow nursery conditions that often exceed 15°C in summer around Kodiak Island (Laurel et al., 2012). However, the laboratory model for SGR underestimated otolith-derived SGR across most years, while the laboratory model for mm/d growth overestimated otolith-derived growth. Growth in mass and growth in length are related but can reflect differences in energy allocation. For example, there is some evidence that seasonality can influence fish growth in the field, with fish adding more length earlier in the season when conditions are favorable for feeding, and then adding more mass later in the season to better prepare for winter conditions (e.g., Hurst and Conover, 2003; Hurst, 2007). Field growth may also exceed laboratory-based growth models due to a number of in situ processes, including higher food quality in the field (e.g., Mazur et al., 2007), favorable conditions in nearshore nursery habitat (e.g., Beck et al., 2001; Hinckley et al., 2019), and the effects of seasonality on growth rates, with juveniles feeding more vigorously in summer months to prepare for winter (e.g., Schwalme and Chouinard, 1999). In 2014, 2015, and 2012, two heatwave influenced years and a cool ocean year, respectively, juvenile Pacific Cod exhibited SGR in the field that were modestly higher than predicted from the laboratory temperature-dependent growth rate model. We note that the 2012 cohort of Pacific Cod was the strongest on record in the GOA since 1977, and growth was likely elevated in response to particularly favorable ocean conditions in that year and the production of multiple cohorts of juveniles (Hinckley et al., 2019). In the unfavorable ocean conditions occurring during the marine heatwave event in 2014 and 2015, only the fastest growing individuals were likely to survive to the juvenile life history stage, inflating the observed growth response of the survivors (e.g., Ricker, 1969; Litvak and Leggett, 1992; Moss et al., 2005). The variability between observed growth rates in the field and classified growth rates in the stress-scape suggests that future iterations of the stress-scape may benefit from added parameters related to selective mortality, foraging conditions, and additional environmental parameters related to ocean conditions.
In this analysis, we assume that the effects of temperature and pCO2 on Pacific Cod growth are additive. We do not account for potential interaction between these two environmental variables as further studies are needed to elucidate the interactive effects between warming and ocean acidification on the growth of Pacific Cod early life stages. We acknowledge that the potential for interactive effects between temperature and pCO2 on juvenile Pacific Cod growth may increase uncertainty in stress-scape classification and contribute to the deviation between Pacific Cod growth observed in the field compared to growth classified by the stress-scape. In general, early life stages of marine organisms tend to exhibit an increased sensitivity to the effects of ocean acidification in the presence of elevated water temperatures due to a heightened stress response (Harvey et al., 2013; Kroeker et al., 2013, 2014). However, fishes tend to be less sensitive than marine invertebrates to increased carbon dioxide in the water column even in the face of elevated temperature conditions due to their increased metabolism (e.g., Enzor et al., 2017), highly developed acid/base regulatory systems (e.g., Melzner et al., 2009), and potential for energy reallocation (e.g., Laubenstein et al., 2018). Studies examining the effects of elevated carbon dioxide concentrations on the early life stages of Alaskan gadids (including Walleye Pollock, a congener of Pacific Cod that shares a similar growth response at the juvenile stage), show limited impacts to growth response (Hurst et al., 2012, 2019). Therefore, we expect interactive effects of temperature and ocean acidification on SGR are likely minimal for juvenile Pacific Cod.
While previous dynamic classifications of the ocean include lower trophic level responses to environmental forcing (e.g., chl-a or carbon; Kavanaugh et al., 2014, 2018), the incorporation of fish physiology represents a notable revision and test of non-linear responses of higher trophic levels. Furthermore, our sensitivity analyses suggests that exclusion of biological responses results in an overly simple classification model. The bivariate prSOM (without growth rate) exhibited fewer output stress-scape classes compared to the full model and grouped several output classes together. In addition, the bivariate prSOM was unable to distinguish between heatwave conditions in 2014–2016 and more normal conditions in 2010–2013, with the dominant class remaining the same across all six years. Due to the quadratic relationship of Pacific Cod growth to ocean temperature (Hurst et al., 2010; Laurel et al., 2016), output classes in the full dynamic stress-scape model were able to successfully distinguish between similar growth rates that occurred in response to two different temperature ranges by classifying them into two separate output classes (classes 1 & 2). By including the biologically relevant growth response into the stress-scape, we could distinguish temperatures that were high enough to negatively impact growth rate from temperatures that were conducive to ideal growth. The utility of dynamic stress-scapes in capturing non-linear growth patterns could be expanded to include more complex biological patterns as indicators of changing ocean conditions, such as those associated with prey quality and quantity (e.g., Daly et al., 2017), predation (e.g., Fennie et al., 2020), or metabolic rate (e.g., Chung et al., 2019).
Machine learning models show promise in classifying marine heatwave events because they can generalize to regions of the ocean where there is limited data by being trained on more data-rich regions with similar conditions and then using the model to detect heatwave conditions. While there are several recent, well-received approaches to classifying marine heatwave events (e.g., Hobday et al., 2016, 2018; Oliver et al., 2018), many of these approaches exclude biological response in their classification schema for marine heatwaves. Our dynamic stress-scape classification of the GOA, which uses sea-surface measurements, successfully distinguished between marine heatwave conditions in 2014–2016 and more historically normal ocean conditions in 2010–2013. In general, stress-scape classes that corresponded to heatwave conditions exhibited higher SST, moderate pCO2 concentrations, and elevated juvenile Pacific Cod SGR. Stress-scapes that corresponded to more normal ocean conditions exhibited moderate SST, lower pCO2 concentrations, and moderate SGR. Dynamic stress-scapes effectively present the biological footprint of remotely sensed oceanographic data by characterizing spatio-temporal changes in the modeled SGR of juvenile Pacific Cod. The stress-scape framework shows promise in for merging spatio-temporal data and biological models to understand how heatwave conditions across a wide spatial and temporal range might affect living marine resources.
Our methods are not without limitations. The prSOM algorithm was chosen for this analysis because its topology preserving nature and its sensitivity to non-linear data. However, the prSOM implementation does not use k-fold cross validation to avoid overfitting, which would improve the classification results. Future efforts would include t-distributed stochastic neighbor embedding (t-SNE; Maatan and Hinton, 2008), which provides a well-defined “optimal solution,” lends itself to visualization of higher dimensional data (e.g., >3) and thus inclusion of additional in juvenile Pacific Cod fitness variables. Additionally, HAC can have issues when the geometry of the data is not flat (as is the case with the relationship between sea-surface temperature and growth rate). The DBSCAN (Ester et al., 1996) and OPTICS (Ankerst et al., 1999) algorithms could replace Hierarchical Agglomerative Clustering and be used in conjunction with a prSOM or t-SNE machine learning algorithm to provide a better stress-scape classification that distinguishes regions where the temperature is warm enough to constrain growth from regions where the temperature is conducive to optimal growth. Our visual results are a preliminary effort to introduce a visual system. In future work we will consider incorporating immersive visualization techniques for visual exploration of the ocean and coordinated multi-view visualization on the different types and sources of data for decision making. Efforts to expand on visualization within the fisheries community suggest interest in developing fast, scalable and explorative tools for fisheries scientists to understand the relationship between ocean variables, marine resources, and the algorithms used to process data from these two domains (Hermann and Moore, 2009).
Finally, our temperature-dependent growth model does not incorporate factors like prey quality, prey quantity, ocean currents, or ocean conditions at depth. Individual Based Modeling (IBM) and Regional Ocean Modeling Systems (ROMS) incorporate many of these factors and can be used to generate a spatial footprint of fish dispersal (Stockhausen et al., 2019). Information on the dominant dispersal pathways of Pacific Cod early life stages from an IBM/ROMS model could be paired with the stress-scapes by developing the duration of residence in each stress-scape. On a related note, IBM/ROMS models could be used to improve the data sampling used to train the prSOM. Currently, remotely sensed data are sampled uniformly at random at each epoch, where a different random sample is used at each iteration, but the distribution is uniform. The IBM/ROMS models could be used to estimate the probability of a juvenile Pacific Cod encountering potentially stressful conditions at each ocean pixel. This distribution could be used to inform the sampling method at each epoch of the prSOM so that the training data is more likely to represent the environmental context of the juvenile Pacific Cod. Our stress-scape framework is not intended as a substitute for an IBM, and it does not offer a comprehensive reconstruction of juvenile Pacific Cod growth history in the GOA (but see Hinckley et al. (2019) for an IBM currently in place for GOA Pacific Cod). Rather, the stress-scape represents a new way to visualize changes in both environmental conditions and Pacific Cod growing conditions before and during a marine heatwave event. Pacific Cod otoliths are used in the context of this study to support and serve as a point of comparison to the stress-scape classification of SST, pCO2, and laboratory-derived juvenile Pacific Cod SGR.
In summary, stress-scapes offer three new and novel features relevant to the fields of marine resource management, ocean ecology and fisheries science. First, stress-scapes are based on both remotely sensed data and models that approximate biological response to remotely sensed environmental data. Second, machine learning models can generalize to regions of ocean where there is limited data by being trained on data that share similar conditions. Third, stress-scapes use machine learning algorithms that train on spatially relevant data, in this case based on temperature-growth models to approximate the growth rate of juvenile Pacific Cod. This produces an output that is potentially relevant for management in multiple fields and at multiple spatial and temporal scales. Environmentally, the stress-scapes are useful metrics that can benefit ecosystem-based and dynamic approaches to management of marine resources (Kavanaugh et al., 2016). From a management perspective, the biological relevance of the stress-scapes can be used to trace or even forecast potentially stressful conditions for a focus species. Downstream effects on socioeconomic conditions of resource users could also be identified by aligning the occurrence and longevity of stress-scape classes in space and time with economic indicators and extraction data. The process is extremely flexible and can handle large amounts of many types of time series data, furthering its utility.
Data Availability Statement
The raw data supporting the conclusions of this article will be made available by the authors, without undue reservation.
Ethics Statement
Ethical review and approval was not required for the animal study because the field work was conducted solely by NOAA’s Alaska Fisheries Science Center Laboratory in Newport, Oregon. Animal tissue, in this case otoliths, was provided to OSU several years after collection. This research was carried out in accordance with all applicable institutional and national guidelines at the time that the study was conducted; all work followed American Fisheries Society policies on the Guidelines for Use of Fishes in Research (https://fisheries.org/docs/policy_useoffishes.pdf) and AVMA (American Veterinary Medical Association) Guidelines on Euthanasia (olaw.nih.gov/sites/default/files/Euthanasia2007.pdf). There was no formal ethics review of this study because NOAA National Marine Fisheries Service does not have an Institutional Animal Care and Use Committee (IACUC) or an ethics approval processes for research on fishes and, at the time of these collection (2010–2016), OSU was not involved in the research.
Author Contributions
All authors conceived of and designed the project. JB, HT, and WK executed the research, analyzed the data, and wrote the manuscript with feedback from YZ, JM, BL, and MK. JB led the prSOM implementation, execution, analysis, and interpretation with input from YZ and MK. HT and BL compiled Pacific Cod growth data for incorporation into the prSOM. WK compiled environmental data for incorporation into the prSOM with assistance from MK. HT and JM completed otolith analyses and interpretation. BL provided fish samples.
Funding
This project was supported by the National Science Foundation Research Traineeship (NSF-NRT) award #1545188, Risk and Uncertainty Quantification and Communication in Marine Science and Policy. Research was also partially supported by the North Pacific Research Board grant no. 1903. JB, WK, and MK were supported by NASA awards 80NSSC18K0412 (Dynamic seascapes to support a biogeographic framework for a global marine biodiversity observing network) and 80NSSC20M0008 (Marine Biodiversity Observing Network in the Northern California Current: Understanding Patterns and Drivers of Biodiversity and Ecosystem Functioning from Plankton to Seascapes).
Conflict of Interest
The authors declare that the research was conducted in the absence of any commercial or financial relationships that could be construed as a potential conflict of interest.
Acknowledgments
We greatly appreciated feedback on the development and implementation on this project from Lorenzo Ciannelli and the rest of the NSF-NRT leadership team at Oregon State University: Flaxen Conway, Ana Spalding, Julia Jones, and Katherine Hoffman. We are grateful to Tom Hurst for providing data and guidance related to Pacific Cod laboratory growth curves and to Thomas Murphy for assistance with processing the juvenile Pacific Cod otolith data used in this project. This effort was supported by a NSF-funded Research Traineeship at Oregon State University, NSF Grant 1545188 (NRT-DESE: Risk and Uncertainty Quantification in Marine Science). HT, JM, and BL were partially supported by the North Pacific Research Board grant no. 1903. In addition, JB, WK, and MK were supported by NASA awards 80NSSC18K0412 (Dynamic seascapes to support a biogeographic framework for a global marine biodiversity observing network) and 80NSSC20M0008 (Marine Biodiversity Observing Network in the Northern California Current: Understanding Patterns and Drivers of Biodiversity and Ecosystem Functioning from Plankton to Seascapes).
Supplementary Material
The Supplementary Material for this article can be found online at: https://www.frontiersin.org/articles/10.3389/fmars.2021.656088/full#supplementary-material
Supplementary Video 1 is located here: https://figshare.com/articles/media/14356751 and Supplementary Video 2 is located here: https://figshare.com/articles/media/14356793.
References
Amaya, D. J., Miller, A. J., Xie, S. P., and Kosaka, Y. (2020). Physical drivers of the summer 2019 North Pacific Marine Heatwave. Nat. Commun. 11:1903. doi: 10.1038/s41467-020-15820-w
Ankerst, M., Breunig, M. M., Kriegel, H. P., and Sander, J. (1999). “OPTICS: ordering points to identify the clustering structure,” in Proceedings of the ACM SIGMOD’98 International Conference on Management of Data, Seattle, WA.
Anouar, F., Badran, F., and Thiria, S. (1998). Probabilistic self-organizing map and radial basis function networks. Neurocomputing 3, 83–96. doi: 10.1016/s0925-2312(98)00026-5
Barbeaux, S., Ferriss, B., Palsson, W., Shotwell, K., Spies, I., Wang, M., et al. (2020). “Assessment of the Pacific cod stock in the Gulf of Alaska,” in Stock Assessment and Fishery Evaluation Report for the Groundfish Resources of the Gulf of Alaska, Compiled by the Plan Team for the Groundfish Fisheries of the Gulf of Alaska, ed. North Pacific Fishery Management Council (Washington, DC: NOAA). Available online at: https://www.fisheries.noaa.gov/resource/data/2020-assessment-pacific-cod-stock-gulf-alaska (accessed January 2, 2021).
Barreto, G. A., and Aguayo, L. (2009). “Time series clustering for anomaly detection using competitive neural networks,” in Proceedings of the International Workshop on Self-Organizing Maps, Berlin.
Beauchamp, D. A. (2009). Bioenergetic ontogeny: linking climate and mass-specific feeding to life-cycle growth and survival of salmon. Am. Fish. Soc. Symp. 70, 1–19.
Beck, M. W., Heck, K. L., Able, K. W., Childers, D. L., Eggleston, D. B., Gillanders, B. M., et al. (2001). The identification, conservation, and management of estuarine and marine nurseries for fish and invertebrates. Bioscience 51, 633–641. doi: 10.1641/0006-3568(2001)051[0633:ticamo]2.0.co;2
Bond, N. A., Cronin, M. F., Freeland, H., and Mantua, N. J. (2015). Causes and impacts of the 2014 warm anomaly in the NE Pacific. Geophys. Res. Lett. 42, 3414–3420. doi: 10.1002/2015gl063306
Bopp, L., Resplandy, L., Orr, J. C., Doney, S. C., Dunne, J. P., Gehlen, M., et al. (2013). Multiple stressors of ocean ecosystems in the 21st century: projections with CMIP5 models. Biogeosciences 10, 6225–6245. doi: 10.5194/bg-10-6225-2013
Breece, M. W., Fox, D. A., Dunton, K. J., Frisk, M. G., Jordaan, A., and Oliver, M. J. (2016). Dynamic seascapes predict the marine occurrence of an endangered species: atlantic Sturgeon Acipenser oxyrinchus oxyrinchus. Methods Ecol. Evol. 7, 725–733. doi: 10.1111/2041-210X.12532
Campana, S. E., and Jones, C. M. (1992). “Analysis of otolith microstructure data,” in Otolith Microstructure Examination and Analysis, Vol. 117, eds D. K. Stevenson and S. E. Campana (London: Canadian Special Publication of Fisheries and Aquatic Sciences), 73–100.
Campana, S. E., and Thorrold, S. R. (2001). Otoliths, increments, and elements: keys to a comprehensive understanding of fish populations? Can. J. Fish. Aquat. Sci. 58, 30–38.
Chen, S., and Hu, C. (2019). Environmental controls of surface water pCO2 in different coastal environments: observations from marine buoys. Cont. Shelf Res. 183, 73–86. doi: 10.1016/j.csr.2019.06.007
Chung, M. T., Trueman, C. N., Godiksen, J. A., Holmstrup, M. E., and Gronkjaer, P. (2019). Field metabolic rates of teleost fishes are recorded in otolith carbonate. Commun. Biol. 2:24. doi: 10.1038/s42003-018-0266-5
Cripps, G., Flynn, K. J., and Lindeque, P. K. (2016). Ocean acidification affects the phyto-zoo plankton trophic transfer efficiency. PLoS One 11:e0151739. doi: 10.1371/journal.pone.0151739
Dahlke, F. T., Wohlrab, S., Butzin, M., and Poertner, H. O. (2020). Thermal bottlenecks in the life cycle define climate vulnerability of fish. Science 369, 65–70. doi: 10.1126/science.aaz3658
Daly, E. A., Brodeur, R. D., and Auth, T. D. (2017). Anomalous ocean conditions in 2015: impacts on spring Chinook salmon and their prey field. Mar. Ecol. Prog. Ser. 566, 169–182. doi: 10.3354/meps12021
Di Lorenzo, E., and Mantua, N. (2016). Multi-year persistence of the 2014/15 North Pacific marine heatwave. Nat. Clim. Chang. 6, 1042–1047. doi: 10.1038/nclimate3082
DiMaria, R. A., Miller, J. A., and Hurst, T. P. (2010). Temperature and growth effects on otolith elemental chemistry of larval Pacific cod, Gadus macrocephalus. Environ. Biol. Fish. 89, 453–462. doi: 10.1007/s10641-010-9665-2
Doyle, M. J., and Mier, K. L. (2016). Early life history pelagic exposure profiles of selected commercially important fish species of the Gulf of Alaska. Deep Sea Res. Part II Top. Stud. Oceanogr. 132, 162–193. doi: 10.1016/j.dsr2.2015.06.019
Enzor, L. A., Hunter, E. M., and Place, S. P. (2017). The effects of elevated temperature and ocean acidification on the metabolic pathways of notothenioid fish. Conserv. Physiol. 2017:cox019. doi: 10.1093/conphys/cox019
Ester, M., Kriegel, H. P., Sander, J., and Xu, X. (1996). “A density-based algorithm for discovering clusters in large spatial databases with noise,” in Proceedings of the 2nd International Conference on Knowledge Discovery and Data Mining, (Portland, OR: AAAI Press), 226–231.
Evans, W., Mathis, J. T., and Cross, J. N. (2014). Calcium carbonate corrosivity in an Alaskan inland sea. Biogeosciences 11, 365–379. doi: 10.5194/bg-11-365-2014
Fabry, V. J., McClintock, J. B., Mathis, J. T., and Grebmeier, J. M. (2009). Ocean acidification at high latitudes: the bellwether. Oceanography 22, 160–171. doi: 10.5670/oceanog.2009.105
Feely, R. A., Sabine, C. L., Lee, K., Berelson, W., Kleypas, J., Fabry, V. J., et al. (2004). Impact of anthropogenic CO2 on the CaCO3 system in the oceans. Science 305, 362–366. doi: 10.1126/science.1097329
Fennie, H. W., Sponaugle, S., Daly, E. A., and Brodeur, R. D. (2020). Prey tell: what quillback rockfish early life history traits reveal about their survival in encounters with juvenile coho salmon. Mar. Ecol. Prog. Ser. 650, 7–18. doi: 10.3354/meps13300
Finn, R. N., Ronnestad, I., van der Meeren, T., and Fyhn, H. J. (2002). Fuel and metabolic scaling during the early life stages of Atlantic cod Gadus morhua. Mar. Ecol. Prog. Ser. 243, 217–234. doi: 10.3354/meps243217
Francis, R. I. C. C. (1990). Back-calculation of fish length: a critical review. J. Fish. Biol. 36, 883–902. doi: 10.1111/j.1095-8649.1990.tb05636.x
GEBCO Bathymetric Compilation Group 2020 (2020). The GEBCO_2020 Grid - a Continuous Terrain Model of the Global Oceans and Land. Liverpool: British Oceanographic Data Centre, National Oceanography Centre, doi: 10.5285/a29c5465-b138-234d-e053-6c86abc040b9
Gregor, L., Lebehot, A. D., Kok, S., and Monteiro, P. M. S. (2019). Data from: Global Surface-Ocean Partial Pressure of Carbon Dioxide (pCO2) Estimates from a Machine Learning Ensemble: CSIR-ML6 v2019a (NCEI Accession 0206205). Washington, DC: NOAA.
Hales, B., Strutton, P. G., Saraceno, M., Letelier, R., Takahasi, T., Feely, R., et al. (2012). Satellite-based prediction of pCO2 in coastal waters of the eastern North Pacific. Prog. Oceanogr. 103, 1–15. doi: 10.1016/j.pocean.2012.03.001
Harvey, B. P., Gwynn-Jones, D., and Moore, P. J. (2013). Meta-an.alysis reveals complex marine biological responses to the interactive effects of ocean acidification and warming. Ecol. Evol. 3, 1016–1030. doi: 10.1002/ece3.516
Hermann, A. J., and Moore, C. W. (2009). “Visualization in fisheries oceanography: new approaches for the rapid exploration of coastal ecosystems,” in Computers in Fisheries Research, 2nd Edn, ed. M. E. Moksness (Dordrecht: Springer), 317–336. doi: 10.1007/978-1-4020-8636-6_10
Hermann, A. J., Hinckley, S., Megrey, B. A., and Napp, J. M. (2001). Applied and theoretical considerations for constructing spatially explicit individual-based models of marine larval fish that include multiple trophic levels. ICES J. Mar. Sci. 58, 1030–1041. doi: 10.1006/jmsc.2001.1087
Hinckley, S., Hermann, A. J., and Megrey, B. A. (1996). Development of a spatially explicit, individual-based model of marine fish early life history. Mar. Ecol. Prog. Ser. 139, 47–68.
Hinckley, S., Stockhausen, W. T., Coyle, K. O., Laurel, B. J., Gibson, G. A., Parada, C., et al. (2019). Connectivity between spawning and nursery areas for Pacific cod (Gadus macrocephalus) in the Gulf of Alaska. Deep Sea Res. Part II 165, 113–126. doi: 10.1016/j.dsr2.2019.05.007
Hobday, A. J., Alexander, L. V., Perkins, S. E., Smale, D. A., Straub, S. C., Oliver, E. C. J., et al. (2016). A hierarchical approach to defining marine heatwaves. Prog. Oceanogr. 141, 227–238. doi: 10.1016/j.pocean.2015.12.014
Hobday, A. J., Oliver, E. C. J., Sen Gupta, A., Benthuysen, J. A., Burrows, M. T., Donat, M. G., et al. (2018). Categorizing and naming marine heatwaves. Oceanography 31:205. doi: 10.5670/oceanog.2018.205
Holsman, K. K., Haynie, A. C., Hollowed, A. B., Reum, J. C. P., Aydin, K., Hermann, A. J., et al. (2020). Ecosystem-based fisheries management forestalls climate-driven collapse. Nat. Commun. 11:4579. doi: 10.1038/s4167-020-18300-3
Hurst, T. P. (2007). Causes and consequences of winter mortality in fishes. J. Fish. Biol. 71, 315–345. doi: 10.1111/j.1095-8649.2007.01596.x
Hurst, T. P., and Conover, D. O. (2003). Seasonal and interannual variation in the allometry of energy allocation in juvenile striped bass. Ecology 84, 3360–3369. doi: 10.1890/02-0562
Hurst, T. P., Copeman, L. A., Haines, S. A., Meredith, S. D., Daniels, K., and Hubbard, K. M. (2019). Elevated CO2 alters behavior, growth, and lipid composition of Pacific cod larvae. Mar. Environ. Res. 145, 52–65. doi: 10.1016/j.marenvres.2019.02.004
Hurst, T. P., Fernandez, E. R., Mathis, J. T., Miller, J. A., Stinson, C. M., and Ahgeak, E. F. (2012). Resiliency of juvenile walleye pollock to projected levels of ocean acidification. Aquat. Biol. 17, 247–259. doi: 10.3354/ab00483
Hurst, T. P., Laurel, B. J., and Ciannelli, L. (2010). Ontogenetic patterns and temperature-dependent growth rates in early life stages of Pacific Cod (Gadus macrocephalus). Fish. B NOAA 108, 382–392.
Irwin, A. J., and Oliver, M. J. (2009). Are ocean deserts getting larger? Geophys. Res. Lett. 2009:GL039883. doi: 10.1029/2009GL039883
Kavanaugh, M. T., Abdala, F. N., Ducklow, H., Glover, D., Fraser, W., Martinson, D., et al. (2015). Effect of continental shelf canyons on phytoplankton biomass and community composition along the western Antarctic Peninsula. Mar. Ecol. Prog. Ser. 524, 11–26. doi: 10.3354/meps11189
Kavanaugh, M. T., Church, M. J., Davis, C. O., Karl, D. M., Letelier, R. M., and Doney, S. C. (2018). ALOHA from the edge: reconciling three decades of in situ Eulerian observation and geographic variability in the North Pacific Subtropical Gyre. Front. Mar. Sci. 5:130. doi: 10.3389/fmars.2018.00130
Kavanaugh, M. T., Hales, B., Saraceno, M., Spitz, Y. H., White, A. E., and Letelier, R. M. (2014). Hierarchical and dynamic seascapes: a quantitative framework for scaling pelagic biogeochemistry and ecology. Prog. Oceanogr. 120, 291–304. doi: 10.1016/j.pocean.2013.10.013
Kavanaugh, M. T., Oliver, M. J., Chavez, F. P., Letelier, R. M., Muller-Karger, F., and Doney, S. C. (2016). Seascapes as a new vernacular for pelagic ocean monitoring, management and conservation. ICES J. Mar. Sci. 73, 1839–1850. doi: 10.1093/icesjms/fsw086
Klajbor, W. C. (2020). Management Applications of Remotely Sensed Dynamic Seascapes: Two Case Studies. Master’s thesis. Corvallis, OR: Oregon State University.
Koenigstein, S., Mark, F. C., Goessling-Reisemann, S., and Reuter, H. (2016). Modelling climate change impacts on marine fish populations: process-based integration of ocean warming, acidification and other environmental drivers. Fish and Fisheries 17, 972–1004.
Kroeker, K. J., Gaylord, B., Hill, T. M., Hosfelt, J. D., Miller, S. H., and Sanford, E. (2014). The role of temperature in determining species’ vulnerability to ocean acidification: a case study using Mytilus galloprovincialis. PLoS One 9:e100353. doi: 10.1371/journal.pone.0100353
Kroeker, K. J., Kordas, R. L., Crim, R., Hendriks, I. E., Ramajo, L., Singh, G. S., et al. (2013). Impacts of ocean acidification on marine organisms: quantifying sensitivities and interaction with warming. Glob. Chang. Biol. 19, 1884–1896.
Laubenstein, T. D., Rummer, J. L., Nicol, S., Parsons, D. M., Pether, S. M. J., Pope, S., et al. (2018). Correlated effects of ocean acidification and warming on behavioral and metabolic traits of a large pelagic fish. Diversity 10:35. doi: 10.3390/d10020035
Laurel, B., Hunsicker, M. E., Ciannelli, L., Hurst, T. P., Duffy-Anderson, J., O’Malley, R., et al. (2021). Regional warming exacerbates match/mismatch vulnerability for cod larvae in Alaska. Pro. Oceanogr. 193:102555.
Laurel, B., and Rogers, L. (2020). Loss of spawning habitat and prerecruits of Pacific Cod during a Gulf of Alaska heatwave. Can. J. Fish. Aquat. Sci. 77, 644–650. doi: 10.1139/cjfas-2019-0238
Laurel, B. J., Copeman, L. A., and Parrish, C. C. (2012). Role of Temperature on lipid/fatty acid composition in Pacific cod (Gadus macrocephalus) eggs and unfed larvae. Mar. Biol. 159, 2025–2034. doi: 10.1007/s00227-012-1989-3
Laurel, B. J., Ryer, C. H., Knoth, B., and Stoner, A. W. (2009). Temporal and ontogenetic shifts in habitat use of juvenile Pacific cod (Gadus macrocephalus). J. Exp. Mar. Biol. Ecol. 377, 28–35. doi: 10.1016/j.jembe.2009.06.010
Laurel, B. J., Spencer, M., Iseri, P., and Copeman, L. A. (2016). Temperature-dependent growth and behavior of juvenile Arctic cod (Boreogadus saida) and co-occurring North Pacific gadids. Polar Biol. 39, 1127–1135.
Laurel, B. J., Stoner, A. W., Ryer, C. H., Hurst, T. P., and Abookire, A. A. (2007). Comparative habitat associations in juvenile Pacific cod and other gadids using seines, baited cameras, and laboratory techniques. J. Exp. Mar. Biol. Ecol. 351, 42–55. doi: 10.1016/j.jembe.2007.06.005
Lebbah, M., Jaziri, R., Bennani, Y., and Chenot, J. H. (2015). Probabilistic self-organizing map for clustering and visualizing non-iid data. Int. J. Comput. Intell. Appl. 14:1550007. doi: 10.1142/S1469026815500078
Leising, A., Schroeder, I. D., Bograd, S. J., Abell, J., Durazo, R., Gaxiola, G., et al. (2015). State of the California current 2014-15: impacts of the warm-water “Blob”. Cal. Coop. Ocean. Fish. 56, 31–68.
Lischka, S., Buedenbender, J., Boxhammer, T., and Riebesell, U. (2011). Impact of ocean acidification and elevated temperatures on early juveniles of the polar shelled pteropod Limacina helicina: mortality, shell, degradation, and shell growth. Biogeosciences. 8, 919–932. doi: 10.5194/bg-8-919-2011
Litvak, M. K., and Leggett, W. C. (1992). Age and size-selective predation on larval fishes: the bigger-is-better hypothesis revisited. Mar. Ecol. Prog. Ser. 81, 13–24.
Mathis, J. T., Cooley, S. R., Lucey, N., Colt, S., Ekstrom, J., Hurst, T., et al. (2015). Ocean acidification risk assessment for Alaska’s fishery sector. Prog. Oceanogr. 136, 71–91. doi: 10.1016/j.pocean.2014.07.001
Mathis, J. T., Cross, J. N., and Bates, N. R. (2011). The role of ocean acidification in systemic carbonate mineral suppression in the Bering Sea. Geophys. Res. Lett. 38:L19602.
Mazur, M. M., Wilson, M. T., Dougherty, A. B., Buchheister, A., and Beauchamp, D. A. (2007). Temperature and prey quality effects on growth of juvenile walleye pollock Theragra chalcogramma (Pallas): a spatially explicit bioenergetics approach. J. Fish Biol. 70, 816–836. doi: 10.1111/j.1095-8649.2007.01344.x
Melzner, F., Gutowska, M. A., Langenbuch, M., Dupont, S., Lucassen, M., Thorndyke, M. C., et al. (2009). Physiological basis for high CO2 tolerance in marine ectothermic animals: pre-adaptation through lifestyle and ontogeny? Biogeosciences 6, 2313–2331. doi: 10.5194/bg-6-2313-2009
Miller, J. A., DiMaria, R. A., and Hurst, T. P. (2016). Patterns of larval source distribution and mixing in early life stages of Pacific cod (Gadus macrocephalus) in the southeastern Bering Sea. Deep Sea Res. Part II Top. Stud. Oceanogr. 134, 270–282. doi: 10.1016/j.dsr2.2014.12.012
Montes, E., Djurhuus, A., Muller-Karger, F. E., Otis, D., Kelble, C. R., and Kavanaugh, M. T. (2020). Dynamic satellite seascapes as a biogeographic framework for understanding phytoplankton assemblages in the Florida Keys National Marine Sanctuary, United States. Front. Mar. Sci. 7: 575.
Moss, J. H., Beauchamp, D. A., Cross, A. D., Myers, K. W., Farley, E. V., Murphy, J. M., et al. (2005). Evidence for size-selective mortality after the first summer of ocean growth by Pink Salmon. T. Am. Fish. Soc. 134, 1313–1322. doi: 10.1577/t05-054.1
Narimatsij, Y., Hattori, T., Ueda, Y., Matsuzaka, H., and Shiogaki, M. (2007). Somatic growth and otolith microstructure of larval and juvenile Pacific cod Gadus macrocephalus. Fish. Sci. 73, 1257–1264.
Oliver, E. C. J., Donat, M. G., Burrows, M. T., Moore, P. J., Smale, D. A., Alexander, L. V., et al. (2018). Longer and more frequent marine heatwaves over the past century. Nat. Commun. 9:1324. doi: 10.1038/s41467-018-03732-9
Pannella, G. (1971). Fish otoliths: daily growth layers and periodical patterns. Science 173, 1124–1127. doi: 10.1126/science.173.4002.1124
Piatt, J. F., Parrish, J. K., Renner, H. M., Schoen, S. K., Jones, T. T., Arimitsu, M. L., et al. (2020). Extreme mortality and reproductive failure of common murres resulting from the northeast Pacific marine heatwave of 2014-2016. PLoS One 15:e0226087. doi: 10.1371/journal.pone.0226087
Reygondeau, G., Longhurst, A., Martinez, E., Beaugrand, G., Antoine, D., and Maury, O. (2013). Dynamic biogeochemical provinces in the global ocean. Glob. Biogeochem. Cy. 27, 1046–1058. doi: 10.1002/gbc.20089
Reynolds, R. W., Rayner, N. A., Smith, T. M., Stokes, D. C., and Wang, W. (2002). Data from: an improved in situ and satellite SST analysis for climate. J. Clim. 15, 1609–1625. doi: 10.1175/1520-0442(2002)015<1609:aiisas>2.0.co;2
Richardson, A. J., Risien, C., and Shillington, F. A. (2003). Using self-organizing maps to identify patterns in satellite imagery. Prog. Oceanogr. 59, 223–239. doi: 10.1016/j.pocean.2003.07.006
Ricker, W. E. (1969). Effects of size-selective mortality and sampling bias on estimates of growth, mortality, production, and yield. Can. J. Fish. Aquat. Sci. 26, 479–541.
Rogers, L. A., Wilson, M. T., Duffy-Anderson, J. T., Kimmel, D. G., and Lamb, J. F. (2020). Pollock and “the Blob”: impacts of a marine heatwave on walleye pollock early life stages. Fish. Oceanogr. 30, 142–158. doi: 10.1111/fog.12508
Saraceno, M., Provost, C., and Lebbah, M. (2006). Biophysical regions identification using an artificial neuronal network: a case study in the South Western Atlantic. Adv. Space Res. 37, 793–805. doi: 10.1016/j.asr.2005.11.005
Schwalme, K., and Chouinard, G. A. (1999). Seasonal dynamics in feeding, organ weights, and reproductive maturation of Atlantic cod (Gadus morhua) in the southern Gulf of St. Lawrence. ICES J. Mar. Sci. 56, 303–319. doi: 10.1006/jmsc.1999.0458
Sogard, S. M. (1997). Size-selective mortality in the juvenile stage of teleost fishes: a review. Bull. Mar. Sci. 60, 1129–1157.
Stockhausen, W. T., Coyle, K. O., Hermann, A. J., Blood, D., Doyle, M. J., Gibson, L., et al. (2019). Running the gauntlet: connectivity between natal and nursery areas for Pacific Ocean perch (Sebastes alutus) in the Gulf of Alaska, as inferred from a biophysical individual-based model. Deep Sea Res. Part II Top. Stud. Oceanogr. 165, 74–88. doi: 10.1016/j.dsr2.2018.05.016
Suryan, R. M., Arimitsu, M. L., Coletti, H. A., Hopcroft, R. R., Lindeberg, M. R., Barbeaux, S. J., et al. (2021). Ecosystem response persists after a prolonged marine heatwave. Sci. Rep. 11:6235. doi: 10.1038/s41598-021-83818-5
Thalmann, H. L., Daly, E. A., and Brodeur, R. D. (2020). Two anomalously warm years in the northern California current: impacts on early marine Steelhead diet composition, morphology, and potential survival. Trans. Am. Fish. Soc. 149, 369–382. doi: 10.1002/tafs.10244
Tibshirani, R., Walther, G., and Hastie, T. (2001). Estimating the number of clusters in a data set via the gap statistic. J. R. Stat. Soc. Ser. B Stat. Methodol. 63, 411–423. doi: 10.1111/1467-9868.00293
Werner, R. G. (2002). “Habitat requirements,” in Fishery Science: the Unique Contributions of Early Life Stages, eds L. A. Fuiman and R. G. Werner (Ames, IA: Blackwell Science), 161–182.
Williams, C. R., Dittman, A. H., McElhany, P., Shallin Busch, D., Maher, M., Bammler, O., et al. (2019). Elevated CO2 impairs olfactory-mediated neural and behavioral responses and gene expression in ocean-phase coho salmon (Oncorhynchus kisutch). Glob. Chang. Biol. 25, 963–977. doi: 10.1111/gcb.14532
Keywords: stress-scapes, Gulf of Alaska, machine learning, visualization, Pacific cod, multiple environmental stressors
Citation: Blaisdell J, Thalmann HL, Klajbor W, Zhang Y, Miller JA, Laurel BJ and Kavanaugh MT (2021) A Dynamic Stress-Scape Framework to Evaluate Potential Effects of Multiple Environmental Stressors on Gulf of Alaska Juvenile Pacific Cod. Front. Mar. Sci. 8:656088. doi: 10.3389/fmars.2021.656088
Received: 20 January 2021; Accepted: 12 April 2021;
Published: 12 May 2021.
Edited by:
Kelly Ortega-Cisneros, University of Cape Town, South AfricaReviewed by:
Matthew Siskey, University of Washington, United StatesCaihong Fu, Department of Fisheries and Oceans, Canada
Copyright © 2021 Blaisdell, Thalmann, Klajbor, Zhang, Miller, Laurel and Kavanaugh. This is an open-access article distributed under the terms of the Creative Commons Attribution License (CC BY). The use, distribution or reproduction in other forums is permitted, provided the original author(s) and the copyright owner(s) are credited and that the original publication in this journal is cited, in accordance with accepted academic practice. No use, distribution or reproduction is permitted which does not comply with these terms.
*Correspondence: Josiah Blaisdell, blaisdjo@oregonstate.edu