- 1Lancaster University Environment Centre, Lancaster University, Lancaster, United Kingdom
- 2Marine Biodiversity Exploitation and Conservation (MARBEC), University of Montpellier, Le Centre national de la recherche scientifique (CNRS), Institut de Recherche pour le Développement (IRD), Ifremer, Montpellier, France
The response-and-effect framework is a trait-based approach that seeks to break down the mechanistic links between ecosystem disturbances, species' traits, and ecosystem processes. We apply this framework to a review of the literature on coral reef fish traits, in order to illustrate the research landscape and structure a path forward for the field. Traits were categorized into five broad groupings: behavioral, life history, morphological, diet, and physiological. Overall, there are fewer studies linking effect traits to ecosystem processes (number of papers on herbivory, n = 14; predation, n = 12; bioerosion, n = 2; nutrient cycling, n = 0) than there are linking response traits to disturbances (climate change, n = 26; fishing, n = 20; pollution, n = 4). Through a network analysis, we show that the size and diet of fish are two of the most common response and effect traits currently used in the literature, central to studies on both ecosystem disturbances and processes. Behavioral and life history traits are more commonly shown to respond to disturbances, while morphological traits tend to be used in capturing ecosystem processes. Pearson correlation coefficients quantifying the strength of the relationships between the most commonly studied process, herbivory, and key effect traits (size, gregariousness, and diel activity) are provided. We find that the most popular cluster of traits used in functional diversity metrics (e.g., functional richness, functional dispersion) is comprised of size, diet, space use/position in the water column, diel activity, gregariousness, and mobility, which encompass three of the broad trait categories. Our assessment of the literature highlights that more research is needed to support an evidence-based selection of traits to understand and predict ecosystem functioning. In synthesizing the literature, we identify research gaps and provide an avenue toward a more robust trait-selection process.
Introduction
Trait-based approaches in ecology and conservation are increasingly applied as the fields shift toward prioritizing an understanding of ecosystem functioning and maintaining ecosystem services (Madin et al., 2016a; Kissling et al., 2018; Barnett et al., 2019). Such an approach allows for mechanistic insight into how species interact with, react to, and shape their habitats (Violle and Jiang, 2009; McLean et al., 2018). The application of trait-based functional ecology to coral reef ecosystems is still in its nascent stages (Bellwood et al., 2019). The launch of the Coral Trait Database in 2016 (Madin et al., 2016b) was a milestone to consolidate the use of coral traits, much like the publication of the first terrestrial plant functional trait handbook (Pérez-Harguindeguy et al., 2013), or the terrestrial invertebrate functional trait handbook (Moretti et al., 2017). Similarly, for coral reef fish, several crucial review papers and projects have begun to provide direction and clarity around which traits could be measured consistently, what is meant by the term ecosystem functioning, and what a novel functional approach might look like (Kublicki, 2010; Villéger et al., 2017; Bellwood et al., 2019; Brandl et al., 2019; Woodhead et al., 2019; Quimbayo et al., 2021).
The “response-and-effect framework” posits that it is useful to determine which traits respond to environmental gradients (“response traits”) and which traits affect ecosystem processes (“effect traits”) (Diaz and Cabido, 2001; Loreau et al., 2001; Lavorel and Garnier, 2002; Grime, 2006; Suding et al., 2008). This concept runs parallel to that of the distinction between Eltonian and Grinnellian dimensions of a niche, where the Grinnellian dimension refers to the resource needs of a species, and the Eltonian dimension refers to the impact of a species on the environment (Devictor et al., 2010). The use of such a framework provides a practical way of addressing how disturbances are likely to affect population dynamics and ecosystem functioning as a whole (Díaz et al., 2013; Salguero-Gómez et al., 2018). The trait structure of a community not only affects its sensitivity to disturbance (McLean et al., 2019), but also its capacity to support long-term functioning (Debouk et al., 2015; Duffy et al., 2016). Response traits have also been proposed as a basis for building Essential Biodiversity Variables, allowing for the effective monitoring of biodiversity change over time (Kissling et al., 2018). By identifying traits that overlap as both response and effect traits, or correlated response and effect traits, predictions about how disturbances could affect ecosystem processes can be made (Gross et al., 2008; Suding et al., 2008).
Several studies have found that functional diversity metrics respond to disturbances but also determine potential ecosystem functioning (Diaz and Cabido, 2001; Mouillot et al., 2013; Sitters et al., 2016). The use of trait-based approaches to estimate the functional diversity of coral reef fishes has become common (e.g., Richardson et al., 2017; Floeter et al., 2018; Mbaru et al., 2019). Yet, the number and selection of traits used in functional indices may greatly influence the outcomes and conclusions that can be drawn from analyses. The creation of functional groups and the use of trait-based approaches to understanding ecological dynamics is only useful if the prior selection of traits and functional groups are ecologically relevant to the questions at hand. Therefore, when applying the response-and-effect framework, it is necessary to determine which traits should be used as response traits and which traits should be used as effect traits, and where there is sufficient evidence mechanistically linking these traits to disturbances and processes.
In this paper, we review the literature on coral reef fish response and effect traits. We do this by asking which fish traits have been investigated in relation to responding to disturbances (response traits) or affecting ecosystem processes (effect traits) and how many papers have studied each of these links. We also ask which traits are being used together and why. We then explore the consistency of the direction of influence for comparable traits and extract quantitative data linking predominant traits with a commonly assessed ecosystem process. In synthesizing the literature, we provide guidance for an evidence-based selection of traits for functional research in coral reef ecology and conservation, and establish where future research and experimentation is needed to strengthen the field.
Materials and Methods
We examined response traits in relation to three disturbances and effect traits in relation to four processes common on coral reefs. The disturbances were pollution, fishing, and climate change (Hughes et al., 2010, 2017). The processes were herbivory, bioerosion, predation, and nutrient recycling (Villéger et al., 2017; Brandl et al., 2019). These limits to inclusion of disturbances and processes were applied to structure the review through the response-and-effect framework.
A comprehensive search of the literature was conducted using the Web of Science database (1974–2018). An initial search on September 26, 2018 used the terms: Topic Sentence (TS) = ((fish* AND coral AND reef*) AND trait* AND (“functional diversity” OR “functional evenness” OR “functional richness” OR “functional dispersion” OR “functional divergence” OR “functional redundancy” OR “functional group*” OR “functional complimentary”)) OR TS = ((“coral reef” OR “coral reefs”) AND (fish OR fishes) AND (trait OR “life history”) AND (function OR functions OR functional OR process OR processes OR disturbance OR disturbances)). This search yielded 380 titles. Further search terms were used to find papers specifically related to relevant ecosystem processes and/or disturbances. For example: TS = ((fish* AND coral AND reef*) AND trait* AND “climate change”). A total of 227 papers were found with these follow up search terms. Furthermore, the reference lists of four review papers that appeared in the searches were “snowballed”. A total of 17 new references were added to the database using this method.
Paper inclusion criteria were that (1) one or more of the processes or disturbances were being investigated in relation to (2) one or more traits of coral reef fish. Papers were excluded if (1) they were looking specifically at larval fish traits (e.g., pelagic larval duration), (2) the process or disturbance was not one that is being reviewed for this paper, (3) the paper was not accessible or not in English, and/or (4) the paper was a review article (although references were checked and snowballed). Papers were first filtered by titles, then abstracts, and finally full body texts. From a total of 624 papers accumulated with the three search strategies, 80 papers met the above criteria and were included in the systematic review (see PRISMA flow diagram, Supplementary Figure 1).
The traits, processes, and disturbances being studied in each paper were identified. Where possible, the direction of the relationship between the trait and process or disturbance was assessed. After a full list of traits had been accumulated, similar traits were grouped together to generate a succinct selection of relevant traits. For example, “size” was used as a way to group together traits such as “total length,” “maximum length,” and “body mass.” Traits were classified as either “behavioral,” “life history,” “morphological,” “diet and trophic level,” or “physiological” (Table 1).
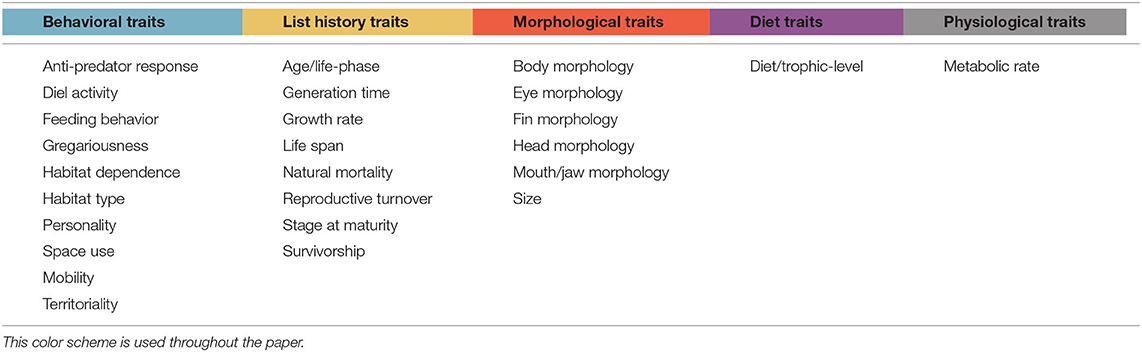
Table 1. Broad groupings of traits, where blue corresponds to behavioral traits, yellow to life-history traits, red to morphological traits, purple to diet traits, and gray to physiological traits.
In order to address the first question, “which traits have been investigated in relation to responding to disturbances (response traits) or affecting ecosystem processes (effect traits), and how many papers have studied each of these links?,” a weighted and directed tripartite network diagram was produced using the R package “igraph” (Csárdi, 2019). The diagram illustrates the number of papers, displayed as thickness of the lines (edges), linking traits to disturbances or processes (nodes).
To address the second research question, “which traits are being used together?,” the R packages “igraph” (Csárdi, 2019) and “CINNA” (Ashtiani et al., 2019) were used to produce an undirected, weighted network diagram. This diagram shows the links between traits used together in papers, where the size of the nodes shows the number of papers using the trait, and the size of the edges illustrates the frequency of trait combinations being included together in papers. The centrality of the nodes shows how commonly traits were used in combination with other traits. These centrality measures were quantified for the five most central traits. Centrality metrics used include degree centrality, subgraph centrality, and the topological coefficient. These metrics were selected as the top three most informative centrality measures, based on the output of a Principal Component Analysis (Ashtiani et al., 2018). Degree centrality can be defined as the number of nodes linked to a given node (Zhang et al., 2007), while subgraph centrality accounts for the participation of a node in the network's sub graphs and deals with more complex networks (Estrada and Rodríguez-Velázquez, 2005), and the topological coefficient quantifies the extent to which neighbors share pairs of nodes (Doncheva et al., 2012). The median rank for the centrality metrics and the individual metric values were plotted using the R package “ggplot2” (Wickam, 2016).
To illustrate the traits being used together to generate functional diversity and related metrics, a Principal Coordinate Analysis (PCoA) (Kenkel, 2006) was carried out using the R package “ape” (Paradis et al., 2019). The analysis was conducted on a Jaccard's dissimilarity matrix based on a presence/absence matrix (Cheetham and Hazel, 1969) of traits within papers looking at functional diversity metrics. Two principal component axes, explaining 49% of the variation in the distance matrix, are illustrated. The number of clusters of traits within the plot was selected using the R package “NbClust.” This package determines the optimum number of clusters based on 30 indices (Charrad et al., 2014). Clusters were illustrated as convex hulls grouping traits together.
The type of evidence used in each paper to test/support disturbance-trait or trait-process links was then categorized as one of the following: ex situ, experiment; ex situ, measurement; ex situ, specimen measurement; in situ + ex situ, measurement; in situ, experiment; in situ, observation; modeling/theoretical approach; other. For example, if a paper assessed the effect of climate change-associated rises in temperature on reef fish boldness behavior in a controlled experimental lab environment, it would be counted in the “ex situ, experiment” category.
Next, we assessed the direction of the relationship between continuous or ordinal traits and processes/disturbances. Trait-process/disturbance linkages were assessed as either being positive, negative, or insignificant based on trends reported in the papers. For Bayesian analyses, where statistical significance is not relevant, only the direction of influence was recorded. For example, if a response trait, such as “generation time,” was shown to decrease in response to a disturbance such as “fishing,” it would be classified as a “negative” relationship. Similarly, if a process, such as “herbivory,” measured as the amount of algae removed in a given time/space, was shown to increase with the “size” (effect trait) of fish, the relationship would be classified as “positive.” Categorical trait relationships were excluded from this analysis, but paper result summaries are provided as a Supplementary Table 1.
To test the quantitative applicability of the framework to coral reef fish, we investigated the effect size of effect traits on the process of herbivory. Herbivory was selected as the ecosystem process for which to extract quantitative effect sizes, because (1) it was the most commonly studied process found in our search, and (2) herbivory was the process with the most consistent measurement in the literature (bite rate or impact). Three of the most common traits related to herbivory in the literature—size, gregariousness and diel activity—were selected as effect traits to assess. Diet was excluded from the quantitative assessment because its relevance is in relation to diet categories which is implicit for herbivory. Because the initial search term limited papers to those using the term “trait,” whereas some papers use traits without explicitly naming them as such, an additional search was conducted to identify papers which may not have used the terms “trait” or “function” but had quantified herbivory. An additional four papers were found looking at the impact of traits on herbivory and directly measuring the process.
Where effect sizes were extracted from the literature, they were converted into Pearson r correlation coefficients according to Beltramini and Wolf (1987) and Friedman (1982). Pearson's r was selected because it allows for contrasts between two or more groups without computational corrections and is easily interpreted as it sits on a limited scale from−1 to 1 (Rosenthal and DiMatteo, 2001). Relevant statistical information needed to compute the effect size was extracted where available. WebPlotDigitizer (Rohatgi, 2019) was used to extract raw data from figures. Where data was provided but not analyzed in relation to traits of interest (e.g., Humphries et al., 2014), a simple correlation was conducted using raw data. For size bins/categories, the average size was used. In papers presenting data on trait-bite-rate relationships for more than one species or more than one site, average effect sizes and 95% confidence intervals are calculated. Additionally, for each trait, an average Pearson's r based on all the papers is presented with 95% confidence intervals. All statistical analyses were performed using R software version 3.5.2. (R Core Team, 2018).
Results
Based on our search results (See PRISMA flow diagram, Supplementary Figure 1), the most studied disturbance affecting coral reef fish traits is climate change (number of papers, n = 26), followed by fishing (n = 20), and then pollution (n = 4). Climate change encompassed papers assessing the impacts of increasing temperatures, rising levels of CO2, extreme weather events and coral bleaching. Fewer papers investigated ecosystem processes using an explicit trait-based approach. The process most studied was herbivory (n = 14), then predation (n = 12), bioerosion (n = 2), and finally no papers were identified through our search terms that quantified nutrient transfer through a trait-based approach (n = 0). “Predation” captured papers assessing prey traits, predator traits, or the interaction between both. The most studied disturbance-response trait links were between size and fishing (n = 16), size and climate change (n = 15), diet and fishing (n = 11), and diet and climate change (n = 8). The most studied effect trait-process links were diet and herbivory (n = 9), size and predation (n = 8), and size and herbivory (n = 6). Size and diet had the greatest number of papers that link the traits both to disturbances and processes, making them both well-studied response and effect traits (Figure 1; Supplementary Figure 2).
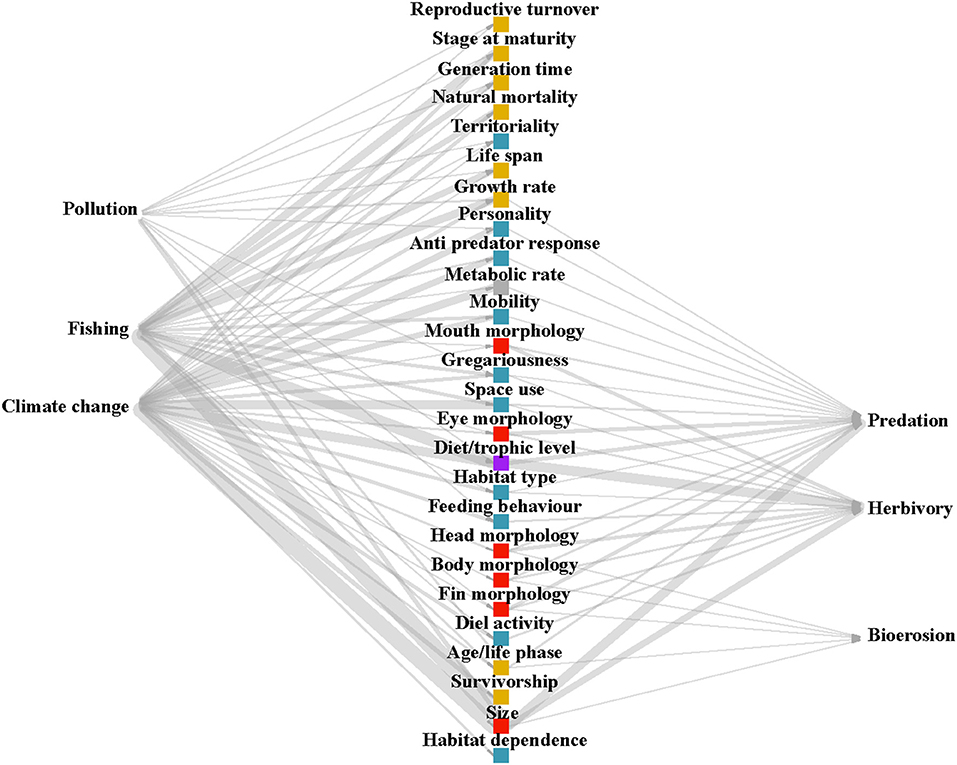
Figure 1. Tripartite network diagram showing the number of papers linking each disturbance and process to response and effect traits. The color of trait points corresponds to broad trait groupings (blue: behavioral, yellow: life-history, red: morphological, gray: physiological, purple: diet).
The network analysis plot, based on the traits of all papers included in the database, illustrates three key findings. Firstly, size and diet, followed by space use, gregariousness, and growth rate, are the most commonly used traits in the literature (Figure 2). Secondly, trait categories, for example, behavioral or morphological (depicted by color), tend to group together in network space. Size, a morphological trait, and gregariousness, a behavioral trait, are the exceptions to this, being situated in the middle of the plot (Figure 2; Supplementary Figure 3). Thirdly, size is almost consistently ranked as the most central trait, followed by diet, gregariousness, habitat type and feeding behavior (Supplementary Figure 4). High centrality scores indicate that these traits as the ones most commonly used with combinations of other traits.
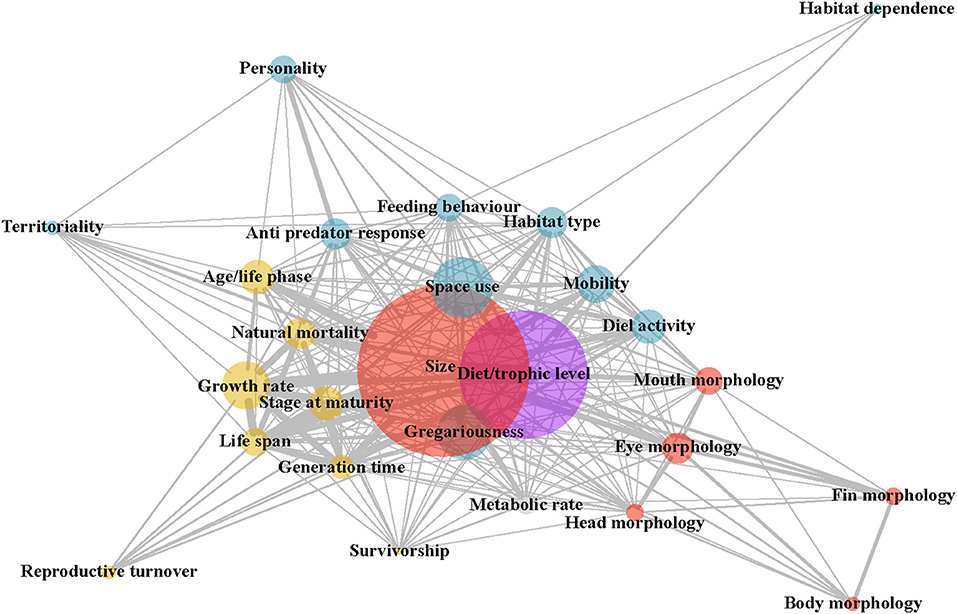
Figure 2. Network diagram showing the use of traits together within papers. Each node corresponds to a trait, with its size representing the number of papers using that trait. The color of trait nodes corresponds to broad trait groupings (blue: behavioral, yellow: life-history, red: morphological, gray: physiological, purple: diet). The thickness of the edges (i.e., lines) illustrates the number of papers in which two traits being connected were used together. The spatial position of the node indicates which traits it is most often used in conjunction with, where the more central nodes represent traits used frequently with all other traits. Rankings of centrality scores (median of degree centrality, subgraph centrality, and topological coefficient) showed size to be the most central trait, diet and gregariousness as the second most central traits, and habitat type and feeding behavior as the third most central traits (see Supplementary Figure 4).
A total of 17 papers used traits to calculate functional diversity metrics for coral reef fish, or to derive functional entities. Functional diversity indices were most commonly calculated using size (n = 15), diet (n = 14), and space use (n = 9). The traits most commonly used together are size, diet, gregariousness, mobility, diel activity, and space use (e.g., position in the water column) (n = 5) (Figure 3). Two other groupings of traits were also apparent: age/life phase with reproductive turnover; and eye morphology with mouth morphology, feeding behavior, habitat type and territoriality. These groupings were based on Euclidean space in the PCoA axes and the traits encompassed were not necessarily used consistently all together in the literature. For example, habitat type was used in five papers; some of these papers also used traits located in the cluster of the most frequently applied six traits. In Brandl et al. (2016), habitat type is used along with diet, size, and territoriality to examine how coral reef fish functional diversity responds to a disturbance in the form of a tropical cyclone. Age/life phase and reproductive turnover represent a lesser studied cluster of traits, produced by papers that include broader life history strategies in functional analyses (e.g., Tuya et al., 2018).
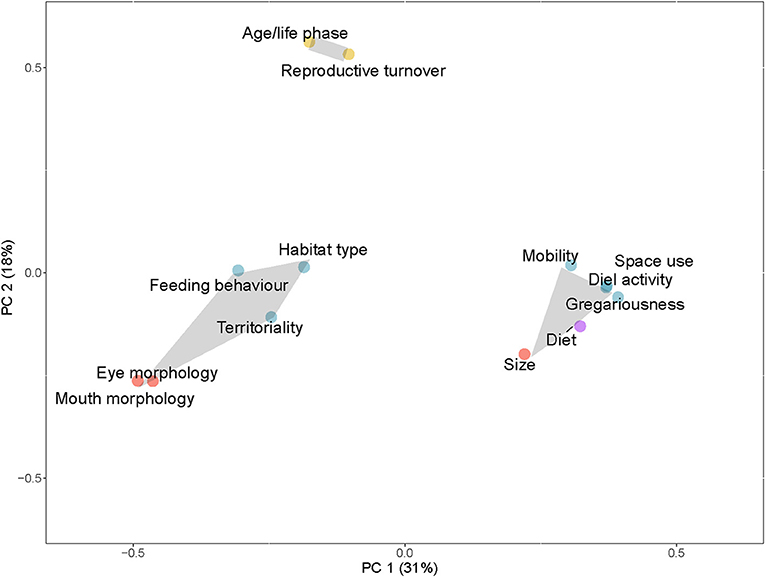
Figure 3. PCoA plot of traits used to estimate functional diversity or to determine functional entities. Three clusters show traits commonly used together in functional diversity metrics. Color of trait points corresponds to broad trait groupings (blue: behavioral, yellow: life-history, red: morphological, gray: physiological, purple: diet).
Overall, the evidence for the majority of functional diversity papers is based on in situ observational evidence (n = 10; total in situ observational n = 36). The number of papers based on this type of evidence is more than double the number of papers based on ex situ experimental (n = 14) and in situ experimental (n = 14) evidence. Trait-relationships based on ex situ measurements (n = 9) and purely theoretical or modeling-based papers (n = 8) were less common. Ex situ specimen trait measurements (n = 1) and a combination of in situ and ex situ measurements (n = 1) were each only represented by one paper (Figure 4).
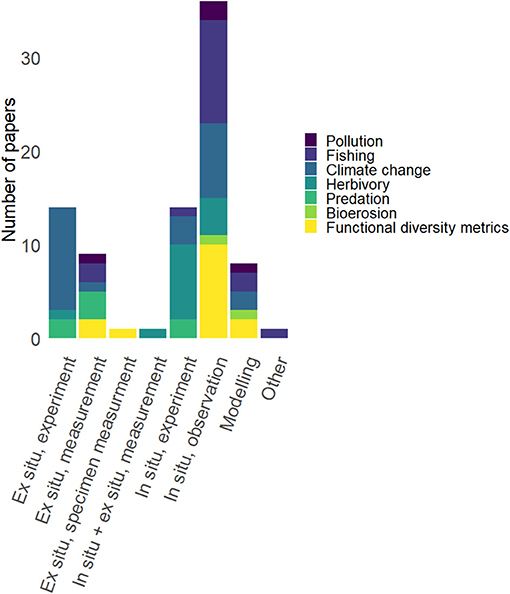
Figure 4. Number of papers (colored according to disturbance, process, or functional diversity) in each evidence category.
The direction of influence of disturbances on response traits and effect traits on ecosystem processes shows the consistency of findings across the limited set of papers identified in the review (Figure 5). Most papers found a negative influence of pollution on the size of fish (n = 3). There was an even split between the number of papers finding an insignificant and positive effect of pollution on the trophic level of coral reef fish (n = 2). There was 100% agreement on the negative relationship between fishing and the life history traits of life span (n = 3) and generation time (n = 3), showing that all papers framed as trait-based and/or functional in the literature identified, found that high fishing pressure results in short life spans and generation times. Fishing had a negative impact on gape size (n = 1), a mostly negative impact on the size (n = 10) and trophic level (n = 5) of fish, but a positive influence on natural mortality (n = 3), and a mostly positive impact on growth rate (n = 4). All papers assessing the impact of climate change on anti-predator responses (n = 4) found a negative relationship. On the other hand, climate change was found to have a positive relationship with metabolic rate (n = 4). There was a mixed effect of climate change on fish size and the growth rate of fish. Climate change was either found to insignificantly affect fish trophic level or have a negative impact on trophic level (n = 2) (Figure 5).
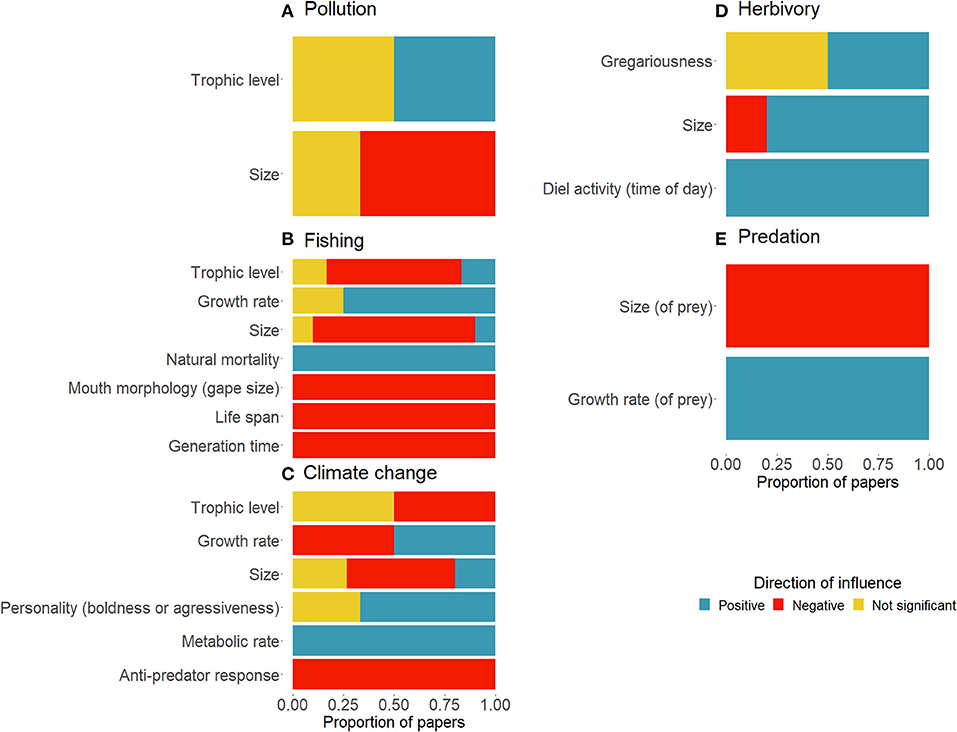
Figure 5. Proportion of papers linking traits positively (blue), negatively (red) or without significance (yellow) to (A) pollution, (B) fishing, (C) climate change, (D) herbivory, and (E) predation.
The small sample size of papers looking at continuous traits linking to measured ecosystem processes showed that there was a positive impact of time of day on herbivory (n = 1), and a mostly positive impact of size on herbivory (n = 5). Gregariousness was found to either have an insignificant or positive impact on herbivory (n = 2). Few papers quantified the process of predation in trait-based terms. However, of those that did, there was a negative effect of the size of prey on the rate of predation (n = 2) and a positive effect of the growth rate of prey on the extent of predation (n = 2) (Figure 6).
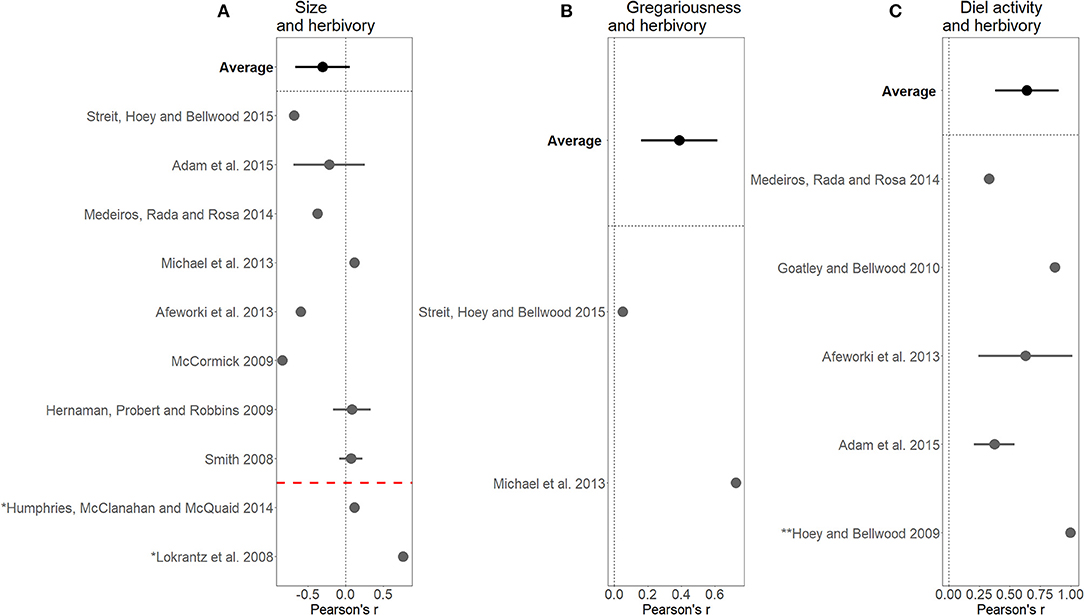
Figure 6. Pearson's r correlation coefficients with 95% confidence intervals for papers linking (A) size and herbivory, (B) gregariousness and herbivory, (C) diel activity and herbivory. Averages of Pearson scores for all the papers are highlighted in bold for each graph. The red dotted line in (A) separates out two papers excluded from average as they measured herbivory in a form other than bite rate. * Bite impact rather than rate (e.g., amount of algae removed). ** Bite rate standardized by size.
The initial search results highlighted that herbivory was the most consistently quantified ecosystem process and was typically measured as bite rate (e.g., bites/minute) or bite impact (e.g., kg × bites/minute). A Pearson correlation coefficient was calculated for each paper quantifying the relationship between effect traits and this measure of herbivory. There was a negative correlation between fish size and bite rate, as shown by the average of papers above the red dotted line in Figure 6A. However, papers looking at fish size and measures of bite impact (below the red line in Figure 6A) tended to find a positive correlation. A strong average positive, correlation between gregariousness and herbivory was identified, but note, this is based on only two studies. A positive relationship between diel activity and herbivory was also found. Diel activity was sometimes measured as a categorical trait [e.g., nocturnal and diurnal in Hoey and Bellwood (2009)] or on different time-scales [e.g., minutes after sunrise in Goatley and Bellwood (2010)], and the correlation coefficient reflects the strong magnitude of influence rather than the direction of influence (Figure 6).
Discussion
Our review highlights four main findings: (1) size and diet are used as both response and effect traits with a relatively large number of studies investigating their importance to the range of different disturbances and processes explored in this paper; (2) nutrient cycling and bioerosion are understudied processes in the trait-based coral reef fish literature; (3) there is a distinct clustering of trait types (e.g., morphological traits, life history traits, etc.) in functional diversity studies, in addition to a cluster of six mixed trait types frequently used together (size, diet, position in the water column, gregariousness, mobility, diel activity); (4) because traits are not consistently coded across the literature, amassing an informative sample size to quantify the effect size of disturbance-trait or trait-process relationships is a challenge. Nevertheless, using a small sample size of papers measuring the relationship between key traits and the process of herbivory, we highlight variation in even the most seemingly well-known interactions. We reflect on these findings and propose a way forward for trait-based approaches in coral reef fish ecology and conservation.
Trends in the Literature
Size and diet were identified as both response and effect traits (Figure 1). As illustrated in the network diagram, both traits are centrally located, with size having a median centrality ranking as the top trait, and diet ranking alongside gregariousness as the second most central trait, indicating their use in conjunction with a range of other traits within papers (Figure 2; Supplementary Figure 4). Size is also demonstrated to have a consistent relationship with herbivory, whereby bite rate is negatively correlated with size and bite impact is positively correlated with size (Figure 6). These findings support the view that size acts as a super-trait, as it scales with, and therefore shapes, a range of other functional traits (Jacob et al., 2011). However, the relationship between size and diet or trophic level is not linear, but rather, it is mediated by traits such as body depth, tooth shape, and mouth width (Keppeler et al., 2020).
In comparison to ecosystem processes, trait-based approaches to studying the effects of disturbances on coral reef fish (response) traits were more numerous. Specifically, fishing and climate change were both studied in 20 or more papers, whereas only four papers focused on pollution (Figure 1). Disturbances can interact additively or synergistically to affect the coral reef environment at a number of different levels ranging from microbial to large fish (Darling et al., 2010; Ban et al., 2014; Zaneveld et al., 2016). While fishing pressure and pollution could involve, in some cases, local solutions, climate change is likely to necessitate urgent global cooperation and decision making (Mumby et al., 2013; Hughes et al., 2017). The level of uncertainty associated with global decision making has led scientists to consider the consequences of different possible climate change scenarios (Hoegh-Guldberg et al., 2007; Pandolfi, 2015). A trait-based approach could be particularly useful to understand what novel ecosystem configurations might arise under these different scenarios (Graham et al., 2014; McClure et al., 2019).
The most commonly studied ecosystem processes were herbivory and predation. This focus in the literature is likely due to the emphasis of herbivory as a key process that prevents phase shifts to non-coral benthic communities (Hughes, 1994; Bozec et al., 2016; Cramer et al., 2017). Unlike herbivory, where only consumer traits were being studied, predation was often researched in terms of both consumer (predator) and prey traits. Studying the interactions of predators and prey contributes to an understanding of community dynamics that support ecosystem functioning (Schmitz, 2017). Although this review was limited to investigating fish traits, the interaction of fish traits with coral traits or algal traits represents a similar avenue to exploring the relationship between community producer-consumer dynamics (Rasher et al., 2013).
Gaps in the Literature
Few papers explored relationships between effect traits and bioerosion and nutrient cycling. While bioerosion is a widely recognized process on coral reefs (Lokrantz et al., 2008; Bellwood et al., 2012), only two papers were identified that measured it using an explicit trait-based approach. Such gaps in the literature highlight the need for further research quantifying such ecosystem processes, so that they can be more confidently linked to coral reef fish traits. However, since conducting the review (September 2018), there have already been strides taken to fill in research gaps and drive forward the application of trait-based approaches to estimate important functions and processes on coral reefs. For example, when this review was conducted, no papers were found linking traits to nutrient cycling, even though the process of nutrient cycling is important to the productivity of the ecosystem (Allgeier et al., 2016). A notable addition to the literature addressing this gap is a paper and companion R package proposing a trait-based approach to model nutrient cycling (Schiettekatte et al., 2020). The authors use traits such as body size, life stage, and diet to model fish ingestion and excretion rates, and accurately predict these rates for three species. Similarly, another notable publication in the field proposes a trait-based methodology and R package to facilitate the estimation of reef fish productivity (Morais and Bellwood, 2020). While productivity was not considered as a process in this review, it is an essential indicator of ecosystem functioning. The productivity of consumers on the reef (process) is demonstrated to respond to habitat degradation (disturbance) through a trait-mediated pathway (Morais et al., 2020). Thus, while the approach is not explicitly framed in the response-and-effect framework, it applies the logic of overlapping response and effect traits to demonstrate the value of traits in detailing the mechanisms through which disturbances affect ecosystem functioning.
Trait Centrality and Clustering
The results of the network and centrality analyses highlight which traits are most commonly being used together. Amongst the evidence base, there is a distinct clustering by trait type (Figure 2). Results from the ordination plot also show that the six traits popularized in Mouillot et al. (2014) (size, diet, space use/position in the water column, diel activity, gregariousness, and mobility) are frequently grouped together to compute functional diversity metrics (Figure 3). These traits cover all broad trait categories except for life history traits and physiological traits. Physiological traits, such as metabolic rate, are often difficult to obtain and vary regionally (Killen et al., 2017). Conversely, life history traits are easily obtainable (Thorson et al., 2017). However, we found that they are not commonly used in functional diversity studies and form their own cluster in the PCoA analysis (Figure 3). This is also partly attributable to the conservative definition we used for the term “life history” traits; in this paper, “life history” traits are restricted to those directly associated with survival and reproduction, as outlined in the seminal work by Stearns (1976). Such traits might compliment the selected six traits to better reflect the response diversity of coral reef fish, as such traits are frequently used to measure the response of coral reef fish to disturbances (Figure 1; Supplementary Figure 3). Nevertheless, the six traits capture a broad range of response and effect traits that have been linked to both ecosystem processes and disturbances. Three of the six traits were assessed quantitatively with respect to their impact on the process of herbivory; several studies show the importance of size, gregariousness, and diel activity in influencing the bite rates and algal removal rates of fish (Figure 6). Furthermore, the six traits have been used to effectively elucidate both global and small-scale ecosystem changes (Stuart-Smith et al., 2013; Brandl et al., 2016) and the ease at which they are obtainable likely means they have great potential for further use in functional studies (Bellwood et al., 2019).
Conversely, morphological traits, which form a cluster driven by ecosystem processes (Supplementary Figure 3), and are sometimes used as stand-alone traits in studies looking at functional morphospace (e.g., Goatley et al., 2010; Quimbayo et al., 2021) (Figure 3), are not as easily available, and as such have typically been used in studies conducted on historical records, at a small geographical scale, or for few species (e.g., Munday et al., 2011; Fox and Bellwood, 2013; Streit et al., 2015). Thus, there is scope to build upon morphological trait databases, so that ecosystem functioning can be better understood at a larger scale (Kiørboe et al., 2018). If such traits become more readily available, they might start being used in conjunction with behavioral and life history traits to bridge together research being conducted on the impact of disturbances and ecosystem functioning.
Although this review provides a broad overview of coral reef fish traits through a response-and-effect framework, it should be noted that it does not extensively cover all of the literature dealing with traits. This is partly because the term “trait” is extremely broad and partly because systematic reviews are always somewhat biased through search terms and database algorithms (Pullin and Stewart, 2006; Drucker et al., 2016). Nevertheless, one of the purposes in this paper was to bring some structure to the coral reef fish trait literature through the response-and-effect framework.
Toward a Trait-Based Approach for Coral Reef Fish Ecology
Identify Overlapping or Correlated Response and Effect Traits
A dichotomy does not exist between response and effect traits. On the contrary, many traits, especially those related to resource use, both respond to disturbances and affect ecosystem processes (Diaz and Cabido, 2001). In our review of the literature, both size and diet were found to be extensively used as response and effect traits (Figure 1). Identifying traits that are both response and effect, as well as response/effect traits that are strongly correlated, allows for a parsimonious prediction of the impacts of disturbances on ecosystem functioning (Suding et al., 2008). Correlated traits can be identified through multivariate ordination techniques. For example, Beukhof et al. (2019) demonstrate how traits positioned in close proximity in a PCA of trait-space (e.g., length and fecundity) follow similar temporal trends when exposed to environmental disturbances.
In cases where two traits are known to correlate, and one of those traits is known to respond to an ecosystem disturbance, while the other is known to affect an ecosystem process, they can be used together to harness predictive capacity. Working with microbial communities, Amend et al. (2016) found that response traits affected by drought that were strongly correlated with traits responsible for ecosystem processes allowed for the effective prediction of shifts in the functioning of microbial communities with disturbances characteristic of global change. Similarly, this review determined that the traits metabolic rate (positive relationship) and anti-predator responses (negative relationship) are linked to climate change (Figure 5). However, both traits have also been linked to the process of predation (White et al., 2013; Ferrari et al., 2015) (Figure 1). Therefore, such traits provide an opportunity for determining the pathways through which disturbances can affect ecosystem processes.
Establish Causation and Build the Predictive Abilities of Trait-Based Approaches
One concern with the response-and-effect framework could be the implied causation in the relationships between disturbances and response traits and effect traits and ecosystem processes. While the framework does attempt to structure the direction of correlations by explaining mechanisms, causation is a notoriously hard concept to prove within science (Anjum and Mumford, 2018). However, it is generally accepted that if hypothesized causal relationships have supporting data that can be theoretically justified, used, and applied, directionality in such relationships can be recognized. Such justifications underlie the processes of mechanistic and causal modeling (Connolly et al., 2017). In this review, the effect traits demonstrated to impact the process of herbivory (Figure 6) were able to be identified as effect traits, because there was a plausible causal pathway. Considering another example: size-selective fishing is proposed as the mechanism underlying a shift in the size structure of fish communities. The clear causal pathway and breadth of observational evidence supporting this disturbance-response trait link, in addition to the predictive power that comes with assuming this causal relationship, illustrates the value and purpose of structuring traits according to the response-and-effect framework. Like many of the tools applied decades ago to understand ecosystem functioning, the response-and-effect framework originates in the terrestrial plant ecology field. One of the central goals of the approach is to enable the prediction of changes in community composition and ecosystem functioning (Lavorel and Garnier, 2002).
Body size is somewhat of an anomalous trait, as it is easily recorded and has great functional importance. For other traits with less well-known causal pathways, building up an evidence base of observations under a range of conditions is important. Moreover, experimentation could be used as a controlled method of assessing causality. While this approach may not always be feasible or appropriate, small-scale controlled experiments can further test or corroborate relationships observed on a large scale (Figure 4). For example, disturbances associated with climate change provide natural experiments on a global scale (e.g., Keith et al., 2018; Richardson et al., 2018). Observations following such events offer useful information about response traits to form hypotheses that can be further tested through experimentation (e.g., Biro et al., 2010).
Favor Continuous Traits or Standardized Trait Categories, and Consider Intraspecific Variation
This review demonstrates the centrality of size in the literature (Figure 2) and its versality as a response and effect trait (Figure 1). Moreover, size can be measured on a continuous scale. In order to model the overall direction of a response or effect trait across a number of studies, consistency in measurement is essential. With categorical data, a range of potential errors get introduced in the effort to standardize (Nakagawa and Cuthill, 2007). Categorical traits have also been shown to decrease the quality of functional space (Maire et al., 2015). Maintaining consistency of categories and/or using continuous traits allows for useful meta-analyses to be compiled for a range of disturbance-trait-process relationships.
In addition to being a super-trait, size provides an avenue for investigating the importance of intraspecific variation. Intraspecific variation is a burgeoning field of research within functional ecology (Albert et al., 2011; Allgeier et al., 2017; Des Roches et al., 2018). Where substantial variability exists within species, it may no longer be sufficient to use species-level trait data (Bolnick et al., 2011). One example of the importance of intraspecific variability is illustrated in a paper by Barneche et al. (2018). The authors show that there is hyperallometric scaling in reproductive output; larger coral reef fish mothers, within the same species, have a far greater reproductive output than smaller mothers. The functional trait of an individual fish might also depend on its sex, age, or size at which it was sampled. For example, many species undergo ontogenetic shifts in their diet; it has been found that some piscivores are able to expand their diet breadth as they grow older and bigger, and their gape size can accommodate larger prey (Dunic and Baum, 2017). This diversity of size amongst individuals of a species, can be easily recorded. The size and species identity of fish is often collected using routine visual surveying techniques (Caldwell et al., 2016). Indeed, total length is a relatively simple trait to measure observationally and non-invasively in situ (Villéger et al., 2017).
Diet, on the other hand, is not typically measured for each fish during a survey, unless it is one of the explicit aims of the research. Rather, species are assigned a diet category post data collection using expert knowledge, published literature, or databases such as Fishbase (e.g., MacNeil et al., 2015). More recently, Parravicini et al. (2020) highlight the disagreement in the literature about broad reef fish trophic guilds, and provide a standardized set of diet classifications using phylogeny and maximum body size to predict trophic guild with high accuracy. However, improvements can still be made by accounting for intraspecific variation. Intraspecific variation linked to ontogenetic shifts in diet could be accounted for by assigning diet to an individual fish count based both on species information and size, where that species-level data exists. Further, technological developments such as video surveys coupled with deep learning may allow the automated estimation of diet and other individual fish characteristics beyond size (Villon et al., 2018; Li et al., 2020). Progress has also been made using gut content DNA metabarcoding to rapidly and confidently estimate diet across diverse food webs. With DNA barcode libraries expanding, there is the potential to estimate high resolution diet across large scales (Casey et al., 2019). The incorporation of between-individual variation to a trait-based approach, with traits such as size and diet, would allow for a more dynamic view of environment~trait~function relationships—a dynamism which is essential to scaling up to population dynamics, whereby such dynamics ultimately shape multiple interacting ecosystem processes (Salguero-Gómez et al., 2018).
Conclusions
This review demonstrates the ability of the response-and-effect framework to guide future research directions based on the understanding that environmental changes will undoubtedly produce functional changes. Evidence suggests that some traits provide a crucial link between fish responses to disturbances and effects on ecosystem processes. However, the evidence base is thin for linking effect traits to many processes. Thus, if an emphasis on the conservation of ecosystem functioning on coral reefs is to be made, there is much scope to develop a more concrete understanding of how traits link to individual processes and eventually the multifunctionality of the reef. Identifying overlapping traits, causation, and improving our ability to capture intra-species trait information will greatly advance this endeavor.
Data Availability Statement
The original contributions presented in the study are included in the article/Supplementary Material, further inquiries can be directed to the corresponding author/s.
Author Contributions
JH-H collected data, did the data analysis, and wrote the first draft of the manuscript. NG and DM helped to conceive the project, provided supervision, data analysis suggestions, and editorial comments. All authors contributed to the article and approved the submitted version.
Funding
This research was funded through a Doctoral Training Programme grant from NERC ENVISION (grant number: NE/L002604/1).
Conflict of Interest
The authors declare that the research was conducted in the absence of any commercial or financial relationships that could be construed as a potential conflict of interest.
Supplementary Material
The Supplementary Material for this article can be found online at: https://www.frontiersin.org/articles/10.3389/fmars.2021.640619/full#supplementary-material
References
Albert, C. H., Grassein, F., Schurr, F. M., Vieilledent, G., and Violle, C. (2011). When and how should intraspecific variability be considered in trait-based plant ecology? Perspectiv. Plant. Ecol. Evol. System. 13, 217–225. doi: 10.1016/j.ppees.2011.04.003
Allgeier, J. E., Adam, T. C., and Burkepile, D. E. (2017). The importance of individual and species-level traits for trophic niches among herbivorous coral reef fishes. Proc. Royal Soc. B Biol. Sci. 284:20170307. doi: 10.1098/rspb.2017.0307
Allgeier, J. E., Valdivia, A., Cox, C., and Layman, C. A. (2016). Fishing down nutrients on coral reefs. Nat. Commun. 7:12461. doi: 10.1038/ncomms12461
Amend, A. S., Martiny, A. C., Allison, S. D., Berlemont, R., Goulden, M. L., Lu, Y., et al. (2016). Microbial response to simulated global change is phylogenetically conserved and linked with functional potential. ISME J. 10, 109–118. doi: 10.1038/ismej.2015.96
Anjum, R. L., and Mumford, S. (2018). Causation in Science and Methods of Scientific Discovery. Oxford: Oxford University Press. doi: 10.1093/oso/9780198733669.001.0001
Ashtiani, M., Mirzaie, M., and Jafari, M. (2019). CINNA: an R/CRAN package to decipher central informative nodes in network analysis. Bioinformatics. 35, 1436–1437. doi: 10.1093/bioinformatics/bty819
Ashtiani, M., Salehzadeh-Yazdi, A., Razaghi-Moghadam, Z., Hennig, H., Wolkenhauer, O., Mirzaie, M., et al. (2018). A systematic survey of centrality measures for protein-protein interaction networks. BMC Syst. Biol. 12:80. doi: 10.1186/s12918-018-0598-2
Ban, S. S., Graham, N. A. J., and Connolly, S. R. (2014). Evidence for multiple stressor interactions and effects on coral reefs. Glob Change Biol. 2014, 681–697. doi: 10.1111/gcb.12453
Barneche, D. R., Ross Robertson, D., White, C. R., and Marshall, D. J. (2018). Fish reproductive-energy output increases disproportionately with body size. Science 360, 642–645. doi: 10.1126/science.aao6868
Barnett, L. A. K., Jacobsen, N. S., Thorson, J. T., and Cope, J. M. (2019). Realizing the potential of trait-based approaches to advance fisheries science. Fish Fisheries 20, 1034–1050. doi: 10.1111/faf.12395
Bellwood, D. R., Hoey, A. S., and Hughes, T. P. (2012). Human activity selectively impacts the ecosystem roles of parrotfishes on coral reefs. Proc. Royal Soc. B Biol. Sci. 279, 1621–1629. doi: 10.1098/rspb.2011.1906
Bellwood, D. R., Streit, R. P., Brandl, S. J., and Tebbett, S. B. (2019). The meaning of the term “function” in ecology: a coral reef perspective. Funct. Ecol. 33, 948–961. doi: 10.1111/1365-2435.13265
Beltramini, R. F., and Wolf, F. M. (1987). Meta-analysis: quantitative methods for research synthesis. J. Mark. Res. 24:452. doi: 10.2307/3151396
Beukhof, E., Dencker, T. S., Pecuchet, L., and Lindegren, M. (2019). Spatio-temporal variation in marine fish traits reveals community-wide responses to environmental change. Marine Ecol. Progr. Series 610, 205–222. doi: 10.3354/meps12826
Biro, P. A., Beckmann, C., and Stamps, J. A. (2010). Small within-day increases in temperature affects boldness and alters personality in coral reef fish. Proc. Royal Soc. B Biol. Sci. 277, 71–77. doi: 10.1098/rspb.2009.1346
Bolnick, D. I., Amarasekare, P., Araújo, M. S., Bürger, R., Levine, J. M., Novak, M., et al. (2011). Why intraspecific trait variation matters in community ecology. Trends Ecol. Evol. 26, 183–192. doi: 10.1016/j.tree.2011.01.009
Bozec, Y. M., O'Farrell, S., Bruggemann, J. H., Luckhurst, B. E., and Mumby, P. J. (2016). Tradeoffs between fisheries harvest and the resilience of coral reefs. Proc. Natl. Acad. Sci. U. S. A. 113, 4536–4541. doi: 10.1073/pnas.1601529113
Brandl, S. J., Emslie, M. J., and Ceccarelli, D. M. (2016). Habitat degradation increases functional originality in highly diverse coral reef fish assemblages. Ecosphere 7:e01557. doi: 10.1002/ecs2.1557
Brandl, S. J., Rasher, D. B., Côt,é, I. M., Casey, J. M., Darling, E. S., Lefcheck, J. S., et al. (2019). Coral reef ecosystem functioning: eight core processes and the role of biodiversity. Front. Ecol. Environ. 17, 445–454. doi: 10.1002/fee.2088
Caldwell, Z. R., Zgliczynski, B. J., Williams, G. J., and Sandin, S. A. (2016). Reef fish survey techniques: assessing the potential for standardizing methodologies. PLoS ONE 11:e0153066. doi: 10.1371/journal.pone.0153066
Casey, J. M., Meyer, C. P., Morat, F., Brandl, S. J., Planes, S., and Parravicini, V. (2019). Reconstructing hyperdiverse food webs: gut content metabarcoding as a tool to disentangle trophic interactions on coral reefs. Methods Ecol. Evol. 10, 1157–1170. doi: 10.1111/2041-210X.13206
Charrad, M., Ghazzali, N., Boiteau, V., and Niknafs, A. (2014). NbClust: an R package for determining the relevant number of clusters in a data set. J. Statist. Softw. 61, 1–36. doi: 10.18637/JSS.V061.I06
Cheetham, A. H., and Hazel, J. E. (1969). Binary (presence-absence) similarity coefficients. J. Paleontol. 43, 1130–1136.
Connolly, S. R., Keith, S. A., Colwell, R. K., and Rahbek, C. (2017). Process, mechanism, and modeling in macroecology. Trends Ecol. Evol. 32, 835–844. doi: 10.1016/j.tree.2017.08.011
Cramer, K. L., O'Dea, A., Clark, T. R., Zhao, J. X., and Norris, R. D. (2017). Prehistorical and historical declines in caribbean coral reef accretion rates driven by loss of parrotfish. Nat. Commun. 8:14160. doi: 10.1038/ncomms14160
Darling, E. S., Mcclanahan, T. R., and Côté, I. M. (2010). Combined effects of two stressors on kenyan coral reefs are additive or antagonistic, not synergistic. Conservation Lett. 3, 122–130. doi: 10.1111/j.1755-263X.2009.00089.x
Debouk, H., de Bello, F., and Sebastià, M.-T. (2015). Functional trait changes, productivity shifts and vegetation stability in mountain grasslands during a short-term warming. PLoS ONE 10:e0141899. doi: 10.1371/journal.pone.0141899
Des Roches, S., Post, D. M., Turley, N. E., Bailey, J. K., Hendry, A. P., Kinnison, M. T., et al. (2018). The ecological importance of intraspecific variation. Nat. Ecol. Evol. 2, 57–64. doi: 10.1038/s41559-017-0402-5
Devictor, V., Clavel, J., Julliard, R., Lavergne, S., Mouillot, D., Thuiller, W., et al. (2010). Defining and measuring ecological specialization. J. Appl. Ecol. 47, 15–25. doi: 10.1111/j.1365-2664.2009.01744.x
Diaz, S., and Cabido, M. (2001). Vive la différence: plant functional diversity matters to ecosystem processes. Trends Ecol. Evol. 16, 646–655. doi: 10.1016/S0169-5347(01)02283-2
Díaz, S., Purvis, A., Cornelissen, J. H. C., Mace, G. M., Donoghue, M. J., Ewers, R. M., et al. (2013). Functional traits, the phylogeny of function, and ecosystem service vulnerability. Ecol. Evol. 3, 2958–2975. doi: 10.1002/ece3.601
Doncheva, N. T., Assenov, Y., Domingues, F. S., and Albrecht, M. (2012). Topological analysis and interactive visualization of biological networks and protein structures. Nat. Protocols 7, 670–685. doi: 10.1038/nprot.2012.004
Drucker, A. M., Fleming, P., and Chan, A. W. (2016). Research techniques made simple: assessing risk of bias in systematic reviews. J. Invest. Dermatol. 136, e109–e114. doi: 10.1016/j.jid.2016.08.021
Duffy, J. E., Lefcheck, J. S., Stuart-Smith, R. D., Navarrete, S. A., and Edgar, G. J. (2016). Biodiversity enhances reef fish biomass and resistance to climate change. Proc. Natl. Acad. Sci. U. S. Am. 113, 6230–6235. doi: 10.1073/pnas.1524465113
Dunic, J. C., and Baum, J. K. (2017). Size structuring and allometric scaling relationships in coral reef fishes. J. Anim. Ecol. 86, 577–589. doi: 10.1111/1365-2656.12637
Estrada, E., and Rodríguez-Velázquez, J. A. (2005). Subgraph centrality in complex networks. Phys. Rev. E Statist. Non-linear Soft Matter Phys. 71, 1–9. doi: 10.1103/PhysRevE.71.056103
Ferrari, M. C. O., Munday, P. L., Rummer, J. L., Mccormick, M. I., Corkill, K., Watson, S. A., et al. (2015). Interactive effects of ocean acidification and rising sea temperatures alter predation rate and predator selectivity in reef fish communities. Glob. Change Biol. 21, 1848–1855. doi: 10.1111/gcb.12818
Floeter, S. R., Bender, M. G., Siqueira, A. C., and Cowman, P. F. (2018). Phylogenetic perspectives on reef fish functional traits. Biol. Rev. 93, 131–151. doi: 10.1111/brv.12336
Fox, R. J., and Bellwood, D. R. (2013). Niche partitioning of feeding microhabitats produces a unique function for herbivorous rabbitfishes (perciformes, siganidae) on coral reefs. Coral Reefs 32, 13–23. doi: 10.1007/s00338-012-0945-5
Friedman, H. (1982). Simplified determinations of statistical power, magnitude of effect and research sample sizes. Educ. Psychol. Measure. 42, 521:526. doi: 10.1177/001316448204200214
Goatley, C. H. R., and Bellwood, D. R. (2010). Biologically mediated sediment fluxes on coral reefs: sediment removal and off-reef transportation by the surgeonfish ctenochaetus striatus. Marine Ecol. Progr. Series 415, 237–245. doi: 10.3354/meps08761
Goatley, C. H. R., Bellwood, D. R., and Bellwood, O. (2010). Fishes on coral reefs: changing roles over the past 240 million years. Paleobiology 36, 415–427. doi: 10.1666/09035.1
Graham, N. A. J., Cinner, J. E., Norström, A. V., and Nyström, M. (2014). Coral reefs as novel ecosystems: embracing new futures. Curr. Opin. Environ. Sustain. Elsevier 2014, 9–14. doi: 10.1016/j.cosust.2013.11.023
Grime, J. (2006). Plant Strategies, Vegetation Processes, and Ecosystem Properties. Chichester: Wiley.
Gross, N., Robson, T. M., Lavorel, S., Albert, C., Le Bagousse-Pinguet, Y., and Guillemin, R. (2008). Plant response traits mediate the effects of subalpine grasslands on soil moisture. New Phytol. 180, 652–662. doi: 10.1111/j.1469-8137.2008.02577.x
Hoegh-Guldberg, O., Mumby, P. J., Hooten, A. J., Steneck, R. S., Greenfield, P., Gomez, E., et al. (2007). Coral reefs under rapid climate change and ocean acidification. Science 318, 1737–1742. doi: 10.1126/science.1152509
Hoey, A. S., and Bellwood, D. R. (2009). Limited functional redundancy in a high diversity system: single species dominates key ecological process on coral reefs. Ecosystems 12, 1316–1328. doi: 10.1007/s10021-009-9291-z
Hughes, T. P. (1994). Catastrophes, phase shifts, and large-scale degradation of a caribbean coral reef. Science 199, 1302–1310. doi: 10.1126/science.265.5178.1547
Hughes, T. P., Barnes, M. L., Bellwood, D. R., Cinner, J. E., Cumming, G. S., Jackson, J. B. C., et al. (2017). Coral reefs in the anthropocene. Nat. Res. 31, 82–90. doi: 10.1038/nature22901
Hughes, T. P., Graham, N. A. J., Jackson, J. B. C., Mumby, P. J., and Steneck, R. S. (2010). Rising to the challenge of sustaining coral reef resilience. Trends Ecol. Evol. 25, 633–642. doi: 10.1016/j.tree.2010.07.011
Humphries, A. T., McClanahan, T. R., and McQuaid, C. D. (2014). Differential impacts of coral reef herbivores on algal succession in Kenya. Marine Ecol. Progr. Series 504, 119–132. doi: 10.3354/meps10744
Jacob, U., Thierry, A., Brose, U., Arntz, W. E., Berg, S., Brey, T., et al. (2011). The role of body size in complex food webs: a cold case. Adv. Ecol. Res. 45, 181–223. doi: 10.1016/B978-0-12-386475-8.00005-8
Keith, S. A., Baird, A. H., Hobbs, J.-P. A., Woolsey, E. S., Hoey, A. S., Fadli, N., et al. (2018). Synchronous behavioural shifts in reef fishes linked to mass coral bleaching. Nat. Climate Change 8, 986–991. doi: 10.1038/s41558-018-0314-7
Kenkel, N. C. (2006). On selecting an appropriate multivariate analysis. Can. J. Plant Sci. 86, 663–676. doi: 10.4141/P05-164
Keppeler, F. W., Montaña, C. G., and Winemiller, K. O. (2020). The relationship between trophic level and body size in fishes depends on functional traits. Ecol. Monogr. 90, 1–19. doi: 10.1002/ecm.1415
Killen, S. S., Marras, S., Nadler, L., and Domenici, P. (2017). The role of physiological traits in assortment among and within fish shoals. Philos. Trans. Royal Soc. B Biol. Sci. 372:20160233. doi: 10.1098/rstb.2016.0233
Kiørboe, T., Visser, A., and Andersen, K. H. (2018). A trait-based approach to ocean ecology. ICES J. Marine Sci. 75, 1849–1863. doi: 10.1093/icesjms/fsy090
Kissling, W. D., Walls, R., Bowser, A., Jones, M. O., Kattge, J., Agosti, D., et al. (2018). Towards global data products of essential biodiversity variables on species traits. Nat. Ecol. Evol. 2, 1531–1540. doi: 10.1038/s41559-018-0667-3
Kublicki, M. (2010). GASPAR General Approach to Species-Abundance Relationships in a Context of Global Change, Reef Fish Species as a Model. Centre for the Synthesis and Analysis of Biodiversity.
Lavorel, S., and Garnier, E. (2002). Predicting changes in community composition and ecosystem functioning from plant traits: revisiting the Holy Grail. Funct. Ecol. 2002, 545–556. doi: 10.1046/j.1365-2435.2002.00664.x
Li, D., Wang, Z., Wu, S., Miao, Z., Du, L., and Duan, Y. (2020). Automatic recognition methods of fish feeding behavior in aquaculture: a review. Aquaculture 528:735508. doi: 10.1016/j.aquaculture.2020.735508
Lokrantz, J., Nyström, M., Thyresson, M., and Johansson, C. (2008). The non-linear relationship between body size and function in parrotfishes. Coral Reefs 27, 967–974. doi: 10.1007/s00338-008-0394-3
Loreau, M., Naeem, S., Inchausti, P., Bengtsson, J., Grime, J. P., Hector, A., et al. (2001). Biodiversity and ecosystem functioning: current knowledge and future challenges. Science 294, 804–808. doi: 10.1126/science.1064088
MacNeil, M. A., Graham, N. A. J., Cinner, J. E., Wilson, S. K., Williams, I. D., Maina, J., et al. (2015). Recovery potential of the world's coral reef fishes. Nature 520, 341–344. doi: 10.1038/nature14358
Madin, J. S., Anderson, K. D., Andreasen, M. H., Bridge, T. C. L., Cairns, S. D., Connolly, S. R., et al. (2016b). The coral trait database, a curated database of trait information for coral species from the global oceans. Sci. Data 3:160017. doi: 10.1038/sdata.2016.17
Madin, J. S., Hoogenboom, M. O., Connolly, S. R., Darling, E. S., Falster, D. S., Huang, D., et al. (2016a). A trait-based approach to advance coral reef science. Trends Ecol. Evol. 31, 419–428. doi: 10.1016/j.tree.2016.02.012
Maire, E., Grenouillet, G., Brosse, S., and Villéger, S. (2015). How many dimensions are needed to accurately assess functional diversity? a pragmatic approach for assessing the quality of functional spaces. Glob. Ecol. Biogeogr. 24, 728–740. doi: 10.1111/geb.12299
Mbaru, E. K., Graham, N. A. J., McClanahan, T. R., and Cinner, J. E. (2019). Functional traits illuminate the selective impacts of different fishing gears on coral reefs. J. Appl. Ecol. 57, 241–252. doi: 10.1111/1365-2664.13547
McClure, E., Richardson, L., Graba-Landry, A., Loffler, Z., Russ, G., Hoey, A., et al. (2019). Cross-Shelf differences in the response of herbivorous fish assemblages to severe environmental disturbances. Diversity 11:23. doi: 10.3390/d11020023
McLean, M., Auber, A., Graham, N. A. J., Houk, P., Villéger, S., Violle, C., et al. (2019). Trait structure and redundancy determine sensitivity to disturbance in marine fish communities. Glob. Change Biol. 25, 3424–3437. doi: 10.1111/gcb.14662
McLean, M., Mouillot, D., Lindegren, M., Engelhard, G., Villéger, S., Marchal, P., et al. (2018). A climate-driven functional inversion of connected marine ecosystems. Curr. Biol. 28, 3654–3660.e3. doi: 10.1016/j.cub.2018.09.050
Morais, R. A., and Bellwood, D. R. (2020). Principles for estimating fish productivity on coral reefs. Coral Reefs 39, 1221–1231. doi: 10.1007/s00338-020-01969-9
Morais, R. A., Depczynski, M., Fulton, C. J., Marnane, M. J., Narvaez, P., Huertas, V., et al. (2020). Severe coral loss shifts energetic dynamics on a coral reef. Funct. Ecol. 34, 1507–1518. doi: 10.1111/1365-2435.13568
Moretti, M., Dias, A. T. C., de Bello, F., Altermatt, F., Chown, S. L., Azcárate, F. M., et al. (2017). Handbook of protocols for standardized measurement of terrestrial invertebrate functional traits. Funct. Ecol. 31, 558–567. doi: 10.1111/1365-2435.12776
Mouillot, D., Graham, N. A. J., Villéger, S., Mason, N. W. H., and Bellwood, D. R. (2013). A functional approach reveals community responses to disturbances. Trends Ecol. Evol. 2013, 167–177. doi: 10.1016/j.tree.2012.10.004
Mouillot, D., Villéger, S., Parravicini, V., Kulbicki, M., Arias-González, J. E., Bender, M., et al. (2014). Functional over-redundancy and high functional vulnerability in global fish faunas on tropical reefs. Proc. Natl. Acad. Sci. U. S. A. 111, 13757–13762. doi: 10.1073/pnas.1317625111
Mumby, P. J., Perry, C. T., Carricart-Ganivet, J. P., Kennedy, E. V., Wisshak, M., Form, A. U., et al. (2013). Avoiding coral reef functional collapse requires local and global action. Curr. Biol. 23, 912–918. doi: 10.1016/j.cub.2013.04.020
Munday, P. L., Gagliano, M., Donelson, J. M., Dixson, D. L., and Thorrold, S. R. (2011). Ocean acidification does not affect the early life history development of a tropical marine fish. Marine Ecol. Progr. Series 423, 211–221. doi: 10.3354/meps08990
Nakagawa, S., and Cuthill, I. C. (2007). Effect size, confidence interval and statistical significance: a practical guide for biologists. Biol. Rev. 82, 591–605. doi: 10.1111/j.1469-185X.2007.00027.x
Pandolfi, J. M. (2015). Incorporating uncertainty in predicting the future response of coral reefs to climate change. Ann. Rev. Ecol. Evol. System. 46, 281–303. doi: 10.1146/annurev-ecolsys-120213-091811
Paradis, E., Blomber, S., Bolker, B., Brown, J., Claude, J., Cuong Hoa, S., et al. (2019). Package ‘Ape’: Analyses of Phylogenetics and Evolution Depends R (>= 3.2.0). Comprehensive R Archive Network.
Parravicini, V., Casey, J. M., Schiettekatte, N. M. D., Brandl, S. J., Pozas-Schacre, C., Carlot, J., et al. (2020). Delineating reef fish trophic guilds with global gut content data synthesis and phylogeny. PLoS Biol. 18:e3000702. doi: 10.1371/journal.pbio.3000702
Pérez-Harguindeguy, N., Díaz, S., Garnier, E., Lavorel, S., Poorter, H., Jaureguiberry, P., et al. (2013). New handbook for standardised measurement of plant functional traits worldwide. Austr. J. Botany 61, 167–234. doi: 10.1071/BT12225
Pullin, A. S., and Stewart, G. B. (2006). Guidelines for systematic review in conservation and environmental management. Conserv. Biol. 20, 1647–1656. doi: 10.1111/j.1523-1739.2006.00485.x
Quimbayo, J. P., Silva, F. C., Mendes, T. C., Ferrari, D. S., Danielski, S. L., Bender, M. G., et al. (2021). Life-history traits, geographical range and conservation aspects of reef fishes from the Atlantic and Eastern Pacific. Ecology. e03298. doi: 10.1002/ecy.3298. [Epub ahead of print].
R Core Team (2018). R: The R Project for Statistical Computing. Available online at: https://www.r-project.org/ (accessed Febraury 12, 2019).
Rasher, D. B., Hoey, A. S., and Hay, M. E. (2013). Consumer diversity interacts with prey defenses to drive ecosystem function. Ecology 94, 1347–1358. doi: 10.1890/12-0389.1
Richardson, L. E., Graham, N. A. J., Pratchett, M. S., Eurich, J. G., and Hoey, A. S. (2018). Mass coral bleaching causes biotic homogenization of reef fish assemblages. Glob. Change Biol. 24, 3117–3129. doi: 10.1111/gcb.14119
Richardson, L. E., Graham, N. A. J., Pratchett, M. S., and Hoey, A. S. (2017). Structural complexity mediates functional structure of reef fish assemblages among coral habitats. Environ. Biol. Fishes 100, 193–207. doi: 10.1007/s10641-016-0571-0
Rosenthal, R., and DiMatteo, M. R. (2001). Meta-analysis: recent developments in quantitative methods for literature reviews. Ann. Rev. Psychol. 52, 59–82. doi: 10.1146/annurev.psych.52.1.59
Salguero-Gómez, R., Violle, C., Gimenez, O., and Childs, D. (2018). Delivering the promises of trait-based approaches to the needs of demographic approaches, and vice versa. Funct. Ecol. 32, 1424–1435. doi: 10.1111/1365-2435.13148
Schiettekatte, N. M. D., Barneche, D. R., Villéger, S., Allgeier, J. E., Burkepile, D. E., Brandl, S. J., et al. (2020). Nutrient limitation, bioenergetics and stoichiometry: a new model to predict elemental fluxes mediated by fishes. Funct. Ecol. 34, 1857–1869. doi: 10.1111/1365-2435.13618
Schmitz, O. (2017). Predator and prey functional traits: understanding the adaptive machinery driving predator–prey interactions. F1000Research 6:1767. doi: 10.12688/f1000research.11813.1
Sitters, H., Di Stefano, J., Christie, F., Swan, M., and York, A. (2016). Bird functional diversity decreases with time since disturbance: does patchy prescribed fire enhance ecosystem function? Ecol. Appl. 26, 115–127. doi: 10.1890/14-1562
Stearns, S. (1976). Life-history tactics: a review of the ideas. Quart. Rev. Biol. 51, 3–47. doi: 10.1086/409052
Streit, R. P., Hoey, A. S., and Bellwood, D. R. (2015). Feeding characteristics reveal functional distinctions among browsing herbivorous fishes on coral reefs. Coral Reefs 34, 1037–1047. doi: 10.1007/s00338-015-1322-y
Stuart-Smith, R. D., Bates, A. E., Lefcheck, J. S., Duffy, J. E., Baker, S. C., Thomson, R. J., et al. (2013). Integrating abundance and functional traits reveals new global hotspots of fish diversity. Nature 501, 539–542. doi: 10.1038/nature12529
Suding, K. N., Lavorel, S., Chapin, F. S., Cornelissen, J. H. C., Díaz, S., Garnier, E., et al. (2008). Scaling environmental change through the community-level: a trait-based response-and-effect framework for plants. Glob. Change Biol. 14, 1125–1140. doi: 10.1111/j.1365-2486.2008.01557.x
Thorson, J. T., Munch, S. B., Cope, J. M., and Gao, J. (2017). Predicting life history parameters for all fishes worldwide. Ecol. Appl. 27, 2262–2276. doi: 10.1002/eap.1606
Tuya, F., Herrero-Barrencua, A., Bosch, N. E., Abreu, A. D., and Haroun, R. (2018). Reef fish at a remote tropical island (Principe Island, Gulf of Guinea): disentangling taxonomic, functional and phylogenetic diversity patterns with depth. Marine Freshw. Res. 69, 395–402. doi: 10.1071/MF17233
Villéger, S., Brosse, S., Mouchet, M., Mouillot, D., and Vanni, M. J. (2017). Functional ecology of fish: current approaches and future challenges. Aquatic Sci. 79, 783–801. doi: 10.1007/s00027-017-0546-z
Villon, S., Mouillot, D., Chaumont, M., Darling, E. S., Subsol, G., Claverie, T., et al. (2018). Ecological informatics a deep learning method for accurate and fast identi fi cation of coral reef fi shes in underwater images. Ecol. Inform. 48, 238–244. doi: 10.1016/j.ecoinf.2018.09.007
Violle, C., and Jiang, L. (2009). Towards a trait-based quantification of species niche. J. Plant Ecol. 2, 87–93. doi: 10.1093/jpe/rtp007
White, J. R., Meekan, M. G., McCormick, M. I., and Ferrari, M. C. O. (2013). A comparison of measures of boldness and their relationships to survival in young fish. PLoS ONE 8:900. doi: 10.1371/journal.pone.0068900
Woodhead, A. J., Hicks, C. C., Norström, A. V., Williams, G. J., and Graham, N. A. J. (2019). Coral reef ecosystem services in the anthropocene. Funct. Ecol. 33, 1023–1034. doi: 10.1111/1365-2435.13331
Zaneveld, J. R., Burkepile, D. E., Shantz, A. A., Pritchard, C. E., McMinds, R., Payet, J. P., et al. (2016). Overfishing and nutrient pollution interact with temperature to disrupt coral reefs down to microbial scales. Nat. Commun. 7:11833. doi: 10.1038/ncomms11833
Keywords: ecosystem processes, environmental disturbances, functional diversity, coral reef ecology, ecosystem function, trait-based ecology, systematic review
Citation: Hadj-Hammou J, Mouillot D and Graham NAJ (2021) Response and Effect Traits of Coral Reef Fish. Front. Mar. Sci. 8:640619. doi: 10.3389/fmars.2021.640619
Received: 11 December 2020; Accepted: 26 February 2021;
Published: 25 March 2021.
Edited by:
Hajime Kayanne, The University of Tokyo, JapanReviewed by:
Sergio R. Floeter, Federal University of Santa Catarina, BrazilAndrew Robert Halford, Pacific Community (SPC), New Caledonia
Copyright © 2021 Hadj-Hammou, Mouillot and Graham. This is an open-access article distributed under the terms of the Creative Commons Attribution License (CC BY). The use, distribution or reproduction in other forums is permitted, provided the original author(s) and the copyright owner(s) are credited and that the original publication in this journal is cited, in accordance with accepted academic practice. No use, distribution or reproduction is permitted which does not comply with these terms.
*Correspondence: Jeneen Hadj-Hammou, j.hadj-hammou@lancaster.ac.uk