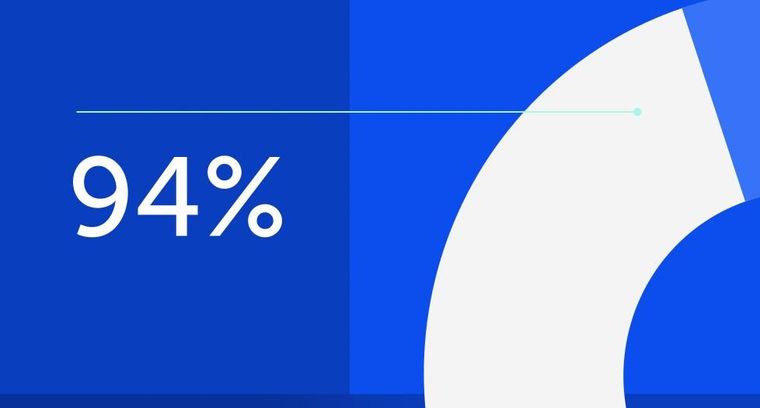
94% of researchers rate our articles as excellent or good
Learn more about the work of our research integrity team to safeguard the quality of each article we publish.
Find out more
METHODS article
Front. Mar. Sci., 30 November 2020
Sec. Ocean Observation
Volume 7 - 2020 | https://doi.org/10.3389/fmars.2020.572680
This article is part of the Research TopicMarine Biodiversity Observation Network (MBON)View all 16 articles
Marine hard-bottom communities are undergoing severe change under the influence of multiple drivers, notably climate change, extraction of natural resources, pollution and eutrophication, habitat degradation, and invasive species. Monitoring marine biodiversity in such habitats is, however, challenging as it typically involves expensive, non-standardized, and often destructive sampling methods that limit its scalability. Differences in monitoring approaches furthermore hinders inter-comparison among monitoring programs. Here, we announce a Marine Biodiversity Observation Network (MBON) consisting of Autonomous Reef Monitoring Structures (ARMS) with the aim to assess the status and changes in benthic fauna with genomic-based methods, notably DNA metabarcoding, in combination with image-based identifications. This article presents the results of a 30-month pilot phase in which we established an operational and geographically expansive ARMS-MBON. The network currently consists of 20 observatories distributed across European coastal waters and the polar regions, in which 134 ARMS have been deployed to date. Sampling takes place annually, either as short-term deployments during the summer or as long-term deployments starting in spring. The pilot phase was used to establish a common set of standards for field sampling, genetic analysis, data management, and legal compliance, which are presented here. We also tested the potential of ARMS for combining genetic and image-based identification methods in comparative studies of benthic diversity, as well as for detecting non-indigenous species. Results show that ARMS are suitable for monitoring hard-bottom environments as they provide genetic data that can be continuously enriched, re-analyzed, and integrated with conventional data to document benthic community composition and detect non-indigenous species. Finally, we provide guidelines to expand the network and present a sustainability plan as part of the European Marine Biological Resource Centre (www.embrc.eu).
Healthy ecosystems and the biodiversity they harbor are a prerequisite for the sustainable future of our planet (Rockström et al., 2009). Scientists are now more than ever forced to provide evidence to understand, and where possible counteract, the factors causing severe change in the biological composition of these environments. Such knowledge is critical as human pressures increase and accumulate, especially in coastal zones, from a combination of factors including traffic, wastewater discharges, energy production, aquaculture and fisheries, recreation, and tourism (Lotze et al., 2006; Worm et al., 2006; OSPAR, 2009). A key limitation to improved understanding of the impact of human activities on marine ecosystems is the ability to generate comparative biological time-series data at a large spatial scale (Richardson and Poloczanska, 2008; Dailianis et al., 2018; Guidi et al., 2020). There is therefore strong pressure on biological monitoring programs to implement standardized and scalable methods to assess status and change in marine biological communities in order to support marine research and policy (Bourlat et al., 2013; Borja et al., 2016; Danovaro et al., 2016; Bean et al., 2017; Bevilacqua et al., 2020). These methods need to fulfill several important criteria, including the implementation of common standards and protocols and to generate material samples and data that are FAIR: findable, accessible, interoperable, and re-usable (Tanhua et al., 2019).
Monitoring subtidal hard-bottom habitats is a challenge as they are three-dimensionally complex and inherently difficult to access (Bianchi et al., 2004; Beisiegel et al., 2017). In contrast to soft-bottom environments, which are widely sampled in a standardized fashion across countries and regions (e.g., with box corers or grab samplers), hard-bottom communities are usually studied by individual assessments in smaller areas using video recordings and scientific diving. A promising approach, however, is the use of artificial substrates—passive samplers that can record the community composition on the seafloor in a standardized way. Artificial Substrate Units (ASU), for example, have been used for many years in individual field experiments (Menge et al., 2002; Gobin and Warwick, 2006), while some European monitoring programs also use settlement plates for monitoring non-indigenous species (HELCOM, 2013). Another popular system is provided by Autonomous Reef Monitoring Structures (ARMS) (www.oceanarms.org). These are three-dimensional units consisting of stacked settlement plates attached to the sea floor (David et al., 2019). Because of their three-dimensional structure, mimicking the complexity of hard bottom marine substrates, ARMS attract both sessile and motile benthic organisms. These monitoring systems were originally developed during the Census of Marine Life and are currently in broad use for integrated studies combining morphological identification with metabarcoding (Leray and Knowlton, 2015; Pearman et al., 2016, 2018; Cahill et al., 2018).
DNA-metabarcoding has been advocated for some years as a potential method for rapid, effective, and scalable measurements of community composition in marine habitats (Bourlat et al., 2013; Borja et al., 2016; Bean et al., 2017). Recent studies have also tested the applicability of this method for hard-bottom monitoring and non-indigenous species (NIS) detection, comparing genetic with conventional methods (Pearman et al., 2016; Kelly et al., 2017; Cahill et al., 2018; Couton et al., 2019). Most of these studies concluded that metabarcoding adds substantial value for monitoring marine biological communities, especially when combined with other methods in biological monitoring programs.
The use of metabarcoding for rapid identification of species in marine communities, however, is not without limitations (e.g., Cahill et al., 2018). One obvious drawback is the still incomplete taxonomic reference databases, which limit positive identifications and can also lead to misidentifications (Meiklejohn et al., 2019; Weigand et al., 2019; Hestetun et al., 2020). It should be noted that alternative morphological-based identification also has flaws as the declining number of taxonomic experts and large numbers of cryptic species mean that mis-identification is commonplace, with important consequences especially for non-indigenous species (NIS) detection (e.g., Viard et al., 2019). Another important consideration of metabarcoding is the sensitivity of the results to primer choice and sequencing methods. A recent study by van der Loos and Nijland (2020) showed that there is a high degree of inconsistency in metabarcoding studies, which are often explorative and designed for a specific purpose, and hence not directly comparable. There is a need to move beyond episodic metabarcoding studies and build up time-series of genetic data (Danovaro et al., 2016; David et al., 2019).
Here we describe a contribution to the Marine Biodiversity Observation Network (MBON) consisting of Autonomous Reef Monitoring Structures (ARMS). The goal of the ARMS-MBON is to establish long-term assessments of status and change in hard-bottom communities in the European continental seas using standardized methodology and generating FAIR data. The network is initiated and maintained by European marine infrastructure programs and intends to contribute a community of practice to the Group on Earth Observations Biodiversity Observation Network, GEO BON (Kissling et al., 2018).
ARMS observatories were established in the vicinity of marinas, ports, marine protected areas (MPAs), and long-term ecological research (LTER) sites between December 2017 and May 2020 (Supplementary Table 1). The duration of sample events varied between short (2–3 months) and long (12–24 months) periods, and was repeated annually, when possible. Some deployment periods had to be extended during the COVID-19 pandemic due to travel and diving restrictions. In several cases, ARMS are deployed as part of national monitoring programs (e.g., Limfjord, Laeso, Swedish West coast, Getxo).
Each sampling event produces at least three fractions (40 μm sessile, 100–500 μm, and 500 μm −2 mm motile) as well as a stack of plate and specimen images. Images are analyzed by individual partners, while material samples are stored at −20°C and shipped for processing by the Institute of Marine Biology, Biotechnology and Aquaculture (IMBBC) of the Hellenic Centre for Marine Research (HCMR) in Greece. Detailed protocols for DNA extraction, PCR amplification, and sequencing are available on the website (http://www.arms-mbon.eu/) under Molecular Standard Operating Procedures (MSOP). In summary, DNA extractions are performed with the DNeasy PowerSoil Kit (Qiagen), while PCR amplification follow a two-step PCR protocol for: the mitochondrial cytochrome c oxidase subunit I (COI), the nuclear 18S small ribosomal subunit (18S rRNA) (except for the samples of 2018), and the nuclear Internal Transcribed Spacer (ITS1). Resulting amplicons are further sequenced using Illumina MiSeq Reagent Kit v3 (2 × 300 bp). Currently, samples are processed and sequenced as batches twice a year. In the future, samples may be processed more frequently and potentially involving additional partners, if these follow the established protocols.
All raw sequence files produced by the network are submitted to the European Nucleotide Archive, ENA (Amid et al., 2020), and processed with the Pipeline for Environmental DNA Metabarcoding Analysis, PEMA (Zafeiropoulos et al., 2020). Repeatable workflow procedures for integrated processing of image and sequence data are currently under development as part of the LifeWatch-ERIC Internal Joint Initiative on non-indigenous species. The final cleaned sequence files are stored in the PlutoF data management system (Abarenkov et al., 2010) where they are accessible to all members of the network.
During the pilot phase, we used two ARMS for parallel sampling trials with alternative preservation methods, namely Dimethyl sulfoxide (DMSO) vs. ethanol (EtOH). One ARMS was located in a Marine Protected Area in Sweden, while the other one was located in a marina in Greece (Supplementary Table 1). The samples from these two sites were used to analyze the effects of the preservation method and compare the community composition between localities and fractions, as well as for identifying non-indigenous species.
Currently, the network maintains 20 ARMS observatories in 14 European countries, as well as Greenland and Antarctica, ranging from tropical waters to polar environments (Figure 1, Supplementary Table 1). All major European continental seas are sampled, with exception of the Black Sea. To date, the network has deployed a total number of 134 ARMS units. The first sampling campaign (2018) deployed 20 ARMS across 11 sites, the second campaign (2019) deployed 53 ARMS across 18 sites, and the third campaign (2020) currently deploys 61 ARMS across 20 sites (Figure 1, Supplementary Table 1).
Figure 1. Overview of the ARMS-MBON observatories. (A) Deployed ARMS; (B,C) Geographical overview of currently established observatories. (D) Growth metrics of the network over time (for details see Supplementary Table 1). Numbers in parentheses (B,C) indicate the number of ARMS deployed in 2018, 2019, and 2020, respectively.
During the pilot phase, we customized the methodology of the global ARMS Program at the Smithsonian Institution (www.oceanarms.org/) to match the purpose of ARMS-MBON. Detailed protocols for sampling and sample processing are documented in the Handbook and in the molecular standard operating procedures (MSOP) available at the ARMS-MBON website (www.arms-mbon.eu). In addition, we also developed a specific partner registration sheet to allow new partners to register new observatories and receive consultation as well as training.
To date, 74% of all concluded sampling events have been sequenced (120 out of 162 material samples). The status of sequence processing and data publication is shown in Supplementary Table 2 and will be updated regularly on the ARMS-MBON website (www.arms-mbon.eu).
A model agreement for sample storage and Data Policy was developed in compliance with the Convention on Biological Diversity and the Nagoya Protocol on Access and Benefit Sharing (ABS) for the utilization of genetic resources in a fair and equitable way. Important documents associated with this model agreement include a Data Management Plan (DMP), Material Transfer Agreement (MTA), and Documents for Access and Benefit Sharing (ABS). All documents are available on the ARMS-MBON website (http://www.arms-mbon.eu/).
Data are published as packages—one for each ARMS (i.e., sampling event) and allow for continuous enrichment, as well as integration of sequence and image data during analysis (Exter et al., 2020). Detailed explanations of the ARMS-MBON data formats are given in the Supplementary Material. All data are published under the Open Access license CC BY 4.0, with a moratorium period of 1 year starting from the point when raw sequence data become available to the partner network. Two examples with data from the ARMS used in the case study are available under the Data Availability Statement. Data from all remaining and future sampling events will become automatically available under the same license after the embargo period, starting in May 2021.
Comparison of sequence reads derived from DMSO and ethanol preserved samples showed a higher yield for DMSO in the sessile fraction and a lower yield for DMSO in the motile fraction from Greece (500 μm), while DMSO and EtOH yield were almost equal in the sessile factions from Sweden (Figure 2). Despite this substantial variation of sequence reads in relation to the fixative, the representation of taxa, as well as the resulting species composition, remained very similar across both preservation methods (Figures 2, 3).
Figure 2. Bar chart showing the relative abundances of the main taxa based on the COI gene and image analysis, at the phylum level, across the different fractions of two ARMS (Greece ARMS: Crete_1HERP_180928-190128, Sweden ARMS: Koster_VH2_180418-180906).
Figure 3. Overview over variance and overlaps in composition of communities obtained from different fractions, preservations, as well as from plate images of two ARMS (Greece ARMS: Crete_1HERP_180928-190128, Sweden ARMS: Koster_VH2_180418-180906). (A) Non-metric Multi-dimensional Scaling (NMDS) plot at class level deducted from COI sequence and image analysis. (B) Venn diagram showing the overlap in the species identified from genetic data and images.
Combined analysis of genetic and image data resulted in 72 identified species from the Swedish ARMS, with 8% overlap between genetic and image-based species observations (Figure 3, Supplementary Table 3). In comparison, the analysis of the Greek ARMS resulted in 69 identified species with only 4% overlap between genetic and image-based communities, highlighting the high degree of complementarity of image and genetic data collected by the ARMS.
Comparison of the taxonomic composition between the three genetic fractions (40 μm sessile, 100–500 μm, and 500 μm −2 mm motile) shows a shift in taxonomic dominance between fractions and hence also indicates a high degree of complementarity (Figure 2). While the sessile fractions are dominated by sequences from chordates (tunicates), the motile fractions are co-dominated by arthropods, nematodes, mollusks, and single cellular eukaryotes. The complementarity between the fractions is also reflected by the distance between motile and sessile fractions in the NMDS plot (Figure 3).
We matched species observations obtained in the case study against the EASIN Catalog of Alien Species (https://easin.jrc.ec.europa.eu/easin/Catalogue) as well as the AquaNIS database (Olenin et al., 2014) and identified 16 non-indigenous species (NIS) that are either alien or cryptogenic in the region of detection (Table 1). Most of the NIS were tunicates, while all but one NIS were detected on the Greek ARMS. Such high numbers of NIS can be explained by the substantial biological invasion in the Eastern Mediterranean, as well as the placement of the Greek ARMS in a marina. In contrast, the low number of NIS on the Swedish ARMS can be explained by its placement in a Marine Protected Area. However, some NIS observations still need to be confirmed, either by taxonomic experts (e.g., in the case of Botryllus spp. in Greece) or by higher confidence estimates (e.g., in the case of Ostraea angasi). We also identified previously unknown NIS, as for example the first record of Anteaeolidiella lurana in the Eastern Mediterranean, a little-known nudibranch species which is suspected to have expanded its distribution range through shipping transport (Bariche et al., 2020).
Table 1. List of identified non-indigenous species (NIS) resulting from matching the complete species lists from two samples (Greece ARMS: Crete_1HERP_180928-190128, Sweden ARMS: Koster_VH2_180418-180906) against EASIN and AquaNIS databases.
Our results indicate that DMSO preservation does not impair the biological analysis, confirming earlier results by Ransome et al. (2017) who likewise found that DMSO is an appropriate preservative for estimating the sessile community in ARMS. Importantly, DMSO samples can be shipped across the network more easily. For this reason, all samples in ARMS-MBON are preserved in DMSO following the protocol of Seutin et al. (1991).
The low overlap between the communities identified by image and genetic analysis (Figure 3) demonstrates the strengths and weaknesses of both conventional and novel identification methods, while it also indicates how these methods complement each other. Genetic identifications are usually confined by incomplete reference libraries (Hestetun et al., 2020), while image-based identifications are confined by the available taxonomic expertise and image resolution. These limitations decline as genetic and image reference libraries continue to grow and computational image-based identification methods improve in the coming years. This will likely result in an increased number of identified species from ARMS.
Our case study shows that ARMS are well suited for hard-bottom monitoring as they provide standardized genetic data that can be continuously enriched and re-analyzed to document benthic community composition, obtain quantitative estimates from image analysis, and detect non-indigenous species. Although the species composition measured by ARMS might not always accurately reflect the biotic composition of surrounding natural substrates due to e.g., selective effects of the substrate (Chase et al., 2016; Sanabria-Fernandez et al., 2018), the standardized method still allows for comparative studies of species assemblages across broad spatial and temporal scales.
ARMS-MBON membership is open to new partners who may register through the program website (www.arms-mbon.eu), although support from central services provided by the European Marine Biological Resource Centre (EMBRC) may be subject to eligibility and availability of resources. Any new partner is expected to set up and maintain at least one observatory in coastal waters and follow the methods and protocols for sampling and sample processing. Partners will also be able to further develop and customize any of the current protocols and drive the scientific application of the data generated by the network. Examples include adaptation of the sampling design to collect data for descriptors in the Marine Strategy Framework Directive (MSFD). As an example, Descriptor 2 (D2) of the MSFD directs EU member states to develop monitoring schemes and management strategies to keep non-indigenous species (NIS) introduced by human activities at levels that do not adversely alter the ecosystems. Given that ports and marinas constitute major introduction hotspots and dispersion pathways for NIS (Ulman et al., 2017), the establishment of ARMS-MBON observatories in such environments is expected to enhance the timely detection of new NIS across the European regional seas. So far, at least nine observatories in the network are deploying ARMS for this purpose, while at least four observatories are part of national MSFD monitoring schemes in Sweden, Denmark, Greece, and Spain.
Another potential application area is the collection of data for calculating Essential Biodiversity Variables (EBV), as suggested by Kissling et al. (2018). EBVs emphasize standardized repeated measures of the same community in the same location at short to long time intervals required for reporting biodiversity change (Pereira et al., 2013). In the future, ARMS-MBON time-series data should be tested for such applications in EBVs.
Presence surveys provided by ARMS-MBON can provide occurrence data for prioritized taxa, including the AZTI Marine Biotic Index (Borja and Muxika, 2005) and Non-indigenous species (NIS). Finally, species traits can be derived from plate images recording the prevalence or relative composition of calcifying species or filter-feeding groups that might indicate important trends in ocean acidification or nutrient levels.
The ambition of ARMS-MBON is to maintain the current network and expand the coverage of the observatories with, spatially and temporally, more dense sampling events. The sustainability of the initiative as a long-term monitoring and observation asset needs to be ensured in as many sites as possible. This will be partly achieved by support from the European Marine Biological Resource Centre (EMBRC-ERIC), which provides access to marine biodiversity, ecosystems, experimental facilities, and expertise to facilitate research using marine organisms (www.embrc.eu). As Europe's research infrastructure (RI) for accessing and studying marine biodiversity, and with a functional lifespan of at least 20 years, EMBRC can offer a solid base for the long-term support of the ARMS-MBON for eligible partners in Europe.
EMBRC is currently developing an “Omics Observation network” to be deployed across its 9 member states, which is expected to cover pelagic and benthic sampling. Through the network of RIs in Europe, EMBRC will also work to develop new bioinformatics tools and analysis pipelines with its partners, such as ELIXIR and LifeWatch. The ambition is to ensure integration of ARMS-MBON (the European pilot described in this paper) into global efforts, such as the Global ARMS Program (www.oceanarms.org), Genomic Observatories Network (Davies et al., 2014), Global Omics Observatory Network GLOMICON (Buttigieg et al., 2019), as well as regional infrastructure networks in other regions, such as those under the umbrella of the World Association of Marine Stations (IOC) or the Partnership for Observation of the Global Ocean (POGO) to expand the ARMS-MBON further in the future.
The EMBRC “Omics Observatory” aims to ensure long-term, sustainable observation of marine biodiversity, integrated in a global setting. As such, the European research infrastructure can support the ARMS-MBON by providing increased visibility of the initiative to researchers and stakeholders in Europe and beyond. It will provide a platform for training and maintaining standards in the network, as well as expanding the coverage by integrating new sites from EMBRC. Furthermore, it is likely that the coordination and sequencing costs of the ARMS-MBON sites in EMBRC can be covered by its “Omics Observatory,” ensuring data collection from these sites for the foreseeable future. Such an initiative provides an important demonstration and prototype for mainstreaming biodiversity observations at the molecular level into biodiversity monitoring programs and supports efforts led by a merger of the Genomic Observatories Network and GLOMICON (https://glomicon.org/) to establish an “Omics BON” (Buttigieg et al., 2019).
The datasets presented in this study can be found in the Integrated Marine Information System (IMIS) datasets catalog under the following address: http://www.assembleplus.eu/information-system?module=dataset&dasid=6405. The names of the repository/repositories and accession number(s) can also be found in the article/Supplementary Material.
MO, CP, KE, and CA conceived the paper. MO coordinated the network. KE coordinated the data management. CP coordinated the sample processing and sequencing. All authors have contributed in an equal manner to the presented results in this paper and also revised the manuscript.
This ARMS-MBON network is funded by the infrastructure programs ASSEMBLE Plus (grant no. 730984) and the European Marine Biological Resource Centre, EMBRC. Both programs establish and maintain the core network and provide services and consultation for deployment, sample processing, sequencing, data management, and analysis. Funding for ARMS observatories in the North Sea Region was provided by the INTERREG project GEANS (North Sea Program of the European Regional Development Fund of the European Union) and the Swedish Agency for Marine and Water Management (grant no. 3181-2019), and the Flanders LifeWatch contribution (Research Foundation Flanders grant I000819N). The ARMS observatory in Roscoff also received support from the Aquanis 2.0 project (FONDATION Total). Data management and analysis was funded by Swedish LifeWatch grant from the Swedish Research council (grant no. 2017-00634) as well as the EOSC NORDIC project (grant no. 857652). Guiding documents to obtain ABS clearance for access to genetic resources were developed in the framework of projects INTERREG EBB (EAPA_501/2016) and H2020 EOSC-Life (grant no. 824087).
The authors declare that the research was conducted in the absence of any commercial or financial relationships that could be construed as a potential conflict of interest.
We thank the LifeWatch ERIC and the Global ARMS Program for the helpful advice, especially Laetitia Plaisance at the Smithsonian Institution in Washington D.C. The ECIMAT Marine Station would like to thank Alberto Meizoso and Guillermo Díaz-Agras (Marine Biology Station of A Graña, University of Santiago de Compostela), Enrique Poza, Roberto Gómez and José González for their help on ARMS construction and deployment, and Prof. Pedro Gomes, Prof. Filipe Costa (CBMA, University of Minho) for help with sampling and lab processing and technical support. The MBA wish to thank John Bishop and Christine Wood for sample processing and technical support. HCMR thanks Argyro Zenetos for help confirming of NIS observations. We are grateful to the participants of JRA5 (Scientific Diving) of the AssemblePlus program for their support in ARMS deployment in several of our observatories. The project is an activity of the Genomic Observatories network. We wish to remember Dawn Field, who founded both the Genomics Standards Consortium and the Genomic Observatories. JT was supported by a Marie Sklodowska-Curie Individual Fellowship (IF) under contract number 797387. This is publication ISEM 2020–283.
The Supplementary Material for this article can be found online at: https://www.frontiersin.org/articles/10.3389/fmars.2020.572680/full#supplementary-material
Supplementary Table 1. Overview of observatories and sample events of the ARMS-MBON pilot phase.
Supplementary Table 2. Overview of genetic data generated during the ARMS-MBON pilot phase.
Supplementary Table 3. Output file consisting of seven spreadsheets showing the results of the sequence analysis of two samples (Greece ARMS: Crete_1HERP_180928-190128, Sweden ARMS: Koster_VH2_180418-180906) with ASV relative abundances across the various fractions and taxonomic assignments. Confidence estimates are provided for ASV and after each taxonomic level. Assignments with confidence estimates lower than 0.20 were not included in the statistical analysis and the lowest taxonomic level with higher confidence estimate was used instead. The eighth sheet (named “image-based observations”) shows the results of the manual species identification from image data, with measures of abundance and reference to the ARMS plate of origin.
Supplementary Material. Explanation of the ARMS-MBON data formats.
Abarenkov, K., Tedersoo, L., Nilsson, R. H., Vellak, K., Saar, I., Veldre, V., et al. (2010). PlutoF-a web based workbench for ecological and taxonomic research, with an online implementation for fungal ITS sequences. Evol. Bioinform. 6, 189–196. doi: 10.4137/EBO.S6271
Amid, C., Alako, B. T. F., Kadhirvelu, V. B., Burdett, T., Burgin, J., Fan, J., et al. (2020). The European Nucleotide Archive in 2019. Nucleic Acids Res. 48, D70–D76. doi: 10.1093/nar/gkz1063
Bariche, M., Al-Mabruk, S. A. A., Ates, M. A., Buyuk, A., Crocetta, F., Dritsas, M., et al. (2020). New alien Mediterranean biodiversity records (March 2020). Mediterr. Mar. Sci. 21, 129–145. doi: 10.12681/mms.21987
Bean, T. P., Greenwood, N., Beckett, R., Biermann, L., Bignell, J. P., Brant, J. L., et al. (2017). A review of the tools used for marine monitoring in the UK: Combining historic and contemporary methods with modeling and socioeconomics to fulfill legislative needs and scientific ambitions. Front. Mar. Sci. 4, 263. doi: 10.3389/fmars.2017.00263
Beisiegel, K., Darr, A., Gogina, M., and Zettler, M. L. (2017). Benefits and shortcomings of non-destructive benthic imagery for monitoring hard-bottom habitats. Mar. Pollut. Bull. 121, 5–15. doi: 10.1016/j.marpolbul.2017.04.009
Bevilacqua, S., Katsanevakis, S., Micheli, F., Sala, E., Rilov, G., Sarà, G., et al. (2020). The status of coastal benthic ecosystems in the Mediterranean Sea: evidence from ecological indicators. Front. Mar. Sci. 7, 475. doi: 10.3389/fmars.2020.00475
Bianchi, C. N., Pronzato, R., Cattaneo-Vietti, R., Benedetti-Cecchi, L., Morri, C., Pansini, M., et al. (2004). “Mediterranean marine benthos: a manual of methods for its sampling and study,” in Biologia Marina Mediterranea, Vol. 11, eds M. C. Gambi, M. Dappiano (Genoa: SIBM), 185–216.
Borja, A., Elliott, M., Andersen, J. H., Berg, T., Carstensen, J., Halpern, B. S., et al. (2016). Overview of integrative assessment of marine systems: the ecosystem approach in practice. Front. Mar. Sci. 3. 20. doi: 10.3389/fmars.2016.00020
Borja, A., and Muxika, H. (2005). Guidelines for the use of AMBI (AZTI's Marine Biotic Index) in the assessment of the benthic ecological quality. Mar. Pollut. Bull. 50, 787–789. doi: 10.1016/j.marpolbul.2005.04.040
Bourlat, S. J., Borja, A., Gilbert, J., Taylor, M. I., Davies, N., Weisberg, S. B., et al. (2013). Genomics in marine monitoring: new opportunities for assessing marine health status. Mar. Pollut. Bull. 74, 19–31. doi: 10.1016/j.marpolbul.2013.05.042
Buttigieg, P. L., Janssen, F., Macklin, J., and Pitz, K. (2019). The Global Omics Observatory Network: Shaping standards for long-term molecular observation. Biodivers. Inf. Sci. Stand. 3, e36712. doi: 10.3897/biss.3.36712
Cahill, A. E., Pearman, J. K., Borja, A., Carugati, L., Carvalho, S., Danovaro, R., et al. (2018). A comparative analysis of metabarcoding and morphology-based identification of benthic communities across different regional seas. Ecol. Evol. 8, 8908–8920. doi: 10.1002/ece3.4283
Chase, A. L., Dijkstra, J. A., and Harris, L. G. (2016). The influence of substrate material on ascidian larval settlement. Mar. Pollut. Bull. 106, 35–42. doi: 10.1016/j.marpolbul.2016.03.049
Couton, M., Comtet, T., Le Cam, S., Corre, E., and Viard, F. (2019). Metabarcoding on planktonic larval stages: an efficient approach for detecting and investigating life cycle dynamics of benthic aliens. Manag. Biol. Invasion 10, 657–689. doi: 10.3391/mbi.2019.10.4.06
Dailianis, T., Smith, C. J., Papadopoulou, N., Gerovasileiou, V., Sevastou, K., Bekkby, T., et al. (2018). Human activities and resultant pressures on key European marine habitats: an analysis of mapped resources. Mar. Pol. 98, 1–10. doi: 10.1016/j.marpol.2018.08.038
Danovaro, R., Carugati, L., Berzano, M., Cahill, A. E., Carvalho, S., Chenuil, A., et al. (2016). Implementing and innovating marine monitoring approaches for assessing marine environmental status. Front. Mar. Sci. 3:213. doi: 10.3389/fmars.2016.00213
David, R., Uyarra, M. C., Carvalho, S., Anlauf, H., Borja, A., Cahill, A. E., et al. (2019). Lessons from photo analyses of Autonomous Reef Monitoring Structures as tools to detect (bio-)geographical, spatial, and environmental effects. Mar. Pollut. Bull. 141, 420–429. doi: 10.1016/j.marpolbul.2019.02.066
Davies, N., Field, D., Amaral-Zettler, L., Clark, M. S., Deck, J., Drummond, A., et al. (2014). The founding charter of the Genomic Observatories Network. Gigascience 3, 2. doi: 10.1186/2047-217X-3-2
Exter, K., Decruw, C., Portier, M., Gerovasileiou, V., Pavloudi, C., and Obst, M. (2020). Genomics Observatory Use-Case: the challenge to standardise image and sequence data to Darwin Core format. Biodivers. Inf. Sci. Stand. 4, e58938. doi: 10.3897/biss.4.58938
Gobin, J. F., and Warwick, R. M. (2006). Geographical variation in species diversity: a comparison of marine polychaetes and nematodes. J. Exp. Mar. Biol. Ecol. 330, 234–244. doi: 10.1016/j.jembe.2005.12.030
Guidi, L., Guerra, A. F, Canchaya, C., Curry, E., Foglini, F., Irisson, J.-O., et al. (2020). “Big data in marine science,” in Future Science Brief 6 of the European Marine Board, eds B. Alexander, J. J. Heymans, A. Muñiz Piniella, P. Kellett, J. Coopman (Ostend: European Marine Board), 1–52. doi: 10.5281/zenodo.3755793
HELCOM (2013). HELCOM ALIENS 2- Non-Native Species Port Survey Protocols, Target Species Selection and Risk Assessment Tools for the Baltic Sea, 34.
Hestetun, J. T., Bye-Ingebrigtsen, E., Nilsson, R. H., Glover, A. G., Johansen, P. O., and Dahlgren, T. G. (2020). Significant taxon sampling gaps in DNA databases limit the operational use of marine macrofauna metabarcoding. Mar Biodivers. 50, 70. doi: 10.1007/s12526-020-01093-5
Kelly, R. P., Closek, C. J., O'Donnell, J. L., Kralj, J. E., Shelton, A. O., and Samhouri, J. F. (2017). Genetic and manual survey methods yield different and complementary views of an ecosystem. Front. Mar. Sci. 3:283. doi: 10.3389/fmars.2016.00283
Kissling, W. D., Ahumada, J. A., Bowser, A., Fernandez, M., Fernandez, N., Garcia, E. A., et al. (2018). Building essential biodiversity variables (EBVs) of species distribution and abundance at a global scale. Biol. Rev. 93, 600–625. doi: 10.1111/brv.12359
Leray, M., and Knowlton, N. (2015). DNA barcoding and metabarcoding of standardized samples reveal patterns of marine benthic diversity. Proc. Natl. Acad. Sci. U. S. A.,112, 2076–2081. doi: 10.1073/pnas.1424997112
Lotze, H. K., Lenihan, H. S., Bourque, B. J., Bradbury, R. H., Cooke, R. G., Kay, M. C., et al. (2006). Depletion, degradation, and recovery potential of estuaries and coastal seas. Science. 312, 1806–1809. doi: 10.1126/science.1128035
Meiklejohn, K. A., Damaso, N., and Robertson, J. M. (2019). Assessment of BOLD and GenBank - their accuracy and reliability for the identification of biological materials. PLoS ONE 14:e217084. doi: 10.1371/journal.pone.0217084
Menge, B. A., Sanford, E., Daley, B. A., Freidenburg, T. L., Hudson, G., and Lubchenco, J. (2002). Inter-hemispheric comparison of bottom-up effects on community structure: insights revealed using the comparative-experimental approach. Ecol. Res. 17, 1–16. doi: 10.1046/j.1440-1703.2002.00458.x
Olenin, S., Narščius, A., Minchin, D., David, M., Galil, B., Gollasch, S., et al. (2014). Making non-indigenous species information systems practical for management and useful for research: an aquatic perspective. Biol Conserv. 173, 98–107. doi: 10.1016/j.biocon.2013.07.040
OSPAR (2009). Trend Analysis of Maritime Human Activities and Their Collective Impact on the OSPAR Maritime Area, 443. ISBN: 978-1-906840-83-9.
Pearman, J. K., Anlauf, H., Irigoien, X., and Carvalho, S. (2016). Please mind the gap - visual census and cryptic biodiversity assessment at central Red Sea coral reefs. Mar. Environ. Res. 118, 20–30. doi: 10.1016/j.marenvres.2016.04.011
Pearman, J. K., Leray, M., Villalobos, R., Machida, R. J., Berumen, M. L., Knowlton, N., et al. (2018). Cross-shelf investigation of coral reef cryptic benthic organisms reveals diversity patterns of the hidden majority. Sci Rep. 8:8090. doi: 10.1038/s41598-018-26332-5
Pereira, H. M., Ferrier, S., Walters, M., Geller, G. N., Jongman, R. H. G., Scholes, R. J., et al. (2013). Essential biodiversity variables. Science 339, 277–278. doi: 10.1126/science.1229931
Ransome, E., Geller, J. B., Timmers, M., Leray, M., Mahardini, A., Sembiring, A., et al. (2017). The importance of standardization for biodiversity comparisons: a case study using autonomous reef monitoring structures (ARMS) and metabarcoding to measure cryptic diversity on Moorea coral reefs, French Polynesia. PLoS One. 12:e0175066. doi: 10.1371/journal.pone.0175066
Richardson, A. J., and Poloczanska, E. S. (2008). Ocean science - Under-resourced, under threat. Science 320, 1294–1295. doi: 10.1126/science.1156129
Rockström, J., Steffen, W., Noone, K., Persson, A., Chapin, F. S., Lambin, E. F., et al. (2009). A safe operating space for humanity. Nature 461, 472–475. doi: 10.1038/461472a
Sanabria-Fernandez, J. A., Lazzari, N., Riera, R., and Becerro, M. A. (2018). Building up marine biodiversity loss: artificial substrates hold lower number and abundance of low occupancy benthic and sessile species. Mar. Environ. Res. 140, 190–199. doi: 10.1016/j.marenvres.2018.06.010
Seutin, G., White, B. N., and Boag, P. T. (1991). Preservation of avian blood and tissue samples for DNA analyses. Can. J. Zool.-Rev. Can. Zool. 69, 82–90. doi: 10.1139/z91-013
Tanhua, T., Pouliquen, S., Hausman, J., O'Brien, K., Bricher, P., de Bruin, T., et al. (2019). Ocean FAIR data services. Front. Mar. Sci. 6, 440. doi: 10.3389/fmars.2019.00440
Ulman, A., Ferrario, J., Occhpinti-Ambrogi, A., Arvanitidis, C., Bandi, A., Bertolino, M., et al. (2017). A massive update of non-indigenous species records in Mediterranean marinas. PeerJ. 5, e3954. doi: 10.7717/peerj.3954
van der Loos, L., and Nijland, R. (2020). Biases in bulk: DNA metabarcoding of marine communities and the methodology involved. Mol. Ecol. doi: 10.1111/mec.15592. [Epub ahead of print].
Viard, F., Roby, C., Turon, X., Bouchemousse, S., and Bishop, J. (2019). Cryptic diversity and database errors challenge non-indigenous species surveys: an illustration with Botrylloides spp. in the English Channel and Mediterranean Sea. Front. Mar. Sci. 6:615. doi: 10.3389/fmars.2019.00615
Weigand, H., Beermann, A. J., Ciampor, F., Costa, F. O., Csabai, Z., Duarte, S., et al. (2019). DNA barcode reference libraries for the monitoring of aquatic biota in Europe: gap-analysis and recommendations for future work. Sci. Total Environ. 678, 499–524. doi: 10.1016/j.scitotenv.2019.04.247
Worm, B., Barbier, E. B., Beaumont, N., Duffy, J. E., Folke, C., Halpern, B. S., et al. (2006). Impacts of biodiversity loss on ocean ecosystem services. Science 314, 787–790. doi: 10.1126/science.1132294
Keywords: benthic invertebrates, Marine Strategy Framework Directive (MSFD), Essential Biodiversity Variables (EBVs), Essential Ocean Variables (EOVs), European Marine Biological Resource Centre (EMBRC), non-indigenous species (NIS), Genomic Observatories, marine biodiversity assessment
Citation: Obst M, Exter K, Allcock AL, Arvanitidis C, Axberg A, Bustamante M, Cancio I, Carreira-Flores D, Chatzinikolaou E, Chatzigeorgiou G, Chrismas N, Clark MS, Comtet T, Dailianis T, Davies N, Deneudt K, de Cerio OD, Fortič A, Gerovasileiou V, Hablützel PI, Keklikoglou K, Kotoulas G, Lasota R, Leite BR, Loisel S, Lévêque L, Levy L, Malachowicz M, Mavrič B, Meyer C, Mortelmans J, Norkko J, Pade N, Power AM, Ramšak A, Reiss H, Solbakken J, Staehr PA, Sundberg P, Thyrring J, Troncoso JS, Viard F, Wenne R, Yperifanou EI, Zbawicka M and Pavloudi C (2020) A Marine Biodiversity Observation Network for Genetic Monitoring of Hard-Bottom Communities (ARMS-MBON). Front. Mar. Sci. 7:572680. doi: 10.3389/fmars.2020.572680
Received: 15 June 2020; Accepted: 05 November 2020;
Published: 30 November 2020.
Edited by:
Frank Edgar Muller-Karger, University of South Florida, United StatesReviewed by:
Lara Jane Atkinson, University of Cape Town, South AfricaCopyright © 2020 Obst, Exter, Allcock, Arvanitidis, Axberg, Bustamante, Cancio, Carreira-Flores, Chatzinikolaou, Chatzigeorgiou, Chrismas, Clark, Comtet, Dailianis, Davies, Deneudt, de Cerio, Fortič, Gerovasileiou, Hablützel, Keklikoglou, Kotoulas, Lasota, Leite, Loisel, Lévêque, Levy, Malachowicz, Mavrič, Meyer, Mortelmans, Norkko, Pade, Power, Ramšak, Reiss, Solbakken, Staehr, Sundberg, Thyrring, Troncoso, Viard, Wenne, Yperifanou, Zbawicka and Pavloudi. This is an open-access article distributed under the terms of the Creative Commons Attribution License (CC BY). The use, distribution or reproduction in other forums is permitted, provided the original author(s) and the copyright owner(s) are credited and that the original publication in this journal is cited, in accordance with accepted academic practice. No use, distribution or reproduction is permitted which does not comply with these terms.
*Correspondence: Matthias Obst, bWF0dGhpYXMub2JzdEBtYXJpbmUuZ3Uuc2U=; orcid.org/0000-0003-0264-9631
Disclaimer: All claims expressed in this article are solely those of the authors and do not necessarily represent those of their affiliated organizations, or those of the publisher, the editors and the reviewers. Any product that may be evaluated in this article or claim that may be made by its manufacturer is not guaranteed or endorsed by the publisher.
Research integrity at Frontiers
Learn more about the work of our research integrity team to safeguard the quality of each article we publish.