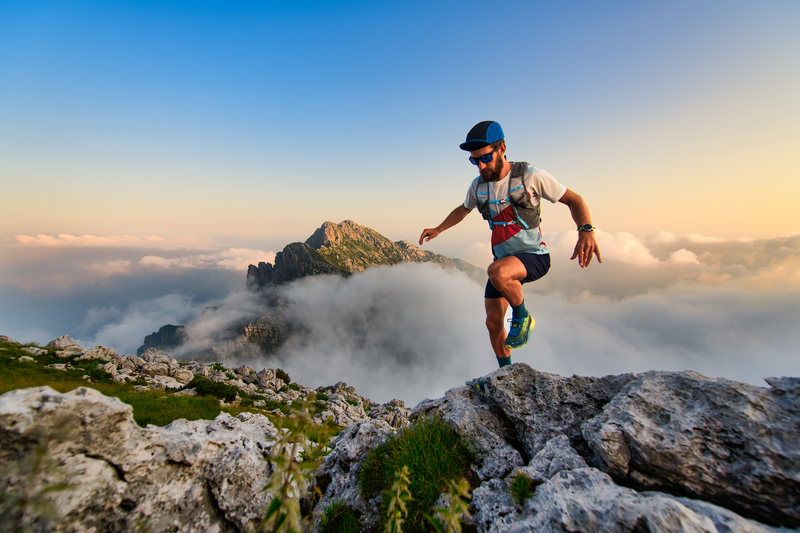
94% of researchers rate our articles as excellent or good
Learn more about the work of our research integrity team to safeguard the quality of each article we publish.
Find out more
ORIGINAL RESEARCH article
Front. Mar. Sci. , 23 July 2021
Sec. Marine Ecosystem Ecology
Volume 8 - 2021 | https://doi.org/10.3389/fmars.2021.611981
This article is part of the Research Topic Oceanography and Benthic Ecology of Patagonian fjords - 500 years from the Discovery of the Strait Magellan View all 16 articles
Fjords are sensitive areas affected by climate change and can act as a natural laboratory to study microbial ecological processes. The Chilean Patagonian fjords (41–56°S), belonging to the Subantarctic ecosystem (46–60°S), make up one of the world’s largest fjord systems. In this region, Estuarine Water (EW) strongly influences oceanographic conditions, generating sharp gradients of oxygen, salinity and nutrients, the effects of which on the microbial community structure are poorly understood. During the spring of 2017 we studied the ecological patterns (dispersal and oceanographic factors) underlying the microbial community distribution in a linear span of 450 km along the estuarine-influenced Chilean Patagonian fjords. Our results show that widespread microbial dispersion existed along the fjords where bacterioplankton exhibited dependence on the eukaryotic phytoplankton community composition. This dependence was particularly observed under the low chlorophyll-a conditions of the Baker Channel area, in which a significant relationship was revealed between SAR11 Clade III and the eukaryotic families Pyrenomonadaceae (Cryptophyte) and Coccomyxaceae (Chlorophyta). Furthermore, dissolved oxygen and salinity were revealed as the main drivers influencing the surface marine microbial communities in these fjords. A strong salinity gradient resulted in the segregation of the Baker Channel prokaryotic communities from the rest of the Patagonian fjords. Likewise, Microbacteriaceae, Burkholderiaceae and SAR11 Clade III, commonly found in freshwater, were strongly associated with EW conditions in these fjords. The direct effect of EW on the microbial community structure and diversity of the fjords exemplifies the significance that climate change and, in particular, deglaciation have on this marine region and its productivity.
One of the world’s largest fjord systems is located in the Patagonian region of southern Chile (41–56°S) (Iriarte et al., 2010). This fragmented coastal region, which possesses several islands, rivers and channels (Iriarte et al., 2014; Cuevas et al., 2019), is characterized by high primary production due to the occurrence of phytoplankton blooms throughout the year (Iriarte et al., 2016; Montero et al., 2017). During the austral summer in the Patagonian fjords, high primary production results in carbon sequestration (∼5 mol C m–2 year–1) that is considered high relative to Patagonia’s Atlantic coast (∼1.6 mol C m–2 year–1) (Torres et al., 2011). Rivers and rainfall runoff, together with glacier melting and vertical mixing, explain this high primary production due to nutrient input (Iriarte et al., 2010; Montero et al., 2017; Cuevas et al., 2019). The Patagonian fjord system is also characterized by sharp vertical and horizontal oxygen and salinity gradients (Iriarte et al., 2014; Cuevas et al., 2019), which are produced by advective transport and mixing of Estuarine Water (EW) with Sub-Antarctic Water (SAAW) (Silva and Vargas, 2014; Vargas et al., 2018). This mixing of EW and SAAW will also likely result in the mixing of entire microbial communities and nutrients in a process termed “community coalescence” (Rillig et al., 2015).
The Patagonian fjord region is considered a vulnerable ecosystem that is highly sensitive to climatic change and anthropogenic activity (Iriarte et al., 2010; Iriarte, 2018; González et al., 2019). Climate forcing by the El Niño-Southern Oscillation and the Southern Annular Mode has been linked to inter-annual variations in environmental conditions, such as higher sea surface temperature, changes in wind direction and intensity, and decreased freshwater discharge to the marine system due to drought (Garreaud, 2018; León-Muñoz et al., 2018). These regional variations can affect the marine microbial community, modifying primary production, and they have even been proposed as possible triggers of harmful algal blooms in the area (Iriarte, 2018; Moreno-Pino et al., 2018). For example, a harmful algal bloom of Pseudochattonella cf. verruculosa observed in the summer of 2016 was the largest reported to date in this area (León-Muñoz et al., 2018). Additionally, activities and processes, such as aquaculture, freshwater runoff and increased glacier melt (Iriarte, 2018; González et al., 2019), have had an impact on the carbon and nitrogen nutrient input to these coastal areas (Silva and Vargas, 2014; Vargas et al., 2018), subsequently affecting microbial activity (Olsen et al., 2017; González et al., 2019). However, the overall effect of these processes on the local microbial ecology is still unknown.
Most efforts to study microbial communities in the Patagonian fjords have focused on the northern region (41–45°S) (Gutiérrez et al., 2015, 2018; Iriarte et al., 2016; Montero et al., 2017), Baker Channel (48°S) (Gutiérrez et al., 2015) and Cape Horn area (52–56°S) (Moreno-Pino et al., 2018), while microbial communities in the central area (47–52°S) remain underexplored. Analyses of the microbial ecology in this area have shown high heterogeneity of the phytoplankton community, dominated during spring blooms by the diatom genera Skeletonema, Chaetoceros, Rhizosolenia, and Thalassiosira (Iriarte et al., 2016; Montero et al., 2017). Additionally, oxygen and salinity were recently suggested as drivers of vertical water stratification that affects the bacterial community distribution of the Puyuhuapi fjord in northern Patagonia (44°S) (Gutiérrez et al., 2018). To date, no broader study has focused on the structure and diversity patterns of bacterioplankton across the Patagonian fjord region.
Here we investigate the influence of EW on the microbial community structure and diversity in the central Patagonian fjord system (47–52°S) over a linear span of 450 km. This survey is necessary to identify the general patterns of microbial communities and specific microorganisms that can be used as possible biological indicators of change in the water conditions of this southern ecosystem, which is susceptible to climatic change and anthropogenic activity. The primary objectives of our analyses were to (i) characterize the environmental conditions, such as temperature, salinity, oxygen and chlorophyll-a, in the Estuarine Waters of the central Patagonian fjords; (ii) assess the effect of the environmental conditions and geographic distance on the community structures of both bacterioplankton (bacteria and archaea) and eukaryotic phytoplankton; and finally, (iii) determine the influence of environmental drivers on relevant taxa in the region.
The central Patagonian fjord region sampled in this study stretched 450 km, from the Penas Gulf (47°S) to Nelson Strait (52°S), as shown in Figure 1. The extent to which areas in this region are influenced by Estuarine and Sub-Antarctic Waters (or modified SAAW) differs. The study transect comprised 23 stations from the Penas Gulf (47.7°S, station 2) to Nelson Strait (51.4°S, station 48) and was divided into ten geographic areas according to the information provided by the Chilean Hydrographic and Oceanographic Service of the Navy. From north to south, the sampling stations were as follows: Penas Gulf (station 2); Baker Channel (stations 10, 8, 7, and 5, oriented in an E–W direction); Bernardo (stations 18C and 18A), Iceberg (station 21B), Falcon (stations 30 and 25) and Penguin (station 33) fjords; Messier Channel (stations 19, 24, 35, 43, and 44); Europa (stations 39 and 36) and Peel (stations 72, 75, and 70) fjords; and Nelson Strait (stations 68 and 48) (Figure 1). Sampling was performed onboard the scientific research vessel “Cabo de Hornos” (AGS61), operated by the Chilean navy’s Hydrographic and Oceanographic Service as part of the program “Cruceros de Investigación Marina en Areas Remotas” (CIMAR). Specifically, this CIMAR23f campaign was conducted from October 17 to November 20, 2017.
Figure 1. Geographic location and study area of the CIMAR23f campaign. (A) Chilean Patagonian fjords as part of the Subantarctic region (46–60°S). Red denotes the Chilean political division within South America. The black dashed square delimits the Patagonian fjord study area. (B) Study area (47.5–52°S) and sampling station locations. Colored circles and numbers represent the location and identification number, respectively, of each station. South Pacific Ocean (SPO), South Atlantic Ocean (SAO).
Surface water at the 23 stations was sampled at two depths (5 and 10 m) using a Niskin bottle-rosette system (46 samples in total). Water samples were analyzed for dissolved oxygen (DO), chlorophyll-a (Chl-a) and used for microbial biomass concentration. Concurrent with water sample collection, temperature and conductivity profiles for the whole column water were obtained using a Seabird 19 CTD. For Chl-a, 1 L water samples were pre-filtered through 200-μm nylon mesh, filtered through 0.22-μm cellulose membrane filters and then stored at −20°C until analysis. The Chl-a concentration (mg m–3) was subsequently determined fluorometrically using the method described by Parsons et al. (1984). DO (O2) concentrations (μM) were determined by the modified Winkler method proposed by Strickland and Parsons (1972). For microbial community analysis, seawater (10–15 L) was subjected to serial prefiltration through 200-μm nylon mesh followed by 20-μm polycarbonate filters using a Cole Parmer peristaltic pump system (Model 7553–70, 6 – 600 rpm) at 50 – 100 mL min–1 for an average of 1 h. Microbial biomass in the filtrate was then concentrated onto 0.22-μm pore size Sterivex units (Durapore; Millipore) and stored at −20°C until analysis.
DNA was extracted according to a modified protocol described in Tillett and Neilan (2000). Briefly, the Sterivex membrane filters were manually removed from the housing before DNA extraction. Next, the biomass on the Sterivex filters was resuspended in xanthogenate buffer [1% potassium ethyl xanthogenate (Sigma-Aldrich, United States), 100 mM Tris-HCl (pH 7.4), 20 mM EDTA (pH 8), 800 mM ammonium acetate] with 1% SDS. The mixture was incubated at 65°C for 2 h (hand-shaken every 30 min). After incubation, the tubes were placed on ice for 30 min. The DNA was then extracted with phenol-chloroform-isoamyl alcohol (25:24:1), and the residual phenol was eliminated with chloroform-isoamyl alcohol (24:1). The extract was cleaned by overnight precipitation with cold isopropanol (−20°C) and subsequently washed with 70% ethanol. DNA was quantified using a Qubit 2.0 Fluorometer (Thermo Fisher Scientific, United States). The quality was assessed by spectrophotometry (A260/A280 ratio) and the integrity was verified by standard 1% agarose gel electrophoresis.
The V4–V5 hypervariable region of the 16S rRNA gene was amplified using the primers 515F (5′-GTGYCAGCMG CCGCGGTAA-3′) and 926R (5′-CCGYCAATTYMTTTRAGT TT-3′), according to Parada et al. (2016). Sequencing was conducted on the Illumina MiSeq platform at the Argonne National Laboratory (Lemont, IL, United States). Raw sequences of the 16S rRNA gene were demultiplexed using the q2-demux plugin implemented in the QIIME2 pipeline (Bokulich et al., 2018; Bolyen et al., 2019). The paired-end sequences were trimmed and merged using DADA2 (Callahan et al., 2016) to obtain amplicon sequencing variants (ASVs). The taxonomy of the 16S rRNA ASVs was assigned using the q2-feature-classifier against the SILVA132 database (Quast et al., 2013) via the “classify consensus vsearch” method (Rognes et al., 2016). ASVs that were taxonomically annotated as “Mitochondria” and “Chloroplast” were excluded from the subsequent bacterial and archaeal community analysis. To obtain the taxonomic identity of eukaryotic phytoplankton (phytoplankton community), ASVs that were annotated as “Chloroplast” were separated and re-annotated using the same methodology applied to the aforementioned ASVs but against the PhytoRef database (Decelle et al., 2015), as described in Fuentes et al. (2019). Finally, to remove singletons and rare taxa, ASVs were filtered by total count sum (>4) and prevalence in at least 10% of the samples (Supplementary Table 1). All 16S rRNA sequences used in this study have been deposited in the NCBI Sequence Read Archive (SRA) under BioProject ID PRJNA670217.
Amplicon sequencing variants analyses were conducted using R packages (R Core Team, 2020), including phyloseq (McMurdie and Holmes, 2013) and ampvis2 (Andersen et al., 2018). Graphical support for the microbial community was conducted with ggplot2 (Wickham, 2016), dendextend (Galili, 2015) and ggmap (Kahle and Wickham, 2013). The Ocean Data View (ODV) software was used for oceanographic variables (Schlitzer, 2020). Maps of the oceanographic conditions by depth were made with a spatial resolution of 1 km (GEBCO 2015 grid) and implemented with coastlines and fill coastlines layers. Diversity analyses were performed with the stats (R Core Team, 2020) and vegan (Oksanen et al., 2019) packages in R. Alpha diversity was measured as the number of distinct ASVs or taxonomic ranks in each sample (observed richness). The Shannon and Pielou indexes were applied exclusively to clean ASVs. For clustering and ordinate analysis, the double-zero effect and rare taxa weight were standardized by Hellinger transformation in ASV filter count data. Hellinger-transformed counts were then used to calculate Euclidean distances among samples (Hellinger distance matrix) (Legendre and Gallagher, 2001). Oceanographic variables were standardized using the z-score method (mean 0, variance 1). Standardized variables were then used to calculate Euclidean distances among the samples. The Ward2 algorithm (Murtagh and Legendre, 2014), implemented in the vegan package, was used to construct the final dendrogram. Silhouette validation criteria, combined with calculated p-values for hierarchical clustering (multiscale bootstrap) implemented in the pvclust package (Suzuki et al., 2019), were used to select the number of clusters (k) in each dataset (Rousseeuw, 1987). Exploratory analysis of the main factors affecting the microbial community structure (i.e., prokaryotic plankton and eukaryotic phytoplankton) was conducted via principal component analysis (PCA) using the ‘envfit’ test function in the vegan package.
Briefly, microbial network analyses were conducted in R using spieceasi (Kurtz et al., 2015) and plotted with ggnet (Briatte, 2020). The best taxonomic hit grouped the clean prokaryotic and eukaryotic ASVs. For network reconstruction and interaction estimation, the neighborhood selection method (Meinshausen and Bühlmann method) (Meinshausen and Bühlmann, 2006) was applied with an nlambda of 20. The network was processed in igraph (Csardi and Nepusz, 2006) for further attribute analyses (i.e., degree and betweenness). Regression coefficient values calculated by the SPIEC-EASI method were used to estimate the positive or negative correlation of edges.
The relation between environmental variables (i.e., temperature, DO, salinity, and Chl-a) and ecological indexes (i.e., Shannon and Pielou) were studied via permutational analysis of variance (PERMANOVA) (Anderson, 2001) using the adonis2 function in the R vegan package (9,999 permutations). Classification of fjord areas was included as the explanatory factor for environmental (df = 9, n = 46) and ecological (df = 9, n = 45) indexes. Additionally, Spearman’s rank correlation (rho) was performed among environmental variables (p < 0.01) (Kendall, 1948). To study the relationship among the environmental and ecological dissimilarity matrices, a Mantel test with a Spearman’s rank correlation (rho) was performed in R. Mantel tests (9,999 permutations), facilitated by the mantel function in the vegan package, were performed between the Hellinger distance matrix (i.e., prokaryotic plankton and eukaryotic phytoplankton), Euclidean distance matrix (independent and joined variables) or the matrix of the Haversine distance between geographical coordinates (i.e., latitude and longitude) among samples (Mantel, 1967; Legendre and Fortin, 2010). Adjusted p-values were obtained using the stats package and Benjamini–Hochberg (BH) post hoc tests (Benjamini and Hochberg, 1995). Distance decay analysis was performed as a simple linear regression analysis of the Jaccard similarity index log between samples versus Haversine distance between sites. Statistical analyses were performed using R software with the stats, vegan, hmisc (Harrell, 2020) and corrplot (Wei and Simko, 2017) packages.
To explore spatial autocorrelation in the collected data, a Mantel correlogram analysis was performed with the vegan package function “mantel.correlog,” using the stations’ latitude and longitude values (XY option), and 9,999 permutations (Supplementary Figure 1). The spatial autocorrelation observed for the oceanographic conditions between neighboring samples was then considered using two approaches, as suggested by Dale and Fortin (2002), and applied to all statistical analyses in this study. First, the p-values were adjusted to a more restrictive type I error (i.e., p < 0.01) for non-permutational analyses [i.e., Spearman’s rank correlation (rho), linear regression and PCA envfit]. Second, when possible, analyses that allow randomization or permutation methods were applied (i.e., PERMANOVA, Mantel test and bootstrap).
Oceanographic conditions in the Patagonian fjord region showed spatial autocorrelation (Supplementary Figure 1) and an evident freshwater influence along the study area, as illustrated by salinity, temperature and DO profiles (Supplementary Figure 2). The vertical distribution of salinity evidenced a two-layer structure with a remarkable halocline within the first meters (∼0–30 m), especially at sites directly influenced by freshwater (i.e., stations 10, 18A, 21B, 30, 39, and 72). A similar pattern was observed for the DO and temperature profiles (Supplementary Figure 2), with a clear oxycline and thermocline in the first meters (∼0–30 m) of the water column. In addition, temperature profiles displayed thermal inversions at some stations (Supplementary Figure 2).
At 5 and 10 m depth, where microbial communities were studied, temperature showed an overall similar pattern, with more considerable differences between depths at the Falcon, Iceberg and Penguin fjords (Figure 2A). Along the study area, mean temperature values ranged between 7.05°C (Falcon Fjord) and 10.86°C (Iceberg Fjord) (Table 1), with significant differences among defined areas (PERMANOVA R2 = 0.97; p = 0.0398). Salinity values at 5 and 10 m were similar (Figure 2B), except for the Baker Channel area (Figure 2B). The study sites did not show significant differences in salinity (PERMANOVA R2 = −10.24; p = 0.9751), with mean values normally below 28 (Table 1). Only stations influenced by oceanic water, specifically those located in the Messier Channel and Penas Gulf areas (Figure 1), showed salinity values above 28 (Figure 2B and Table 1).
Figure 2. Oceanographic conditions of the Chilean Subantarctic Patagonian fjord region. Study area and station locations during the CIMAR23f campaign from October 17 to November 20, 2017 (austral spring). (A) Temperature, (B) Salinity, (C) Dissolved oxygen, and (D) Chlorophyll-a.
The mean DO concentrations in this study ranged between 281.74 and 402.67 μM (Table 1), with non-significant differences between areas (PERMANOVA R2 = 0.80; p = 0.3567). DO concentrations and saturation percentages demonstrated a well-oxygenated surface layer, with higher DO values at 5 m, especially in the Falcon and Penguin fjords (Figure 2C and Table 1), rather than at 10 m depth where several areas (Baker Channel, Bernardo, Iceberg and Falcon fjords) showed undersaturated mean values (oxygen saturation <100%, Supplementary Table 2). Chl-a values over 3 mg m–3 were detected at both 5 and 10 m in the Messier Channel (stations 35, 43, and 44) and Peel Fjord (stations 70 and 72) areas (Figure 2D). Meanwhile, the lowest Chl-a concentrations (<1 mg m–3) were observed in the Penas Gulf, Baker Channel and Nelson Strait areas (Figure 2D). Although mean values ranged from 0.12 to 9.60 mg m–3, non-significant differences between areas were detected for Chl-a (PERMANOVA R2 = −1.27; p = 0.8678) (Table 1).
Among the environmental variables (temperature, salinity and DO), non-significant (p > 0.01) correlations were detected. However, Spearman’s correlation analysis showed significant associations between depth and these variables (i.e., temperature, salinity and DO). For instance, depth was negatively correlated with DO (R = −0.463; p < 0.01) and temperature (R = −0.364; p < 0.05), but positively correlated with salinity (R = 0.477; p < 0.001) (Supplementary Figure 3). Temperature, salinity and DO conditions in the region were used to identify the presence of estuarine freshwater (EFW; S < 11), estuarine brackish water (EBW; S = 11–21) and estuarine saltwater (ESW; S = 21–31) in the first ∼24 m, followed by modified Subantarctic water (MSAAW; S = 31–33) between ∼25–69 m and Subantarctic water (SAAW; S > 33) below 70 m (Supplementary Figure 2).
Alpha diversity analyses were performed to determine the microbial community diversity of species within each fjord. The bacterioplankton communities and eukaryotic phytoplanktonic (hereafter referred to as phytoplankton) communities showed similar alpha diversity patterns among the study areas, with the highest observed richness values observed in the Messier Channel, Peel Fjord and Baker Channel, and the lowest observed richness values in the Penas Gulf (Supplementary Table 3). PERMANOVA, which was applied to investigate the difference between fjord areas, showed a significant difference in the diversity (Shannon index) and evenness (Pielou index) of ASVs from the bacterioplankton communities (PERMANOVA: Shannon index R2 = 0.56, p = 0.0003; Pielou index R2 = 0.40, p = 0.0252). Conversely, a non-significant difference was found for phytoplankton using the same indexes (PERMANOVA: Shannon index R2 = 0.36, p = 0.0617; Pielou index R2 = 0.29, p = 0.1756).
To determine the variation in species composition (i.e., beta diversity) among samples in the Patagonian fjords and the effect of oceanographic variables, a PCA plus envfit analysis was performed. The PCA results show that the first three axes (i.e., PC1, PC2, and PC3) explained 73.8 and 46.6% of the variance for bacterioplankton and phytoplankton, respectively (Figure 3). DO was identified as the main factor inducing variation in the diversity of both communities in the Patagonian fjords (Figure 3). In the Europa Fjord, bacteria and phytoplankton responded differentially to DO concentrations. Additionally, lower salinity values showed an influence on Baker Channel bacterioplankton (Figure 3A). Accordingly, salinity seemed to induce separation of the bacterioplankton assemblages from the Baker Channel and Penas Gulf (Figure 3A). This effect was more pronounced for the bacterioplankton communities located in the Baker Channel at 5 m depth, where the mean salinity value was 15 (Table 1).
Figure 3. Principal component analysis (PCA) of the 45 samples analyzed for bacterioplankton (A) and phytoplankton (B) communities. The left side of panels (A,B) shows PC1 and PC2, while the right side shows PC1 and PC3. Samples were collected from 5 m (triangles) and 10 m (circles) depth. Only oceanographic variables with values of significance (p < 0.01) via envfit were plotted (black arrow and red labels). The relative contribution (eigenvalue) of each axis to the total inertia in the data is indicated as a percentage on the axis titles.
Beta diversity-based cluster analyses of samples were performed to determine regional diversity (Figure 4 and Supplementary Figure 4). The results reveal two separate clusters of bacterioplankton (B1 and B2) and four clusters of phytoplankton (P1–P4) (Figure 4A). A geographical division of the Patagonian fjords is reflected in the cluster distribution of both communities (Figure 4A). The more northern stations, represented by samples from Penas Gulf (47°S) and Baker Channel (48°S), grouped with samples from south of Messier Channel (51°S) (clusters B2 and P4). The central area from Bernardo Fjord to Falcon Fjord (48.8–49.5°S), instead grouped exclusively as part of clusters B1 and P1. Finally, stations in the southern fjord region (50–52°S; Figure 1) were heterogeneously represented by a high diversity of groups dispersed within the six different clusters.
Figure 4. Cluster analysis of the Patagonian fjord microbial community distribution. (A) Geographical distribution of bacterioplankton clusters (colored areas labeled as B1 and B2) and phytoplankton clusters (colored areas labeled as P1 to P4) throughout the Chilean Patagonian fjords. Baker Channel (BK), Bernardo Fjord (BF), Messier Channel (MC), Iceberg Fjord (IF), Falcon Fjord (FF), Penguin Fjord (PF), Europa Fjord (EF), Peel Fjord (PlF), Nelson Strait (NS). Última Esperanza Sound is also shown due to its substantial fluvial contribution to the region. (B) Beta diversity correlation of communities. Mantel test with Spearman’s correlation (rho) of the Hellinger distance matrix between the microbial communities. (C) Microbial community distance decay was calculated as the linear regression of the log Jaccard similarity index and the linear distance among samples. Bacterioplankton communities (dark blue). Phytoplankton communities (red).
The effect of oceanographic variables on beta diversity was then assessed using a Mantel test with Spearman’s correlation (rho). The results confirm that the environment modulates microbial community clusters differentially. For instance, the bacterioplankton community structure of cluster B2 correlated much stronger with salinity and DO (R = 0.5875, p = 0.0018) than that of cluster B1 (R = 0.243, p = 0.0283) (Table 2). Meanwhile, phytoplankton of cluster P1 showed a weak but significant correlation with temperature (R = 0.3205, p = 0.0093), and those of cluster P4 showed a moderate correlation with salinity (R = 0.4729, p = 0.0283) (Table 2). Finally, geographical distance (i.e., Haversine distance) between samples, as well as the correlation of diversity between communities, were studied. Geographic distance showed a weak correlation for both communities (bacterioplankton R = 0. 2398, p = 0.0024; phytoplankton R = 0.2265, p = 0.0018), thus their dissimilarity weakly correlated with the distance between samples. However, a high correlation was observed between the bacterioplankton and phytoplankton communities (R = 0.687, p = 0.0018) (Supplementary Table 4 and Figure 4B).
To identify the microbial taxonomic composition, and the impact of environmental variables on specific taxa, we focused on identifying the microbial core taxa based on the decreasing prevalence of taxa among samples (Supplementary Table 5). The results showed that no bacterioplankton or phytoplankton ASVs were shared by all samples (n = 45), signifying a highly variable microbial community in the study area. However, when analyzing bacterioplankton at the species level, as well as phytoplankton at the phylum level, it was possible to detect core taxa (Supplementary Table 5). Fifteen bacterioplankton families (9 orders), dominated by Flavobacteriaceae (Flavobacteriales), Rhodobacteraceae (Rhodobacterales) and Pelagibacteraceae SAR11 Clade I (SAR11 clades) (Supplementary Table 6), represented the core taxa and the most abundant bacterioplankton taxa in these Patagonia fjords. In contrast, although the phytoplankton composition was dominated by the families Thalassiosiraceae, Skeletonemataceae, and Bathycoccaceae (Supplementary Table 7), none represented the core (Supplementary Table 5). To confirm if the observed community differences respond to geographic barriers, a distance decay analysis was performed. The results showed a non-significant (p > 0.01) association between the shared ASVs (Jaccard similarity index) and geographical distance (km) between sites (ln prokaryotic R2 = 0.007, p = 0.03; phytoplankton R2 = 0.008, p = 0.04) (Figure 4C). The same dispersion analysis performed at different taxonomic levels shows a higher dispersion of taxa in the Patagonian fjord communities.
Additionally, we analyzed the effect of environmental factors on specific taxa to identify potential microbial indicators of environmental change within the Patagonian fjords. Here, we defined microbial indicators as those families with a moderate to strong correlation (i.e., R ≥ 0.5 or ≤−0.5) with one or more oceanographic factors. The analysis identified 29 families (dominated by bacteria) that were influenced by at least one oceanographic factor, while eight families were affected by more than one factor. As expected, DO was identified as a critical factor, affecting 14 families (bacteria 12; archaea 2) (Figure 5). Additionally, several families including Gimesiaceae, Beijerinckiaceae and the NS9 marine group, showed a conserved pattern of correlations and were significantly associated with low temperature and low DO concentrations. In contrast, the bacterial families SAR11 Clade III, Burkholderiaceae and Microbacteriaceae were negatively correlated with salinity and Chl-a. These bacterial families also showed a positive correlation with the eukaryotic families Coccomyxaceae and Pyrenomonadaceae. Furthermore, Coccomyxaceae and Pyrenomonadaceae, which were negatively correlated with Chl-a, were the only phytoplankton families to show a significant correlation with an abiotic factor (Figure 5).
Figure 5. Indicator families from the Patagonian fjord microbial communities. Correlation analysis between environmental variables and fjord families. Only taxa with a significant correlation (p < 0.01) and a moderate to strong effect (R ≥ 0.5 or ≤–0.5) with at least one oceanographic factor are shown. The color gradient represents the Spearman’s correlation coefficient values. Only values with significance (p < 0.01), after Benjamini–Hochberg (BH) FDR correction, are shown. The labels are colored by domain according to the legend at the bottom of the plot. Clade III refers to Pelagibacteraceae subgroup III.
Finally, the relationship previously observed in the Mantel test (Figure 4C) between bacterioplankton and phytoplankton taxa (612 and 33 ASVs, respectively) was analyzed in more detail via network reconstruction (Figure 6). The analysis yielded high modularity within the community. This network was mainly dominated by generalist bacteria present in more than one cluster (Figure 6A), while most connected nodes belonged to ASVs within the major bacterioplankton groups (clusters B1 and B2). All phytoplankton ASVs were located, with high connectivity, at the network center (Figure 6A). According to the beta diversity analysis, phytoplankton ASVs that were abundant in cluster P1 correlated more with cluster B1 while ASVs from cluster P4 interacted more with cluster B2. Subsequently, to better understand specific inter-domain interactions, we performed a new correlation analysis by selecting all bacterioplankton families that correlated (i.e., R ≥ 0.5 or ≤−0.5) with phytoplanktonic taxa. The results show interactions between 27 bacterioplankton and 10 phytoplankton families (Figure 6B). The Pyrenomonadaceae and Coccomyxaceae families accounted for most of the positive correlations with bacteria, especially Schleiferiaceae, Spirosomaceae, Sporichthyaceae, and TRA3-20 (Figure 6B). At the same time, Noelaerhabdaceae (order Isochrysidales) exhibited stronger negative correlations with bacteria (i.e., Methylophilaceae) (Figure 6B).
Figure 6. Patagonian fjord microbial network and inter-domain interactions. (A) Microbial co-occurrence network. The node color (ASV) was assigned according to the group with the highest relative abundance observed for the node. Vertices are colored according to the positive (green) or negative (red) correlation between nodes. (B) Correlation between microbial families influenced by interactions with phytoplankton (R ≥ 0.5 or ≤–0.5). The color gradient presents the degree of pair Spearman’s correlation, with blue representing positive correlations and dark yellow representing negative correlations. Only values of significance (p < 0.01), after Benjamini–Hochberg (BH) FDR correction, are shown. The labels are colored by domain according to the legend at the bottom of the plot. Clades I and III refer to Pelagibacteraceae subgroups I and III, respectively.
Oceanographic conditions along the study area showed a clear stratification in the water column. This stratification is associated with the presence of the Estuarine Water (EW) in the first 24 m, followed by the Modified Sub-Antarctic Water (MSAAW) and deeper down by the Sub-Antarctic Water (SAAW) as has been previously described in the area (Silva and Vargas, 2014). Additionally, 3 types of EW, previously identified in the area (Silva and Guzmán, 2006), could be distinguished in the first meters of the water column. At 5 and 10 m depth, the Estuarine Salty Water (ESW) clearly dominates in the study area, with only Estuarine Brackish Water (EBW) present at 5 m in the Baker Channel and the Iceberg fjord. In agreement with previous results from central Patagonia (∼47–53°S) (Silva and Vargas, 2014; Torres et al., 2014), a low salinity, high oxygen surface layer was detected throughout the study area, even in stations located far from a direct freshwater input (i.e., stations 2, 43, 48, and 68), denoting a strong influence of freshwater along the region. Substantial fluvial input has been described in the region, mainly related to Baker Channel and Última Esperanza Sound (González et al., 2013; Silva and Vargas, 2014). The freshwater contribution from rivers and glaciers is characterized by low nitrate and phosphate, but high silicate and organic matter, playing a key role in local dynamics through mixing/stratification processes and influencing the phytoplankton community (González et al., 2013; Silva and Vargas, 2014; Vargas et al., 2018). The salinity and DO conditions associated with EW have been shown to influence the establishment of estuarine microbial communities in northern Patagonia (41°S), delimiting their ecological niches (Gutiérrez et al., 2018). This agrees with our observation in the central Patagonian area, where salinity and DO are also significant drivers of the bacterioplankton structure. These results show a different response of the fjord microbial community structure relative to the general pattern observed in other oceanographic regions where temperature is the main driver (Sunagawa et al., 2015). Our results also confirm the presence of Estuarine Waters, mainly Estuarine Salty Water (ESW, S = 21–31) along the study area, highlighting a conserved pattern of estuarine influence along 450 km of the Chilean Patagonian fjord region. Freshwater input with low nutrients (nitrate and phosphate) and high sediment material, which limits light penetration in the column water, probably negatively affects phytoplankton biomass. In this regard, the lowest Chl-a concentrations observed in this study, namely in the Baker Channel and Nelson Strait areas, were correlated with a direct freshwater influence. In contrast, the highest Chl-a values were detected along the Messier Channel area (stations 19, 24, 35, 43, and 44), where local dynamics allow for the arrival of SAAW, which is characterized by nutrient-rich waters (Silva and Vargas, 2014; Cuevas et al., 2019). The relationship between low Chl-a and high freshwater input has been previously reported in several areas of Patagonia (Vargas et al., 2018; Cuevas et al., 2019) and other glacier regions, such as Maxwell Bay (King George Island, Antarctic Peninsula) (Meredith et al., 2018).
Freshwater and intertidal waters are characterized by a higher microbial richness than marine waters (Wang et al., 2012). This is reflected in the present study, with areas such as Peel Fjord and Baker Channel showing higher richness and sharp environmental gradients in the surface of the water column, which can be explained by the mixing of sediment and microorganisms from freshwater input (Wang et al., 2012; Rocca et al., 2020). In contrast, due to its connection with an enclosed area containing many fjords, the high richness observed in the Messier Channel area was probably associated with the arrival of sediments and EW, thus accumulating the microbial richness of these fjords. The mixing of these microbial communities (i.e., community coalescence) triggers a structural change that shapes the communities of the Patagonian fjords. However, as in other aquatic environments, the mixing process in this region is not random but facilitated by estuarine circulation, specifically driven by the constant mixing of EW and SAAW that can generate sharp gradients, as observed in this study. This prompts constant community coalescence and the selection of species better adapted to dispersion and mixing (Castledine et al., 2020; Mestre and Höfer, 2020). However, heterogeneous water mixing (i.e., differential mixing between water masses along the fjord system) was also noticed between stations in the study area. These different gradients can also induce variations in the community coalescence mixing ratio and microbial structure (Rillig et al., 2015; Castledine et al., 2020). This was demonstrated by our cluster analysis, in that geographically heterogeneous community structures respond differentially to the measured oceanographic conditions, even when several taxa are shared between clusters, emphasizing the importance of biotic interactions in these communities. Furthermore, evidence suggests that the microbial coalescence process favors system function over the prevalence of input members, selecting the best-adapted taxa, even from the rare biosphere, to ensure productivity of the system (Rillig and Mansour, 2017; Sierocinski et al., 2017; Rocca et al., 2020). Further analysis is now needed to determine if the primary productivity pattern is conserved within different communities of the Patagonian fjords.
The network analysis suggests high modularity in the Patagonian communities (i.e., bacterioplankton and phytoplankton), observing two divergent modules made up of positive biotic connections within modules and negative connections between modules. Modular communities, such as those observed here, have been proposed to emerge after particular coalescence events. These coalescence events produce mixed communities that are adapted to the new resulting environment, while maintaining most of their original species interactions. However, modularity also can be observed when a structured environment limits the mixing between communities, thereby supporting localized interactions (Castledine et al., 2020). The lack of overlap between the two observed modules and their different ecological potentials could be associated here with the gradients caused by heterogeneous mixing of the water masses in the Patagonian fjords (i.e., EW and SAAW); that is, local conditions limit mixing of the communities. Nevertheless, some strong biotic connections were observed between bacterioplankton and phytoplankton at the community and family levels. In the Baker Channel, some localized correlations associated with its differential and specific ecological conditions were also observed. The low dispersion barriers in the area allow dispersion of microorganisms in conjunction with local selection, limiting microbial interactions. In that sense a few ubiquitous and more generalist (i.e., better-adapted) families were distributed throughout the microbial communities of the different fjords. New experiments are now needed to better address the differential mixing ratios affecting the community structures, interactions and productivity in the Patagonian fjords.
Along the 450 km of the Patagonian fjords studied here, the bacterioplankton community was characterized by the dominance of Flavobacteriales, Rhodobacterales and SAR11 Clade I (Pelagibacteraceae). These bacterial groups have been previously described as abundant in this region (Gutiérrez et al., 2018) and in other fjords, such as Arctic Svalbard (Cardman et al., 2014). Flavobacteriales and Rhodobacterales are commonly attached to phytoplankton cells during blooms (Buchan et al., 2014) and are specialized for algal polysaccharide remineralization (Teeling et al., 2016; Kappelmann et al., 2019). In that sense, the high abundance of these bacteria, which were collected during the spring of 2017, could be related to the typical seasonal occurrence of spring phytoplankton blooms (Gutiérrez et al., 2018; Cuevas et al., 2019; Saldías et al., 2019). In agreement with Gutierrez et al., who studied the surface waters of Puyuhuapi fjord in northern Patagonia (Gutiérrez et al., 2018), our results show that the abundant and global marine generalist SAR11 is ubiquitous in the Patagonian fjords. However, the present study also reveals the presence of different sub-clades of SAR11 that are associated with different niches. In particular, SAR11 Clade I (i.e., ecotype SAR11 Clade Ia.1), which has been mainly associated with cold marine waters at high latitudes, was the most abundant sub-clade present in the Patagonian fjords. Meanwhile, Clade III (i.e., ecotype SAR11 Clade III.b), related to freshwater (Brown et al., 2012; Giovannoni, 2017), was associated with the low salinity (i.e., EW) and low Chl-a waters, such as those observed in the Baker Channel. Nevertheless, the conclusions presented in this study should be taken with a degree of caution given the inherent limitations of current molecular techniques applied to measure the full diversity or taxonomy of microbial communities. Limitations in DNA extraction and library construction that may over- or under-represent estimates of microbial communities members have not yet been solved (Oyola et al., 2012; Vincent et al., 2017). In addition, a major technical limitation in this study was the use of a single molecular marker (i.e., the 16S rRNA gene), which is unable to resolve the finest taxonomic discrepancies and relies heavily on database annotation (Rosselló-Móra and Amann, 2015).
The Patagonian fjord phytoplanktonic community was dominated by the orders Thalassiosirales (Thalassiosiraceae and Skeletonemataceae) and Mamiellales (Bathycoccaceae), which have been previously described in the region (Takeuchi and Kohshima, 2004; González et al., 2013; Iriarte et al., 2013; Fernández et al., 2017; Montero et al., 2017; Fuentes et al., 2019). However, the families Pyrenomonadaceae and Coccomyxaceae exhibited a higher correlation with bacterioplankton families. Pyrenomonadaceae and Coccomyxaceae were especially abundant in areas of low salinity and low Chl-a, such as the Baker Channel. These two families are mainly comprised of genera identified in terrestrial and freshwater environments (Guiry and Guiry, 2020), supporting the introduction of microorganisms into these fjords by freshwater inputs. The distinctive oceanographic conditions found in the Baker area explain the segregated microbial communities when compared to the rest of the Patagonian fjord areas and reveal possible specific interactions between the phytoplankton families Coccomyxaceae and Pyrenomonadaceae and some bacterial families (i.e., Schleiferiaceae, Spirosomaceae, Sporichthyaceae, TRA3-20, and SAR11 Clade III). How these interactions impact the system, and if these interactions are direct (i.e., mutualism) or indirect (i.e., trophic cascades), should be addressed in future studies.
Microorganisms are crucial for the formation of an ecosystem. Thus, the essential role of the microbiome within the environmental structure can be studied and associated in relation to normal or perturbed ecological conditions (Glasl et al., 2017; Astudillo-García et al., 2019). Consequently, microbiomes have been used to assess the impact of climate change in highly sensitives ecosystems, such as agricultural soils (Schloter et al., 2018), coral reefs (Glasl et al., 2017), and fjords (Gutiérrez et al., 2015; Eregno et al., 2018). Microbial communities respond rapidly to environmental changes, making them a potential tool to complement current monitoring systems (Glasl et al., 2017; Rocca et al., 2020). However, both the environment and microbial communities are highly dynamic, so the use of these communities as biological indicators presents different challenges, such as understanding system stability and how temporal variation impacts the community structure (Astudillo-García et al., 2019). The use of microbial communities or specific taxa to analyze the health of a natural system will largely depend on our knowledge of the microbial diversity, ecology and stability of the system (Astudillo-García et al., 2019). Exploring the diversity of the microbial diversity is important for understanding their complexity, variation and conservation within an specific environment, which will ultimately improve our understanding of how microorganisms interact with the system (Colwell, 1997; Astudillo-García et al., 2019). Efforts to obtain information on the microbial communities of a given system also help to establish a reference for the analysis of other similar regions.
In the Patagonia region, inter-annual variations (e.g., El Niño-Southern Oscillation and Southern Annular Mode) (Garreaud, 2018) can impact the planktonic community, causing increased harmful algal blooms (León-Muñoz et al., 2018). In this study, the negative associations of SAR11 Clade III with salinity and Chl-a conditions highlight this taxon as a potential indicator of freshwater input into the fjords, especially in the Baker Channel area, which features high freshwater runoff, sediment load penetration and decreased light penetration (González et al., 2013). SAR11 Clade III has not only been previously associated with freshwater (Giovannoni, 2017), but it has also been suggested as a tool to monitor climate change mediated by ocean warming (i.e., by following the migration of tropical ecotypes to polar regions) (Brown et al., 2012). Considering that global climate change is influencing precipitation in the Patagonia region (Garreaud, 2018), with precipitation trends decreasing over north-central Patagonia and increasing south of 50°S (Garreaud et al., 2013), changes in the abundance of SAR11 Clade III could also be a potential indicator of possible climate change effects in the region.
In contrast to the conditions observed in freshwater-influenced stations, Beijerinckiaceae, Gimesiaceae (PVC group; Planctomycetes) and the NS9 marine group (Flavobacteriia; Flavobacteriales) were identified as potential indicators of the low temperature and low DO waters upwelled from the MSAAW. These bacteria have been associated with different depths in the water column (Muck et al., 2019). The NS9 marine group has been commonly found below the deep chlorophyll maximum (DCM) (Cram et al., 2015). Planctomycetes are highly diverse (i.e., genetically) and widespread in aquatic environments (Dedysh and Ivanova, 2019), in which the Gimesiaceae family, in particular, has been associated with deep waters, mainly in lakes (Rojas-Jimenez et al., 2019). In this region, due to the potential changes in freshwater input under the current climate change scenario, alterations to the typical salinity and oxygen concentration could disrupt the dominance of certain taxa in the surface of the column water. This could modify the interactions between surface and subsurface microorganisms in the Patagonian fjords. Further analyses are needed to fully understand how these changes in community structure and interactions could impact the high productivity of the Patagonian fjord system.
The microbial dispersion observed in the central Patagonian fjord area could be due to both estuarine circulation and the normal coalescence process that homogenize microbial communities over hundreds of kilometers within this ecosystem. However, particular local conditions seem to influence the interactions of microorganisms that are possibly co-evolving in these communities. Thus, our results suggest that Patagonia fjords are an ideal location to study selective ecological pressure on microbial coalescent communities. In addition, the microbial communities in Patagonia showed that while high dispersion homogenizes the community, established biotic interactions allow bacterioplankton and phytoplankton to respond differentially to environmental factors. Thus, the significant association identified in this study between bacterioplankton and phytoplankton (i.e., beta diversity and family-level microbial composition) suggests that although abiotic factors directly or indirectly affect particular taxa within the microbial assemblages (e.g., indicators), biological interactions can permeate the entire community structure of the Patagonian fjords. However, particular taxa, such as the SAR11 Clade III, Burkholderiaceae and Microbacteriaceae families, should be further analyzed and potentially used as indicators of freshwater input into the Patagonian fjord region, an ecosystem highly susceptible to climate change. Meanwhile, Gimesiaceae, Beijerinckiaceae and the NS9 marine group could be used to monitor the impact of marine waters upwelled from the SAAW or MSAAW in these fjords. Due to changes in freshwater input under the current climate change scenario, alterations to the typical salinity and DO concentration may disrupt common interactions between surface and subsurface microorganisms in the region. Altogether, this study of the marine microbial communities in the Patagonia fjords, as indicators of environmental variations in Subantarctic fjords, may help us to understand the potential impact of climate change on Patagonian biodiversity and productivity, and ultimately in other fjords worldwide.
The datasets generated for this study can be found in online repositories. The names of the repository/repositories and accession number(s) can be found below: National Center for Biotechnology Information (NCBI), Sequence Read Archive (SRA), BioProject accession ID PRJNA670217.
JT-L collected and processed the samples in the field. JT-L, JC-A, PA-R, JA, and IM processed and analyzed sequence data, analyzed oceanographic data, helped with the discussion, and critically reviewed and edited the manuscript for journal submission. JT-L and BD wrote the manuscript. All authors contributed to the article and approved the submitted version.
This research was funded by “Cruceros de Investigación Marina en Areas Remotas (CIMAR)” – CIMAR Fiordos 23 (CONA C23F 17-02) of the “Servicio Hidrográfico y Oceanográfico de la Armada” (SHOA), the Center for Climate and Resilience Research (CR)2 (ANID/FONDAP/1511000), and the Center for Genome Regulation (CRG) (ANID/FONDAP/15200002). Additionally, JT-L was supported by the National Agency for Research and Development (ANID) National Doctoral Scholarship (no. 21171048) during the analysis and writing of this manuscript. JA thanks ANID National Doctoral Scholarship (no. 21130515) for additional funding support.
The authors declare that the research was conducted in the absence of any commercial or financial relationships that could be construed as a potential conflict of interest.
All claims expressed in this article are solely those of the authors and do not necessarily represent those of their affiliated organizations, or those of the publisher, the editors and the reviewers. Any product that may be evaluated in this article, or claim that may be made by its manufacturer, is not guaranteed or endorsed by the publisher.
We would like to thank the scientific research ship “Cabo de Hornos” (AGS61) of the Chilean navy, their crew and the “Servicio Hidrográfico y Oceanográfico de la Armada” (SHOA) for their help and logistical support during the CIMAR23f campaign. We also thank Christina Ridley for her help in correcting the English version of the manuscript, and the BLOOM laboratory team, especially Antonieta Jojot, Juan Ayala, and Viviana Catalan, for sampling and sample processing. The comments of the editor and reviewers helped us to improve the manuscript.
The Supplementary Material for this article can be found online at: https://www.frontiersin.org/articles/10.3389/fmars.2021.611981/full#supplementary-material
Andersen, K. S., Kirkegaard, R. H., Karst, S. M., and Albertsen, M. (2018). ampvis2: an R package to analyse and visualise 16S rRNA amplicon data. Biorxiv [Preprint] doi: 10.1101/299537
Anderson, M. J. (2001). A new method for non-parametric multivariate analysis of variance. Austral. Ecol. 26, 32–46. doi: 10.1111/j.1442-9993.2001.01070.pp.x
Astudillo-García, C., Hermans, S. M., Stevenson, B., Buckley, H. L., and Lear, G. (2019). Microbial assemblages and bioindicators as proxies for ecosystem health status: potential and limitations. Appl. Microbiol. Biotechnol. 103, 6407–6421. doi: 10.1007/s00253-019-09963-0
Benjamini, Y., and Hochberg, Y. (1995). Controlling the false discovery rate: a practical and powerful approach to multiple testing. J. R. Stat. Soc. Ser. B 57, 289–300. doi: 10.1111/j.2517-6161.1995.tb02031.x
Bokulich, N. A., Kaehler, B. D., Rideout, J. R., Dillon, M., Bolyen, E., Knight, R., et al. (2018). Optimizing taxonomic classification of marker-gene amplicon sequences with QIIME 2’s q2-feature-classifier plugin. Microbiome 6:90. doi: 10.1186/s40168-018-0470-z
Bolyen, E., Rideout, J. R., Dillon, M. R., Bokulich, N. A., Abnet, C. C., Al-Ghalith, G. A., et al. (2019). Reproducible, interactive, scalable and extensible microbiome data science using QIIME 2. Nat. Biotechnol. 37, 852–857. doi: 10.1038/s41587-019-0209-9
Briatte, F. (2020). Ggnet: Functions to Plot NetworksWith ggplot2. R package version 0.1.0. Available online at: https://github.com/briatte/ggnet (accessed November 28, 2020).
Brown, M. V., Lauro, F. M., DeMaere, M. Z., Muir, L., Wilkins, D., Thomas, T., et al. (2012). Global biogeography of SAR11 marine bacteria. Mol. Syst. Biol. 8:595. doi: 10.1038/msb.2012.28
Buchan, A., LeCleir, G. R., Gulvik, C. A., and Gonzalez, J. M. (2014). Master recyclers: features and functions of bacteria associated with phytoplankton blooms. Nat. Rev. Microbiol. 12, 686–698. doi: 10.1038/nrmicro3326
Callahan, B. J., McMurdie, P. J., Rosen, M. J., Han, A. W., Johnson, A. J. A., and Holmes, S. P. (2016). DADA2: high-resolution sample inference from illumina amplicon data. Nat. Methods 13, 581–583. doi: 10.1038/nmeth.3869
Cardman, Z., Arnosti, C., Durbin, A., Ziervogel, K., Cox, C., Steen, A. D., et al. (2014). Verrucomicrobia are candidates for polysaccharide-degrading bacterioplankton in an arctic fjord of svalbard. Appl. Environ. Microbiol. 80, 3749–3756. doi: 10.1128/AEM.00899-14
Castledine, M., Sierocinski, P., Padfield, D., and Buckling, A. (2020). Community coalescence: an eco-evolutionary perspective. Philos. Trans. R. Soc. B Biol. Sci. 375:20190252. doi: 10.1098/rstb.2019.0252
Colwell, R. R. (1997). Microbial diversity: the importance of exploration and conservation. J. Ind. Microbiol. Biotechnol. 18, 302–307. doi: 10.1038/sj.jim.2900390
Cram, J. A., Chow, C. E. T., Sachdeva, R., Needham, D. M., Parada, A. E., Steele, J. A., et al. (2015). Seasonal and interannual variability of the marine bacterioplankton community throughout the water column over ten years. ISME J. 9, 563–580. doi: 10.1038/ismej.2014.153
Csardi, G., and Nepusz, T. (2006). The igraph software package for complex network research. InterJournal 1695, 1–9.
Cuevas, L. A., Tapia, F. J., Iriarte, J. L., González, H. E., Silva, N., and Vargas, C. A. (2019). Interplay between freshwater discharge and oceanic waters modulates phytoplankton size-structure in fjords and channel systems of the chilean patagonia. Prog. Oceanogr. 173, 103–113. doi: 10.1016/j.pocean.2019.02.012
Dale, M. R. T., and Fortin, M. J. (2002). Spatial autocorrelation and statistical tests in ecology. Ecoscience 9, 162–167. doi: 10.1080/11956860.2002.11682702
Decelle, J., Romac, S., Stern, R. F., Bendif, E. M., Zingone, A., Audic, S., et al. (2015). PhytoREF: a reference database of the plastidial 16S rRNA gene of photosynthetic eukaryotes with curated taxonomy. Mol. Ecol. Resour. 15, 1435–1445. doi: 10.1111/1755-0998.12401
Dedysh, S. N., and Ivanova, A. A. (2019). Planctomycetes in boreal and subarctic wetlands: diversity patterns and potential ecological functions. FEMS Microbiol. Ecol. 95:fiy227. doi: 10.1093/femsec/fiy227
Eregno, F. E., Tryland, I., Tjomsland, T., Kempa, M., and Heistad, A. (2018). Hydrodynamic modelling of recreational water quality using Escherichia coli as an indicator of microbial contamination. J. Hydrol. 561, 179–186. doi: 10.1016/j.jhydrol.2018.04.006
Fernández, L. D., Hernández, C. E., Schiaffino, M. R., Izaguirre, I., and Lara, E. (2017). Geographical distance and local environmental conditions drive the genetic population structure of a freshwater microalga (Bathycoccaceae; Chlorophyta) in Patagonian lakes. FEMS Microbiol. Ecol. 93:fix125. doi: 10.1093/femsec/fix125
Fuentes, S., Arroyo, J. I., Rodríguez-Marconi, S., Masotti, I., Alarcón-Schumacher, T., Polz, M. F., et al. (2019). Summer phyto- and bacterioplankton communities during low and high productivity scenarios in the Western Antarctic Peninsula. Polar Biol. 42, 159–169. doi: 10.1007/s00300-018-2411-5
Galili, T. (2015). Dendextend: an R package for visualizing, adjusting, and comparing trees of hierarchical clustering. Bioinformatics 31, 3718–3720. doi: 10.1093/bioinformatics/btv428
Garreaud, R., Lopez, P., Minvielle, M., and Rojas, M. (2013). Large-scale control on the patagonian climate. J. Clim. 26, 215–230. doi: 10.1175/JCLI-D-12-00001.1
Garreaud, R. D. (2018). Record-breaking climate anomalies lead to severe drought and environmental disruption in western Patagonia in 2016. Clim. Res. 74, 217–229. doi: 10.3354/cr01505
Giovannoni, S. J. (2017). SAR11 bacteria: the most abundant plankton in the oceans. Ann. Rev. Mar. Sci. 9, 231–255. doi: 10.1146/annurev-marine-010814-015934
Glasl, B., Webster, N. S., and Bourne, D. G. (2017). Microbial indicators as a diagnostic tool for assessing water quality and climate stress in coral reef ecosystems. Mar. Biol. 164:91. doi: 10.1007/s00227-017-3097-x
González, H. E., Castro, L. R., Daneri, G., Iriarte, J. L., Silva, N., Tapia, F., et al. (2013). Land–ocean gradient in haline stratification and its effects on plankton dynamics and trophic carbon fluxes in Chilean Patagonian fjords (47–50°S). Prog. Oceanogr. 119, 32–47. doi: 10.1016/j.pocean.2013.06.003
González, H. E., Nimptsch, J., Giesecke, R., and Silva, N. (2019). Organic matter distribution, composition and its possible fate in the Chilean North-Patagonian estuarine system. Sci. Total Environ. 657, 1419–1431. doi: 10.1016/j.scitotenv.2018.11.445
Guiry, M. D., and Guiry, G. M. (2020). AlgaeBase. Available online at: https://www.algaebase.org (accessed August 2, 2020).
Gutiérrez, M. H., Galand, P. E., Moffat, C., and Pantoja, S. (2015). Melting glacier impacts community structure of bacteria, archaea and fungi in a Chilean Patagonia fjord. Environ. Microbiol. 17, 3882–3897. doi: 10.1111/1462-2920.12872
Gutiérrez, M. H., Narváez, D., Daneri, G., Montero, P., Pérez-Santos, I., and Pantoja, S. (2018). Linking seasonal reduction of microbial diversity to increase in winter temperature of waters of a Chilean Patagonia Fjord. Front. Mar. Sci. 5:277. doi: 10.3389/fmars.2018.00277
Harrell, F. E. Jr. (2020). Hmisc: Harrell Miscellaneous. R package version 4.5.0. Available online at: https://cran.r-project.org/package=Hmisc (accessed February 28, 2021).
Iriarte, J., León-Muñoz, J., Marcé, R., Clément, A., and Lara, C. (2016). Influence of seasonal freshwater streamflow regimes on phytoplankton blooms in a Patagonian fjord. New Zeal. J. Mar. Freshw. Res. 51, 304–315. doi: 10.1080/00288330.2016.1220955
Iriarte, J. L. (2018). Natural and human influences on marine processes in Patagonian subantarctic coastal waters. Front. Mar. Sci. 5:360. doi: 10.3389/fmars.2018.00360
Iriarte, J. L., González, H. E., and Nahuelhual, L. (2010). Patagonian Fjord ecosystems in southern Chile as a highly vulnerable region: problems and needs. Ambio 39, 463–466. doi: 10.1007/s13280-010-0049-9
Iriarte, J. L., Pantoja, S., and Daneri, G. (2014). Oceanographic processes in Chilean fjords of Patagonia: from small to large-scale studies. Prog. Oceanogr. 129, 1–7. doi: 10.1016/j.pocean.2014.10.004
Iriarte, J. L., Pantoja, S., González, H. E., Silva, G., Paves, H., Labbé, P., et al. (2013). Assessing the micro-phytoplankton response to nitrate in Comau Fjord (42 S) in Patagonia (Chile), using a microcosms approach. Environ. Monit. Assess. 185, 5055–5070. doi: 10.1007/s10661-012-2925-1
Kahle, D., and Wickham, H. (2013). ggmap: spatial visualization with ggplot2. R J. 5, 144–161. doi: 10.32614/rj-2013-014
Kappelmann, L., Krüger, K., Hehemann, J.-H., Harder, J., Markert, S., Unfried, F., et al. (2019). Polysaccharide utilization loci of North Sea Flavobacteriia as basis for using SusC/D-protein expression for predicting major phytoplankton glycans. ISME J. 13, 76–91. doi: 10.1038/s41396-018-0242-6
Kurtz, Z. D., Müller, C. L., Miraldi, E. R., Littman, D. R., Blaser, M. J., and Bonneau, R. A. (2015). Sparse and compositionally robust inference of microbial ecological networks. PLoS Comput. Biol. 11:e1004226. doi: 10.1371/journal.pcbi.1004226
Legendre, P., and Fortin, M. J. (2010). Comparison of the Mantel test and alternative approaches for detecting complex multivariate relationships in the spatial analysis of genetic data. Mol. Ecol. Resour. 10, 831–844. doi: 10.1111/j.1755-0998.2010.02866.x
Legendre, P., and Gallagher, E. D. (2001). Ecologically meaningful transformations for ordination of species data. Oecologia 129, 271–280. doi: 10.1007/s004420100716
León-Muñoz, J., Urbina, M. A., Garreaud, R., and Iriarte, J. L. (2018). Hydroclimatic conditions trigger record harmful algal bloom in western Patagonia (summer 2016). Sci. Rep. 8:1330. doi: 10.1038/s41598-018-19461-4
Mantel, N. (1967). The detection of disease clustering and a generalized regression approach. Cancer Res. 27, 209–220.
McMurdie, P. J., and Holmes, S. (2013). phyloseq: an R package for reproducible interactive analysis and graphics of microbiome census data. PLoS One 8:e61217. doi: 10.1371/journal.pone.0061217
Meinshausen, N., and Bühlmann, P. (2006). High-dimensional graphs and variable selection with the Lasso. Ann. Stat. 34, 1436–1462. doi: 10.1214/009053606000000281
Meredith, M. P., Falk, U., Bers, A. V., Mackensen, A., Schloss, I. R., Ruiz Barlett, E., et al. (2018). Anatomy of a glacial meltwater discharge event in an Antarctic cove. Philos. Trans. R. Soc. A Math. Phys. Eng. Sci. 376:20170163. doi: 10.1098/rsta.2017.0163
Mestre, M., and Höfer, J. (2020). The microbial conveyor belt: connecting the globe through dispersion and dormancy. Trends Microbiol 29, 482–492. doi: 10.1016/j.tim.2020.10.007
Montero, P., Pérez-Santos, I., Daneri, G., Gutiérrez, M. H., Igor, G., Seguel, R., et al. (2017). A winter dinoflagellate bloom drives high rates of primary production in a Patagonian fjord ecosystem. Estuar. Coast. Shelf Sci. 199, 105–116. doi: 10.1016/j.ecss.2017.09.027
Moreno-Pino, M., Krock, B., De la Iglesia, R., Echenique-Subiabre, I., Pizarro, G., Vásquez, M., et al. (2018). Next generation sequencing and mass spectrometry reveal high taxonomic diversity and complex phytoplankton-phycotoxins patterns in Southeastern Pacific fjords. Toxicon 151, 5–14. doi: 10.1016/j.toxicon.2018.06.078
Muck, S., De Corte, D., Clifford, E. L., Bayer, B., Herndl, G. J., and Sintes, E. (2019). Niche differentiation of aerobic and anaerobic ammonia oxidizers in a high latitude deep oxygen minimum zone. Front. Microbiol 10:2141. doi: 10.3389/fmicb.2019.02141
Murtagh, F., and Legendre, P. (2014). Ward’s hierarchical agglomerative clustering method: which algorithms implement ward’s criterion? J. Classif. 31, 274–295. doi: 10.1007/s00357-014-9161-z
Oksanen, J., Blanchet, F. G., Friendly, M., Kindt, R., Legendre, P., McGlinn, D., et al. (2019). Vegan: Community Ecology Package. R package version 2.5.7. Available online at: https://cran.r-project.org/package=vegan (accessed November 28, 2020).
Olsen, L. M., Hernández, K. L., Ardelan, M., Van Iriarte, J. L., Bizsel, K. C., and Olsen, Y. (2017). Responses in bacterial community structure to waste nutrients from aquaculture: an in situ microcosm experiment in a Chilean fjord. Aquac. Environ. Interact. 9, 21–32. doi: 10.3354/aei00212
Oyola, S. O., Otto, T. D., Gu, Y., Maslen, G., Manske, M., Campino, S., et al. (2012). Optimizing illumina next-generation sequencing library preparation for extremely at-biased genomes. BMC Genomics 13:1. doi: 10.1186/1471-2164-13-1
Parada, A. E., Needham, D. M., and Fuhrman, J. A. (2016). Every base matters: assessing small subunit rRNA primers for marine microbiomes with mock communities, time series and global field samples. Environ. Microbiol. 18, 1403–1414. doi: 10.1111/1462-2920.13023
Parsons, T. R., Maita, Y., and Lalli, C. M. (1984). A Manual of Chemical and Biological Methods for Seawater Analysis. Oxford: Pergamon Press.
Quast, C., Pruesse, E., Yilmaz, P., Gerken, J., Schweer, T., Yarza, P., et al. (2013). The SILVA ribosomal RNA gene database project: improved data processing and web-based tools. Nucleic Acids Res. 41, 590–596. doi: 10.1093/nar/gks1219
R Core Team. (2020). R: A Language and Environment for Statistical Computing. R version 3.6.3. Available online at: https://www.r-project.org/ (accessed February 28, 2021).
Rillig, M. C., Antonovics, J., Caruso, T., Lehmann, A., Powell, J. R., Veresoglou, S. D., et al. (2015). Interchange of entire communities: microbial community coalescence. Trends Ecol. Evol. 30, 470–476. doi: 10.1016/j.tree.2015.06.004
Rillig, M. C., and Mansour, I. (2017). Microbial ecology: community coalescence stirs things up. Curr. Biol. 27, R1280–R1282. doi: 10.1016/j.cub.2017.10.027
Rocca, J. D., Simonin, M., Bernhardt, E. S., Washburne, A. D., and Wright, J. P. (2020). Rare microbial taxa emerge when communities collide: freshwater and marine microbiome responses to experimental mixing. Ecology 101:e02956. doi: 10.1002/ecy.2956
Rognes, T., Flouri, T., Nichols, B., Quince, C., and Mahé, F. (2016). VSEARCH: a versatile open source tool for metagenomics. PeerJ 4:e2584. doi: 10.7717/peerj.2584
Rojas-Jimenez, K., Araya-Lobo, A., Quesada-Perez, F., Akerman-Sanchez, J., Delgado-Duran, B., Ganzert, L., et al. (2019). Variation of bacterial communities along the vertical gradient in Lake Issyk Kul, Kyrgyzstan. Biorxiv. [Preprint] doi: 10.1101/864355 Biorxiv: 864355,
Rosselló-Móra, R., and Amann, R. (2015). Past and future species definitions for Bacteria and Archaea. Syst. Appl. Microbiol. 38, 209–216. doi: 10.1016/j.syapm.2015.02.001
Rousseeuw, P. J. (1987). Silhouettes: a graphical aid to the interpretation and validation of cluster analysis. J. Comput. Appl. Math. 20, 53–65. doi: 10.1016/0377-0427(87)90125-7
Saldías, G. S., Sobarzo, M., and Quiñones, R. (2019). Freshwater structure and its seasonal variability off western Patagonia. Prog. Oceanogr. 174, 143–153. doi: 10.1016/j.pocean.2018.10.014
Schlitzer, R. (2020). Ocean Data View. Version 5.2.0. Available online at: https://www.odv.awi.de (accessed February 18, 2020).
Schloter, M., Nannipieri, P., Sørensen, S. J., and van Elsas, J. D. (2018). Microbial indicators for soil quality. Biol. Fertil. Soils 54, 1–10. doi: 10.1007/s00374-017-1248-3
Sierocinski, P., Milferstedt, K., Bayer, F., Großkopf, T., Alston, M., Bastkowski, S., et al. (2017). A single community dominates structure and function of a mixture of multiple methanogenic communities. Curr. Biol. 27, 3390–3395. doi: 10.1016/j.cub.2017.09.056
Silva, N., and Guzmán, D. (2006). Condiciones oceanográficas físicas y químicas, entre boca del guafo y fiordo aysén (crucero cimar 7 fiordos). Cienc. Y Tecnol. del Mar 29, 25–44.
Silva, N., and Vargas, C. A. (2014). Hypoxia in chilean patagonian fjords. Prog. Oceanogr. 129, 62–74. doi: 10.1016/j.pocean.2014.05.016
Strickland, J. D. H., and Parsons, T. R. (1972). A Practical Handbook of Seawater Analysis, 167th Edn. Ottawa, CA: Fisheries research board of Canada.
Sunagawa, S., Coelho, L. P., Chaffron, S., Kultima, J. R., Labadie, K., Salazar, G., et al. (2015). Structure and function of the global ocean microbiome. Science 348:1261359. doi: 10.1126/science.1261359
Suzuki, R., Terada, Y., and Shimodaira, H. (2019). Pvclust: Hierarchical Clustering with P-Values via Multiscale Bootstrap Resampling. R package version 2.2.0. Available online at: https://cran.r-project.org/package=pvclust (accessed November 19, 2019).
Takeuchi, N., and Kohshima, S. (2004). A snow algal community on Tyndall Glacier in the Southern Patagonia Icefield, Chile. Arctic Antarct. Alp. Res. 36, 92–99. doi: 10.1657/1523-04302004036
Teeling, H., Fuchs, B. M., Bennke, C. M., Krüger, K., Chafee, M., Kappelmann, L., et al. (2016). Recurring patterns in bacterioplankton dynamics during coastal spring algae blooms. Elife 5:e11888. doi: 10.7554/eLife.11888
Tillett, D., and Neilan, B. A. (2000). Xanthogenate nucleic acid isolation from cultured and environmental cyanobacteria. J. Phycol. 36, 251–258. doi: 10.1046/j.1529-8817.2000.99079.x
Torres, R., Pantoja, S., Harada, N., González, H. E., Daneri, G., Frangopulos, M., et al. (2011). Air-sea CO2 fluxes along the coast of Chile: from CO2 outgassing in central northern upwelling waters to CO2 uptake in southern Patagonian fjords. J. Geophys. Res. Ocean 116, 1–17. doi: 10.1029/2010JC006344
Torres, R., Silva, N., Reid, B., and Frangopulos, M. (2014). Silicic acid enrichment of subantarctic surface water from continental inputs along the Patagonian archipelago interior sea (41-56°S). Prog. Oceanogr. 129, 50–61. doi: 10.1016/j.pocean.2014.09.008
Vargas, C. A., Cuevas, L. A., Silva, N., González, H. E., De Pol-Holz, R., and Narváez, D. A. (2018). Influence of glacier melting and river discharges on the nutrient distribution and DIC Recycling in the Southern Chilean Patagonia. J. Geophys. Res. Biogeosciences 123, 256–270. doi: 10.1002/2017JG003907
Vincent, A. T., Derome, N., Boyle, B., Culley, A. I., and Charette, S. J. (2017). Next-generation sequencing (NGS) in the microbiological world: how to make the most of your money. J. Microbiol. Methods 138, 60–71. doi: 10.1016/j.mimet.2016.02.016
Wang, Y., Sheng, H. F., He, Y., Wu, J. Y., Jiang, Y. X., Tam, N. F. Y., et al. (2012). Comparison of the levels of bacterial diversity in freshwater, intertidal wetland, and marine sediments by using millions of illumina tags. Appl. Environ. Microbiol. 78, 8264–8271. doi: 10.1128/AEM.01821-12
Wei, T., and Simko, V. (2017). R Package “Corrplot”: Visualization of a Correlation Matrix. R version 0.89. Available online at: https://github.com/taiyun/corrplot (accessed June 6, 2021).
Keywords: Patagonian fjords, Subantarctic, Estuarine Water, bacterioplankton, eukaryotic phytoplankton, microbial indicator
Citation: Tamayo-Leiva J, Cifuentes-Anticevic J, Aparicio-Rizzo P, Arroyo JI, Masotti I and Díez B (2021) Influence of Estuarine Water on the Microbial Community Structure of Patagonian Fjords. Front. Mar. Sci. 8:611981. doi: 10.3389/fmars.2021.611981
Received: 29 September 2020; Accepted: 25 June 2021;
Published: 23 July 2021.
Edited by:
Giorgio Bavestrello, University of Genoa, ItalyReviewed by:
Sokratis Papaspyrou, University of Cádiz, SpainCopyright © 2021 Tamayo-Leiva, Cifuentes-Anticevic, Aparicio-Rizzo, Arroyo, Masotti and Díez. This is an open-access article distributed under the terms of the Creative Commons Attribution License (CC BY). The use, distribution or reproduction in other forums is permitted, provided the original author(s) and the copyright owner(s) are credited and that the original publication in this journal is cited, in accordance with accepted academic practice. No use, distribution or reproduction is permitted which does not comply with these terms.
*Correspondence: Beatriz Díez, YmRpZXpAYmlvLnB1Yy5jbA==
Disclaimer: All claims expressed in this article are solely those of the authors and do not necessarily represent those of their affiliated organizations, or those of the publisher, the editors and the reviewers. Any product that may be evaluated in this article or claim that may be made by its manufacturer is not guaranteed or endorsed by the publisher.
Research integrity at Frontiers
Learn more about the work of our research integrity team to safeguard the quality of each article we publish.