- 1School of Earth, Ocean and Climate Sciences, Indian Institute of Technology Bhubaneswar, Arugul, India
- 2Department of Earth and Climate Science, Indian Institute of Science Education and Research, Pune, India
The role of COVID-19 pandemic lockdown in improving air quality was reported extensively for land regions globally. However, limited studies have explored these over oceanic areas close to high anthropogenic activities and emissions. The Bay of Bengal (BoB) basin is one such region adjacent to the highly populated South Asian region. We find that Aerosol Optical Depth (AOD) over the BoB declined by as much as 0.1 or 30% during the peak lockdown of April 2020 compared to long-term climatology during 2003–2019. Simultaneously, the sea surface temperature (SST) rose by 0.5–1.5°C over the central and north-western parts of the BoB with an average increase of 0.83°C. We show that up to 30% of this observed warming is attributable to reduced atmospheric aerosols. The study highlights the importance of anthropogenic emissions reduction due to COVID lockdown on short-term changes to SST over ocean basins with implications to regional weather.
Introduction
COVID-19 was declared a pandemic by the World Health Organization (WHO) in March 2020, which subsequently spread to most countries globally, significantly changing their socio-economic landscapes (Buheji et al., 2020; Martin et al., 2020). Nations across the globe have taken various measures like social distancing, travel restrictions, and complete lockdowns of different durations to slow its spread. Numerous studies have documented multiple impacts of these lockdowns and the associated decrease in anthropogenic emissions in improving the air quality (e.g., Baldasano, 2020; Bauwens et al., 2020; Collivignarelli et al., 2020; Kanniah et al., 2020; Li et al., 2020; Mahato et al., 2020; Muhammad et al., 2020; Otmani et al., 2020; Pandey and Vinoj, 2020; Stratoulias and Nuthammachot, 2020). It is well known that atmospheric aerosols directly affect the surface radiation budget by absorbing and scattering the incoming solar radiation. They also modify the cloud microphysical properties (by acting as cloud condensation nuclei and ice nuclei, changing the size of cloud droplets and hence its albedo) (Twomey, 1977; Yu et al., 2006; Andreae and Rosenfeld, 2008; Boucher et al., 2013). In addition, the absorbing aerosols also change the atmosphere by altering the vertical temperature structure (Johnson et al., 2004; Koch and Del Genio, 2010), thereby affecting the atmospheric convection, droplet evaporation, and the overall cloud cover (called the semi-direct effect, Hansen et al., 1997). All these processes modulate the surface reaching solar radiation (Satheesh et al., 2006; Vinoj et al., 2010) and potentially modulate the surface temperature (Rajeev et al., 2008; Dave et al., 2020).
The Indian Ocean rim countries (especially in South Asia) have undergone significant economic growth in recent times with increased emissions and thus atmospheric aerosol loading (Moorthy et al., 2013). The continental outflow, especially from the heavily aerosol laden Indo-Gangetic Plains (IGP), dominates the aerosol loading over the BoB region (Satheesh et al., 2001, 2006; Vinoj et al., 2004, 2010; Moorthy et al., 2009; Nair et al., 2014; Tiwari et al., 2016) during pre-monsoon (March to May) and the winter (December to February) season. Thus BoB is heavily affected by fine anthropogenic particulates as indicated by the high Angstrom Exponent (AE) (see Figure 1) compared to the Arabian Sea (Vinoj and Satheesh, 2003). A study done by Kedia and Ramachandran (2008) found that the anthropogenic contribution to AOD over the Bay of Bengal could be as high as 68–75%. The peak lockdown due to the pandemic occurred during April, coinciding with the period of high aerosol loading over the Indo-Gangetic Plains and hence the BoB (Satheesh et al., 2006; Vinoj and Pandey, 2016).
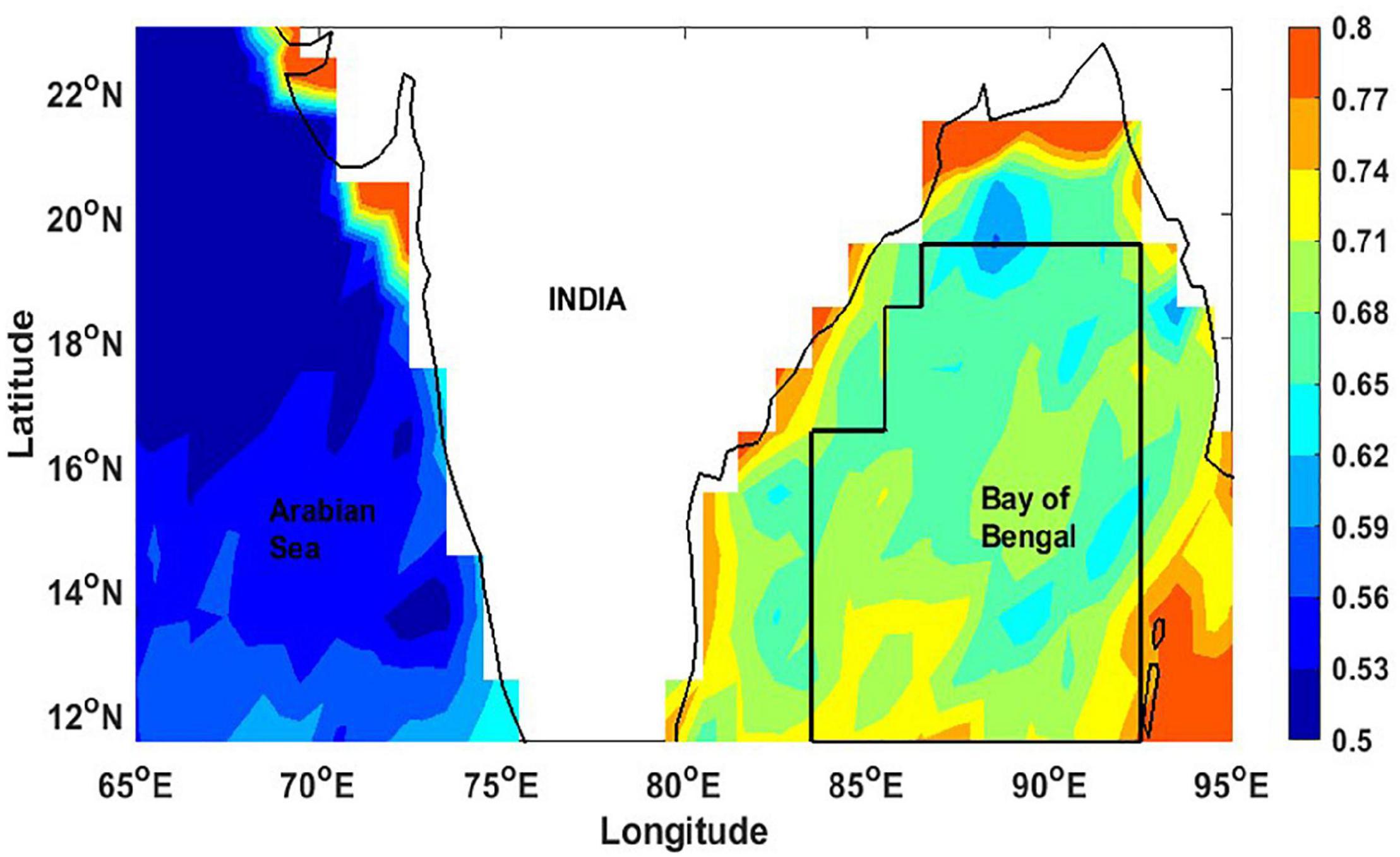
Figure 1. The monthly mean Angstrom Exponent (AE) climatology over the oceanic regions around India during the period 2003–2018 for April.
The sea surface temperature (SST) is an essential parameter for weather and plays a crucial role in regulating the Earth’s climate. Previous studies using in situ and satellite-based measurements show that the BoB aerosols exert a radiative forcing of −20 to −30 W m–2 at the ocean surface (Satheesh, 2002; Dey et al., 2004; Vinoj et al., 2004; Satheesh et al., 2010). An observational study carried out over the Equatorial Indian Ocean showed that aerosols due to Indonesian forest fire exert a surface radiative forcing of −46 W m–2, thereby cooling the ocean surface by 1°C (Rajeev et al., 2008). Similar effects due to aerosol loading and SST were also documented by other studies (Cheng et al., 2005; Dwyer et al., 2010; Patil et al., 2019; Diao et al., 2021; Luo et al., 2021). However, most of these are based on model simulations and hence lack observational constraints.
The COVID-19 lockdown halted activities across large sections of the society by confining the population to their homes resulting in a significant decline in atmospheric anthropogenic aerosol loading (Pandey et al., 2016; Kanniah et al., 2020; Ranjan et al., 2020) as much as 40–60% over the South Asian region. The reduction in emissions and their influence extended far beyond their sources, affecting the air quality over places afar, including oceanic regions downwind such as the Bay of Bengal (BoB) (Pandey and Vinoj, 2020). Despite a good understanding of large-scale, long-range transport of aerosols to BoB from landmass around it (Vinoj et al., 2004; Kumar et al., 2008; Kulshrestha and Kumar, 2014), no study has attempted to understand the effect of aerosols on the SST over this region. Similar areas affected by long-range transport also exist over the South China Sea, north-west Pacific, equatorial Eastern, and the North Atlantic Ocean (Prospero, 1990; Lin et al., 2007; Zhu et al., 2020). Several reasons make such studies unviable. One primary reason is the lack of high-quality aerosol and SST observations from satellites. However, the availability of high-quality datasets since 2000 has made it possible to explore such cases in detail. In addition, the highly dynamic nature and the role of multiple factors affecting SSTs make it difficult to explore these relationships, if any.
Some of the factors affecting SSTs are solar insolation, warm/cold water advection, and other marine processes, namely ocean currents, upwelling/downwelling phenomena, and eddies (Leeuwenburgh and Stammer, 2001; Shinoda, 2005; Krishna, 2008; Mandal et al., 2019; Buckley et al., 2020). In addition, the BoB also receives a sizable freshwater influx from the rivers and undergoes near-surface ocean-atmosphere interactions, which alters the upper ocean processes (Parampil et al., 2010). Past studies have revealed that the circulation in the BoB is primarily wind and buoyancy-driven (Schott and McCreary, 2001; Schott et al., 2002; Liu and Alexander, 2007), which also modulates the surface and subsurface properties in the BoB. These multiple influences induce large variability in SST, with a monotonic decrease toward the South (Srivastava et al., 2018), coinciding with the spatial variability of aerosol loading (Satheesh et al., 2010), indicating a potential relationship.
The lockdown presented a unique opportunity to explore the effect of an unprecedented and substantial decline in aerosols over this region since the start of satellite measurements. This article quantifies the change to SST due to changes in aerosol loading over the Bay of Bengal during the peak pandemic lockdown period of April 2020 using long-term satellite datasets.
Data and Methods
The aerosol optical depth (AOD) data used in this study is obtained from the NASA satellite datasets archived at https://giovanni.gsfc.nasa.gov/giovanni/ for April from 2003 to 2020. All the variables are at a spatial resolution of 1° × 1°. Several investigators have used aerosol optical depth (AOD), a column-averaged measure, to study atmospheric particulate matter load (Pandey and Vinoj, 2020; Ranjan et al., 2020). The Moderate Resolution Imaging Spectroradiometer (MODIS) Terra/Aqua aerosol products are of high quality and validated by multiple studies over India’s land and oceanic regions (e.g., Vinoj et al., 2004, 2010; Jethva et al., 2007; Mhawish et al., 2017). The retrieval algorithms are updated periodically (Remer et al., 2005) to improve their accuracy. The latest version of combined MODIS Terra (MOD08_D3) and Aqua (MYD08_D3) collection 6 (Levy et al., 2013) level 3 products is used. The MODIS AOD is estimated in the following wavelengths: 0.47, 0.55, and 0.65 μm with an error of ±0.05 ± 15%τ (Kaufman and Tanré, 1998). In this study, AOD at 0.55 μm is used. The Angstrom Exponent (AE) product used in this study is estimated using 412 nm and 470 nm as the reference wavelengths. The latest collection (collection 6) (Platnick et al., 2017) product significantly reduces uncertainty. Unlike land, the ocean surface is spatially homogeneous, allowing coarser-resolution datasets for the analysis. In addition, level 3 datasets are used for research involving large spatial scales, thereby avoiding small scale heterogeneities, which is not of interest in this study. Observations from MODIS is widely utilized for aerosol and air quality research (Gupta et al., 2006, 2013; Pandey et al., 2016, 2017; Vinoj and Pandey, 2016; Mhawish et al., 2017; Jethva et al., 2018; Mukherjee et al., 2018; Rupakheti et al., 2019) over this region and also globally.
The sea surface temperature (SST), cloud fraction (CFR), and total column water vapor (TWV) is obtained from Atmospheric Infrared Sounder (AIRS) onboard NASA’s Aqua satellite. The AIRS is a hyperspectral atmospheric sounder with 2378 infrared channels and four visible/near-infrared channels providing daily long-term global observations (Chahine et al., 2006; Pagano et al., 2006). This study uses the level 3 daily products (AIRSX3STD V007) from the latest version of the datasets (Tian et al., 2020). Past studies have shown good agreement between AIRS and radiosonde data within mission-specific accuracy bounds (Kahn et al., 2008; Prasad and Singh., 2009; Milstein and Blackwell, 2016).
Quality Check and Screening
The MODIS aerosol retrievals ensure high fidelity in their products. They are considered the best available for long-term scientific studies due to their stringent quality control and cloud screening measures. In addition to their regular quality checks, we also carried out a few data reduction strategies for additional confidence. Sometimes, the satellites may report slightly higher aerosol optical depth due to sub-grid cloud contamination even though a significant portion of the grids may be cloud-free. This may lead to a slight increase in the AOD.
Similarly, bright clouds close to clear pixels may lead to stray additional light leaking into nearby pixels leading to reduced AOD. Therefore, all datasets are screened for outliers using percentile-based criteria (top and bottom 5th percentile datasets are removed) though errors are remote. Considering the high quality of MODIS products, this data reduction is not an essential requirement and does not impact the overall results. However, this is done for better confidence in the quantifications made in this study. Also, there is no specific reason for using the 5th percentile for data reduction.
In addition, most meteorological parameters have a distinct seasonality over this region. Therefore, to avoid correlations induced due to these seasonality’s, the anomalies/residuals are calculated by subtracting the weekly running mean (calculated using a 7-day window centered on the time point). This allowed the removal of seasonality (within the month) and long-term trend-induced co-variability between AOD and SST. This is an essential requirement as BoB has strong seasonality in both aerosol and sea surface temperature distribution, which can alter the relationship between the two.
Analysis Method
The changes in SSTs due to change in AOD were estimated using two different methods. (1) Using the raw SST and AOD using simple linear regression analysis, but only for clear sky conditions by including only data with CFR < 5% and the total column water vapor below the 20th percentile for each grid point. This is an additional check to avoid any effect due to clouds and water vapor. A linear regression analysis was done using data from all grid points over the whole BoB. The regression coefficients thus obtained provide an estimate of the change in SST as a function of AOD. (2) Using multiple linear regression analysis by using residuals of SST, AOD, TWC, and CFR. Here a regression model is developed at each grid point and is applied to estimate the change in SST only due to change in AOD at that specific grid. The statistical significance of the regression is calculated using the t-test.
Additional care was taken to use only grids (see Figure 1) at least 1° away from the coast (especially Northern Bay). This was essential to remove any effect of current-induced SST changes close to the coast and avoid any false relationships that may arise due to coastal processes such as upwelling and freshwater flux that may change SSTs (Krishna, 2008). In addition, aerosol retrieval errors are significantly high closer to the coast due to the land-ocean boundary (different algorithms used for the AOD retrievals) and the potential effects of sediment concentrations on the overall surface-atmosphere reflectance errors in aerosol retrievals from satellite sensors (Anderson et al., 2013).
Results and Discussion
Climatological SST and AOD Over the BoB
The climatological mean AOD and SST for April are shown in Figures 2A,D, respectively. These are the climatological spatial patterns expected over this region. The AOD shows a spatial gradient that declines from the North to the South. The high AOD is observed over the Northern BoB with values as high as 0.6–0.8. They become lower as one moves south by more than 50% (0.2–0.3) within a small distance. This is because the head Bay is affected more by the advection of aerosols from North India (especially the Indo-Gangetic Plains) and the coastal regions of India, Bangladesh, and Myanmar (Sumanth et al., 2004; Vinoj et al., 2004; Nair et al., 2009). The high population density and numerous industries over the region mean that emissions over these source regions are relatively high. Northwesterly winds during the period further act as conduits transporting these aerosols to the BoB (Lakshmi et al., 2017). Southern BoB is affected by the surrounding coastal regions and aerosols from the Arabian Sea, which is less affected by anthropogenic activities leading to lower AOD (Nair et al., 2009). One can also see a decrease in loading as we move further away from the coasts. Even in a climatological sense, the high AOD to the North matches with the lower SST below indicating an inverse relationship between AOD and SST. However, due to the possibility of cool freshwater influx from the rivers such as the Ganges and Brahmaputra during this period, it is impossible to argue that this reduced SST is solely caused by the atmospheric aerosols.
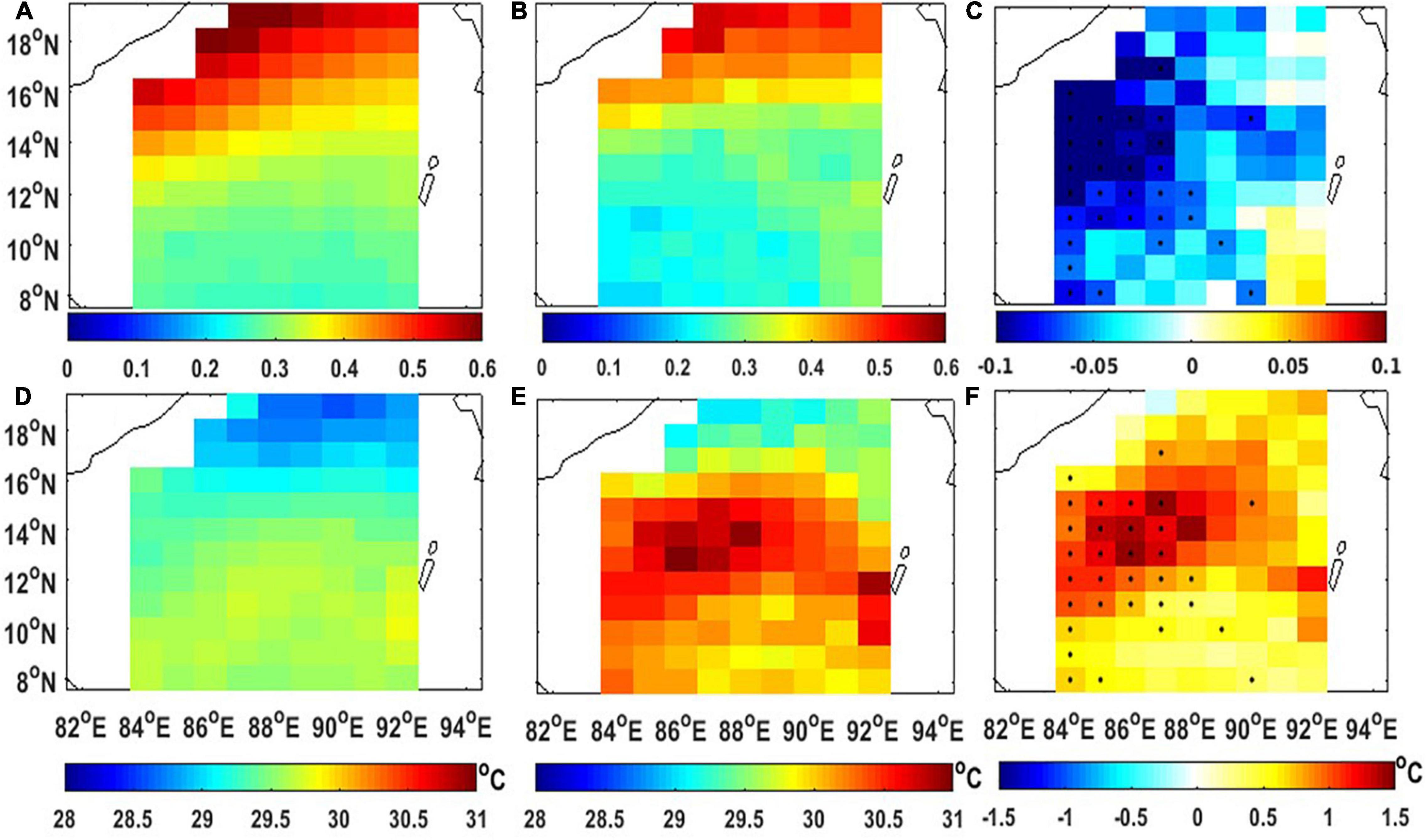
Figure 2. (A) Climatological aerosol loading during April 2003–2019. (B) Aerosol loading during April 2020. (C) AOD anomaly (dots signify regions where AOD change is more than 20%). (D) Climatological SST during April 2003–2019. (E) SST during April 2020. (F) SST anomaly (dots signify regions where AOD change is more than 20%).
Figure 2C shows the anomalies in AOD for the period (April 2020 climatology). It is clear that AOD decreased during this period coinciding with the world’s most extensive lockdown affecting more than a billion people in the sub-continent. The AOD decreased by 0.05–0.15 (30–60%) over the Northwest part of BoB. The area average decline was about 0.1 over the whole domain (Figures 2B,C). This decline is substantial, knowing that AOD changes even over developed countries were lower than this (Acharya et al., 2020; Sanap, 2021). Even considering the high aerosol-laden IGP, these numbers are substantial (greater than 20–30% for this period). The high decline in percentages corresponds to regions in the south Bay of Bengal due to their ordinarily low loading conditions. During the same period over land, the AOD decreased by 0.4 (Pandey and Vinoj, 2020; Soni et al., 2021). The IGB (major source region for aerosols over BoB) showed the maximum change in AOD.
As already mentioned, the SST is lower over N. BoB and increases toward the South (Figure 2D). The spatial distribution and gradients for aerosol and SST are opposite over BoB. In April 2020 (Figure 2E), the SST has increased throughout the basin, but more so over North-Western parts during the lockdown period. The Figures 2C,F shows the difference between climatology and 2020 for AOD and SST, respectively. It is found that the decline in AOD spatially matches the pattern of increase in SST (Figure 2F). The spatial pattern of change in SST and AOD points to the possibility of aerosol decline leading to a substantial increase in SST. The negative relationship in the spatial pattern of AOD and SST (high AOD corresponding to low SST and vice versa) in a climatological sense and the similar and consistent spatial pattern of change during April 2020 points to a possibility of physical causation. Thus both in climatological sense and anomalies (and their exact match in the spatial pattern) during April 2020, the AOD and SST indicate a strong negative relationship.
Earlier studies have established that winds and freshwater forcing are the dominating factors affecting the temporal variability of SST in the BoB (Duncan and Han, 2009; Seo et al., 2009; Akhil et al., 2014; Jana et al., 2015; Srivastava et al., 2018). The buoyancy flux plays a significant role in changing the region’s SST (and MLD) during the winter monsoon (January and February). Similarly, wind stress forcing modulates the SST during the summer monsoon period. Investigating the intraseasonal variability of SST in the Indian Ocean during boreal summer using Hybrid Coordinate Ocean Model (HYCOM), Duncan and Han (2009) found that wind plays a much more significant role in altering SSTs than either shortwave fluxes or precipitation. Low wind speed conditions over BoB characterize April; hence insolation is the dominant contributor to the variability in SST. This indicates that aerosol-induced changes to solar insolation may significantly impact the SST variability during this period. The SST variability decreases from North to South, with the northern BoB showing higher SST variability compared to the southern portion (Srivastava et al., 2018). This is similar to the variability in aerosol loading as well.
Thus, SST’s over the BoB is influenced by aerosols. This is not surprising as studies have shown in the past that aerosols lead to the large top of the atmosphere cooling of the order of −1 to −2 W m–2 (Andreae et al., 2005). These are expected to be substantially larger in highly polluted regions with extensive aerosol loading such as BoB with top of the atmosphere (surface) forcing being of the order of −6 to −10 W m–2 (−30 to −35 W m–2) (Vinoj et al., 2004; Ramachandran, 2005).
Quantifying the SST Change Due to Aerosols
An attempt is made to estimate the change in SST as a function of AOD using daily raw AOD and SST datasets during April 2003–2019 (510 days of data over a large spatial scale with 101 grid points). These datasets have undergone the initial quality checks mentioned in the section “Data and Methods” and correspond to method 1. Figure 3 shows the contour density plot to establish the relationship between SST and AOD. The SST decreases with an increase in AOD. The value of the slope implies that an increase in unit AOD leads to a roughly 1.64°C decrease in temperature. Therefore, this simple estimate reveals that, on average, a 0.1 difference in AOD may cause SST to change by 0.16°C.
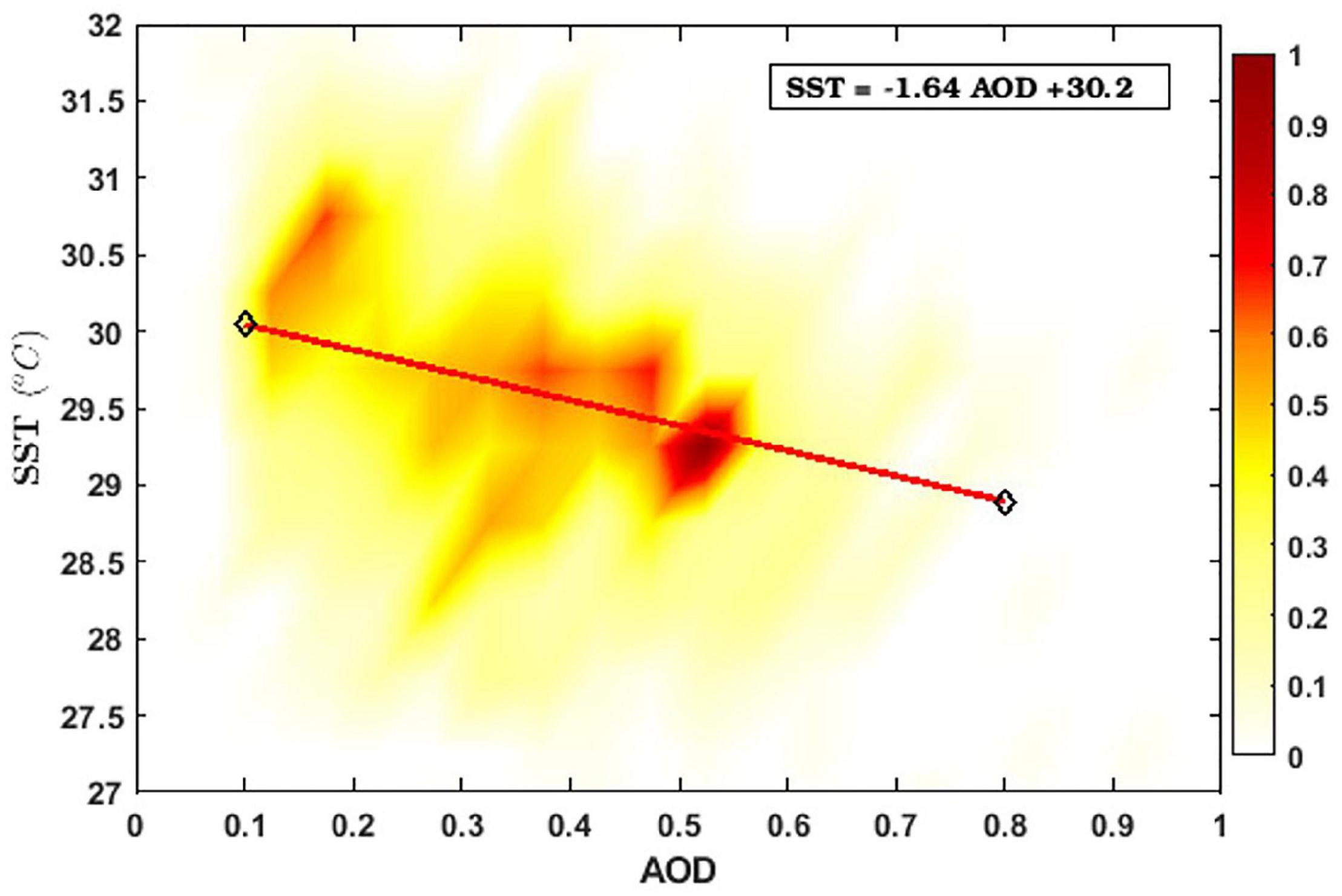
Figure 3. The scatter plot showing the relationship between SST and AOD for April 2003–2019. The color bar represents the number density at each pixel normalized by the maximum over the whole SST-AOD space.
The above assessment is made using raw AOD and SST after the initial screening for outliers and avoiding coastal grids and clouds but may be influenced by seasonality, trends, and other atmospheric parameters. Also, this estimate is valid only for this region for this period. If one has to find the SST change elsewhere, these numbers may vary. This is mainly due to differences in aerosol properties and the specific processes that influence SST over different regions. For example, a stagnant ocean region may show a more significant sensitivity of SST to change in atmospheric constituents and solar insolation than a more dynamic ocean surface. This is also an average estimate for the whole study region. Rajeev et al. (2008) showed that a change in aerosol loading of 0.8 led to a cooling of ∼1°C over the Equatorial Indian Ocean due to the Indonesian forest fire-induced aerosols during September/October 1997. This sensitivity (∼1.25°C for a unit change in AOD) is slightly lower than our estimate of 1.64°C. This may be due to the change in season and differing oceanic processes relevant for that particular region. The SST’s are also much warmer during April than September/October. In addition, other marine processes such as eddies may strengthen or dampen the sensitivity of SST to changes in aerosols.
Quantifying the SST Change Due to Aerosols Without Clouds and Water Vapor
A multi-linear regression analysis was carried out (method 2) incorporating AOD, TWV, and CFR in place of simple linear regression to remove the effects induced by other parameters such as CFR and TWV if any. The sensitivity of SST to AOD is calculated by setting the regression coefficients corresponding to all other parameters to zero at each grid point over the BoB.
The total change in SST observed during April 2020 (Figure 4A) and that owing to aerosols is shown in Figure 4B (method 1) and Figure 4C (method 2), respectively. The only difference between Figures 4B,C is that one corresponds to change in SST estimated using a single regression coefficient applied to all grid points over BoB and the other where regression coefficients are calculated separately at each grid point. That is the reason for the similarity in the spatial pattern of Figures 4B, Figure 2C. Both these estimates are based on regression coefficients developed using long-term datasets covering 2003–2019 and applying them to change in AOD observed during 2020. The SST change due to aerosols is seen to be statistically significant at most pixels, where the change in SST is high (Figure 4C). The aerosols have a higher effect on SST closer to the coasts (North West) (see Figure 2C), as expected. These regions most affected by emissions from the surrounding landmass coincided with a significant decline in AOD during April 2020. Large spatial variability is observed in the warming attributable to aerosols, with the most considerable warming exceeding 1.5°C. Such differences may be possible due to various reasons, including variations in aerosols loading, aerosol type, sea surface properties such as salinity, the thickness of the mixed layer, barrier layer, etc.
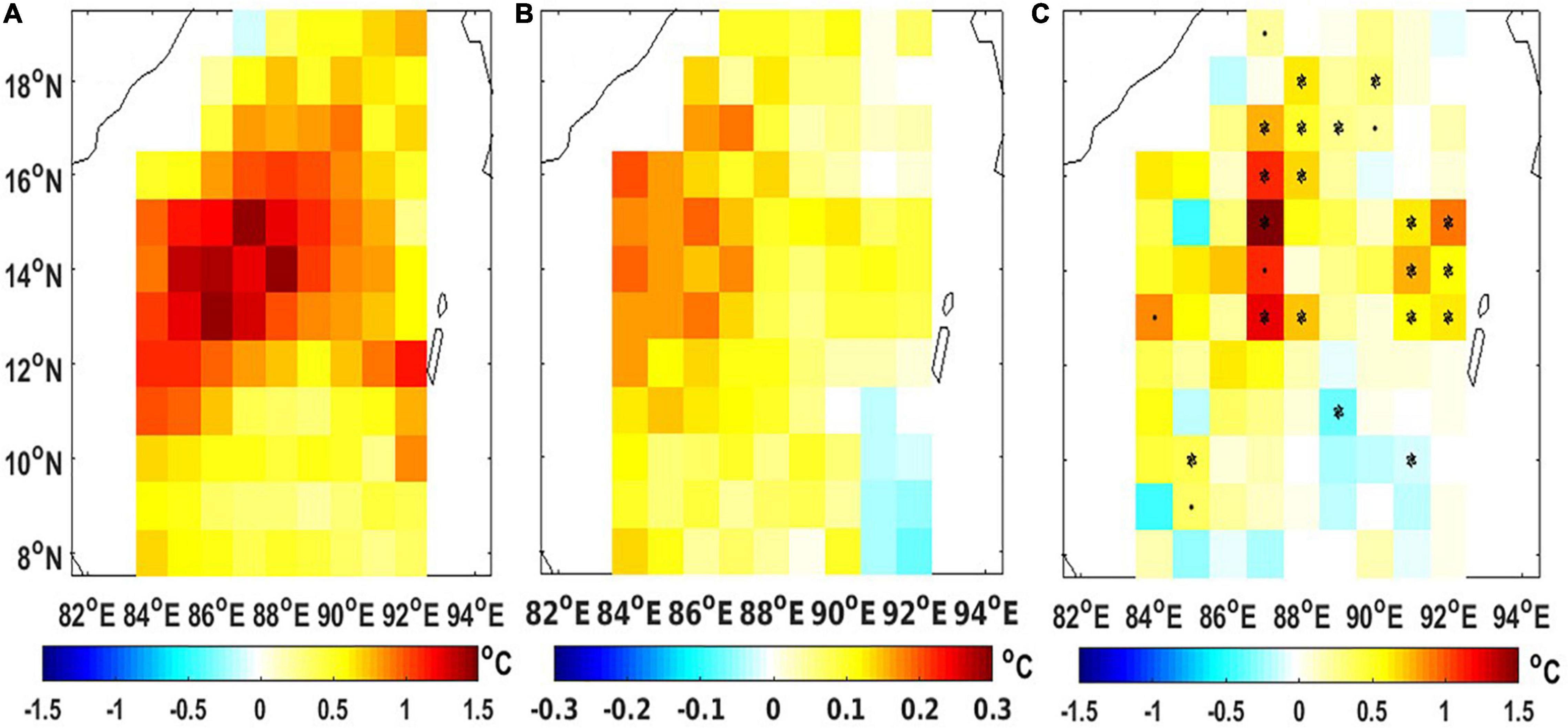
Figure 4. (A) The observed spatial pattern of change in SST. (B) Change in SST calculated using method 1. (C) Change in SST estimated using method 2 (dots and stars represent statistical significance at 90 and 95% levels, respectively).
Detailed analysis of all these factors is beyond the scope of this work and hence is not attempted. However, most grids show warming close to or greater than 0.2°C (Figures 4B,C, shown as yellow contours). These numbers are comparable to those obtained earlier in method 1 (an average of 0.16°C corresponding to a 0.1 change in AOD). This spatial pattern (Figure 4B) for April 2020 coinciding with the high-temperature anomaly (Figure 4A) provides confidence that AOD decline led to the observed warming. Thus, it may be said that the SST change is in part modulated by a significant decline in aerosol loading due to COVID-19 lockdown over the BoB.
Overall, the whole BoB region shows an average observed warming of 0.83°C during April 2020 (see Figure 5), while the average aerosol effect is estimated to be ∼0.26°C, which is ∼32% of the overall warming.
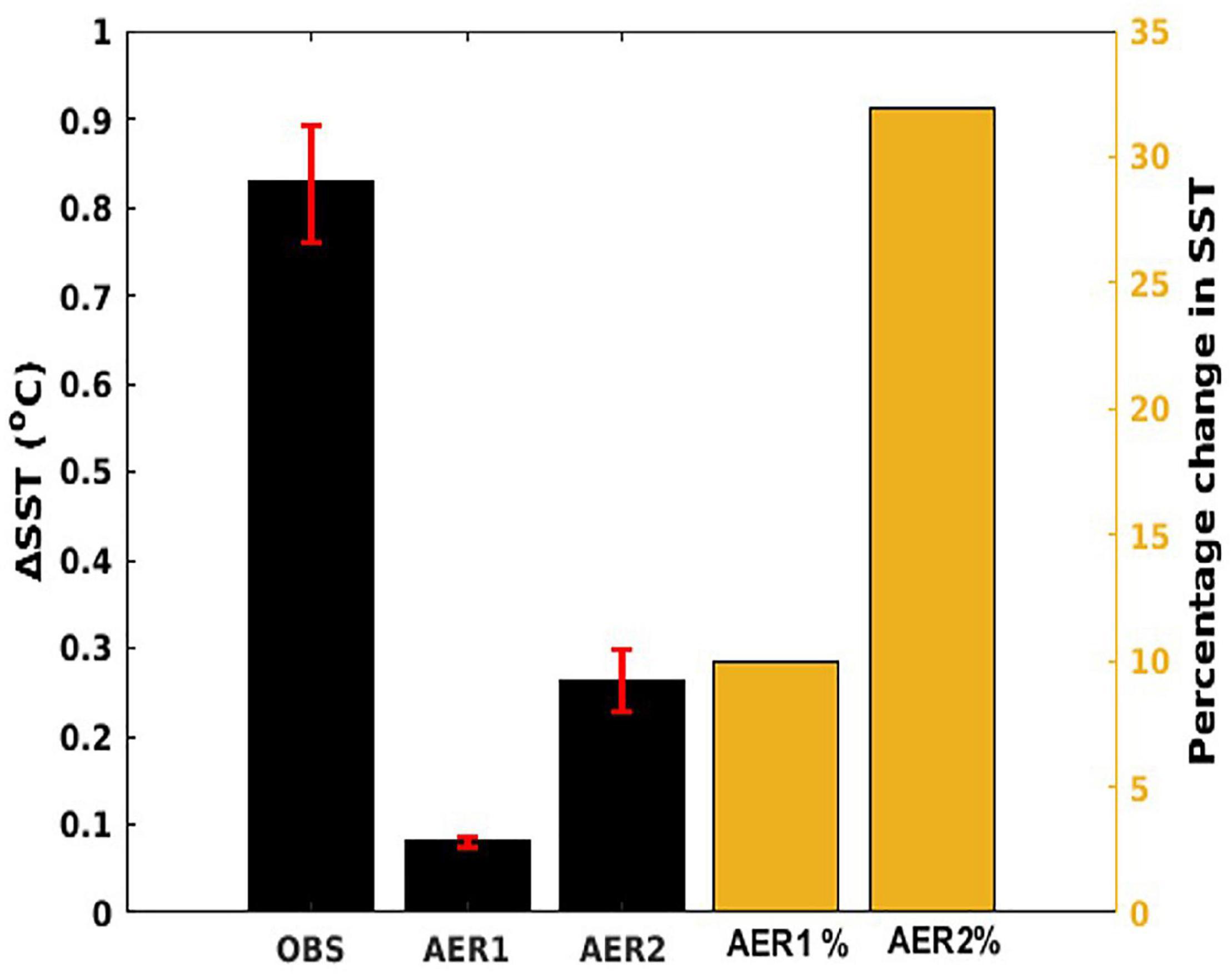
Figure 5. The total warming observed during April 2020 and that attributed to aerosols (AER1 and AER2). The yellow bar represents the aerosol-led warming in percentage (corresponding to the y-axis shown to the right). The error bar represents the standard error estimated from the spatial distribution.
One crucial aspect that needs to be mentioned here is the significant difference in change in SST between methods 1 and 2. In method 1, the effect of CFR is minimized, but the presence of water vapor (though only data less than the 20th percentile is used) appears to dampen the aerosol impact on SST. Also, in method 2, it is found that the regression coefficients for TWV were overall positive, indicating a warming effect. Such warming due to TWV is not surprising as past studies have suggested that irradiance in the long wave (LW) spectrum increases with increasing water vapor and subsequent changes to downwelling radiation (Obregón et al., 2015). Pandey and Vinoj (2020) showed that humidity increased by 10–15% over the Northern BoB during the lockdown. Such an increase in water vapor content may induce warming [as indicated by the positive regression coefficient from method 2 (not shown)], thus reducing the overall aerosol effect. Stanhill (2011) also demonstrated a more significant sensitivity of surface temperature to LW than short wave (SW) due to water vapor. Overall, method 1 shows aerosol-induced warming of ∼10%, whereas method 2, which minimizes both the effect of CFR and TWV, shows amplified aerosol-induced warming of ∼30%.
Some Limitations of the Study
There are a few limitations in the current study. The aerosol-induced warming of ∼10–30% is expected to be a lower bound as only the direct effect under clear sky conditions is studied. The aerosols also alter radiation balance indirectly by modifying cloud properties such as effective cloud droplet radius and thus cloud albedo and are not addressed in this article. Our preliminary analysis also indicates a decline in cloud fraction during this period (not shown). In addition, another related study by Bhowmick et al. (2020) showed that warm-core eddies over this region might have also led to the increased overall SST during the lockdown. This also points to the possibility of high sensitivity to aerosols (compared to Rajeev et al., 2008) over these warm-core eddies as their interaction with water mass around them is minimal. Thus, it may be said that the aerosol effect on SST, in reality, maybe higher if their impact through cloud modifications is considered. However, quantifying these effects is beyond the scope of this work and hence not attempted.
Conclusion
This study explored the impact of COVID-19 lockdown during April 2020, the resultant decline in aerosol loading, and its effect on SST over the BoB using satellite observations. Our study reveals that the lockdown-induced decrease in the AOD over the Bay of Bengal was ∼30% (corresponding AOD of 0.05–0.1). Simultaneously, the SSTs over the whole basin increased by ∼+0.83°C (up to 1.5°C in certain pockets). Spatially, the decline in aerosol loading matched well with the areas showing a substantial increase in SSTs (especially over North and North-West of BoB). The analysis indicates a rise in SST of the order of +0.26°C (∼32%) attributed to aerosols’ effect. The SSTs of regions closer to the coast and the North Central part of BoB were the most affected by changes in aerosol loading. Thus, our study shows that reduced aerosols due to the recent COVID-19 lockdowns have increased the SST partially in the BoB with implications to regional weather.
Data Availability Statement
All dataset presented in this study are available in the public domain downloadable from https://giovanni.gsfc.nasa.gov/giovanni/. Any further inquiries can be directed to the corresponding author.
Author Contributions
VV, DS, and KL conceived the idea. TS carried out all the analyses and prepared the plots. VV, TS, and DS wrote the manuscript with inputs from all co-authors. All authors contributed equally in discussions.
Conflict of Interest
The authors declare that the research was conducted in the absence of any commercial or financial relationships that could be construed as a potential conflict of interest.
Publisher’s Note
All claims expressed in this article are solely those of the authors and do not necessarily represent those of their affiliated organizations, or those of the publisher, the editors and the reviewers. Any product that may be evaluated in this article, or claim that may be made by its manufacturer, is not guaranteed or endorsed by the publisher.
Acknowledgments
The authors would like to thank the NASA Giovanni archive for providing all the datasets used in this study. TS would like to thank IIT Bhubaneswar for providing the necessary infrastructure to carry out this work as part of an internship at the institute. TS would also like to thank the Infosys Foundation for providing the funds required to continue his studies and the Ministry of Science and Technology, DST, Government for financial assistance through the INSPIRE scholarship.
References
Acharya, P., Barik, G., Gayen, B. K., Bar, S., Maiti, A., Sarkar, A., et al. (2020). Revisiting the levels of Aerosol Optical Depth in South-Southeast Asia, Europe and USA amid the COVID-19 pandemic using satellite observations. Environ. Res. 193:110514. doi: 10.1016/j.envres.2020.110514
Akhil, V. P., Durand, F., Lengaigne, M., Vialard, J., Keerthi, M. G., Gopalakrishna, V. V., et al. (2014). A modeling study of the processes of surface salinity seasonal cycle in the Bay of Bengal. J. Geophys. Res.-Oceans 119, 3926–3947. doi: 10.1002/2013JC009632
Anderson, J. C., Wang, J., Zeng, J., Leptoukh, G., Petrenko, M., Ichoku, C., et al. (2013). Long-term statistical assessment of Aqua-MODIS aerosol optical depth over coastal regions: bias characteristics and uncertainty sources. Tellus B 65:20805. doi: 10.3402/tellusb.v65i0.20805
Andreae, M. O., and Rosenfeld, D. (2008). Aerosol–cloud–precipitation interactions. Sci. Rev. 89, 13–41.
Andreae, M. O., Jones, C. D., and Cox, P. M. (2005). Strong present-day aerosol cooling implies a hot future. Nature 435, 1187–1190. doi: 10.1038/nature03671
Baldasano, J. M. (2020). COVID-19 lockdown effects on air quality by NO2 in the cities of Barcelona and Madrid (Spain) Sci. Total Environ. 741:140353. doi: 10.1016/j.scitotenv.2020.140353
Bauwens, M., Compernolle, S., Stavrakou, T., Müller, J. F., van Gent, J., Eskes, H., et al. (2020). Impact of coronavirus outbreak on NO2 pollution assessed using TROPOMI and OMI observations. Geophys. Res. Lett. 47:e2020GL087978. doi: 10.1029/2020GL087978
Bhowmick, S. A., Agarwal, N., Sharma, R., Sundar Venkatesan, R., Anoopa Prasad, C., and Navaneeth, K. N. (2020). Cyclone Amphan: oceanic conditions pre- and post-cyclone using in situ and satellite observations. Curr. Sci. 119, 1510–1151. doi: 10.18520/cs/v119/i9/1510
Boucher, O., Randall, D., Artaxo, P., Bretherton, C., Feingold, G., Forster, P., et al. (2013). “Clouds and aerosols,” in Climate change 2013: The physical science basis, contribution of working group I to the fifth assessment report of the intergovernmental panel on climate change, ed. T. F. Stocker (Cambridge, UK: Cambridge University Press), 571–658.
Buckley, J. M., Mingels, B., and Tandon, A. (2020). The impact of lateral advection on SST and SSS in the northern Bay of Bengal during 2015. Deep Sea Res. Part II 172:104653. doi: 10.1016/j.dsr2.2019.104653
Buheji, M., da Costa, Cunha, K., Beka, G., MavriĆ, B., Leandro, et al. (2020). The extent of COVID-19 pandemic socio-economic impact on global poverty. a global integrative multidisciplinary review. Am. J. Econ. 2020, 213–224. doi: 10.5923/j.economics.20201004.02
Chahine, M. T., Pagano, T. S., Aumann, H. H., Atlas, R., Barnet, C., Blaisdell, J., et al. (2006). Zhou AIRS: improving weather forecasting and providing new data on greenhouse gases Bull. Am. Meteorol. Soc. 87, 911–926. doi: 10.1175/BAMS-87-7-911
Cheng, Y., Lohmann, U., Zhang, J., Luo, Y., Liu, Z., and Lesins, G. (2005). Contribution of change in sea surface temperature and aerosol loading to the decreasing precipitation trend in southern China. J. Clim. 18, 1381–1390. doi: 10.1175/JCLI3341.1
Collivignarelli, M. C., Abbà, A., Bertanza, G., Pedrazzani, R., Ricciardi, P., and Miino, M. C. (2020). Lockdown for CoViD-2019 in Milan: what are the effects on air quality? Sci. Tot. Environ. 2020:732.
Dave, P., Bhushan, M., and Venkataraman, C. (2020). Absorbing aerosol influence on temperature maxima: An observation based study over India. Atmosph. Environ. 223:117237. doi: 10.1016/j.atmosenv.2019.117237
Dey, S., Sarkar, R. P., and Singh. (2004). Comparison of aerosol radiative forcing over the Arabian Sea and the Bay of Bengal. Adv. Space Res. 33, 1104–1108. doi: 10.1016/S0273-1177(03)00737-3
Diao, C., Xu, Y., and Xie, S.-P. (2021). Anthropogenic aerosol effects on tropospheric circulation and Sea surface temperature (1980–2020): separating the role of zonally asymmetric forcings, atmos. Chem. Phys. Discuss. [Preprint]. doi: 10.5194/acp-2021-407
Duncan, B., and Han, W. (2009). Indian Ocean intraseasonal sea surface temperature variability during boreal summer: Madden-Julian Oscillation versus sub monthly forcing and processes. J. Geophys. Res.-Oceans 114:C05002. doi: 10.1029/2008JC004958
Dwyer, J. G., Norris, J. R., and Ruckstuhl, C. (2010). Do climate models reproduce observed solar dimming and brightening over China and Japan? J. Geophys. Res. Atmosph. 115:945. doi: 10.1029/2009JD012945
Gupta, P., Christopher, S. A., Wang, J., Gehrig, R., Lee, Y., and Kumar, N. (2006). Satellite remote sensing of particulate matter and air quality assessment over global cities. Atmos. Environ. 40, 5880–5892. doi: 10.1016/j.atmosenv.2006.03.016
Gupta, P., Khan, M. N., Silva, A., and Patadia, F. (2013). MODIS aerosol optical depth observations over urban areas in Pakistan: quantity and quality of the data for air quality monitoring. Atmos. Pollut. Res. 4, 43–52. doi: 10.5094/APR.2013.005
Hansen, J. E., Sato, M., and Ruedy, R. (1997). Radiative forcing and climate response. J. Geophys. Res. 102, 6831–6864. doi: 10.1029/96jd03436
Jana, S., Gangopadhyay, A., and Chakraborty, A. (2015). Impact of seasonal river input on the Bay of Bengal simulation. Cont. Shelf Res. 104, 45–62. doi: 10.1016/j.csr.2015.05.001
Jethva, H., Chand, D., Torres, O., Gupta, P., Lyapustin, A., and Patadia, F. (2018). Agricultural Burning and Air Quality over Northern India: A Synergistic Analysis using NASA’s A-train Satellite Data and Ground Measurements. Aerosol. Air Qual. Res. 18, 1756–1773. doi: 10.4209/aaqr.2017.12.0583
Jethva, H., Satheesh, S. K., and Srinivasan, J. (2007). Evaluation of MODIS C004 aerosol retrievals at Kanpur, Indo-Gangetic Basin. J. Geophys. Res. 112:D14216. doi: 10.1029/2006JD007929
Johnson, B. T., Shine, K. P., and Forster, P. M. (2004). The semi-direct aerosol effect: Impact of absorbing aerosols on marine stratocumulus. Q. J. R. Meteorol. Soc. 130, 1407–1422. doi: 10.1256/qj.03.61
Kahn, B. H., Chahine, M. T., Stephens, G. L., Mace, G. G., Marchand, R. T., Wang, Z., et al. (2008). Cloud type comparisons of AIRS, CloudSat, and CALIPSO cloud height and amount. Atmos. Chem. Phys. 8, 1231–1248. doi: 10.5194/acp-8-1231-2008
Kanniah, K. D., Zaman, N. A. F. K., Kaskaoutis, D. G., and Latif, M. T. (2020). COVID-19’s impact on the atmospheric environment in the Southeast Asia region. Sci. Tot. Environ. 2020:736.
Kaufman, Y. J., and Tanré, D. (1998). Algorithm for remote sensing of tropospheric aerosol from MODIS. Modis. Atbd Mod. 9, 1–85.
Kedia, S., and Ramachandran, S. (2008). Features of aerosol optical depths over the Bay of Bengal and the Arabian Sea during pre-monsoon season: Variabilities and anthropogenic influence. J. Geophys. Res. 113:D11201. doi: 10.1029/2007JD009070
Koch, D., and Del Genio, A. D. (2010). Black carbon semi-direct effects on cloud cover: Review and synthesis. Atmos. Chem. Phys. 10, 7685–7696. doi: 10.5194/acp-10-7685-2010
Krishna, M. (2008). View on Bay of Bengal upwelling area on the basis of 19-years satellite sea surface temperature. Int. J. Digital Earth 1, 304–314. doi: 10.1080/17538940802149965
Kulshrestha, U., and Kumar, B. (2014). Air mass trajectories and long-range transport of pollutants: review of wet deposition scenario in South Asia. Adv. Meteorol. 2014, 1–14. doi: 10.1155/2014/596041
Kumar, A., Sudheer, A. K., and Sarin, M. M. (2008). Chemical characteristics of aerosols in MABL of Bay of Bengal and Arabian Sea during spring inter-monsoon: a comparative study. J. Earth Syst. Sci. 117: 325e332.
Lakshmi, N. B., Nair, V. S., and Suresh Babu, S. (2017). Vertical structure of aerosols and mineral dust over the Bay of Bengal from multisatellite observations. J. Geophys. Res. Atmosph. 122, 12,845–12,861. doi: 10.1002/2017JD027643
Leeuwenburgh, O., and Stammer, D. (2001). The effect of ocean currents on sea surface temperature anomalies. J. Phys. Oceanogr. 31, 2340–2358. doi: 10.1175/1520-0485(2001)031<2340:teooco>2.0.co;2
Levy, R. C., Mattoo, S., Munchak, L. A., Remer, L. A., Sayer, A. M., Patadia, F., et al. (2013). The Collection 6 MODIS aerosol products over land and ocean. Atmos. Meas.Tech. 6, 2989–3034. doi: 10.5194/amt-6-2989-2013
Li, L., Li, Q., Huang, L., Wang, Q., Zhu, A., Xu, J., et al. (2020). Air quality changes during the COVID-19 lockdown over the Yangtze River Delta Region: an insight into the impact of human activity pattern changes on air pollution variation. Sci. Tot. Environ. 2020:732.
Lin, I. I., Chen, J. P., Wong, G. T. F., Huang, C.-W., and Lien, C.-C. (2007). Aerosol input to the South China Sea: results from the moderate resolution imaging spectroradiometer, the quick scatterometer, and the measurements of pollution in the troposphere sensor. Deep Sea Res. II 54, 1589–1601. doi: 10.1016/j.dsr2.2007.05.013
Liu, Z., and Alexander, M. (2007). Atmospheric bridge, oceanic tunnel, and global climatic teleconnections. Rev. Geophys. 45:RG2005. doi: 10.1029/2005RG000172
Luo, B., Minnett, P. J., Zuidema, P., Nalli, N. R., and Akella, S. (2021). Saharan dust effects on North Atlantic sea-surface skin temperatures. J. Geophys. Res. 126:e2021JC017282. doi: 10.1029/2021JC017282
Mahato, S., Pal, S., and Ghosh, K. G. (2020). Effect of lockdown amid COVID-19 pandemic on air quality of the megacity Delhi. India. Sci. Total Environ. 730:139086. doi: 10.1016/j.scitotenv.2020.139086
Mandal, S., Sil, S., Pramanik, S., Arunraj, K. S., and Jena, B. K. (2019). Characteristics and evolution of a coastal mesoscale eddy in the western Bay of Bengal monitored by high-frequency radars Dyn. Atmos. Oceans 88:101107. doi: 10.1016/j.dynatmoce.2019.101107
Martin, A., Markhvida, M., and Hallegatte, S. (2020). Socio-Economic Impacts of COVID-19 on Household Consumption and Poverty. Econ. Dis. CliCha 4, 453–479. doi: 10.1007/s41885-020-00070-3
Mhawish, A., Banerjee, T., Broday, D. M., Misra, A., and Tripathi, S. N. (2017). Evaluation of MODIS Collection 6 aerosol retrieval algorithms over Indo- Gangetic Plain: implications of aerosols types and mass loading. Rem. Sens. Environ. 201, 297–313. doi: 10.1016/j.rse.2017.09.016
Milstein, A. B., and Blackwell, W. J. (2016). Neural network temperature and moisture retrieval algorithm validation forAIRS/AMSU and CrIS/ATMS. J. Geophys. Res. Atmos. 121, 1414–1430. doi: 10.1002/2015jd024008
Moorthy, K., Nair, Vijayakumar, S., Babu, Suresh, S., et al. (2009). Spatial and vertical heterogeneities in aerosol properties over oceanic regions around India: Implications for radiative forcing. Q. J. R. Meteorol. Soc. 135, 2131–2145. doi: 10.1002/qj.525
Moorthy, K., Suresh Babu, S., Manoj, M. R., and Satheesh, S. K. (2013). Buildup of aerosols over the Indian Region. Geophys. Res. Lett. 40, 1011–1014. doi: 10.1002/grl.50165
Muhammad, S., Long, X., and Salman, M. (2020). COVID-19 pandemic and environmental pollution: A blessing in disguise? Sci. Total Environ. 728:138820. doi: 10.1016/j.scitotenv.2020.138820
Mukherjee, T., Asutosh, A., Pandey, S. K., Yang, L., Panwar, A., Gogoi, P. P., et al. (2018). Increasing Potential for Air Pollution over Megacity New Delhi: A Study Based on 2016 Diwali Episode. Aerosol. Air Q. Res. 9:18. doi: 10.4209/aaqr.2017.11.0440
Nair, P. R., George, S. K., Aryasree, S., and Jacob, S. S. (2014). Chemical composition of aerosols over Bay of Bengal during pre-monsoon: Dominance of anthropogenic sources. J. Atmos. Sol. Terr. Phys. 109, 54–65. doi: 10.1016/j.jastp.2014.01.004
Nair, V. S., Moorthy, K. K., Babu, S. S., and Satheesh, S. K. (2009). Optical and physical properties of atmospheric aerosols over the Bay of Bengal during ICARB. J. Atmos. Sci. 66, 2640–2658. doi: 10.1175/2009jas3032.1
Obregón, M. A., Costa, M. J., Serrano, A., and Silva, A. M. (2015). Effect of water vapor in the SW and LW downward irradiance at the surface during a day with low aerosol load. IOP Conf. Ser. 28:012009. doi: 10.1088/1755-1315/28/1/012009
Otmani, A., Benchrif, A., Tahri, M., Bounakhla, M., El Bouch, M., and Krombi, M. H. (2020). Impact of COVID-19 lockdown on PM10, SO2 and NO2 concentrations in Salé City (Morocco). Sci. Total Environ. 735:139541. doi: 10.1016/j.scitotenv.2020.139541
Pagano, T. S., Chahine, M. T., Aumann, H. H., and Tian, B. (2006). “Climate research with the atmospheric infrared sounder,” in Proc. SPIE 6362, Remote Sensing of Clouds and the Atmosphere XI, (Mass: Kluwer Acad), 63621K. doi: 10.1117/12.689148
Pandey, S. K., and Vinoj, V. (2020). Surprising Changes in Aerosol Loading over India amid COVID-19 Lockdown. Aerosol. Air Qual. Res. 21:200466. doi: 10.4209/aaqr.2020.07.0466
Pandey, S. K., Bakshi, H., and Vinoj, V. (2016). “Recent changes in dust and its impact on aerosol trends over the Indo-Gangetic Plain (IGP),” in Proc. of SPIE, Remote Sensing of the Atmosphere, Clouds, and Precipitation VI, (Cambridge, UK: Cambridge University Press), Vol. 14, 98761Z. doi: 10.1117/12.2223314
Pandey, S. K., Vinoj, V., Landu, K., and Babu, S. S. (2017). Declining pre-monsoon dust loading over South Asia: Signature of a changing regional climate. Sci. Rep. 7:16062. doi: 10.1038/s41598-017-16338-w
Parampil, S. R., Gera, A., Ravichandran, M., and Sengupta, D. (2010). Intraseasonal response of mixed layer temperature and salinity in the Bay of Bengal to heat and freshwater flux. J. Geophys. Res. 115:C05002. doi: 10.1029/2009JC005790
Patil, N., Venkataraman, C., Muduchuru, K., Ghosh, S., and Mondal, A. (2019). Disentangling sea-surface temperature and anthropogenic aerosol influences on recent trends in South Asian monsoon rainfall. Clim. Dyn. 52, 2287–2302. doi: 10.1007/s00382-018-4251-y
Platnick, S., Meyer, K. G., King, M. D., Wind, G., Amarasinghe, N., Marchant, B., et al. (2017). The MODIS cloud optical and microphysical products: Collection 6 updates and examples from Terra and Aqua. IEEE Trans. Geosci. Remote Sens. 55, 502–525. doi: 10.1109/TGRS.2016.2610522
Prasad, A. K., and Singh, R. P. (2009). Validation of MODIS Terra, AIRS, NCEP/DOE AMIP-II Reanalysis-2, and AERONET Sun photometer derived integrated precipitable water vapour using ground-based GPS receivers over India. J. Geophys. Res. Atmos. 2009:114.
Prospero, J. M. (1990). “Mineral-aerosol transport to the North Atlantic and North Pacific: The impact of African and Asia source,” in The Long-Range Atmospheric Transport of Natural and Contaminant Substances, ed. A. H. Knap (Mass: Kluwer Acad), 59–86. doi: 10.1007/978-94-009-0503-0_4
Rajeev, K., Parameswaran, K., and Nair, S. K. (2008). Meenu Observational evidence for the radiative impact of Indonesian smoke in modulating the sea surface temperature of the equatorial Indian Ocean. J. Geophys. Res. 2008:113. doi: 10.1029/2007jd009611
Ramachandran, S. (2005). Aerosol radiative forcing over Bay of Bengal and Chennai: Comparison with maritime, continental, and urban aerosol models. J. Geophys. Res. 110:D21206. doi: 10.1029/2005JD005861
Ranjan, A. K., Patra, A. K., and Gorai, A. K. (2020). Effect of lockdown due to SARS COVID-19 on aerosol optical depth (AOD) over urban and mining regions in India. Sci. Total Environ. 745:141024. doi: 10.1016/j.scitotenv.2020.141024
Remer, L. A., Kaufman, Y. J., Tanre, D., and Mattoo, S. (2005). The MODIS aerosol algorithm, products and validation. J. Atmos. Sci. 62, 947–973. doi: 10.1175/JAS3385.1
Rupakheti, D., Kang, S., Bilal, M., Gong, J., Xia, X., and Cong, Z. (2019). Aerosol optical depth climatology over Central Asian countries based on Aqua-MODIS Collection 6.1 data: Aerosol variations and sources. Atmos. Environ. 207, 205–214. doi: 10.1016/j.atmosenv.2019.03.020
Sanap, S. D. (2021). Global and regional variations in aerosol loading during COVID-19 imposed lockdown. Atmos. Environ. 246:118132. doi: 10.1016/j.atmosenv.2020.118132
Satheesh, S. K. (2002). Radiative forcing by aerosols over Bay of Bengal region. Geophys. Res. Lett. 29:2083. doi: 10.1029/2002GL015334
Satheesh, S. K., Krishna Moorthy, K., and Srinivasan, J. (2006). Spatial and Temporal Heterogeneity in Aerosol Properties and Radiative Forcing over Bay of Bengal: Sources, Role of Aerosol Transport and Implications to Regional Climate. J. Geophys. Res. 111:D08202. doi: 10.1029/2005JD006374
Satheesh, S. K., Moorthy, K. K., and Das, I. (2001). Aerosol spectral optical depths over the Bay of Bengal. Arabian Sea Ind. Ocean Curr. Sci. 81, 1617–1625.
Satheesh, S. K., Vinoj, V., and Krishna Moorthy, K. (2010). Assessment of aerosol radiative impact over oceanic regions adjacent to Indian subcontinent using multi-satellite analysis. Adv. Meteorol. 2010:139186. doi: 10.1155/2010/139186
Schott, F. A., and McCreary, J. P. (2001). The monsoon circulation of the Indian Ocean. Progr. Oceanogr. 51, 1–123. doi: 10.1016/S0079-6611(01)00083-0
Schott, F. A., Dengler, M., and Schoenefeldt, R. (2002). The shallow overturning circulation of the Indian Ocean. Progr. Oceanogr. 53, 57–103. doi: 10.1016/S0079-6611(02)00039-3
Seo, H., Xie, S. P., Murtugudde, R., Jochum, M., and Miller, A. J. (2009). Seasonal effects of Indian Ocean freshwater forcing in a regional coupled model. J. Climat. 22, 6577–6596. doi: 10.1175/2009JCLI2990.1
Shinoda, T. (2005). Impact of the diurnal cycle of solar radiation on intraseasonal SST variability in the western equatorial Pacific. J. Climate 18, 2628–2636. doi: 10.1175/jcli3432.1
Soni, M., Verma, S., Jethava, H., Payra, S., Lamsal, L., Gupta, P., et al. (2021). Impact of COVID-19 on the air quality over china and india using long-term (2009-2020) multi-satellite data. Aerosol. Air. Qual. Res. 21:200295. doi: 10.4209/aaqr.2020.06.0295
Srivastava, A., Dwivedi, S., and Mishra, A. K. (2018). Investigating the role of air-sea forcing on the variability of hydrography, circulation, and mixed layer depth in the Arabian Sea and Bay of Bengal. Oceanologia 60, 169–186. doi: 10.1016/j.oceano.2017.10.001
Stratoulias, D., and Nuthammachot, N. (2020). Air quality development during the COVID-19 pandemic over a medium-sized urban area in Thailand. Sci. Total Environ. 746:141320. doi: 10.1016/j.scitotenv.2020.141320
Stanhill, G. (2011). The role of water vapor and solar radiation in determining temperature changes and trends measured at Armagh, 1881-2000. J. Geophys. Res. 116:D03105. doi: 10.1029/2010JD014044
Sumanth, E., Mallikarjuna, K., Stephen, Joshi, Moole, Mahesh, et al. (2004). Measurements of aerosol optical depths and black carbon over Bay of Bengal during post-monsoon season. Geophys. Res. Lett. 31:L16115.
Tian, B., Manning, E., Roman, J., Thrastarson, H., Fetzer, E. J., and Monarrez, R. (2020). AIRS Version 7 Level 3 Product User Guide.
Tiwari, S., Mishra, A. K., and Singh, A. K. (2016). Aerosol Climatology over the Bay of Bengal and Arabian Sea Inferred from Space-Borne Radiometers and Lidar Observations. Aerosol Air Q. Res. 16, 2855–2868. doi: 10.4209/aaqr.2015.06.0406
Twomey, S. (1977). The influence of pollution on the shortwave albedo of clouds. J. Atmos. Sci. 34, 1149–1152. doi: 10.1175/1520-0469(1977)034<1149:tiopot>2.0.co;2
Vinoj, V., Babu, S. S., Satheesh, S. K., Moorthy, K. K., and Kaufman, Y. J. (2004). Radiative forcing by aerosols over the Bay of Bengal region derived from shipborne, island-based, and satellite (Moderate-Resolution Imaging Spectroradiometer) observations. J. Geophys. Res. 109:D05203. doi: 10.1029/2003JD004329
Vinoj, V., and Pandey, S. (2016). “Towards understanding the variability of aerosol characteristics over the Indo-Gangetic Plain,” in Proc. SPIE 9882, Remote Sensing and Modeling of the Atmosphere, Oceans, and Interactions VI, (Mass: Kluwer Acad), 988205. doi: 10.1117/12.2223315
Vinoj, V., and Satheesh, S. K. (2003). Measurements of aerosol optical depth over Arabian Sea during summer monsoon season. Geophys. Res. Lett. 30, 67-1–67-4. doi: 10.1029/2002GL016664
Vinoj, V., Satheesh, S. K., and Krishna Moorthy, K. (2010). Optical, radiative and source characteristics of aerosols at a remote island, Minicoy in the southern Arabian Sea. J. Geophys. Res. 115:D01201. doi: 10.1029/2009JD011810
Yu, H., Kaufman, Y. J., Chin, M., and Feingold, G. (2006). A review of measurement-based assessments of the aerosol direct radiative effect and forcing. Atmos. Chem. Phys. 6, 613–666. doi: 10.5194/acp-6-613-2006
Keywords: atmospheric aerosols, sea surface temperature, COVID-19 lockdown, Bay of Bengal, anthropogenic contribution
Citation: Sarin TS, Vinoj V, Swain D, Landu K and Suhas E (2021) Aerosol Induced Changes in Sea Surface Temperature Over the Bay of Bengal Due to COVID-19 Lockdown. Front. Mar. Sci. 8:648566. doi: 10.3389/fmars.2021.648566
Received: 31 December 2020; Accepted: 16 July 2021;
Published: 10 August 2021.
Edited by:
Xi Xiao, Zhejiang University, ChinaReviewed by:
Junyu He, Zhejiang University, ChinaJing Ma, Nanjing University of Information Science and Technology, China
Copyright © 2021 Sarin, Vinoj, Swain, Landu and Suhas. This is an open-access article distributed under the terms of the Creative Commons Attribution License (CC BY). The use, distribution or reproduction in other forums is permitted, provided the original author(s) and the copyright owner(s) are credited and that the original publication in this journal is cited, in accordance with accepted academic practice. No use, distribution or reproduction is permitted which does not comply with these terms.
*Correspondence: V. Vinoj, vinoj@iitbbs.ac.in