- 1Maurice Lamontagne Institute, Fisheries and Oceans Canada, Mont-Joli, QC, Canada
- 2Institut des Sciences de la Mer de Rimouski, Québec-Océan – Université du Québec à Rimouski, Rimouski, QC, Canada
- 3Pacific Biological Station, Fisheries and Oceans Canada, Nanaimo, BC, Canada
- 4Centre d’Etudes Biologiques de Chizé, UMR 7372 CNRS – Université de la Rochelle, Villiers-en-Bois, France
Blue whale survival and fitness are highly contingent on successful food intake during an intense feeding season. Factors affecting time spent at the surface or at depth in a prey patch are likely to alter foraging effort, net energy gain, and fitness. We specifically examined the energetic consequences of a demonstrated reduction in dive duration caused by vessel proximity, and of krill density reductions potentially resulting from krill exploitation or climate change. We estimated net energy gain over a simulated 10-h foraging bout under baseline conditions, and three scenarios, reflecting krill density reductions, vessel interactions of different amplitudes, and their combined effects. Generally, the magnitude of the effects increased with that of krill density reductions and duration of vessel proximity. They were also smaller when peak densities were more accessible, i.e., nearer to the surface. Effect size from a reduction in krill density on net energy gain were deemed small to moderate at 5% krill reduction, moderate to large at 10% reduction, and large at 25 and 50% reductions. Vessels reduced cumulated net energy gain by as much as 25% when in proximity for 3 of a 10-h daylight foraging period, and by up to 47–85% when continuously present for 10 h. The impacts of vessel proximity on net energy gain increased with their duration. They were more important when whales were precluded from reaching the most beneficial peak densities, and when these densities were located at deeper depths. When krill densities were decreased by 5% or more, disturbing foraging blue whales for 3 h could reduce their net energy gain by ≥30%. For this endangered western North Atlantic blue whale population, a decrease in net energy gain through an altered krill preyscape or repeated vessel interactions is of particular concern, as this species relies on a relatively short feeding season to accumulate energy reserves and to fuel reproduction. This study highlights the importance of distance limits during whale-watching operations to ensure efficient feeding, as well as the vulnerability of this specialist to fluctuations in krill densities.
Introduction
Sufficient food provisioning is essential to the growth, survival, and reproduction of individuals (Emlen, 1966; Pyke et al., 1977). In capital breeders and other migrating species, the vast majority of lipid accumulation relies on a spatiotemporally narrow window of food abundance. Accumulated energy reserves are then used for their yearly needs to sustain the individual during reproduction and periods of fasting or reduced food availability (Houston et al., 2007). Rorqual whales are capital breeders that adopt this strategy, although sporadic feeding has been documented during migration when suitable areas are encountered (McWilliams et al., 2004; Skagen, 2006; Silva et al., 2013; Owen et al., 2016). Rorquals feed on dense aggregations of zooplankton or small schooling fish using a distinct feeding strategy called lunge filter feeding (Goldbogen et al., 2011). However, the high energetic cost of lunge feeding constrains rorquals to target dense prey patches to maintain high foraging efficiency and allow for fat accumulation (Goldbogen et al., 2011; Guilpin et al., 2019).
The blue whale, Balaenoptera musculus, is the largest of the rorquals. This species is ubiquitous to all oceans and feeds almost exclusively on euphausiids or krill, a keystone species of pelagic food webs that is heterogeneously distributed in space and time (Kawamura, 1980; Mauchline and Fisher, 1980; Watkins and Murray, 1998). Blue whales have been shown to follow optimality predictions when feeding on krill by increasing the number of lunges per dive with increasing feeding depth (Doniol-Valcroze et al., 2011). Additionally, they modulate their behavior both in response to krill density and foraging depth (Hazen et al., 2015). For instance, they tend to optimize oxygen consumption when krill densities are low or located at shallow depths and to maximize energy intake when krill densities are high or located at deeper depths (Hazen et al., 2015). Capital breeders such as blue whales might be particularly sensitive to alterations to their prey field or prey access, and in the context of climate change and worldwide increase in anthropogenic activity, there is a need to understand how animals cope with natural or human-induced changes to their environment.
In recent years, climate change and increased anthropogenic activity may be adding to the stochasticity and natural variability in krill availability, density and patchiness. By affecting water temperature, sea ice extent and other environmental factors, climate change has been acting on krill abundance and distribution, species composition, and life cycle in both hemispheres (Flores et al., 2012; McBride et al., 2014). Krill exploitation for use primarily as fish food in aquaculture, medical or pharmaceutical purposes, or for human consumption is a common activity in the Antarctic and around Japan, and its potential as a new fishery resource is periodically considered by different countries including Canada (Nicol et al., 2012). Decreases in krill abundance from climate change and fisheries have been shown to hinder foraging success in penguins (Alonzo et al., 2003; Cresswell et al., 2008). Given that prey density directly influences the rate of net energy gain, any reductions in the krill preyscape would impact blue whale energetics likewise.
In the western North Atlantic, blue whales seasonally visit the Estuary and Gulf of St. Lawrence (EGSL) to feed (Sears and Calambokidis, 2002). In this region from 1999 to 2009, their diet was composed on average of 70% of Thysanoessa raschii, and 30% of Meganyctiphanes norvegica (Gavrilchuk et al., 2014), the two dominant krill species in the area. These two species differ in aggregative behavior, mean depth, temperature preferences, optimal ecological niche as well as energy content (Plourde et al., 2014b; McQuinn et al., 2015; Cabrol et al., 2019). Surface salinity affects daytime distribution of the two species, while changing water temperature experienced by adult krill may have consequences for development, growth, and reproduction (Richardson, 2008; Plourde et al., 2014a, c; Benkort et al., 2019), and ultimately biomass and density (Richardson, 2008). With climate change, M. norvegica – a temperate to boreal species (Sameoto, 1976) – might be favored over Thysanoessa spp. (i.e., T. raschii and Thysanoessa inermis) – adapted to cold environments (Mauchline and Fisher, 1980). A change in the relative abundance and density of the two krill species, or in the depth of their center of mass, is likely to affect the energetics and foraging efficiency of blue whales feeding in the EGSL. Similarly, operations such as the introduction of a krill fishery, that would reduce krill density and biomass, could lead to a decrease in the number of krill patches with densities suitable for foraging blue whales and to an increased patchiness of blue whale habitat, affecting their foraging efficiency.
Other anthropogenic activities might also affect foraging efficiency by acting on prey access rather than density. This is the case for instance of anthrophonic disturbances related to marine traffic or whale-watching activities, underwater noise and vessel collision risk (Pirotta et al., 2018) that interfere with normal behavior. Whale watching operations can reduce foraging activity of marine mammals by triggering avoidance responses and changing diving patterns (Lusseau et al., 2009; Christiansen et al., 2013; Senigaglia et al., 2016; Schuler et al., 2019). A reduction in net energy gain can lead not only to immediate physiological repercussions but also to long-term consequences on population dynamics (Pirotta et al., 2019). In the EGSL, marine traffic and whale-watching are industry sectors that generate thousands of transits through blue whale habitat annually (Chion et al., 2009; Aulanier et al., 2016). In this area, close proximity between vessels and blue whales occurs on a regular basis, and for prolonged periods during daytime, as the species is the target of whale-watching activities (Martins, 2012). Particularly when within 400 m, vessels have been shown to reduce blue whale feeding opportunities by decreasing their surface and dive times (Lesage et al., 2017b). The relative proportion of lost foraging time from vessel exposure also increased exponentially with prey depth (Lesage et al., 2017b). This lost foraging time through a decrease in the number of feeding lunges per dive has resulted in lesser net energy gain in other rorqual species (Christiansen et al., 2013; Di Clemente et al., 2018). Consequently, vessel proximity may be affecting the energetics and foraging efficiency of disturbed whales. These effects might be exacerbated when cumulated with other stressors such as fisheries or climate change on prey abundance and density.
Blue whales in the western North Atlantic are listed as endangered under the Species at Risk Act of Canada and the Endangered Species Act of the United States of America. Their low abundance and apparently low calving rate raise concerns about their nutritional status and cumulative impacts of the changes to their environment, either natural or man-induced, on their foraging efficiency, fitness and population size. In this study, we examined how changes in krill density and reductions in time spent foraging affected net energy gain of foraging blue whales through model simulations based on empirical data of their foraging behavior. Ultimately, these results can help estimate the amount of additional pressure if any that this population can sustain in light of the long-term changes in climate, and chronic exposure to anthropogenic activity.
Materials and Methods
We used model simulations to explore the energetic consequences of both human disturbances and variations in krill preyscape on blue whale energy gains. Each scenario comprised a set of simulations to investigate the effects of reductions in krill density, of reduced dive durations as a result of vessel proximity, and of the combined effect of both while accounting for foraging depths. Consequences on blue whale energetics and the ability to accumulate energy were integrated over a 10-h daylight foraging bout, which corresponded to the maximum duration of whale-watching activities and potential vessel proximity in the study area during summer (Martins, 2012; Lesage et al., 2017b). However, we did not consider the longer term impacts of these energetic consequences because we lacked data on seasonal changes in blue whale behavior and on the strategies that blue whales may use to cope with energy deficits.
Model Framework
We simulated individual time-series of foraging dives to investigate immediate and cumulated energetic consequences of the different scenarios. The model framework allowed the integration of dive-specific parameters such as feeding depth and depth-specific krill densities when calculating energy intake and expenditures (Figure 1). We used Monte Carlo methods to account for the uncertainty and variability in parameters, and for estimating net energy gain. Dive parameters for the simulations were based on the literature or the analysis of data loggers (Table 1). We assumed that a whale initiating a foraging dive at a given depth foraged in the same way and in the same location for the 10-h foraging bout. We also did not take into account the time needed to fill the forestomach or its clearing rate given that our model was based on empirical diving rate data, which already incorporated these factors.
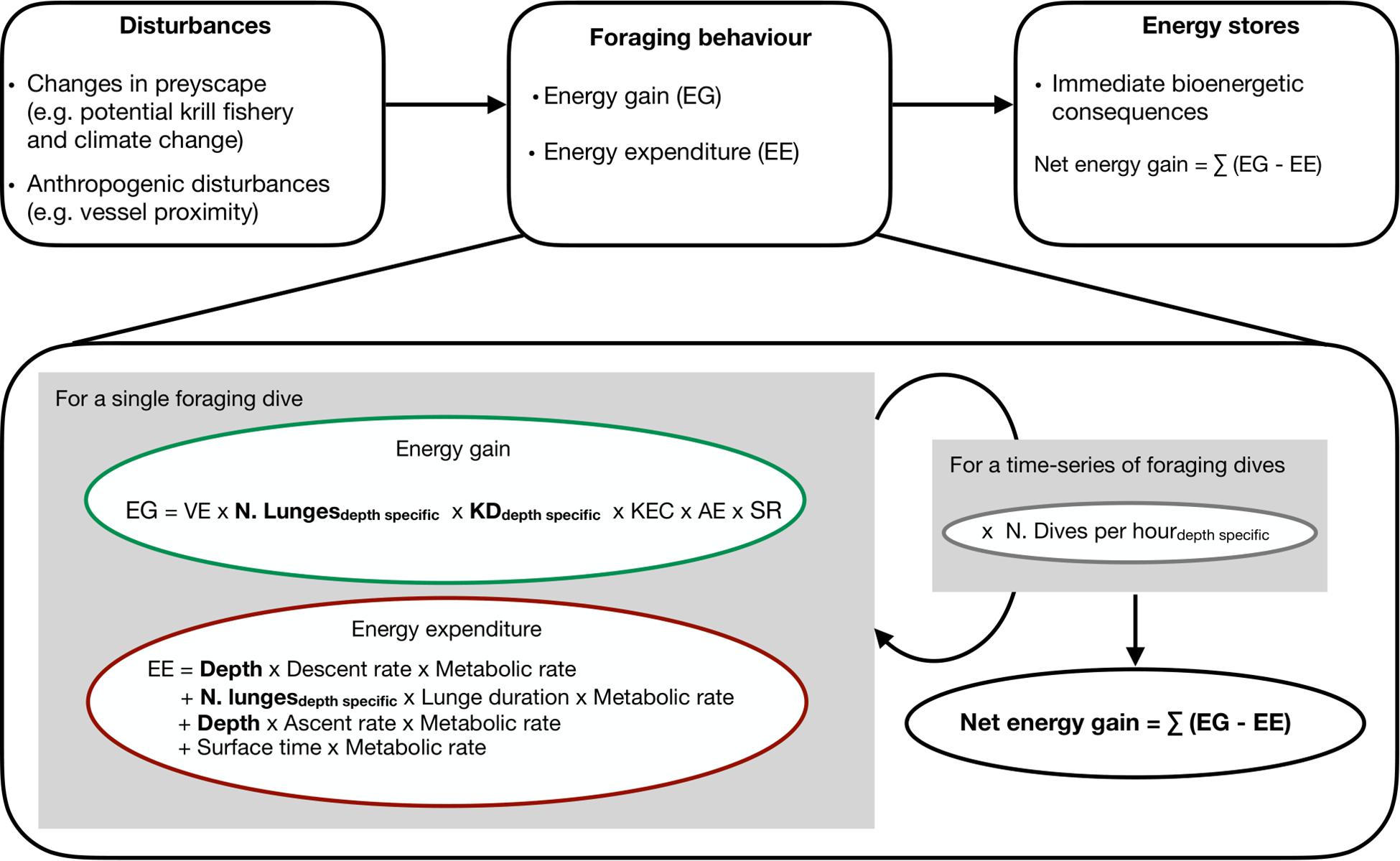
Figure 1. Bioenergetic model framework to assess immediate effects on net energy gain of changes in preyscape as a result of ocean warming or in prey access as a result of vessel proximity. Parameters and their value are provided in Table 1.
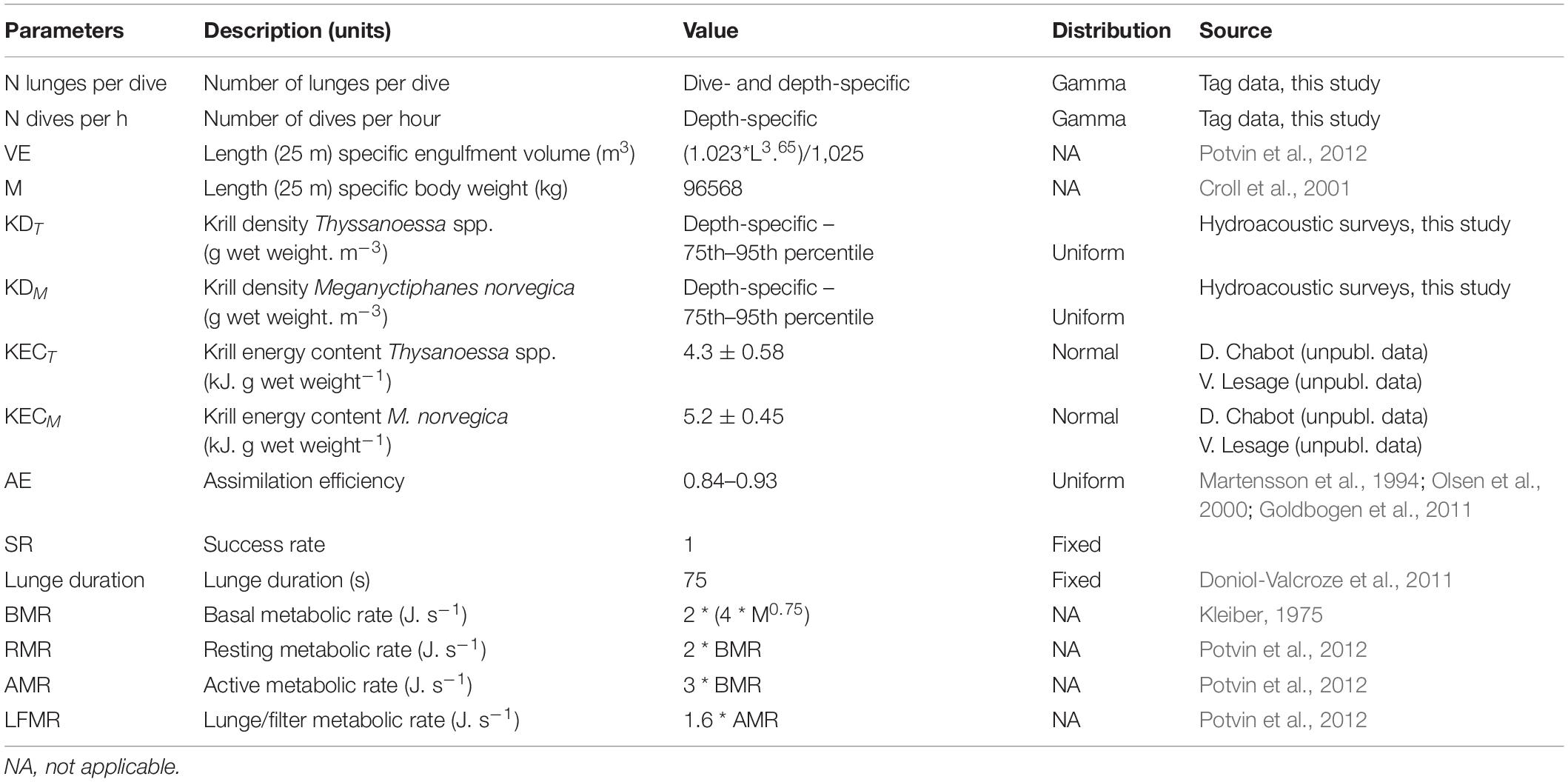
Table 1. Input parameters, associated sampling distributions and/or values and, data sources used in the bioenergetic framework for estimating net energy gain.
We calculated the net energy gain (MJ) as the difference between the energy intake and energy expenditure cumulated over each hour of foraging (i):
The energy intake (kJ) during a dive was defined as:
and accounts for the number of lunges per dive, which depends on feeding depth, body-length dependent volume of engulfment VE (m3), species-specific krill density KED (g wet weight.m–3), krill-specific energy content KEC (kJ.g wet weight–1), the assimilation efficiency AE, and success rate SR.
The energy expended per dive depends on the targeted depth. Typically, blue whale foraging dives consist of a descent during which 40% is passive gliding, followed by one or more lunges at depth, a steadily powered ascent phase and recovery time at the surface (Goldbogen et al., 2011). Blue whales are negatively buoyant and start gliding at a depth of about 18 m when targeting depths exceeding 36 m (Williams et al., 2000). Accordingly, the energy expended (kJ) during a foraging dive at depths of 36 m or more, was modeled as follows:
where Depth (m) ∗ Descent rate (m.s–1) and Depth (m) ∗ Ascent rate (m.s–1) respectively represent the time (s) required to descend to the targeted depth or to ascend back to the surface, and where N. lunges per dives ∗ Lunge duration represents the time (s) available for foraging at the targeted depth (m). Each feeding dive was followed by a period spent at the surface, specific to feeding depth, and for which costs were calculated based on the resting metabolic rate. Recovery time at the surface was shown to increase with feeding depth of the preceding dive (Doniol-Valcroze et al., 2011), and therefore this relationship was extrapolated from empirical data.
The specific metabolic rates: basal metabolic rate (BMR), resting metabolic rate (RMR), active metabolic rate (AMR), and lunge/filter metabolic rate (LFMR) were taken from the literature (Hemmingsen, 1960; Kleiber, 1975; Croll et al., 2001; Goldbogen et al., 2006; Potvin et al., 2012). Values and sources for all parameters are presented in Table 1. The energy expended (kJ) when foraging at depths shallower than 36 m was modeled in the same way, but without considering passive gliding during descent:
Energy associated with urine production was assumed to be included in basal metabolism, which represents the energy needed for maintenance activities, including vital organs such as kidneys. Energy expenditure from the heat increment of feeding, i.e., energy associated with digesting food, depends on the size and composition of the meal, and should ideally be included as a separate parameter in bioenergetics models. However, given there is no value specific to large whales for this parameter, numerous studies investigating the energetics of large whales (e.g., right whales and blue whales) have not taken these costs into account (Wiedenmann et al., 2011; Fortune et al., 2013; Pirotta et al., 2018). Instead, heat increment of feeding has been assumed to be part of the active metabolic rate (Nordøy et al., 1995).
Details on other parameters, such as depth-specific diving rate or time spent at the surface after a dive, which were extracted from the analysis of biologging data, and on krill densities obtained from systematic hydroacoustic surveys, are presented in the Supplementary Material.
Simulated Scenarios
The energetic consequences of krill density reductions or of repeated vessel interactions were each examined under baseline conditions and three different scenarios using Monte Carlo simulations (Supplementary Table S1). We proceeded with 1,000 iterations, which were each comprised of a time-series of feeding dives performed over a 10-h foraging bout.
Scenario 1: Baseline
This scenario used vertical distributions of krill densities measured in two regions, i.e., the St. Lawrence Estuary (SLE) and the North western Gulf of St. Lawrence (NWG), during systematic hydroacoustic surveys conducted in August 2008–2015 in the EGSL (Quebec, Canada, 49° 43′N – 65° 11′W) (Figure 2, data analysis is presented in details in the Supplementary Material). Blue whales feed in three different habitats in the SLE (Doniol-Valcroze et al., 2012), the mean feeding depth of which corresponds well with the center of mass of krill patches documented in this region (i.e., 30, 80, and 130 m for Thysanoessa spp., and 60 and 80 m for M. norvegica) (Figure 3 and McQuinn et al., 2015). While a comparable study documenting blue whale foraging depth does not exist for the NWG, the center of mass for Thysanoessa spp. and M. norvegica remain in the range of those plausible or documented in the SLE, or in other regions such as the Pacific (i.e., 30, 60, 120, and 160 m for Thysanoessa spp., and 60, 100, 140, and 180 m for M. norvegica) (Goldbogen et al., 2013; Hazen et al., 2015; Irvine et al., 2019). Therefore, we ran simulations for feeding depths corresponding to krill peak densities in each of the two regions, and separately for the two krill species (Figure 3).
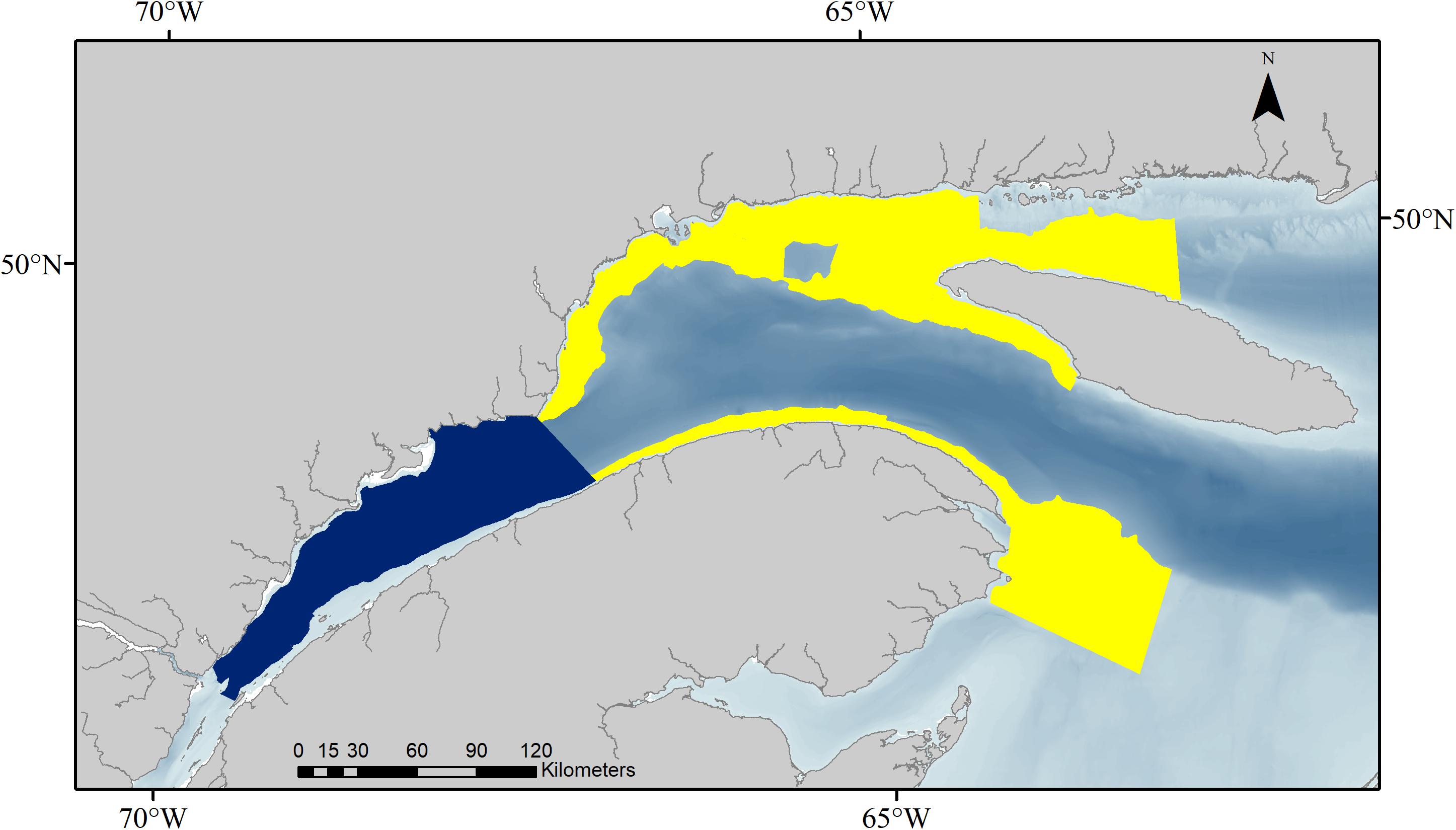
Figure 2. Two regions, i.e., the Estuary of the St. Lawrence (dark blue) and the northwestern Gulf of the St. Lawrence (yellow) including the Gaspé peninsula, used to determine in situ vertical distributions of krill densities.
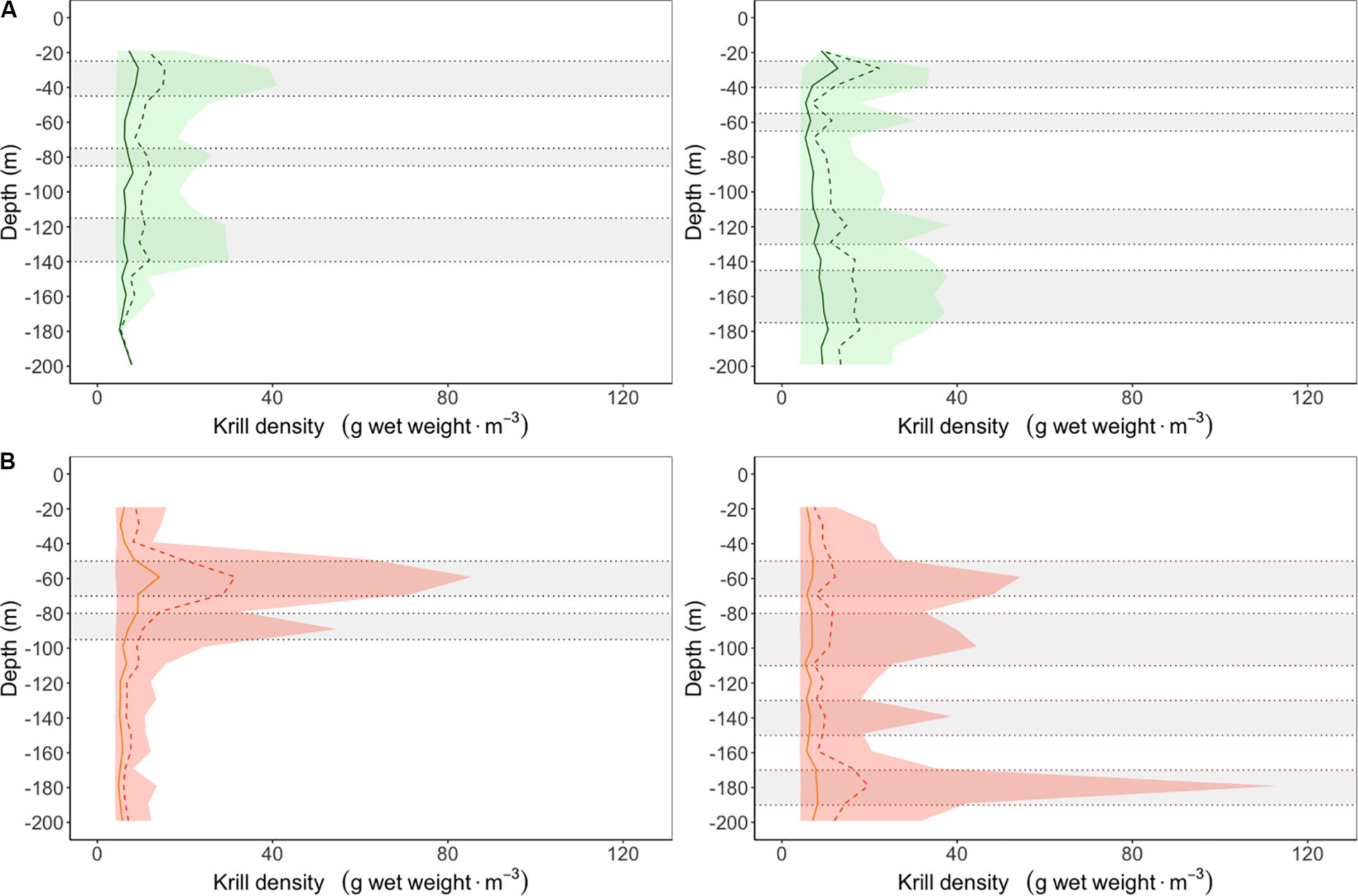
Figure 3. Vertical density profiles for (A) Thysanoessa spp. (green) and (B) Meganyctiphanes norvegica (orange) for the St. Lawrence Estuary (left) and the northwestern Gulf of St. Lawrence (right) with identified high density layers used as targeted feeding depth for simulated dive time-series (gray horizontal ribbons). Solid vertical lines represent the median, the dashed lines are the 75th percentiles and the colored areas are the 5–95% quantiles.
Scenario 2: Reduction in Krill Biomass Density
This scenario investigates the effect of reducing krill densities on net energy gain. We modeled a decrease in krill density relative to the baseline scenario for the two species separately and distributed this decrease homogeneously over the baseline vertical distribution. Hypothetical reductions in krill density of 5, 10, 25, and 50% were examined, each using a set of 1,000 iterations.
Scenario 3: Disturbances From Vessels Proximity
A recent study indicated that the presence of vessels within 400 m of blue whales in the SLE resulted in a 36% reduction in dive time, equivalent to a mean dive duration of 4 min, and a maximum foraging effort of 12 dives per hour (Lesage et al., 2017b). In this scenario, we investigated the effect of limiting dive time to a maximum of 4 min on blue whale capacity to accumulate energy, while allowing reachable depth and number of lunges per dive to vary for each dive. In other words, disturbed whales were allowed to vary the number of lunges per dive according to feeding depth, but with the constraint of a maximum dive duration of 4 min. Krill density was depth-specific for each dive. We simulated conditions reflecting vessel proximity within 400 m of foraging blue whales for a portion (i.e., 3-h – from hour 5 to 8) and the full potential duration (10 h) of whale-watching activities in the St. Lawrence Estuary (Martins, 2012). Therefore in the scenario of a 3-h vessel proximity, a whale would be constrained to adjust feeding depth and exploit the highest densities that are reachable within the 4-min dive limit during these 3 h. They would be allowed to resume foraging at the initial target depth for the next 7 h. In the scenario where vessel proximity lasted for the full daytime foraging bout, i.e., 10 h, whales would be deprived from reaching certain deeper depths under the constraints of the 4-min dive limit for the entire period. While effects on net energy gain for a 3 h vessel proximity are presented relative to the baseline scenarios for the same depth categories, they are presented for the 10 h relative to the baseline scenario providing the highest net energy gain, i.e., they are not depth-specific.
Scenario 4: Combined Effect of Reduction in Krill Density and Vessel Disturbance
Reductions in krill density might occur in areas where vessel-related effects take place and thus, these two effects might be additive (Hin et al., 2019; Pirotta et al., 2019). To account for potential combined effects, we examined scenarios where krill density was reduced by 5, 10, 25, and 50% from baseline densities, while blue whales foraged in conditions of vessel proximity for 3 and 10 h.
Statistical Analysis
We assessed the sensitivity of the energy gain function to the uncertainty in parameters using a partial correlation coefficient sensitivity analysis and the “pcc” function of the R package “sensitivity” (Pujol et al., 2016. Sensitivity: Global Sensitivity Analysis of Model Outputs. R package version 1.17.0). This method accounted for the correlation between feeding rate and energy expenditure, and identified parameters with the largest influence on energy gain, across all scenarios. While all parameters from the energy gain equations (Eqs. 1 and 2) figured in the sensitivity analysis, the energy expenditure associated with each dive (Eq. 3) was entered as a single parameter, thereby incorporating variability associated with depth-specific feeding. This allowed the analysis to be focused on uncertainty from krill density and number of lunges performed, while avoiding autocorrelation of multiple parameters associated with energy expenditure. Metabolic rates are weight-specific, and estimates from the literature are not associated with uncertainty and thus a single, fixed value, was used given our model was for a 25-m whale.
We assessed each scenario and its effect on blue whale net energy gain against the baseline scenario using Cohen’s d value, which represents the difference between the mean net energy gain of one of the krill-reduction and/or vessel-proximity scenarios and the baseline scenario scaled by their pooled standard deviation (Cohen, 1977). Cohen’s d values indicate a small (0.2–0.5), moderate (0.5–0.8), or large (>0.8) effect size (Cohen, 1977). Simulations and statistical analyses were conducted under the R programming language (R Development Core Team, 2017).
Results
Baseline simulations using in situ krill vertical distributions indicated that blue whales had the potential to accumulate three times more energy by feeding on the most accessible peak of krill densities, i.e., nearest to the surface. For instance, mean net energy gain was more than 3 times higher in the SLE when feeding on peak densities of M. norvegica at 50–70 m versus 80–90 m (Figure 4A), or on peak densities of Thysanoessa spp. at 25–45 m versus 115–145 m (Supplementary Figure S1A). Only in the NWG, where peak densities of M. norvegica at 170–190 m were double those at 50–70 m, was net energy gain similar at the two feeding depths (Supplementary Figure S2A in the NWG for M. norvegica). M. norvegica also offered a higher potential for net energy gain than Thysanoessa spp. in the two regions, regardless of feeding depth. This was particularly the case in the SLE, with mean net energy gain for blue whales reaching a maximum of 4,526 and 2,772 MJ for M. norvegica, as opposed to 1,070 and 675 MJ for Thysanoessa spp. in the SLE and NWG, respectively (Figure 4A, Supplementary Figures S1A, S2A, S3A, and Supplementary Table S1).
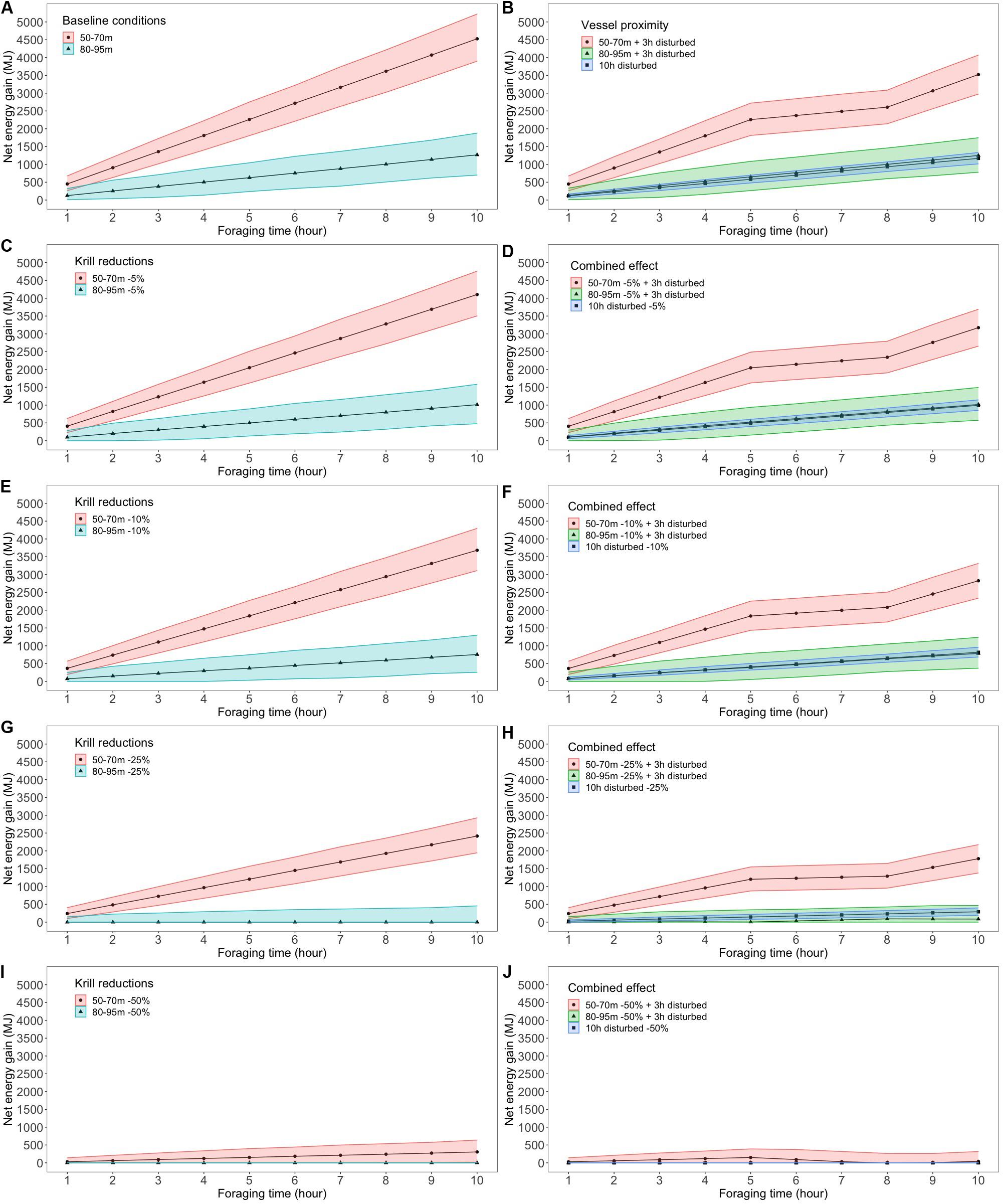
Figure 4. Net energy gain (mean and 5–95% CI) accumulated over a 10 h foraging period when feeding on M. norvegica in the St. Lawrence Estuary, (A) under baseline conditions measured in situ, (B) when exposed to vessel proximity within 400 m for 3 h (from hour 5 to hour 8) and 10 h, (C,E,G,I) under krill density reductions of 5, 10, 25, or 50% relative to baseline, and (D,F,H,J) while foraging on reduced krill densities when exposed to vessel proximity. Patterns for Thysanoessa spp. and the NWG are presented in the Supplementary Material.
Simulations of various scenarios of krill reduction and vessel proximity highlighted significant differences in blue whale net energy gains made over a 10-h daylight foraging bout compared to the baseline. Generally, the magnitude of the effects increased with that of krill density reductions and the duration of vessel proximity. Trends in net energy gain as a result of krill density reduction and vessel proximity scenarios were similar for the two krill species, and the two regions, except for the particular case of Thysanoessa spp. in the SLE. Therefore, detailed results are presented only for the scenarios that involved M. norvegica in the SLE, with results for the other species and regions being presented in the Supplementary Material. However, the relative range of the effect sizes of the different scenarios on net energy gain is presented below for both krill species and the two regions.
As expected, reducing krill density decreased the net energy potentially gained by a blue whale feeding at any depth compared to the baseline scenario. The energy deficit increased with the size of the krill density reduction, for the two species and regions (Figures 4C,E,G,I and Supplementary Figures S1C,E,G,I, S2C,E,G,I, S3C,E,G,I). However, net energy losses were overall smaller both in absolute terms (in MJ) and in percentage for a given scenario when peak densities were more accessible, i.e., closer to the surface (Figures 4C,E,G,I, 5). For instance, a reduction of 5% in M. norvegica densities in the SLE caused a 9% reduction in net energy gain when peak densities were at 50–70 m (422/4,526 MJ), compared to 20% (257/1,268 MJ) when peak densities were at deeper depths (80–90 m) (Supplementary Table S1). In addition, large effects were observed when initial peak densities were low. In Thysanoessa spp. for instance, even small to moderate reductions in krill densities decreased net energy gain to 0 (Figure 3, Supplementary Table S1, and Supplementary Figures S1C,E,G,I, S3C,E,G,I), with effect sizes considered moderate at 5% reduction (Cohen’s d values >0.51) and large at 10, 25, and 50% reduction (Cohen’s d values >1.16, Figure 5). In comparison, the effects of a decrease in krill density were considered small for M. norvegica at 5% reduction (Cohen’s d values >0.28), moderate to large at 10% reduction (Cohen’s d values >0.45) and large at 25 and 50% reduction (Cohen’s d values >1.17, Figure 5) depending on whether whales were feeding in the SLE or NWG.
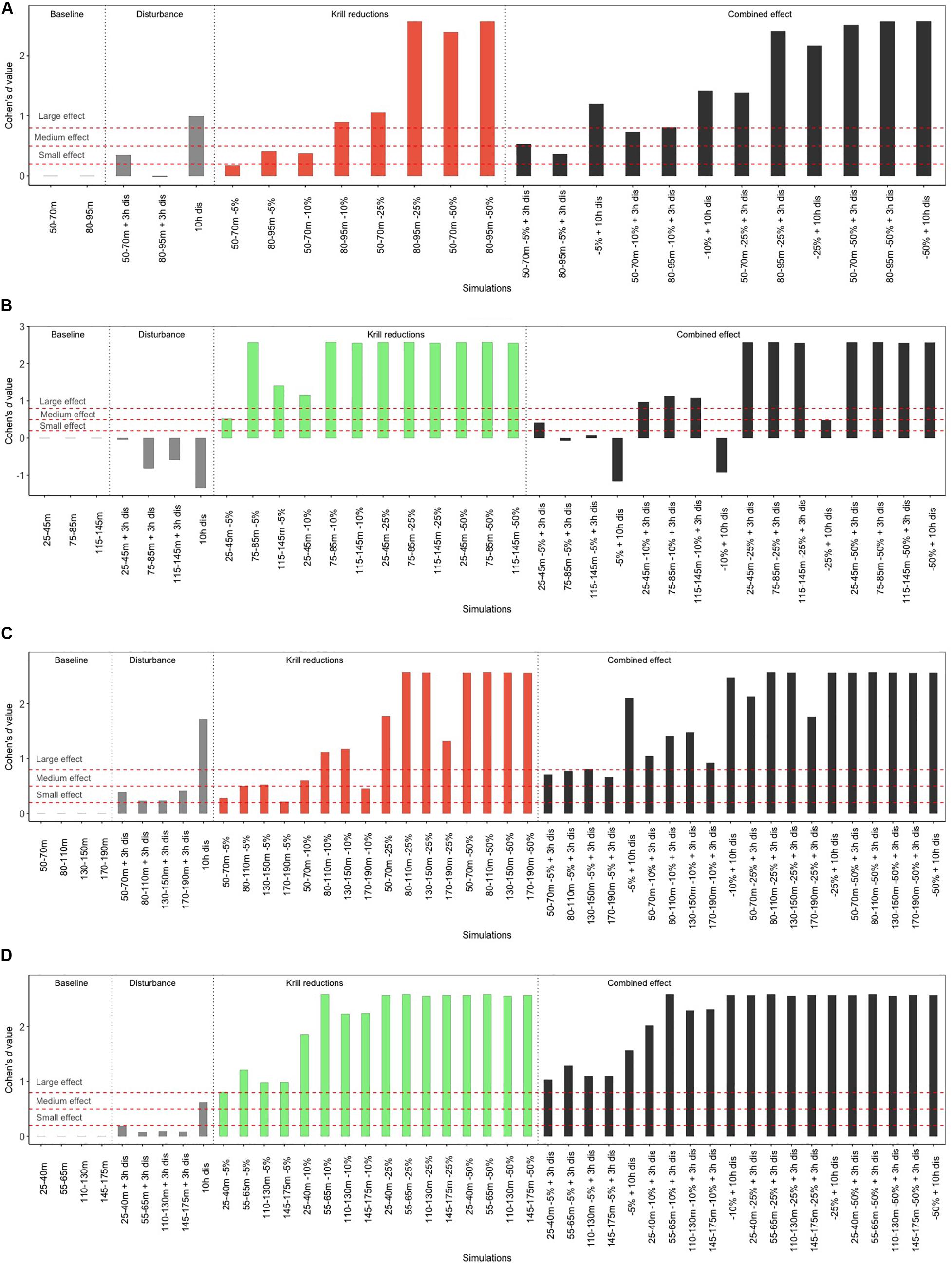
Figure 5. Effect of the various scenarios on net energy gain after a 10 h foraging period of a blue whale feeding on (B,D) Thysanoessa spp. or (A,C) M. norvegica, (A,B) in the St. Lawrence Estuary and (C,D) in the North western Gulf of St. Lawrence. The red dashed lines represent the threshold values of Cohen’s d above which effects are considered small (0.2), medium (0.5), and large (>0.8). Negative Cohen’s d value indicates a positive effect.
Vessel proximity to foraging whales, with the limit they imposed on dive duration (4 min) and feeding rate (12 dives per h), led to the majority (70%) of dives being constrained to depths of 30 m or less (Figure 6). The impacts of vessel proximity on net energy gain of foraging whales increased with the duration of vessel interaction, were more important when whales were precluded from reaching the most beneficial krill density peaks and when these densities were located at deeper depths (Figures 4, 5 and Supplementary Figures S2B, S3B). The impacts of vessel proximity also depended on initial feeding depth and associated peak densities. For instance, an undisturbed whale foraging in the SLE at intermediate depths (50–70 m) on densities of M. norvegica performed on average 10 dives and 25 lunges⋅h–1, and gained 4,526 MJ over the 10 h foraging bout. During the 3 h when vessels were in proximity, their feeding rate declined to 12 dives and 15 lunges⋅h–1, and whales were likely unable to reach depths where they were initially feeding. Adding the 7 h of undisturbed foraging (during which feeding depth, the number of dives, and the number of lunges were unconstrained) resulted in a 22.2% reduction in net energy gain compared to the baseline over the 10-h foraging bout, i.e., 3,521 MJ (Supplementary Table S1). In comparison, an undisturbed whale targeting less dense krill densities at deeper depths (80–95 m) was able to perform only 7 dives and 26 lunges⋅h–1, gaining nearly 3 times less energy than a similarly undisturbed whale feeding near the surface (1,268 MJ). In this case, vessel proximity over 3 h did not further constrain the whale feeding rate (which was already within the set limit of 12 dives⋅h–1), but it limited feeding depth to shallow waters through the 4-min dive limit where densities were lower than at deeper depths (Figure 3). Unconstrained diving to 80–195 m for the remaining 7 h led to no overall change in net energy gain in this scenario (i.e., 1,251 MJ, Supplementary Table S1). Whales having vessels in proximity during most of their daytime foraging bout had their feeding rate limited for the full 10 h of vessel proximity, leading to net energy gains that were 74% lower compared to undisturbed whales feeding at depths allowing the highest energy accumulation, an effect considered large based on Cohen’s d value (>1.8) (Figure 5 and Supplementary Table S1). There were scenarios where vessel proximity did not lead to a negative effect on net energy gain, notably when undisturbed whales foraged near the surface (25–45 m) on the highest densities of Thysanoessa spp. in the SLE.
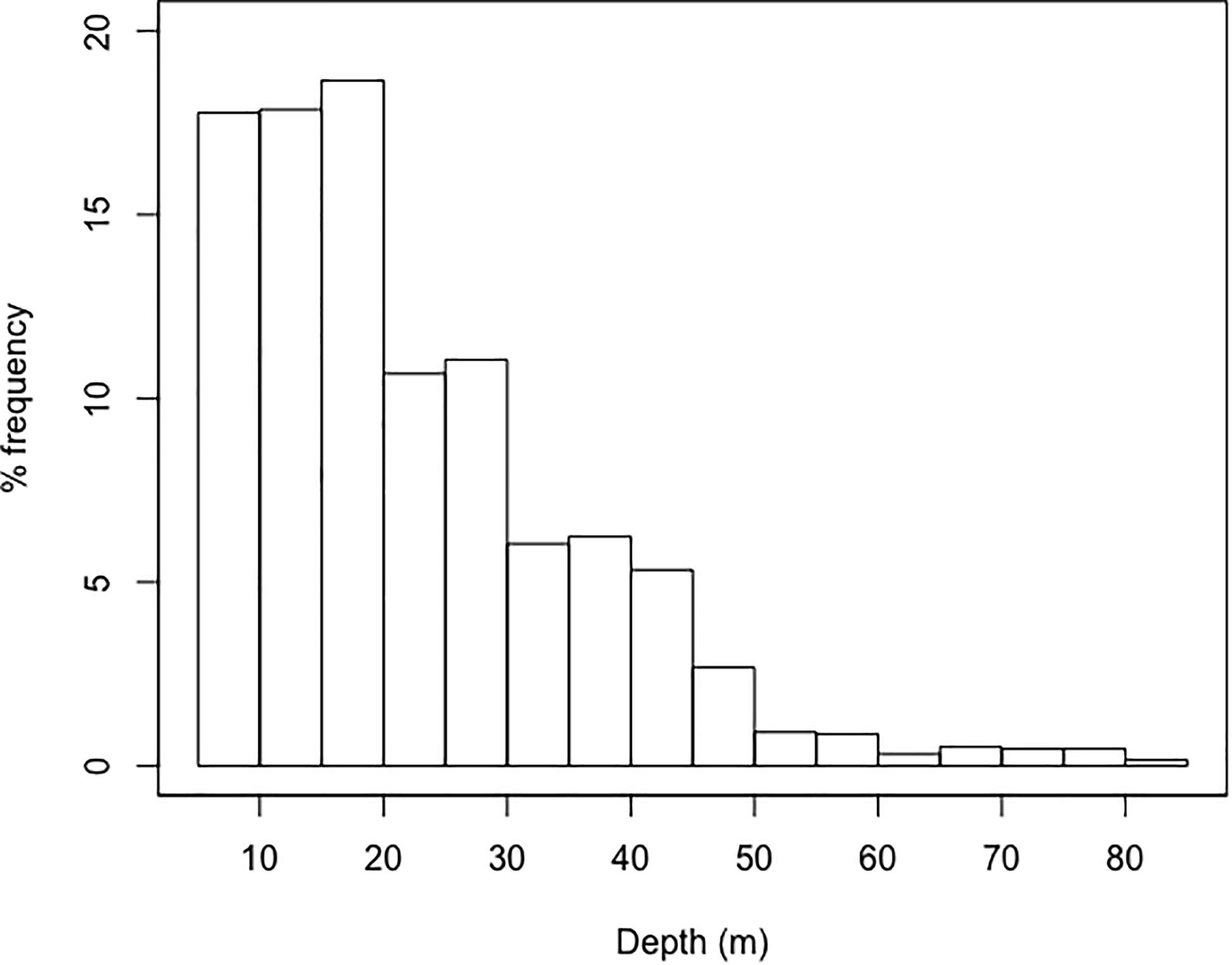
Figure 6. Frequency (%) distribution of feeding depths when exposed to vessel proximity within 400 m while feeding, thus when dives are limited to 4 min or less.
In the last scenario, effects from krill density reductions were combined with those from vessel proximity. As a result, effects on net energy gain generally reflected those from the two stressors separately. Specifically, vessel proximity and krill density reductions net energy losses were smaller when peak densities were high, and more accessible. Impacts of these two factors on net energy gain increased with krill density reductions, and with vessel proximity persistence over time (Figures 4D,F,H,J, 5). For whales foraging on peak densities of M. norvegica in the SLE, a density reduction of 25% or more, combined with vessel proximity of 3 h or more, invariantly resulted in a medium to large effect, equivalent to approximately 60–100% decrease in net energy gain depending on feeding depth (Figure 5 and Supplementary Table S1). A whale foraging optimally, i.e., on peak densities of M. norvegica nearest to the surface, but that have been reduced by 50%, with vessels in proximity for 3 h, would accumulate less than half the energy they would have acquired under the baseline scenario (mean net energy gain of 1,781 MJ versus 4,526 MJ under baseline) (Supplementary Table S1). Extending this perturbation to 10 h would lead to a 60% reduction in energy accumulated over that period compared to baseline conditions (i.e., 2,747 MJ) (Supplementary Table S1). For M. norvegica in the SLE and both species in the NWG, even a small reduction in krill densities (5%), when combined to medium (3 h) exposure to vessels can lead to a net energy deficit of 19–38% when feeding at intermediate or shallow depths. The scale of the effect varied for the two species and regions for a given depth and scenario according to krill densities and depths where net energy gain was the highest (see Supplementary Material for further results). Comparing the effect size between the two stressors, a 3 h vessel proximity to a foraging blue whale would result in the same reduction in net energy gain as a 5% krill density reduction in most cases. Foraging during 10 h with vessels in continuous proximity would be equivalent to foraging on krill densities that are reduced by 5–10% (Figure 5).
The sensitivity analysis highlighted the number of lunges per dive, krill density and krill energy content as the three parameters contributing the most to the uncertainty in net energy gain (Sensitivity partial correlation coefficients: 0.96, 0.87, and 0.62, respectively).
Discussion
Habitat degradation, either natural and/or human-induced, can have detrimental effects on foraging efficiency, fitness and population trends (Williams et al., 2006; Pirotta et al., 2018). Blue whale foraging behavior in the SLE conforms to optimality predictions, with feeding dives near the surface yielding the highest feeding rates (Doniol-Valcroze et al., 2011). Adding to this that the krill densities measured in situ in the EGSL were generally denser at shallower depths than at deeper depths (Figure 3), we predicted that the highest potential for energy accumulation was when whales were feeding on these shallow peaks densities and were targeting M. norvegica over T. raschii. We also showed that the energy deficit caused by krill density reductions or vessel proximity, or their combination, can be significant, even when vessels are in proximity for relatively short periods of time (3 h). Generally, effects varied with the density and depth of the krill peaks accessible to blue whales, and increased with the magnitude of krill density reductions, and with the duration of vessel proximity.
Krill densities, like any other marine resource, naturally fluctuate in abundance according to environmental conditions (Reid et al., 2010; Flores et al., 2012; Sydeman et al., 2013), and long-lived predators are particularly well adapted to cope with these fluctuations (Benoit-Bird et al., 2013; Abrahms et al., 2019; Dunn et al., 2020). Our simulations showed that blue whales are in all likelihood well adapted to cope with fluctuations of 5%, but that they may incur considerable energy loss at higher density fluctuations. A link between food availability and calving rate has been documented in several species including blue whales (e.g., Ward et al., 2009; Seyboth et al., 2016; Meyer-Gutbrod and Greene, 2018). In blue whales from Antarctica, a simulated reduction of 50% in mean swarm density during an entire feeding season of 120 days resulted in an estimated 80% decrease in calving rate (Wiedenmann et al., 2011). Blue whales appear to track food resource based on long-term stored information rather than short-term proximate cues (Abrahms et al., 2019), a behavior that might limit their ability to respond and adjust to rapidly changing environmental conditions despite a certain degree of behavioral plasticity in blue whales (Abrahms et al., 2019) as in other marine predators (Ronconi and Burger, 2008). Behavioral plasticity might be particularly insufficient for mitigating the consequences of climate change, which are accelerating and exacerbating the natural variability of prey resources (Sydeman et al., 2013). Important foraging habitats for blue whales have been identified in several areas across eastern Canada, including the EGSL but also in Newfoundland waters and on the Scotian Shelf (Lesage et al., 2018). These regions offer a high diversity of habitats, and are heterogeneous in their seascape (Loncarevic et al., 1992; Galbraith et al., 2018), in vessel traffic density (e.g., Simard et al., 2014), and in krill densities (McQuinn et al., 2015; Plourde et al., 2016). In the case where krill densities would be inadequate in a given region, or where vessel exposure would limit energy gains, whales would theoretically have the opportunity to move to other areas of recurrent krill aggregations within the EGSL region or outside of it, assuming of course that these habitats exist in these other regions at the time they are needed.
Changes in zooplankton community composition in the EGSL have been documented over the last two decades (Richardson, 2008; Blais et al., 2019). Although studies specifically examining climate change effects are still scarce for krill communities typical of the EGSL, changes in composition are also expected for the latter given the overlapping but distinct optimal temperature niche of the two main krill species (Sameoto, 1976; Mauchline and Fisher, 1980; Ollier et al., 2018). Water salinity through its effect on light penetration influences the vertical distribution and density of krill in the EGSL (Plourde et al., 2014b) as well as their temperature exposure, physiological state, condition, and survival (Flores et al., 2012; McBride et al., 2014). The forecasted 0.6–1.2°C increase in seawater temperature the EGSL over the next 50 years (Hutchings et al., 2012; Long et al., 2016; Galbraith et al., 2018) might lead to a niche expansion of the more temperate species, M. norvegica (Sameoto, 1976). Although the effects of a potential increase in species-specific krill density are not explicitly addressed in this study, their general trend could be derived directly from the percent changes presented in the Supplementary Material (by making them positive).
Meganyctiphanes norvegica offered a higher potential for net energy gain than Thysanoessa spp., with particularly high energetic benefits when peak densities were near the surface. While blue whales are known to feed on both M. norvegica and Thysanoessa spp., their diet is likely to vary across their range depending on local availability (Lesage et al., 2018). M. norvegica is the dominant krill species in other, warmer blue whale foraging areas such as the Scotian shelf or the waters off southern Newfoundland (Cochrane et al., 1991, 2000) and is likely to represent their main prey, although specific blue whale diet is unknown for these areas. In the EGSL, Thysanoessa spp. comprised 70% of blue whale diet between 1995–2009 according to quantitative isotopic models (Gavrilchuk et al., 2014). This is in agreement with the stronger spatial association of this krill species with blue whale observations made during hydroacoustic surveys in 2009–2013 (McQuinn et al., 2016), and which suggests that M. norvegica densities worth exploiting might not be that common in the EGSL. Hydroacoustic surveys used in our study to derive total krill densities were conducted in 2008–2015, i.e., mainly after the study on blue whale diet, and indicated densities that were similar for the two krill species or greater for M. norvegica since 2010 (Figure 3). However, M. norvegica peak densities are typically found at deeper depths than Thysanoessa spp. (Figure 3; McQuinn et al., 2015, 2016), reducing the relative benefit of exploiting this resource over Thysanoessa spp. Considering its higher energetic value, and that our results showed that blue whales accumulated the most energy when feeding on M. norvegica, a change in the dominance ratio with climate change in favor of M. norvegica might be beneficial to blue whales and therefore mitigate at least in part the reduced krill density in the EGSL.
We showed that the level of impact from vessel proximity is dependent on resource accessibility. If krill is present in sufficient densities near the surface, then the negative effects from close vessel proximity, which imposes a limit on dive time and dive rate, would not be as limiting as if adequate krill densities are only available at deeper depth, where they become out of reach with a dive-duration limit of 4 min (>30 m). In the SLE, blue whales forage in four types of habitat that vary in feeding depth and whale behavior, and that are used differently depending on tidal phase (Doniol-Valcroze et al., 2012). Feeding depths follow a bimodal distribution, with a strong peak near the surface and a weaker peak between 50 and 100 m (Doniol-Valcroze et al., 2012). Indeed, blue whales in the SLE and NWG exhibited the strongest spatial association with shallow krill patches over deep aggregations between 2009 and 2013 (McQuinn et al., 2016). These results suggest that a given vessel-proximity event might have differential effects on blue whale foraging efficiency depending on where and when it occurs in their habitat. At times or in areas where sufficient krill densities that may allow for a positive net energy gain are unavailable near the surface, effects of vessel proximity on the capacity of blue whales to accumulate energy reserves might be more important than depicted in our study, even for short periods of vessel exposure.
The timing of peak whale-watching activities is inextricably linked to the presence of whales. In the EGSL, the whale-watching fleet comprises over 20 vessels proposing multiple departures a day from May to October. Their activities result in prolonged and recurrent vessel proximity to foraging blue whales (Martins, 2012) and what might lead to negligible to major energy deficits per day. Whether the net energy deficits predicted in our study would have long-term effects on blue whale body condition is unknown, and depends on the nature and magnitude of the consequences (Houston et al., 2012; Pirotta et al., 2018), which in turn depend on the recurrence of exposure and the whale’s capacity to compensate for un-capitalized energy at other times. Individuals generally show resilience and plasticity by adjusting foraging effort to the naturally changing preyscape (Costa et al., 1989; Sigler et al., 2009). In the context of anthropogenic disturbance, this plasticity likely allows some degree of compensation for lost feeding opportunities. While some studies show a relatively high resilience to disturbance in some populations (e.g., New et al., 2013a, 2014), others provide strong support for a limited capacity to compensate for lost opportunities (e.g., New et al., 2013b; McHuron et al., 2018). For example, a simulation study on Californian sea lions indicated that both short and infrequent disturbances (<1 month, 1 year only) and prolonged and repetitive disturbances (lasting several months and occurring yearly) could have detrimental effects on recruitment and population size, depending on the severity of the behavioral response to disturbance (McHuron et al., 2018). In the case of blue whales exposed to whale-watching activities, compensating for lost foraging opportunities and associated energetic shortcomings would need to occur on undisturbed day or daylight hours, during twilight and/or at night, or through an extension of their feeding season. Biologging data indicates that foraging accounts on average for 69% of the daily activity budget of blue whales in the SLE (Doniol-Valcroze and Lesage, unpublished data), thus leaving little time or opportunity for compensation. It is noteworthy that in scenarios of vessel proximity, we assumed based on Lesage et al. (2017b) that whales reduced time spent at depth and at the surface. However, their analysis was based on surface behavior only and was thus unsuitable for assessing whether the average reduction in dive time was caused by a reduction in the duration of foraging dives or by a change in behavior with total cessation of feeding (Lusseau et al., 2009). In the latter case, this would represent a total loss of foraging opportunity until whales find another suitable and undisturbed location to forage.
The availability of prey resources may also modulate the impacts of disturbances by acting synergistically. For example, a modeling exercise indicated that long-finned pilot whales should be able to withstand longer periods of disturbance when resources are abundant than when they are more scarce (Hin et al., 2019). Another simulation study involving blue whales further indicated that, beyond the instantaneous potential shortfall in net energy gain associated with a disturbance event, the recurrence of anthropogenic disturbances (e.g., every year) and its combined effect with poor environmental conditions might result in strong negative effects on their reproductive success, as they tend to prioritize their own survival over investment into an offspring (Pirotta et al., 2019). In our study area, whether the changes we modeled could affect blue whale’s capacity to accumulate adequate energy reserves to reproduce successfully or to survive is unknown, and depends on the persistence of vessel interactions and on food abundance. However, there are indications of a low calving rate for this western North Atlantic blue whale population (Mingan Island Cetacean studies, Unpublished data), which may be an indication of difficulties in foraging. When food resources are limited, the additional pressure from short-term or prolonged vessel-proximity might exacerbate the negative effects of prey limitation on their net energy gain. Modeling the effects of vessel-proximity and food shortage over longer time periods (e.g., for annual life cycle or a reproductive cycle) would be evaluated best if cumulated over a feeding season or a full reproductive cycle and would help determine their biological significance for blue whales. However, this would require a model that incorporates energy gains from feeding at other times of day, search time for food patches and non-foraging behavior, and in the case of extrapolation over a full reproductive cycle, breeding and migration costs. The model would also need to incorporate the unknown mechanisms that blue whales implement for coping with energy deficits, which may include increased foraging effort that day, or an extension of the feeding season, assuming that the foraging schedule can accommodate these additions. Energy gains from these compensatory strategies would depend on a number of factors such as prey density and search time in-between food patches, parameters that vary directly with prey densities and thus which are dependent on one of the effects we are testing here (effect of a fishery or climate-driven change in prey densities). We felt that the uncertainty associated with such predictions would be so high that they would be in the end, uninformative given the information currently available for parameterizing the model.
As a first attempt to quantify the energetic consequences of potential change in krill density and/or anthropogenic disturbances for this specific endangered blue whale population, we made a number of assumptions, which should be addressed in future work. For simplicity, we assumed continuous foraging for a period of 10 h during which whales stayed in the same foraging “mode,” i.e., they fed at the same depth for the entire period. However, foraging is often not continuous but rather organized in bouts of intense activity separated by periods of time allocated to other activities, or until foraging comes to a stop due to physiological requirements (e.g., clearing of the forestomach, digestion, replenishing oxygen stores) (Sibly et al., 1990; Boyd, 1996). While records in the Pacific indicate a median feeding-bout duration of 3.3 h for blue whales (range 0.2–34.9 h) (Irvine et al., 2019), a value similar to what has been observed in the SLE (2 h on average, range 0.1–13 h; Doniol-Valcroze and Lesage, Unpublished data), the long period spent foraging daily by SLE blue whales (average 69%, Doniol-Valcroze and Lesage, Unpublished data) suggests that pauses between foraging bouts are likely short. Individuals should theoretically end foraging when a prey patch is depleted, and resume foraging when they encounter another prey patch of sufficient density. However, both of these behavioral modes are time dependent and were not considered in our study (Mori, 1998; Watanabe et al., 2014). We assumed constant prey density while depletion of a food patch is inevitable and influences predator foraging decisions (Thompson and Fedak, 2001; Sparling et al., 2007; Thums et al., 2013; Akiyama et al., 2019), and did not allow a whale to find an alternative patch (Sims et al., 2006; Thums et al., 2011). The hydroacoustic surveys used covered most of the areas visited by blue whales over a feeding season (Lesage et al., 2017a) and provided us with a global krill vertical density distribution rather than densities of specific krill layers or swarms. It was therefore reasonable to assume that whales were feeding at the same general location for the 10-h foraging period. However, we might have overestimated net energy gain during this 10-h portion of daylight foraging by not accounting for activities other than foraging (i.e., resting and traveling).
From a management perspective, the findings of this study are important as they transpose behavioral responses into energetic consequences for foraging blue whales. They also bring support to the currently applied regulations in the Saguenay-St. Lawrence Marine Park, which impose a 400-m exclusion zone for vessels around blue whales, as a way to mitigate potential impacts on this endangered species. In addition, they highlight the need to extend these limits to other important habitats for blue whales, where vessel interactions might be chronic. Our study also underscored the importance of limiting the duration of vessel proximity, especially if vessels are within 400 m of a blue whale and in conditions where krill densities might be reduced. In the Saguenay-St. Lawrence Marine Park, a single vessel has a 1-h viewing time limit, with a 1-h interval between successive observations (Regulations Saguenay-St. Lawrence Marine Park, 2020). A potential management measure could be to implement cumulative vessel time viewing limits around blue whales to decrease total duration of potential disturbance.
For a species like the blue whale, which relies on a limited feeding season for building energy reserves, changes in energy gain through an altered krill preyscape and/or anthropogenic disturbances is of concern. This study showed that these changes not only would have a detrimental effect on net energy gain, but there is the possibility of these changes to acting synergistically or exacerbating one another. Under conditions where krill densities would decrease due to climate change or krill exploitation, disturbing foraging blue whales would undoubtedly affect their capacity to accumulate energy stores over a feeding season. An estimation of the energy budget over a reproductive cycle of blue whales is needed to determine a threshold above which these changes would jeopardize their reproductive success. Although future work needs to investigate the fine scale diving behavior of disturbed individuals, our results to date could be integrated into models simulating the population consequences of disturbances to estimate their effect on vital rates and population dynamics, providing a longer time-scale perspective on the energetics of this endangered population.
Data Availability Statement
The datasets presented in this article are not readily available because the tag data and hydroacoustic data are still under exploitation. The datasets generated for this study are available on request. Requests to access the datasets should be directed to VL, dmVyb25pcXVlLmxlc2FnZUBkZm8tbXBvLmdjLmNh (tag data); IM, aWFuLm1jUXVpbm5AZGZvLW1wby5nYy5jYQ== (hydroacoustic data); and MG, bWFyaWUuZ3VpbHBpbkBnbWFpbC5jb20= (generated datasets).
Ethics Statement
The animal study was reviewed and approved by The Canadian Animal Care Committee, through the Maurice Lamontagne Institute committee.
Author Contributions
VL and MG conceived the model framework. MG led the data analysis and wrote the manuscript. VL and GW obtained funding to support the research. IM provided the hydroacoustic surveys data and guidance for the analysis of it. VL, GW, PB, and TJ-D provided guidance on the model and simulations. All authors provided guidance on model framework and reviewed the manuscript.
Funding
This work was financially supported by the Species at Risk and Oceans Management programs of Fisheries and Oceans Canada, and by the strategic partnership grant (STPGP 447363) from the Natural Sciences and Engineering Research Council of Canada (NSERC) awarded to GW and VL. PB was funded by Fisheries Science and Ecosystem Research Program from the Government of Canada through an NSERC (Natural Sciences and Engineering Research Council of Canada) visiting fellowship.
Conflict of Interest
The authors declare that the research was conducted in the absence of any commercial or financial relationships that could be construed as a potential conflict of interest.
Acknowledgments
We thank all DFO personnel and crewmembers aboard the Frederick G. Creed involved in the systematic hydroacoustic surveys. We thank F. Paquet for validating the hydroacoustic data as well as the editor and reviewers for their helpful suggestions which greatly improved the manuscript. This study is contributing to the program of Québec–Océan, a strategic cluster of oceanography scientists and their partners, funded by the Fonds de Recherche du Québec – Nature et technologies (FRQNT).
Supplementary Material
The Supplementary Material for this article can be found online at: https://www.frontiersin.org/articles/10.3389/fmars.2020.00626/full#supplementary-material
References
Abrahms, B., Hazen, E. L., Aikens, E. O., Savoca, M. S., Goldbogen, J. A., Bograd, S. J., et al. (2019). Memory and resource tracking drive blue whale migrations. Proc. Natl. Acad. Sci. U.S.A. 116, 5582–5587. doi: 10.1073/pnas.1819031116
Akiyama, Y., Akamatsu, T., Rasmussen, M. H., Iversen, M. R., Iwata, T., Goto, Y., et al. (2019). Leave or stay? Video-logger revealed foraging efficiency of humpback whales under temporal change in prey density. PLoS One 14:e0211138. doi: 10.1371/journal.pone.0211138
Alonzo, S. H., Switzer, P. V., and Mangel, M. (2003). An ecosystem-based approach to management: using individual behaviour to predict the indirect effects of Antarctic krill fisheries on penguin foraging. J. Appl. Ecol. 40, 692–702. doi: 10.1046/j.1365-2664.2003.00830.x
Aulanier, F., Simard, Y., Roy, N., Gervaise, C., and Bandet, M. (2016). Spatial-temporal exposure of blue whale habitats to shipping noise in St. Lawrence system. DFO Can. Sci. Advis. Sec. Res. Doc. 90, 4–26.
Benkort, D., Plourde, S., Winkler, G., Cabrol, J., Ollier, A., Cope, L.-E., et al. (2019). Individual-based modeling explains the contrasted seasonality in size, growth, and reproduction of the sympatric Arctic (Thysanoessa raschii) and Nordic krill (Meganyctiphanes norvegica) in the St. Lawrence Estuary, eastern Canada. Limnol. Oceanogr. 64, 1–21. doi: 10.1002/lno.11032
Benoit-Bird, K. J., Battaile, B. C., Heppell, S. A., Hoover, B., Irons, D., Jones, N., et al. (2013). Prey patch patterns predict habitat use by top marine predators with diverse foraging strategies. PLoS One 8:53348. doi: 10.1371/journal.pone.0053348
Blais, M., Galbraith, P. S., Plourde, S., Scarratt, M., Devine, L., and Lehoux, C. (2019). Chemical and biological oceanographic conditions in the estuary and gulf of St. Lawrence during 2017. DFO Can. Sci. Advis. Sec. Res. 2019:56.
Boyd, I. L. (1996). Temporal scales of foraging in a marine predator. Ecology 77, 426–434. doi: 10.2307/2265619
Cabrol, J., Trombetta, T., Amaudrut, S., Aulanier, F., Sage, R., Tremblay, R., et al. (2019). Trophic niche partitioning of dominant North-Atlantic krill species, Meganyctiphanes norvegica, Thysanoessa inermis, and T. raschii. Limnol. Oceanogr. 64, 165–181. doi: 10.1002/lno.11027
Chion, C., Turgeon, S., Michaud, R., Landry, J.-A., and Parrott, L. (2009). Portrait de la navigation dans le parc marin du Saguenay-Saint-Laurent. Parcs. Canada 2009, 1–86.
Christiansen, F., Rasmussen, M., and Lusseau, D. (2013). Whale watching disrupts feeding activities of minke whales on a feeding ground. Mar. Ecol. Prog. Ser. 478, 239–251. doi: 10.3354/meps10163
Cochrane, N. A., Sameoto, D. D., and Herman, A. W. (2000). Scotian Shelf euphausiid and silver hake population changes during Scotian Shelf euphausiid and silver hake population changes during 1984 - 1996 measured by multi-frequency acoustics. ICES J. Mar. Sci. 57, 122–132. doi: 10.1006/jmsc.1999.0563
Cochrane, N. A., Sameoto, D. D., Herman, A. W., and Neilson, J. L. (1991). Multiple-frequency acoustic backscattering and zooplankton aggregations in the Inner Scotian shelf basins. Can. J. Fish. Aquat. Sci. 48, 340–355. doi: 10.1139/f91-046
Cohen, J. (1977). Statistical Power Analysis For The Behavioral Science. New York, NY: Academic Press.
Costa, D. P., Croxall, J. P., and Duck, C. D. (1989). Foraging energetics of Antarctic fur seals in relation to changes in prey availability. Ecology 70, 596–606. doi: 10.2307/1940211
Cresswell, K. A., Wiedenmann, J., and Mangel, M. (2008). Can macaroni penguins keep up with climate- and fishing-induced changes in krill? Polar Biol. 31, 641–649. doi: 10.1007/s00300-007-0401-0
Croll, D. A., Acevedo-Gutiérrez, A., Tershy, B. R., and Urbán-Ramírez, J. (2001). The diving behavior of blue and fin whales: is dive duration shorter than expected based on oxygen stores? Comp. Biochem. Physiol. Part A Mol. Integr. Physiol. 129, 797–809. doi: 10.1016/S1095-6433(01)00348-8
Di Clemente, J., Christiansen, F., Pirotta, E., Steckler, D., Wahlberg, M., and Pearson, H. C. (2018). Effects of whale watching on the activity budgets of humpback whales, Megaptera novaeangliae (Borowski, 1781), on a feeding ground. Aquat. Conserv. Mar. Freshw. Ecosyst. 28, 810–820. doi: 10.1002/aqc.2909
Doniol-Valcroze, T., Lesage, V., Giard, J., and Michaud, R. (2011). Optimal foraging theory predicts diving and feeding strategies of the largest marine predator. Behav. Ecol. 22, 880–888. doi: 10.1093/beheco/arr038
Doniol-Valcroze, T., Lesage, V., Giard, J., and Michaud, R. (2012). Challenges in marine mammal habitat modelling: evidence of multiple foraging habitats from the identification of feeding events in blue whales. Endanger. Spec. Res. 17, 255–268. doi: 10.3354/esr00427
Dunn, R. E., Wanless, S., Daunt, F., Harris, M. P., and Green, J. A. (2020). A year in the life of a north Atlantic seabird: behavioural and energetic adjustments during the annual cycle. Sci. Rep. 10, 1–11. doi: 10.1038/s41598-020-62842-x
Emlen, J. M. (1966). The role of time and energy in food preference. Am. Nat. 100, 611–617. doi: 10.1086/282455
Flores, H., Atkinson, A., Kawaguchi, S., Krafft, B. A., Milinevsky, G., Nicol, S., et al. (2012). Impact of climate change on Antarctic krill. Mar. Ecol. Prog. Ser. 458, 1–19. doi: 10.3354/meps09831
Fortune, S. M. E., Trites, A. W., Mayo, C. A., Rosen, D. A. S., and Hamilton, P. K. (2013). Energetic requirements of North Atlantic right whales and the implications for species recovery. Mar. Ecol. Prog. Ser. 478, 253–272. doi: 10.3354/meps10000
Galbraith, P. S., Chassé, J., Caverhill, C., Nicot, P., Gilbert, D., Lefaivre, D., et al. (2018). Physical oceanographic conditions in the gulf of St. Lawrence during 2017. DFO Can. Sci. Advis. Sec. Res. Doc. 50:79.
Gavrilchuk, K., Lesage, V., Ramp, C., Sears, R., Bérubé, M., Bearhop, S., et al. (2014). Trophic niche partitioning among sympatric baleen whale species following the collapse of groundfish stocks in the Northwest Atlantic. Mar. Ecol. Prog. Ser. 497, 285–301. doi: 10.3354/meps10578
Goldbogen, J. A., Calambokidis, J., Oleson, E., Potvin, J., Pyenson, N. D., Schorr, G., et al. (2011). Mechanics, hydrodynamics and energetics of blue whale lunge feeding: efficiency dependence on krill density. J. Exp. Biol. 214, 131–146. doi: 10.1242/jeb.054726
Goldbogen, J. A., Calambokidis, J., Shadwick, R. E., Oleson, E. M., McDonald, M. A., and Hildebrand, J. A. (2006). Kinematics of foraging dives and lunge-feeding in fin whales. J. Exp. Biol. 209, 1231–1244. doi: 10.1242/jeb.02135
Goldbogen, J. A., Southall, B. L., DeRuiter, S. L., Calambokidis, J., Friedlaender, A. S., Hazen, E. L., et al. (2013). Blue whales respond to simulated mid-frequency military sonar. Proc. Biol. Sci. 280:20130657. doi: 10.1098/rspb.2013.0657
Guilpin, M., Lesage, V., McQuinn, I., Goldbogen, J., Potvin, J., Jeanniard-du-Dot, T., et al. (2019). Foraging energetics and prey density requirements of western North Atlantic blue whales in the Estuary and Gulf of St. Lawrence, Canada. Mar. Ecol. Prog. Ser. 625, 205–223. doi: 10.3354/meps13043
Hazen, E. L., Friedlaender, A. S., and Goldbogen, J. A. (2015). Blue whales (Balaenoptera musculus) optimize foraging efficiency by balancing oxygen use and energy gain as a function of prey density. Sci. Adv. 1:e1500469. doi: 10.1126/sciadv.1500469
Hemmingsen, A. M. (1960). Energy metabolism as related to body size and respiratory surfaces, and its evolution. Rep. Stem Meml. Hosp. Nordisk Insulin Lab. 9:110.
Hin, V., Harwood, J., and de Roos, A. M. (2019). Bio-energetic modeling of medium-sized cetaceans shows high sensitivity to disturbance in seasons of low resource supply. Ecol. Appl. 29, 1–19. doi: 10.1002/eap.1903
Houston, A. I., Prosser, E., and Sans, E. (2012). The cost of disturbance: a waste of time and energy? Oikos 121, 597–604. doi: 10.1111/j.1600-0706.2011.19594.x
Houston, A. I., Stephens, P. A., Boyd, I. L., Harding, K. C., and McNamara, J. M. (2007). Capital or income breeding? A theoretical model of female reproductive strategies. Behav. Ecol. 18, 241–250. doi: 10.1093/beheco/arl080
Hutchings, J. A., Côté, I. M., Dodson, J. J., Fleming, I. A., Jennings, S., Mantua, N. J., et al. (2012). Climate change, fisheries, and aquaculture: trends and consequences for Canadian marine biodiversity. Environ. Rev. 20, 220–311. doi: 10.1139/a2012-013
Irvine, L. M., Palacios, D. M., Lagerquist, B. A., and Mate, B. R. (2019). Scales of blue and fin whale feeding behavior off California, USA, with implications for prey patchiness. Front. Ecol. Evol. 7:338. doi: 10.3389/fevo.2019.00338
Kawamura, A. (1980). A review of food of balaenopterid whales. Sci. Rep. Whales Res. Inst. 32, 155–197.
Kleiber, M. (1975). The Fire Of Life: An Introduction To Animal Energetics, 2nd Edn, Huntington, NY: Robert E. Krieger.
Lesage, V., Gavrilchuk, K., Andrews, R. D., and Sears, R. (2017a). Foraging areas, migratory movements and winter destinations of blue whales from the western North Atlantic. Endanger. Spec. Res. 34, 27–43. doi: 10.3354/esr00838
Lesage, V., Omrane, A., Doniol-Valcroze, T., and Mosnier, A. (2017b). Increased proximity of vessels reduces feeding opportunities of blue whales in the St. Lawrence Estuary, Canada. Endanger. Spec. Res. 32, 351–361. doi: 10.3354/esr00825
Lesage, V., Gosselin, J., Lawson, J. W., Mcquinn, I., Moors-Murphy, H., Plourde, S., et al. (2018). Habitats important to blue whales (Balaenoptera musculus) in the Western North Atlantic. DFO Can. Sci. Advis. Sec. Res. 80:50.
Loncarevic, B. D., Piper, D. J. W., and Fader, G. B. J. (1992). Application of high-quality bathymetry to geological interpretation on the scotian shelf. Geosci. Canada 19, 5–13.
Long, Z., Perrie, W., Chassé, J., Brickman, D., Guo, L., Drozdowski, A., et al. (2016). Impacts of climate change in the Gulf of St. Lawrence. Atmos. Ocean 54, 337–351. doi: 10.1080/07055900.2015.1029869
Lusseau, D., Bain, D. E., Williams, R., and Smith, J. C. (2009). Vessel traffic disrupts the foraging behavior of southern resident killer whales Orcinus orca. Endanger. Spec. Res. 6, 211–221. doi: 10.3354/esr00154
Martensson, P.-E., Nordøy, E. S., and Blix, A. S. (1994). Digestibility of krill (Euphausia superba and Thysanoessa sp.) in minke whales (Balaenoptera acutorostrata) and crabeater seals (Lobodon carcinophagus). Br. J. Nutr. 72, 713–716. doi: 10.1079/BJN19940073
Martins, C. C. A. (2012). Study of Baleen Whale’s Ecology and Interaction with Maritime Traffic Activities to Support Management of Complex Socio-ecological System. Ph.D. thesis, Université de Montréal, Montréal.
McBride, M. M., Dalpadado, P., Drinkwater, K. F., Godø, O. R., Hobday, A. J., Hollowed, A. B., et al. (2014). Krill, climate, and contrasting future scenarios for Arctic and Antarctic fisheries. ICES J. Mar. Sci. 71, 1934–1955. doi: 10.1093/icesjms/fsu002
McHuron, E. A., Schwarz, L. K., Costa, D. P., and Mangel, M. (2018). A state-dependent model for assessing the population consequences of disturbance on income-breeding mammals. Ecol. Modell. 385, 133–144. doi: 10.1016/j.ecolmodel.2018.07.016
McQuinn, I. H., Gosselin, J., Bourassa, M., Mosnier, A., St-Pierre, J.-F., Plourde, S., et al. (2016). The spatial association of blue whales (Balaenoptera musculus) with krill patches (Thysanoessa spp. and Meganyctiphanes norvegica) in the estuary and northwestern Gulf of St. Lawrence. DFO Can. Sci. Advis. Sec. Res. Doc. 104, 4–19.
McQuinn, I. H., Plourde, S., St. Pierre, J.-F., and Dion, M. (2015). Spatial and temporal variations in the abundance, distribution, and aggregation of krill (Thysanoessa raschii and Meganyctiphanes norvegica) in the lower estuary and Gulf of St. Lawrence. Prog. Oceanogr. 131, 159–176. doi: 10.1016/j.pocean.2014.12.014
McWilliams, S. R., Guglielmo, C., Pierce, B., and Klaassen, M. (2004). Flying, fasting, and feeding in birds during migration: a nutritional and physiological ecology perspective. J. Avian Biol. 35, 377–393. doi: 10.1111/j.0908-8857.2004.03378.x
Meyer-Gutbrod, E. L., and Greene, C. H. (2018). Uncertain recovery of the North Atlantic right whale in a changing ocean. Glob. Chang. Biol. 24, 455–464. doi: 10.1111/gcb.13929
Mori, Y. (1998). The optimal patch use in divers: optimal time budget and the number of dive cycles during bout. J. Theor. Biol. 190, 187–199. doi: 10.1006/jtbi.1997.0550
New, L. F., Clark, J. S., Costa, D. P., Fleishman, E., Hindell, M. A., Klanjšček, T., et al. (2014). Using short-term measures of behaviour to estimate long-term fitness of southern elephant seals. Mar. Ecol. Prog. Ser. 496, 99–108. doi: 10.3354/meps10547
New, L. F., Harwood, J., Thomas, L., Donovan, C., Clark, J. S., Hastie, G., et al. (2013a). Modelling the biological significance of behavioural change in coastal bottlenose dolphins in response to disturbance. Funct. Ecol. 27, 314–322. doi: 10.1111/1365-2435.12052
New, L. F., Moretti, D. J., Hooker, S. K., Costa, D. P., and Simmons, S. E. (2013b). Using energetic models to investigate the survival and reproduction of beaked whales (family Ziphiidae). PLoS One 8:e68725. doi: 10.1371/journal.pone.0068725
Nicol, S., Foster, J., and Kawaguchi, S. (2012). The fishery for Antarctic krill - recent developments. Fish Fish. 13, 30–40. doi: 10.1111/j.1467-2979.2011.00406.x
Nordøy, E. S., Folkow, L. P., Martensson, P.-E., and Blix, A. S. (1995). “Food requirements of northeast Atlantic minke whales,” in Whales, Seals, Fish and Man. Developments in Marine Biology 4, eds C. S. Baker, L. M. Herman, and A. Perry (Amsterdam: Elsevier Science), 307–317. doi: 10.1016/s0163-6995(06)80032-6
Ollier, A., Chabot, D., Audet, C., and Winkler, G. (2018). Metabolic rates and spontaneous swimming activity of two krill species (Euphausiacea) under different temperature regimes in the St. Lawrence Estuary, Canada. J. Crustac. Biol. 2018:ruy028. doi: 10.1093/jcbiol/ruy028
Olsen, M. A., Blix, A. S., Utsi, T. H., Sørmo, W., and Mathiesen, S. D. (2000). Chitinolytic bacteria in the minke whale forestomach. Can. J. Microbiol. 46, 85–94. doi: 10.1139/w99-112
Owen, K., Kavanagh, A. S., Warren, J. D., Noad, M. J., Donnelly, D., Goldizen, A. W., et al. (2016). Potential energy gain by whales outside of the Antarctic: prey preferences and consumption rates of migrating humpback whales (Megaptera novaeangliae). Polar Biol. 11:e0156698. doi: 10.1007/s00300-016-1951-9
Pirotta, E., Booth, C. G., Costa, D. P., Fleishman, E., Kraus, S. D., Lusseau, D., et al. (2018). Understanding the population consequences of disturbance. Ecol. Evol. 8, 9934–9946. doi: 10.1002/ece3.4458
Pirotta, E., Mangel, M., Costa, D. P., Goldbogen, J., Harwood, J., Hin, V., et al. (2019). Anthropogenic disturbance in a changing environment: modelling lifetime reproductive success to predict the consequences of multiple stressors on a migratory population. Oikos 128, 1340–1357. doi: 10.1111/oik.06146
Plourde, S., Grégoire, F., Lehoux, C., Galbraith, P. S., Castonguay, M., and Ringuette, M. (2014a). Effect of environmental variability on the Atlantic Mackerel (Scomber scombrus L.) stock dynamics in the Gulf of St. Lawrence. Fish. Ocean. 24, 347–363. doi: 10.1111/fog.12113
Plourde, S., McQuinn, I. H., Maps, F., St-Pierre, J.-F., Lavoie, D., and Joly, P. (2014b). Daytime depth and thermal habitat of two sympatric krill species in response to surface salinity variability in the Gulf of St Lawrence, eastern Canada. ICES J. Mar. Sci. 71, 272–281. doi: 10.1093/icesjms/fst023
Plourde, S., Starr, M., Devine, L., St-Pierre, J.-F., St-Amand, L., Joly, P., et al. (2014c). Chemical and biological oceanographic conditions in the Estuary and Gulf of St. Lawrence during 2011 and 2012. DFO Can. Sci. Advis. Sec. Res. Doc. 49, 5–46.
Plourde, S., Lehoux, C., McQuinn, I. H., and Lesage, V. (2016). Describing krill distribution in the western North Atlantic using statistical habitat models. DFO Can. Sci. Advis. Sec. Res. Doc. 2016, 111–134.
Potvin, J., Goldbogen, J. A., and Shadwick, R. E. (2012). Metabolic expenditures of lunge feeding rorquals across scale: implications for the evolution of filter feeding and the limits to maximum body size. PLoS One 7:e44854. doi: 10.1371/journal.pone.0044854
Pujol, G., Iooss, B., Janon, A., Boumhaout, K., Roustant, O., Song, E., et al. (2016). Sensitivity: Global Sensitivity Analysis of Model Outputs. R Package Version 1.12.1. Available online at: https://CRAN.R-project.org/package=sensitivity (accessed October 12, 2018).
Pyke, G., Pulliam, H. R., and Charnov, E. L. (1977). Optimal Foraging?: A selective review of theory and tests. Q. Rev. Biol. 52, 137–154. doi: 10.1086/409852
R Development Core Team (2017). R: A Language And Environment For Statistical Computing. Vienna: R Foundation for Sta- tistical Computing.
Reid, K., Watkins, J. L., Murphy, E. J., Trathan, P. N., Fielding, S., and Enderlein, P. (2010). Krill population dynamics at South Georgia: implications for ecosystem-based fisheries management. Mar. Ecol. Prog. Ser. 399, 243–252. doi: 10.3354/meps08356
Richardson, A. J. (2008). In hot water: zooplankton and climate change. ICES J. Mar. Sci. 65, 279–295. doi: 10.1093/icesjms/fsn028
Ronconi, R. A., and Burger, A. E. (2008). Limited foraging flexibility: increased foraging effort by a marine predator does not buffer against scarce prey. Mar. Ecol. Prog. Ser. 366, 245–258. doi: 10.3354/meps07529
Saguenay-St. Lawrence Marine Park (2020). Regulations. Available online at: http://parcmarin.qc.ca/wp-content/uploads/2016/10/ParcMarin-Regulations_v2_www-1.pdf (accessed June 17, 2020).
Sameoto, D. D. (1976). Distribution of sound scattering layers caused by euphausiids and their relationship to chlorophyll a concentrations in the Gulf of St. Lawrence Estuary. J. Fish. Res. Board Canada 33, 681–687. doi: 10.1139/f76-084
Schuler, A. R., Piwetz, S., Di Clemente, J., Steckler, D., Mueter, F., and Pearson, H. C. (2019). Humpback whale movements and behavior in response to whale-watching vessels in Juneau, AK. Front. Mar. Sci. 6:710. doi: 10.3389/fmars.2019.00710
Sears, R., and Calambokidis, J. (2002). Update COSEWIC Status Report on the Blue Whale Balaenoptera musculus in Canada, in COSEWIC Assessment And Update Status Report On the Blue Whale Balaenoptera musculus in Canada. Comm Status Endanger Wildl Canada Ottawa, 1-32. Available online at: http://www.sararegistry.gc.ca/virtual_sara/files/cosewic/sr_blue_whale_e.pdf
Senigaglia, V., Christiansen, F., Bejder, L., Gendron, D., Lundquist, D., Noren, D. P., et al. (2016). Meta-analyses of whale-watching impact studies: comparisons of cetacean responses to disturbance. Mar. Ecol. Prog. Ser. 542, 251–263. doi: 10.3354/meps11497
Seyboth, E., Groch, K. R., Dalla Rosa, L., Reid, K., Flores, P. A. C., and Secchi, E. R. (2016). Southern right whale (Eubalaena australis) reproductive success is influenced by krill (Euphausia superba) density and climate. Sci. Rep. 6:28205. doi: 10.1038/srep28205
Sibly, R. M., Nott, H. M. R., and Fletcher, D. (1990). Splitting behaviour into bouts. Anim. Behav. 39, 63–69. doi: 10.1016/S0003-3472(05)80726-2
Sigler, M. F., Tollit, D. J., Vollenweider, J. J., Thedinga, J. F., Csepp, D. J., Womble, J. N., et al. (2009). Steller sea lion foraging response to seasonal changes in prey availability. Mar. Ecol. Prog. Ser. 388, 243–261. doi: 10.3354/meps08144
Silva, M. A., Prieto, R., Jonsen, I., Baumgartner, M. F., and Santos, R. S. (2013). North Atlantic blue and fin whales suspend their spring migration to forage in middle latitudes: building up energy reserves for the journey? PLoS One 8:e076507. doi: 10.1371/journal.pone.0076507
Simard, Y., Roy, N., Giard, S., and Yayla, M. (2014). Canadian year-round shipping traffic atlas for 2013: volume 1, East coast marine waters. Can. Tech. Rep. Fish. Aquat. Sci. 3091:327.
Sims, D. W., Witt, M. J., Richardson, A. J., Southall, E. J., and Metcalfe, J. D. (2006). Encounter success of free-ranging marine predator movements across a dynamic prey landscape. Proc. Biol. Sci. 273, 1195–1201. doi: 10.1098/rspb.2005.3444
Skagen, S. K. (2006). Migration stopovers and the conservation of arctic-breeding Calidridine Sandpipers. Auk 123:313. doi: 10.1093/auk/123.2.313
Sparling, C. E., Georges, J. Y., Gallon, S. L., Fedak, M., and Thompson, D. (2007). How long does a dive last? Foraging decisions by breath-hold divers in a patchy environment: a test of a simple model. Anim. Behav. 74, 207–218. doi: 10.1016/j.anbehav.2006.06.022
Sydeman, W. J., Santora, J. A., Thompson, S. A., Marinovic, B., and Lorenzo, E. D. (2013). Increasing variance in North Pacific climate relates to unprecedented ecosystem variability off California. Glob. Chang. Biol. 19, 1662–1675. doi: 10.1111/gcb.12165
Thompson, D., and Fedak, M. A. (2001). How long should a dive last? A simple model of foraging decisions by breath-hold divers in a patchy environment. Anim. Behav. 61, 287–296. doi: 10.1006/anbe.2000.1539
Thums, M., Bradshaw, C. J. A., and Hindell, M. A. (2011). In situ measures of foraging success and prey encounter reveal marine habitat-dependent search strategies. Ecology 92, 1258–1270. doi: 10.1890/09-1299.1
Thums, M., Bradshaw, C. J. A., Sumner, M. D., Horsburgh, J. M., and Hindell, M. A. (2013). Depletion of deep marine food patches forces divers to give up early. J. Anim. Ecol. 82, 72–83. doi: 10.1111/j.1365-2656.2012.02021.x
Ward, E. J., Holmes, E. E., and Balcomb, K. C. (2009). Quantifying the effects of prey abundance on killer whale reproduction. J. Appl. Ecol. 46, 632–640. doi: 10.1111/j.1365-2664.2009.01647.x
Watanabe, Y. Y., Ito, M., and Takahashi, A. (2014). Testing optimal foraging theory in a penguin-krill system. Proc. R. Soc. Lond. Ser. B 281:20132376. doi: 10.1098/rspb.2013.2376
Watkins, J. L., and Murray, A. W. A. (1998). Layers of Antarctic krill, Euphausia superba: are they just long krill swarms? Mar. Biol. 131, 237–247. doi: 10.1007/s002270050316
Wiedenmann, J., Cresswell, K. A., Goldbogen, J. A., Potvin, J., and Mangel, M. (2011). Exploring the effects of reductions in krill biomass in the Southern Ocean on blue whales using a state-dependent foraging model. Ecol. Model. 222, 3366–3379. doi: 10.1016/j.ecolmodel.2011.07.013
Williams, R., Lusseau, D., and Hammond, P. S. (2006). Estimating relative energetic costs of human disturbance to killer whales (Orcinus orca). Biol. Conserv. 133, 301–311. doi: 10.1016/j.biocon.2006.06.010
Keywords: net energy gain, foraging energetics, krill density, climate change, whale-watching interaction, Estuary and Gulf of St. Lawrence
Citation: Guilpin M, Lesage V, McQuinn I, Brosset P, Doniol-Valcroze T, Jeanniard-du-Dot T and Winkler G (2020) Repeated Vessel Interactions and Climate- or Fishery-Driven Changes in Prey Density Limit Energy Acquisition by Foraging Blue Whales. Front. Mar. Sci. 7:626. doi: 10.3389/fmars.2020.00626
Received: 14 May 2020; Accepted: 08 July 2020;
Published: 03 August 2020.
Edited by:
Rebecca Dunlop, The University of Queensland, AustraliaReviewed by:
Elizabeth McHuron, University of California, Santa Cruz, United StatesGail Schofield, Queen Mary University of London, United Kingdom
Copyright © 2020 Guilpin, Lesage, McQuinn, Brosset, Doniol-Valcroze, Jeanniard-du-Dot and Winkler. This is an open-access article distributed under the terms of the Creative Commons Attribution License (CC BY). The use, distribution or reproduction in other forums is permitted, provided the original author(s) and the copyright owner(s) are credited and that the original publication in this journal is cited, in accordance with accepted academic practice. No use, distribution or reproduction is permitted which does not comply with these terms.
*Correspondence: Marie Guilpin, bWFyaWVndWlscGluQGdtYWlsLmNvbQ==