- 1Hawai‘i Institute of Marine Biology, University of Hawai‘i at Mānoa, Kāne‘ohe, HI, United States
- 2ARC Centre of Excellence for Coral Reef Studies, James Cook University, Townsville, QLD, Australia
- 3Physics and Marine Geophysical Laboratory, College of Science and Engineering, James Cook University, Townsville, QLD, Australia
- 4NOAA / NESDIS / STAR Coral Reef Watch, College Park, MD, United States
- 5Earth System Science Interdisciplinary Center / Cooperative Institute for Satellite Earth System Studies, University of Maryland, College Park, College Park, MD, United States
- 6Marine Laboratory, University of Guam, Mangilao, GU, United States
Coral diseases contribute to the decline of reef communities, but factors that lead to disease are difficult to detect. In the present study, we develop a multi-species model of colony-scale risk for the class of coral diseases referred to as White Syndromes, investigating the role of current or past conditions, including both environmental stressors and biological drivers at the colony and community scales. Investigating 7 years of coral survey data at five sites in Guam we identify multiple environmental and ecological associations with White Syndrome, including a negative relationship between short-term heat stress and White Syndrome occurrence, and strong evidence of increasing size-dependent White Syndrome risk across coral species. Our findings result in a generalized model used to predict colony-scale White Syndrome risk for multiple species, highlighting the value of long-term monitoring efforts to detect drivers of coral disease.
Introduction
Diseases naturally persist at low prevalence in coral communities, but may emerge into high-prevalence, destructive outbreaks over short time periods under conducive conditions. Acute and chronic coral tissue loss diseases increase coral host mortality (Sutherland et al., 2004; Raymundo et al., 2008) and contribute to the broader decline of coral reefs alongside other impacts including bleaching from marine heatwaves (Le Nohaïc et al., 2017), reduced recruitment on reefs (Hughes et al., 2019), and losses in functional redundancy (Pratchett et al., 2014; Descombes et al., 2015; Richardson et al., 2018). The temporal and spatial variability of coral disease, its naturally low prevalence, and the sparse data on conditions preceding disease, pose significant challenges to predicting patterns in disease occurrence.
Variability in disease prevalence reflects a complex suite of antecedent environmental and biological conditions. Thermal stress and resultant bleaching have previously been identified as a driver of coral disease, including high-mortality outbreaks (Bruno et al., 2007; Brandt and McManus, 2009; Miller et al., 2009). The history of thermal stress at a site has found widespread use as an indicator of disease risk, but its effect is variable, due in part to the varied response of coral species to heat stress (Heron et al., 2010; Randall et al., 2014; McClanahan et al., 2015). Chronic nutrient enrichment and increased chlorophyll-a (Chl-a) have both been linked to increased prevalence and severity of coral disease and bleaching (Redding et al., 2013; Thompson et al., 2014; Vega Thurber et al., 2014). Sediment stress and turbidity from offshore dredging and extreme runoff events due to land-use change are associated with increased coral disease prevalence on adjacent reefs (Pollock et al., 2014; Sheridan et al., 2014; Otaño-Cruz et al., 2017). Proximity to human populations, degrading water quality, thermal stress, and additional anthropogenic stressors precipitate either chronic infection or large-scale, multi-year outbreaks (Aeby et al., 2011a,b) such as those currently occurring in the Caribbean (Walton et al., 2018). Also at the colony- or community-scale, fragmentation and sediment stress (particularly terrigenous sediments) impacting corals both increase prevalence of tissue loss diseases when combined with thermal stress (Brandt et al., 2013).
In combination with environmental stress, biological drivers at the scale of individual colonies or the coral community may increase the probability of disease occurrence. However, the relationship between prior biological interactions and current disease occurrence at these scales is poorly described, in part due to a scarcity of longitudinal studies where disease and its biological drivers are repeatedly observed at fine scales over time. At the scale of individual coral colonies, reduced diversity of coral-microbial communities has been associated with increased disease susceptibility in Acroporid corals (Pollock et al., 2019) and shifts in microbial composition linked to tissue loss diseases in the Caribbean (Rosenberg et al., 2007; Séré et al., 2013; Bourne et al., 2016; Meyer et al., 2019). Evidence at the colony-scale supports that even limited removal of tissue or skeleton by fish may impact coral fitness (Rotjan and Lewis, 2008), that high prevalence of corallivorous gastropods is associated with outbreaks of White Syndrome (Antonius and Riegl, 1998), and Drupella spp. may aid in the transmission of coral diseases (Nicolet et al., 2013).
Coral colony size may influence disease susceptibility through increased area for pathogen attack and opportunities for physical damage (Lamb et al., 2014, 2016) or from age-related decreases in microbial diversity as a barrier to pathogen colonization (Pollock et al., 2019) or other factors associated with senescence, and has been observed as a significant colony-scale factor in previous studies of coral disease (Caldwell et al., 2020). Likewise, colony morphology may influence disease lesion dynamics, driving tissue loss and recovery rates (Lozada-Misa et al., 2015). In addition, diverse fish communities have been hypothesized to reduce disease risk at the community scale by reducing the abundance of potential vectors (Raymundo et al., 2009; Zaneveld et al., 2016). Fragmentation and bleaching may shift the composition of coral communities toward increased disease susceptibility or reduced capacity for recovery following these disturbances (Ostrander et al., 2000; Thompson et al., 2014; Holbrook et al., 2018).
The broad suite of biological drivers associated with coral disease requires further study using survey methods that adequately capture the accumulation of stress to coral hosts with sufficient temporal frequency, and the varied responses of different coral species to stressors. Importantly, the unique combination of environmental and biological conditions that precede disease may be specific to disease type and/or geographic region. For example, examinations of individual diseases have helped to identify factors driving patterns of Porites tissue loss (Porites white syndrome), revealing a strong association with reductions in butterflyfish (Chaetodontidae) abundance in Hawaii, the Philippines, and the Great Barrier Reef (Raymundo et al., 2009; Williams et al., 2010). Comparisons such as these highlight the importance of modeling individual disease risk to identify factors driving patterns, which consider the unique local environments, ecology, and prior conditions that exist across coral habitats.
Improved prediction of coral disease at large scales requires thorough testing of biological and environmental covariates on a variety of coral species, using surveys designed to detect local disease occurrence through time. Stratified random survey designs are widespread but poorly equipped to detect local stress accumulation, and longitudinal studies are rare due to the cost of repeatedly surveying coral communities over long periods of time. This has led to a paucity of data to link disease occurrence to prior environmental or biological conditions – relationships that may ultimately allow prediction of disease risk at these locations and others that may lack longitudinal data. Here, we investigate relationships between the suite of coral diseases referred to as White Syndromes (WS) and current or past conditions, including both environmental stressors and biological drivers at the colony and community scale. “White syndrome” is a collective term used mainly in the Indo-Pacific, describing acute and rapid tissue loss that leaves behind bare skeleton, which can be rapidly colonized by algae. It is currently unknown whether variation in patterns of tissue loss is due to differences in host response or differences in causative agents (or both) as limited evidence suggests more than one causal agent (reviewed in Bourne et al., 2015). White Syndromes are the most commonly reported and widespread coral disease in the Indo-Pacific, in terms of both host species and geographic range, and may be either acute or chronic. Using insights from longitudinal surveys at five locations in Guam, we developed a predictive model of WS risk, improving our understanding of drivers for this suite of diseases and providing a powerful tool in managing future WS risk and impact.
Methods
Field Sites
In 2009, we identified five sites on shallow (1-3 m) coral reef flats on the northwestern coast of Guam (approx. 144.7°E, 13.5°N). At each site, we established three belt transects (20 × 1 m) within 500 m of shore to capture the distinct coral communities of each site. Each of the 15 transects was marked at both ends by a stake hammered and epoxied into the substrate or a large zip tie attached to existing reef structure and marker GPS coordinates recorded; this allowed repeated sampling of the transect. The five sites span a range of water quality and anthropogenic stress along the western coastline of Guam (see Redding et al., 2013 for a description of anthropogenic stressors at sites) (Figure 1; Table 1; Supplementary Figures 1-3; and Supplementary Table 1).
Survey Methods
A total of 72,904 colony-scale coral health surveys were conducted during quarterly surveys (weather permitting) between 2009 and 2018. Within each belt transect, snorkelers recorded the species, size, and health condition of each coral colony (i.e., healthy, disease type, evident predation, algal overgrowth, bleaching), following methods of Myers and Raymundo (2009). To limit observer bias, all taxonomic assessments and disease observations were conducted by a single expert observer (Raymundo) who confirmed visual classification of disease type. Taxonomic identification was based on Randall and Myers (1983), Wallace (1999), Veron and Stafford-Smith (2000) and verified via photographs by D. Burdick (pers. comm.) (Randall and Myers, 1983; Wallace, 1999; Veron and Stafford-Smith, 2000). Representative photographs of major species used in final analyses are included as Supplementary Figure 4. Multiple adjacent coral colonies of the same species were estimated as individuals only when physically separated with clear tissue or skeletal borders. Clusters of staghorn corals falling within the transect band were considered distinct colonies when thickets were entirely disconnected or where it could be visually determined that branches were not connected between clusters. All colonies within the belt were visually inspected, binned into one of six size classes using maximum colony diameter, and the decision tree designed by Raymundo et al. (2008) was employed to assess tissue loss and partial mortality. A positive identification of white syndrome was based on the presence of an area of bare white skeleton devoid of tissue and an active progressing front marked by tissue erosion along the margin, often accompanied by mucous secretion and/or loss of pigmentation in the tissue bordering the disease front (Work and Aeby, 2006). In addition, the absence of a colored band or deformed skeletal features eliminated other known diseases. In larger lesions, recruitment of early successional green filamentous algae onto skeleton exposed for at least 1 week was an additional feature. Lastly, an absence of coral predators (Acanthaster planci and Drupella spp.) near or within the colony supported the assessment of disease.
As monitoring progressed, it became clear to surveyors that patterns of tissue loss indicative of WS differed between major taxa (Supplementary Figure 5). WS lesions on Staghorn Acropora spp. manifested as a band of tissue loss progressing quickly, most commonly from the base of a branch, upward. A rapid rate of infection was indicated by predominance of recently denuded skeleton relative to skeleton newly colonized by early successional stage green filamentous algae. Porites spp. WS lesions were generally concentric, multifocal patches of tissue loss that developed anywhere on the colony, resulting in long-term chronic infection. Porites responded to infection with excessive mucous production in infected tissue and paling of tissue ahead of the progressing front. Pavona spp. lesions generally began along the upper frond margins, progressing downward along the frond while exposing white denuded skeleton. Finally, Pocillopora spp. infections began at the base of a branch and progressed slowly, as indicated by a larger proportion of dead, heavily fouled skeleton and typically a single branch displaying active tissue loss. Regardless of species surveyed, old-dead or heavily fouled dead skeleton alone was not considered evidence of an active WS lesion, and only colonies with evidence of active WS lesions were marked as having WS present.
Disease Driver Generation
White Syndrome drivers were generated and grouped for variable selection according to hypotheses described in Table 2, with additional data handling described below. Season of survey, colony size, and species were obtained from the survey data. Season was categorized as Winter (cool, low precipitation) or Summer (warm, high precipitation) based on the survey month. Size data was converted from categorical size classes into a continuous variable, normalized to each species’ average size: categorical colony sizes [Size Class 1 (0-10 cm), 2 (11-30 cm), 3 (31-60 cm), 4 (61-100 cm), 5 (1-2 m), or 6 (2-5 m)] was converted to a continuous variable by calculating the midpoint of each size class (example: Size Class 1 is 0-10 cm, midpoint taken is 5 cm), log transforming the midpoint value, and standardizing using the mean and standard deviation of each species, resulting in a species-specific relative colony size. This process allows the model-estimated effect of colony size to be interpreted across coral species as the change in WS risk per unit of standard deviation from the species’ average colony size. Surveys prior to 2011, which did not include size class data, were removed. Coral species groups were defined within the survey methodology and categorized to genus only if species could not be identified in the field using morphological attributes (e.g. “Massive Porites”). Coral species groups with fewer than 10 confirmed cases of WS from 2009 to 2018 were removed – constraining model fitting to only surveys of species observed as (at-least) moderately susceptible to WS (Supplementary Figure 2, N = 59,585). A total of 13 coral species or species groups remained for analysis (Supplementary Table 1).
Community characteristics for each survey and transect were summarized from the survey data. While some transect-scale biological predictors such as percent hard coral cover (using line intercept methods) were already available for the surveyed areas, other drivers required summarization of colony-scale biological variables to describe the local coral community. Diversity of coral species on transect during each survey period was calculated as an Inverse Simpson’s Diversity Index where 0 indicated a species monoculture and 1 indicated infinite diversity. Proportion of coral colonies susceptible to WS (species with ten or more confirmed cases of WS from 2009 to 2018) was calculated by taking the ratio of WS-susceptible colonies surveyed over the total number of colonies observed on transect during each survey period. Proportion of WS-susceptible colonies on transect was additionally used to estimate the percent of hard coral cover occupied by WS-susceptible species. In addition to recorded colony scale presence-absence observations, prevalence of damselfish predation (farming behavior), gastropod predation, macroalgal overgrowth, and bleaching on transect was calculated for the current survey period, and also as a mean prevalence across all surveys carried out in the year prior to the current survey. Bleaching Pressure, a measure of projected impact of temperature stress, was calculated as the product of a surveyed colony’s species-specific Bleaching Response Index (Swain et al., 2016) and the maximum observed Degree Heating Week (DHW) value at a site within 1 year prior to a coral survey.
Satellite-based environmental variables provided a history of environmental conditions in the vicinity of each site. Sea surface temperature (SST) and derived metrics produced by NOAA Coral Reef Watch (CRW) describe thermal conditions and stress at ∼5 km resolution. These include measures of year-round (SST anomaly) and summertime (HotSpot, DHW, Hot Snap) thermal stress, as well as winter temperatures (the prior winter condition metric was calculated as the CRW Winter Condition value at each site at the end of the previous winter; Heron et al., 2010). Heat stress for the period immediately before a survey (short-term) was represented by using daily SST-based metrics from the same day, and prior (long-term) heat stress was represented by means or maximums of thermal stress metrics over the year prior to a coral survey date. VIIRS ocean color data were used to describe the local ocean color parameters chlorophyll-a (Chl-a) concentration, a proxy for ocean productivity, and the diffuse attenuation coefficient at 490 nm, Kd (490), an indicator of turbidity (driver groupings summarized in Table 2). We note that Guam winters are characterized by low rainfall, resulting in low Chl-a and turbidity, compared to summertime conditions where increased rainfall results in elevated Chl-a and turbidity (in part due to runoff). Ocean color data density was variable and typically insufficient to provide continuous time-series for comparison with disease observations. To overcome this, the sparse, daily 750 m data were aggregated by taking the 90th percentile value from a 7-day time window and 61-pixel (∼8.25 km) rosette spatial window, see Geiger et al., unpublished. Weekly ocean color data were summarized as mean or maximum values over 1-month (short-term) or 1-year (long-term) windows prior to a coral survey date.
Variable Selection and Random Effects Specification
After driver generation and data handling, additional steps were taken to ensure robust and repeatable variable selection. In the interest of comparing disease drivers as equally as possible, the dataset was filtered using the complete.cases() function from the R package stats (R Core Team, 2018) to produce a consensus dataset that featured only complete cases for all drivers to be tested (no NA values, N = 28,687). Removal of incomplete observations ensured that the number of coral surveys used to train models of WS remained constant regardless of which drivers were included. The consensus dataset (Figure 2) was subsequently split into two random partitions representing approximately 75% (n = 21,514) and 25% (n = 7,173) of the total dataset using the createDataPartition() function from the R package Caret (Kuhn, 2020) while respecting the distribution of WS cases through time across sites, transects, and species. The 75% partition was used for model fitting and the 25% partition was reserved for later validation of model fit, sensitivity, and predictions of WS (i.e., using out-of-sample data).
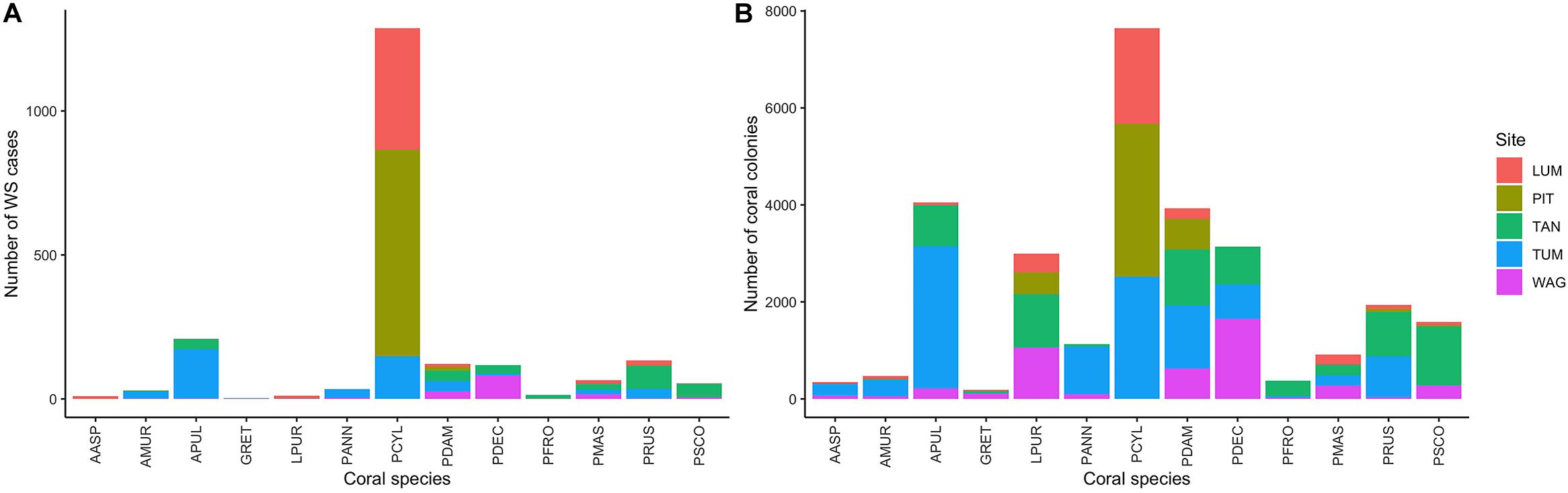
Figure 2. (A) Number of White Syndrome (WS) cases and (B) total counts of coral colonies per species and site in the full consensus dataset.
A generalized linear mixed model (glmm) of WS via the R package lme4 included season and a random effect structure for year, species, and transect nested within site to account for the unique temporal, spatial, and biological structure of the longitudinal dataset (Bates et al., 2015). The season in which a coral survey occurred (summer or winter) was included as a covariate in all models rather than a random effect to reflect interest in tropical seasonality and its interaction with other variables as a driver of WS.
Base model specification with random effects:
Using this base model specification, variable selection was carried out for each group of variables representing our hypotheses (Table 2). Each potential WS driver in a hypothesis group was tested individually in the base model specification (random effects plus season) to determine standalone performance as a WS predictor. Variables were removed from further consideration if they, when tested individually, induced model singularity, increased model convergence above a threshold of 0.01, or were did not explain a significant proportion of model variance. A group model was then fit using the base model specification and including all variables from a single hypothesis group that were found to be significant predictors of WS in individual models. Multicollinearity was common among significant WS predictors from a single hypothesis group. Group models were further reduced by removing the variable with the highest variance inflation factor (VIF) above a threshold of four and refitting the newly reduced group model. Once all covariates in a group model had acceptable VIF, non-significant drivers with the lowest Z-score or high standard error (typically above 1-1.5) were removed and the model refit to the remaining variables. This process was repeated until each hypothesis group of variables was reduced to a single WS predictor or a set of predictors that could coexist without impacting model stability. These variables were then flagged for further variable selection in later, full models of WS risk occurrence. The full process of variable selection and model testing is pictured in Supplementary Figure 6.
After variable selection was performed separately on each hypothesis group, all environmental variables flagged for inclusion were joined in an initial full model of colony-scale WS occurrence. Variable selection for the full model proceeded using the same guidelines applied to hypothesis group models, with the addition of two caveats. First, if two variables were functionally similar (example: both describe short-term warming) then the variable with the highest standard error (or lowest Z score) was removed. Second, if two competing variables performed similarly, the variable that would be easiest to attain at large scales (example: does not require prior in situ site history) was retained. After this process was used to arrive at a full model that included environmental variables, these steps were repeated to include variables from hypothesis groups describing concurrent or past biological covariates such as gastropod or damselfish predation (Supplementary Figure 6). A third round of variable selection using the above criteria was applied to this intermediate full model, reducing the set of WS covariates to those found in the final model specification.
The covariate prior winter condition exhibited sensitivity to the random selection of validation data. To estimate the range of estimated effects for prior winter condition, 100 additional random 75% partitions of the consensus dataset were generated, and the final model specification fit to each data set. After each model fit, estimates for all fixed effects and model conditional R2 were stored for later summarization.
Model Testing and Validation
After model selection exercises, model performance on reserved out-of-sample coral surveys (n = 7,173) was assessed on criteria of model specificity (true negative), sensitivity (true positive), and balanced accuracy. Model performance was measured as the conditional R2 (variance explained by fixed and random effects combined) of the model when fitted to the reserved dataset and calculated using the function r.squaredGLMM() in the R package MuMIn (Bartoń, 2020). It should be noted that predictions from binomial models, including the Bernoulli, are probabilities and require the use of a probability decision rule. This decision rule determines the model-estimated probability of WS required to classify an observation as disease-positive. While not applied here, tuning of probability decision rules using localized data can allow improved model predictions. Model specificity and sensitivity were calculated by generating predictions of WS presence for each colony-scale observation in the reserved data using the predict() function in the R package lme4 (Bates et al., 2015) and applying a pre-defined probability decision rule. For each colony-scale observation, predicted WS outcome was compared to true WS occurrence and categorized as a correct or incorrect classification. This process was repeated 1000 times for the entire training dataset at 1000 probability cutoff decision rule steps equally spaced between 0.01 and 0.99 to estimate model classification rates.
In addition, to gain a broader understanding of model performance across a range of probability decision rules and at the transect scale, 1000 independent realizations of predicted WS outcome were generated for each colony surveyed in the reserved dataset using probability decision rules randomly sampled from a uniform distribution ranging from 0 to 1. These data were summarized at the transect scale to assess performance of the colony-scale model when predicting transect-level trends in WS occurrence through time without the use of an informed probability decision rule.
Results
After variable selection exercises, a total of seven fixed effects remained in the final fitted model trained on 21,514 coral health observations across 13 coral species and 7 years of data (Table 3). The final model fit featured a marginal R2 (R2m, fit of fixed effects only) of 13.9% and a conditional R2 (R2c, fit of fixed plus random effects) of 41.9%. Overall model random effects accounted for approximately 28% of variation in WS cases observed. Of these random effects the most variation in WS was attributed to coral species (∼70%), followed by the coral community (transect) (∼28%), survey site (∼1%), and year (<1%). Among the seven fixed effects included in the final model all were highly significant predictors of WS except for prior winter condition. The significance of prior winter condition as a predictor of WS was found to be sensitive to the random selection of data for the data split, despite training data retaining the proportionality of WS occurrence in the complete dataset. Additional model fits on 100 random splits of the complete dataset (described in Variable selection and random effects specification) determined that a small number of WS cases occurred in the dataset for which prior winter condition was a strongly positive predictor (Figure 3).
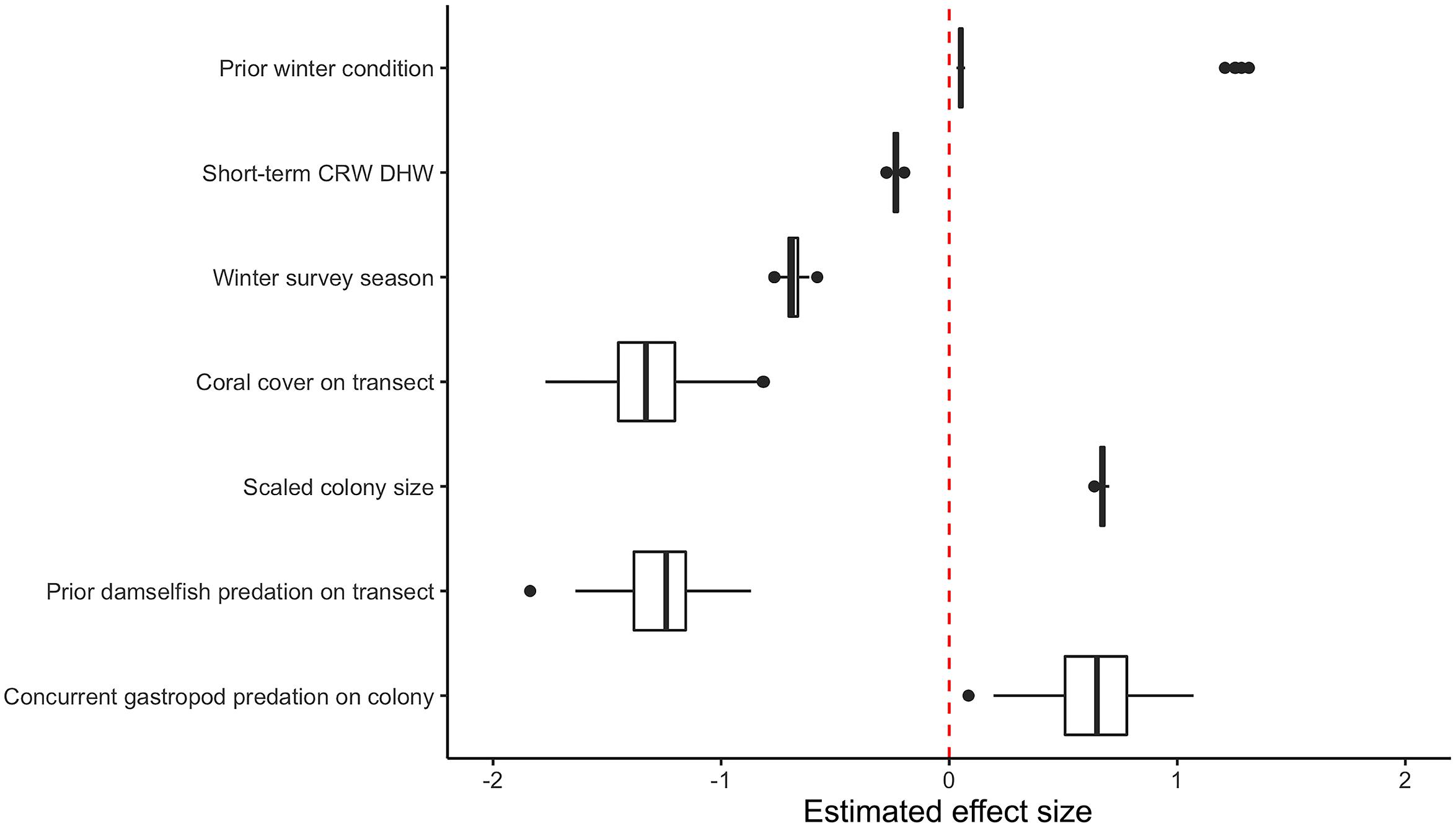
Figure 3. Boxplot of model fixed effect estimates after fitting on 100 random subsets of original consensus dataset. Two distinct populations are visible for the estimated effect size of prior winter condition.
As its inclusion would not significantly impact model performance for WS cases outside of this small group, prior winter condition remained in the model to aid prediction of WS where this driver is important. This exercise of additional model fits also determined that all other WS predictors included were robust to any substantial biases imparted by the data partitioning process (Figure 3). When summarized at the transect level across all sites, colony-scale predictions of WS with randomized decision rule values featured a fit of approximately 58% in training data (75% of available data) and 47% in model testing data (reserved 25% of available data). In both cases the model tended to underestimate when WS occurrence was very high (Figure 4).
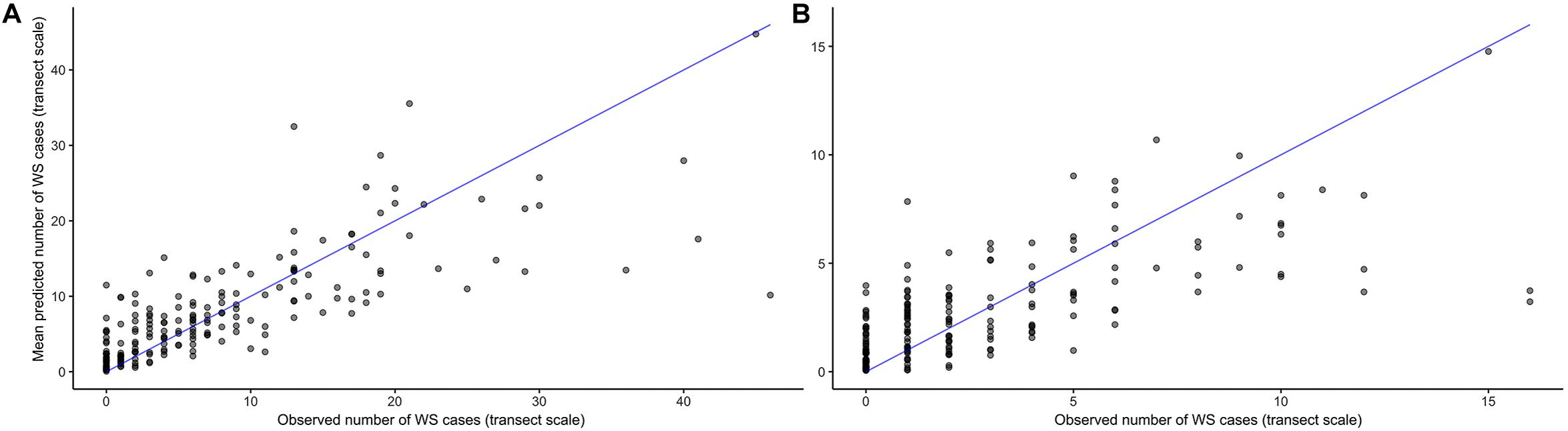
Figure 4. Agreement between observed and predicted White Syndrome cases at the transect scale using 1000 random probability decision rule values for in-sample training data (A), and out-of-sample testing data (B). Model predictions tend to underestimate where White Syndrome occurrence is very high.
Model Interpretation
Factors persisting throughout variable selection and found to be significant in the final fitted model represent key hypotheses on the importance of both biological and environmental drivers of WS.
Colonies surveyed in winter were significantly less likely to be observed with WS compared to those surveyed during summer, and this trend was most evident in sites dominated by Porites cylindrica and Acropora cf. pulchra. Occurrence of WS generally decreased with increasing coral cover, though this relationship varied by species and at the community level depending on which coral species was predominant. Coral colony size was found to be the most reliable predictor of WS risk for all species: colonies larger than average for each species experienced greatly increased WS risk (Figure 5 and Supplementary Figure 7). Increased short-term heat stress (measured as DHW) was consistently associated with decreased probability of WS (Figure 6 and Supplementary Figure 8) across coral species, whilst warmer prior winter conditions were strongly associated with increased WS in a limited number of observations. Increased prevalence of recent damselfish predation on transect within the past year resulted in decreased risk of WS at the colony-scale, whereas concurrent gastropod predation on a colony was strongly associated with increased likelihood that WS was observed on the same colony.
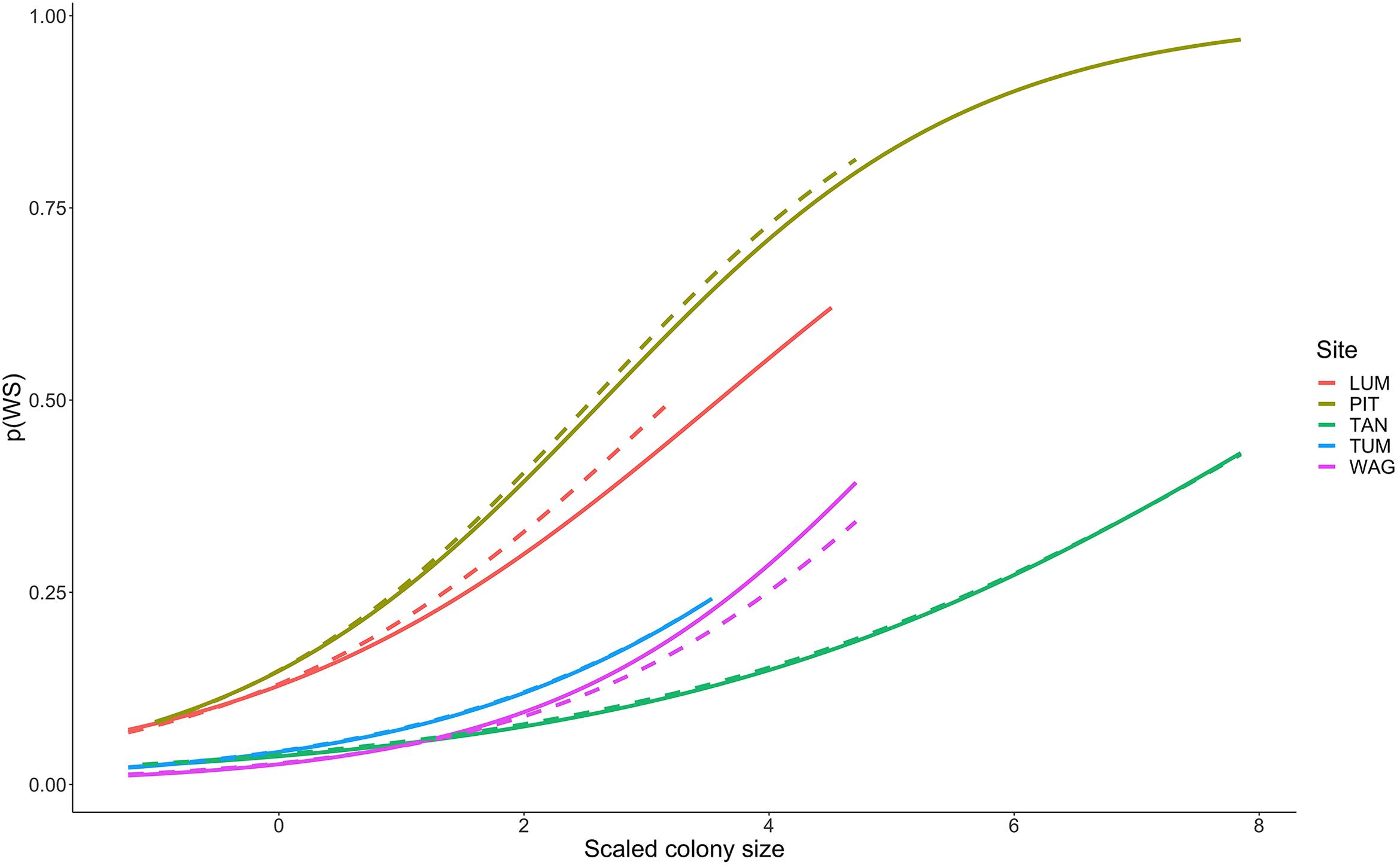
Figure 5. Increasing probability of White Syndrome with increased scaled colony size. Solid lines represent predicted White Syndrome probability on training data, dashed lines represent predictions for reserved data. Species-level plots in Supplementary Figure 7.
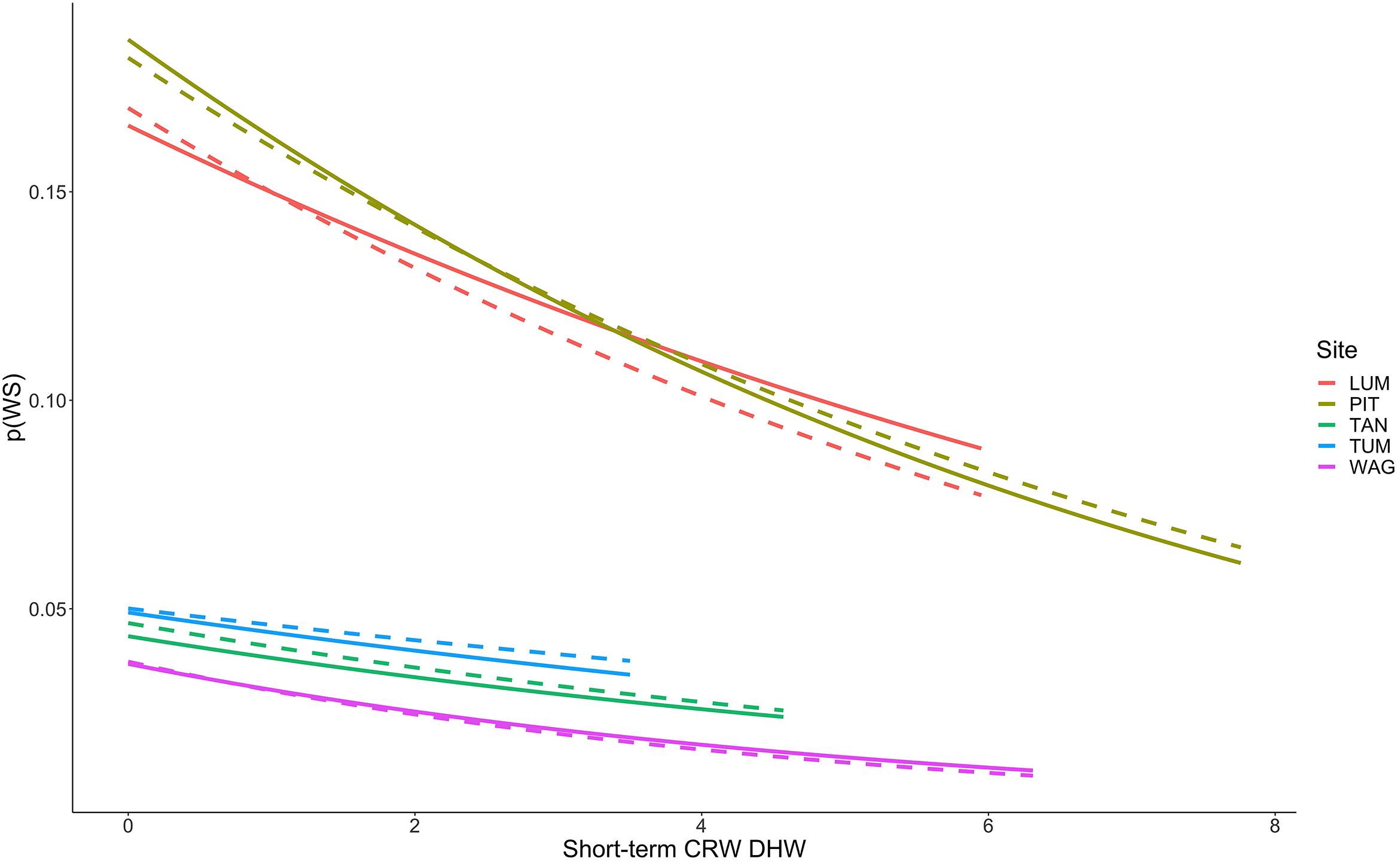
Figure 6. Decreasing probability of White Syndrome with increased Degree Heating Week values. Solid lines represent predicted White Syndrome probability on training data, dashed lines represent predictions for reserved data. Species-level plots in Supplementary Figure 8.
Several hypothesis groups (Table 2) were not represented in the final model after variable selection, suggesting limited or no contribution to WS occurrences observed in this dataset of non-outbreak WS occurrence. Neither diversity of coral species on a transect, nor proportion of susceptible colonies on a transect, were significant predictors of WS risk; coral size was the only significant driver from Hypothesis Group 2. There was no evidence that prior bleaching, estimated bleaching susceptibility, or the estimated impact of prior heat stress (Bleaching Pressure) increased WS occurrence (Hypothesis Group 3). Long-term heat stress (Hypothesis Group 6), such as prior maximum temperature anomalies or Hot Snap values, also showed no significant contribution to the probability of WS at the time of a survey. Ocean color, including estimates of acute or chronic stress from Chl-a and turbidity measured as Kd (490), were not included in the final model as reliable predictors of WS (Hypothesis Groups 7 and 8). While concurrent gastropod predation (Hypothesis Group 9) and prior damselfish predation (Hypothesis group 10) were selected as factors, no measure of past or present macroalgal overgrowth (Hypothesis Group 11) persisted through the variable selection process.
Model Performance
When predicting WS occurrence in the absence of an informed probability decision rule, the final model tended to underestimate where WS cases on a transect (20 m2) exceeded six in reserved data or 20 in training data (Figure 7). Model performance was maximized in communities dominated by Porites cylindrica (sites PIT or LUM) and communities featuring a mix of Acropora cf. pulchra with Porites cylindrica and Pocillopora damicornis (site TUM) – this result was expected based on the large proportion of WS cases affecting these species. Predictions from 1000 randomly selected probability decision rules provided reliable estimates of WS throughout much of the training and testing datasets (Figure 7). Improved detection of spikes in WS frequency could be achieved by tuning prediction outcomes through the use of an informed probability decision rule, at the cost of increased model sensitivity and false positives. Analysis of model specificity across 1000 probability decision rules and sensitivity indicated that overall accuracy was maximized at a decision rule of p(WS) = 0.081, where specificity was 0.72 and sensitivity was 0.76. Visual assessment of model fit suggested that a decision rule of p(WS) = 0.25 provided reliable detection of WS spikes in Porites cylindrica or Acropora cf. pulchra-dominated communities at the cost of over-predicting low-frequency WS (Supplementary Figure 9). However, in all cases the model tended to under-predict a sharp increase in WS occurrence at Tumon in mid-to-late 2016. Finally, while prior damselfish predation appears ecologically valuable to the prediction of WS, removal of this driver for WS prediction without prior site visits is possible. A model fit without prior damselfish predation prevalence featured an R2m of 0.12 and an overall R2c of 0.40, approximately a 2% reduction in overall fit.
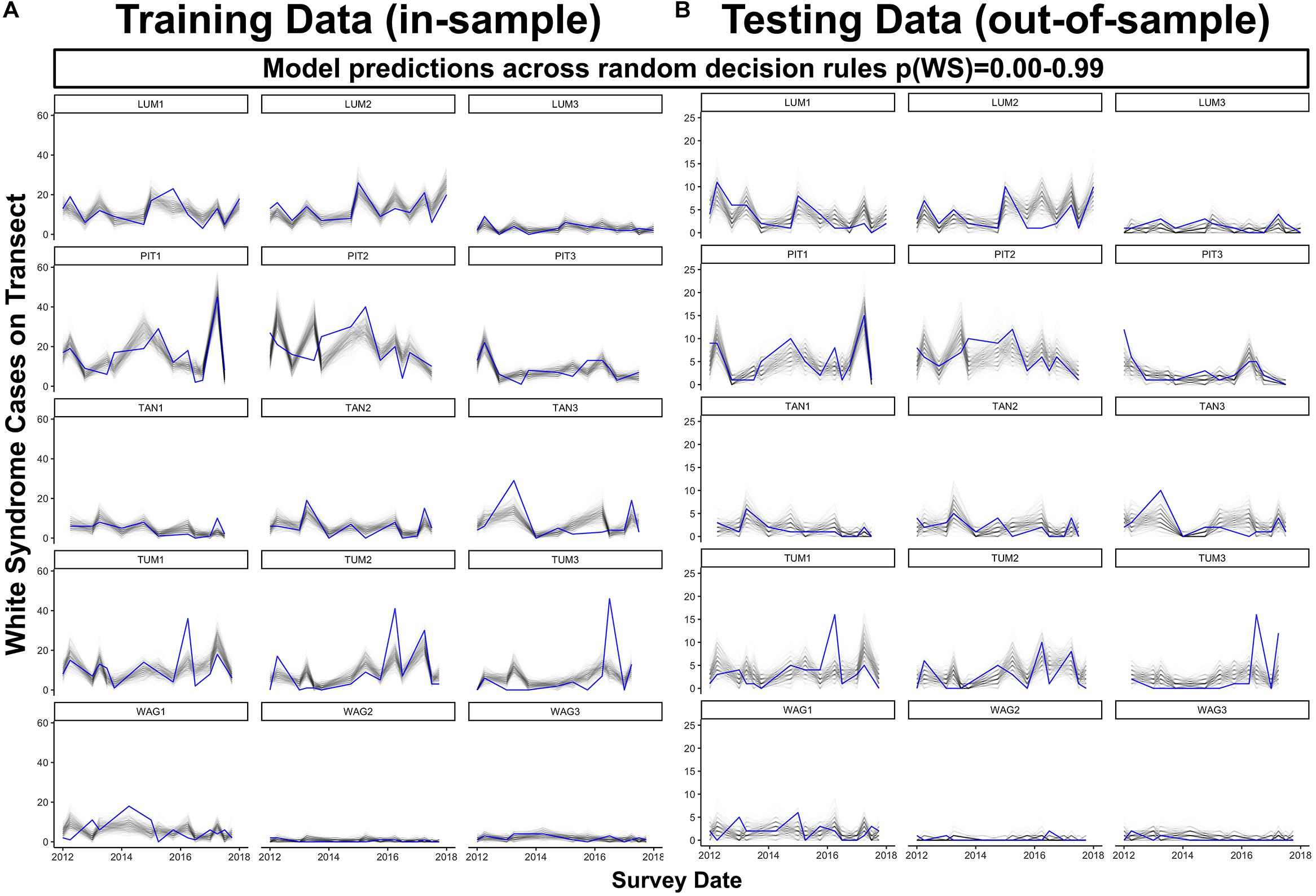
Figure 7. Predicted (black) and observed (blue) total WS cases per transect through time for both model training data (A), in-sample) and reserved model testing data (B), out-of-sample) across 1000 colony-scale model realizations using decision rules randomly selected from a uniform distribution ranging from 0.00 to 0.99. For plotting clarity, 250 of the total 1000 model realizations are plotted.
Discussion
Coral disease is difficult to predict, and many of the environmental and ecological factors that contribute to its occurrence are poorly specified. Laboratory studies alone cannot capture the full complexity and range of factors that contribute to coral disease in natural settings, and often are best combined with in situ experimentation or monitoring strategies (Boyett et al., 2007; Sokolow, 2009; Vega Thurber et al., 2014). Effective management of coral disease, and actions toward prevention of outbreaks, requires informed predictions of when and where coral diseases will arise. Current understanding of conditions associated with coral disease is largely based on stratified random surveys, which do not track coral communities affected by disease through time, and are unable to observe local stress accumulation in situ (Winston et al., 2019). Stratified random strategies are useful for the detection of coral disease or host stress responses such as bleaching over large areas but inferences from stratified random surveys are limited by the lack of colony- and transect- scale prior observations that can identify critical drivers that precede disease onset. Supplementing stratified random data with evidence from longitudinal studies allows models of coral disease to consider which prior conditions may increase or decrease future disease risk.
Seasonality of White Syndromes
Like many tropical islands, Guam is well-known for seasonality that features cool, dry winter conditions and warm, wet summer conditions. Here, a significant effect of season suggests that coral colonies surveyed in winter were less likely to be observed with active WS lesions. Winter reductions in WS were most evident in PIT and TUM, both of which are dominated by either Porites cylindrica or Acropora cf. pulchra. At LUM, which also features Porites cylindrica, seasonal effects were less apparent. In TAN, where coral communities are more diverse and feature high abundance of Leptastraea purpurea and Psammocora spp., WS occurrence is higher in winter than summer. While we have confidence in the model results indicating that WS risk is reduced during winter months, we also caution that these results may be specific to those species that contributed most to model training data (Porites cylindrica and Acropora cf. pulchra). For affected species, decreased WS risk during Winter may be due to hypothesized associations between colder temperatures and decreased pathogen load in the water column or coral surface mucus layer (Heron et al., 2010; Mao-Jones et al., 2010). Exploring potential mechanisms between winter temperatures and disease risk will be an important next step to inform predictive models of coral disease such as WS under warming climates.
Coral Cover, Diversity, and Colony Size
Coral colony size was the most reliable predictor of WS risk. Regardless of coral species or the site where a survey was conducted, corals larger than average for their species were more likely to have active WS lesions - this finding is unique for its uniformity across 13 coral species. Coral colony size has long been considered a factor in the susceptibility of corals to bleaching and disease and has well-documented effects on the ecology of coral reefs (Sammarco, 1980; McCook et al., 2001; Roff et al., 2006; Albright et al., 2010; Jokiel et al., 2015; Doropoulos et al., 2016; Caldwell et al., 2020). Prior research has found substantially increased disease risk with increases in host colony size (Caldwell et al., 2018), and researchers have posed both age- and size-related hypotheses to explain these patterns. Age related hypotheses suggest either that older corals senesce, resulting in reduced immune function or that corals experience a process of microbial winnowing, wherein the host microbiome becomes less diverse and more specialized to the local environment over time (van Oppen and Blackall, 2019). Such decreases in diversity have previously been associated with increased host susceptibility to disease (Pollock et al., 2019; van Oppen and Blackall, 2019). Size-related explanations suggest that larger colonies have an increased surface area – and, therefore, increased susceptibility - for injury or pathogen attack. Our findings are more consistent with the hypothesis of age-dependence, given the similarity of effect across species even when the effect of size was normalized by species. That is, this effect was consistent between species that varied greatly in maximum size.
Overall – and contrary to prior studies (Bruno et al., Heron et al) – WS decreased with increasing coral cover; however, this effect varied by site and species (Supplementary Figure 10). For example, while Porites cylindrica showed reduced WS risk with increased coral cover at all sites where it occurred, Acropora cf. pulchra exhibited decreased disease risk at three sites (LUM, WAG, TAN) and a marked increase with coral cover at site TUM. Similarly, Pocillopora damicornis experienced reduced WS risk with increasing coral cover at all sites except for TAN, where WS risk increased. This variation among sites and species could be driven by the differences in relative contribution of different taxa to coral cover by site, or it may highlight that diseases classified as WS here may, in fact, be diseases with distinct causation or host-response between taxa. Our results indicate that the effect of coral cover on disease risk (negative in this study, and positive in some prior studies) is not consistent and that coral cover as an indicator of reef health must be considered alongside other site or transect-level variables. Diversity of coral species on a transect, another common metric of reef health, was not found to significantly influence WS risk in any model.
Prior Bleaching and Heat Susceptibility
Coral bleaching is a response to accumulated oxidative stress, and bleaching is frequently posited as a precursor to coral diseases such as WS. In at least one longitudinal study conducted during the 2005 Caribbean bleaching event a positive correlation was found between incidence of White Plague and prior bleaching at the colony-scale for Montastraea faveolata (Brandt and McManus, 2009), but explicit evidence linking these phenomena is lacking (Ban et al., 2013). Short-term longitudinal evidence from northeast Australia (Beaver Reef) suggests that moderate bleaching and WS may combine to produce increased tissue loss, but that these effects are less apparent in severely bleached colonies where mortality is likely regardless of WS presence or absence (Brodnicke et al., 2019). Since the survey data used in this study revisited fixed transects rather than individually marked colonies, we are unable to determine if prior bleaching at the colony-scale is predictive of WS on the same colony. However, this study did allow us to estimate WS risk at the colony-scale in response to prior bleaching prevalence observed on the same transect. We observed no significant effect of prior bleaching on the probability of a colony having WS, regardless of whether the bleaching prevalence value used represented a maximum within the past year or was weighted by these maxima. It is well known that coral species respond differently to heat stress, and we attempted to incorporate this knowledge by testing both the Bleaching Response Index (BRI) (Swain et al., 2016) and the BRI weighted by maximum thermal stress (DHW) experienced by a colony within 1 year prior to a survey. None of these variables were significant predictors of WS risk at the colony-scale. Following these results, we propose that the physiological stress of bleaching on its own is not a sufficient predictor for the onset of WS and encourage further study on the mechanisms of coral disease onset to improve predictive models. We also highlight that caution should be used when attempting to generate disease predictions based the expectation that disease follows bleaching, as these assumptions may lead to erroneously high estimates of coral disease.
Winter Warming
Winter warming in the form of prior winter condition is a highly significant and strong predictor of WS for a small population of cases captured in our survey data. Under further investigation, it does not appear that these cases belong to a particular coral species or a particular survey site. It is possible there was a unique combination of conditions that made WS occurrences highly correlated with prior winter condition in a subset of surveys, but we did not find evidence to support this hypothesis. Instead, we suggest that the disease classified as WS in these cases, while visually similar to other WS recorded, may have been a different disease or type of WS that responds to different drivers. Appearance and progression patterns of WS lesions varied among taxa (as described in Methods) and, where monitored, progression rates of tissue loss have also been shown to vary as well (Lozada-Misa et al., 2015). These findings support recent conclusions that identification of coral diseases by visual signs alone may be insufficient (Caldwell et al., 2018), reinforcing the need for additional methods such as histology to allow comprehensive disease description (Work and Aeby, 2006) and more accurate field identification.
Long-Term Heat Stress
Evidence exists that corals may remain compromised for several months or more following bleaching or thermal stress events (Thomas and Palumbi, 2017), and a history of thermal stress has been found to increase the risk of diseases similar to WS in Caribbean coral communities (Randall et al., 2014). We hypothesized that current WS occurrence may be associated with the maximum heat stress a colony may have experienced within the past year prior to the date it was surveyed. The longitudinal nature of our survey data allowed this hypothesis to be tested on four commonly used metrics of thermal stress from CRW: SST Anomaly, Hotspot, DHW, and Hot Snap. None of these variables persisted to the final model as reliable predictors of colony-scale WS in Guam. While prior heat stress can certainly impact coral health, we found no evidence at our survey locations that annual maxima prior to a survey were informative drivers of observed WS. While this appears to conflict with the significant effect of season in the model (summer disease occurrence being greater than winter), instead it simply demonstrates that these more specific thermal stress metrics do not provide additional explanatory power, compared to season alone. In addition, season captures more underlying variation in precipitation and other factors as well as temperature. Further research is needed to determine whether these findings represent a recovery of the coral host from prior heat stress events, or if background disease levels and heat stress are not as directly linked as previously thought.
Short-Term Heat Stress
Thermal stress such as DHW is widely considered a strong predictor of coral bleaching, and coral bleaching from thermal stress is frequently considered antecedent to many coral diseases such as White Syndrome by increasing coral host susceptibility to disease (Mydlarz et al., 2009; Ruiz-Moreno et al., 2012; Pollock et al., 2019). We hypothesized that short-term heat stress would be associated with increased WS risk at the colony-scale. Of those variables tested as part of this hypothesis group, only Degree Heating Weeks (DHW) persisted to the final model as a reliable predictor of WS. DHW is zero during winter months, when we observed reduced WS occurrence, and represents accumulated heat stress over a 12-week period prior to a coral survey date. Counterintuitively, however, we observed decreased occurrence of WS with increased values of DHW, and these results were consistent across coral species regardless of site with the exception of Leptastraea purpurea, Acropora aspera, and Acropora muricata (Figure 6). Probability of WS on L. purpurea showed no trend with DHW, whereas Acropora aspera and A. muricata both demonstrated increased WS risk over the limited range of DHW under which they were observed (approximately 0 to 1 DHW). Broadly, these findings represent a departure from traditionally hypothesized relationships wherein coral disease increases with prior bleaching (discussed above) or heat stress. One explanation for these results could be high bleaching and mortality after periods of elevated DHW, resulting in fewer colonies on transect available to express WS. No clear relationship is evident in these data to support this hypothesis as (i) total transect area remained constant throughout the study and (ii) the total number of colonies surveyed on each transect showed no relationship with increasing DHW values. Another explanation for these results is that satellite-based measures, such as DHW used here, assess temperature stress at a spatial scale much larger than that of individual colonies and do not necessarily assess the true temperature experienced by coral colonies in reef microhabitats. CRW DHW values at the five sites ranged from 0 to 10.28 (mean 0.33)°C-weeks in the raw CRW data, and from 0 to 7.76 (mean 0.81)°C-weeks in the consensus model-training data. From 2009 to 2018 in raw CRW data the DHW threshold of 4°C-weeks, at which significant coral bleaching can be expected, was only crossed three times – once in late 2013, late 2016, and late 2017. While bleaching was observed outside of these time periods as well, severe bleaching expected at DHW above 4°C-weeks was observed in each of these years (Raymundo et al., 2019), suggesting that CRW DHW values in Guam adequately reflect thermal stress experienced by corals at the survey locations. These findings suggest further investigation is needed into alternative, perhaps more mechanistic explanations for the relationship observed here between short-term heat stress and WS.
If the relationship observed here between WS and DHW is not an artifact of data handling or the reduced temperature variability of tropical regions, then it suggests that the link between short-term thermal stress and White Syndromes is not as expected. All of these hypotheses are valuable points of consideration for improved prediction and management of coral disease in tropical regions. In light of this we suggest further research on the potential influence of increased temperatures on pathogen load in the water column, how thermal stress affects coral susceptibility to disease as well as pathogen virulence, and the role of temperature microhabitats not captured by satellite metrics as refugia from, or sources of, disease. Based on our results we recommend caution when statistically linking increased disease directly to either increased bleaching or the thermal stress that may precede bleaching until these mechanisms are better resolved.
Short and Long-Term Ocean Color
Satellite ocean color, including metrics of Chl-a concentration or turbidity approximated by Kd (490), have infrequently been included in predictive models of coral disease despite their established utility in lakes and open oceans (McClain, 2009). Limitations do exist when using these measures in coastal near-shore environments where bottom reflectance can skew data and exacerbate issues of low data recovery due to cloud cover. The methods developed above and in Geiger et al., unpublished represent a promising and practical step forward by addressing issues of low data density in near-shore environments. Using both short-term maximums and long-term means of ocean color metrics, we hypothesized that increased Chl-a or turbidity would be associated with an increase in coral disease. While clear differences in ocean color were apparent between some survey sites, no relationship between WS and short- or long-term ocean color metrics was found. These results may suggest that WS in Guam occurs through mechanisms largely independent of water quality. Alternatively, the coarse spatial scale of the rosette used to spatially summarize VIIRS ocean color data (approximately 8.25 km) could have led to poor differentiation between survey locations. Of equal importance could be that the technique used here aggregating ocean color data to 1-week time periods for the benefit of increased data density may have also resulted in poor detection of acute stressors such as short-term runoff events common to high islands. Given the documented in situ and experimental relationships between nutrient concentration or sedimentation and increased coral disease (Bruno et al., 2003; Redding et al., 2013; Pollock et al., 2014; Vega Thurber et al., 2014), we feel satellite ocean color measures may still be of great use and encourage further exploration of these methods to better understand the link between water quality (as measured by ocean color) and coral disease.
Gastropod Predation
Corallivorous gastropods have been suggested as direct vectors of coral disease and are generally regarded as damaging to coral health (Antonius and Riegl, 1998; Nicolet et al., 2013). We hypothesized that gastropod predation at the colony or community (transect) scale is associated with an increase in WS occurrence, and this study supported this hypothesis: we found that colonies with evidence of gastropod predation at the time of survey were significantly more likely to also exhibit active WS lesions. Prevalence of gastropod predation at the transect scale at the time of survey, or in the past year, were not significantly associated with colony-scale WS occurrence. Importantly, while these findings reinforce that there may be a link between gastropod predation and coral diseases such as WS (perhaps through vectoring of pathogens), they do not suggest a causal relationship without further experimentation. The role of gastropod predation may differ by disease, or gastropods may be responding to the presence of necrotic tissue on the coral surface and largely inconsequential to the spread of disease. Alternatively, consumption of infected necrotic tissues by gastropods may lead to reduced disease spread if gastropod movement among colonies is low. A neutral role of gastropod predation is supported by the lack of a relationship between prior gastropod predation prevalence and observed WS frequency. Additional research and manipulative experiments are required to better understand the role of gastropods in coral health.
Damselfish Predation (Farming Behavior)
Damselfish farming is unique for its easy identification through distinctive feeding scars, which characterize the removal of tissue and underlying coral skeleton to create substrate for algal colonization, in contrast to butterflyfish feeding scars, which remove individual polyps without damaging the coral skeleton (Raymundo et al., 2008). It has previously been suggested that even limited removal of tissue or skeleton may result in reduced coral health and fitness (Rotjan and Lewis, 2008). While butterflyfish have been suggested as a potential vector of coral disease, relatively little is known about the role of damselfish to disease risk, especially those which may persist on or near corals to farm algae (Raymundo et al., 2008, 2009; Casey et al., 2015). In addition, the presence of normal levels of damselfish farming may simply be an indicator of a healthy reef community. Our results found that increased prior transect-level prevalence of damselfish farming within the past year significantly associated with decreased WS at the colony-scale. Concurrent damselfish farming at the colony or transect scale did not persist through variable selection. These results suggest that damselfish farming behavior may play an important role in the early prevention of coral disease or maintenance of coral health. Importantly, damselfish farming within the year prior to a coral survey is the only predictor in this model that requires longitudinal in situ knowledge of a survey site. We suggest closer inspection of the potential mitigating or inhibitory role damselfish farming behavior may have on coral diseases such as WS, such as whether they act to remove diseased tissue to establish new algae farms, ultimately reducing local pathogen load.
Macroalgal Overgrowth
It is well documented that many coral reefs are in decline, experiencing phase shifts toward algal-dominated states, and that habitats with high concentrations of algal exudates also support a high load of potential pathogens (Ostrander et al., 2000; Szmant, 2002; Adam et al., 2011; D’Angelo and Wiedenmann, 2014; Casey et al., 2015; Bourne et al., 2016). We hypothesized that increased macroalgal overgrowth at the colony scale, or its increased prevalence across a transect, would indicate compromised coral health and be associated with increased disease. No evidence was found to support this hypothesis for the occurrence of WS in Guam, and no variable describing macroalgal overgrowth persisted through variable selection. These results support the conclusion that typical indicators of reef decline, such as prior heat stress and macroalgal overgrowth, may not themselves be reliable predictors of WS at the colony-scale, or at least do not represent proximate causal relationships. To accurately predict coral diseases such as WS at the colony-scale we encourage a better understanding of the specific mechanisms behind the onset of coral disease within degraded reef habitats.
Model Performance at Transect Scale
This study demonstrates the development of a colony-scale model of coral disease that can predict both colony-scale and transect-scale disease occurrence with relative accuracy. Model performance in all datasets was maximized when predicting WS in coral communities, which featured a large proportion of Porites cylindrica, as these communities also contributed the most WS cases toward model training. The stochastic realizations of the model tended to both underestimate and overestimate WS counts in coral communities that were dominated by Acropora cf. pulchra. Future data collection in Guam or other Pacific high islands could be targeted to better capture WS occurring on Acropora cf. pulchra to improve model performance. Alternatively, careful selection of probability cutoff decision rules by managers implementing this model could guard against systematic over or underprediction of White Syndrome, at the cost of a more rigid model interpretation versus a mean of stochastic realizations. While balanced colony-scale accuracy was maximized at a probability cutoff of p(WS) = 0.076, we observed more reliable model predictions at the transect-scale without overprediction in Acropora cf. pulchra dominated communities at a more conservative probability cutoff of p(WS) = 0.25. These findings advance our current understanding of coral disease forecasting by suggesting that disease forecasts should use colony-scale data if available, such as colony size, and aim to account for community-level ecological factors such as coral cover and damselfish predation prevalence when estimating disease responses.
Results in Context
While the model presented here represents only five sites in Guam, it provides compelling evidence that diseases visually presenting as White Syndrome are driven by both past and present environmental and ecological factors at multiple scales. Importantly, this model was based on mostly low-prevalence WS data with limited high-prevalence observations, and drivers in outbreak scenarios may vary from those found to be significant here. Rather than developing a model of WS separately for each species in the dataset, we have demonstrated that a species-generalized model of WS is capable of predicting low disease occurrences across a range of coral communities and may be adjusted to require only a single time point of in situ data. Jointly fitting multiple species means that the model is not tightly fit for any single coral species but represents a valuable step towards generalized coral disease models that might be used to estimate coral disease in remote reefs or at large spatial scales using predictions of coral cover and colony size based on limited prior site data. Two primary limitations of this study include the largely undescribed etiology of White Syndromes, and the visual similarity of these conditions across coral species. White Syndrome lesions on colonies of different coral species may represent different diseases altogether. Additionally, differences in WS etiology across coral species may represent differences in driving factors for a shared pathogen. The inability to distinguish diseases within White Syndromes in situ limits both survey data, modeling efforts, and inference. We investigated the suite of White Syndrome conditions occurring across host species with the goal of assessing disease predictors in general. This exercise has highlighted that factors commonly associated with coral diseases, namely thermal stress, Chl-a concentration, and prior bleaching may be unreliable predictors of future disease risk and, in some cases, reduce the likelihood of WS. In addition, this study reinforces that coral colony size, and perhaps age-related factors, require further study as drivers of WS and perhaps other coral diseases. These findings emphasize the variety of factors associated with WS, the value of longitudinal data for disentangling them, and the challenging nature of modeling coral disease at large spatial scales.
Data Availability Statement
The raw data supporting the conclusions of this article will be made available by the authors, without undue reservation.
Author Contributions
AG led analysis of the data with consultation by MJD, JMC, SFH, EG, and LJR. SFH and EG provided satellite temperature and ocean color data for use in analysis. LJR provided coral survey data and site background information for use in analysis and writing. All authors contributed directly to writing and editing of the manuscript.
Funding
Ongoing survey work in Guam is funded under NOAA grant NA15NOS4820039. Analysis was supported by the NASA Ecological Forecasting program grant NNX17AI21G. This is contribution #1831 from the Hawai‘i Institute of Marine Biology and #11170 from the School of Oceanography and Earth Science and Technology.
Conflict of Interest
Frontiers Media SA remains neutral with regard to jurisdictional claims in published maps and institutional affiliations.
The authors declare that the research was conducted in the absence of any commercial or financial relationships that could be construed as a potential conflict of interest.
Acknowledgments
We would like to thank the following people for their help in collecting the survey data: Roxanna Myers, Paula Lozada-Misa, Roxy Diaz, Valeri Lapacek, and Ashton Williams. We would also like to thank Dr. Jake Ferguson at the University of Hawai‘i at Mānoa for his insight on variable selection.
Supplementary Material
The Supplementary Material for this article can be found online at: https://www.frontiersin.org/articles/10.3389/fmars.2020.601469/full#supplementary-material
Supplementary Figure 1 | Dot plot of species colony counts and transect composition through time for all recorded species. Dot size indicates number of colonies observed at each time point and survey location.
Supplementary Figure 2 | Dot plot of species colony counts and transect composition through time for modeled coral species. Dot size indicates number of colonies observed at each time point and survey location.
Supplementary Figure 3 | Dot plot of White Syndrome (WS) prevalence through time for modeled coral species. Dot size indicates WS prevalence within each species at each time point and survey location.
Supplementary Figure 4 | Reference photographs of coral species used in model analysis (also see Supplementary Table 1).
Supplementary Figure 5 | Reference photographs of characteristic lesions from several coral species observed, representing typical variation in tissue loss classified as White Syndrome.
Supplementary Figure 6 | Depiction of the variable selection process where red coloration indicates rejection of a variable due to violation of variable selection criteria and green indicates persistence of a variable to the next step of variable selection. Diamonds indicate decision points for each variable tested.
Supplementary Figure 7 | Plots indicating relationship between probability of White Syndrome with increased scaled colony size across sites and species modeled. Notably, most species demonstrate increased risk of WS as size increases from their species-specific average.
Supplementary Figure 8 | Plots indicating association between short-term heat stress (measured as CRW DHW) and probability of White Syndrome across sites and species modeled. For species where decreasing relationships between p(WS) and DHW are found, these trends appear consistent across study sites.
Supplementary Figure 9 | Predicted (black) and observed (blue) total WS cases per transect through time for both model training data (left, in-sample), in-sample) and reserved model testing data (right, out-of-sample) based on a p(WS) = 0.25 probability decision rule.
Supplementary Figure 10 | The influence ofcoral cover on colony-scale probability of White Syndrome varies by site (left) and by species (right). Left panel: Solid lines represent predicted White Syndrome probability on training data, dashed lines represent predictions for reserved data.
Supplementary Table 1 | Table of species codes used in survey data and corresponding scientific names. Species appearing in the consensus dataset have had their code and scientific name bolded.
References
Adam, T. C., Schmitt, R. J., Holbrook, S. J., Brooks, A. J., Edmunds, P. J., Carpenter, R. C., et al. (2011). Herbivory, connectivity, and ecosystem resilience: response of a coral reef to a large-scale perturbation. PLoS One 6:e0023717. doi: 10.1371/journal.pone.0023717
Aeby, G. S., Williams, G. J., Franklin, E. C., Haapkyla, J., Harvell, C. D., Neale, S., et al. (2011a). Growth anomalies on the coral genera Acropora and Porites are strongly associated with host density and human population size across the Indo-Pacific. PLoS One 6:e16887. doi: 10.1371/journal.pone.0016887
Aeby, G. S., Williams, G. J., Franklin, E. C., Kenyon, J., Cox, E. F., Coles, S., et al. (2011b). Patterns of coral disease across the Hawaiian Archipelago: relating disease to environment. PLoS One 6:e20370. doi: 10.1371/journal.pone.0020370
Albright, R., Mason, B., Miller, M. W., and Langdon, C. (2010). Ocean acidification compromises recruitment success of the threatened Caribbean coral Acropora palmata. Proc. Natl. Acad. Sci. U.S.A. 107, 20400–20404. doi: 10.1073/pnas.1007273107/-/DCSupplemental.www.pnas.org/cgi//10.1073/pnas.1007273107
Antonius, A., and Riegl, B. (1998). Coral diseases and Drupella cornus invasion in the Red Sea. Coral Reefs 17:48. doi: 10.1007/s003380050093
Ban, S. S., Graham, N. A. J., and Connolly, S. R. (2013). Relationships between temperature, bleaching and white syndrome on the Great Barrier Reef. Coral Reefs 32, 1–12. doi: 10.1007/s00338-012-0944-6
Bates, D., Mächler, M., Bolker, B. M., and Walker, S. C. (2015). Fitting linear mixed-effects models using lme4. J. Stat. Softw. 67, 1–48. doi: 10.18637/jss.v067.i01
Bourne, D. G., Ainsworth, T. D., Pollock, F. J., and Willis, B. L. (2015). Towards a better understanding of white syndromes and their causes on Indo-Pacific coral reefs. Coral Reefs 34, 233–242. doi: 10.1007/s00338-014-1239-x
Bourne, D. G., Morrow, K. M., and Webster, N. S. (2016). Insights into the Coral microbiome: underpinning the health and resilience of reef ecosystems. Annu. Rev. Microbiol. 70, 317–340. doi: 10.1146/annurev-micro-102215-095440
Boyett, H. V., Bourne, D. G., and Willis, B. L. (2007). Elevated temperature and light enhance progression and spread of black band disease on staghorn corals of the Great Barrier Reef. Mar. Biol. 151, 1711–1720. doi: 10.1007/s00227-006-0603-y
Brandt, M. E., and McManus, J. W. (2009). Disease incidence is related to bleaching extent in reef-building corals. Ecology 90, 2859–2867. doi: 10.1890/08-0445.1
Brandt, M. E., Smith, T. B., Correa, A. M. S., and Vega-Thurber, R. (2013). Disturbance driven colony fragmentation as a driver of a coral disease outbreak. PLoS One 8:e57164. doi: 10.1371/journal.pone.0057164
Brodnicke, O. B., Bourne, D. G., Heron, S. F., Pears, R. J., Stella, J. S., Smith, H. A., et al. (2019). Unravelling the links between heat stress, bleaching and disease: fate of tabular corals following a combined disease and bleaching event. Coral Reefs 38, 591–603. doi: 10.1007/s00338-019-01813-9
Bruno, J. F., Petes, L. E., Harvell, C. D., and Hettinger, A. (2003). Nutrient enrichment can increase the severity of coral diseases. Ecol. Lett. 6, 1056–1061. doi: 10.1046/j.1461-0248.2003.00544.x
Bruno, J. F., Selig, E. R., Casey, K. S., Page, C. A., Willis, B. L., Harvell, C. D., et al. (2007). Thermal stress and coral cover as drivers of coral disease outbreaks. PLoS Biol. 5:e124. doi: 10.1371/journal.pbio.0050124
Caldwell, J. M., Aeby, G., Heron, S. F., and Donahue, M. J. (2020). Case-control design identifies ecological drivers of endemic coral diseases. Sci. Rep. 10, 1–11. doi: 10.1038/s41598-020-59688-8
Caldwell, J. M., Donahue, M. J., and Harvell, C. D. (2018). Host size and proximity to diseased neighbours drive the spread of a coral disease outbreak in Hawai‘i. Proc. R. Soc. B Biol. Sci. 285:20172265. doi: 10.1098/rspb.2017.2265
Casey, J. M., Connolly, S. R., and Ainsworth, T. D. (2015). Coral transplantation triggers shift in microbiome and promotion of coral disease associated potential pathogens. Sci. Rep. 5:11903. doi: 10.1038/srep11903
D’Angelo, C., and Wiedenmann, J. (2014). Impacts of nutrient enrichment on coral reefs: new perspectives and implications for coastal management and reef survival. Curr. Opin. Environ. Sust. 7, 82–93. doi: 10.1016/j.cosust.2013.11.029
Descombes, P., Wisz, M. S., Leprieur, F., Parravicini, V., Heine, C., and Olsen, S. M. (2015). Forecasted coral reef decline in marine biodiversity hotspots under climate change. Glob. Change Biol. 21, 2479–2487. doi: 10.1111/gcb.12868
Doropoulos, C., Roff, G., Bozec, Y., Zupan, M., Werminghausen, J., and Mumby, P. J. (2016). Characterizing the ecological trade- offs throughout the early ontogeny of coral recruitment. Ecol. Monogr. 86, 20–44. doi: 10.1890/15-0668.1
Heron, S. F., Willis, B. L., Skirving, W. J., Mark Eakin, C., Page, C. A., Miller, I. R., et al. (2010). Summer hot snaps and winter conditions: modelling white syndrome outbreaks on great barrier reef corals. PLoS One 5:e12210. doi: 10.1371/journal.pone.0012210
Holbrook, S. J., Adam, T. C., Edmunds, P. J., Schmitt, R. J., Carpenter, R. C., Brooks, A. J., et al. (2018). Recruitment drives spatial variation in recovery rates of resilient coral reefs. Sci. Rep. 8, 1–11. doi: 10.1038/s41598-018-25414-8
Hughes, T. P., Kerry, J. T., Baird, A. H., Connolly, S. R., and Chase, T. J. (2019). Global warming impairs stock–recruitment dynamics of corals. Nature 568, 387–390. doi: 10.1038/s41586-019-1081-y
Jokiel, P., Rodgers, K. U., and Stender, Y. (2015). Assessment of Coral Settlement Distributions and Environmental Conditions. Avaliable at: https://data.nodc.noaa.gov/coris/library/NOAA/CRCP/other/grants/NA13NOS4820014/FY13-Project-8_SKohala-Coral-Assessment-final-report-April-2015-1.pdf (accessed June 1, 2018).
Kuhn, M. (2020). caret: Classication and Regression Training (p. R Package version 6.0-86). Avaliable at: https://cran.r-project.org/web/packages/caret/caret.pdf (accessed March 20, 2020).
Lamb, J. B., True, J. D., Piromvaragorn, S., and Willis, B. L. (2014). Scuba diving damage and intensity of tourist activities increases coral disease prevalence. Biol. Conserv. 178, 88–96. doi: 10.1016/j.biocon.2014.06.027
Lamb, J. B., Wenger, A. S., Devlin, M. J., Ceccarelli, D. M., Williamson, D. H., and Willis, B. L. (2016). Reserves as tools for alleviating impacts of marine disease. Philos. Trans. R. Soc. B Biol. Sci. 371. doi: 10.1098/rstb.2015.0210
Le Nohaïc, M., Ross, C. L., Cornwall, C. E., Comeau, S., Lowe, R., McCulloch, M. T., et al. (2017). Marine heatwave causes unprecedented regional mass bleaching of thermally resistant corals in northwestern Australia. Sci. Rep. 7, 1–11. doi: 10.1038/s41598-017-14794-y
Lozada-Misa, P., Kerr, A., and Raymundo, L. J. (2015). Contrasting lesion dynamics of white syndrome among the scleractinian corals Porites spp. PLoS One 10:e0129841. doi: 10.1371/journal.pone.0129841
Mao-Jones, J., Ritchie, K. B., Jones, L. E., and Ellner, S. P. (2010). How microbial community composition regulates coral disease development. PLoS Biol. 8:e1000345. doi: 10.1371/journal.pbio.1000345
McClain, C. R. (2009). A decade of satellite ocean color observations. Annu. Rev. Mar. Sci. 1, 19–42. doi: 10.1146/annurev.marine.010908.163650
McClanahan, T. R., Maina, J., and Ateweberhan, M. (2015). Regional coral responses to climate disturbances and warming is predicted by multivariate stress model and not temperature threshold metrics. Clim. Change 131, 607–620. doi: 10.1007/s10584-015-1399-x
McCook, L. J., Jompa, J., and Diaz-Pulido, G. (2001). Competition between corals and algae on coral reefs: a review of evidence and mechanisms. Coral Reefs 19, 400–417. doi: 10.1007/s003380000129
Meyer, J. L., Castellanos-Gell, J., Aeby, G. S., Häse, C. C., Ushijima, B., and Paul, V. J. (2019). Microbial community shifts associated with the ongoing stony coral tissue loss disease outbreak on the Florida reef tract. Front. Microbiol. 10:2244. doi: 10.3389/fmicb.2019.02244
Miller, J., Muller, E., Rogers, C., Waara, R., Atkinson, A., Whelan, K. R. T., et al. (2009). Coral disease following massive bleaching in 2005 causes 60% decline in coral cover on reefs in the US Virgin Islands. Coral Reefs 28, 925–937. doi: 10.1007/s00338-009-0531-7
Mydlarz, L. D., Couch, C. S., Weil, E., Smith, G., and Harvell, C. D. (2009). Immune defenses of healthy, bleached and diseased Montastraea faveolata during a natural bleaching event. Dis. Aquat. Organ. 87, 67–78. doi: 10.3354/dao02088
Myers, R. L., and Raymundo, L. J. (2009). Coral disease in micronesian reefs: a link between disease prevalence and host abundance. Dis. Aquat. Organ. 87, 97–104. doi: 10.3354/dao02139
Nicolet, K. J., Hoogenboom, M. O., Gardiner, N. M., Pratchett, M. S., and Willis, B. L. (2013). The corallivorous invertebrate Drupella aids in transmission of brown band disease on the Great Barrier Reef. Coral Reefs 32, 585–595. doi: 10.1007/s00338-013-1010-8
Ostrander, G. K., Armstrong, K. M., Knobbe, E. T., Gerace, D., and Scully, E. P. (2000). Rapid transition in the structure of a coral reef community: the effects of coral bleaching and physical disturbance. Proc. Natl. Acad. Sci. U.S.A. 97, 5297–5302. doi: 10.1073/pnas.090104897
Otaño-Cruz, A., Montañez-Acuña, A. A., Torres-López, V., Hernández-Figueroa, E. M., and Hernández-Delgado, E. A. (2017). Effects of changing weather, oceanographic conditions, and land uses on spatio-temporal variation of sedimentation dynamics along near-shore coral reefs. Front. Mar. Sci. 4:249. doi: 10.3389/fmars.2017.00249
Pollock, F. J., Lamb, J. B., Field, S. N., Heron, S. F., Schaffelke, B., Shedrawi, G., et al. (2014). Sediment and turbidity associated with offshore dredging increase coral disease prevalence on nearby reefs. PLoS One 9:e102498. doi: 10.1371/journal.pone.0102498
Pollock, F. J., Lamb, J. B., van de Water, J. A. J. M., Smith, H. A., Schaffelke, B., Willis, B. L., et al. (2019). Reduced diversity and stability of coral-associated bacterial communities and suppressed immune function precedes disease onset in corals. R. Soc. Open Sci. 6:190355. doi: 10.1098/rsos.190355
Pratchett, M. S., Hoey, A. S., and Wilson, S. K. (2014). Reef degradation and the loss of critical ecosystem goods and services provided by coral reef fishes. Curr. Opin. Environ. Sust. 7, 37–43. doi: 10.1016/j.cosust.2013.11.022
R Core Team, (2018). R: A language and environment for statistical computing. Vienna: R Foundation for Statistical Computing.
Randall, C. J., Jordan-Garza, A. G., Muller, E. M., and Van Woesik, R. (2014). Relationships between the history of thermal stress and the relative risk of diseases of Caribbean corals. Ecology 95, 1981–1994. doi: 10.1890/13-0774.1
Randall, R. H., and Myers, R. F. (1983). Guide to the Coastal Resources of Guam: Vol. 2. The Corals. Mangilao: University of Guam Press.
Raymundo, L. J., Burdick, D., Hoot, W. C., Miller, R. M., Brown, V., Reynolds, T., et al. (2019). Successive bleaching events cause mass coral mortality in Guam. Micronesia. Coral Reefs 38, 677–700. doi: 10.1007/s00338-019-01836-2
Raymundo, L. J., Couch, C. S., Bruckner, A. W., and Harvell, C. D. (2008). Coral Disease Handbook Guidelines for Assessment, Monitoring & Management. Melbourne: Currie Communications.
Raymundo, L. J., Halford, A. R., Maypa, A. P., and Kerr, A. M. (2009). Functionally diverse reef-fish communities ameliorate coral disease. Proc. Natl. Acad. Sci. U.S.A. 106, 17067–17070. doi: 10.1073/pnas.0900365106
Redding, J. E., Myers-Miller, R. L., Baker, D. M., Fogel, M., Raymundo, L. J., and Kim, K. (2013). Link between sewage-derived nitrogen pollution and coral disease severity in Guam. Mar. Pollut. Bull. 73, 57–63. doi: 10.1016/j.marpolbul.2013.06.002
Richardson, L. E., Graham, N. A. J., Pratchett, M. S., Eurich, J. G., and Hoey, A. S. (2018). Mass coral bleaching causes biotic homogenization of reef fish assemblages. Glob. Change Biol. 24, 3117–3129. doi: 10.1111/gcb.14119
Roff, G., Hoegh-Guldberg, O., and Fine, M. (2006). Intra-colonial response to Acroporid “white syndrome” lesions in tabular Acropora spp. (Scleractinia). Coral Reefs 25, 255–264. doi: 10.1007/s00338-006-0099-4
Rosenberg, E., Koren, O., Reshef, L., Efrony, R., and Zilber-Rosenberg, I. (2007). The role of microorganisms in coral health, disease and evolution. Nat. Rev. Microbiol. 5, 355–362. doi: 10.1038/nrmicro1635
Rotjan, R. D., and Lewis, S. M. (2008). Impact of coral predators on tropical reefs. Mar. Ecol. Prog. Ser. 367, 73–91. doi: 10.3354/meps07531
Ruiz-Moreno, D., Willis, B. L., Page, A. C., Weil, E., Cróquer, A., Vargas-Angel, B., et al. (2012). Global coral disease prevalence associated with sea temperature anomalies and local factors. Dis. Aquat. Organ. 100, 249–261. doi: 10.3354/dao02488
Sammarco, P. W. (1980). Diadema and its relationship to coral spat mortality: grazing, competition, and biological disturbance. J. Exp. Mar. Biol. Ecol. 45, 245–272. doi: 10.1016/0022-0981(80)90061-1
Séré, M. G., Tortosa, P., Chabanet, P., Turquet, J., Quod, J. P., and Schleyer, M. H. (2013). Bacterial communities associated with Porites white patch syndrome (PWPS) on three western Indian Ocean (WIO) coral reefs. PLoS One 8:e83746. doi: 10.1371/journal.pone.0083746
Sheridan, C., Baele, J. M., Kushmaro, A., Fréjaville, Y., and Eeckhaut, I. (2014). Terrestrial runoff influences white syndrome prevalence in SW Madagascar. Mar. Environ. Res. 101, 44–51. doi: 10.1016/j.marenvres.2014.08.003
Sokolow, S. (2009). Effects of a changing climate on the dynamics of coral infectious disease: a review of the evidence. Dis. Aquat. Organ. 87, 5–18. doi: 10.3354/dao02099
Sutherland, K. P., Porter, J. W., and Torres, C. (2004). Disease and immunity in Caribbean and Indo-Pacific zooxanthellate corals. Mar. Ecol. Progr. Ser. 266, 273–302. doi: 10.3354/meps266273
Swain, T. D., Vega-Perkins, J. B., Oestreich, W. K., Triebold, C., DuBois, E., Henss, J., et al. (2016). Coral bleaching response index: a new tool to standardize and compare susceptibility to thermal bleaching. Glob. Change Biol. 22, 2475–2488. doi: 10.1111/gcb.13276
Szmant, A. M. (2002). Nutrient enrichment on coral reefs: is it a major cause of coral reef decline? Estuaries 25, 743–766. doi: 10.1007/BF02804903
Thomas, L., and Palumbi, S. R. (2017). The genomics of recovery from coral bleaching. Proc. R. Soc. B Biol. Sci. 284:20171790. doi: 10.1098/rspb.2017.1790
Thompson, A., Schroeder, T., Brando, V. E., and Schaffelke, B. (2014). Coral community responses to declining water quality: whitsunday Islands. Great Barrier Reef, Australia. Coral Reefs 33, 923–938. doi: 10.1007/s00338-014-1201-y
van Oppen, M. J. H., and Blackall, L. L. (2019). Coral microbiome dynamics, functions and design in a changing world. Nat. Rev. Microbiol. 17, 557–567. doi: 10.1038/s41579-019-0223-4
Vega Thurber, R. L., Burkepile, D. E., Fuchs, C., Shantz, A. A., Mcminds, R., and Zaneveld, J. R. (2014). Chronic nutrient enrichment increases prevalence and severity of coral disease and bleaching. Glob. Change Biol. 20, 544–554. doi: 10.1111/gcb.12450
Veron, J., and Stafford-Smith, M. (2000). Corals of the World, ed. M. Stafford-Smith, (Townsville: Australian Institute of Marine Science and CRR Ald Pty Ltd), 1–3.
Wallace, C. (1999). Staghorn Corals of the World: A Revision of the Genus Acropora. Clayton: CSIRO PUBLISHING.
Walton, C. J., Hayes, N. K., and Gilliam, D. S. (2018). Impacts of a regional, multi-year, multi-species coral disease outbreak in southeast Florida. Front. Mar. Sci. 5:323. doi: 10.3389/fmars.2018.00323
Williams, G. J., Aeby, G. S., Cowie, R. O. M., and Davy, S. K. (2010). Predictive modeling of coral disease distribution within a reef system. PLoS One 5:e9264. doi: 10.1371/journal.pone.0009264
Winston, M., Couch, C., Ferguson, M., Huntington, B., Swanson, D., and Vargas-Ángel, B. (2019). Ecosystem Sciences Division Standard Operating Procedures: Data Collection for Rapid Ecological Assessment Benthic Surveys, 2018 Update. Washington, DC: NOAA, 65. doi: 10.25923/39jh-8993 NOAA Technical Memorandum, NMFS-PIFSC.
Work, T. M., and Aeby, G. S. (2006). Systematically describing gross lesions in corals. Dis. Aquat. Organ. 70, 155–160. doi: 10.3354/dao070155
Keywords: coral, disease, predictive, modeling, management, White Syndrome
Citation: Greene A, Donahue MJ, Caldwell JM, Heron SF, Geiger E and Raymundo LJ (2020) Coral Disease Time Series Highlight Size-Dependent Risk and Other Drivers of White Syndrome in a Multi-Species Model. Front. Mar. Sci. 7:601469. doi: 10.3389/fmars.2020.601469
Received: 01 September 2020; Accepted: 03 November 2020;
Published: 08 December 2020.
Edited by:
Noga Stambler, Bar-Ilan University, IsraelReviewed by:
Esti Kramarsky-Winter, Ben-Gurion University of the Negev, IsraelDouglas Fenner, Independent Researcher, Pago Pago, American Samoa
Copyright © 2020 Greene, Donahue, Caldwell, Heron, Geiger and Raymundo. This is an open-access article distributed under the terms of the Creative Commons Attribution License (CC BY). The use, distribution or reproduction in other forums is permitted, provided the original author(s) and the copyright owner(s) are credited and that the original publication in this journal is cited, in accordance with accepted academic practice. No use, distribution or reproduction is permitted which does not comply with these terms.
*Correspondence: Austin Greene, QXVzdGluTEdASGF3YWlpLmVkdQ==