- Ecology and Biodiversity Research Group, Biology Department, Vrije Universiteit Brussel (VUB), Brussels, Belgium
Mangrove forests are dynamic ecosystems found along low-lying coastal plains along tropical, subtropical, and some warm-temperate coasts, predominantly on tidal flats fringing deltas, estuaries, bays, and oceanic atolls. These landforms present varied hydrodynamic and geomorphological settings for mangroves to persist and could influence the extent of within-site propagule transport and subsequent local regeneration. In this study, we examined how different landform characteristics may influence local genetic diversity, kinship, and neighborhood structure of mangrove populations. To do so, we considered independent populations of Avicennia marina, one of the most abundant and widespread mangrove species, located in estuarine and coastal bay environments spread across the Western Indian Ocean region. A transect approach was considered to estimate kinship-based fine-scale spatial genetic structure using 15 polymorphic microsatellite markers in 475 adult A. marina trees from 14 populations. Elevated kinship values and significant fine-scale structure up to 30, 60, or 90 m distances were detected in sheltered systems void of river discharge, suggesting a setting suitable for very local propagule retention and establishment within a neighborhood. Slopes of a linear regression over restricted distance within 150 m were significantly declining in each sheltered transect. Contrastingly, such a spatial structure has not been detected for A. marina transects bordering rivers in the estuarine systems considered, or alongside partially sheltered creeks, suggesting that recruitment here is governed by unrelated carried-away mixed-origin propagules. South African populations showed strong inbreeding levels. In general, we have shown that A. marina populations can locally experience different modes of propagule movement, explained from their position in different coastal landforms. Thus, the resilience of mangroves through natural regeneration is achieved by different responses in coastal landforms characterized by different hydrodynamic conditions, which can be important information for their management and protection within the variety of coastal environments.
Introduction
The ability of a species to persist in changing environments depends on the interacting effects of phenotypic plasticity, the potential to adapt to new conditions, and the capacity to disperse and colonize suitable habitats (Hampe, 2011; Reed et al., 2011; Urban et al., 2014; Fox et al., 2019). Hence, in order to predict the response of species to changing environmental conditions, it is important to understand the physical and biological processes that structure their dispersal and connectivity patterns. Such knowledge provides insight into the ecology and biogeographical ranges of species; nonetheless, it is also being increasingly used to inform their local conservation (Balbar and Metaxas, 2019).
Direct measurements of dispersal pathways and estimation of connectivity can be challenging, particularly in nearshore marine and intertidal coastal species where the transport of offspring may cover extensive distances. Gene flow in these systems is determined by the interaction of coastal topography, habitat availability, and “chaotic” flow fields (Banks et al., 2007; Johansson et al., 2008; Nicastro et al., 2008; White et al., 2010; Durrant et al., 2018), resulting in connectivity patterns that are typically stochastic in nature (Siegel et al., 2008; Williams and Hastings, 2013). As an example, erratic on-shelf currents were found to restrict dispersal and limit population connectivity in a coastal snail species with long pelagic larval duration (Teske et al., 2015). Habitat heterogeneities may influence dispersal (and establishment) and thus create distinct patterns of spatial genetic diversity. Coastal bays, for example, may act as retention zones promoting settlement and recruitment, due to reduced flow rates, recirculation, and oceanographic features such as fronts and eddies that originate from the interaction of alongshore coastal currents with headlands (Graham and Largier, 1997; Archambault and Bourget, 1999; Shanks et al., 2003; Roughan et al., 2005). Estuaries are characterized by flow dynamics at the interface between freshwater river discharge and saline coastal waters, whereas sheltered parts of coastal bays undergo circulation dynamics in the absence of river flow. Differences in genetic structure and diversity have been observed between bays and open coasts for a variety of species associated with differences in hydrodynamic stress (Nicastro et al., 2008). Similarly, evidence for genetic differentiation between estuarine and open-coast ecotypes of the seaweed Hormosira banksii has been found (Coleman et al., 2018). Despite the importance of identifying the influence of environmental settings on a species’ dispersal characteristics and the genetic structure of populations, only recently did a few studies consider potential differences in genetic structure and diversity between mangrove populations that occupy different environmental settings (Hasan et al., 2018; Chablé Iuit et al., 2020; Triest et al., 2020), whereas several fine-scale genetic structure (FSGS) analyses delivered relevant information and revealed patterns at the within-site level (Céron-Souza et al., 2012; Mori et al., 2015; Millán-Aquilar et al., 2016; Do et al., 2019).
Mangroves represent characteristic intertidal forests along the coastline of tropical, subtropical, and some warm-temperate regions. They function as important nursery habitats for a variety of fish species and invertebrates (Lefcheck et al., 2019), and they support the livelihoods of coastal human populations as a source of food (e.g., fish) and wood products (Barbier et al., 2011). Their robust and complex aerial root networks dissipate wind and swell waves and present a natural defense against coastal erosion and storm damage (Gedan et al., 2011; Temmerman et al., 2013). Moreover, mangroves have been recognized in the context of climate change mitigation through their important role in carbon sequestration (Howard et al., 2017). Despite the numerous ecosystem services that mangroves provide, they are facing a number of threats across different parts of their range, such as the conversion to aquaculture and agriculture, urban development, and pollution, driving deforestation and fragmentation (Richards and Friess, 2016; Thomas et al., 2017; Bryan-Brown et al., 2020).
The genetic structure and diversity of mangrove populations is determined by the cumulative effect of insect, wind, and bird pollination (Hermansen et al., 2014; Wee et al., 2015) and the transport, establishment, and survivorship of water-buoyant propagules (Rabinowitz, 1978). Hence, genetic structure in these systems depends on the interaction of biological factors, such as propagule abundance, predation, and establishment (e.g., Rabinowitz, 1978; Smith, 1987; McKee, 1995; Clarke et al., 2001; Balke et al., 2011), and abiotic factors, such as the local atmospheric and oceanographic circulation regimes (including tides) (e.g., Stieglitz and Ridd, 2001; Van der Stocken et al., 2015, 2019b). The relative influence of these factors may differ strongly among the coastal settings where mangroves are found, including bays, estuaries, and atolls, and may control local establishment and forest structure (Thom, 1982; Twilley, 1995). Rhizophora mangle L. showed FSGS in different hydrological conditions of Caribbean mangroves (Yucatan, Mexico) broadly categorized as a bay, lagoon, or coast and indicated an extended structure along a river (Chablé Iuit et al., 2020). A comparison of FSGS in Avicennia and Rhizophora species from the same coastal area demonstrated a longer distance dispersal effect of the larger-sized and elongated propagules of Rhizophora. Avicennia mostly shows higher kinship values over similar within-site distances than Rhizophora (Céron-Souza et al., 2012; Do et al., 2019).
In this study, we tested the hypothesis that, through its influence on dispersal and establishment, differences in the physical nature of these coastal landforms influence the extent of within-site genetic structure in Avicennia mangrove populations. FSGS results from propagule dispersal within a neighborhood and settlement processes in the near past, of which the effects have structured populations of current adult trees. Bays are expected to harbor sheltered zones, whereas mangroves in estuarine habitats are also exposed to the hydrodynamic forces provided by river flow. More specifically, based on a genetic diversity analysis of A. marina populations positioned within a variety of coastal bay and estuarine environments, we aimed to (1) examine and compare FSGS for populations in different physical settings and (2) estimate which coastal landform would allow for a locally established structure. To do so, we examined FSGS of the pioneer mangrove species A. marina (Forsk.) Vierh., using samples from 14 populations located in different coastal settings as could be found in the Western Indian Ocean (WIO) region. To ensure a high resolution for detecting kinship-based FSGS, we selected 15 nuclear microsatellite markers that were polymorph at the within-site level.
Materials and Methods
Study Area
We considered mangrove populations from different coastal bay and estuarine environments that are well separated geographically, an important condition to test our hypothesis by avoiding pseudo-replicates within the same local system or along the same river. We sampled either in sheltered zones without any direct influence from river discharge in the bay or in fringing tidal rivers in estuarine systems. We assume that contrasting local hydrodynamics associated with different coastal landforms will reflect different FSGSs regardless of their inter-population variability at the regional scale, as it concerns a within-site process. Data were collected from 14 different mangrove populations in the WIO region (Figure 1), located in an overarching broad category of either a coastal bay or estuarine setting, though showing important differences in their physical nature (Table 1). More specifically, sampled populations are located along the coastline of Mozambique (MOZ1–MOZ6), the Republic of South Africa (SAF1–SAF4), northwestern Madagascar (MAD1 and MAD2), and the oceanic islands Mayotte (MAY) and Europa (EUR). Eight populations are from coastal bays (of which six represent a sheltered environment void of rivers and two with tidal creeks), and six populations are bordering a river within an estuarine environment (Supplementary Figure 1 and Table 1). Variability of environmental settings could be noted. Within a broad “coastal bay” class, MOZ4 is a population along a sand bar though also expanding further across a wide creek, and MOZ6 was located along a creek, thereby exposed to hydrokinetic energy form multiple directions, in contrast to six sheltered populations void of creeks or continuous river flow (Supplementary Figure 1). In the “estuary” class, two river populations are located in an estuary with temporarily closed mouth conditions (SAF1 and SAF3), restricting tidal exchange (Supplementary Figure 1). Additionally, SAF4 consists of a small estuarine population that was sampled partly along a river and partly in a higher intertidal patch.
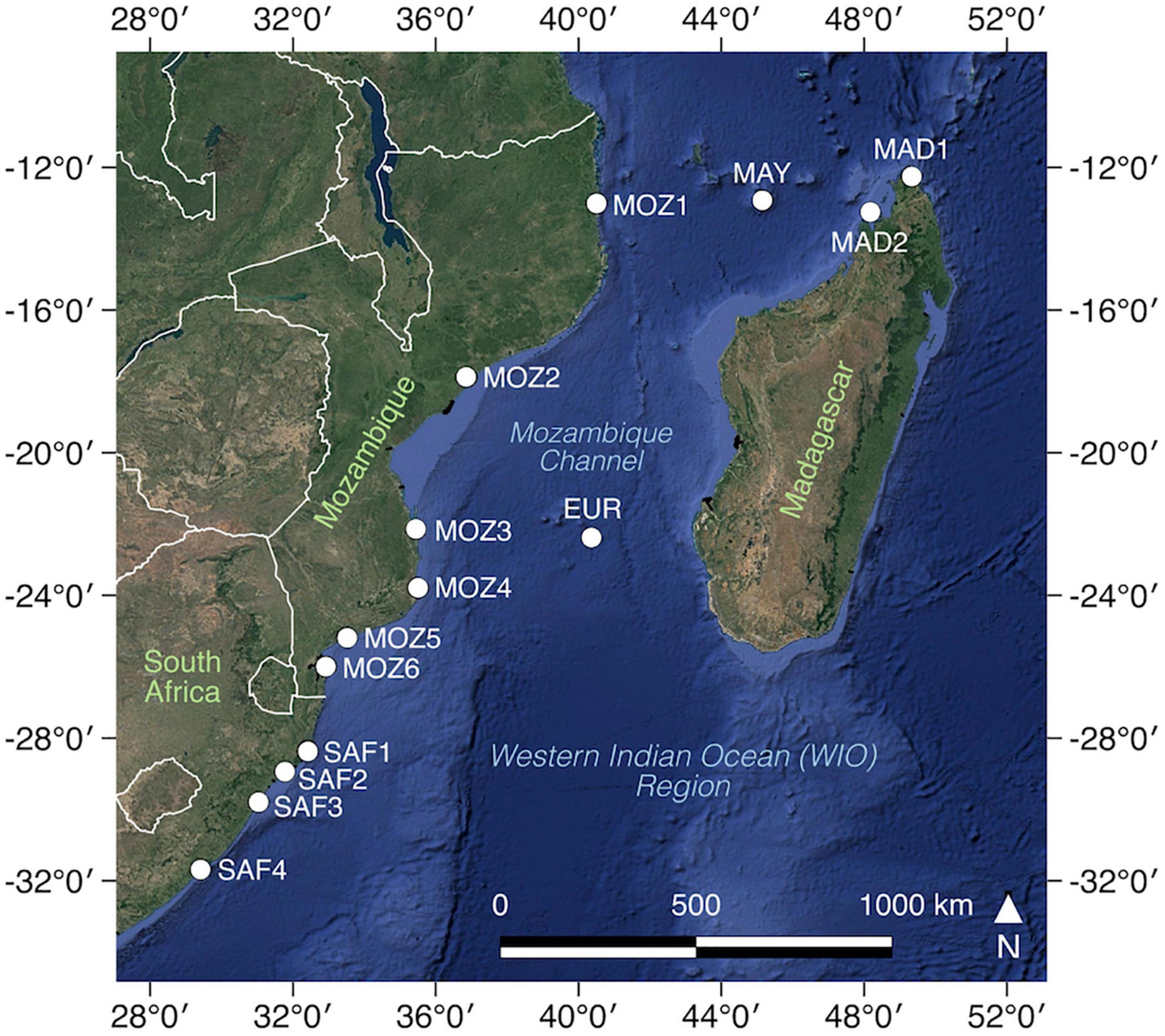
Figure 1. Sampled Avicennia marina populations in the Western Indian Ocean Region. Population codes are denoted in Table 1. Coastal landform maps with transect details of each population are in Supplementary Figure 1. Map generated using the Quantum Geographical Information System, version 2.18.13 (www.qgis.org). Basemap source: Google Satellite. Country boundaries are from Natural Earth.
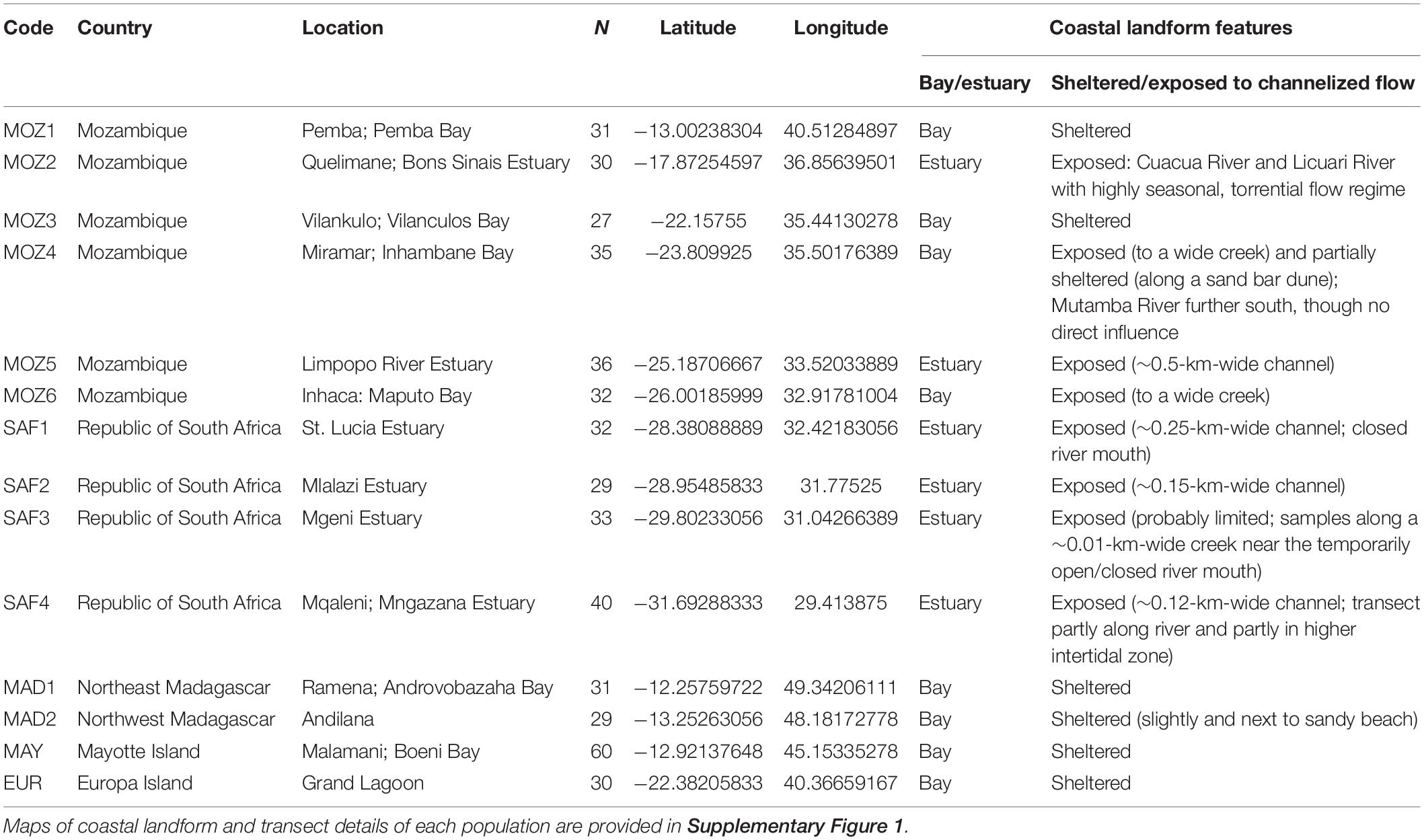
Table 1. Location details and coastal landform features of 14 Avicennia marina populations in the Western Indian Ocean region, considered for fine-scale genetic analysis.
Study Species and Sample Collection
Avicennia marina is a widely distributed and pioneer mangrove species found across the Indo-Pacific, between latitudes 25°N and 38°S. In the studied area of the WIO, this mangrove species is very common. A total of 475 A. marina individual trees were sampled (Table 1). GPS coordinates of every individual tree along each transect were taken. Transect locations either were in a non-channelized, tidal-influenced sheltered area or, alternatively, were at close hydrological proximity along the edge of a river or across a creek. Transects were 200 to 4,680 m depending on the available mangrove patches within the area. Gaps between mangrove patches were included in the total distance because the fine-scale analysis focused on pairs of individual trees within short distances of 150 m, regardless of the patch where they occur. The number of sampled trees was 34 on average and ranged from 26 to 40 per transect. Short-distance intervals of at least 30 m were considered whenever A. marina trees were present over that short distance.
DNA Extraction and Microsatellite Primers
Genomic DNA was extracted from approximately 20 mg of dried leaf tissue using the EZNA SP plant DNA Mini Kit (Omega Bio-Tek, Norcross, GA, United States). Two multiplex polymerase chain reactions (PCRs) consisted of 15 microsatellite markers in total, chosen for their allelic polymorphism within a population, hence suitable for fine-scale analysis (Supplementary Table 1). Seven markers for A. marina were previously developed by Geng et al. (2007), three by Maguire et al. (2000), one by Teixeira et al. (2003), and four by Triest et al. (2020). Primers were fluorescent-labeled with four different dye labels (6FAM/VIC/NED/PET), and a primer mix was made by mixing 0.2 μM of each primer together. Multiplex PCRs consisted of 6.25 μl Master Mix (Qiagen Multiplex PCR Kit), 1.25 μl primer mix, 2.5 μl H2O, and 2.5 μl genomic DNA. PCR was performed in a thermal cycler (Bio-Rad MyCycler) with the following conditions: an initial denaturation of 95°C for 15 min followed by 35 cycles of 30 s denaturation at 95°C, 90 s annealing at 57°C, and 80 s elongation at 72°C followed by a final extension of 30 min at 60°C. PCR products were separated on an ABI3730xl sequencer (Macrogen, Seoul, South Korea), and allele sizes were determined with GeneMarker V2.60 (SoftGenetics LLC, State College, PA, United States).
Genetic Analyses
Prior to population- and individual-based data analyses, we tested for genotypic disequilibrium, potential null alleles, and overall resolution of the 15 microsatellite markers in A. marina. A linkage test between all pairs of loci (1,000 permutations) gave no genotypic disequilibrium at the 0.05 level using FSTAT (v.2.9.3) (Goudet, 2001). No scoring errors, large allele dropouts, or null alleles were indicated using MICRO-CHECKER (van Oosterhout et al., 2004). The probability of identity (PI), namely, whether two individuals could share an identical multilocus genotype by chance using GenAlEx (v.6.5; Peakall and Smouse, 2012), gave a cumulative probability of identity for all polymorphic loci in each site of 1.7 × 10–2 to 7.9 × 10–9, thereby providing ample resolution, even for siblings, potentially present in our fine-scale sampling design, which reached a PI of 1.2 × 10–2 to 4.2 × 10–4.
Basic descriptive population genetic variables of A. marina were measured for each site: total number of alleles (A), mean number of alleles (AM), effective number of alleles (AE), allelic richness (AR) for 17 diploid samples, observed heterozygosity (HO), unbiased expected heterozygosity (uHE), and population inbreeding coefficient (FIS)—with a 1,000-permutation test—using FSTAT and GenAlEx. The descriptive genetic structure among sites (FST), inbreeding within sites (FIS), overall inbreeding (FIT), and a pairwise genotypic differentiation matrix (FST) were calculated via AMOVA-FST at 999 random permutations using GenAlEx v.6.5. The overall FIJ kinship coefficient (Loiselle et al., 1995) for all pairs of individuals of within-site comparisons was tested as a first exploratory approach and obtained for an equal number of pairwise comparisons (in casu 825 pairs) within each class. These were generated at 10 distance classes of 0.04, 0.08, 0.14, 0.22, 0.31, 0.45, 0.77, 1.20, 1.95, and 4.68 km, as obtained by SPAGeDi 1.5a (Hardy and Vekemans, 2002) and using the within category as a reference. On the basis of that overall outcome and obtained significant kinship values, we then subsequently tested the FIJ kinship coefficient again for all within-site comparisons using five shorter equal distance classes within 0.5 km (0–0.1, 0.1–0.2, 0.2–0.3, 0.3–0.4, and 0.4–0.5 km) and within 0.15 km (0–0.03, 0.03–0.06, 0.06–0.09, 0.09–0.12, and 0.12–0.15 km). The latter distance classes yielded the best resolution and were considered throughout this study to estimate the fine-scale spatial autocorrelation of individuals of mangrove populations for each transect level (N = 14). We computed the slope (-b) of linear regressions between pairwise genetic coefficients and geographical distance over the restricted distance of 0.15 km, using SPAGeDi with 1,000 permutations. The Sp-statistic which is proposed as an informative parameter about survival strategy for diploids was calculated as Sp = −blog/(1 − F1) (Vekemans and Hardy, 2004), where blog is the slope of the ln regression and F1 represents the average kinship coefficient (FIJ) between neighboring individuals in the first distance class (0–30 or 0–60 m in this study).
A Fisher exact test for a 2 × 2 contingency table was obtained for the presence/absence of FSGS and for two scenarios with the predictor groups “bay” (N = 8) versus “estuary” (N = 6) and the predictor groups “sheltered” (N = 6) versus “exposed” (N = 8) (see Table 1 for these categories of each population). Additionally, a Mann–Whitney U-test was performed for the same two scenarios using three informative variables of an FSGS: the Fij kinship values of the significant distance class (zero in case of no FSGS), the b-slope values, and the Sp-statistic value. Two groups of sites classified under “sheltered” (N = 6) or “exposed” (N = 8) were each considered for a spatial autocorrelation within 0.15 km (0–0.03, 0.03–0.06, 0.06–0.09, 0.09–0.12, and 0.12–0.15 km) and tested for significance with 1,000 permutations using each within category as a reference. The same two groups (sheltered and exposed) were tested for differences in their AR, HO, HE, FIS, and FST using 1,000 permutations in FSTAT.
Results
Genetic Diversity Levels and Overall Structure
A total of 92 (22–65 per population) alleles were observed in the 15 loci considered, with a mean number of alleles (AM) ranging between 1.5 and 4.3, an effective number of alleles (AE) between 1.2 and 2.6, and an adjusted allelic richness (AR) between 1.4 and 3.9 (Table 2). The overall observed heterozygosity (HO = 0.251) was lower than the expected heterozygosity (uHE = 0.315). The within-population inbreeding (mean FIS = 0.232; p < 0.001) ranged from 0.094 to 0.642 and was significant for eight transects, of which the South African estuarine mangrove populations showed the highest level of inbreeding (Table 2). Overall, we observed very similar amounts for basic population genetic variables for A. marina populations in the different coastal landforms. The lowest allele and gene diversities, coinciding with the highest inbreeding values, were observed in two South African estuarine populations (SAF1 and SAF2). However, low but significant levels of inbreeding could also be observed in the highly diverse coastal bay populations of MOZ1, MAY, and EUR.
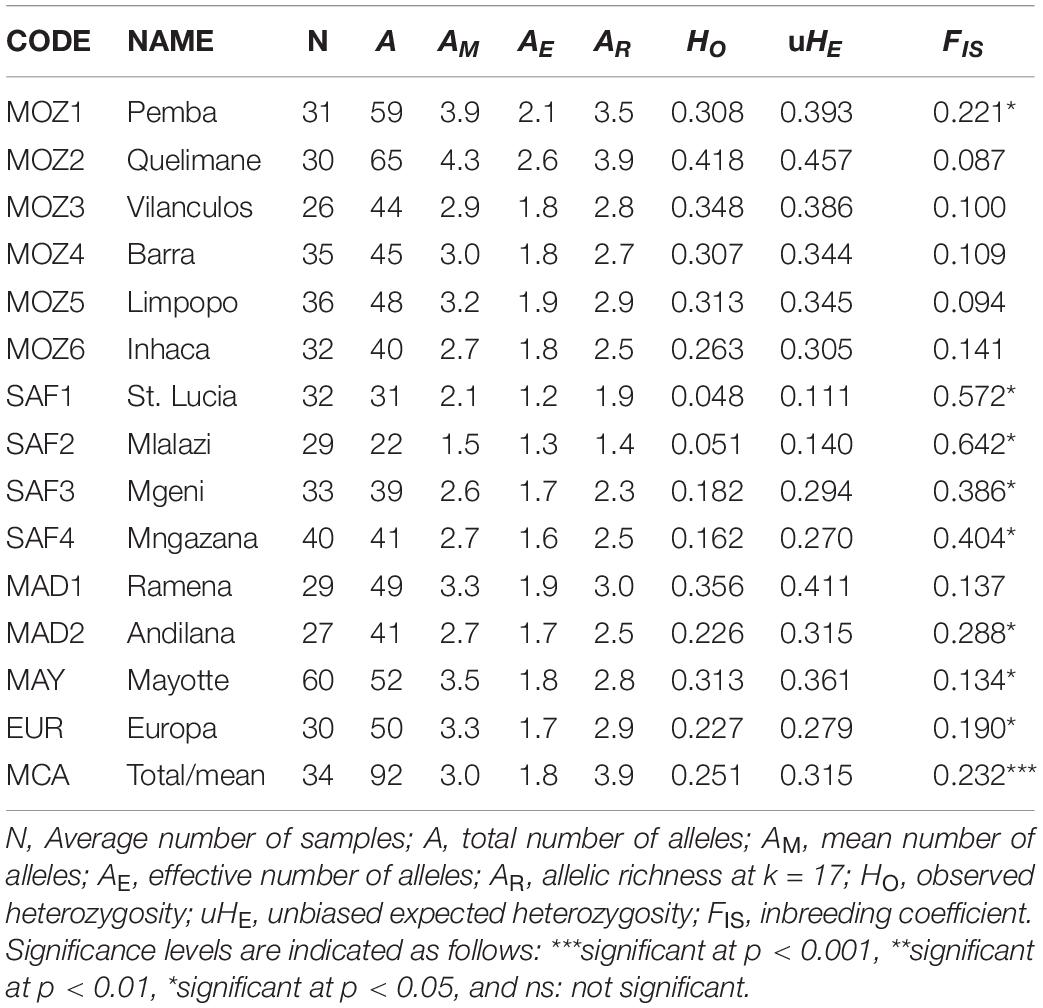
Table 2. Population genetic variables of 14 Avicennia marina populations in the Western Indian Ocean region and used for fine-scale genetic structure analysis.
AMOVA results revealed that only 16% of the genetic variation was explained among individuals, whereas 54% of A. marina genetic variation came from within individuals and 30% was explained among the different populations (Table 3). Pairwise genetic differentiation ranged from 11% to 65% with all populations being significantly different at p < 0.001 (Supplementary Table 2). Estimates of genetic divergence were lowest along the coastline of Mozambique (e.g., FST = 0.109 between MOZ3 and MOZ5; FST = 0.100 between MOZ2 and MOZ3) and highest along the South African coast (e.g., FST = 0.648 between SAF1 and SAF2, Supplementary Table 2).
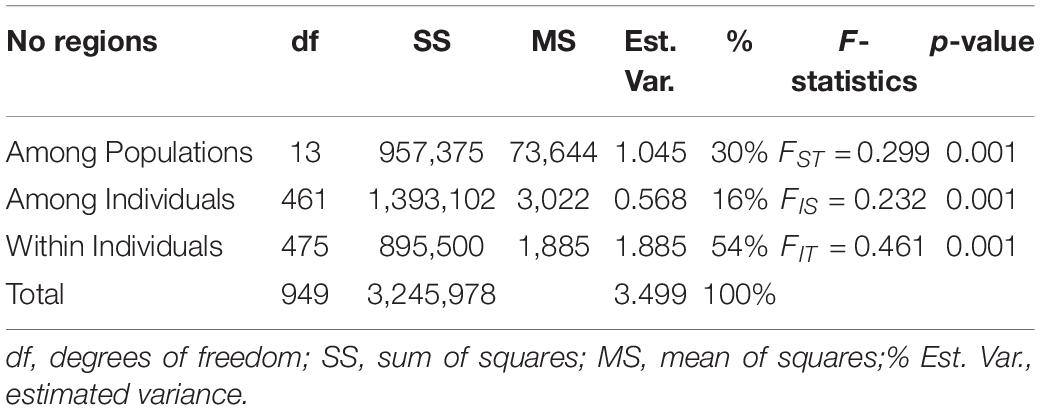
Table 3. Summary of AMOVA and F-statistics of Avicennia marina for 14 populations in the Western Indian Ocean region.
Fine-Scale Genetic Structure
The overall FIJ kinship coefficient for all within-site comparisons considering even sample sizes in 10 distance classes revealed positive kinship values within the shortest distance classes of 0.04 km (FIJ = 0.023; p < 0.001) and of 0.08 km (FIJ = 0.009; p = 0.015; Figure 2). The kinship value (FIJ) decreased significantly over the full distance (slope of a linear regression b = −0.009 at p = 0.007). A detailed analysis of FSGS for each separate A. marina transect revealed an intra-individual kinship ranging from FIJ = 0.087 to FIJ = 0.649, the latter being in agreement with the population inbreeding values (Table 4). When considering distance classes of 30, 60, 90, 120, and 150 m, in order to detect a spatial structure from the autocorrelation of individuals within each transect separately (N = 14), most of the significant elevated kinship values appeared within 30 and 60 m, and once at 90 m (Table 4). The following populations showed a significant FSGS up to 30 m (MAY and EUR), up to 30 and 60 m (MAD1 and MAD2), up to 60 m (MOZ3), or up to 90 m (MOZ1). In fact, these six sheltered populations showed FSGS, not only for the multiple loci but also for several (three to five) single loci (Table 4). The slopes of the linear regressions (over restricted distance within 150 m) were all declining and ranged from b = −1.223 (p = 0.002) in MAD2 to b = −0.154 (p = 0.054) in MAY (Table 4). The regression slope was not significant when higher kinship values were further at 60 or 90 m. Most estuarine populations along the African coast did not show any sign of spatial structure (MOZ2, MOZ4, MOZ5, MOZ6, SAF1, and SAF3) for multiple loci and were not informative for any single locus (Table 4). Only SAF2 and SAF4 showed higher kinship values at 30 m, though only for one or two loci, which coincides with homozygosity, fixed loci, and pronounced inbreeding (Table 2). The outlier value for SAF2 (Table 4) should be interpreted cautiously because of a limited number of pairwise comparisons giving a coefficient of variation lower than 50%, which is considered insufficient. SAF4 consists of a small estuarine population that was sampled partly along a river and partly in a higher intertidal, but sample numbers were too low to analyze both conditions separately.
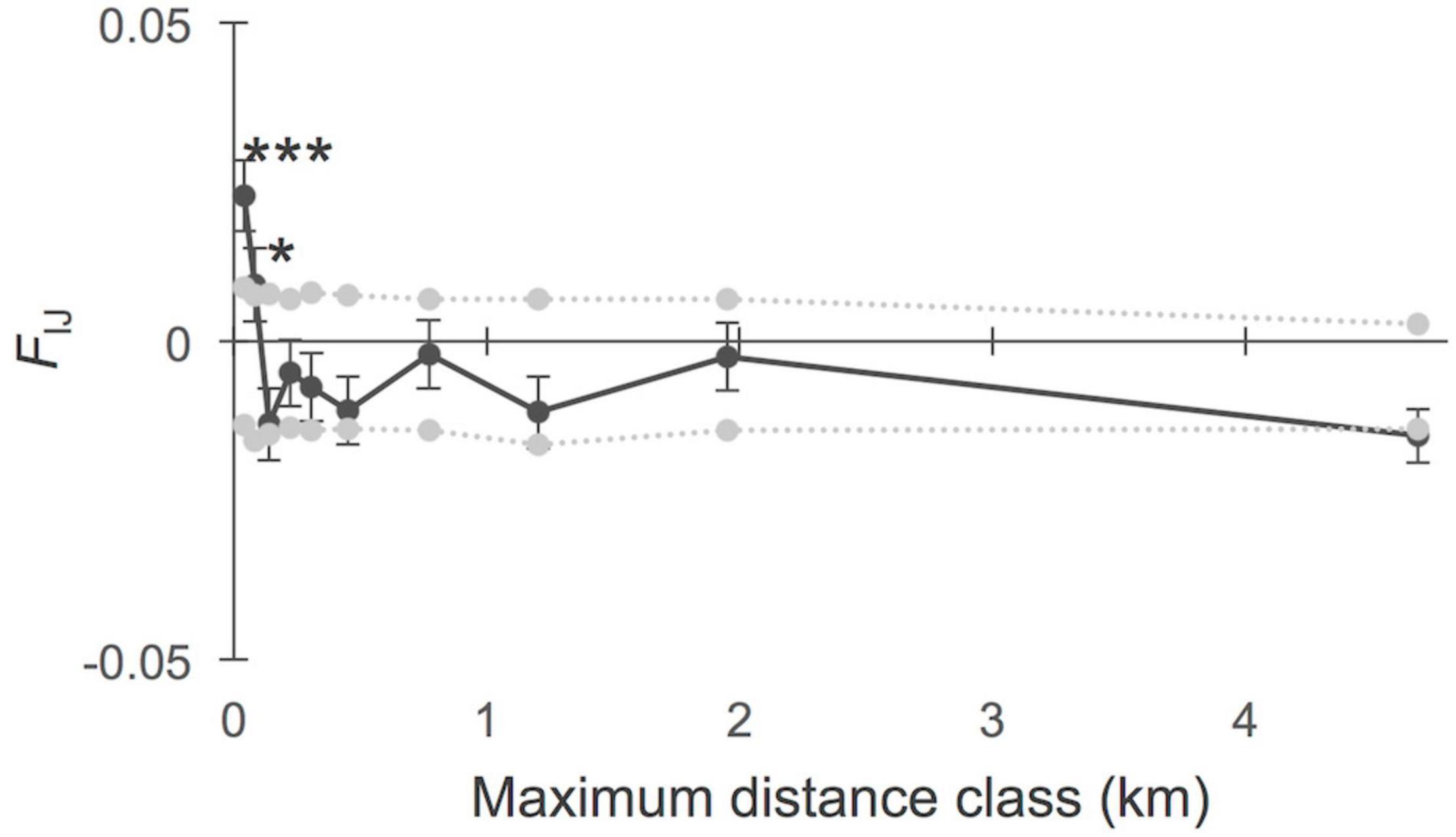
Figure 2. Fine-scale genetic structure of Avicennia marina populations (N = 14) from mangroves in the Western Indian Ocean region, using even sample size over 10 distance classes. Significant elevated kinship (FIJ) values were only within the shortest distance classes of 41 m (***p < 0.001) and 84 m (*p < 0.05) though not observed beyond hundreds of meters or a few kilometers. The slope of the regression over the full distance was b = –0.009 (p < 0.01).
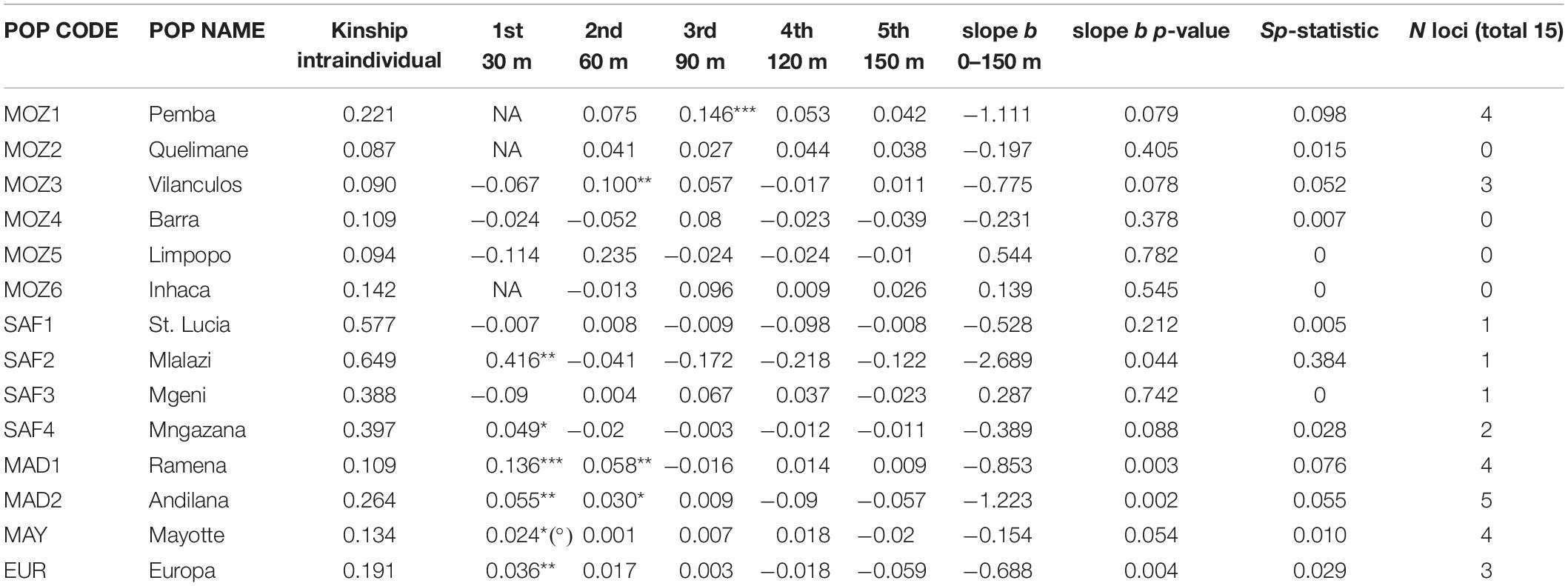
Table 4. Kinship values for five distance classes and linear slope b over 0–150 m for 14 Avicennia marina populations in the Western Indian Ocean region (***p < 0.001; **p < 0.01; *p < 0.05); Sp-statistic as an informative parameter for survival strategy; (°) FIJ of Mayotte within 15 m; NA, not applicable because of the too low proportion of individuals represented in the interval; N loci, the number of single loci out of 15 that showed significant higher kinship values for at least one of the distance classes.
A Fisher exact test of the presence/absence of FSGS for the predictor groups “bay” versus “estuary” was non-significant, whereas it was found significant (p < 0.01) for the predictor groups “sheltered” versus “exposed.” A Mann–Whitney U-test for comparison of the same abovementioned groups for their b-slope (of the FSGS) appeared non-significant for the groups “bay” versus “estuary” in contrast to the groups “sheltered” versus “exposed” which were found to be different at p = 0.04. A similar Mann–Whitney U-test for kinship value was not significant for “bay” versus “estuary,” whereas “sheltered” versus “exposed” was different at p = 0.02.
The Sp-statistic was zero or extremely low (Sp = 0–0.007) for five “exposed” sites fringing a river (MOZ4, MOZ5, MOZ6, SAF1, and SAF3) but were most elevated (Sp = 0.010–0.098) for six “sheltered” sites (MOZ1, MOZ3, MAD1, MAD2, MAY, and EUR). Within the “estuary” category, MOZ2 and SAF4 had an intermediate value (Sp = 0.015 and 0.028, respectively), whereas SAF2 showed an outlier value (Sp = 0.384). The latter outlier corresponds to the site with the lowest allelic richness (AR = 1.4), highest inbreeding levels (FIS = 0.642), and a coefficient of variation < 50%, hence uninformative. A Mann–Whitney U-test showed that the Sp-value was significantly higher for the category “sheltered” (N = 6) than for “exposed” (N = 8; p = 0.02).
Based on these findings, we considered “sheltered” versus “exposed” systems for further fine-scale spatial autocorrelation estimates beyond the site level. The analysis of FSGS within the six populations of the category “sheltered,” considering five distance classes of 30 m interval (Figure 3), revealed significant kinship values for the shortest distance classes at 30 m (FIJ = 0.031, p < 0.001; N pairs = 455) and 60 m (FIJ = 0.017, p = 0.002, N pairs = 450). The linear slope of the regression over 150 m was b = −0.561 (p < 0.001). The eight “exposed” sites (Figure 4) showed no significant FSGS with weak kinship values at 30 m (FIJ = 0.015, p > 0.05, N pairs = 140). The linear slope of the regression over 150 m was b = −0.350 (p > 0.05). A comparison of population genetic variables between the two groups of coastal settings considered in this study revealed no significant (p > 0.05) differences in their levels of AR, HO, HE, FIS, or FST, thereby indicating the local effect of coastal landforms on FSGS, regardless of the basic diversity of populations from independent systems.
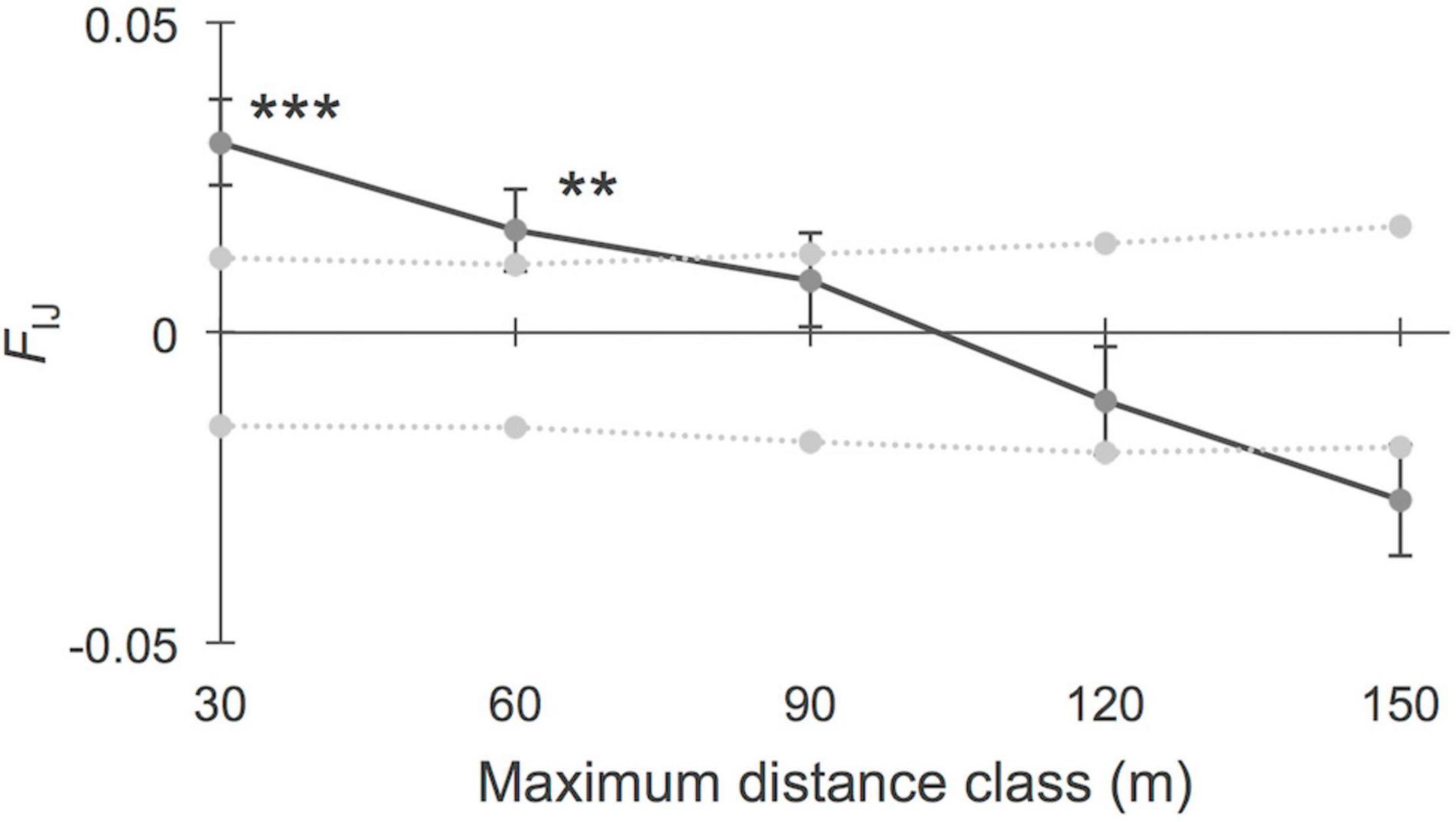
Figure 3. Fine-scale genetic structure of Avicennia marina populations (N = 6) from sheltered mangroves in bays in the Western Indian Ocean region. Five distance classes of 30 m interval showed significant kinship (FIJ) values for the first distance classes (***p < 0.001; **p < 0.01). The slope of the regression over 150 m was b = –0.56 (p < 0.001).
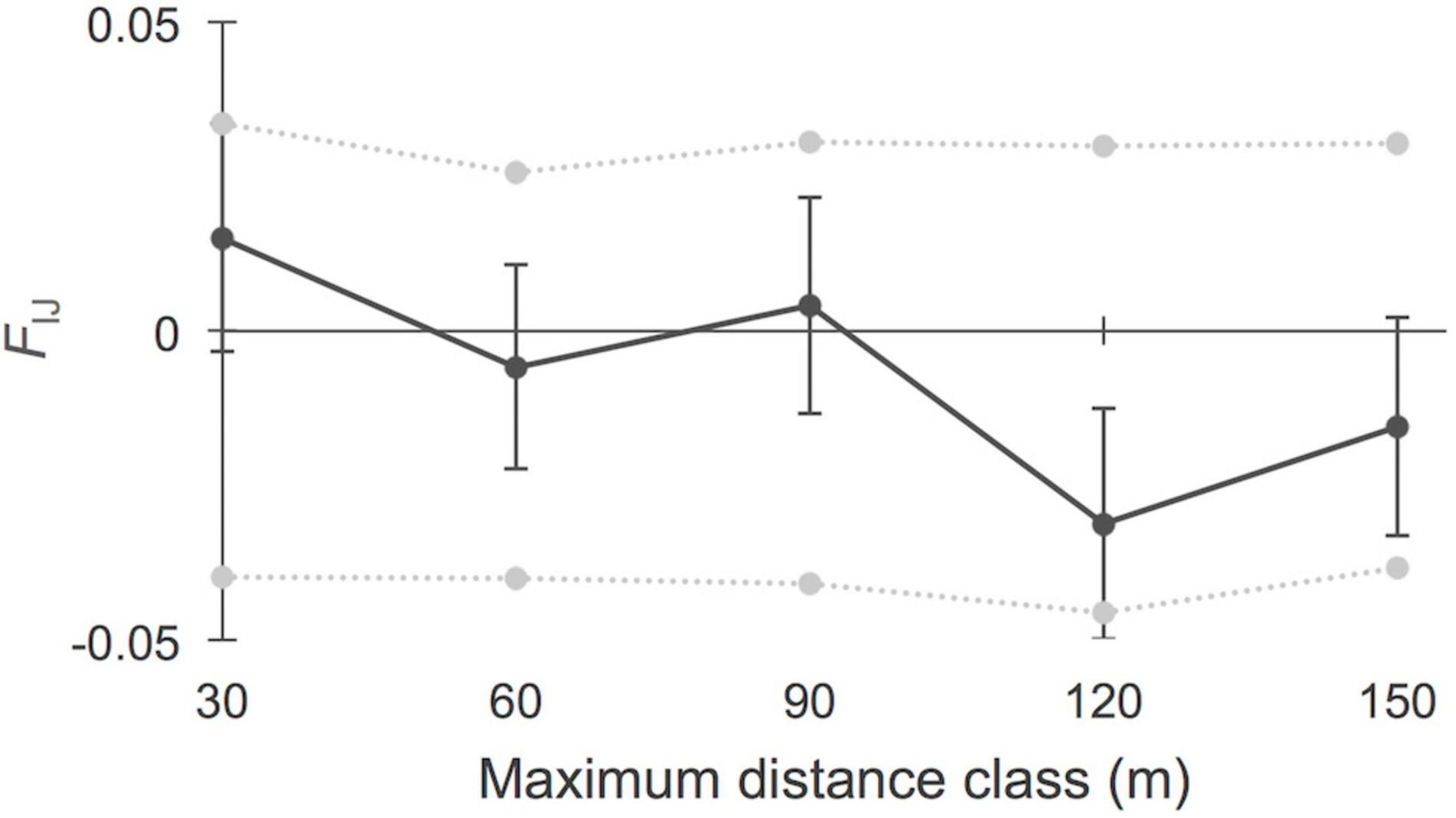
Figure 4. Fine-scale genetic structure of Avicennia marina populations (N = 8) along exposed mangroves bordering estuarine rivers and creeks in the Western Indian Ocean region. Five distance classes of 30 m interval showed no significant kinship (FIJ) values. The slope of the regression over 150 m was b = –0.35 (non-significant).
Discussion
Mangroves typically thrive in low-energy coastal habitats, such as tidal flats fringing estuaries and coastal bays. The geophysical energies in these environments still may differ strongly. Estuaries are characterized by flow dynamics at the interface between freshwater river flow and saline coastal waters, whereas coastal bays undergo circulation dynamics where river discharge may be absent. In this study on the widely distributed mangrove species A. marina, we estimated the potential effect of different coastal landforms on the fate of propagule dispersal and establishment of populations, as indicated from their FSGS. Since local hydrodynamics control the within-site distribution of mangrove propagules and influence seedling establishment, variations in hydrodynamic forces between these environments resulted in observable variations of FSGS.
Our results revealed a clear tendency for FSGS over short distances of 30 to 60 m in the sheltered populations considered in our study. These environmental settings are presumed to be of lower hydrodynamic energy, such as found in high intertidal A. marina zones (Triest et al., 2020). Obviously, when sheltered conditions are prevailing throughout the life span of an A. marina patch, an FSGS may remain detectable. Of the high propagule load that can be expected from the high fecundity values observed for A. marina (Clarke, 1992), only a reduced proportion may viably reach a suitable habitat, anchor, and develop into fully grown adult trees (Dahdouh-Guebas et al., 1998; Balke et al., 2011). Reduced flow rates may restrict dispersal and promote local establishment and regeneration, with the established trees thus leaving a trace of elevated kinship values compared to the population’s average kinship value. Such traces have also been detected in other studies within spatial stretches of a few meters up to several hundreds of meters (Mori et al., 2015; Do et al., 2019; Chablé Iuit et al., 2020; Triest et al., 2020), and it has been shown that kinship can also be enhanced when populations became severely fragmented and confined within artificial dikes (Hasan et al., 2018). Sp-values for A. marina, whenever showing FSGS, are among the ranges reported for mixed mating systems in general (Vekemans and Hardy, 2004) and, more importantly, a dispersal mode suggesting local conditions of retention. FSGS detectable up to distances of 30 m (or 60 m) corresponded to more sheltered environmental settings that are void of large rivers. The Sp-values that we obtained for A. marina in sheltered sites (Sp = 0.010–0.098, average Sp = 0.053) are mostly higher than the Sp-values previously reported for Avicennia germinans (L.) Stearn populations from Northwestern Mexico, which ranged from Sp = 0.002 to 0.015 in adult trees, although these could increase for saplings up to Sp = 0.035 (Millán-Aquilar et al., 2016).
In contrast, our findings showed that dynamic conditions for non-sheltered areas, resulting from the interplay of physical forces such as river flow, tides, and winds, left no trace of FSGS. Reduced levels or absence of FSGS can be expected particularly for lower-intertidal mangroves fringing the same water channel (e.g., MOZ5, SAF1, and SAF3), where longer hydroperiods and the interaction of river flow and tides may promote high longitudinal (i.e., along-channel) connectivity. This is consistent with recent findings from a study exploring the FSGS along eight A. marina transects, in relation to the local water channel network structure (Triest et al., 2020). In that study, admixed gene pools without any FSGS were found in mangrove sites located along an estuarine river channel that runs near parallel to the directionality of the tidal currents. In addition, the lack of a clear genetic structure for most along-channel sites, even at distances as short as 5–20 m, resulted in a very low Sp-statistic (Sp = 0.001–0.008; Triest et al., 2020) that is within the low range of Sp-values that we found in this study for non-sheltered sites. An overall estimate of Sp = 0.019 was obtained for A. germinans in Caribbean and Pacific estuaries of Panama (Céron-Souza et al., 2012). The first distance classes considered in each of these studies and our study are comparable, being 0–30 or 0–60 m (this study), 0–50 m (Millán-Aquilar et al., 2016), and 0–100 m (Céron-Souza et al., 2012). However, despite comparable minimal distances, the range of Sp-values for these Avicennia species is only comparable for the non-sheltered systems considered in this study, whereas Sp-values for our “bay” systems are among the highest and correspond to gravity dispersal (hence a very restricted/short-distance dispersal).
Avicennia trees produce water-buoyant propagules that consist of a single embryo surrounded by a thin pericarp (Tomlinson, 2016). As these propagules are rather small, “resembling flattened olives” (Rabinowitz, 1978), retention rates during dispersal through the forest’s root and stem network are expected to be low, compared to propagules from other mangrove species (Van der Stocken et al., 2019b). Several studies on A. marina have reported propagule floating and viability periods of several days to weeks (Clarke et al., 2001; Clarke and Myerscough, 1991; Steinke, 1986), but floating periods of several months have been reported in other Avicennia species (Alleman and Hester, 2011; Rabinowitz, 1978) and may vary greatly among estuaries (Steinke, 1986). Gene dispersal of A. germinans and R. mangle was considered to remain usually limited within estuaries (Céron-Souza et al., 2012). A comparison of FSGS in Avicennia and Rhizophora species from the same coastal site allows us to examine the potential effect of the larger-sized and elongated propagules of Rhizophora. Avicennia mostly shows higher kinship values over similar distances than Rhizophora (Céron-Souza et al., 2012; Do et al., 2019). R. mangle showed an FSGS up to 90 m in different hydrological conditions of Caribbean mangroves (Yucatan, Mexico) such as a bay, lagoon, or coast and up to 240 m along a river (Chablé Iuit et al., 2020). These most likely reflect lower hydrodynamic energy when compared to the Cameroon estuary complex in a high-rainfall area where Rhizophora racemosa showed no or only limited autocorrelation within 25 m (Ngeve et al., 2017).
Propagule loads that encounter no or few nearby physical barriers while being transported away from the location of the parent tree may become in a sense diluted and mixed with unrelated propagules from other local provenances and settle as a mixture from different cohorts at suitable locations. For mangrove patches resulting from the establishment of such “mixed” propagule cohorts, the kinship value becomes very low or is usually absent and shows no relationship with the neighborhood, hence no FSGS. The Sp-values (often 0 but up to Sp = 0.028) that we found for A. marina in sites bordering a river or a creek is among the ranges reported for outcrossing trees in general (Vekemans and Hardy, 2004) and of dispersal over considerable distances beyond the near surroundings of the parent tree. While most sites fringing a river were clearly without FSGS, two sites (SAF2 and SAF4) showed elevated kinship values at the shortest distance class considered (30 m). This involved methodological constraints due to high levels of inbreeding with fixed loci and only one variable microsatellite locus contributing to kinship estimation. An aberrant outlier value of Sp = 0.384 for SAF2 (Mlalazi River) far outside ranges reported by Vekemans and Hardy (2004) points at a methodological issue as it is not based on evidence of congruent polymorphism and thus remains uninformative to reflect a local structure. The identified pressures in SAF2 are sedimentation of a river mouth that is kept open to allow expansion and that in SAF4 are harvesting, trampling, and sand extraction for the same locations mentioned under Mlalazi and Mngazana by Adams and Rajkaran (2020). The South African estuarine populations SAF1 and SAF3 also showed strong inbreeding levels that are most likely due to the recurrent closing of the river mouth, lowering the chance of external propagule input as discussed by De Ryck et al. (2016) in comparison to East African Avicennia populations. The pressures in mangrove areas of SAF1 and SAF3 are related to mouth restriction such as freshwater inflow and siltation of these same locations mentioned under St. Lucia and Mngeni by Adams and Rajkaran (2020). However, these estuary types did not render a detectable FSGS, suggesting that wind action or temporal interaction of river flow and tides during open-mouth conditions may be sufficient to mix propagules before their establishment. Additionally, during closure periods with freshwater floods, there could be sufficient mixing of propagules, however associated with a die-back of A. marina (Adams and Rajkaran, 2020).
Overall, our findings provide evidence that coastal regions, characterized by different “energy signatures” (sensu Twilley, 1995), can be an important variable to consider in (mangrove) dispersal and connectivity studies that aim to explain local patterns of genetic structure. Large-scale patterns of dispersal and population connectivity in mangroves have been estimated using Lagrangian particle-tracking methods in combination with output from ocean general circulation models (e.g., Van der Stocken et al., 2019a), whereas other studies have used maps and general descriptions of large-scale ocean surface currents to explain observed mangrove population genetic structure (e.g., De Ryck et al., 2016; Wee et al., 2020). While such studies become increasingly possible due to the continuous efforts to increase the availability of oceanographic data and information products, estimating local- and regional-scale dispersal and connectivity patterns within and among mangrove areas in close proximity (a few km to tens of km) remains challenging (Chablé Iuit et al., 2020; Triest et al., 2020). In contrast to large-scale data products, high-resolution input data required for local simulations (e.g., topography, time series of water level, and discharge) are seldom readily available and often require complex and expensive field measurement campaigns. Very important pressures on mangrove areas are the estuary mouth dynamics and anthropogenic-induced habitat removal or freshwater inflow reduction causing a change in population structure and overall degradation with eventual die-back of Avicennia trees and shrubs (Adams and Rajkaran, 2020). From our study, we can put forward that the resilience of mangroves through natural regeneration is achieved by different responses for settings with different hydrodynamic conditions, which can be important information for their management and protection within the variety of coastal environments. Hence, examining the genetic structure of mangrove species in different coastal and marine landforms can be considered an important step toward a more comprehensive understanding of the influence of different coastal settings on patterns of dispersal and establishment in mangrove forests. Indeed, environmental conditions may have changed from the moment of propagule establishment and during the time of development into the mature stands that are usually being sampled in genetic studies. Highly polymorphic genetic data at the individual mangrove tree level, such as used in this study, therefore may shed light on how mangrove trees—once at the seedling stage—became established in mangrove patches through hydrochorous dispersal that may have happened over various distances ranging from the shortest distances near the parent tree neighborhood (i.e., gravity dispersed) up to greater distances within or beyond the coastal landform harboring mangrove areas.
Data Availability Statement
The datasets presented in this study can be found in online repositories. The names of the repository/repositories and accession number(s) can be found below: https://www.ncbi.nlm.nih.gov/genbank/, MT713342-MT713346, https://datadryad.org/stash, https://doi.org/10.5061/dryad.8pk0p2nkt.
Author Contributions
LT did the conceptualization, data curation, formal analysis, funding acquisition, investigation, methodology, resources, visualization, writing-original draft, and writing-review and editing. TV did the conceptualization, investigation, methodology, visualization, writing-original draft, and writing-review and editing. Both authors contributed to the article and approved the submitted version.
Funding
This work was supported by the Vrije Universiteit Brussel (VUB; grant number BAS42), the Marie-Curie International Research Staff Exchange Scheme “Coastal Research Network on Environmental Changes—CREC” (EC grant agreement no. 247514), and a mobility scholarship from the “Leopold III-fonds voor natuuronderzoek en natuurbehoud vzw.” TV is supported by the EU Horizon 2020 Framework Programme for Research and Innovation under the Marie Skłodowska-Curie actions Individual Fellowship (MSCA-IF) with grant agreement no. 896888 (GLOMAC).
Conflict of Interest
The authors declare that the research was conducted in the absence of any commercial or financial relationships that could be construed as a potential conflict of interest.
Acknowledgments
The authors sincerely thank Rosa van der Ven (VUB), Dennis De Ryck (VUB), Hajaniaina Andrianavalonarivo Ratsimbazafy (VUB), and François Fromard (CNRS), for collecting materials.
Supplementary Material
The Supplementary Material for this article can be found online at: https://www.frontiersin.org/articles/10.3389/fmars.2021.617855/full#supplementary-material
References
Adams, J. B., and Rajkaran, A. (2020). Changes in mangroves at their southernmost African distribution limit. Estuar. Coast. Shelf Sci. 247:106862. doi: 10.1016/j.ecss.2020.106862
Alleman, L. K., and Hester, M. W. (2011). Reproductive ecology of black mangrove (Avicennia germinans) along the Louisiana coast: propagule production cycles, dispersal limitations, and establishment elevations. Estuaries Coasts 34, 1068–1077. doi: 10.1007/S12237-011-9404-8
Archambault, P., and Bourget, E. (1999). Influence of shoreline configuration on spatial variation of meroplanktonic larvae, recruitment and diversity of benthic subtidal communities. J. Exp. Mar. Biol. Ecol. 238, 161–184. doi: 10.1016/S0022-0981(98)00146-4
Balbar, A. C., and Metaxas, A. (2019). The current application of ecological connectivity in the design of marine protected areas. Glob. Ecol. Conserv. 17:e00569. doi: 10.1016/j.gecco.2019.e00569
Balke, T., Bouma, T. J., Horstman, E. M., Webb, E. L., Erftemeijer, P. L. A., and Herman, P. M. J. (2011). Windows of opportunity: thresholds to mangrove seedling establishment on tidal flats. Mar. Ecol. Prog. Ser. 440, 1–9. doi: 10.3354/meps09364
Banks, S. C., Piggott, M. P., Williamson, J. E., Bové, U., Holbrook, N. L., and Beheregaray, L. B. (2007). Oceanic variability and coastal topography shape genetic structure in a long-dispersing sea urchin. Ecology 88, 3055–3064. doi: 10.1890/07-0091.1
Barbier, E. B., Hacker, S. D., Kennedy, C., Koch, E. W., Stier, A. C., and Silliman, B. R. (2011). The value of estuarine and coastal ecosystem services. Ecol. Monogr. 81, 169–193. doi: 10.1890/10-1510.1
Bryan-Brown, D. N., Connolly, R. M., Richards, D. R., Adame, F., Friess, D. A., and Brown, C. J. (2020). Global trends in mangrove forest fragmentation. Sci. Rep. 10:7117. doi: 10.1038/s41598-020-63880-1
Céron-Souza, I., Bermingham, E., McMillan, O. W., and Jones, F. A. (2012). Comparative genetic structure of two mangrove species in Caribbean and Pacific estuaries of Panama. BMC Evol. Biol. 12:205. doi: 10.1186/1471-2148-12-205
Chablé Iuit, L. R., Machkour-M’Rabet, S., Espinoza-Ávalos, J., Hernández-Arana, H. A., López-Adame, H., and Hénaut, Y. (2020). Genetic structure and connectivity of the red mangrove at different geographic scales through a complex transverse hydrological system from freshwater to marine ecosystems. Diversity 12:48. doi: 10.3390/d12020048
Clarke, P. J. (1992). Predispersal mortality and fecundity in the grey mangrove (Avicennia marina) in southeastern Australia. Aust. J. Ecol. 17, 161–168. doi: 10.1111/j.1442-9993.1992.tb00794.x
Clarke, P. J., Kerrigan, R. A., and Westphal, C. J. (2001). Dispersal patterns and early growth in 14 tropical mangroves: do early life history traits correlate with patterns of adult distribution. J. Ecol. 89, 648–659. doi: 10.1046/j.0022-0477.2001.00584.x
Clarke, P. J., and Myerscough, P. J. (1991). Buoyancy of Avicennia marina propagules in South-Eastern Australia. Aust. J. Bot. 39, 77–83. doi: 10.1071/BT9910077
Coleman, M. A., Clark, J. S., Doblin, M. A., Bishop, M. J., and Kelaher, B. P. (2018). Genetic differentiation between estuarine and open coast ecotypes of a dominant ecosystem engineer. Mar. Freshw. Res. 70, 977–985. doi: 10.1071/MF17392
Dahdouh-Guebas, F., Verneirt, M., Tack, J. F., van Speybroeck, D., and Koedam, N. (1998). Propagule predators in Kenyan mangroves and their possible effect on regeneration. Mar. Freshw. Res. 49, 345–350. doi: 10.1071/MF97108
De Ryck, D. J. R., Koedam, N., Van der Stocken, T., Van der Ven, R. M., Adams, J., and Triest, L. (2016). Dispersal limitation of the mangrove Avicennia marina at its South African range limit in strong contrast to connectivity in its core East African region. Mar. Ecol. Prog. Ser. 545, 123–134. doi: 10.3354/MEPS11581
Do, B. T. N., Koedam, N., and Triest, L. (2019). Avicennia marina maintains genetic structure whereas Rhizophora stylosa connects mangroves in a flooded, former inner sea (Vietnam). Estuar. Coast. Shelf Sci. 222, 195–204. doi: 10.1016/j.ecss.2019.04.005
Durrant, H. M. S., Barrett, N. S., Edgar, G. J., Coleman, M. A., and Burridge, C. P. (2018). Seascape habitat patchiness and hydrodynamics explain genetic structuring of kelp populations. Mar. Ecol. Prog. Ser. 587, 81–92. doi: 10.3354/meps12447
Fox, R. J., Donelson, J. M., Schunter, C., Ravasi, T., and Gaitán-Espitia, J. D. (2019). Beyond buying time: the role of plasticity in phenotypic adaptation to rapid environmental change. Philos. Trans. R. Soc. B 374:20180174. doi: 10.1098/rstb.2018.0174
Gedan, K. B., Kirwan, M. L., Wolanski, E., Barbier, E. B., and Silliman, B. R. (2011). The present and future role of coastal wetland vegetation in protecting shorelines: answering recent challenges to the paradigm. Clim. Change 106, 7–29. doi: 10.1007/s10584-010-0003-7
Geng, Q. F., Lian, C. L., Tao, M., Li, Q., and Hogetsus, T. (2007). Isolation and characterization of 10 new compound microsatellite markers for a mangrove tree species, Avicennia marina (Forsk.) Vierh. (Avicenniaceae). Mol. Ecol. Notes 7, 1208–1210. doi: 10.1111/j.1471-8286.2007.01834.x
Goudet, J. (2001). FSTAT version 2.9.3: a program to estimate and test gene diversities and fixation indices (update from version 1.2, Goudet 1995): a computer program to calculate F-statistic. J. Hered. 86, 485–486. doi: 10.1093/oxfordjournals.jhered.a111627
Graham, W. M., and Largier, J. L. (1997). Upwelling shadows as nearshore retention sites: the example of northern Monterey Bay. Cont. Shelf Res. 17, 509–532. doi: 10.1016/S0278-4343(96)00045-3
Hampe, A. (2011). Plants on the move: the role of seed dispersal and initial population establishment for climate-driven range expansions. Acta Oecol. 37, 666–673. doi: 10.1016/j.actao.2011.05.001
Hardy, O. J., and Vekemans, X. (2002). SPAGeDi: a versatile computer program to analyse spatial genetic structure at the individual or population levels. Mol. Ecol. Notes 2, 618–620. doi: 10.1046/j.1471-8286.2002.00305.x
Hasan, S., Triest, L., Afrose, S., and De Ryck, D. (2018). Migrant pool model of dispersal explains strong connectivity of Avicennia officinalis within Sundarban mangrove areas: effect of fragmentation and replantation. Estuar. Coast. Shelf Sci. 214, 38–47. doi: 10.1016/j.ecss.2018.09.007
Hermansen, T. D., Britton, D. R., Ayre, D. J., and Minchonton, T. E. (2014). Identifying the real pollinators? Exotic honeybees are the dominant flower visitors and only effective pollinators of Avicennia marina in Australian temperate mangroves. Estuaries Coasts 37, 621–635. doi: 10.1007/s12237-013-9711-3
Howard, J., Sutton-Grier, A., Herr, D., Kleypas, J., Landis, E., McLeod, E., et al. (2017). Clarifying the role of coastal and marine systems in climate mitigation. Front. Ecol. Environ. 15, 42–50. doi: 10.1002/fee.1451
Johansson, M. L., Banks, M. A., Glunt, K. D., Hassel-Finnegan, H. M., and Buonaccorsi, V. P. (2008). Influence of habitat discontinuity, geographical distance, and oceanography on fine−scale population genetic structure of copper rockfish (Sebastes caurinus). Mol. Ecol. 17, 3051–3061. doi: 10.1111/j.1365-294X.2008.03814.x
Lefcheck, J. S., Hughes, B. B., Johnson, A. J., Pfirrmann, B. W., Rasher, D. B., Smyth, A. R., et al. (2019). Are coastal habitats important nurseries? A meta-analysis. Conserv. Lett. 12:e12645. doi: 10.1111/conl.12645
Loiselle, B., Sork, V. L., Nason, J., and Graham, C. (1995). Spatial genetic structure of a tropical understory shrub, Psychotria officinalis (Rubiaceae). Am. J. Bot. 82, 1420–1425. doi: 10.1002/j.1537-2197.1995.tb12679.x
Maguire, T. L., Edwards, K. J., Saenger, P., and Henry, R. (2000). Characterisation and analysis of microsatellite loci in a mangrove species, Avicennia marina (Forsk.) Vierh. (Avicenniaceae). Theor. Appl. Genet. 101, 279–285. doi: 10.1007/s001220051480
McKee, K. L. (1995). Seedling recruitment patterns in a Belizean mangrove forest: effects of establishment ability and physico-chemical factors. Oecologia 101, 448–460. doi: 10.1007/BF00329423
Millán-Aquilar, O., Manzano-Sarabia, M., Nettel-Hernanz, A., Dodd, R. S., Hurtado-Oliva, M. A., and Velázquez-Velázquez, E. (2016). Genetic diversity of the black mangrove Avicennia germinans (L.) stearn in Northwestern Mexico. Forests 7:197. doi: 10.3390/f7090197
Mori, G. M., Zucchi, M. I., and Souza, A. P. (2015). Multiple-geographic-scale genetic structure of two mangrove tree species: the roles of mating system, hybridization, limited dispersal and extrinsic factors. PLoS One 10:e0118710. doi: 10.1371/journal.pone.0118710
Ngeve, M., Van der Stocken, T., Menemenlis, D., Koedam, N., and Triest, L. (2017). Hidden founders? Strong bottlenecks and fine-scale genetic structure in mangrove populations of the Cameroon Estuary complex. Hydrobiologia 803, 189–207. doi: 10.1007/s10750-017-3369-y
Nicastro, K. R., Zardi, G. I., McQuaid, C. D., Teske, P. R., and Barker, N. P. (2008). Coastal topography drives genetic structure in marine mussels. Mar. Ecol. Prog. Ser. 368, 189–195. doi: 10.3354/meps07607
Peakall, R., and Smouse, P. E. (2012). GenAlEx 6.5: genetic analysis in Excel. Population genetic software for teaching and research – an update. Bioinformatics 28, 2537–2539. doi: 10.1111/j.1471-8286.2005.01155.x
Rabinowitz, D. (1978). Dispersal properties of mangrove propagules. Biotropica 10, 47–57. doi: 10.2307/2388105
Reed, T. E., Schindler, D. E., and Waples, R. S. (2011). Interacting effects of phenotypic plasticity and evolution on population persistence in a changing climate. Conserv. Biol. 25, 56–63. doi: 10.1111/j.1523-1739.2010.01552.x
Richards, D. R., and Friess, D. A. (2016). Rates and drivers of mangrove deforestation on Southeast Asia, 2000–2012. Proc. Natl. Acad. Sci. U.S.A. 113, 344–349. doi: 10.1073/pnas.1510272113
Roughan, M., Mace, A. J., Largier, J. L., Morgan, S. G., Fisher, J. L., and Carter, M. L. (2005). Subsurface recirculation and larval retention in the lee of a small headland: a variation on the upwelling shadow theme. J. Geophys. Res. 110:C10027. doi: 10.1029/2005JC002898
Shanks, A. L., McCulloch, A., and Miller, J. (2003). Topographically generated fronts, very nearshore oceanography and the distribution of larval invertebrates and holoplankters. J. Plankton Res. 25, 1251–1277. doi: 10.1093/plankt/fbg090
Siegel, D. A., Mitarai, S., Costello, C. J., Gaines, S. D., Kendall, B. E., Warner, R. R., et al. (2008). The stochastic nature of larval connectivity among nearshore marine populations. Proc. Natl. Acad. Sci. U.S.A. 105, 8974–8979. doi: 10.1073/pnas.0802544105
Smith, T. J. III (1987). Seed predation in relation to tree dominance and distribution in mangrove forests. Ecology 68, 266–273. doi: 10.2307/1939257
Steinke, T. D. (1986). A preliminary study of buoyancy behavior in Avicennia marina propagules. S. Afr. J. Bot. 52, 559–565. doi: 10.1016/S0254-6299(16)31492-2
Stieglitz, T., and Ridd, P. V. (2001). Trapping of mangrove propagules due to density-driven secondary circulation in the Normanby River estuary, NE Australia. Mar. Ecol. Prog. Ser. 211, 131–142. doi: 10.3354/meps211131
Teixeira, S., Arnaud-Haond, S., Duarte, C. M., and Serrao, E. (2003). Polymorphic microsatellite DNA markers in the mangrove tree Avicennia alba. Mol. Ecol. Notes 3, 544–546. doi: 10.1046/j.1471-8286.2003.00505.x
Temmerman, S., Meire, P., Bouma, T. J., Herman, P. M. J., Ysebaert, T., and De Vriend, H. J. (2013). Ecosystem-based coastal defence in the face of global change. Nature 504, 79–83. doi: 10.1038/nature12859
Teske, P. R., Sandoval-Castillo, J., van Sebille, E., Waters, J., and Beheregaray, L. B. (2015). On-shelf larval retention in a coastal broadcast spawner. Mar. Ecol. Prog. Ser. 532, 1–12. doi: 10.3354/meps11362
Thom, B. G. (1982). “Mangrove ecology a geomorphological perspective,” in Mangrove Ecosystems in Australia, ed. B. F. Clough (Canberra, ACT: Australian National University Press), 3–17.
Thomas, N., Lucas, R., Bunting, P., Hardy, A., Rosenqvist, A., and Simard, M. (2017). Distribution and drivers of global mangrove forest change, 1996-2010. PLoS One 12:e0179302. doi: 10.1371/journal.pone.0179302
Triest, L., Van der Stocken, T., Akinyi, A. A., Sierens, T., Kairo, J., and Koedam, N. (2020). Channel network structure determines genetic connectivity of landward–seaward Avicennia marina populations in a tropical bay. Ecol. Evol. 10, 12059–12075. doi: 10.1002/ece3.6829
Twilley, R. R. (1995). “Properties of mangrove ecosystems related to the energy signature of coastal environments,” in Maximum Power: The Ideas and Applications of H.T. Odum, ed. C. A. S. Haii (Boulder, CO: University of Colorado Press), 43–62.
Urban, M. C., Richardson, J. L., and Freidenfelds, N. A. (2014). Plasticity and genetic adaptation mediate amphibian and reptile responses to climate change. Evol. Appl. 7, 88–103. doi: 10.1111/eva.12114
Van der Stocken, T., Carroll, D., Menemenlis, D., Simard, M., and Koedam, N. (2019a). Global-scale dispersal and connectivity in mangroves. Proc. Natl. Acad. Sci. U.S.A. 116, 915–922. doi: 10.1073/pnas.1812470116
Van der Stocken, T., Vanschoenwinkel, B., De Ryck, D. J. R., Bouma, T. J., Dahdouh-Guebas, F., and Koedam, N. (2015). Interaction between water and wind as a driver of passive dispersal in mangroves. PLoS One 10:e0121593. doi: 10.1371/journal.pone.0121593
Van der Stocken, T., Wee, A. K. S., De Ryck, D. J. R., Vanschoenwinkel, B., Friess, D. A., Dahdouh-Guebas, F., et al. (2019b). A general framework for propagule dispersal in mangroves. Biol. Rev. 94, 1547–1575. doi: 10.1111/brv.12514
van Oosterhout, C., Hutchinson, W. F., Wills, D. P. M., and Shipley, P. (2004). MICRO-CHECKER: software for identifying and correcting genotyping errors in microsatellite data. Mol. Ecol. Notes 4, 535–538. doi: 10.1111/j.1471-8286.2004.00684.x
Vekemans, X., and Hardy, O. J. (2004). New insights from fine-scale spatial genetic structure analyses in plant populations. Mol. Ecol. 13, 921–935. doi: 10.1046/j.1365-294X.2004.02076.x
Wee, A. K. S., Low, S. Y., and Webb, E. L. (2015). Pollen limitation affects reproductive outcome in the bird-pollinated mangrove Bruguiera gymnorrhiza (Lam.) in a highly urbanized environment. Aquat. Bot. 120, 240–243. doi: 10.1016/j.aquabot.2014.09.001
Wee, A. K. S., Noreen, A. M. E., Ono, J., Takayama, K., Kumar, P. P., Tan, H. T. W., et al. (2020). Genetic structure across a biogeographical barrier reflect dispersal potential of four Southeast Asian mangrove plant species. J. Biogr. 47, 1258–1271. doi: 10.1111/jbi.13813
White, C., Selkoe, K. A., Watson, J., Siegel, D. A., Zacherl, D. C., and Toonen, R. J. (2010). Ocean currents help explain population genetic structure. Proc. R. Soc. B 277, 1685–1694. doi: 10.1098/rspb.2009.2214
Keywords: Avicennia, coastal wetland, bay, estuary, fine-scale genetic structure, microsatellites
Citation: Triest L and Van der Stocken T (2021) Coastal Landform Constrains Dispersal in Mangroves. Front. Mar. Sci. 8:617855. doi: 10.3389/fmars.2021.617855
Received: 15 October 2020; Accepted: 24 May 2021;
Published: 28 June 2021.
Edited by:
Christos Dimitrios Arvanitidis, Hellenic Centre for Marine Research (HCMR), GreeceReviewed by:
Stefano Cannicci, The University of Hong Kong, Hong Kong, SAR ChinaPanagiotis Kasapidis, Hellenic Centre for Marine Research (HCMR), Greece
Copyright © 2021 Triest and Van der Stocken. This is an open-access article distributed under the terms of the Creative Commons Attribution License (CC BY). The use, distribution or reproduction in other forums is permitted, provided the original author(s) and the copyright owner(s) are credited and that the original publication in this journal is cited, in accordance with accepted academic practice. No use, distribution or reproduction is permitted which does not comply with these terms.
*Correspondence: Ludwig Triest, ltriest@vub.be