- 1Department of Earth and Environment, Florida International University, Miami, FL, United States
- 2Institute of Environment, Florida International University, Miami, FL, United States
- 3Florida Fish and Wildlife Conservation Commission, Florida Fish and Wildlife Research Institute, St. Petersburg, FL, United States
- 4South Florida Natural Resources Center, National Park Service, Homestead, FL, United States
- 5Atlantic Oceanographic and Meteorological Laboratory, National Oceanic and Atmospheric Administration, Miami, FL, United States
Seagrasses are threatened worldwide due to anthropogenic and natural disturbances disrupting the multiple feedbacks needed to maintain these ecosystems. If the disturbance is severe enough, seagrass systems may undergo a regime shift to a degraded system state that is resistant to recovery. In Florida Bay, Florida, United States, two recent, large-scale disturbances (a drought-induced seagrass die-off in 2015 and Hurricane Irma in 2017) have caused 8,777 ha of seagrass beds to degrade into a turbid, unvegetated state, causing a large sediment plume. Using satellite imagery digitization and long-term seagrass cover data, we investigate the expansion of this sediment plume between 2008 and 2020 and the potential interaction of this sediment plume with seagrass recovery in two focal basins in Florida Bay affected by the die-off, Johnson and Rankin. The average size of the sediment plume increased by 37% due to the die-off and Hurricane Irma, increasing from an average of 163.5 km2 before the disturbances to an average of 223.5 km2. The expansion of the plume was basin-specific, expanding into Johnson after the 2015 seagrass die-off with expansive and long-lasting effects, but only expanding into Rankin after Hurricane Irma with less severe and short-term effects. Furthermore, the sediment plume was negatively correlated with seagrass cover in Johnson, but held no relationship with seagrass cover in Rankin. Thus, different disturbances can act upon seagrass ecosystems at varying scales with varying consequences. This study illustrates the advantage of combining satellite imagery with field data to monitor disturbances as well as highlights the importance of investigating disturbances of seagrass ecosystems at various scales to comprehend seagrass resilience in the context of future extreme events.
Introduction
Seagrass communities are a vital part of coastal ecosystems worldwide, providing many ecosystem services such as carbon storage (Mcleod et al., 2011; Fourqurean et al., 2012; Duarte et al., 2013), habitat for fish and other fauna (Gillanders, 2006; Unsworth et al., 2019), sediment stabilization (Bos et al., 2007), and primary production (Duarte and Chiscano, 1999). However, seagrasses around the world are declining due to anthropogenic and natural disturbances, threatening the balance of these shallow water ecosystems (Orth et al., 2006; Waycott et al., 2009). One of the main causes of seagrass decline is the disruption of natural feedbacks that promote seagrass growth and sustenance (Duarte, 2002; Orth et al., 2006). Stabilizing feedbacks (i.e., negative feedback loops) control seagrass ecosystems at multiple scales (Maxwell et al., 2017; O’Brien et al., 2017), from supporting mesograzer populations at the meter scale (Valentine and Duffy, 2006; Duffy et al., 2015) to genetic diversity at the ecosystem scale (Procaccini et al., 2007; Reynolds et al., 2013). For example, grazing by sea urchins results in the reduction of aboveground seagrass biomass, which increases the predation pressure on sea urchins. The predation pressure leads to a decrease in urchin population, thus allowing for the seagrass to recover (Heck and Valentine, 1995). External disturbances can result in the disruption of one or more of these stabilizing feedbacks, creating destabilizing feedbacks (i.e., positive feedback loops) that drive seagrass decline (Nyström et al., 2012; Maxwell et al., 2017; O’Brien et al., 2017). An example of a destabilizing feedback is the decline of seagrass, which increases sediment resuspension events. The water column becomes more turbid, which in turn lowers the light available for photosynthesis and leads to further seagrass loss and further sediment resuspension (Nyström et al., 2012; Maxwell et al., 2017; O’Brien et al., 2017; Figure 1).
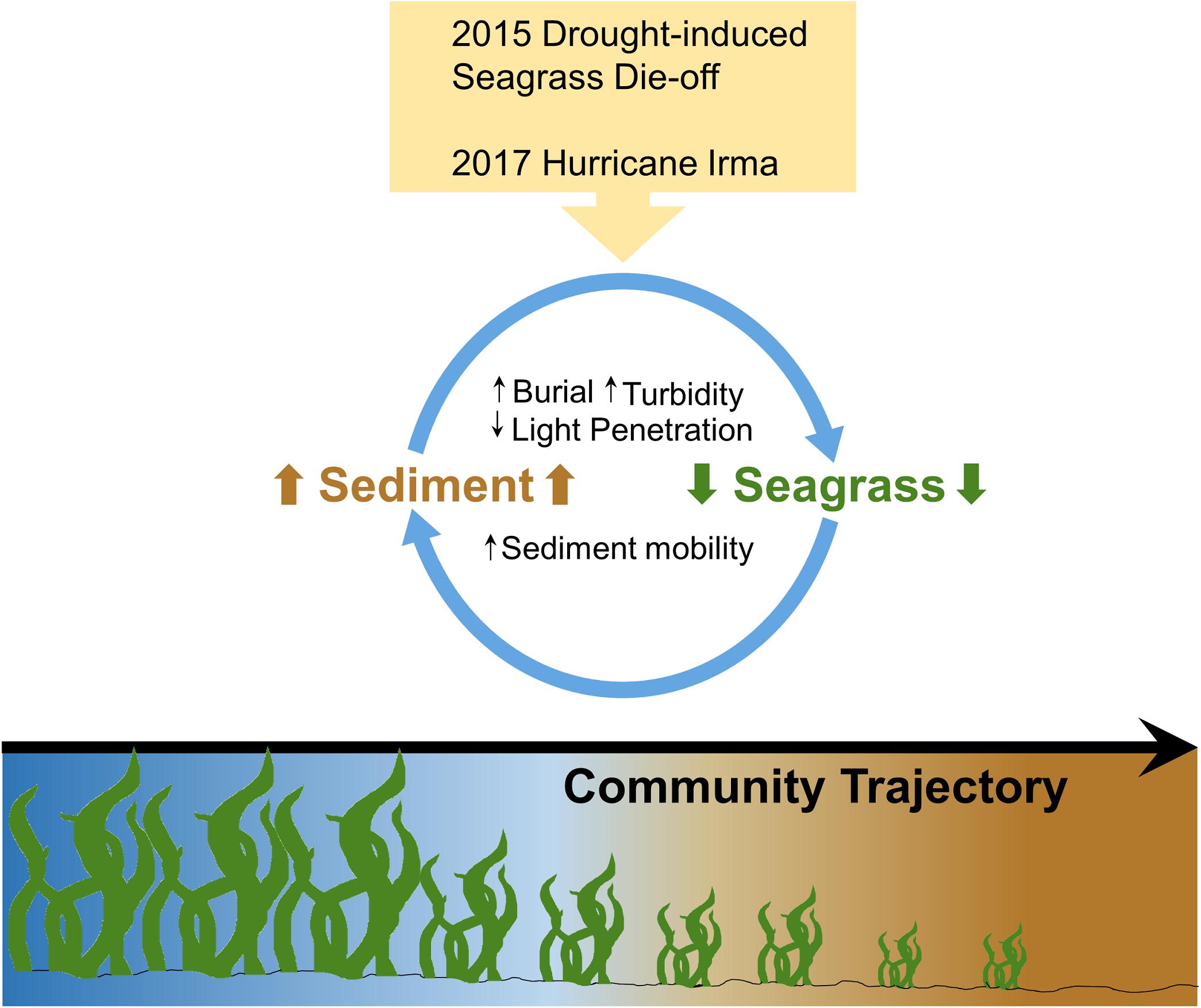
Figure 1. Conceptual diagram illustrating the turbidity feedback loop within Florida Bay seagrass ecosystems. Two large disturbances (a 2015 seagrass die-off and the 2017 Hurricane Irma) caused a reduction in seagrass cover, which increased sediment mobility and thus, the amount of sediment in the water column, which in turn decreased light penetration while increasing turbidity and burial (Figure adapted from Nyström et al., 2012).
How seagrass ecosystems respond to these external disturbances is dependent upon the frequency and severity of the disturbance (O’Brien et al., 2017). Strong, or frequent disturbances can result in regime shifts, or a change from one ecosystem state to another, at the spatial scale of the disturbance (Nyström et al., 2012; O’Brien et al., 2017). For example, removal of seagrass due to propeller scarring changes the ecosystem state within the width of the scar (often <1 m) from vegetated to unvegetated. Without further disturbance, the seagrass recovers within a year and reaches the original ecosystem state within 6 years (Dawes et al., 1997; Kenworthy et al., 2002). However, if the disturbance is severe or frequent enough, ecosystems can become locked into a degraded state through the creation of new stabilizing feedbacks. If the destabilizing feedback loops are strong enough, the system will experience a lag in recovery (i.e., hysteresis) and may enter an alternative stable state (Scheffer et al., 2001; Beisner et al., 2003; Petraitis et al., 2009; Nyström et al., 2012).
Seagrass ecosystems are particularly vulnerable to changes into alternative stable states due to the number and strength of feedbacks within these systems (Maxwell et al., 2017). One example of the long-term impact that hysteresis can have on a seagrass ecosystem is the Dutch Wadden Sea (Scheffer et al., 2001; van der Heide et al., 2007). The construction of a large dam and a seagrass wasting disease in the 1930s resulted in the collapse of large eelgrass (Zostera marina) meadows, which led to an unvegetated, turbid alternative stable state that still persists despite restoration efforts (van Katwijk and Hermus, 2000; van Katwijk et al., 2000; van der Heide et al., 2007). Unfortunately, the number of coastal ecosystems experiencing shifts to degraded alternative stable states has risen since the mid 20th century (Duarte et al., 2009; Carstensen et al., 2011; Maxwell et al., 2017). Proper management of coastal ecosystems, specifically seagrass ecosystems, requires more information on the causes and impacts of such regime shifts.
Florida Bay, the estuary between the Florida mainland and the Florida Keys, has a long history of anthropogenic and natural disturbances, including the reduction of freshwater inputs from water management practices, altered water circulation associated with the completion of the Flagler Over-Sea Railroad in 1912, and a number of hurricanes and tropical storms (Fourqurean and Robblee, 1999). Such disturbances have resulted in a reduced exchange between Florida Bay and the Atlantic Ocean, increased water residence time, changes in water circulation patterns, higher salinities, more frequent algal blooms, and decreased seagrass diversity (i.e., an increase in Thalassia testudinum-dominated communities, Rudnick et al., 2005; Madden et al., 2009). A combination of high bottom water temperatures, hypersalinity and prolonged bottom water anoxia caused a large-scale seagrass die-off between 1987 and 1991 that affected 27,000 ha of T. testudinum, and led to loss of submerged aquatic vegetation (SAV) coverage, increased water column production and turbidity, and trophic shifts (Robblee et al., 1991; Fourqurean and Robblee, 1999). After nearly a decade of persistent algal blooms, the system required an additional 6–10 years to return to pre-die-off levels of T. testudinum coverage (Hall et al., 2016). However, a second drought-induced seagrass die-off occurred in 2015, leading to another potential regime shift (Hall et al., 2016; Figure 2). The region was disturbed again in 2017, when Hurricane Irma passed through Florida Bay as a category 4 hurricane, impacting areas of Florida Bay and the Florida Keys (Wilson et al., 2020; Figure 2). Large and frequent disturbance events such as these could induce a state of turbidity that influences the growth and stability of seagrass habitats (Figure 1).
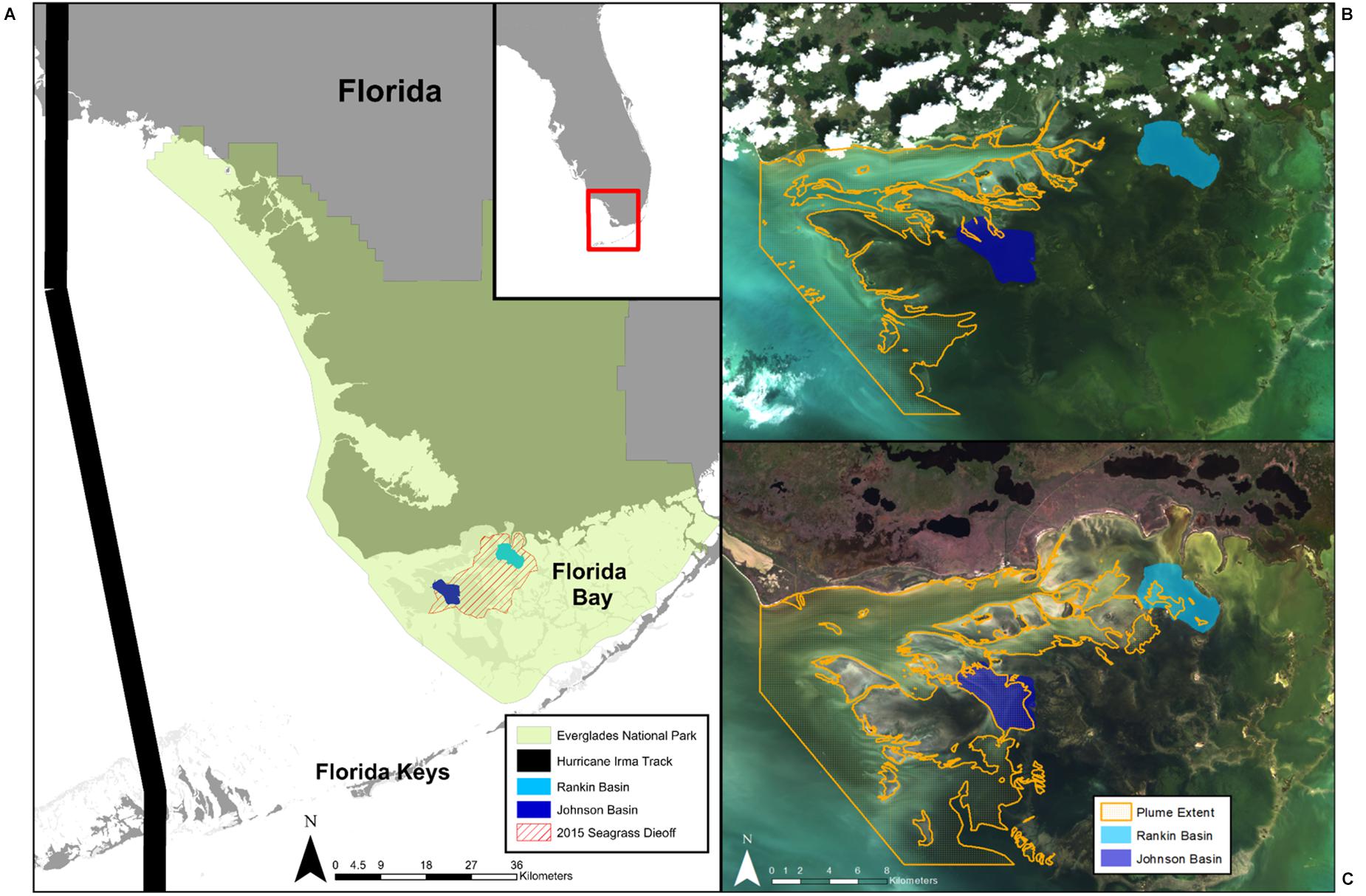
Figure 2. (A) Map of Florida Bay showing the location of our two study basins (Rankin and Johnson), the extent of the 2015 seagrass die-off, and the track of Hurricane Irma in 2017. Everglades National Park is denoted by green shading. Extent of the sediment plume overlaid over satellite imagery shows the (B) smallest (119.6 km2; 10/03/2009), and (C) largest plume areas (249.2 km2; 11/29/2018) observed in the study.
In this article, we describe the expansion of a heretofore undocumented sediment plume in the western region of Florida Bay in relation to these two recent disturbances: the 2015 seagrass die-off and Hurricane Irma in 2017. Furthermore, we investigate the potential interaction of the sediment plume with seagrass recovery. Using a remote sensing approach, we addressed the following questions: (a) To what extent did the sediment plume expand after each of the two disturbances (pre vs. post comparisons)? (b) Which disturbance had a greater effect on the expanse of the plume? and (c) Is there an interaction between sediment plume coverage and changes in seagrass cover? We addressed these questions by delineating changes in plume extent over 2008–2020 and in relation to the timing of the two disturbances using satellite imagery. We then compared changes in plume extent relative to long term seagrass cover data across two focal basins of interest, Johnson and Rankin, both affected and undergoing recovery from the 2015 seagrass event. Based on these data, we discuss the potential impact of this expanding sediment plume on seagrass communities throughout Florida Bay and what to expect in the future.
Materials and Methods
Study Site
Florida Bay is the largest estuary in Florida (2,200 km2), located at the southern end of the Florida peninsula and of Everglades National Park (ENP, Figure 2). The bay consists of a patchwork of shallow interconnected basins (1–2 m depth), mud banks (<0.5 m depth), seagrass meadows, mangrove islands, and narrow tidal channels. Florida Bay has restricted water exchange and high residence time, which can make regions of the bay prone to hypersalinity (Nuttle et al., 2000; Rudnick et al., 2005). Importantly, Florida Bay has undergone major changes over the past century as a result of anthropogenic impacts associated with the construction of the railroad across the Florida Keys and drainage and impoundment of freshwater wetlands upstream (Fourqurean and Robblee, 1999), resulting in increased salinity, decreased water exchange, and changes in benthic macrophyte communities (Rudnick et al., 2005; Madden et al., 2009).
Today, a large portion of Florida Bay functions as a reverse estuary with chronic hypersalinity conditions prevailing in the north-central part of the bay during the low precipitation and freshwater inflow periods of the dry season (December–May; Kelble et al., 2007). Relative to historical conditions, freshwater flows have been reduced by 60%, with nearshore present-day salinities being 5–20 ppt higher than pre-drainage (Marshall et al., 2009). These conditions make the bay vulnerable to drought events, which in 1987 and 2015 resulted in massive seagrass die-off events, affecting approximately 30% of the bay (Zieman et al., 1999; Hall et al., 2016). Two basins, Johnson and Rankin, were chosen as focal basins for this study because they are located in the north-central part of the bay affected by the 2015 die-off, and because long long-term seagrass cover monitoring data exists, which are useful to examine recovery trends (Hall et al., 2016; Figure 2). Additionally, the basins were affected by Hurricane Irma, which passed through Florida Bay as a category 4 hurricane in September 2017, disturbing benthic communities and significantly altering the circulation of water in the bay (Liu et al., 2020).
Satellite Imagery Processing
LandSat imagery from three LandSat missions (LandSat 5, LandSat 7, and LandSat 8)1 were used to map the sediment plume. LandSat satellites are a very popular tool in coastal mapping due to a relatively short revisit rate (2 weeks), high resolution (30 m), long time series (LandSat 5 was launched in 1985), and availability. LandSat satellites collect data in 7 bands across the visible and infrared spectrums. Two LandSat images per year from 2008 to 2020 were used to map the plume expansion for a total of 24 images. 2008 was chosen as the early cutoff since it matches the time series of total seagrass cover data provided by the Florida Fish and Wildlife Commission Fish and Wildlife Research Institute (FWC-FWRI). Google Earth Engine, an online coding platform, was used to process each satellite image (Gorelick et al., 2017). Google Earth Engine is a popular tool for seagrass mapping at regional scales due to its availability, ease of use, and batch processing that allows multiple satellite images to be analyzed at once (Lyons et al., 2012; Zhang et al., 2019; Wang et al., 2020).
Atmospherically and geometrically corrected LandSat images (courtesy of the U.S. Geological Survey) were loaded into Google Earth Engine to mask clouds, land, and shallow banks. Masking each image removes the areas of the satellite image that are not the focus of the study. Images were chosen based on a visual inspection of cloud cover, where images needed to have less than 10% cloud cover to be considered. Clouds were masked via the U.S. Geological Survey (USGS) quality assessment algorithm, which uses the CFMask algorithm (Foga et al., 2017) to calculate pixels with cloud cover and shadow. Land and shallow banks were masked using a Florida Bay Basin shapefile provided by FWC-FWRI (Figure 3A). Banks were masked due to the lack of seagrass data and difficulty of differentiating sediment plume from bottom. Masked LandSat images were downloaded from Google Earth Engine, keeping the bands in the visible and near-infrared spectrums.
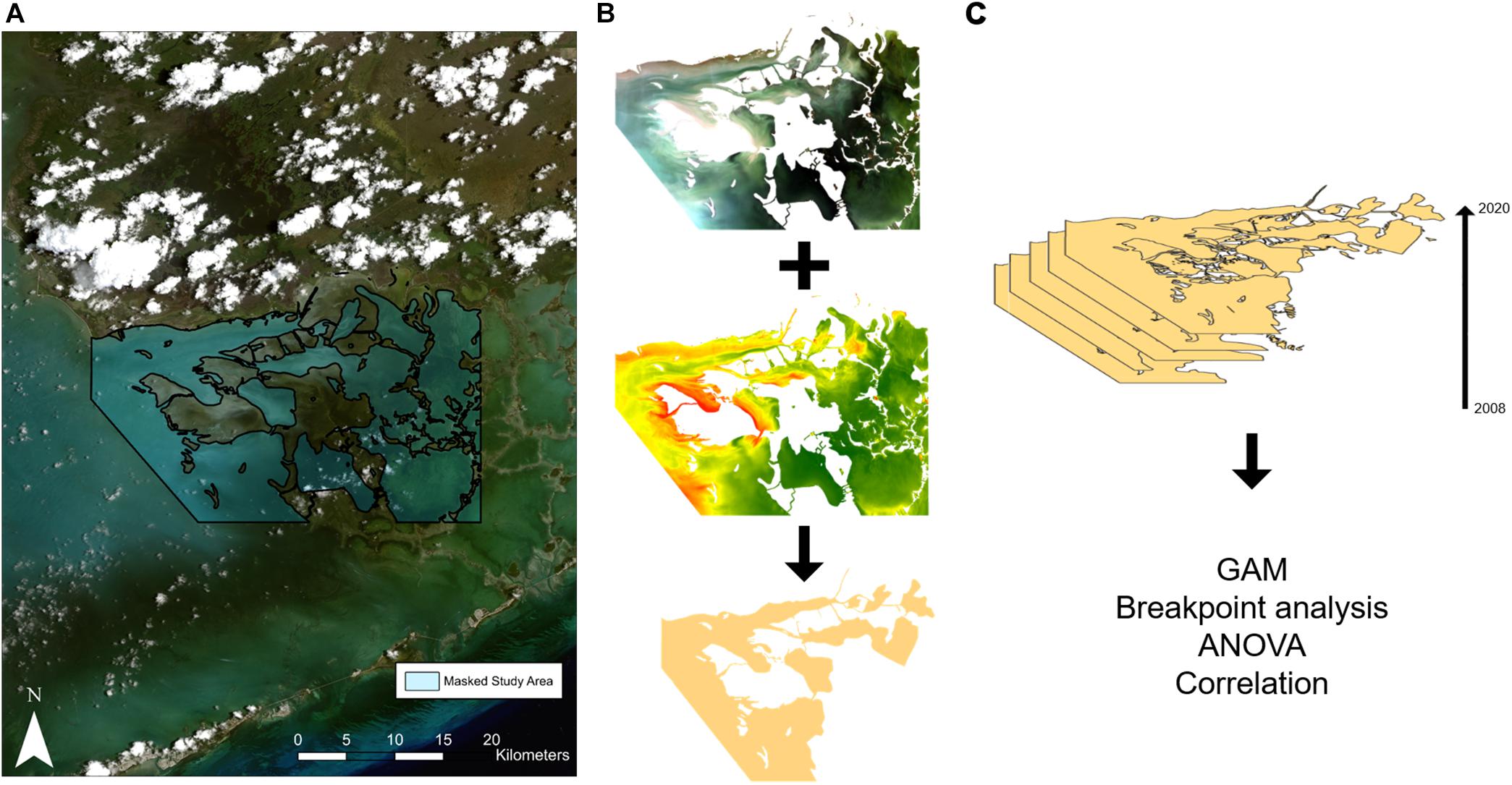
Figure 3. Illustration of the methods employed in this study. LandSat images were loaded into Google Earth Engine and (A) masked to remove clouds, land, and shallow banks. (B) The masked satellite images and an approximate suspended sediment concentration (SSC) map were downloaded used for sediment plume delineation. (C) A total of 24 images were delineated from 2008 to 2020, GAMs were used to model the expansion of the plume, breakpoint analysis was used to determine a significant change in sediment plume size while ANOVAs and correlation analysis were used to determine the impact of the sediment plume on seagrass recovery.
Sediment Plume Delineation
The areal extent of the sediment plume was delineated using manual digitalization. Manual digitization, also known as photo interpretation, has been used in coastal mapping for many decades (Roelfsema et al., 2009) and continues to be a popular method of coastal ecosystem mapping (Sherwood et al., 2017). Manual delineation was used in this study due to a lack of field training data as well as the presence of optically similar areas (i.e., sandy bottom vs. sediment plume). Two image interpreters were trained to delineate two classes: sediment plume with no light penetration (i.e., the bottom was not visible) and sediment plume with some light penetration (i.e., the bottom was visible; Figure 3B). Algal blooms within our area of study were not delineated. Accuracy assessment was performed by comparing delineations from 2017 to 2020 with turbidity measured from grab samples taken within the area of the plume by ENP. A turbidity measurement of >8 NTU was considered turbid, corresponding to an average Secchi depth of less than 1 m (Effler, 1988).
To aid in delineation, approximate suspended sediment concentration (SSC) was mapped using the algorithm developed in Islam et al. (2001). This algorithm assumes a linear relationship between the red band and the sediment concentration and was chosen because it best visually represented the sediment plume in Florida Bay out of several algorithms considered by Pereira et al. (2019). This study utilized delineation instead of an algorithm derived from satellite data to map the sediment plume because the goal of this study was to map the extent of the plume, not SSC. Furthermore, the shallow waters and algal blooms within Florida Bay make isolating a sediment plume difficult and require an algorithm to be derived from extensive field sampling, which was not available for this study. Future work will focus on building upon the work done by Hajigholizadeh and Melesse (2017) to create an algorithm and threshold that maps the sediment plume within Florida Bay.
Seagrass Cover
Seagrass data was obtained from the Fish Habitat Assessment Program (FHAP), established through the Comprehensive Everglades Restoration Plan’s (CERP) Restoration, Coordination and Verification (RECOVER) program to “provide information for the spatial assessment and resolution of inter-annual variability in seagrass communities, and to establish a baseline to monitor responses of seagrass communities to water management alterations associated with CERP activities” (Hall et al., 2016; Hall and Durako, 2019). Monitoring for FHAP is conducted once a year in May–June (with the exception of 2015 when monitoring occurred after the die-off in November) at 30 sites within 17 basins across Florida Bay. At each site, eight 0.5 × 0.5 m quadrats are deployed and benthic macrophyte cover is quantified using the Braun-Blanquet (BB) method (Hall et al., 2016). The BB method is a rapid and highly repeatable visual assessment technique that has been employed in Florida Bay for over two decades (Fourqurean et al., 2001; Furman et al., 2018). The scoring system is as follows: 0 = no presence, 0.1 = 1 shoot, 0.5 = less than 5 shoots, 1 = many shoots but <5% cover, 2 = 5–25% cover, 3 = 25–50% cover, 4 = 50–75% cover, 5 = 75–100% cover. The BB score for total seagrass is then averaged for each site. Our study utilized 30 sites in Johnson Basin and 30 sites in Rankin Basin surveyed each year for a total of 720 seagrass measurements. To determine the relationship between seagrass cover and sediment plume extent, the total seagrass cover from the 30 sites within each basin was averaged to create one BB score per year for each basin.
Data Analyses
To determine the extent of the sediment plume and how it changed over time, the two classes were combined and the area of the plume was calculated for each time step (Figure 3C). A Generalized Additive Model (GAM) was used to model plume size across years using the R package “mgcv” (Wood, 2017). Two models were run in preliminary analyses: one with seasonality and one without seasonality to determine whether seasonality was a significant driver of plume size. A breakpoint analysis was run to determine years in which plume size significantly changed over the 12 years.
In order to relate plume extent to changes in seagrass cover, plume expansion and contraction within Johnson and Rankin Basins were investigated. Shapefiles of Rankin and Johnson were used to determine the proportion of each basin the plume covered within each image. A breakpoint analysis was also run on the Rankin and Johnson Basin time series individually to identify the years in which the plume coverage within each basin significantly changed. For all of the breakpoint analyses, the optimal number of breakpoints in the data was determined by the minimum Bayesian Information Criterion (BIC; Bai and Perron, 2003). Breakpoint analyses were done with the R package “strucchange” (Zeileis et al., 2002, 2003).
In order to examine the interaction of plume expansion and seagrass cover, an analysis of variance (ANOVA) was used to test for differences in the proportion of each basin covered by the sediment plume and seagrass cover before and after the breakpoints between each basin. A Tukey’s HSD was run to identify which time periods significantly differed. Pearson’s correlation tests were run to test the relationship between the proportion of each basin covered by the sediment plume and seagrass cover. Only spring images (n = 12, includes the November measurement after the seagrass die-off) were included in the seagrass ANOVA and correlation analyses since seagrass cover was only monitored once a year in May–June. ANOVA and correlation analyses were done in R v 4.0.3 (R Core Team, 2020).
Results
Accuracy of Sediment Plume Delineation
The areal extent of the sediment plume in western Florida Bay increased over the period of the study (2008–2020). At its largest, the sediment plume covered an area of 249.2 km2, increasing 108% from a minimum of 119.6 km2 during the period of observation (Supplementary Table 1). The overall accuracy of satellite imagery plume delineations tested with grab samples over 2017–2020 was 80.5% (Table 1). However, the majority (69.2%) of that error was due to lower turbidity measurements in the deeper, southern portion of our study area (around Rabbit Key Basin), where the bottom can be obscured by lighter sediment loads due to depth. The overall accuracy increased to 93.1% when the deeper, southern area was excluded from the accuracy assessment.
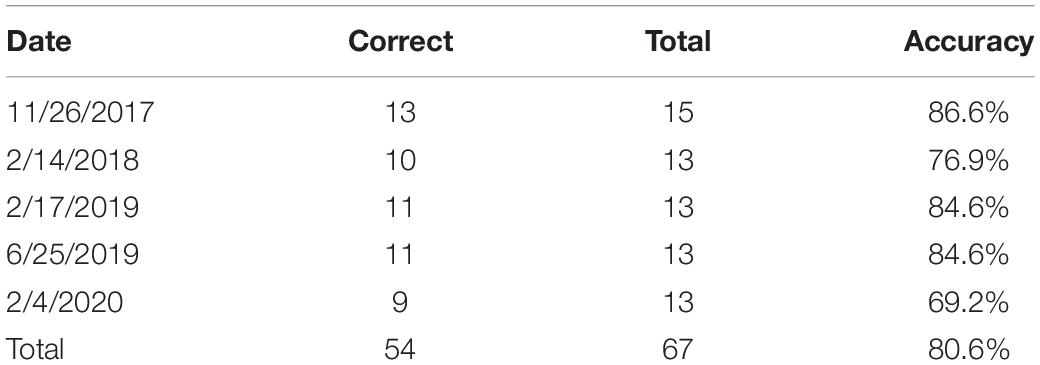
Table 1. Summary of accuracy assessment of images from 2017 until 2020 using grab sample data provided by ENP.
Sediment Plume Expansion Across the Study Area
When considering the full spatial extent of the study, we observed a significant, non-linear increase of the plume over the period examined. The GAM results found that yearly variation significantly affected the size of the plume, explaining 63% of the deviance, and found no evidence of variation as a function of season (e.g., fall vs. spring satellite images; Figure 4 and Table 2). We detected a breakpoint in the sediment plume coverage of the region in November 2016 (95% CI: September 2015–February 2018; Table 3). This date straddled the Fall 2015 seagrass die-off and the Fall 2017 hurricane Irma. The average size of the sediment plume before the breakpoint was 163.5 km2 (±26.8 km2), which increased to 223.5 km2 (±19.9 km2) post-breakpoint–a 37% increase in area.
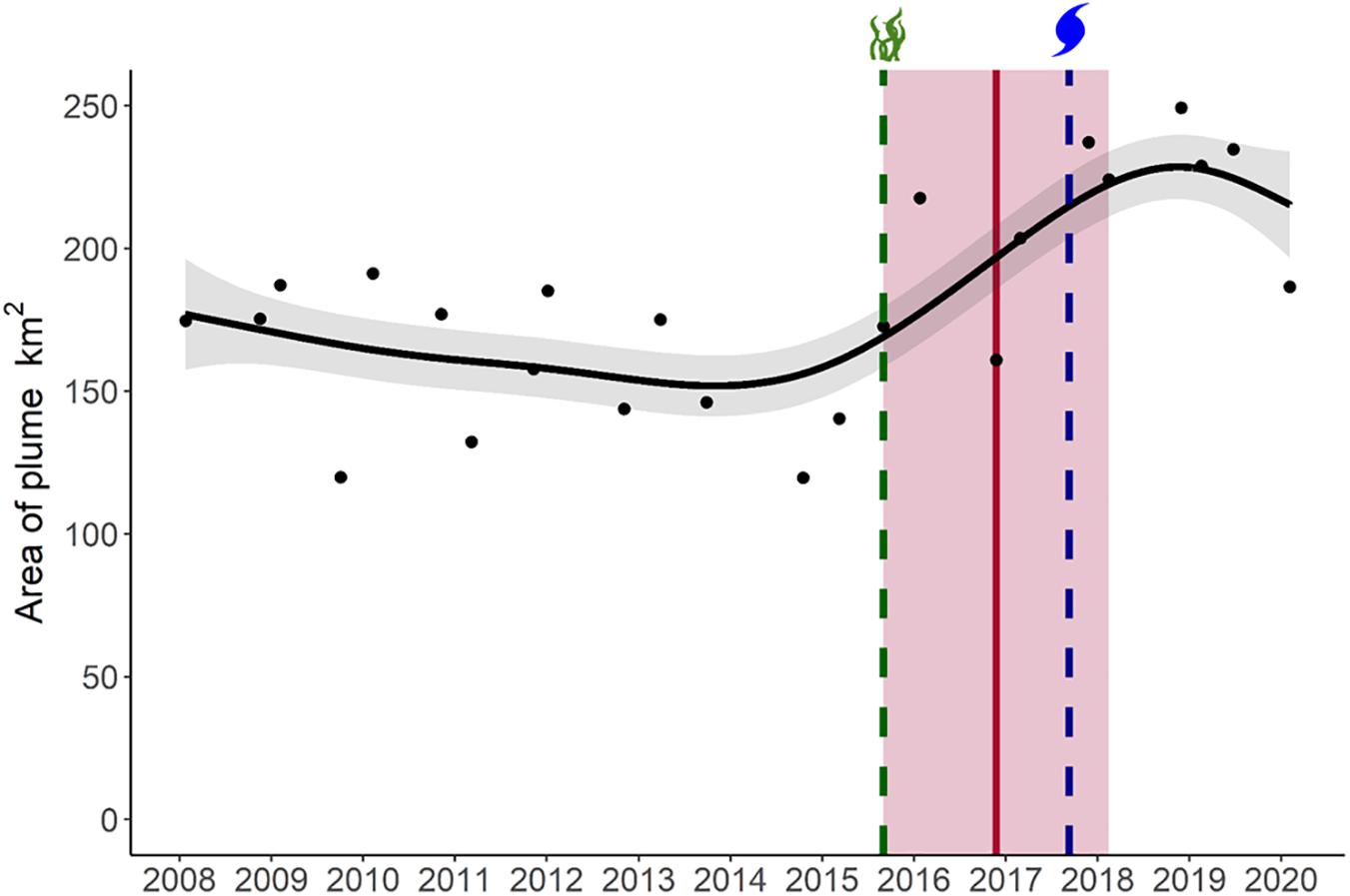
Figure 4. Temporal trend in the sediment plume size in Florida Bay between 2008 and 2020. The black line shows the fitted GAM model and 95% confidence interval (gray shaded area). The vertical red line shows the breakpoint in sediment plume size, with its 95% confidence interval (red shaded area). The vertical green line represents the 2015 seagrass die-off and the vertical blue line represents Hurricane Irma.
Basin Specific Responses to Disturbances and Sediment Plume Coverage
When considering our focal basins, Johnson and Rankin, we found differences in the magnitude, timing and duration of effects of the sediment plume. Both basins exhibited breakpoints, but the breakpoint was earlier, and resulted in more severe and longer lasting effects in the western basin, Johnson (Figure 5). An ANOVA conducted on the proportion of the basins covered by the plume, showed a significant basin and breakpoint interaction, with both Johnson and Rankin having a significantly higher proportion of basin covered by the plume after the change point compared to before (Tukey’s HSD < 0.05; Figure 6A and Table 4). In Johnson, there was a breakpoint in March 2015 (95% CI: October 2014–September 2015; Figure 5 and Table 3), and sustained high sediment coverage through the last data point in the time series. Before the breakpoint, an average of 11.6% (±10.8%) of Johnson was covered by the sediment plume while 78.6% (±13.4%) of Johnson was covered by the plume after the breakpoint (Figure 6A). In contrast, for Rankin, there were two breakpoints. The first breakpoint was February 2017 (95% CI September 2015–November 2017), and the second was November 2018 (95% CI February 2018–February 2020; Figure 5 and Table 4). Here, 0% of Rankin was covered by the plume before the first breakpoint, while 22% (±21%) was covered by the plume after the first breakpoint (Figure 6A). The second breakpoint in Rankin represents a sediment contraction, indicating the short term effects in this basin, and thus, was not considered in further analyses.
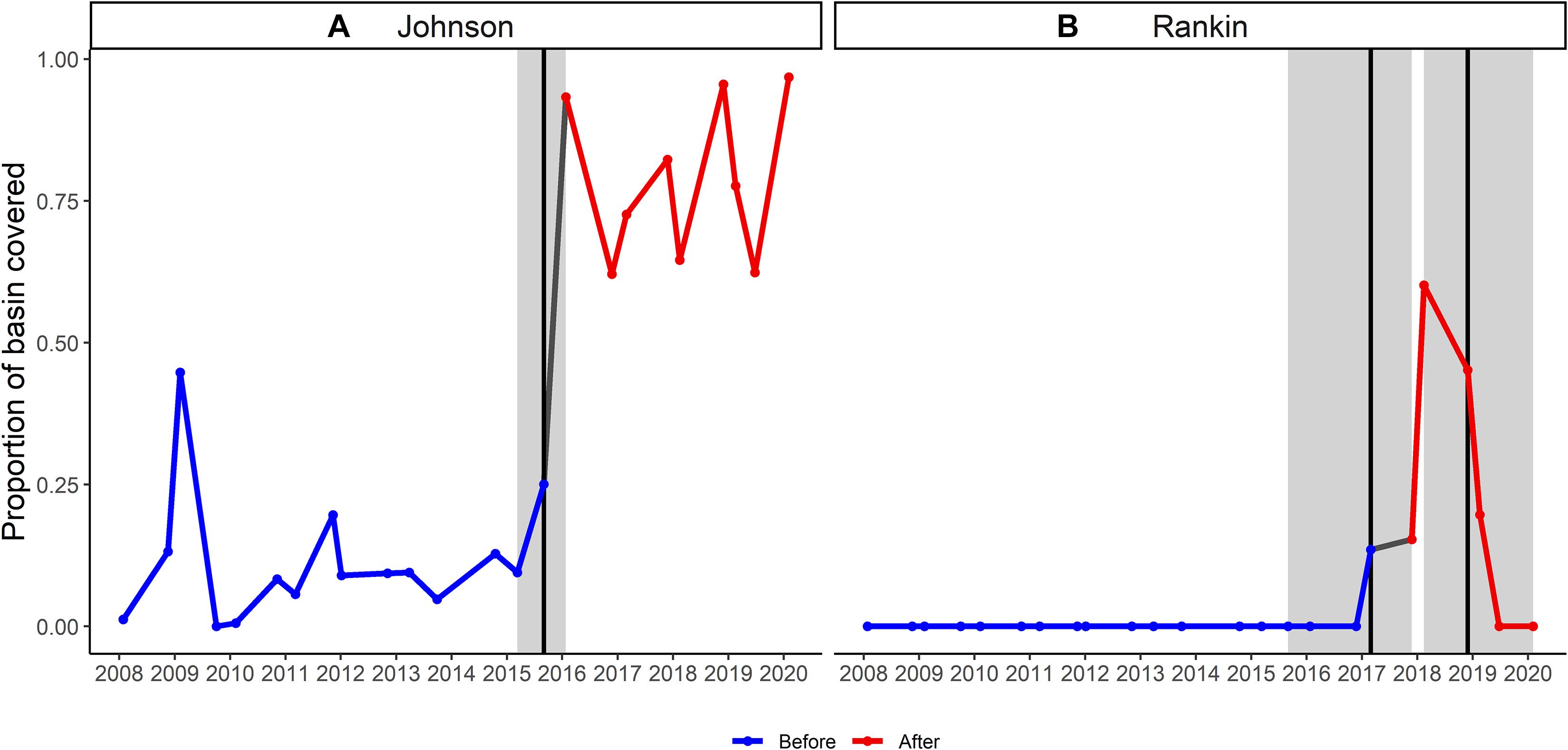
Figure 5. Temporal trend in the proportion of (A) Johnson, and (B) Rankin basins covered by the sediment plume. The blue lines represent the trend before the breakpoint while the red lines represent the trend after the first breakpoint. Breakpoints are shown by vertical black lines with 95% confidence interval in gray shading.
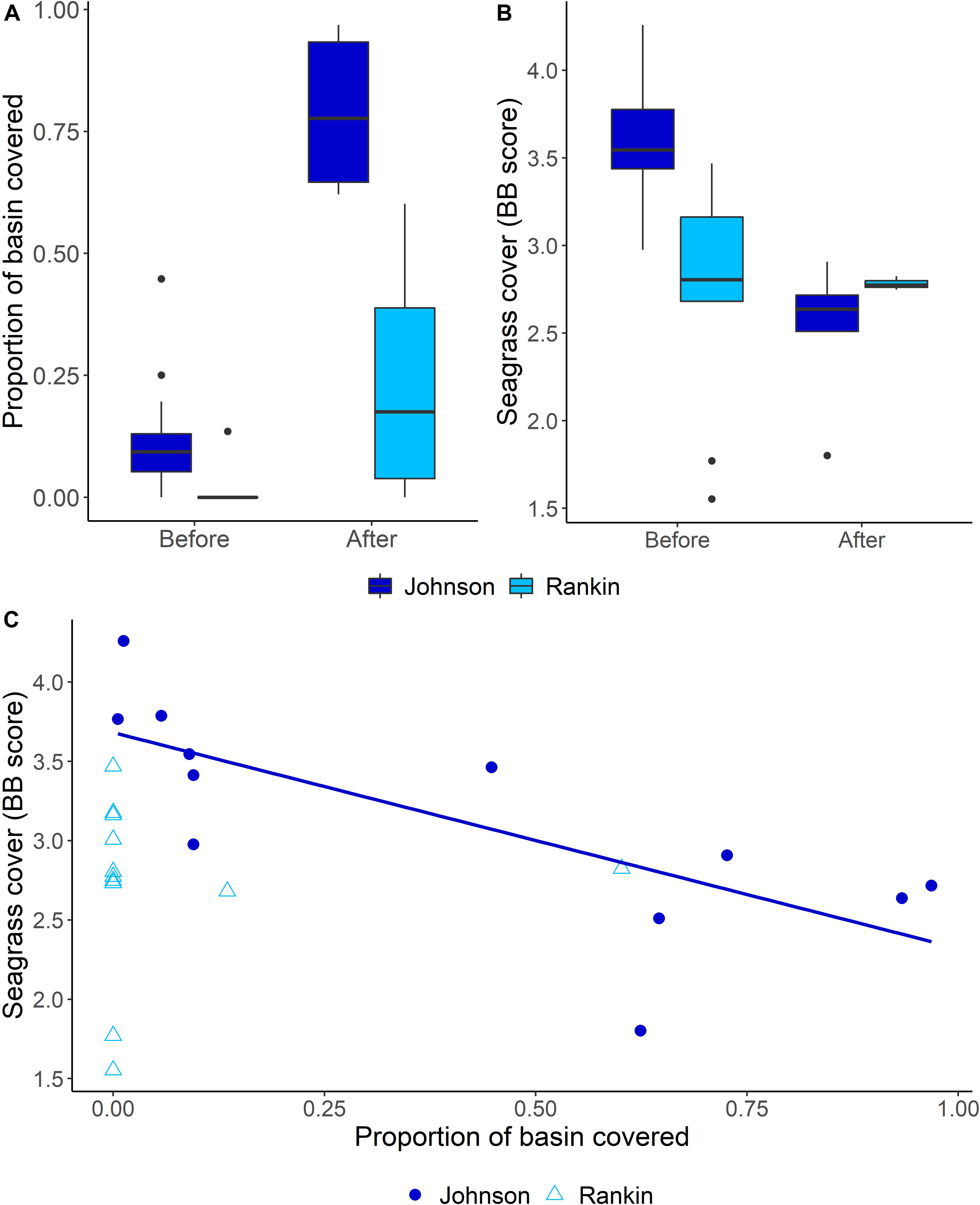
Figure 6. Summary of ANOVA and correlation analyses. (A) Mean proportions (and 95% confidence intervals) of Johnson and Rankin Basins covered by the sediment plume before and after the breakpoints. (B) Mean (and 95% confidence interval) Braun-Blanquet seagrass cover in Johnson and Rankin before and after the breakpoints. (C) Relationship between the proportion of each basin covered by the sediment plume and the mean seagrass cover of each basin shown separately by basin, showing the significant regression line for Johnson Basin. Linear regression model shown for Johnson Basin has an R2 value of 0.55 following the following equation: y = –1.4x + 3.7.
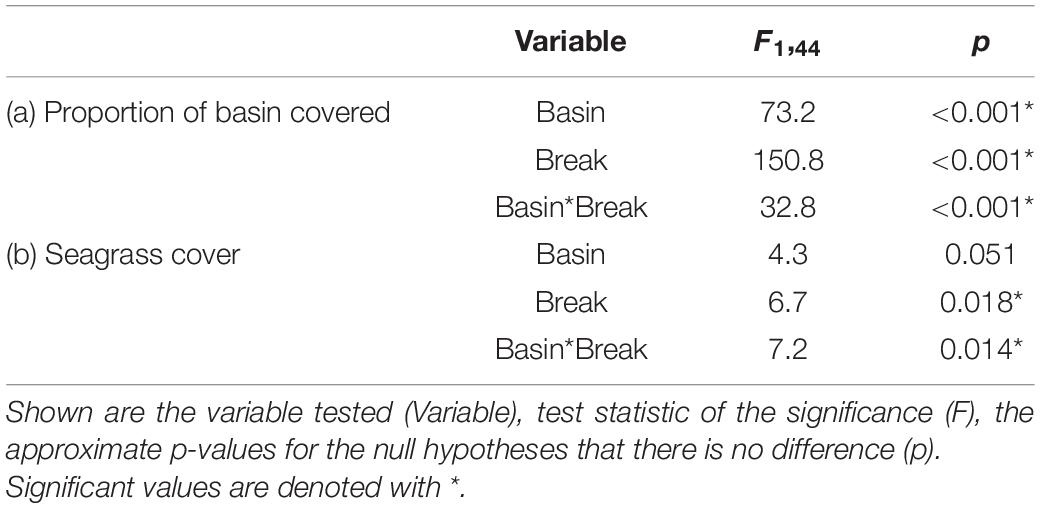
Table 4. Analysis of variance (ANOVA) used to determine significant differences in (a) proportion of basin covered and (b) seagrass cover between basin and before and after the breakpoint.
Interaction Between Sediment Plume Coverage and Changes in Seagrass Cover
Along with differences in the extent of the sediment plume, we also found differences in seagrass cover between basins, and a basin-specific interaction between plume extent and seagrass cover. For seagrass cover, the interaction between basin and before and after the change point was significant (Table 4). Seagrass cover in Johnson basin decreased significantly after its March 2015 breakpoint (Tukey’s HSD < 0.05; Figure 6B and Table 4). The average BB score in Johnson dropped from 3.6 (±0.34; approximately 60% cover) to 2.5 (±0.38; approximately 35% cover) after the breakpoint. In contrast, we found no change in seagrass cover as a function of its February 2017 breakpoint in Rankin. The average BB score of Rankin before the breakpoint was 2.7 (±0.61; approximately 40% cover) and was 2.8 (±0.05; approximately 45% cover) after the breakpoint. Further, in Johnson, there was a negative correlation between the proportion of basin covered by the sediment plume and seagrass cover (r = −0.75, p = 0.005; Figure 6C). There was no correlation between the proportion of basin covered by the sediment plume and seagrass cover in Rankin (r = 0.05, p = 0.88; Figure 6C).
Discussion
Anthropogenic and natural disturbances have jointly contributed to the degradation of seagrass habitats worldwide, including in Florida Bay, which have resulted in two drought-induced seagrass die-offs (Fourqurean and Robblee, 1999; Hall et al., 2016). The most recent seagrass die-off occurred in 2015, causing a potential localized regime shift from a densely vegetated state to a turbid, non-vegetated state (Hall et al., 2016; Hall and Durako, 2019). The system was further perturbed when Hurricane Irma passed over Florida Bay in 2017. In this paper, we describe evidence based on remote sensing and field data of how an expansion of a sediment plume in the western region of Florida Bay resulted from these two disturbances. Overall, we saw a 37% average increase in sediment plume area as the plume expanded eastward post the 2015 die-off (time series breakpoint is November 2016), with peak sediment values reached post Hurricane Irma. The expansion of the plume into individual basins was dependent on the scale and location of the disturbance, expanding in Johnson soon after the die-off but only expanding into Rankin after Hurricane Irma. Further, the effect of the plume in Rankin was smaller and shorter lasting than the more expansive and persistent effects in Johnson. We also investigate the potential interaction of this sediment plume expansion with seagrass, which we found to be basin specific. The sediment plume was negatively related to seagrass cover in the more and earlier impacted Johnson basin while had no relationship in Rankin due to the recovery of seagrass before the sediment plume reached Rankin.
How a system responds to a disturbance is spatially explicit, dependent on the spatial scale (Norkko et al., 2006; Dumbrell et al., 2008) and intensity of a disturbance event (Platt and Connell, 2003; Miller et al., 2011). This is especially true in coastal ecosystems, where different levels of exposure to a disturbance result in a spatial gradient of effects on community composition and function (Fonseca and Bell, 1998; Fonseca et al., 2008; Santos and Lirman, 2012). For example, Stipek et al. (2020) found that seagrass beds closer to pulses of freshwater experienced higher fragmentation rates and higher mortality of small seagrass patches. In our study, the expansion of the sediment plume in response to the seagrass die-off and Hurricane Irma was also spatially explicit due to the unique hydrological dynamics of Florida Bay. Both disturbances contributed to the expansion of the sediment plume, but at different spatial scales. We expected high sediment mobilization after the seagrass die-off due to the loss of the mechanisms that promote sediment stabilization in densely covered SAV habitats (Fonseca and Fisher, 1986; Nyström et al., 2012). Even though the sediment was more easily mobilized after the seagrass die-off, the low water movement within the system, due to restricted water exchange and high residence time (Nuttle et al., 2000; Rudnick et al., 2005), may explain why the plume did not expand immediately after the die-off into certain areas of the Bay, such as in Rankin.
Hurricane Irma was a bay-scale disturbance that drastically moved water throughout the estuary, and the combination of the water movement and increased sediment mobility likely led to the expansion of the sediment plume to its largest extent (Liu et al., 2020). The different spatial extent and location at which the two disturbances acted created a spatially explicit gradient in their effects, as seen by the differences in the timing of breakpoints between basins. Early during the study period, Johnson was on the eastern border of the sediment plume (Figure 2). There was a breakpoint in the proportion of Johnson covered with the sediment plume that corresponded with the seagrass die-off. This was not the case for Rankin, which is located east of Johnson, where the sediment concentration before the disturbances was zero. The sediment plume in Rankin was ephemeral, increasing in size after Hurricane Irma in 2017 but exiting Rankin in 2019.
The timing of disturbances also plays a role in determining effects on an ecosystem (Miller et al., 2011; Santillan et al., 2019). In Florida Bay, Johnson Basin became turbid due to the sediment plume right after the 2015 seagrass die-off while the sediment plume did not migrate to Rankin Basin until 2017. The difference in timing between when each basin experienced the sediment plume may have affected seagrass recovery. Both Johnson and Rankin lost most of their T. testudinum cover in the drought-induced seagrass die-off in 2015 (Hall et al., 2016; Hall and Durako, 2019). Since both systems were T. testudinum-dominated before the die-off, the loss of this species resulted in a severe reduction to the total seagrass cover as well. However, recovery trajectories differ between the two basins (Hall and Durako, 2019; McDonald et al., 2020). Total seagrass cover, dominated by Halodule wrightii (a pioneer species), recovered to near pre-die-off levels in Rankin within 2 years, whereas Johnson continues to lose T. testudinum and was not being recolonized by H. wrightii until 2020 (McDonald et al., 2020). This difference in seagrass recovery may be due to the high proportion of the Johnson covered by the sediment plume that occurred immediately after the die-off.
Several factors acting at various spatial and temporal scales influence seagrass recovery after a disturbance. In Tampa Bay, water clarity was the main driver preventing seagrass recovery (Greening et al., 2011), while genetic material was the limiting factor in the recovery of seagrass in Shark Bay, Australia (Nowicki et al., 2017). The sequence of events (die-off > plume extent > lack of SAV recovery) in Johnson suggests that the seagrass seascape in Johnson entered into a feedback cycle of degradation between seagrass cover and turbidity, preventing recovery (Figure 1). This cycle is common within degraded seagrass habitats and does not allow for seagrass to recover, which is evident in Johnson. A similar pattern occurred in seagrass beds near the Great Barrier Reef, where sediment plumes from dredging prevented the establishment of seagrass (York et al., 2015).
Persistent algal blooms and proximity to freshwater inflows may also play a factor in reducing light penetration and preventing seagrass recovery in Florida Bay. Central Florida Bay experiences seasonal algal blooms originating from anthropogenically altered freshwater deliveries (Boyer et al., 2009; Briceño and Boyer, 2010) and large-scale algal blooms brought on by tropical storms and hurricanes (Glibert et al., 2009; Wahl, 2019). The seagrass die-off in the late 1980’s exacerbated algal blooms in this region, leading to negative effects on seagrass recovery (Robblee et al., 1991; Fourqurean and Robblee, 1999). However, Hall et al. (2021) found no evidence of algal blooms depleting sources of seagrass recruits after the 1980’s die-off. Furthermore, freshwater inflows may be affecting the recovery of seagrass within Florida Bay. H. wrightii, the pioneer seagrass species, is favored over T. testudinum in lower salinity conditions (Herbert et al., 2011). Rankin Basin is located closer to freshwater inflows than Johnson Basin. Therefore, higher freshwater inflows and lower salinities in Rankin Basin may have contributed to a more rapid recovery. Further research is needed to determine the variables affecting seagrass recovery within Florida Bay, especially considering the hydrodynamics drivers that interactively influenced seagrass physiology, productivity and patch formation.
While Johnson Basin experienced high turbidity from the sediment plume right after the die-off, suspended sediment associated with the sediment plume did not reach Rankin until 2 years later. This delay in disturbance may have allowed Rankin to begin recolonizing the benthos as illustrated by the recovery of seagrass cover (by mostly H. wrightii) to a BB score of 2.5 before the sediment plume entered Rankin. Recolonization followed traditional models of SAV succession, wherein macroalgae colonize the bare sediment and then H. wrightii replaces the macroalgae (Den Hartog, 1979; Zieman, 1982). Due to the known function of seagrass habitats for sediment stabilization and deposition in coastal environments (Bos et al., 2007), we hypothesize that the establishment of H. wrightii within Rankin helped stabilize the sediment. Therefore, when Hurricane Irma expanded the plume into Rankin, the system exhibited resistance to the increase in turbidity and prevented the shift into the degrading feedback loop shown in Figure 1. Furthermore, the wind may have played a role in reducing the impact of the sediment plume in Rankin. Southern Florida experiences strong easterly winds in fall and winter (Klink, 1999), which may have aided in removing the plume from Rankin after a couple of years.
Western Florida Bay, including Johnson, faces a more difficult road to recovery. The sediment plume expanded into this region right after the 2015 die-off, preventing any SAV recovery and driving the system into a turbid alternative state that persisted through 2020 in our study. Recovery of seagrass after the die-off in the late 1980’s might present a solution that includes light availability and time. Stumpf et al. (1999) mapped albedo throughout Florida Bay before and after the first seagrass die-off. They found that sediment expanded into the western region starting in 1988 and persisted through 1997 (the duration of their time series), but lost intensity beginning in 1996. Once this loss in intensity (reduction in turbidity) reaches a certain threshold, benthic macroalgae can start recolonizing an area. Benthic macroalgae only need approximately 8–10% of surface irradiance to grow (Choice et al., 2014). Therefore, time may be the only factor required for sediment settlement to occur, reducing turbidity and increasing benthic light intensity to the 8–10% threshold needed for macroalgae recolonization. Macroalgae recolonization then has the potential to create a beneficial feedback loop, leading to increased sediment stabilization. If there is a source of seagrass genetic material available (seed reserve or connectivity to clonal sources), the sediment stabilization may result in seagrass recovery in Johnson and the rest of western Florida Bay (Austin et al., 2017). Florida Bay returned to pre-die-off conditions by 2012 (Hall et al., 2016), illustrating that the system can undergo the lengthy (15 years) recovery process. But it is unknown whether there are enough sources of seagrass, and instead active restoration techniques may be required.
Climate change presents seagrass meadows with an additional set of disturbances, which have various impacts on seagrass distribution and productivity (Short and Neckles, 1999; Duarte et al., 2018). Increased temperature will lead to species distribution changes (Carlson et al., 2018; Duarte et al., 2018), ocean acidification will impact seagrass metabolism (Apostolaki et al., 2014; Zimmerman, 2020), and sea level rise will cause dynamic changes in seagrass areal extent (Albert et al., 2017). How these factors will interact with the current conditions within Florida Bay and the impact climate change will have on seagrass ecosystems is unknown (but see Browder et al., 2002; Carlson et al., 2018; Peñalver et al., 2020). Therefore, it is vital to focus on the resilience (the ability to persist after external disturbances; Holling, 1973; Côté and Darling, 2010) of seagrass within Florida Bay. A healthy ecosystem requires conditions that build resilience in order to withstand multiple disturbances at different spatial scales (Costanza and Mageau, 1999; Standish et al., 2014; Gladstone-Gallagher et al., 2019). Considering recent events, increased resilience of Florida Bay seagrasses is vital to maintain the various ecosystems services that they provide. However, increasing resilience within seagrass systems requires knowledge of parameters, such as genetic and species diversity, trophic interactions, water quality, and connectivity with other coastal systems that drive habitat stability and the ability to recover from compounded disturbance effects (Unsworth et al., 2015). Therefore, further monitoring of how extreme disturbances impact the structure and function of seagrass ecosystems is needed.
Seagrasses around the world are declining due to anthropogenic and natural disturbances disrupting natural feedbacks that promote seagrass growth and sustenance. Our study demonstrates the usage of long-term seagrass monitoring and remote sensing to investigate how two disturbances may interact to impact seagrass ecosystems at multiple scales. We found that a sediment plume may be a contributing factor in preventing seagrass recovery in Florida Bay after a large-scale seagrass die-off and a hurricane. Given that seagrass beds provide many ecosystem services such as carbon sequestration, habitat for fish and other fauna, and sediment stabilization (Bos et al., 2007; Fourqurean et al., 2012; Unsworth et al., 2019), more information is needed to increase seagrass resilience against impacts of future extreme events such as hurricanes and droughts.
Data Availability Statement
The raw data supporting the conclusions of this article will be made available by the authors, without undue reservation.
Author Contributions
JRR, WJ, RS, JSR, BF, CRK, ZF, and CK conceptualized the project. JRR, VB, and JL delineated sediment plumes. BF and MH provided long-term seagrass data. ZF and CK provided turbidity grab sample data. JRR, WJ, and RS ran statistical analyses. JRR, WJ, NV, JOL, and LL prepared first draft. All authors contributed to the manuscript preparation and revision, and read and approved the submitted version.
Funding
Project funded by the Critical Ecosystems Study Initiative (CESI) of the National Park Service, the Munson Foundation through SECOORA, the Everglades Foundation ForEverglades Scholarship and the Institute of Environment Christina Menendez Fellowship, and in collaboration with the Florida Coastal Everglades Long-Term Ecological Research program under National Science Foundation Grant # DEB-1832229. JRR was funded by the Presidential Fellowship from the University Graduate School at Florida International University.
Conflict of Interest
The authors declare that the research was conducted in the absence of any commercial or financial relationships that could be construed as a potential conflict of interest.
Publisher’s Note
All claims expressed in this article are solely those of the authors and do not necessarily represent those of their affiliated organizations, or those of the publisher, the editors and the reviewers. Any product that may be evaluated in this article, or claim that may be made by its manufacturer, is not guaranteed or endorsed by the publisher.
Acknowledgments
We would like to thank the editors and the reviewers for their time and effort. This research was conducted under NPS Permit EVR-2020-SCI-0039. This is contribution #288 from the Coastlines and Oceans Division in the Institute of Environment at Florida International University.
Supplementary Material
The Supplementary Material for this article can be found online at: https://www.frontiersin.org/articles/10.3389/fmars.2021.633240/full#supplementary-material
Footnotes
References
Albert, S., Saunders, M. I., Roelfsema, C. M., Leon, J. X., Johnstone, E., Mackenzie, J. R., et al. (2017). Winners and losers as mangrove, coral and seagrass ecosystems respond to sea-level rise in Solomon Islands. Environ. Res. Lett. 12:094009. doi: 10.1088/1748-9326/aa7e68
Apostolaki, E. T., Vizzini, S., Hendriks, I. E., and Olsen, Y. S. (2014). Seagrass ecosystem response to long-term high CO2 in a Mediterranean volcanic vent. Mar. Environ. Res. 99, 9–15. doi: 10.1016/j.marenvres.2014.05.008
Austin, ÅN., Hansen, J. P., Donadi, S., and Eklöf, J. S. (2017). Relationships between aquatic vegetation and water turbidity: a field survey across seasons and spatial scales. PLoS One 12:e0181419. doi: 10.1371/journal.pone.0181419
Bai, J., and Perron, P. (2003). Computation and analysis of multiple structural change models. J. Appl. Econ. 18, 1–22. doi: 10.1002/jae.659
Beisner, B. E., Haydon, D. T., and Cuddington, K. (2003). Alternative stable states in ecology. Front. Ecol. Environ. 1:376–382. doi: 10.2307/3868190
Bos, A. R., Bouma, T. J., de Kort, G. L. J., and van Katwijk, M. M. (2007). Ecosystem engineering by annual intertidal seagrass beds: sediment accretion and modification. Estuar. Coast. Shelf Sci. 74, 344–348. doi: 10.1016/j.ecss.2007.04.006
Boyer, J. N., Kelble, C. R., Ortner, P. B., and Rudnick, D. T. (2009). Phytoplankton bloom status: chlorophyll a biomass as an indicator of water quality condition in the southern estuaries of Florida, USA. Ecol. Indic. 9, S56–S67.
Briceño, H. O., and Boyer, J. N. (2010). Climatic controls on phytoplankton biomass in a sub-tropical estuary, Florida Bay, USA. Estuar. Coasts 33, 541–553.
Browder, J. A., Zein-Eldin, Z., Criales, M. M., Robblee, M. B., Wong, S., Jackson, T. L., et al. (2002). Dynamics of pink shrimp (Farfantepenaeus duorarum) recruitment potential in relation to salinity and temperature in Florida Bay. Estuaries 25, 1355–1371. doi: 10.1007/BF02692230
Carlson, D. F., Yarbro, L. A., Scolaro, S., Poniatowski, M., McGee-Absten, V., and Carlson, P. R. (2018). Sea surface temperatures and seagrass mortality in Florida Bay: spatial and temporal patterns discerned from MODIS and AVHRR data. Remote Sens. Environ. 208, 171–188. doi: 10.1016/j.rse.2018.02.014
Carstensen, J., Sánchez-Camacho, M., Duarte, C. M., Krause-Jensen, D., and Marbà, N. (2011). Connecting the dots: responses of coastal ecosystems to changing nutrient concentrations. Environ. Sci. Technol. 45, 9122–9132. doi: 10.1021/es202351y
Choice, Z. D., Frazer, T. K., and Jacoby, C. A. (2014). Light requirements of seagrasses determined from historical records of light attenuation along the Gulf coast of peninsular Florida. Mar. Pollut. Bull. 81, 94–102. doi: 10.1016/j.marpolbul.2014.02.015
Costanza, R., and Mageau, M. (1999). What is a healthy ecosystem? Aquat. Ecol. 33, 105–115. doi: 10.1023/A:1009930313242
Côté, I. M., and Darling, E. S. (2010). Rethinking ecosystem resilience in the face of climate change. PLoS Biol. 8:e1000438. doi: 10.1371/journal.pbio.1000438
Dawes, C. J., Andorfer, J., Rose, C., Uranowski, C., and Ehringer, N. (1997). Regrowth of the seagrass Thalassia testudinum into propeller scars. Aquat. Bot. 59, 139–155. doi: 10.1016/S0304-3770(97)00021-1
Den Hartog, C. (1979). Seagrasses and seagrass ecosystems, an appraisal of the research approach. Aquat. Bot. 7, 105–117. doi: 10.1016/0304-3770(79)90015-9
Dumbrell, A. J., Clark, E. J., Frost, G. A., Randell, T. E., Pitchford, J. W., and Hill, J. K. (2008). Changes in species diversity following habitat disturbance are dependent on spatial scale: theoretical and empirical evidence. J. Appl. Ecol. 45, 1531–1539. doi: 10.1111/j.1365-2664.2008.01533.x
Duarte, B., Martins, I., Rosa, R., Matos, A. R., Roleda, M. Y., Reusch, T. B. H., et al. (2018). Climate change impacts on seagrass meadows and macroalgal forests: an integrative perspective on acclimation and adaptation potential. Front. Mar. Sci. 5:190. doi: 10.3389/fmars.2018.00190
Duarte, C. M., and Chiscano, C. L. (1999). Seagrass biomass and production: a reassessment. Aquat. Bot. 65, 159–174. doi: 10.1016/S0304-3770(99)00038-8
Duarte, C. M., Conley, D. J., Carstensen, J., and Sánchez-Camacho, M. (2009). Return to neverland: shifting baselines affect eutrophication restoration targets. Estuar. Coasts 32, 29–36. doi: 10.1007/s12237-008-9111-2
Duarte, C. M., Kennedy, H., Marbà, N., and Hendriks, I. (2013). Assessing the capacity of seagrass meadows for carbon burial: current limitations and future strategies. Ocean Coast. Manag. 83, 32–38. doi: 10.1016/j.ocecoaman.2011.09.001
Duffy, J. E., Reynolds, P. L., Boström, C., Coyer, J. A., Cusson, M., Donadi, S., et al. (2015). Biodiversity mediates top–down control in eelgrass ecosystems: a global comparative-experimental approach. Ecol. Lett. 18, 696–705. doi: 10.1111/ele.12448
Effler, S. W. (1988). Secchi disc transparency and turbidity. J. Environ. Eng. 114, 1436–1447. doi: 10.1061/(ASCE)0733-93721988114:61436
Foga, S., Scaramuzza, P. L., Guo, S., Zhu, Z., Dilley, R. D., Beckmann, T., et al. (2017). Cloud detection algorithm comparison and validation for operational landsat data products. Remote Sens. Environ. 194, 379–390. doi: 10.1016/j.rse.2017.03.026
Fonseca, M. S., and Bell, S. S. (1998). Influence of physical setting on seagrass landscapes near Beaufort, North Carolina, USA. Mar. Ecol. Prog. Ser. 171, 109–121. doi: 10.3354/meps171109
Fonseca, M. S., and Fisher, J. S. (1986). A comparison of canopy friction and sediment movement between four species of seagrass with reference to their ecology and restoration. Mar. Ecol. Prog. Ser. 29, 15–22.
Fonseca, M. S., Kenworthy, W. J., Griffith, E., Hall, M. O., Finkbeiner, M., and Bell, S. S. (2008). Factors influencing landscape pattern of the seagrass Halophila decipiens in an oceanic setting. Estuar. Coast. Shelf Sci. 76, 163–174. doi: 10.1016/j.ecss.2007.06.014
Fourqurean, J. W., Duarte, C. M., Kennedy, H., Marbà, N., Holmer, M., Mateo, M. A., et al. (2012). Seagrass ecosystems as a globally significant carbon stock. Nat. Geosci. 5, 505–509. doi: 10.1038/ngeo1477
Fourqurean, J. W., and Robblee, M. B. (1999). Florida bay: a history of recent ecological changes. Estuaries 22, 345–357. doi: 10.2307/1353203
Fourqurean, J. W., Willsie, A., Rose, C. D., and Rutten, L. M. (2001). Spatial and temporal pattern in seagrass community composition and productivity in south Florida. Mar. Biol. 138, 341–354. doi: 10.1007/s002270000448
Furman, B. T., Leone, E. H., Bell, S. S., Durako, M. J., and Hall, M. O. (2018). Braun-Blanquet data in ANOVA designs: comparisons with percent cover and transformations using simulated data. Mar. Ecol. Prog. Ser. 597, 13–22. doi: 10.3354/meps12604
Glibert, P. M., Heil, C. A., Rudnick, D. T., Madden, C. J., Boyer, J. N., and Kelly, S. P. (2009). Florida bay: water quality status and trends, historic and emerging algal bloom problems. Contrib. Mar. Sci. 38, 5–17.
Gillanders, B. M. (2006). “Seagrasses, fish, and fisheries,” in Seagrasses: Biology, Ecology and Conservation, eds A. W. D. Larkum, R. J. Orth, and C. M. Duarte (Dordrecht: Springer Netherlands), 503–505.
Gladstone-Gallagher, R. V., Pilditch, C. A., Stephenson, F., and Thrush, S. F. (2019). Linking traits across ecological scales determines functional resilience. Trends Ecol. Evol. 34, 1080–1091. doi: 10.1016/j.tree.2019.07.010
Gorelick, N., Hancher, M., Dixon, M., Ilyushchenko, S., Thau, D., and Moore, R. (2017). Google earth engine: planetary-scale geospatial analysis for everyone. Remote Sens. Environ. 202, 18–27. doi: 10.1016/j.rse.2017.06.031
Greening, H. S., Cross, L. M., and Sherwood, E. T. (2011). A multiscale approach to seagrass recovery in Tampa Bay, Florida. Ecol. Rest. 29, 82–93. doi: 10.3368/er.29.1-2.82
Hajigholizadeh, M., and Melesse, A. (2017). Study on spatiotemporal variability of water quality parameters in Florida Bay using remote sensing. J. Remote Sens. GIS 6:207. doi: 10.4172/2469-4134.1000207
Hall, M. O., Bell, S. S., Furman, B. T., and Durako, M. J. (2021). Natural recovery of a marine foundation species emerges decades after landscape-scale mortality. Sci. Rep. 11:6973.
Hall, M., and Durako, M. J. (2019). South Florida Fisheries Habitat Assessment Program Annual Report to South Florida Water Management District. West Palm Beach, FL: South Florida Water Management District.
Hall, M., Furman, B., Merello, M., and Durako, M. (2016). Recurrence of Thalassia testudinum seagrass die-off in Florida Bay, USA: initial observations. Mar. Ecol. Prog. Ser. 560, 243–249. doi: 10.3354/meps11923
Heck, K. L., and Valentine, J. F. (1995). Sea urchin herbivory: evidence for long-lasting effects in subtropical seagrass meadows. J. Exp. Mar. Biol. Ecol. 189, 205–217. doi: 10.1016/0022-0981(95)00012-G
Herbert, D. A., Perry, W. B., Cosby, B. J., and Fourqurean, J. W. (2011). Projected reorganization of Florida Bay seagrass communities in response to the increased freshwater inflow of Everglades restoration. Estuar. Coasts 34, 973–992.
Holling, C. S. (1973). Resilience and Stability of Ecological Systems. Annu. Rev. Ecol. Syst. 4, 1–23. doi: 10.1146/annurev.es.04.110173.000245
Islam, M. R., Yamaguchi, Y., and Ogawa, K. (2001). Suspended sediment in the Ganges and Brahmaputra Rivers in Bangladesh: observation from TM and AVHRR data. Hydrol. Process. 15, 493–509. doi: 10.1002/hyp.165
Kelble, C. R., Johns, E. M., Nuttle, W. K., Lee, T. N., Smith, R. H., and Ortner, P. B. (2007). Salinity patterns of Florida Bay. Estuar. Coast. Shelf Sci. 71, 318–334. doi: 10.1016/j.ecss.2006.08.006
Kenworthy, W. J., Fonseca, M. S., Whitfield, P. E., and Hammerstrom, K. K. (2002). Analysis of seagrass recovery in experimental excavations and propeller-scar disturbances in the Florida keys national marine sanctuary. J. Coast. Res. 37, 75–85.
Klink, K. (1999). Climatological mean and interannual variance of United States surface wind speed, direction and velocity1. Int. J. Climatol. 19, 471–488. doi: 10.1002/(SICI)1097-0088(199904)19:5<471::AID-JOC367<3.0.CO;2-X
Liu, Y., Weisberg, R. H., and Zheng, L. (2020). Impacts of hurricane irma on the circulation and transport in Florida Bay and the charlotte harbor estuary. Estuar. Coasts 43, 1194–1216. doi: 10.1007/s12237-019-00647-6
Lyons, M. B., Phinn, S. R., and Roelfsema, C. M. (2012). Long term land cover and seagrass mapping using Landsat and object-based image analysis from 1972 to 2010 in the coastal environment of South East Queensland, Australia. ISPRS J. Photogramm. Remote Sens. 71, 34–46. doi: 10.1016/j.isprsjprs.2012.05.002
Madden, C. J., Rudnick, D. T., McDonald, A. A., Cunniff, K. M., and Fourqurean, J. W. (2009). Ecological indicators for assessing and communicating seagrass status and trends in Florida Bay. Ecol. Indic. 9, S68–S82. doi: 10.1016/j.ecolind.2009.02.004
Marshall, F. E., Wingard, G. L., and Pitts, P. (2009). A simulation of historic hydrology and salinity in everglades national park: coupling paleoecologic assemblage data with regression models. Estuar. Coasts 32, 37–53. doi: 10.1007/s12237-008-9120-1
Maxwell, P. S., Eklöf, J. S., van Katwijk, M. M., O’Brien, K. R., de la Torre-Castro, M., Boström, C., et al. (2017). The fundamental role of ecological feedback mechanisms for the adaptive management of seagrass ecosystems – a review. Biol. Rev. 92, 1521–1538. doi: 10.1111/brv.12294
McDonald, A., Hall, M., and Furman, B. (2020). Florida Bay Benthic Vegetation. Chapter 6: Everglades Research and Evaluation. South Florida Environmental Report. West Palm Beach, FL: South Florida Water Management District.
Mcleod, E., Chmura, G. L., Bouillon, S., Salm, R., Björk, M., Duarte, C. M., et al. (2011). A blueprint for blue carbon: toward an improved understanding of the role of vegetated coastal habitats in sequestering CO2. Front. Ecol. Environ. 9:552–560. doi: 10.1890/110004
Miller, A. D., Roxburgh, S. H., and Shea, K. (2011). How frequency and intensity shape diversity–disturbance relationships. Proc. Natl. Acad. Sci. U.S.A. 108, 5643–5648. doi: 10.1073/pnas.1018594108
Norkko, A., Rosenberg, R., Thrush, S. F., and Whitlatch, R. B. (2006). Scale- and intensity-dependent disturbance determines the magnitude of opportunistic response. J. Exp. Mar. Biol. Ecol. 330, 195–207. doi: 10.1016/j.jembe.2005.12.027
Nowicki, R., Thomson, J., Burkholder, D., Fourqurean, J., and Heithaus, M. (2017). Predicting seagrass recovery times and their implications following an extreme climate event. Mar. Ecol. Prog. Ser. 567, 79–93. doi: 10.3354/meps12029
Nuttle, W. K., Fourqurean, J. W., Cosby, B. J., Zieman, J. C., and Robblee, M. B. (2000). Influence of net freshwater supply on salinity in Florida Bay. Water Resour. Res. 36, 1805–1822. doi: 10.1029/1999WR900352
Nyström, M., Norström, A. V., Blenckner, T., de la Torre-Castro, M., Eklöf, J. S., Folke, C., et al. (2012). Confronting feedbacks of degraded marine ecosystems. Ecosystems 15, 695–710. doi: 10.1007/s10021-012-9530-6
O’Brien, K. R., Waycott, M., Maxwell, P., Kendrick, G. A., Udy, J. W., Ferguson, A. J. P., et al. (2017). Seagrass ecosystem trajectory depends on the relative timescales of resistance, recovery and disturbance. Mar. Pollut. Bull. 134, 166–176. doi: 10.1016/j.marpolbul.2017.09.006
Orth, R. J., Carruthers, T. J. B., Dennison, W. C., Duarte, C. M., Fourqurean, J. W., Heck, K. L., et al. (2006). A global crisis for seagrass ecosystems. BioScience 56, 987–996.
Peñalver, M. M., Durako, M. J., Furman, B. T., and Hall, M. O. (2020). Multiple stressors result in reduced reproductive effort by Thalassia testudinum in Florida Bay, USA. Mar. Ecol. Prog. Ser. 647, 65–78. doi: 10.3354/meps13395
Pereira, F. J. S., Costa, C. A. G., Foerster, S., Brosinsky, A., and de Araújo, J. C. (2019). Estimation of suspended sediment concentration in an intermittent river using multi-temporal high-resolution satellite imagery. Int. J. Appl. Earth Obs. Geoinf. 79, 153–161. doi: 10.1016/j.jag.2019.02.009
Petraitis, P. S., Methratta, E. T., Rhile, E. C., Vidargas, N. A., and Dudgeon, S. R. (2009). Experimental confirmation of multiple community states in a marine ecosystem. Oecologia 161, 139–148.
Platt, W. J., and Connell, J. H. (2003). Natural disturbances and directional replacement of species. Ecol. Monogr. 73, 507–522. doi: 10.1890/01-0552
Procaccini, G., Olsen, J. L., and Reusch, T. B. H. (2007). Contribution of genetics and genomics to seagrass biology and conservation. J. Exp. Mar. Biol. Ecol. 350, 234–259. doi: 10.1016/j.jembe.2007.05.035
R Core Team (2020). R: A Language and Environment for Statistical Computing. Vienna: R Foundation for Statistical Computing.
Reynolds, L. K., Waycott, M., and McGlathery, K. J. (2013). Restoration recovers population structure and landscape genetic connectivity in a dispersal-limited ecosystem. J. Ecol. 101, 1288–1297. doi: 10.1111/1365-2745.12116
Robblee, M., Barber, T., Carlson, P., Durako, M., Fourqurean, J., Muehlstein, L., et al. (1991). Mass mortality of the tropical seagrass Thalassia testudinum in Florida Bay (USA). Mar. Ecol. Prog. Ser. 71, 297–299. doi: 10.3354/meps071297
Roelfsema, C. M., Phinn, S. R., Udy, N., and Maxwell, P. (2009). An integrated field and remote sensing approach for mapping seagrass cover, Moreton Bay, Australia. J. Spat. Sci. 54, 45–62. doi: 10.1080/14498596.2009.9635166
Rudnick, D. T., Ortner, P. B., Browder, J. A., and Davis, S. M. (2005). A conceptual ecological model of Florida Bay. Wetlands 25, 870–883.
Santillan, E., Seshan, H., Constancias, F., Drautz-Moses, D. I., and Wuertz, S. (2019). Frequency of disturbance alters diversity, function, and underlying assembly mechanisms of complex bacterial communities. NPJ Biofilms Microbiomes 5:8. doi: 10.1038/s41522-019-0079-4
Santos, R. O., and Lirman, D. (2012). Using habitat suitability models to predict changes in seagrass distribution caused by water management practices. Can. J. Fish. Aquat. Sci. 69, 1380–1388. doi: 10.1139/f2012-018
Scheffer, M., Carpenter, S., Foley, J. A., Folke, C., and Walker, B. (2001). Catastrophic shifts in ecosystems. Nature 413, 591–596. doi: 10.1038/35098000
Sherwood, E. T., Greening, H. S., Johansson, J. O. R., Kaufman, K., and Raulerson, G. E. (2017). Tampa Bay (Florida, USA): documenting seagrass recovery since the 1980’s and reviewing the benefits. Southeast. Geographer. 57, 294–319.
Short, F. T., and Neckles, H. A. (1999). The effects of global climate change on seagrasses. Aquat. Bot. 63, 169–196. doi: 10.1016/S0304-3770(98)00117-X
Standish, R. J., Hobbs, R. J., Mayfield, M. M., Bestelmeyer, B. T., Suding, K. N., Battaglia, L. L., et al. (2014). Resilience in ecology: abstraction, distraction, or where the action is? Biol. Conserv. 177, 43–51. doi: 10.1016/j.biocon.2014.06.008
Stipek, C., Santos, R., Babcock, E., and Lirman, D. (2020). Modelling the resilience of seagrass communities exposed to pulsed freshwater discharges: a seascape approach. PLoS One 15:e0229147. doi: 10.1371/journal.pone.0229147
Stumpf, R. P., Frayer, M. L., Durako, M. J., and Brock, J. C. (1999). Variations in water clarity and bottom albedo in Florida Bay from 1985 to 1997. Estuaries 22:431. doi: 10.2307/1353209
Unsworth, R. K. F., Collier, C. J., Waycott, M., Mckenzie, L. J., and Cullen-Unsworth, L. C. (2015). A framework for the resilience of seagrass ecosystems. Mar. Pollut. Bull. 100, 34–46. doi: 10.1016/j.marpolbul.2015.08.016
Unsworth, R. K. F., Nordlund, L. M., and Cullen−Unsworth, L. C. (2019). Seagrass meadows support global fisheries production. Conserv. Lett. 12:e12566. doi: 10.1111/conl.12566
Valentine, J. F., and Duffy, J. E. (2006). “The central role of grazing in seagrass ecology,” in Seagrasses: Biology, Ecology and Conservation, eds A. W. D. Larkum, R. J. Orth, and C. M. Duarte (Dordrecht: Springer Netherlands), 463–501.
van der Heide, T., van Nes, E. H., Geerling, G. W., Smolders, A. J. P., Bouma, T. J., and van Katwijk, M. M. (2007). Positive feedbacks in seagrass ecosystems: implications for success in conservation and restoration. Ecosystems 10, 1311–1322. doi: 10.1007/s10021-007-9099-7
van Katwijk, M. M., Hermus, D. C. R., de Jong, D. J., Asmus, R. M., and de Jonge, V. N. (2000). Habitat suitability of the Wadden Sea for restoration of Zostera marina beds. Helgol. Mar. Res. 54, 117–128. doi: 10.1007/s101520050010
van Katwijk, M. M., and Hermus, D. C. R. (2000). Effects of water dynamics on Zostera marina: transplantation experiments in the intertidal Dutch Wadden Sea. Mar. Ecol. Prog. Ser. 208, 107–118. doi: 10.3354/meps208107
Wahl, T. J. (2019). Temporal Variability of DIN, Chlorophyll, and DOC in Florida Bay following Hurricane Irma. Ph.D. thesis. Chapel Hill, NC: University of North Carolina.
Wang, X., Xiao, X., Zou, Z., Chen, B., Ma, J., Dong, J., et al. (2020). Tracking annual changes of coastal tidal flats in China during 1986–2016 through analyses of landsat images with Google earth engine. Remote Sens. Environ. 238:110987. doi: 10.1016/j.rse.2018.11.030
Waycott, M., Duarte, C. M., Carruthers, T. J. B., Orth, R. J., Dennison, W. C., Olyarnik, S., et al. (2009). Accelerating loss of seagrasses across the globe threatens coastal ecosystems. Proc. Natl. Acad. Sci. U.S.A. 106, 12377–12381. doi: 10.1073/pnas.0905620106
Wilson, S. S., Furman, B. T., Hall, M. O., and Fourqurean, J. W. (2020). Assessment of hurricane IRMA impacts on South Florida seagrass communities using long-term monitoring programs. Estuar. Coasts 43, 1119–1132. doi: 10.1007/s12237-019-00623-0
Wood, S. N. (2017). Generalized Additive Models: An Introduction with R, 2nd Edn. London: Chapman and Hall.
York, P. H., Carter, A. B., Chartrand, K., Sankey, T., Wells, L., and Rasheed, M. A. (2015). Dynamics of a deep-water seagrass population on the great Barrier Reef: annual occurrence and response to a major dredging program. Sci. Rep. 5:13167. doi: 10.1038/srep13167
Zeileis, A., Kleiber, C., Krämer, W., and Hornik, K. (2003). Testing and dating of structural changes in practice. Comput. Stat. Data Anal. 44, 109–123.
Zeileis, A., Leisch, F., Hornik, K., and Kleiber, C. (2002). Strucchange: an r package for testing for structural change in linear regression models. J. Stat. Softw. 7, 1–38.
Zhang, K., Dong, X., Liu, Z., Gao, W., Hu, Z., and Wu, G. (2019). Mapping tidal flats with landsat 8 images and google earth engine: a case study of the china’s eastern coastal zone circa 2015. Remote Sens. 11:924. doi: 10.3390/rs11080924
Zieman, J. C. (1982). The Ecology of the Seagrasses of South Florida: A Community Profile. Washington, D.C: Fish and Wildlife Service.
Zieman, J. C., Fourqurean, J. W., and Frankovich, T. A. (1999). Seagrass die-off in Florida Bay: long-term trends in abundance and growth of turtle grass,Thalassia testudinum. Estuaries 22, 460–470. doi: 10.2307/1353211
Keywords: seagrass, suspended sediment, disturbance, resilience, Florida Bay, Everglades, seagrass die-off, Hurricane Irma
Citation: Rodemann JR, James WR, Santos RO, Furman BT, Fratto ZW, Bautista V, Lara Hernandez J, Viadero NM, Linenfelser JO, Lacy LA, Hall MO, Kelble CR, Kavanagh C and Rehage JS (2021) Impact of Extreme Disturbances on Suspended Sediment in Western Florida Bay: Implications for Seagrass Resilience. Front. Mar. Sci. 8:633240. doi: 10.3389/fmars.2021.633240
Received: 25 November 2020; Accepted: 30 June 2021;
Published: 23 July 2021.
Edited by:
Frank S. Gilliam, University of West Florida, United StatesReviewed by:
Jane Caffrey, University of West Florida, United StatesWade H. Jeffrey, University of West Florida, United States
Copyright © 2021 Rodemann, James, Santos, Furman, Fratto, Bautista, Lara Hernandez, Viadero, Linenfelser, Lacy, Hall, Kelble, Kavanagh and Rehage. This is an open-access article distributed under the terms of the Creative Commons Attribution License (CC BY). The use, distribution or reproduction in other forums is permitted, provided the original author(s) and the copyright owner(s) are credited and that the original publication in this journal is cited, in accordance with accepted academic practice. No use, distribution or reproduction is permitted which does not comply with these terms.
*Correspondence: Jonathan R. Rodemann, SnJvZGUwMTBAZml1LmVkdQ==