- 1Key Laboratory of Vertebrate Evolution and Human Origins, Institute of Vertebrate Paleontology and Paleoanthropology, Chinese Academy of Sciences, Beijing, China
- 2Chinese Academy of Sciences (CAS) Center for Excellence in Life and Paleoenvironment, Beijing, China
- 3Section on Plant Breeding and Genetics, School of Integrative Plant Sciences, Cornell University, Ithaca, NY, United States
- 4Department of Ecology & Evolutionary Biology, University of Connecticut, Stamford, CT, United States
- 5Department of Natural Resources, Cornell University, Ithaca, NY, United States
- 6Applied Ocean Physics and Engineering, Woods Hole Oceanographic Institution, Woods Hole, MA, United States
- 7United States Department of Agriculture – Agriculture Research Service, Ithaca, NY, United States
An assessment of genetic diversity of marine populations is critical not only for the understanding and preserving natural biodiversity but also for its commercial potential. As commercial demand rises for marine resources, it is critical to generate baseline information for monitoring wild populations. Furthermore, anthropogenic stressors on the coastal environment, such as warming sea temperatures and overharvesting of wild populations, are leading to the destruction of keystone marine species such as kelps. In this study, we conducted a fine-scale genetic analysis using genome-wide high-density markers on Northwest Atlantic sugar kelp. The population structure for a total of 149 samples from the Gulf of Maine (GOM) and Southern New England (SNE) was investigated using AMOVA, FST, admixture, and PCoA. Genome-wide association analyses were conducted for six morphological traits, and the extended Lewontin and Krakauer (FLK) test was used to detect selection signatures. Our results indicate that the GOM region is more heterogeneous than SNE. These two regions have large genetic difference (between-location FST ranged from 0.21 to 0.32) and were separated by Cape Cod, which is known to be the biogeographic barrier for other taxa. We detected one significant SNP (P = 2.03 × 10–7) associated with stipe length, and 248 SNPs with higher-than-neutral differentiation. The findings of this study provide baseline knowledge on sugar kelp population genetics for future monitoring, managing and potentially restoring wild populations, as well as assisting in selective breeding to improve desirable traits for future commercialization opportunities.
Introduction
Brown macroalgae in the order Laminariales (Phaeophyceae), or kelp, are keystone species in the near-shore temperate marine environment (Dayton, 1985). As primary producers, they are at the base of many food webs and provide numerous ecosystem functions including detrital production, wave attenuation, habitat modification, and carbon sequestration (Dayton, 1985; Bartsch et al., 2008; Falkenberg et al., 2012; Efird and Konar, 2014; Trebilco et al., 2015). For humans around the globe, kelp has long provided both a food source and bioextracts with various commercial applications (Bartsch et al., 2008).
Besides their important ecological roles and the well-established kelp cultivation practices in coastal Asian countries, there is growing interest in macroalgal cultivation in Europe, South America, and throughout the United States of America (Augyte et al., 2017; Buschmann et al., 2017; Campbell et al., 2019; Grebe et al., 2019; Kim et al., 2019; Goecke et al., 2020). Specifically, there are efforts to selectively breed kelp for large-scale food and bioenergy production (Bjerregaard et al., 2016; Hwang et al., 2019; Goecke et al., 2020) and increasing demand for germplasm banking to support future cultivation as well as restoration research (Barrento et al., 2016; Wade et al., 2020). To assist in the establishment of this nascent industry in Northeastern United States, an understanding of genetic and phenotypic variation across wild kelp populations is essential. Intensive selection pressure during the marine crop domestication process leads to favoring certain phenotypic traits (Zhang et al., 2017). These mechanisms may promote adaptive divergence between cultivated seaweeds and wild populations, and it is, therefore, critical to understand wild phenotypic traits as they undergo domestication. This will foster future breeding and cultivation efforts in a sustainable and informed manner for managers, conservation groups, researchers, and industry.
Our study focused on Saccharina latissima (S. latissima), sugar kelp, which has a circumboreal distribution adapted to temperate cold water. In the Northeastern United States, its southern distributional limit is in Long Island Sound (LIS) in the Southern New England (SNE) region, with one disjunct historic population at an offshore site in New Jersey (Egan and Yarish, 1988). LIS has a coastline of roughly 176 km and ranges from New York City in the west to Fisher’s Island in the east (Mathieson and Dawes, 2017). To the north of LIS, separated by Cape Cod, is the Gulf of Maine (GOM) where a dominant coastal current flows southwestward (Pappalardo et al., 2015). Previous studies on invertebrate larval transport reveal that species boundaries exist at Cape Cod largely set by the interaction of these currents, depth distribution, and in the case of larvae, their pelagic duration (Pappalardo et al., 2015).
Although sugar kelp is a keystone species that is ecologically and commercially important, the evolutionary history of sugar kelp is not entirely known (Bolton, 2010; Starko et al., 2019). Limited research exists on the genetic and phenotypic structure of sugar kelp despite its broad range distributions and likely regional adaptation (Valero et al., 2011). Existing S. latissima populations colonized the eastern and western North Atlantic coasts post-glacially from a north Pacific source via oceanographic flow through the Arctic (Neiva et al., 2018). New evidence also suggests that sugar kelp has radiated at a constant rate (Starko et al., 2019).
Genetic population structure depends on the mode of reproduction and dispersal ability (Valero et al., 2011), and therefore provides insights about gene flow among populations (Durrant et al., 2018). In the marine environment, where direct observation of dispersal can be challenging, genetic tools provide an opportunity to better recognize patterns and scales of population connectivity (Valero et al., 2011). Nearshore kelp cultivation efforts have raised concerns by resource managers of the potential of farm cultivars to introgress with wild populations. However, for kelp species like S. latissima, dispersal distances of meiospores and gametes are generally short, usually not exceeding a few meters (Paine, 1979; Hoffmann and Santelices, 1991). This was demonstrated by Zhang et al. (2017) where genetic structure and relatedness were evaluated in eight wild populations and 17 farmed populations of S. japonica, and they observed that wild populations have not been significantly impacted by gene flow from cultivated populations.
In the Northeast United States, two small-scale studies have investigated the genetic population structure of S. latissima within small geographic ranges (Augyte et al., 2017; Breton et al., 2018). A previous study in the Gulf of Maine (GOM) reported slight genetic differentiation between five S. latissima populations spanning 225 km (Breton et al., 2018). Augyte et al. (2017) also found a slight distinction between the sugar kelp population at one site in Long Island Sound (LIS) (41° N, 72–73° W) as compared to three populations tested to the north in the GOM. However, these and other population-level genetic studies of kelp may have been limited by variation levels in amplified fragment length polymorphisms (Vos et al., 1995) and microsatellites (Richard et al., 2008; Nielsen et al., 2016; Paulino et al., 2016; Neiva et al., 2018). Improvements in the speed, cost, and accuracy of next-generation sequencing (NGS) data can now provide a better resolution for kelp genetics studies. In addition to NGS, reduced representational sequencing such as Genotyping by Sequencing (GBS) (Elshire et al., 2011) and Diversity Arrays Technology (DArT) (Jaccoud et al., 2001) have additional advantages, including reducing the genome complexity, avoiding inherent ascertainment bias in fixed SNP arrays, and decreasing sequencing costs. The high density of SNPs from these methods provides a finer understanding of the evolutionary processes shaping genetic diversity and may be informative about genes affecting complex traits in wild populations.
To aid selective breeding efforts of sugar kelp in the Northeastern United States, it is also important to understand whether variation in commercially valuable traits has a genetic basis or mostly is a result of phenotypic plasticity (Gerard and Mann, 1979; Koehl et al., 2008). Therefore, the objectives of this study were twofold: (1) to explore the finer population structure of sugar kelps in Northeastern United States and (2) to investigate phenotypic variation of commercially valuable traits and test for marker-trait associations. This study is fundamental to understanding the patterns of genetic diversity of sugar kelp in Northeastern United States and can guide future conservation efforts such as germplasm banking. This is especially important as future climate change is expected to produce significant range contractions at low-latitude ranges for several kelps including S. latissima and Laminaria digitata (Wilson et al., 2019; Neiva et al., 2020). Furthermore, our work has the potential to inform recommendations for protecting coastal marine ecosystems by identifying population structure and guiding future kelp breeding and cultivation efforts by building a baseline of knowledge about kelp population diversity and connectivity.
Materials and Methods
Sample Collection and Phenotypic Analysis
A total of 189 wild kelp samples were collected by SCUBA diving at 15 locations (Figure 1 and Table 1) throughout the Northeastern United States between April – June of 2018. Among these samples, we recorded six morphological traits on 165 samples from 15 locations. To ensure sampling consistency, only reproductive blades (i.e., blades having reached full maturity) were used for morphometric measurements. Six commercially important traits were measured: blade length, blade width at 10cm, blade width at the widest portion of the blade, blade thickness, stipe length, and stipe diameter. Collection locations were selected based on known kelp beds and ease of access (Table 1). The population sampled (defined as all 15 locations) was divided into two regions separated by Cape Cod (based on preliminary analyses), with GOM to the north of Cape Cod, and Southern New England (SNE) to the south. Within GOM, samples from one location at Giant’s Staircase were previously identified as Saccharina angustissima (S. angustissima), which is genetically closely related to S. latissima based on mitochondrial DNA and common garden experiments (Mathieson et al., 2008; Augyte et al., 2017, 2018). These samples have a distinctive strap-like morphology. Given preliminary genomic results using the data presented here, these two species are not different genetically. Therefore, we included S. angustissima in our analyses to increase the sample size and power of identifying functional SNPs. Radar plots of the mean and standard deviation of morphological traits were made to visualize diversity (Supplementary Figure 1). Furthermore, pairwise correlations of the six traits were estimated using the cor() function in R (method = “pearson,” option = “na.or.complete”). The correlation was considered statistically significant when P < 0.05.
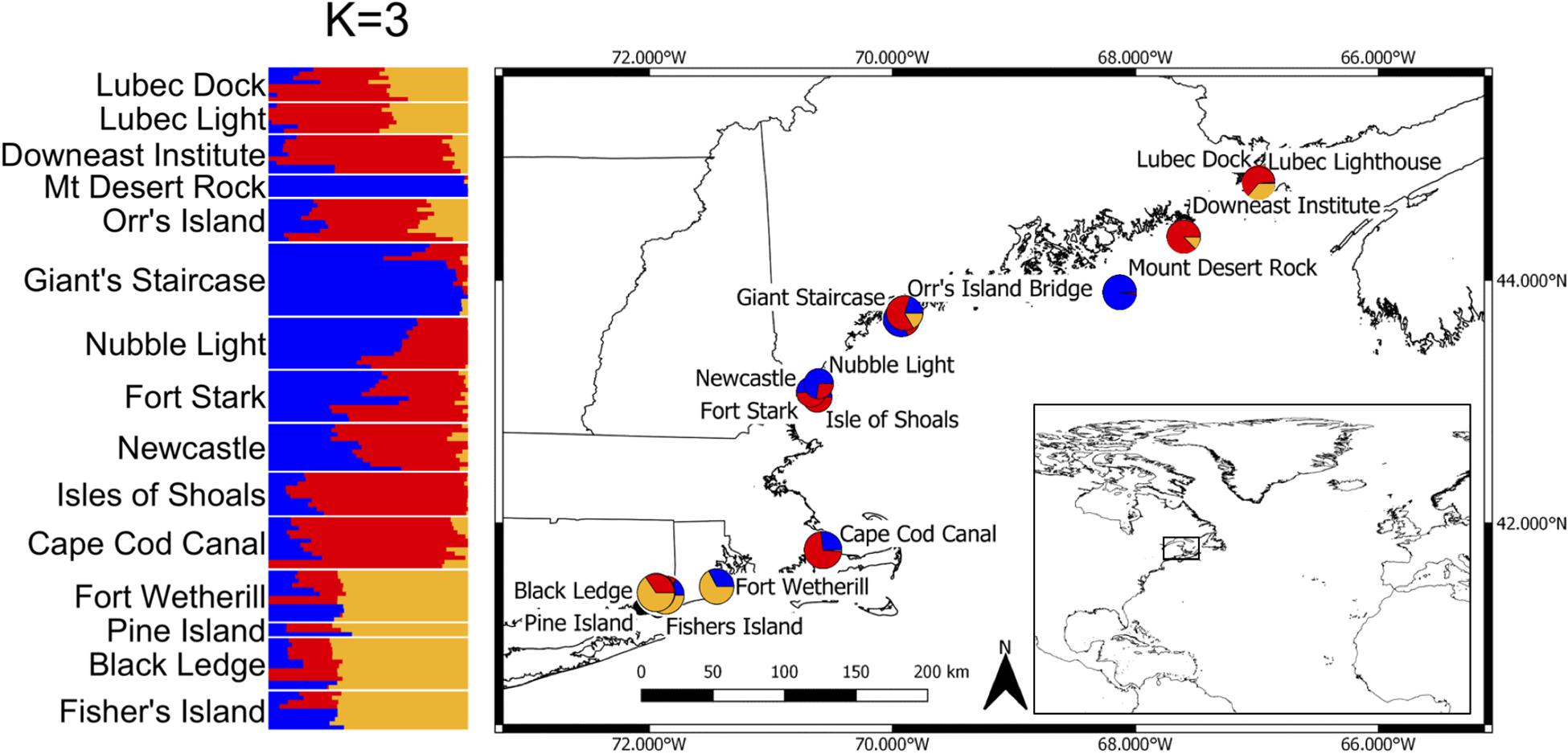
Figure 1. Collection locations of 15 subtidal populations of sugar kelp and the admixture results using conStruct for the 149 kelp samples for K = 3 (left). Admixture proportions are shown as pie plot at their approximate geographic locations (right).
DNA Extraction and Genotyping
Of the 189 collected samples from both GOM and SNE for 15 locations, 149 kelp samples were genotyped (40 samples did not produce high-quality DNA) (Table 1). Meristematic kelp blade tissue was removed and placed in silica gel to desiccate. After transport to UCONN Stamford, Marine Biotechnology Laboratory, samples were ground up with mortar and pestle using liquid nitrogen. Weights of 5 mg per sample were measured and sent to DArT Ltd. (Diversity Arrays Technology, Canberra, Australia) sequencing facility for DNA isolation and genotyping. Genomic DNA was extracted according to the modified CTAB protocol (Doyle and Doyle, 1990). Then DNA quality and quantity were evaluated with a DS-11FX series spectrophotometer (Denovix, Wilmington, DE, United States), followed by running agarose (1.2%) gel electrophoresis. Genotyping was carried out in the following steps: first, reduced genomic representations were generated following the procedures described in detail by Kilian et al. (2012) and Berry et al. (2019). This procedure included the digestion of DNA samples using a combination of PstI and HpaII restriction enzymes, ligation with site-specific adapters, and amplification of adapter-ligated fragments. The adapter-ligated fragments were amplified in 30 rounds of polymerase chain reaction (PCR) using the following reaction conditions: 94°C for 1 min, followed by 30 cycles of 94°C for 20 s, 58°C for 30 s, 72°C for 45 s, with a final extension step at 72°C for 7 min. Then, amplified fragments were sequenced using Hiseq2500 (Illumina, United States) for 77 cycles and single-end reads were generated (approximate 2,500,000 reads per sample). Next, these reads were processed and SNPs were called using proprietary DArT analytical pipelines. Two technical replicates of each sample were processed (from sequence generating to SNP calling) to guarantee the reproducibility of genotypes.
Quality Control for SNPs
A total of 20,242 SNPs were identified and five steps of quality control were applied to the SNP data: (1) Keep only biallelic SNPs; (2) Removal of SNPs with call rate (proportion with non-missing samples) less than 95%; (3) Removal of samples with call rate (proportion with non-missing SNPs) less than 90%; (4) Removal of SNPs with minor allele frequency (MAF) < 0.05; (5) Removal of SNPs severely departing from Hardy-Weinberg-Equilibrium (P < 0.01) in more than 1/4 of all collection sites, to avoid systematic genotyping errors caused by paralogous comparisons. After the quality control, 149 samples and 4,905 SNPs were retained.
Isolation by Distance
The extent of isolation by distance (IBD, Wright, 1931), which posits that populations that are geographically closer tend to be more closely related genetically, was estimated using a Mantel test implemented in the R package VEGAN (Dixon, 2003). In the Mantel test, genetic distance was represented by FST/(1 − FST), and geographical distance was calculated as “the crow flies” (straight line) between our collection sites. The Mantel test was run for GOM and SNE separately (due to clear geographic barrier of Cape Cod) with 999 permutations to assess the significance of the correlation between genetic and geographic distance.
Sequence Diversity and Population Structure
The sequence diversity for samples within each location was assessed by summary statistics of average expected heterozygosity (HE), average observed heterozygosity (HO), and inbreeding coefficient (FIS), using the R package hierfstat (Yang, 2006).
Population structure was assessed through analysis of molecular variance (AMOVA), pairwise FST, Principle Coordinate Analysis (PCoA), and admixture analysis. First, the presence of a hierarchical population structure was investigated using the AMOVA (Excoffier et al., 1992) implemented in the R package poppr (Kamvar et al., 2015). In AMOVA, hierarchical variance components were estimated in three scenarios: combining GOM and SNE locations, GOM only locations, and SNE only locations. Second, pairwise relationships among all locations were investigated by calculating pairwise FST using the R package StAMPP (Pembleton et al., 2013). The AMOVA and pairwise FST rely on geographical assignments, estimating genetic differentiation among different locations. The underlying population structure was further investigated through PCoA implemented in the R package Adegenet (Jombart, 2008). The PCoA is a model-free approach, which is commonly used to find hidden structure among samples where population is not pre-assigned. Admixture analysis was done in R using the conStruct package (Bradburd et al., 2018). Similar to other population structure analyses, this model assumes a number K of distinct ancestral populations, called “layers.” Distinct from other models, conStruct fits IBD within layers. Bradburd et al. (2018) showed this model better captures the true subpopulation structure when compared to conventional non-spatial admixture modeling. Models with different values of K are fit and a best model can be chosen, either based on its cross-validation prediction of covariances in allelic frequencies across samples or based on the contribution of layers to explaining allelic frequency covariance. Due to computational intensity in conducting the cross-validation approach, we used the latter approach. The model was initially fit using values of K = 1 to K = 10 for the full set of samples using a smaller number of iterations (8,000). Based on these results, we reduced the values of K to 1–6 for the full set using a larger number of iterations (50,000 MCMC iterations with a burn-in of 500 iterations that were discarded). The optimal K was chosen based on the Bradburd et al. (2018) method, where each additional layer to be included contributes a minimum of 1% of the total covariance (Supplementary Figure 2).
Genome-Wide Association Analyses of Morphological Traits
A genome-wide association study (GWAS) was conducted on six traits using the 4,905 SNP markers and 98 GOM plus 27 SNE samples (a common set out of the 149 genotyped and 165 phenotyped samples, Table 1). Due to the strong subpopulation structure in this dataset and to account for sampling location variation, the sampling location (15 locations) was included as a categorical fixed effect in the GWAS model using the R package GAPIT (Lipka et al., 2012; Liu et al., 2016). The kinship matrix was estimated using rrBLUP package (Endelman, 2011) with the A.mat() function and included in the GWAS model. The mixed linear model was as follows:
where y was a vector of the response variable for one of the morphological traits, 1 was a vector of ones, μ was the common population mean, b was the vector of location effects, X was an incidence matrix relating location to each individual, α was the additive allele substitution effect of the SNP, and s was the design matrix for SNPs. Elements of the vector s were allele dosages (0–2) for one randomly chosen allele at each locus. Z was an incidence matrix relating the vector of additive polygenic values u to individuals, and e was the error term. The values u and e were assumed to follow multivariate normal distributions, u∼(0, ) and e∼(0, ) respectively, where G was the genomic covariance matrix estimated by GAPIT using the kinship matrix. I was an identity matrix, the genetic variance, and the residual variance. To correct for the multiple testing, a Bonferroni correction was used. The resulting significance level threshold was 0.05/4,905 = 1.02 × 10–5. SNPs with a P-value below this threshold were significantly associated with morphological traits. We then mapped the sequence of this SNP onto the reference genome of a closely related species, S. japonica (Ye et al., 2015), due to the absence of a S. latissima reference genome.
Detection of Selection Signatures
The FLK test (Bonhomme et al., 2010) was used to test for signatures of selection. This test can identify SNPs especially with high differentiation among populations that are outliers relative to expectations under genetic drift, while accounting for the hierarchical structure among populations. We applied the FLK test to 149 genotyped samples from 15 locations which included both GOM and SNE samples. There were five steps for running the FLK analysis with detailed information described by Bonhomme et al. (2010): (1) Compute Reynolds’ genetic distance from SNP data; (2) Build rooted Neighbor-joining tree using the R package APE (Paradis et al., 2004); (3) Compute population kinship matrix from Neighbor-joining tree; (4) Compute FLK test statistic; (5) Simulate empirical distribution of FLK test statistic under the null hypothesis of neutral evolution with 50,000 replicate and return the empirical quantiles of the null distribution. FLK test statistics above 0.995 quantiles were considered to be significant. This procedure was applied to the 4,905 SNPs for all populations. Currently, there is no annotated reference genome for S. latissima. We used bwa-mem (Li, 2013) to align sequences of the outlier loci to the S. japonica reference genome, which contained 50,098 predicted genes, of which 15,017 have annotation information (Ye et al., 2015). We then checked if these mapped sequences were proximal to any annotated S. japonica genes, searching for a range of 1,000 base pairs upstream and downstream of the query sequence (Quinlan and Hall, 2010). The gene ontology (GO) terms associated with identified S. japonica genes (Ye et al., 2015) were grouped using CateGOrizer program (Hu et al., 2008).
Results
Phenotypes of Collected Samples
Large morphological variation was observed across locations for the collected samples (Supplementary Figure 1). Blade lengths ranged from 84.5 ± 37.5 cm for Pine Island to 227.4 ± 22.9 cm for Mount Desert Rock; blade widths at 10 cm ranged from 2.2 ± 0.3 cm for Giant Staircase to 24.6 ± 1.6 cm for Downeast Institute; blade widths at the widest ranged from 3.4 ± 0.3 mm for Giant Staircase to 41.4 ± 2.3 mm for Orr’s Island; stipe diameter ranged from 2.17 ± 0.61 mm for Giant Staircase to 14.43 ± 2.17 mm for Downeast Institute; stipe lengths ranged from 4.8 ± 0.6 cm for Giant Staircase to 122.7 ± 18.9 cm for Lubec Light; blade thickness ranged from 0.8 ± 0.00 mm for Pine Island to 2.28 ± 0.14 mm for Downeast Institute. Most of the pairwise correlations were positive except for the correlation between blade width at 10 cm and blade length which was -0.05 but not significantly different from zero (Figure 2). Among all positive correlations, stipe length was most highly correlated with stipe diameter (0.85), and blade width at the widest portion was most highly correlated with blade width at 10 cm (0.80).
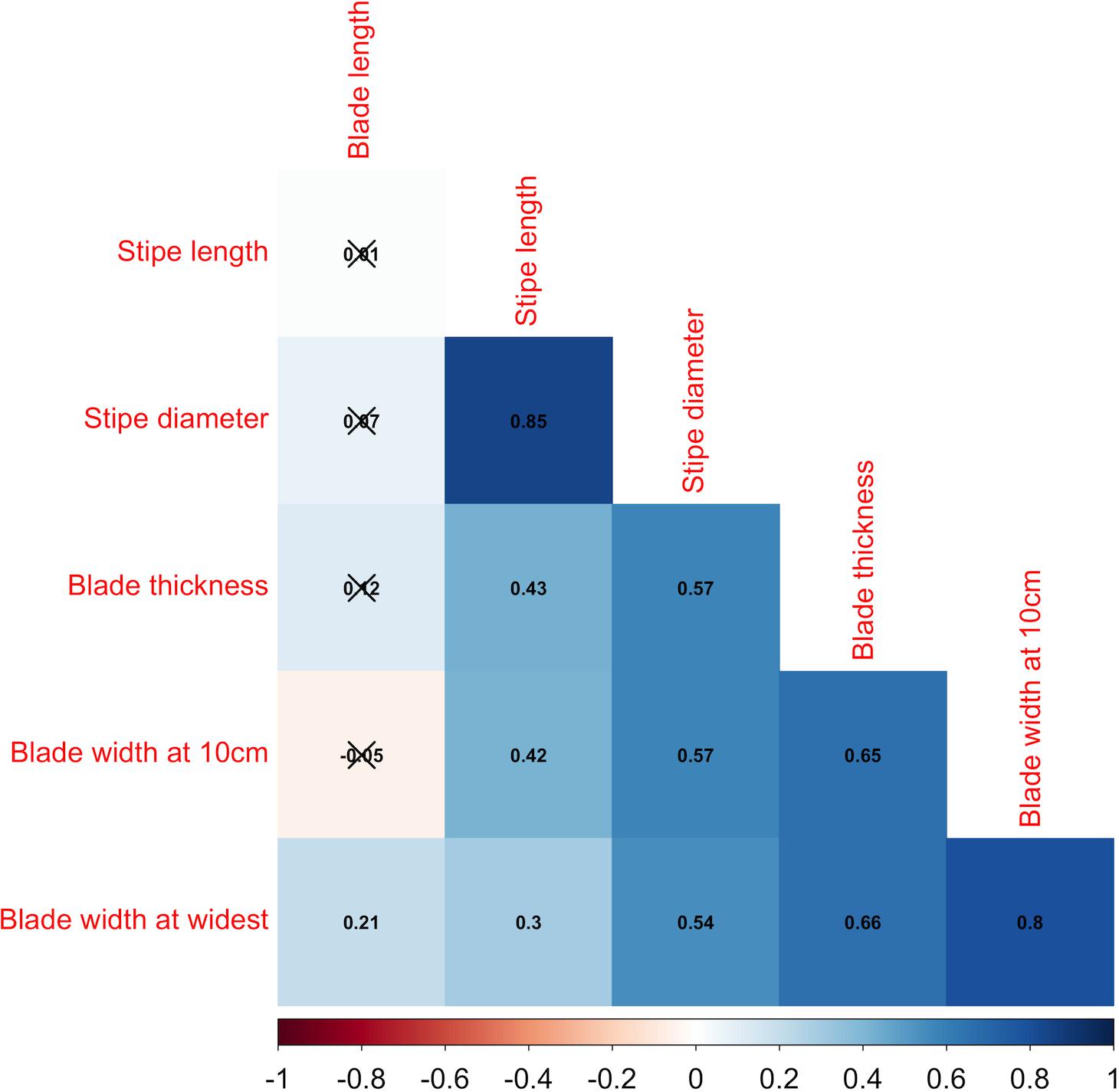
Figure 2. Phenotypic correlations among six morphological traits. The color gradient is shown at the bottom with red representing a negative correlation and blue representing a positive correlation. Non-significant (P > 0.05) correlations were marked with black crosses.
Sequence Diversity
Most locations had a slightly negative inbreeding coefficient (FIS) (Table 1). Observed heterozygosity (Ho) values ranged from 0.27 for Fisher’s Island from GOM to 0.32 for Newcastle from GOM. Pine Island from SNE had the most negative FIS and Fisher’s Island had the most positive FIS among all locations, but there were no obvious geographic trends. Overall, compared with GOM (mean observed heterozygosity = 0.31), SNE exhibited modestly lower genetic diversity (mean observed heterozygosity = 0.28, p = 0.007, t-test).
Population Structure
The AMOVA results indicate that roughly half of the total variation exists within locations (56.4%), while the least variation exists among locations within each region (14.4%, Table 2), similar to what has been reported for kelps in a review by Goecke et al. (2020). Variation among locations was higher in GOM (23.0% of the total variance, average pairwise FST = 0.13) than among locations in SNE (6.8%, FST = 0.03). Regional genetic differentiation between GOM and SNE accounted for 29.26% of overall variance (Table 2), with average inter-regional (between locations from GOM and SNE) FST = 0.26 (range from 0.21 to 0.32) (Figure 3 and Supplementary Table 1). The PCoA revealed two major clusters, dividing samples into GOM and SNE regions (Figure 4A) with clear substructure also found within the GOM region (Figure 4B). The admixture analysis using conStruct revealed three underlying ancestral populations (optimal K is 3) for all our 149 samples (Figure 1). Besides, it shows that there is a clear difference between GOM and SNE, corresponding well to the pairwise FST analysis and supported by the PCoA results (Figures 3, 4). Yet samples from SNE share some ancestry with samples from GOM.
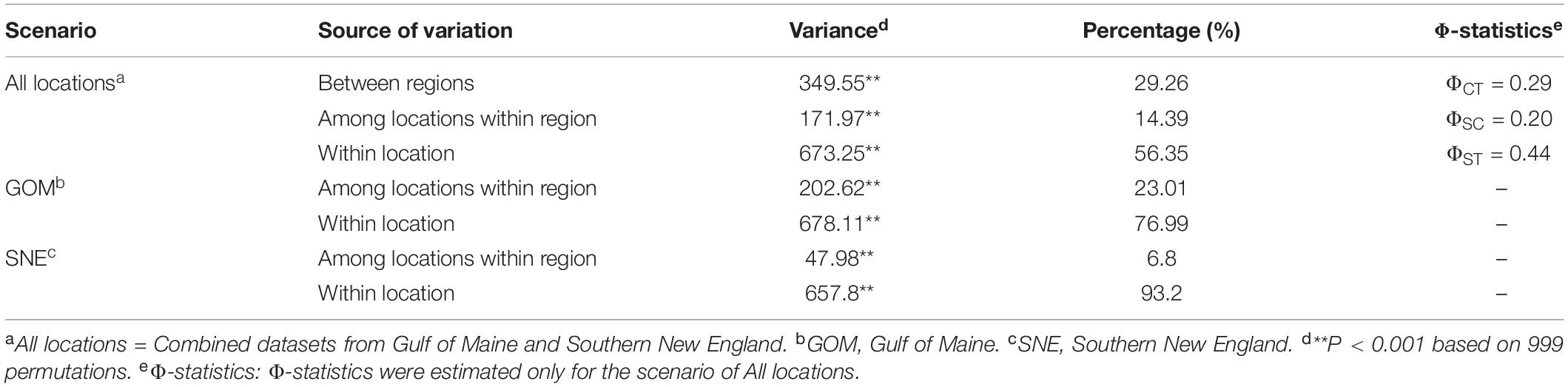
Table 2. Analysis of molecular variance for sugar kelp in Northwest Atlantic using genome-wide single nucleotide polymorphisms data.
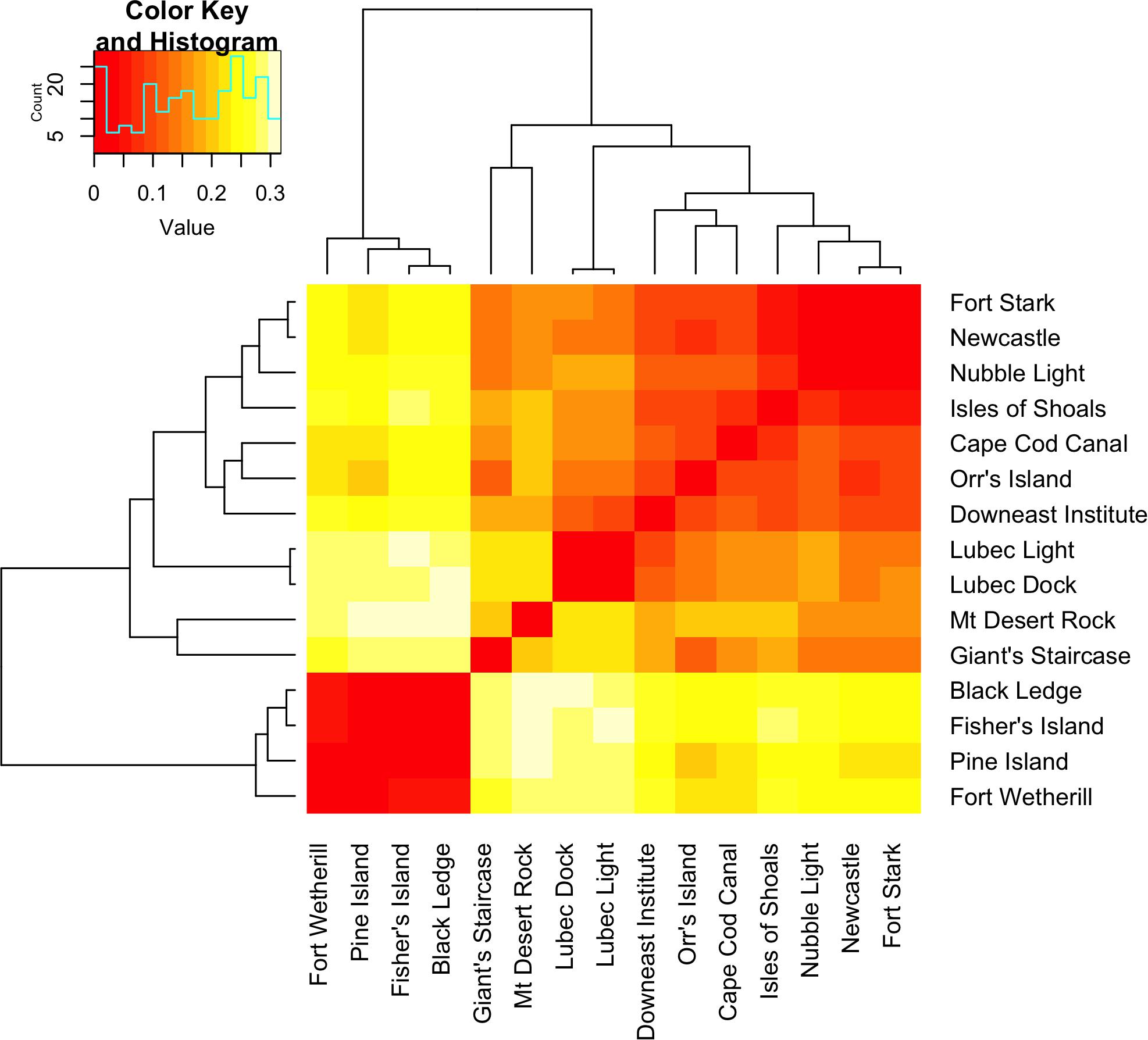
Figure 3. Heat map showing pairwise FST values with hierarchical clustering. Insert graph on the top left is a histogram showing the frequency spectrum of pairwise FST values.
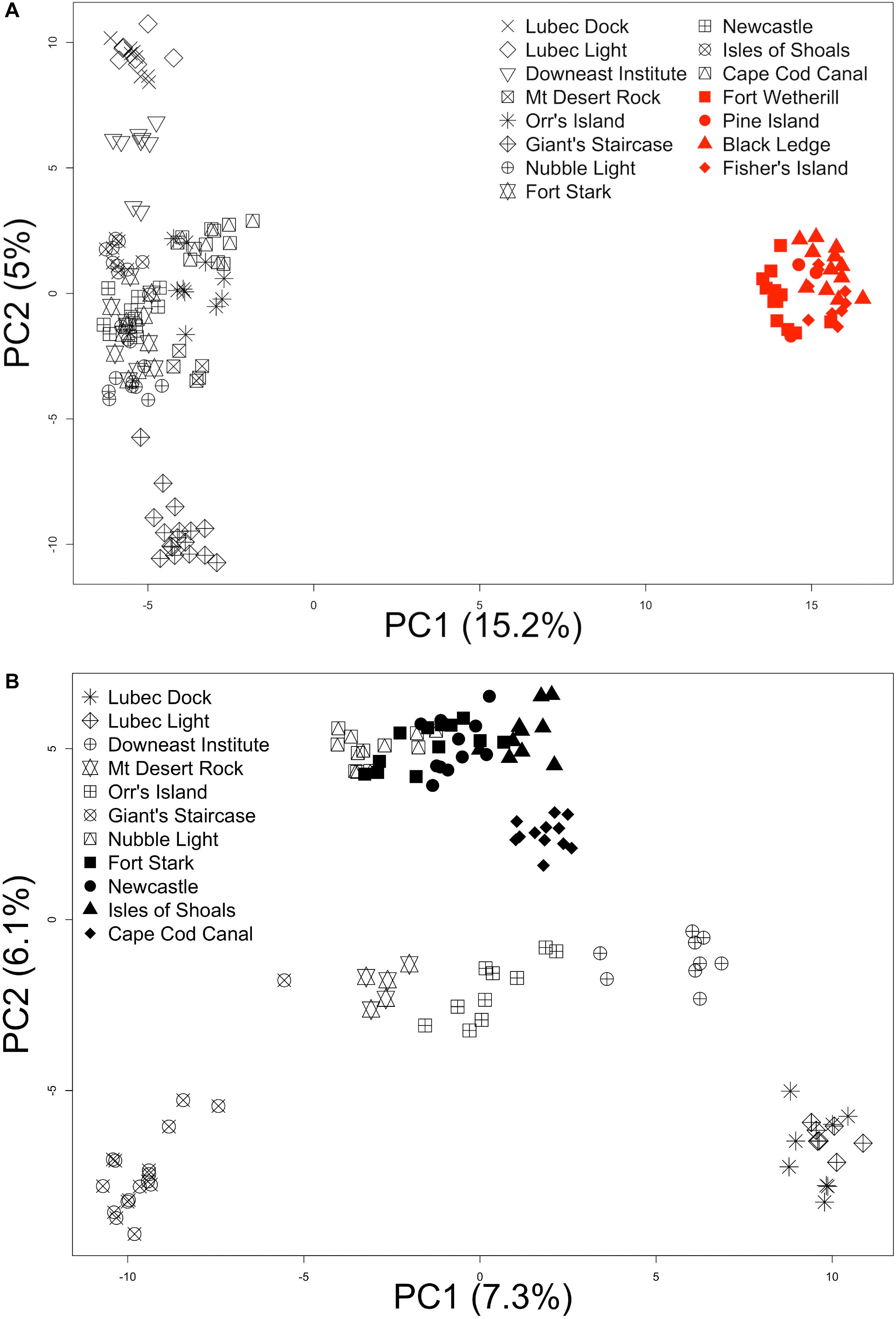
Figure 4. Principal coordinate analysis (PCoA). Samples are labeled according to their collection locations. The two PCs that explain most of the variation are shown in the plots. (A) PCoA of 149 samples from all 15 locations; (B) PCoA of 113 samples from GOM (Gulf of Maine) only.
Isolation by Distance
Testing IBD for each region separately, the Mantel tests (Figure 5) showed a moderately positive correlation for GOM (r = 0.47, P = 0.002) and a strong positive correlation for SNE (r = 0.94, P = 0.125). The strong IBD was not significant for SNE due to the small number of populations (n = 4).
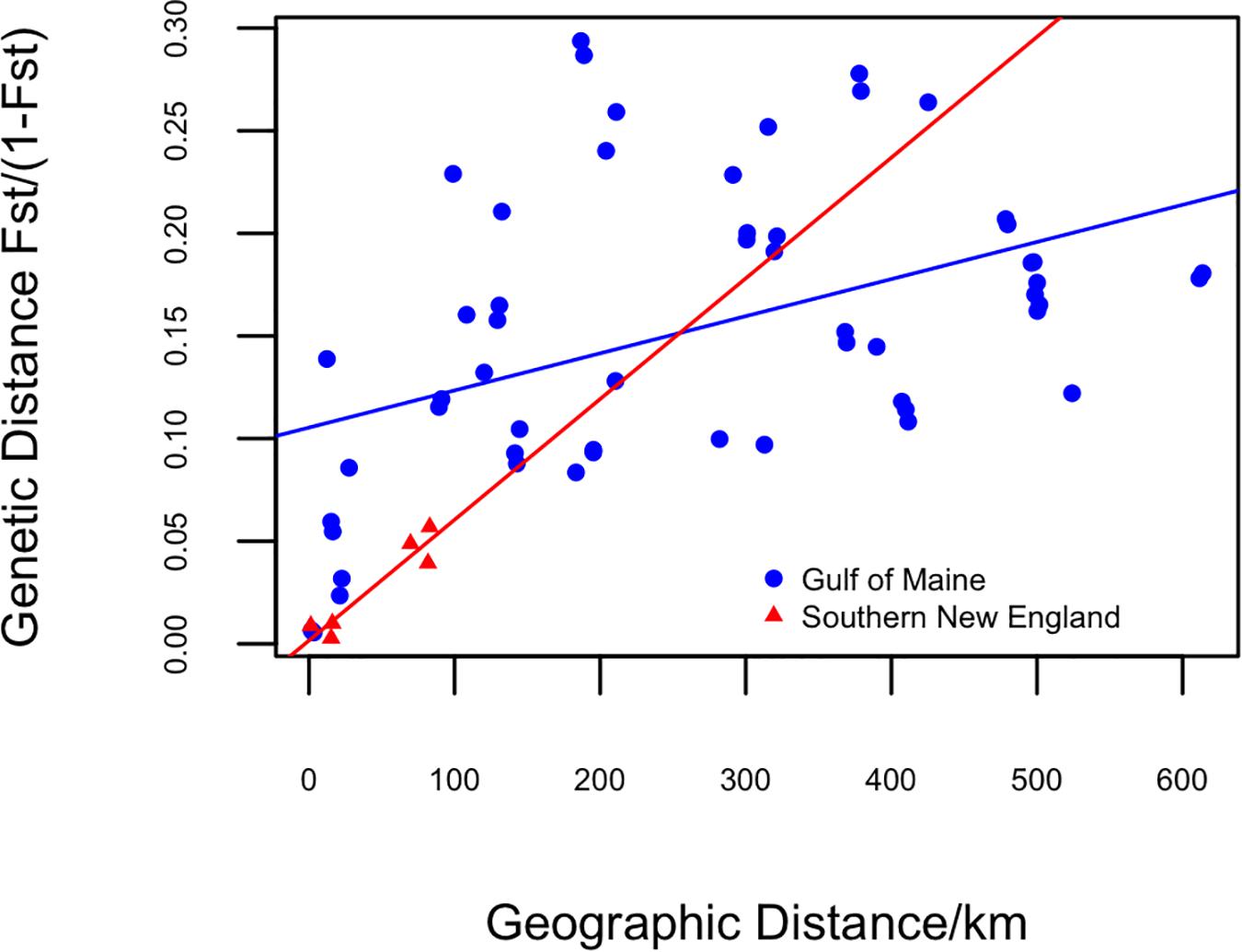
Figure 5. Mantel tests for correlation between genetic distance (y-axis) and geographic distance in kilometers (x-axis) in the Gulf of Maine and Southern New England. Regression lines are drawn for the Gulf of Maine (blue) and Southern New England (red).
Genome-Wide Association Analyses
Only one SNP (37855763-68-T/C, SNP ID assigned by DArT) was significantly associated with a trait (stipe length P = 2.03 × 10–7), with a minor allele frequency of 0.07 overall. The sequence containing this and other notable SNPs is provided in Supplementary Table 2. The sequence containing this SNP mapped to Chromosome 10 of the S. japonica genome and was not within or near a known gene (Ye et al., 2015).
Detection of Selection Signatures
FLK statistics above the 0.995 quantile were considered significant (Figure 6). A total of 248 SNPs were identified, 46% of which were fixed within the SNE region. We were able to align 154 of them onto the S. japonica reference genome and 90 occurred in functional gene regions (Supplementary Table 3). The classification of GO terms related to these genes is presented in Supplementary File S1. The top five GO terms were classified into very general functional groups such as catalytic activity in the metabolism process (other general groups included molecular function, biological process, and binding).
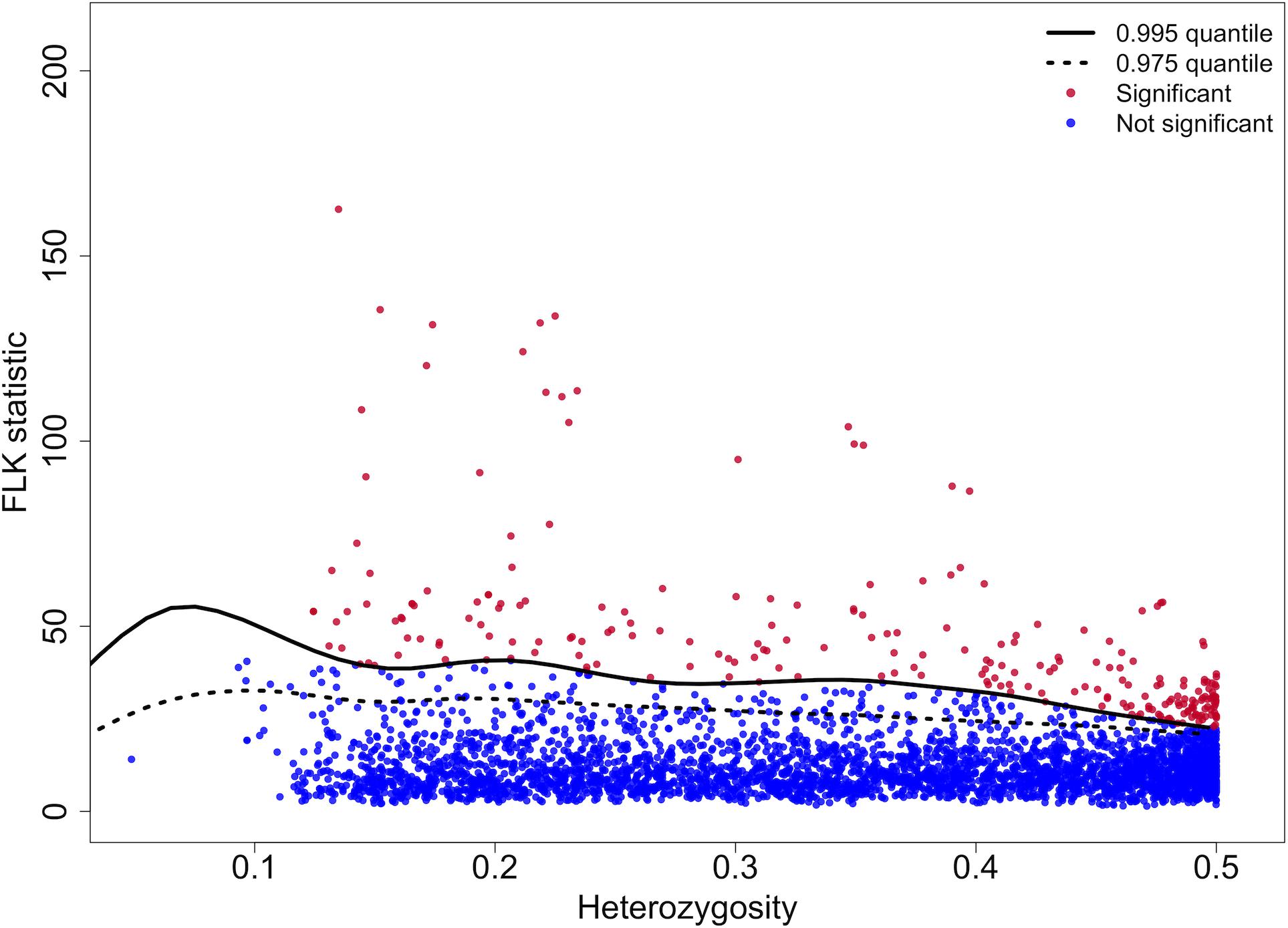
Figure 6. Genome-wide FLK test statistics and simulated 0.995 and 0.975 quantiles. The x-axis is the SNP heterozygosity, and the y-axis is the FLK statistic. The solid line indicates the significance threshold. Significant SNP outliers are highlighted with red color above the solid line, consistent with among-population differentiation above that expected from genetic drift alone.
Discussion
In this study, we investigated the genetic population structure of wild sugar kelp (Saccharina sp.) populations in the Northeastern United States using genome-wide SNP data. We found that most of the variation is geographically distributed in a pattern consistent with genetic drift with stepping stone dispersal among populations and large genetic difference between GOM and SNE. But evidence for non-neutral variation suggests that some differentiation is adaptive.
Population Structure
The major genetic distinction in the Northeastern United States region of the Northwest Atlantic was between GOM and SNE, suggesting Cape Cod acts as a biogeographic barrier for sugar kelp gene flow as it does for many other species (Pappalardo et al., 2015). Over and above what might be expected due simply to sampling asymmetries, patterns of population structure differed between these two regions. The GOM showed three times more differentiation among locations than that in SNE based on hierarchical AMOVA and pairwise FST (Table 2 and Figure 3). The difference in the genetic population structure within the GOM and SNE regions was also qualitatively confirmed by PCoA (Figure 4).
We hypothesized that the difference between the population structure of GOM and SNE might be due to different post-glacial immigration histories. Biogeographic reconstruction suggests the early expansion and diversification of complex kelp (in the order Laminariales) started in the northeast Pacific with dispersal and colonization to other regions via oceanographic flow through the Bering Strait and Arctic (Wares and Cunningham, 2001; Neiva et al., 2018; Starko et al., 2019). Divergence of western and eastern Atlantic populations predates the Last Glacial Maximum (Neiva et al., 2018) when an ice sheet extended onto the continental shelf and reached into the western Atlantic as far south as Long Island, New York (Maggs et al., 2008). North American arctic populations with genetic affinities to both the North Pacific and western Atlantic sugar kelp populations suggest recent hybridization, which also may be typical of previous interglacial periods (Neiva et al., 2018). Thus, the GOM kelp populations may have a greater degree of shared history with arctic populations than does the relatively isolated SNE population at the southern range edge (Neiva et al., 2018). However, this hypothesis needs to be tested with future studies that compare the GOM and SNE populations with kelp populations in the arctic and eastern Atlantic.
Within GOM, samples from one unique location at Giant’s Staircase were previously identified as S. angustissima, due to a distinctive strap-like morphology (Mathieson et al., 2008; Augyte et al., 2017, 2018). Here, the differentiation observed between S. angustissima (Giant’s Staircase) and S. latissima (other locations) was similar to that of S. latissima among locations (Figure 3). For example, the highest FST between S. angustissima and S. latissima (0.29) was no larger than that among S. latissima samples (highest FST 0.32). This is consistent with previous research based on mitochondrial DNA and common garden experiments suggesting that S. angustissima is genetically closely related to S. latissima (Augyte et al., 2018). These patterns motivated combining these two species for the analyses here, because the distinct phenotype found at Giants Staircase has a genetic basis, as suggested by common garden experiments (Augyte et al., 2018). Reproductive isolation of this strap-blade population is refuted by results here, or at least that isolation must be very recent.
Candidate SNPs for Morphology and Selection Signature
To our knowledge, no candidate genes have been reported for morphological traits in sugar kelp. In a closely related species, S. japonica, candidate genes have been reported for blade length and blade width (Wang et al., 2018). We found one SNP (37855763-68-T/C) significantly associated with stipe length, but its position on chromosome 10 of the S. japonica reference genome (Ye et al., 2015) was not near any recognized genes. The stage of kelp growth could potentially affect the GWAS results. We took care to only collect mature adult blades. Though some samples might represent multiple cohorts and be of different maturities, we believe that the main impact this will have on GWAS is to lower its power. It is possible that genotype and maturity could be correlated. If the correlation is across population structure, this effect will be eliminated by linear model components accounting for structure. If the correlation exists within clusters, we think this effect is a legitimate, albeit an indirect, causal mechanism and should therefore be reported as a marker-trait association. In the future, more samples should be collected and compared to an S. latissima reference genome to detect the causal loci affecting sugar kelp morphology, and to better understand the genetic architecture of traits of commercial interest.
There was no overlap between SNPs detected from GWAS and the FLK test because these tests exploit different signals in the data. As implemented here, the association analysis identified a SNP causing variation within locations, whereas the FLK test identified SNPs exceptionally divergent between locations. In addition, the association study adopted a stringent Bonferroni correction to eliminate false positives, resulting in a small set of significant SNPs. The FLK outlier loci were further assessed to potentially build confidence that they are not exclusively false positives. A large proportion of the outlier SNPs were fixed in the SNE region, but not in the GOM region. This SNE fixation might be due to stronger selection pressure in SNE than in GOM, though the small population size of the SNE region increases the chance that drift played a role. Given the current absence of a S. latissima annotated reference genome, aligning sequences against the S. japonica reference genome gave us a list of candidate genes based on existing S. japonica annotations (Supplementary File S1 and Supplementary Table 3). Note that DArT marker sequence is short (Supplementary Table 3), and it is not a complete measurement of variation in the functional gene region but is only a tag of the region. Hence given our current data, we could not draw any conclusions on the functional consequences of these outlier loci or determine if certain pathways are more involved in the adaptation process. Further research is required in this area.
Genetic Diversity in the Northeastern United States
While high-density SNP markers have been used to assess kelp genetics for S. japonica (Zhang et al., 2015), our study is the first of its kind for S. latissima in the Northeastern United States. Previously, genetic diversity in eastern Maine was assessed using 12 microsatellite markers, and samples from five intertidal locations (i.e., Penobscot Bay, Frenchman Bay, Cobscook Bay, Englishman Bay, and Starboard Cove) (Breton et al., 2018). Their range of observed heterozygosity was estimated from 0.283 to 0.339. In European sugar kelp populations, genetic diversity has been estimated with the same 12 microsatellite markers and reported much higher observed heterozygosity at 0.560 (Nielsen et al., 2016). Lower genetic diversity in western North Atlantic benthic taxa relative to Europe has also been reported and hypothesized to result from post-glacial recolonization from east to west (Wares and Cunningham, 2001). In the context of these previous studies, our conStruct results indicate that there is greater complexity than would be expected from a simple IBD pattern of evolution with Cape Cod fragmentation. This raised hypotheses that admixture during post-glacial recolonization events and divergence could be the geographic pattern of genetic differentiation observed. However, we do not have enough evidence given our current data set. These hypotheses require further validation with genome-wide genetic markers from eastern Atlantic and the inference of demographic history.
Compared with GOM (mean observed heterozygosity = 0.31), SNE exhibited modestly lower genetic diversity (mean observed heterozygosity = 0.28, p = 0.007, t-test). If this difference reflects equilibrium effective population size it could be due to either smaller census population sizes in SNE populations at the range limit, or to greater gene flow connectivity among GOM populations than in the SNE. In the future, genome-wide SNP data should be applied to sugar kelp genetic diversity globally to help infer demographic histories as a context within which the role of selection can be inferred. Understanding the mechanisms generating variation in effective population size will be important for predicting adaptive capacity of sugar kelp in response to predict climate change (Hoffmann and Sgrò, 2011; Wilson et al., 2019).
In conclusion, the vicariance caused by Cape Cod in the Northeastern United States has led to ecological diversification and separation of S. latissima gene pools in the GOM and SNE subpopulations. While these results need to be placed into a larger global context, several patterns have clearly emerged. Specifically, the Cape Cod barrier was shown to be a longstanding barrier to gene flow. It is notable that despite this deep regional population structure, the genetic variation found within locations accounted for the greatest proportion of the total, indicating abundant local standing diversity for selection to act on. Breeding samples across these two regions is currently prohibited due to conservation concerns. Nonetheless, similar to other studies (Evankow et al., 2019), our recommendation supports the precautionary principle to only use regional ecotypes for cultivation, and to not interbreed kelp strains separated by Cape Cod. We also reveal a novel SNP association with a morphological trait and additional polymorphisms that may have been subject to selection in sugar kelp. Our study can be used to guide conservation and management decisions as well as future kelp breeding research.
Data Availability Statement
The datasets generated for this study can be found in the online repositories. The names of the repository/repositories and accession number(s) can be found below: NCBI BioProject (accession: PRJNA638172).
Author Contributions
XM, SA, and MH wrote the manuscript, discussed results, and revised the manuscript together. XM and MH performed the analysis. MPH guided population genetic analyses and edited the manuscript. CY offered guidance on collections. SA, DB, and SL collected samples. DB, SL, SA, SU, and MM-R phenotyped the samples. KR guided analyses, provided computational resources, and edited the manuscript. SL, CY, and J-LJ led the project and edited the manuscript. All authors read and approved the manuscript.
Conflict of Interest
The authors declare that the research was conducted in the absence of any commercial or financial relationships that could be construed as a potential conflict of interest.
Acknowledgments
We acknowledge funding support from the U.S. Depaertment of Energy ARPA-E (DE-AR0000915), and the Massachusetts Clean Energy Center (AmplifyMass). XM benefited from the Strategic Priority Research Program (B) (XDB26000000) of CAS during revision process. We acknowledge Christina Marie Rochus for suggestions on the data analyses. We thank Jeff Glaubitz from Cornell Institute of Biotechnology for consultation on bioinformatics. This work has been published as a reprint in bioRxiv (Mao et al., 2020, https://www.biorxiv.org/content/10.1101/2020.04.21.050930v1).
Supplementary Material
The Supplementary Material for this article can be found online at: https://www.frontiersin.org/articles/10.3389/fmars.2020.00694/full#supplementary-material
References
Augyte, S., Lewis, L., Lin, S., Neefus, C. D., and Yarish, C. (2018). Speciation in the exposed intertidal zone: the case of Saccharina angustissima comb. nov. & stat. nov. (Laminariales, Phaeophyceae). Phycologia 57, 100–112. doi: 10.2216/17-40.1
Augyte, S., Yarish, C., Redmond, S., and Kim, J. K. (2017). Cultivation of a morphologically distinct strain of the sugar kelp, Saccharina latissima forma angustissima, from coastal Maine, USA, with implications for ecosystem services. J. Appl. Phycol. 29, 1967–1976. doi: 10.1007/s10811-017-1102-x
Barrento, S., Camus, C., Sousa-Pinto, I., and Buschmann, A. H. (2016). Germplasm banking of the giant kelp: our biological insurance in a changing environment. Algal Res. 13, 134–140. doi: 10.1016/j.algal.2015.11.024
Bartsch, I., Wiencke, C., Bischof, K., Buchholz, C. M., Buck, B. H., Eggert, A., et al. (2008). The genus Laminaria sensu lato: recent insights and developments. Eur. J. Phycol. 43, 1–86. doi: 10.1080/09670260701711376
Berry, O., Richards, Z., Moore, G., Hernawan, U., Travers, M., and Gruber, B. (2019). Oceanic and coastal populations of a harvested macroinvertebrate Rochia nilotica in north-western Australia are isolated and may be locally adapted. Mar. Freshwater Res. 71, 782–793. doi: 10.1071/MF19172
Bjerregaard, R., Valderrama, D., Sims, N., Radulovich, R., Diana, J., Capron, M., et al. (2016). Seaweed Aquaculture for Food Security, Income Generation and Environmental Health Security in Tropical Developing Countries. Washington, DC: World Bank Group.
Bolton, J. J. (2010). The biogeography of kelps (Laminariales, Phaeophyceae): a global analysis with new insights from recent advances in molecular phylogenetics. Helgoland Mar. Res. 64, 263–279. doi: 10.1007/s10152-010-0211-6
Bonhomme, M., Chevalet, C., Servin, B., Boitard, S., Abdallah, J., Blott, S., et al. (2010). Detecting selection in population trees: the Lewontin and Krakauer test extended. Genetics 186, 241–262. doi: 10.1534/genetics.110.117275
Bradburd, G. S., Coop, G. M., and Ralph, P. L. (2018). Inferring continuous and discrete population genetic structure across space. Genetics 210, 33–52. doi: 10.1534/genetics.118.301333
Breton, T. S., Nettleton, J. C., O’Connell, B., and Bertocci, M. (2018). Fine-scale population genetic structure of sugar kelp, Saccharina latissima (Laminariales, Phaeophyceae), in eastern Maine, USA. Phycologia 57, 32–40. doi: 10.2216/17-72.1
Buschmann, A., Camus, C., Infante, J., Neori, A., Israel, Á, Hernández-González, M. C., et al. (2017). Seaweed production: overview of the global state of exploitation, farming and emerging research activity. Eur. J. Phycol. 4, 391–406. doi: 10.1080/09670262.2017.1365175
Campbell, I., Macleod, A., Sahlmann, C., Neves, L., Funderud, J., Øverland, M., et al. (2019). The environmental risks associated with the development of seaweed farming in Europe - prioritizing key knowledge gaps. Front. Mar. Sci. 6:107. doi: 10.3389/fmars.2019.00107
Dayton, P. K. (1985). Ecology of kelp communities. Annu. Rev. Ecol. Syst. 16, 215–245. doi: 10.1146/annurev.es.16.110185.001243
Dixon, P. (2003). VEGAN, a package of R functions for community ecology. J. Veg. Sci. 14, 927–930. doi: 10.1111/j.1654-1103.2003.tb02228.x
Durrant, H. M. S., Barrett, N. S., Edgar, G. J., Coleman, M. A., and Burridge, C. P. (2018). Seascape habitat patchiness and hydrodynamics explain genetic structuring of kelp populations. Mar. Ecol. Prog. Ser. 587, 81–92. doi: 10.3354/meps12447
Efird, T. P., and Konar, B. (2014). Habitat characteristics can influence fish assemblages in high latitude kelp forests. Environ. Biol. Fishes 97, 1253–1263. doi: 10.1007/s10641-013-0211-x
Egan, B., and Yarish, C. (1988). The distribution of the genus Laminaria (Phaeophyta) at its southern limit in the Western Atlantic Ocean. Bot. Mar. 31, 155–161.
Elshire, R. J., Glaubitz, J. C., Sun, Q., Poland, J. A., Kawamoto, K., Buckler, E. S., et al. (2011). A robust, simple genotyping-by-sequencing (GBS) approach for high diversity species. PLoS One 6:e19379. doi: 10.1371/journal.pone.0019379
Endelman, J. B. (2011). Ridge regression and other kernels for genomic selection with R package rrBLUP. Plant Genome J. 4:250. doi: 10.3835/plantgenome2011.08.0024
Evankow, A., Christie, H., Hancke, K., Brysting, A. K., Junge, C., Fredriksen, S., et al. (2019). Genetic heterogeneity of two bioeconomically important kelp species along the Norwegian coast. Conserv. Genet. 20, 615–628. doi: 10.1007/s10592-019-01162-8
Excoffier, L., Smouse, P. E., and Quattro, J. M. (1992). Analysis of molecular variance inferred from metric distances among DNA haplotypes: application to human mitochondrial DNA restriction data. Genetics 131, 479–491.
Falkenberg, L. J., Russell, B. D., and Connell, S. D. (2012). Stability of strong species interactions resist the synergistic effects of local and global pollution in kelp forests. PLoS One 7:e0033841. doi: 10.1371/journal.pone.0033841
Gerard, V. A., and Mann, K. H. (1979). Growth and production of Laminaria longicruris (Phaeophyta) populations exposed to different intensities of water movement. J. Phycol., 15, 33–41. doi: 10.1111/j.1529-8817.1979.tb02958.x
Goecke, F., Klemetsdal, G., and Ergon, Å (2020). Cultivar development of kelps for commercial cultivation - past lessons and future prospects. Front. Mar. Sci. 8:110. doi: 10.3389/fmars.2020.00110
Grebe, G., Byron, C. J., St. Gelais, A., Kotowicz, D. M., and Olson, T. K. (2019). An ecosystem approach to kelp aquaculture in the Americas and Europe. Aquat. Rep. 15:100215. doi: 10.1016/j.aqrep.2019.100215
Hoffmann, A. A., and Sgrò, C. M. (2011). Climate change and evolutionary adaptation. Nature 470, 479–485.
Hoffmann, A. J., and Santelices, B. (1991). Banks of algal microscopic forms: hypotheses on their functioning and comparisons with seed banks. Mar. Ecol. Prog. Ser. 79, 185–194. doi: 10.3354/meps079185
Hu, Z.-L., Bao, J., and Reecy, J. M. (2008). CateGOrizer: a web-based program to batch analyze gene ontology classification categories. Online J. Bioinformat. 9, 108–112.
Hwang, E. K., Yotsukura, N., Pang, S. J., Su, L., and Shan, T. F. (2019). Seaweed breeding programs and progress in eastern Asian countries. Phycologia 58, 484–495. doi: 10.1080/00318884.2019.1639436
Jaccoud, D., Peng, K., Feinstein, D., and Kilian, A. (2001). Diversity arrays: a solid state technology for sequence information independent genotyping. Nucleic Acids Res. 29:e25.
Jombart, T. (2008). Adegenet: a R package for the multivariate analysis of genetic markers. Bioinformatics 24, 1403–1405. doi: 10.1093/bioinformatics/btn129
Kamvar, Z. N., Brooks, J. C., and Grünwald, N. J. (2015). Novel R tools for analysis of genome-wide population genetic data with emphasis on clonality. Front. Genet. 6:208. doi: 10.3389/fgene.2015.00208
Kilian, A., Wenzl, P., Huttner, E., Carling, J., Xia, L., Blois, H., et al. (2012). Diversity arrays technology: a generic genome profiling technology on open platforms. Methods Mol. Biol. 888, 67–89. doi: 10.1007/978-1-61779-870-2_5
Kim, J. K., Stekoll, M., and Yarish, C. (2019). Opportunities, challenges, and future directions of open-water seaweed aquaculture in the United States. Phycologia 58, 446–461. doi: 10.1080/00318884.2019.1625611
Koehl, M. A. R., Silk, W. K., Liang, H., and Mahadevan, L. (2008). How kelp produce blade shapes suited to different flow regimes: a new wrinkle. Integr. Comp. Biol. 48, 834–851. doi: 10.1093/icb/icn069
Li, H. (2013). Aligning sequence reads, clone sequences and assembly contigs with BWA-MEM. Genomics 1303:3997.
Lipka, A. E., Tian, F., Wang, Q., Peiffer, J., Li, M., Bradbury, P. J., et al. (2012). GAPIT: genome association and prediction integrated tool. Bioinformatics 28, 2397–2399. doi: 10.1093/bioinformatics/bts444
Liu, X., Huang, M., Fan, B., Buckler, E. S., and Zhang, Z. (2016). Iterative usage of fixed and random effect models for powerful and efficient Genome-Wide Association Studies. PLoS Genet.:e1005767. doi: 10.1371/journal.pgen.1005767
Maggs, C. A., Castilho, R., Foltz, D., Henzler, C., Jolly, M. T., Kelly, J., et al. (2008). Evaluating signatures of glacial refugia for north atlantic benthic marine taxa. Ecology 89, S108–S122. doi: 10.1890/08-0257.1
Mao, X., Augyte, S., Huang, M., Hare, M. P., Bailey, D., Umanzor, S., et al. (2020). Population genetics of sugar kelp in the Northwest Atlantic region using genome-wide markers. bioRxiv [Preprint]. doi: 10.1101/2020.04.21.050930
Mathieson, A. C., and Dawes, C. J. (2017). Seaweeds of the Northwest Atlantic. Amherst, MA: University of Massachusetts Press.
Mathieson, A. C., Hehre, E. J., Dawes, C. J., and Neefus, C. D. (2008). An historical comparison of seaweed populations from Casco Bay. Maine. Rhodora 110, 1–102. doi: 10.3119/06-23.1
Neiva, J., Paulino, C., Nielsen, M. M., Krause-Jensen, D., Saunders, G. W., Assis, J., et al. (2018). Glacial vicariance drives phylogeographic diversification in the amphi-boreal kelp Saccharina latissima. Sci. Rep. 8:1112. doi: 10.1038/s41598-018-19620-7
Neiva, J., Serrão, E. A., Paulino, C., Gouveia, L., Want, A., Tamigneaux, E., et al. (2020). Genetic structure of amphi-Atlantic Laminaria digitata (Laminariales, Phaeophyceae) reveals a unique range-edge gene pool and suggests post-glacial colonization of the NW Atlantic. Eur. J. Phycol. doi: 10.1080/09670262.2020.1750058
Nielsen, M. M., Paulino, C., Neiva, J., Krause-Jensen, D., Bruhn, A., and Serrao, E. A. (2016). Genetic diversity of Saccharina latissima (Phaeophyceae) along a salinity gradient in the North Sea-Baltic Sea transition zone. J. Phycol. 52, 523–531. doi: 10.1111/jpy.12428
Paine, R. T. (1979). Disaster, catastrophe, and local persistence of the sea palm Postelsia palmaeformis. Science 205, 685–687. doi: 10.1126/science.205.4407.685
Pappalardo, P., Pringle, J. M., Wares, J. P., and Byers, J. E. (2015). The location, strength, and mechanisms behind marine biogeographic boundaries of the east coast of North America. Ecography 38, 722–731. doi: 10.1111/ecog.01135
Paradis, E., Claude, J., and Strimmer, K. (2004). APE: analyses of phylogenetics and evolution in R language. Bioinformatics 20, 289–290. doi: 10.1093/bioinformatics/btg412
Paulino, C., Neiva, J., Coelho, N. C., Aires, T., Marbà, N., Krause-Jensen, D., et al. (2016). Characterization of 12 polymorphic microsatellite markers in the sugar kelp Saccharina latissima. J. Appl. Phycol. 28, 3071–3074. doi: 10.1007/s10811-016-0811-x
Pembleton, L. W., Cogan, N. O. I., and Forster, J. W. (2013). StAMPP: an R package for calculation of genetic differentiation and structure of mixed-ploidy level populations. Mol. Ecol. Resour. 13, 946–952. doi: 10.1111/1755-0998.12129
Quinlan, A. R., and Hall, I. M. (2010). BEDTools: a flexible suite of utilities for comparing genomic features. Bioinformatics 26, 841–842. doi: 10.1093/bioinformatics/btq033
Richard, G. F., Kerrest, A., and Dujon, B. (2008). Comparative genomics and molecular dynamics of DNA repeats in eukaryotes. Microbiol. Mol. Biol. Rev. 72, 686–727. doi: 10.1128/mmbr.00011-08
Starko, S., Soto Gomez, M., Darby, H., Demes, K. W., Kawai, H., Yotsukura, N., et al. (2019). A comprehensive kelp phylogeny sheds light on the evolution of an ecosystem. Mol. Phylo. Evol. 136, 138–150. doi: 10.1016/j.ympev.2019.04.012
Trebilco, R., Dulvy, N. K., Stewart, H., and Salomon, A. K. (2015). The role of habitat complexity in shaping the size structure of a temperate reef fish community. Mar. Ecol. Prog. Ser. 532, 197–211. doi: 10.3354/meps11330
Valero, M., Destombe, C., Mauger, S., Ribout, C., and Enge, C. R. (2011). Using genetic tools for sustainable management of kelps: a literature review and the example of Laminaria digitata. Cah. Biol. Mar. 52, 467–483.
Vos, P., Hogers, R., Bleeker, M., Reijans, M., van de Lee, T., Hornes, M., et al. (1995). AFLP: a new technique for DNA fingerprinting. Nucleic Acids Res. 23, 4407–4414. doi: 10.1093/nar/23.21.4407
Wade, R., Augyte, S., Harden, M., Nuzhdin, S., Yarish, C., and Alberto, F. (2020). Macroalgal germplasm banking for conservation, food security, and industry. PLoS Biol. 18:e3000641. doi: 10.1371/journal.pbio.3000641
Wang, X., Chen, Z., Li, Q., Zhang, J., Liu, S., and Duan, D. (2018). High-density SNP-based QTL mapping and candidate gene screening for yield-related blade length and width in Saccharina japonica (Laminariales, Phaeophyta). Sci. Rep. 8:13591.
Wares, J. P., and Cunningham, C. W. (2001). Phylogeography and historical ecology of the North Atlantic intertidal. Evolution 55, 2455–2469. doi: 10.1111/j.0014-3820.2001.tb00760.x
Wilson, K. L., Skinner, M. A., and Lotze, H. K. (2019). Projected 21st-century distribution of canopy-forming seaweeds in the Northwest Atlantic with climate change. Divers. Distrib. 25, 582–602. doi: 10.1111/ddi.12897
Yang, R. C. (2006). Estimating hierarchical f-statistics. Evolution 52, 950–956. doi: 10.2307/2411227
Ye, N., Zhang, X., Miao, M., Fan, X., Zheng, Y., Xu, D., et al. (2015). Saccharina genomes provide novel insight into kelp biology. Nat. Commun. 6:6986. doi: 10.1038/ncomms7986
Zhang, J., Wang, X., Yao, J., Li, Q., Liu, F., Yotsukura, N., et al. (2017). Effect of domestication on the genetic diversity and structure of Saccharina japonica populations in China. Sci. Rep. 4, 1–11. doi: 10.1038/srep42158
Keywords: Saccharina latissima, population structure, genome-wide analysis, cultivation, Northeastern United States
Citation: Mao X, Augyte S, Huang M, Hare MP, Bailey D, Umanzor S, Marty-Rivera M, Robbins KR, Yarish C, Lindell S and Jannink J-L (2020) Population Genetics of Sugar Kelp Throughout the Northeastern United States Using Genome-Wide Markers. Front. Mar. Sci. 7:694. doi: 10.3389/fmars.2020.00694
Received: 16 April 2020; Accepted: 30 July 2020;
Published: 21 August 2020.
Edited by:
Sophie von der Heyden, Stellenbosch University, South AfricaReviewed by:
Jin Sun, Hong Kong University of Science and Technology, Hong KongZi-Min Hu, Institute of Oceanology (CAS), China
Romina Henriques, Stellenbosch University, South Africa
Copyright © 2020 Mao, Augyte, Huang, Hare, Bailey, Umanzor, Marty-Rivera, Robbins, Yarish, Lindell and Jannink. This is an open-access article distributed under the terms of the Creative Commons Attribution License (CC BY). The use, distribution or reproduction in other forums is permitted, provided the original author(s) and the copyright owner(s) are credited and that the original publication in this journal is cited, in accordance with accepted academic practice. No use, distribution or reproduction is permitted which does not comply with these terms.
*Correspondence: Mao Huang, mh865@cornell.edu
†These authors have contributed equally to this work
‡Present address: Xiaowei Mao, Key Laboratory of Vertebrate Evolution and Human Origins, Institute of Vertebrate Paleontology and Paleoanthropology, Chinese Academy of Sciences, Beijing, China; CAS Center for Excellence in Life and Paleoenvironment, Beijing, China