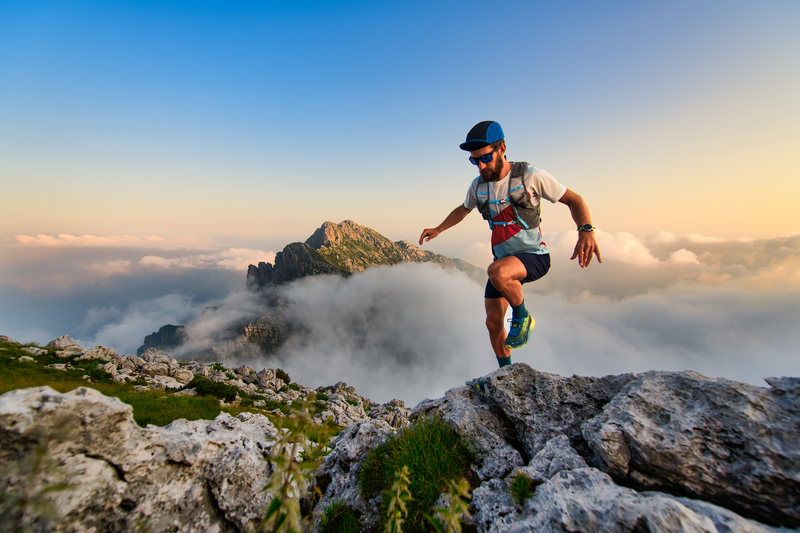
95% of researchers rate our articles as excellent or good
Learn more about the work of our research integrity team to safeguard the quality of each article we publish.
Find out more
MINI REVIEW article
Front. Mar. Sci. , 30 September 2020
Sec. Coral Reef Research
Volume 7 - 2020 | https://doi.org/10.3389/fmars.2020.544290
Increasing sea surface temperature and extreme heat events pose the greatest threat to coral reefs globally, with trends exceeding previous norms. The resultant mass bleaching events, such as those evidenced on the Great Barrier Reef in 2016, 2017, and 2020 have substantial ecological costs in addition to economic and social costs. Advancing remote (nanosatellites, rapid revisit traditional satellites) and in-field (drones) technological capabilities, cloud data processing, and analysis, coupled with existing infrastructure and in-field monitoring programs, have the potential to provide cost-effective and timely information to managers allowing them to better understand changes on reefs and apply effective remediation. Within a risk management framework for monitoring coral bleaching, we present an overview of how remote sensing can be used throughout the whole risk management cycle and highlight the role technological advancement has in earth observations of coral reefs for bleaching events.
Coral reef ecosystems are biodiversity hotspots that comprise only 0.1% of the ocean surface (Roberts et al., 2002; Fisher et al., 2015; Hoegh-Guldberg et al., 2017) yet are valued at $US36 billion annually due to the ecosystem services coral reefs provide, including subsistence and commercial fisheries, tourism and coastal protection (Spalding et al., 2017). It is widely recognized that the greatest threat to coral reefs globally is elevated sea surface temperature (SST) (Brainard et al., 2011; Hughes et al., 2018a) as increases in SST affect the coral’s capacity to reproduce and grow (Lough et al., 2018). On the Great Barrier Reef aerial surveys of large bleached areas correlated with in-water post bleaching mortality, and resultantly changes in species composition (Hughes et al., 2017). The current frequency and intensity of mass bleaching events because of temperature stress is unprecedented, and the full extent of impacts (Figure 1) is largely unknown due to the remoteness and inaccessibility of many of the world’s reefs (McCauley et al., 2013). Despite combined efforts to collect baseline data and document impact and recovery, there are many areas with no information.
Figure 1. Conceptual model of an acute heat event on coral reefs showing coral response pathways. Increased SST, depicted here by the mean annual 2018 SST anomaly (NOAA Coral Reef Watch, 2018), places pressure on coral reefs. Three major outcomes may result (Lough et al., 2018); these include (I) negligible effect – corals are able to maintain structure and function with little to no bleaching present; (II) bleaching – corals are affected by bleaching in areas, reef structure and function is still possible; and (III) mortality – extensive bleaching is apparent resulting in mortality – diversity, structure, and function of reef compromised. Re-bleaching may occur if the reef is subjected to subsequent thermal stress prior to baseline conditions being recovered. This may result in a negligible effect compared to post primary bleaching baseline, or further bleaching. (SST – source: NOAA Coral Reef Watch, 2018).
At the cutting edge of emerging technologies are fine spatial (<5 m), temporal (daily) scale nanosatellites, frequent revisit satellites (e.g., 5 days temporal scale, and 10 m spatial resolution), and low (<120 m) altitude drone systems that can cost-effectively provide data on aspects of the physical and biological status of marine ecosystems in unprecedented detail. Coupled with cloud-based data management and processing, and web-based knowledge delivery systems, a near real-time environmental monitoring system for managers and stakeholders of shallow and intertidal coral communities can be developed. Cloud processing and storage refers to online data storage and processing that uses third party processing and storage services. Applied alongside existing data collected from vessels and in-water surveys, these systems have the potential to identify climate-based threats to coral reefs across a range of spatial and temporal scales that have previously been inaccessible using traditional monitoring programs due to cost and logistical constraints of processing data, and geographical constraints in monitoring.
This paper presents an overview of common current shallow and intertidal coral reef monitoring, assessment, and rapid response strategies in the context of thermal stress. Thermal stress, due to increased SST and heatwave events presents a unique risk, as it is a global hazard requiring both global and local mitigation and monitoring. We address the thermal risk to corals alongside technological advances and develop a risk management framework for monitoring the response to thermal induced coral bleaching. Specifically, we investigate how remote sensing and associated technological advancements can be adopted throughout the entire risk management cycle of thermal induced bleaching events. This framework, to our knowledge, has not previously been applied for this type of risk, in doing so, we propose an adaptive and cost-efficient mechanism for authorities to monitor thermal induced coral bleaching throughout the risk management cycle.
Risk management typically conforms to a cyclic process categorized by pre-event and post-event phases (Figure 2; Joyce et al., 2009). Pre-event tends to be capacity building, consisting of reduction activities that either reduce the likelihood of the event occurring, or reduce its potential impact. This is achieved alongside preparation planning (readiness), including baseline data collection. Post-event includes response and recovery. The response phase focuses on managing risks as they occur, and is often implemented in isolation from other phases (Joyce et al., 2009).
Figure 2. Traditional risk management strategy utilized to develop a mechanism to respond to increasing thermal stress on coral reefs and the potential coral bleaching response. Reduction and readiness form the pre-event phases, whilst recovery, and response, the post-event phases. The tools best suited for each stage are depicted, communication is shown as required throughout the whole cycle.
The reduction phase has two key aims; (I) mitigate the vulnerability of coral reefs to thermal stress and the impacts of coral reef bleaching; and (II) mitigate the causes of thermal stress. Optimally, each aspect should operate concurrently; however, the geographic, cultural, and economic setting may influence the applicability of different mechanisms (Albright and Cooley, 2019).
The demand for environmental vulnerability assessments is increasing as the impacts of climate change become apparent (Wang et al., 2019). The vulnerability of coral reefs to repeated thermal extreme events may be influenced by spatial variability in temperature, and previous heat exposure and recovery time (Palumbi et al., 2014; Ainsworth et al., 2016; Safaie et al., 2018; Thomas et al., 2019); with recent research indicating that those coral able to spawn post a bleaching event (bleached and recovered, partially bleached, or unbleached) may have greater resistance to acute events (Hughes et al., 2019). Extreme thermal events resulting in mortality alter reef assemblages by reducing larval recruitment of key species (Hughes et al., 2019). This subsequently through time reduces overall biodiversity (e.g., species richness), which has been shown to increase the vulnerability of the ecosystem, diminishing its ability to maintain function (Beaugrand et al., 2015), resist disease (Bruno et al., 2007) and introduced species (Wernberg et al., 2011), adapt to future changes (Mora et al., 2016) and potentially hinder recovery. Reducing additional anthropogenic pressures on coral reefs may not minimize the thermal threat, however it may reduce their overall vulnerability (Wooldridge, 2009; Convention on Biological Diversity, 2015), and aid their ability to recover at the local scale.
Ultimately the scientific consensus is that the most successful way to mitigate the cause of thermal stress to coral reefs is to limit temperature increase (Ainsworth et al., 2016; Lough et al., 2018), which will require global action on reducing greenhouse gas emissions (IPCC, 2014). Whilst new earth observation technologies could assist in monitoring, maintaining or reducing greenhouse gas emissions (e.g., carbon and methane) (Doppelt, 2010) to hinder increases in SST, reviewing these strategies is beyond the scope of this framework.
The readiness phase has one main focus; to ensure there is sufficient environmental and biological baseline data and ongoing monitoring of coral reefs so that any change or potential threat (e.g., heat wave) can be detected. In turn, the response phase can then be initiated as efficiently and quickly as possible.
Fundamental to establishing baselines and collecting reliable data is ensuring data is collected timely via an appropriate method and interpreted to reflect the approach used. This has been discussed as a three-dimensional continuum; (I) spatial (area) scale; (II) temporal scale; and (III) spatial resolution (Kamal et al., 2015; Figure 3). The intersection of these nodes along this continuum determines the resolution of data required for the scale of the ecological phenomena, ranging from sub-organism (e.g., number of coral polyps) to landscape (e.g., area of reefs bleached) (Figure 3). The mechanisms used to measure the ecological phenomena will affect the result reported, due to accuracy, precision, and availability of data (Figure 3). Within coral reef monitoring, two main mechanisms are available: in-water, and spatial (satellite and aerial) data collection, though it is the combination of these that is required for optimal monitoring.
Figure 3. The spatial scale being measured and spatial resolution of the data is depicted as a linear relationship. Here we demonstrate the remote sensing method best suited to collect suitable data at major coral reef ecological scales. (Photo credits: “Branch”: N. Thake – AIMS; “Colony,” “Patch,” “Multi-Patch”: S. Hickey; “Reef-scape,” “Bioregion”: AIMS).
Spatial remote sensing has been viewed as advantageous to environmental monitoring, providing cost-efficient observations across large (≥10 km2) and difficult to access geographical areas at extended temporal scales (decadal) (Hedley et al., 2016). However, the tropical marine environment provides difficulties for traditional aerial remote sensing data acquisition, such as the spatial resolution of satellite data (Kachelriess et al., 2014; Hedley et al., 2016), and water clarity (e.g., turbidity, atmospheric noise including clouds, and ability of spectral wavelengths to penetrate water) (Dierssen et al., 2019). As such these methods have not been able to adequately detect fine-to-moderate scale (≤10 m2) coral reef responses to environmental pressures including temperature variations. Recent advancement however has seen satellite SST being monitored and utilized (e.g., NOAA Coral Reef Watch; Strong et al., 2013) to model and predict coral reef bleaching, enabling targeted assessment in locations deemed vulnerable to bleaching or sites of interest (Marshall et al., 2017). Additionally, geomorphic zone and benthic community habitat maps have been developed from structural measurements (Heyward et al., 2018) and through object-based image analysis approaches, which identify and attribute spatially homogeneous pixel clusters within remote sensing datasets, though accuracy is limited by appropriate field data and depth, with higher accuracy in shallower areas where the aerial sensor can penetrate (e.g., <5 m). This approach combines color and textural characteristics of pixel groups in combination with physical attributes such as depth, slope, significant wave height, and spatial relationships between the mapped categories (Hedley et al., 2016; Asner et al., 2017; Roelfsema et al., 2018; Li et al., 2019). Accuracy assessment methods of remotely sensed or predicted data with in-water data should be used to understand the uncertainty around this modeled data (Phillips and Marks, 1996; Meyer et al., 2019).
Traditional high resolution and publicly available satellites have increased revisit times, such as Sentinel two which can revisit locations every 5 days, providing greater opportunity to monitor surface changes. While rapid return, nanosatellites have further increased temporal frequency of image acquisition, with up to daily scenes of the globe (Asner et al., 2017; Planet Team, 2017). These are usually constellations of hundreds of relatively small satellites that together provide daily coverage of the Earth’s surface at fine-to-moderate (≤10 m) spatial resolution (Planet Team, 2017). Whilst still hindered by the same depth issues (<5 m) radiometric issues that influence traditional satellites, with increased temporal frequency (daily) they provide greater opportunity for cloud-free, non-turbid, low, or high tidal, reduced wave conditions to be captured for greater coverage of coral reefs (Hedley et al., 2018; Joyce et al., 2018), increasing the spatial scale and resolution of ecological assessment from these satellites (Figure 3). Whilst currently limited by sensors and spectral bands (e.g., Red, Green, Blue, Infrared are standard output of rapid-return nanosatellites), the cost to produce (Kenyon and Stanton, 2017; Sarzi-Amade et al., 2017) these satellites is far less than traditional satellites.
Low altitude (<120 m) drones provide an avenue to acquire very fine (<1 m) spatial resolution data compared to satellites, which due to being flown at low heights can provide images at a low cost acquired under specific environmental conditions, such as cloud free, low tide, and clear water. However, whilst they bridge a gap between very fine scale in-field data collection and moderate-to-broadscale satellite studies, they also present limitations, including flight time, flight conditions [e.g., wind, staying within line of site (dependent on conditions), data processing, and weight limitations in mounting sensors (Joyce et al., 2018)]. As such their application needs to be specific to the ecological phenomena being captured (Figure 3). Further research is required for best practice experimental design for drones in ecological studies, though Joyce et al. (2018) provides some recommendations for use in marine environments, including optimizing flight times and direction to minimize sun glint and reflection, in conjunction with flying at low tide to further minimize water interference.
Machine learning is a subset of artificial intelligence and statistics that builds models based on supervised input or labeled data. The modeling method itself determines statistical relationships and patterns in datasets with limited predefined model structure or statistical inference. Utilizing earth observation data with machine learning could enable virtual monitoring stations to be set up to alert changes in baseline conditions in near-real-time (Bakker and Ritts, 2018), initiating a move to the response phase quickly.
Access to free and low cost satellite programs (e.g., Landsat missions, Sentinel, Planet Labs, MODIS) has exponentially increased the amount of data available to researchers, enabling access to long-term global datasets that can be utilized for baseline change studies (Zhu et al., 2019). However, their use by environmental researchers has been limited by their capacity to process and analyze such large datasets, and methods to downscale information to scales relevant to ecology and management (Zhu et al., 2019).
The recent development in cloud processing [e.g., Microsoft Azure, Amazon Cloud, and Google Earth Engine (GEE)] and machine learning has been significant, increasing the amount of data available and processing capability, providing an avenue to link fine-scale ecological site data with broader-scale remote sensing data, locally and at a global scale. The development of cloud processing services has been fundamental in determining environmental baselines (Hansen et al., 2013), highlighting locations of possible ecosystem vulnerability, and aiding in the development of The Sustainable Development Goals through near-real time monitoring (Zhu et al., 2019). However, machine learning models are highly data driven and most require vast amounts of training data before they can be applied (Sun et al., 2017). Currently marine earth observation data lacks large libraries of standardized in situ datasets, essential for prototyping, training, tuning and benchmarking machine learning models. While cloud computing and access to specialized computing hardware [i.e., Graphics Processing Units (GPUs)] may be essential to train models and process the large volume of required data, the models are fast to run once they are appropriately trained and can be used for near-real time analysis. It is therefore essential to pre-train the models in advance and in anticipation of thermal impact events, for this reason we have included this in the readiness phase of the framework.
The response phase is traditionally activated when an alert (due to SST exceeding a degree heating week threshold) is triggered in the readiness phase. Current strategies of data acquisition are centerd on responding to impact events (Joyce et al., 2009), as such the current mechanisms employed between aerial and in-water survey techniques tend to be well implemented to provide observations on the extent and degree of bleaching, as exhibited by the 2016 bleaching event on the Great Barrier Reef (Hughes et al., 2018c) that invoked a multi-organization co-ordinated response (Great Barrier Reef Marine Park Authority, 2018). However, new technology can be utilized where current strategies are limited, to increase quantitative measurements, or to increase their efficacy.
In-water monitoring strategies are explored below. These techniques can be utilized in conjunction with key satellites that have short revisit times to overlap field acquisition dates. Such satellites include Planet Dove and RapidEye satellites which have daily global data, and Sentinel two which provides weekly temporal data with greater spectral bands [moderate-to-high resolution (10 m × 10 m pixels)] (Hedley et al., 2018).
Coral monitoring of small reef areas is currently achieved by in situ data collection following well-established ecological survey methods (English et al., 1994; Hill and Wilkinson, 2004). Here we focus on methods applicable to the shallow reef flat and intertidal environments, as these areas are the most suitable to aerial remote sensing. Such methods tend to use sensors to measure point data such as temperature at a fine spatial (e.g., cm) and very fine temporal resolution (e.g., minutes), or transects undertaken by a diver to collect information on benthic condition and community composition (Jonker et al., 2008). In deeper areas not addressed here, manta-tows can be used to cover larger areas of the reef (Hill and Wilkinson, 2004). The advent of (Remotely Operated Vehicles) ROVs has allowed assessments of reefs that are less accessible to scientific divers, increasing the capacity to monitor remote and mesophotic reefs – though not the focus of this framework (Kahng and Kelley, 2007; Armstrong et al., 2019). Corals are either assessed in real time in situ by a person or photos are assessed after the survey (Jonker et al., 2008). Observations in the field are thus able to provide detail on the taxonomic composition and condition of coral communities within a defined area. The ability to collect geo-located photo quadrats representing a footprint along transects collected by divers in combination with automated photo analysis has increased our ability to get large amounts of data consistently whilst maintaining a record that could be revisited to assess the benthos condition and composition (Roelfsema and Phinn, 2010; Beijbom et al., 2015; Roelfsema et al., 2015; González-Rivero et al., 2016). How this information relates to corals outside of the survey area can be difficult and requires extensive sampling designs and uncertainty models (Phillips and Marks, 1996; Meyer et al., 2019). Sampling design in these shallow environments also needs to consider tide in relation to the application of monitoring techniques. An optimal location would occur in a reef flat zone where low tide water level locally is less than 50 cm, and away from the wave zone to allow for optimal drone photos, and high tide water level locally is appropriate to allow for in-water snorkel or SCUBA diver transects (dependent on water level and conditions) to collect in-water diver data and images.
Emerging technologies by first responders can also be utilized as also detailed in section “Spatial Remote Sensing”. For instance, imagery can be captured with a small lightweight inexpensive drone across a range of habitat types and shallow water depths to quantitatively capture the extent of bleaching (Levy et al., 2018), or enable targeted in-water field assessments. Whilst drones can cover a larger area than a diver along a transect (approximately 500 m Euclidean line), they can only cover a relatively small part of a whole reef or reef systems. Drones can also be used in zones such as the reef flat, and emergent shallow reefs, which are difficult to access and often not prioritized in the field, though are most exposed to high thermal conditions (Le Nohaïc et al., 2017), and where other remote monitoring through virtual monitoring stations may be possible (e.g., from satellite data). More traditional aerial remote sensing has included photography from helicopters and planes, this method is capable of documenting reef systems, and has been used to document bleaching events, including the recent Great Barrier Reef bleaching events (Hughes et al., 2018b,c). Through combining current response strategies with the readiness phase of ongoing data analysis, the most vulnerable and resistant sites can be detected, providing necessary but often overlooked spatial information for studying coral resilience (van Oppen and Lough, 2018), microenvironment dynamics (e.g., fine-scale hydrodynamics), and coral reef restoration (Foo and Asner, 2019).
Fine-scale aerial drone imagery (and in-water imagery) provide an opportunity to map the structural complexity of shallow and intertidal coral reef areas. The geolocated and overlapping images can use machine learning software with location and scale reference data to create a structural surface layer, termed “structure-from-motion” (SFM). Common in terrestrial studies to monitor canopy height of forests (Cunliffe et al., 2016), SFM is novel in application to coral reefs though it provides an opportunity to derive rugosity measurements at known locations, when collected with accurate Global Positioning System (GPS) data. This then has the potential to be monitored through time to provide a measurement of coral cover structural complexity. Previous studies have used LiDAR to collect similar elevation and structural measures, however this is expensive, not easy to obtain in remote areas, and is still limited to non-turbid, relatively shallow (<25 m) depths.
The recovery phase is the last phase of the risk management cycle and occurs following the initial response to increased thermal stress. Within this risk framework, the recovery phase assesses the extent to which the coral reef has been able to return to pre-incident conditions (Figure 1). This phase combines the in-water and spatial strategies of the readiness and response phases. Ongoing monitoring is vital to assess any recovery and evaluate any management intervention. For systematic comparison, methods should mirror the readiness and response phases.
A key goal of risk management is to build resilience to increase the reef’s capability for rapid recovery and to adapt to a consecutive or secondary thermal stress events (Horita et al., 2017; Figure 1). However, ecosystems are changing, with changes in climate evident from range shifts of tropical fish and seagrass inhabiting temperate reefs (Wernberg et al., 2013). This is influencing the ecosystem structure and function of the reef, as such, shifting baselines need to be addressed in management plans (Elliott et al., 2015) including, measuring coral bleaching incidence, and the reef’s ability to meet ecosystem services, such as provisioning for fauna habitat (Pratchett et al., 2018). The key capability of remote sensing data and machine learning is to provide spatial context here. Marine spatial planning has been shown to be pivotal in successful marine park plans (Jones et al., 2016). Within this framework the ongoing data collection and machine learning methods could be utilized to highlight areas that have high and low incidence of thermal stress to target for resilience, or restoration activities (Hoegh-Guldberg et al., 2018, 2019).
Global policy and coordinated action have a pivotal role in maintaining the global ecosystem services coral reefs currently provide (Convention on Biological Diversity, 2015). The risk management framework presented is unique in that we specifically target how new and emerging remote sensing or technological advancements can be used throughout the whole risk management cycle to monitor the thermal stress to the world’s coral reefs and the ecosystems they support. Technological advancements in earth observation data collection and analysis provide an avenue to meet local and global reporting demands (e.g., IUCN Red List of Ecosystems; IPCC Paris Agreement) to assess the ongoing vulnerability of ecosystems in near real time.
Whilst limitations remain in the ability of technology as discussed here, the rate of advancement being experienced in machine learning and remote sensing means this ability will improve in the near future. Current advancements in satellite and drone technology is largely limited in application to the reef flat, a traditionally understudied area, though comprising a considerable area of global coral reefs (Vecsei, 2004). Understanding the place of remote sensing and machine learning as tools alongside existing mechanisms is pivotal in ensuring coral reefs are effectively monitored.
All authors participated in the workshop and contributed to the outline and development of the manuscript.
This manuscript was devised at a Spatial Monitoring workshop held by the Australian Institute of Marine Sciences under an Australian Institute of Marine Sciences Capacity Development Funding grant.
The authors declare that the research was conducted in the absence of any commercial or financial relationships that could be construed as a potential conflict of interest.
James Gilmour, Neal Cantin, Simon Harries, and Mark Case also attended and contributed to the workshop where the manuscript was devised. Figure 1 and also parts of the graphical abstract were created by OOID Scientific.
Ainsworth, T. D., Heron, S. F., Ortiz, J. C., Mumby, P. J., Grech, A., Ogawa, D., et al. (2016). Climate change disables coral bleaching protection on the Great Barrier Reef. Science 352, 338–342. doi: 10.1126/science.aac7125
Albright, R., and Cooley, S. (2019). A review of interventions proposed to abate impacts of ocean acidification on coral reefs. Reg. Stud. Mar. Sci. 29:100612. doi: 10.1016/j.rsma.2019.100612
Armstrong, R. A., Pizarro, O., and Roman, C. (2019). “Underwater robotic technology for imaging mesophotic coral ecosystems,” in Mesophotic Coral Ecosystems, eds Y. Loya, K. A. Puglise, and T. C. L. Bridge (Berlin: Springer), 973–988. doi: 10.1007/978-3-319-92735-0_51
Asner, G. P., Martin, R. E., and Mascaro, J. (2017). Coral reef atoll assessment in the South China sea using planet dove satellites. Remote Sens. Ecol. Conserv. 3, 57–65. doi: 10.1002/rse2.42
Bakker, K., and Ritts, M. (2018). Smart Earth: a meta-review and implications for environmental governance. Global Environ. Change 52, 201–211. doi: 10.1016/j.gloenvcha.2018.07.011
Beaugrand, G., Edwards, M., Raybaud, V., Goberville, E., and Kirby, R. R. (2015). Future vulnerability of marine biodiversity compared with contemporary and past changes. Nat. Clim. Change 5, 695–701. doi: 10.1038/nclimate2650
Beijbom, O., Edmunds, P. J., Roelfsema, C., Smith, J., Kline, D. I., Neal, B. P., et al. (2015). Towards automated annotation of benthic survey images: variability of human experts and operational modes of automation. PLoS One 10:e0130312. doi: 10.1371/journal.pone.0130312
Brainard, R. E., Birkeland, C., Eakin, C. M., McElhany, P., Miller, M. W., Patterson, M., et al. (2011). Status Review Report of 82 Candidate Coral Species Petitioned Under the U.S. Endangered SPECIES. NOAA Technical Memorandum NMFS-PIFSC-27. Washington, DC: Act. U.S. Dep. Commer, 530.
Bruno, J. F., Selig, E. R., Casey, K. S., Page, C. A., Willis, B. L., Harvell, C. D., et al. (2007). Thermal stress and coral cover as drivers of coral disease outbreaks. PLoS Biol. 5:e124. doi: 10.1371/journal.pbio.0050124
Convention on Biological Diversity (2015). Priority Action to Achieve, Aichi Biodiversity Target 10 for Coral Reefs and Closely Associated Ecosystems. NewYork, NY: Convention on Biological Diversity, 1–16.
Cunliffe, A. M., Brazier, R. E., and Anderson, K. (2016). Ultra-fine grain landscape-scale quantification of dryland vegetation structure with drone-acquired structure-from-motion photogrammetry. Remote Sens. Environ. 183, 129–143. doi: 10.1016/j.rse.2016.05.019
Dierssen, H. M., Bostrom, K. J., Chlus, A., Hammerstrom, K., Thompson, D. R., and Lee, Z. (2019). Pushing the limits of seagrass remote sensing in the turbid waters of elkhorn slough, california. Remote Sens. 11:1664. doi: 10.3390/rs11141664
Doppelt, B. (2010). Leading Change Toward Sustainability. London: Taylor & Francis Group, doi: 10.4324/9781351278966
Elliott, M., Borja, Á, McQuatters-Gollop, A., Mazik, K., Birchenough, S., Andersen, J. H., et al. (2015). Force majeure: will climate change affect our ability to attain good environmental status for marine biodiversity? Mar. Pollut. Bull. 95, 7–27. doi: 10.1016/j.marpolbul.2015.03.015
English, S., Wilkinson, C., and Baker, V. (1994). Survey Manual for Tropical Marine Resources. Townsvilleia.: Australian Institute of Marine Science.
Fisher, R., O’Leary, R. A., Low-Choy, S., Mengersen, K., Knowlton, N., Brainard, R. E., et al. (2015). Species richness on coral reefs and the pursuit of convergent global estimates. Curr. Biol. 25, 500–505. doi: 10.1016/j.cub.2014.12.022
Foo, S. A., and Asner, G. P. (2019). Scaling up coral reef restoration using remote sensing technology. Front. Mar. Sci. 6:79. doi: 10.3389/fmars.2019.00079
González-Rivero, M., Beijbom, O., Rodriguez-Ramirez, A., Holtrop, T., González-Marrero, Y., Ganase, A., et al. (2016). Scaling up ecological measurements of coral reefs using semi-automated field image collection and analysis. Remote Sens. 8:1:30.
Great Barrier Reef Marine Park Authority (2018). Great Barrier Reef. Annual Report 2017-2018. Townsville, QLD: Great Barrier Reef Marine Park Authority.
Hansen, M. C., Potapov, P. V., Moore, R., Hancher, M., Turubanova, S. A., Tyukavina, A., et al. (2013). High-resolution global maps of 21st-century forest cover change. Science 342, 850–853. doi: 10.1126/science.1244693
Hedley, J. D., Roelfsema, C., Brando, V., Giardino, C., Kutser, T., Phinn, S., et al. (2018). Coral reef applications of sentinel-2: coverage, characteristics, bathymetry and benthic mapping with comparison to Landsat 8. Remote Sens. Environ. 216, 598–614. doi: 10.1016/j.rse.2018.07.014
Hedley, J. D., Roelfsema, C. M., Chollett, I., Harborne, A. R., Heron, S. F., Weeks, S., et al. (2016). Remote sensing of coral reefs for monitoring and management: a review. Remote Sens. 8:118. doi: 10.3390/rs8020118
Heyward, A., Colquhoun, J., Cripps, E., McCorry, D., Stowar, M., Radford, B., et al. (2018). No evidence of damage to the soft tissue or skeletal integrity of mesophotic corals exposed to a 3d marine seismic survey. Mar. Pollut. Bull. 129, 8–13. doi: 10.1016/j.marpolbul.2018.01.057
Hill, J., and Wilkinson, C. (2004). Methods for Ecological Monitoring of Coral Reefs. Australian Institute of Marine Science, Townsville, Version 1, 1-116. Avaliable online at: https://www.cbd.int/doc/case-studies/tttc/tttc-00197-en.pdf (accessed August 25, 2020).
Hoegh-Guldberg, O., Poloczanska, E. S., Skirving, W., and Dove, S. (2017). Coral reef ecosystems under climate change and ocean acidification. Front. Mar. Sci. 4:158. doi: 10.3389/fmars.2017.00158
Hoegh-Guldberg, O., Kennedy, E. V., Beyer, H. L., McClennen, C., and Possingham, H. P. (2018). Securing a long-term future for coral reefs. Trends Ecol. Evol. 33, 936–944. doi: 10.1016/j.tree.2018.09.006
Hoegh-Guldberg, O., Jacob, D., Taylor, M., Bolaños, T. G., Bindi, M., Brown, S., et al. (2019). The human imperative of stabilizing global climate change at 1.5°C. Science 365:eaaw6974. doi: 10.1126/science.aaw6974
Horita, F. E. A., de Albuquerque, J. P., Marchezini, V., and Mendiondo, E. M. (2017). Bridging the gap between decision-making and emerging big data sources: an application of a model-based framework to disaster management in Brazil. Decis. Support Syst. 97, 12–22. doi: 10.1016/j.dss.2017.03.001
Hughes, T. P., Anderson, K. D., Connolly, S. R., Heron, S. F., Kerry, J. T., Lough, J. M., et al. (2018a). Spatial and temporal patterns of mass bleaching of corals in the Anthropocene. Science 359, 80–83. doi: 10.1126/science.aan8048
Hughes, T. P., Barnes, M. L., Bellwood, D. R., Cinner, J. E., Cumming, G. S., Jackson, J. B., et al. (2017). Coral reefs in the Anthropocene. Nature 546, 82-90. doi: 10.1038/nature22901
Hughes, T. P., Kerry, J. T., Baird, A. H., Connolly, S. R., Dietzel, A., Eakin, C. M., et al. (2018b). Global warming transforms coral reef assemblages. Nature 556, 492–496. doi: 10.1038/s41586-018-0041-2
Hughes, T. P., Kerry, J. T., and Simpson, T. (2018c). Large-scale bleaching of corals on the great barrier reef. Ecology 99:501. doi: 10.1002/ecy.2092
Hughes, T. P., Kerry, J. T., Baird, A. H., Connolly, S. R., Chase, T. J., Dietzel, A., et al. (2019). Global warming impairs stock–recruitment dynamics of corals. Nature 568, 387–390. doi: 10.1038/s41586-019-1081-y
Jones, P. J. S., Lieberknecht, L. M., and Qiu, W. (2016). Marine spatial planning in reality: introduction to case studies and discussion of findings. Mar. Policy 71, 256–264. doi: 10.1016/j.marpol.2016.04.026
Jonker, M., Johns, K., and Osborne, K. (2008). “Surveys of benthic reef communities using underwater digital photography and counts of juvenile corals,” in Long-term Monitoring of the Great Barrier Reef (Townsville, QLD: Australian Institute of Marine Science), 10. Available online at: https://www.aims.gov.au/sites/default/files/Sop%20No%2010.pdf
Joyce, K. E., Belliss, S. E., Samsonov, S. V., McNeill, S. J., and Glassey, P. J. (2009). A review of the status of satellite remote sensing and image processing techniques for mapping natural hazards and disasters. Prog. Phys. Geogr. 33, 183–207. doi: 10.1177/0309133309339563
Joyce, K. E., Duce, S., Leahy, S. M., Leon, J., and Maier, S. W. (2018). Principles and practice of acquiring drone-based image data in marine environments. Mar. Freshw. Res.. 70, 952–963. doi: 10.1071/MF17380
Kachelriess, D., Wegmann, M., Gollock, M., and Pettorelli, N. (2014). The application of remote sensing for marine protected area management. Ecol. Indicat. 36, 169–177. doi: 10.1016/j.ecolind.2013.07.003
Kahng, S. E., and Kelley, C. D. (2007). Vertical zonation of megabenthic taxa on a deep photosynthetic reef (50-140 m) in the Au’au Channel. Hawaii. Coral Reefs 26, 679–687. doi: 10.1007/s00338-007-0253-7
Kamal, M., Phinn, S., and Johansen, K. (2015). Object-based approach for multi-scale mangrove composition mapping using multi-resolution image datasets. Remote Sens. 7, 4753–4783. doi: 10.3390/rs70404753
Kenyon, S., and Stanton, D. (2017). “Designing for cost effectiveness results in responsiveness: demonstrating the SSTL X-series,” in Proceedings of the 12th Reinventing Space Conference, ed. S. Hatton (Cham: Springer International Publishing), 97–103. doi: 10.1007/978-3-319-34024-1_7
Le Nohaïc, M., Ross, C. L., Cornwall, C. E., Comeau, S., Lowe, R., McCulloch, M. T., et al. (2017). Marine heatwave causes unprecedented regional mass bleaching of thermally resistant corals in northwestern Australia. Sci. Rep. 7, 1–11. doi: 10.1038/s41598-017-14794-y
Levy, J., Hunter, C., Lukacazyk, T., and Franklin, E. C. (2018). Assessing the spatial distribution of coral bleaching using small unmanned aerial systems. Coral Reefs 37, 373–387. doi: 10.1007/s00338-018-1662-5
Li, J., Knapp, D. E., Schill, S. R., Roelfsema, C., Phinn, S., Silman, M., et al. (2019). Adaptive bathymetry estimation for shallow coastal waters using planet dove satellites. Remote Sens. Environ. 232:111302. doi: 10.1016/j.rse.2019.111302
Lough, J. M., Anderson, K. D., and Hughes, T. P. (2018). Increasing thermal stress for tropical coral reefs: 1871-2017. Sci. Rep. 8, 1–8. doi: 10.1038/s41598-018-24530-9
Marshall, P., Abdulla, A., Ibrahim, N., Naeem, R., and Basheer, A. (2017). Maldives Coral Bleaching Response Plan 2017. Malé: Maldives Marine Research Institute.
McCauley, D. J., Power, E. A., Bird, D. W., McInturff, A., Dunbar, R. B., Durham, W. H., et al. (2013). Conservation at the edges of the world. Biol. Conserv. 165, 139–145. doi: 10.1016/j.biocon.2013.05.026
Meyer, H., Reudenbach, C., Wöllauer, S., and Nauss, T. (2019). Importance of spatial predictor variable selection in machine learning applications–Moving from data reproduction to spatial prediction. Ecol. Model. 411:108815. doi: 10.1016/j.ecolmodel.2019.108815
Mora, C., Graham, N. A. J., and Nyström, M. (2016). Ecological limitations to the resilience of coral reefs. Coral Reefs 35, 1271–1280. doi: 10.1007/s00338-016-1479-z
Palumbi, S. R., Barshis, D. J., Traylor-Knowles, N., and Bay, R. A. (2014). Mechanisms of reef coral resistance to future climate change. Science 344, 895–898. doi: 10.1126/science.1251336
Phillips, D. L., and Marks, D. G. (1996). Spatial uncertainty analysis: propagation of interpolation errors in spatially distributed models. Ecol. Model. 91, 213–229. doi: 10.1016/0304-3800(95)00191-3
Planet Team (2017). Planet Application Program Interface: In Space for Life on Earth. San Francisco, CA: Planet Team, 40.
Pratchett, M. S., Thompson, C. A., Hoey, A. S., Cowman, P. F., and Wilson, S. K. (2018). “Effects of coral bleaching and coral loss on the structure and function of reef fish assemblages,” in Coral Bleaching. Ecological Studies (Analysis and Synthesis), eds M. van Oppen and J. Lough (Cham: Springer), 233, 265–293. doi: 10.1007/978-3-319-75393-5_11
Roberts, C. M., McClean, C. J., Veron, J. E., Hawkins, J. P., Allen, G. R., McAllister, D. E., et al. (2002). Marine biodiversity hotspots and conservation priorities for tropical reefs. Science 295, 1280–1284. doi: 10.1126/science.1067728
Roelfsema, C., Kovacs, E., Ortiz, J. C., Wolff, N. H., Callaghan, D., Wettle, M., et al. (2018). Coral reef habitat mapping: a combination of object-based image analysis and ecological modelling. Remote Sens. Environ. 208, 27–41. doi: 10.1016/j.rse.2018.02.005
Roelfsema, C., Lyons, M., Dunbabin, M., Kovacs, E. M., and Phinn, S. (2015). Integrating field survey data with satellite image data to improve shallow water seagrass maps: the role of AUV and snorkeller surveys? Remote Sens. Lett. 6, 135–144. doi: 10.1080/2150704X.2015.1013643
Roelfsema, C. M., and Phinn, S. R. (2010). Integrating field data with high spatial resolution multispectral satellite imagery for calibration and validation of coral reef benthic community maps. J. Appl. Remote Sens. 4:43527.
Safaie, A., Silbiger, N. J., McClanahan, T. R., Pawlak, G., Barshis, D. J., Hench, J. L., et al. (2018). High frequency temperature variability reduces the risk of coral bleaching. Nat. Commun. 9, 1–12. doi: 10.1038/s41467-018-04074-2
Sarzi-Amade, N., Bauer, T. P., Wertz, J. R., and Rufer, M. (2017). “Sprite, a very low-cost launch vehicle for small satellites,” in Proceedings of the 12th Reinventing Space Conference, ed. S. Hatton Cham: Springer International Publishing, 165–178. doi: 10.1007/978-3-319-34024-1_13
Spalding, M., Burke, L., Wood, S. A., Ashpole, J., Hutchison, J., and zu Ermgassen, P. (2017). Mapping the global value and distribution of coral reef tourism. Mar. Policy 82, 104–113. doi: 10.1016/j.marpol.2017.05.014
Strong, A. E., Arzayus, F., Skirving, W., and Heron, S. F. (2013). “Identifying coral bleaching remotely via coral reef watch – improved integration and implications for changing climate,” in Coral Reefs and Climate Change: Science and Management, eds J. T. Phinney, O. Hoegh-Guldberg, J. Kleypas, W. Skirving, and A. Strong (Washington, DC: American Geophysical Union), 163–180. doi: 10.1029/61CE10
Sun, C., Shrivastava, A., Singh, S., and Gupta, A. (2017). “Revisiting unreasonable effectiveness of data in deep learning era,” in Proceedings of the IEEE International Conference on Computer Vision, 2017, Venice, 843–852. doi: 10.1109/ICCV.2017.97
Thomas, L., López, E. H., Morikawa, M. K., and Palumbi, S. R. (2019). Transcriptomic resilience, symbiont shuffling, and vulnerability to recurrent bleaching in reef-building corals. Mol. Ecol. 28, 3371–3382.
van Oppen, M. J. H., and Lough, J. M. (2018). “Synthesis: coral bleaching: patterns, processes, causes and consequences,” in Bleaching: Patterns, Processes, Causes and Consequences, Vol. Coral, eds M. J. H. van Oppen and J. M. Lough (Berlin: Springer), 343–348. doi: 10.1007/978-3-319-75393-5_14
Vecsei, A. (2004). A new estimate of global reefal carbonate production including forereefs. Glob. Planet. Change 43, 1–18. doi: 10.1016/j.gloplacha.2003.12.002
Wang, W., Li, L., Cao, R., Chen, L., and Wei, K. (2019). Adapting climate change challenge: a new vulnerability assessment framework from the global perspective. J. Cleaner Prod. 217, 216–224. doi: 10.1016/j.jclepro.2019.01.162
Wernberg, T., Russell, B. D., Moore, P. J., Ling, S. D., Smale, D. A., Campbell, A., et al. (2011). Impacts of climate change in a global hotspot for temperate marine biodiversity and ocean warming. J. Exp. Mar. Biol. Ecol. 400, 7–16. doi: 10.1016/j.jembe.2011.02.021
Wernberg, T., Smale, D. A., Tuya, F., Thomsen, M. S., Langlois, T. J., De Bettignies, T., et al. (2013). An extreme climatic event alters marine ecosystem structure in a global biodiversity hotspot. Nat. Clim. Change 3, 78–82. doi: 10.1038/nclimate1627
Wooldridge, S. A. (2009). Water quality and coral bleaching thresholds: formalising the linkage for the inshore reefs of the Great Barrier Reef. Australia. Mar. Pollut. Bull. 58, 745–751. doi: 10.1016/j.marpolbul.2008.12.013
Keywords: coral reefs, remote sensing, drone, SST (sea surface temperature), climate change, disaster and risk management
Citation: Hickey SM, Radford B, Roelfsema CM, Joyce KE, Wilson SK, Marrable D, Barker K, Wyatt M, Davies HN, Leon JX, Duncan J, Holmes TH, Kendrick AJ, Callow JN and Murray K (2020) Between a Reef and a Hard Place: Capacity to Map the Next Coral Reef Catastrophe. Front. Mar. Sci. 7:544290. doi: 10.3389/fmars.2020.544290
Received: 20 March 2020; Accepted: 07 September 2020;
Published: 30 September 2020.
Edited by:
Michael Sweet, University of Derby, United KingdomReviewed by:
Claire M. Spillman, Bureau of Meteorology, AustraliaCopyright © 2020 Hickey, Radford, Roelfsema, Joyce, Wilson, Marrable, Barker, Wyatt, Davies, Leon, Duncan, Holmes, Kendrick, Callow and Murray. This is an open-access article distributed under the terms of the Creative Commons Attribution License (CC BY). The use, distribution or reproduction in other forums is permitted, provided the original author(s) and the copyright owner(s) are credited and that the original publication in this journal is cited, in accordance with accepted academic practice. No use, distribution or reproduction is permitted which does not comply with these terms.
*Correspondence: Sharyn M. Hickey, c2hhcnluLmhpY2tleUB1d2EuZWR1LmF1
Disclaimer: All claims expressed in this article are solely those of the authors and do not necessarily represent those of their affiliated organizations, or those of the publisher, the editors and the reviewers. Any product that may be evaluated in this article or claim that may be made by its manufacturer is not guaranteed or endorsed by the publisher.
Research integrity at Frontiers
Learn more about the work of our research integrity team to safeguard the quality of each article we publish.