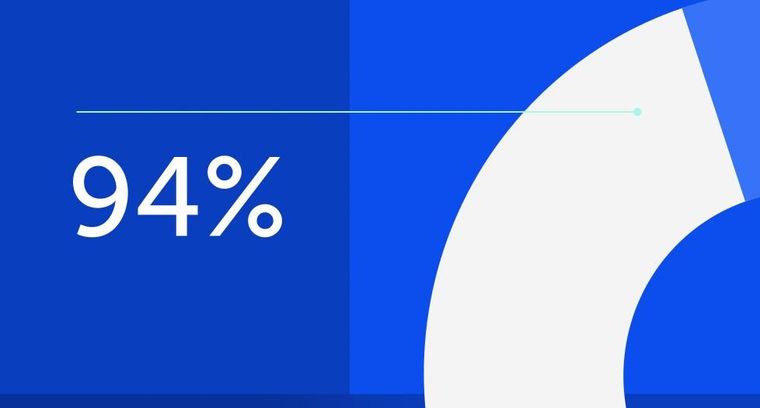
94% of researchers rate our articles as excellent or good
Learn more about the work of our research integrity team to safeguard the quality of each article we publish.
Find out more
ORIGINAL RESEARCH article
Front. Mar. Sci., 28 July 2020
Sec. Marine Fisheries, Aquaculture and Living Resources
Volume 7 - 2020 | https://doi.org/10.3389/fmars.2020.00614
Marine fisheries are social-ecological systems important for human health and livelihoods. However, research approaches that consider human–nature interactions within as well as between adjacent and distant fisheries are scarce. As such, we measured and modeled marine fisheries catches at local and regional scales over 65 years (1950–2014), assessed cross-scalar interactions among fishing types (artisanal, subsistence, industrial, recreational), and predicted future catches using the metacoupling framework, a new approach for evaluating human-nature interactions within and across adjacent and distant fisheries (metacouplings). Across taxa examined (mahi-mahi [Coryphaena hippurus], Atlantic bluefin tuna [Thunnus thynnus], cods [Gadidae]), 75% of catches (8.5 million metric tons [MMT]) were made by nations in their own exclusive economic zones (EEZs; Type 1 fishing). However, catches in adjacent EEZs (Type 2 fishing, 1.0 MMT) and distant EEZs and the high seas (Type 3 fishing, 1.9 MMT) increased substantially for all taxa at certain times, becoming consistently important for tuna and cods after 1980. Moreover, Types 1–3 fishing interacted in ways that affect humans differentially across fisheries. For instance, tuna artisanal and subsistence catches (Type 1) decreased with increasing Type 2 and Type 3 industrial fishing, respectively. Cod subsistence catches declined with increasing Type 2/3 industrial fishing and Type 1 artisanal fishing, whereas fishing-type interactions were largely positive for mahi-mahi, causing catches to increase across sectors. Overall, metacouplings affect humans in positive and negative ways that vary across scales and fisheries systems, galvanizing the need for metacoupling-informed fisheries research, policy, and management programs.
The world faces enormous social-ecological challenges, including climate change, food and nutrition insecurity, biodiversity loss, and water scarcity (Walther et al., 2002; Godfray et al., 2010; Cardinale et al., 2012; Mekonnen and Hoekstra, 2016). On one hand, these challenges reflect human population growth and resource consumption, placing us beyond planetary safe operating spaces for climate change, biosphere integrity, biogeochemical flows, and land-system change (Steffen et al., 2015). On the other hand, it is increasingly possible, amid rapid and long-distance movements of goods, services, information, money, and people throughout the world, to conceptualize and address social-ecological challenges at the speeds and spatiotemporal scales at which they occur. For instance, the telecoupling framework is a new approach for sustainable management of social-ecological systems widely separated in space (Liu et al., 2013). Telecoupling refers to socioeconomic and environmental interactions between social-ecological systems over long distances. For example, demand for soybeans in China is a distal driver of Amazonian deforestation, much like European and American biofuel mandates affect land, food, water, and energy availability throughout the world (Liu et al., 2013; Silva et al., 2017). Telecoupling research has catalyzed scientific and policy advancements in diverse realms such as land-use change, species invasion and migration, tourism, and foreign direct investment (Hulina et al., 2017; Sun et al., 2017). However, because many of humanity’s social-ecological challenges are multiscalar—simultaneously involving local, adjacent, and distant components—there is a need for approaches that transcend telecoupling by accounting for human-nature interactions both within and between adjacent and distant social-ecological systems.
The metacoupling framework encompasses and advances the telecoupling framework by explicitly accounting for multiscalar human-nature linkages (Liu, 2017). Metacouplings are human-nature interactions that occur within particular or local social-ecological systems (intracouplings, hereafter Type 1 interactions) as well as between adjacent systems (pericouplings, Type 2) and between distant systems (telecouplings, Type 3; Figure 1). By incorporating local, adjacent, and distant interactions, the metacoupling framework allows researchers to assess cross-scalar effects (e.g., impacts of Type 3 on Type 1 interactions), feedbacks, and tradeoffs that may not be apparent when focusing on particular scales or systems. In addition, by using the metacoupling framework to evaluate three coupling levels simultaneously, researchers can ascertain the relative importance of each, which is important for determining possible ecological or policy interventions. However, metacoupling research has been largely qualitative to date (Carlson et al., 2018b; Schaffer-Smith et al., 2018), creating a need for approaches to quantitatively model metacouplings, cross-scalar interactions, the relative importance of different couplings, and sustainability outcomes.
Figure 1. Diagram illustrating metacoupling: human-nature interactions (arrows) within a particular or local social-ecological system (Type 1) as well as between adjacent systems (Type 2) and between distant systems (Type 3).
The metacoupling framework has scarcely been applied to fisheries, which are prime examples of multiscalar human-natural systems because they encompass local, regional, and global flows of fish, fish products, money, information, and people (Eriksson et al., 2015; Carlson et al., 2017). For instance, policy and management decisions made by governments and commercial fishing companies at global scales can affect regional distribution of fishing effort and local abundance of fish stocks important for subsistence and artisanal fishers and associated markets (Crona et al., 2015; Carlson et al., 2018a). However, despite growth in social-ecological fisheries research emphasizing international connections among fisheries (Österblom and Folke, 2015; Crona et al., 2016; Tapia-Lewin et al., 2017), multiscalar studies focusing on local, adjacent, and distant interactions among biophysical and socioeconomic issues are scarce. This dearth of metacoupling research is significant because fisheries support food provisioning, food/nutrition security, employment, and livelihoods for billions of people throughout the world. For instance, fish is an important source of inexpensive, accessible protein, micronutrients, and omega-3 fatty acids as well as income and livelihoods for some of the world’s poorest, most food-insecure people (Roos et al., 2003; Bennett et al., 2018). Globally, fish consumption has grown from 9.0 kg/capita in 1961 to 20.5 kg/capita today, with 4.5 billion people relying of fish for at least 15 percent of their animal-derived protein (Béné et al., 2015; Food and Agriculture Organization [FAO], 2018). Moreover, depending on who fishes and when and where fishing occurs, multiscalar linkages between local norms and laws and international conventions will have different regulatory feedbacks on fisher behavior, stock sustainability, fish marketability, and hence income and profits. As such, metacoupling research exploring multiscalar human-nature interactions in fisheries has the potential to benefit human health and well-being and ecosystem conservation alike.
The purpose of this study was to use the metacoupling framework to characterize—and develop a modeling approach to understand—marine fisheries catches and associated local, adjacent, and distant interactions for taxa whose numbers are increasing, decreasing, and stable, with the goal of better managing and governing fisheries as multiscalar social-ecological systems. Fishes of interest included mahi-mahi (Coryphaena hippurus, increasing catches), Atlantic bluefin tuna (Thunnus thynnus, decreasing catches), and cods (family Gadidae, stable catches overall; Collette et al., 2011, 2015; International Union for Conservation of Nature [IUCN], 2019; Pauly et al., 2020). Our first objective was to use the metacoupling framework to evaluate fisheries catches classified as Type 1 (by nations in their own EEZs), Type 2 (in adjacent EEZs), and Type 3 (in distant EEZs and the high seas) in 1950–2014. Our second objective was to develop metacoupling time-series models to understand multiscalar interactions among fishing types (Type 1 artisanal, subsistence, industrial, recreational; Type 2 industrial; Type 3 industrial) and predict future catches. Such an analysis allowed us to test the prediction that metacouplings exhibit social-ecological variability—for instance, distant-water industrial fishing may negatively affect local artisanal and subsistence catches for some, but not all, taxa. Our third objective was to assess how extinction risks and total catches are related to the magnitude and direction of fishing-type interactions for each taxon. Our final objective was to use models to characterize and compare metacouplings in different fisheries and identify pathways for multiscalar, social-ecological fisheries governance that optimizes spatially heterogeneous fisheries services such as food supply, employment, livelihoods, and biodiversity.
The metacoupling framework is related to but advances concepts of teleconnections (environmental interactions between natural systems over distances; Bjerknes, 1969) and globalization (socioeconomic interactions between human systems over distances; Dreher et al., 2008) by simultaneously considering local, adjacent, and distant human-nature interactions. Similarly, the metacoupling framework advances a precursor paradigm (the telecoupling framework; Liu et al., 2013) by assessing social-ecological linkages at multiple spatial scales, rather than long distances only (Type 3 interactions). In the metacoupling framework, all couplings (metacouplings and Types 1, 2, and 3 interactions) are characterized by systems, flows, agents, causes, and effects (Figure 1). Coupled human-natural systems are classified according to their association with flows (movements of fish, fish products, money, information, etc.) as “sending” (systems where flows arise), “receiving” (systems that receive flows), or “spillover” (systems that affect, or are affected by, sending-receiving system interactions). Because fisheries metacouplings involve different types of fishing (artisanal, subsistence, industrial, recreational) with unique flows distributed across distinct spatial areas, a particular coupled human-natural system may be classified in multiple ways (sending, receiving, spillover) with respect to different fishing types. Agents are the autonomous decision-making entities such as individuals, organizations, and governments that facilitate or hinder couplings directly or indirectly through feedbacks, either transparent or hidden (Liu et al., 2013; Liu, 2017). By comparison, causes are environmental, socioeconomic, political, and cultural reasons why couplings occur, and effects are the social-ecological outcomes of couplings.
Three types of fishing were considered in this study: Type 1—fishing by nations within their own EEZs, including territories, collectivities, etc.; Type 2—fishing in EEZs of adjacent nations (those sharing land/maritime borders); and Type 3—fishing in EEZs of distant nations (those not sharing land/maritime borders) and the high seas. Types 2 and 3 fishing were exclusively industrial, so six fishing types were analyzed: Type 1 artisanal, subsistence, industrial, and recreational; Type 2 industrial; Type 3 industrial. For each taxon, catches of the six fishing types summed across the world were treated as quantitative indices of metacouplings.
Metacouplings were characterized and modeled using catches of mahi-mahi, Atlantic bluefin tuna (hereafter tuna), and cods in the world’s 280 EEZs every year from 1950 to 2014. These taxa were chosen because they represent broad population trends, and associated International Union for the Conservation of Nature (IUCN) statuses, exhibited by fish stocks throughout the world; mahi-mahi are generally increasing globally (IUCN Least Concern), tuna are decreasing (Endangered), and cods are relatively stable (Least Concern/Near Threatened/Vulnerable; Collette et al., 2011, 2015; International Union for Conservation of Nature [IUCN], 2019). These taxa were also chosen due to their broad importance throughout the world in terms of biodiversity, food supply, food/nutrition security and livelihoods (i.e., mahi-mahi in developing-country contexts), employment, recreation, and culture (Palko et al., 1982; Cohen et al., 1990; Collette, 1999).
Fisheries catch data were obtained from the Sea Around Us1 (Pauly et al., 2020). This database was created in response to the widely recognized problem of catch underestimation in fisheries data annually submitted to and distributed by the United Nations Food and Agriculture Organization (FAO), a problem that FAO acknowledges (Pauly and Zeller, 2016). For instance, FAO data routinely underestimate artisanal, subsistence, and recreational catches as well as discards (bycatch) and illegally caught fish. The Sea Around Us helps ameliorate this problem by supplementing FAO data with EEZ-specific data sources (e.g., peer-reviewed research, fisheries agency documents, independent research reports) to reconstruct industrial, artisanal, subsistence, and recreational catches in the world’s 280 EEZs every year in 1950–2014 (Pauly et al., 2020). It is preferable to account for artisanal, subsistence, recreational, discarded, and illegal catches that are known to occur—and which are quantified in EEZ-specific, non-FAO data sources—rather than treat these fishing types as “zeroes” (Pauly and Zeller, 2016), as other fisheries data sources tend to do. Overall, the Sea Around Us is a useful, peer-reviewed, regularly updated resource for quantifying and modeling fisheries catches amid widely known limitations of typical marine fisheries data.
The Sea Around Us is structured so that catch (landing and discard) data can be downloaded in spreadsheet form and organized by nations that fished in particular EEZs in particular years. As such, spreadsheets for each taxon were used to identify Types 1–3 fishing by comparing the geographic proximity between fishing nations and EEZs where fishing took place in 1950–2014. Catch data were also subdivided by fishing sector (artisanal, subsistence, industrial, recreational), which enabled assessment of sectorial metacoupling relationships such as effects of Type 3 industrial fishing on Type 1 subsistence fishing. Although we used the Sea Around Us because it included the multiscalar catch data necessary to address our objectives, national and international databases such as FAOSTAT2 and UN Comtrade3 are also conducive for evaluating metacouplings across international trade networks and food systems. For instance, these databases could be used to classify local, regional, and global flows of wheat, soybeans, rice, and other foodstuffs as Type 1, 2, or 3, allowing researchers to compare food-system metacouplings in different areas of the world and generate policy-relevant insights for enhancing food provisioning and safeguarding food/nutrition security.
Industrial fishing is known to reduce artisanal and subsistence catches, alter species composition, and force fishers to migrate to new fishing locations (Pauly, 2006; Belhabib et al., 2014), so it was hypothesized that Types 2 and 3 industrial fishing would negatively affect Type 1 artisanal and subsistence fishing. This effect was predicted to be highly negative for Atlantic bluefin tuna, a species whose catches have declined substantially over 65 years (Pauly et al., 2020) and is thus vulnerable to fishing-type competition, and somewhat negative or neutral for mahi-mahi (increased catches) and cods (stable catches). In addition, Type 2/3 industrial fishing and Type 1 industrial fishing were predicted to negatively affect each other given that these fisheries operate using similar methods and fishing locations and hence potentially compete for the same fish. Type 2/3 industrial fishing was not expected to affect Type 1 recreational catches given the latter sector’s relatively small size, spatial extent, and typical operation in coastal areas away from industrial vessels (Cooke and Cowx, 2004; Arlinghaus and Cowx, 2008).
It was hypothesized that Type 1 fisheries (other than industrial) would generally not affect Type 2/3 industrial catches given the considerably larger size, fishing capacity, and geographic distribution of the latter sectors (Kroodsma et al., 2018). Type 1 artisanal and subsistence fishing were predicted to have neutral or positive effects on each other, except if declining catches promoted Type 1 fishing competition, as seems likely for tuna (Pauly et al., 2020). Type 1 artisanal and subsistence fishing were predicted to have neutral or positive effects on Type 1 industrial and recreational catches, especially for mahi-mahi, a species whose catches have increased considerably over 65 years (Pauly et al., 2020) and hence would be expected to have neutral, if not positive, interactions among fishing types. Moreover, it was hypothesized that Type 1 industrial fishing would have neutral or positive effects on other intranational, Type 1 fisheries, as it is generally not in the interest of nations to fish industrially in ways that impair their other fisheries, although exceptions exist (Carlson et al., 2018a). Type 1 recreational fishing was not expected to affect other Type 1 fisheries given the sector’s relatively small size and different fishing methods and locations relative to artisanal/subsistence/industrial fishing. Finally, it was hypothesized that total catches would increase across taxa as fishing-type interactions became stronger and more positive, the number of positive interactions increased, and the number of negative interactions decreased.
Catch trends were initially evaluated by plotting total catches (metacouplings) as well as Types 1, 2, and 3 catches in 1950–2014 for each taxon. In addition, flows of fish within and between fishing nations and world regions were visualized by constructing Sankey diagrams, wherein catches flow from left to right (sending to receiving systems) in proportion to tonnage (line width). Sankey diagrams depicting Types 1–3 fish flows were constructed using world-region definitions from the United Nations Statistics Division Geoscheme (United Nations Statistics Division [UNSD], 2020).
The above graphical approaches laid a foundation for mathematical modeling of metacoupling interactions among Types 1–3 fishing using time series analysis, particularly autoregressive integrated moving average (ARIMA) models. ARIMA modeling is a widely used approach for time series analysis (Hyndman and Athanasopoulos, 2018) that reliably predicts diverse phenomena such as fisheries catches (Tsitsika et al., 2007), vehicular traffic flow (Williams and Hoel, 2003), and wind speed (Kavasseri and Seetharaman, 2009). In addition, ARIMA modeling of fisheries metacouplings complements recent time series analysis of multiscalar interactions in trade of soybeans, wheat, and corn (Herzberger et al., 2019), helping advance the nascent field of metacoupling research in a manner relevant for biologists, managers, policy makers, and other stakeholders. Moreover, metrics for objectively evaluating ARIMA model performance, including mean absolute scaled error (MASE) and normalized root mean squared error (NRMSE; RMSE/standard deviation), are readily available (Hyndman and Athanasopoulos, 2018). High-performance ARIMA models that capture much of the variation in empirical data have NRMSE and MASE values close to zero, moderate-performance models have values around 1, and low-performance models have values greater than 1.
In the present study, ARIMA models were constructed to assess previous (1950–2014) and predict future (2015–2030) catch trends of mahi-mahi, tuna, and cods for each of six fishing types. These models were also used to evaluate effects of Types 1–3 fishing on each other for each taxon. The ARIMA approach uses underlying properties of time series data, particularly dependent variables’ lagged values and prediction errors, as predictors for modeling and ultimately forecasting future values of dependent variables. In combination with data differencing—calculating differences between current and previous values of dependent variables to stationarize time series—autoregression and moving average approaches in an ARIMA framework tend to enhance model fit relative to either approach independently (Hamilton, 1994; Hyndman and Athanasopoulos, 2018).
ARIMA models contain autoregressive parameters [AR(p)] and moving averages [MA(q)] as well as integrated [I(d)] terms, which stationarize time series by subtracting current values of dependent variables from previous values d times. Although standard ARIMA models do not explicitly account for major factors influencing dependent variables (e.g., effects of gross domestic product and population size/growth on global agricultural trade), they use observed data to capture time-series patterns by accounting for non-stationarity, seasonality, and auto-correlation, which endogenizes effects of major drivers. As such, standard ARIMA models provide an overall picture of time series trends by broadly accounting for major drivers of dependent variables (rather than isolating their effects), unless drivers represent time series themselves. In this case, major drivers can be added to ARIMA models as external regressors (Hamilton, 1994; Hyndman and Athanasopoulos, 2018), as they were in the present study. In fisheries catch models developed herein, the AR(p) term involved modeling natural-log-transformed catches based on their lagged values:
where Ytgf is catch at time t for taxon g (i.e., group) and fishing type f (Type 1 artisanal, subsistence, industrial, and recreational; Type 2 industrial; Type 3 industrial), δ is a constant, p is the AR polynomial order, ϕi terms are AR parameters, y(t–i)gf terms are lagged values of catch for taxon g and type f, and εtgf is an error term for taxon g and type f. The I(d) model term involved subtracting current catches from previous catches d times to stationarize time series:
where ydtgf is ytgf differenced d times and other terms are the same as above. The MA(q) model term involved modeling fisheries catches based on errors between predicted and observed catches over time:
where Ytgf is catch at time t for taxon g and fishing type f, δ is a constant, q is the MA polynomial order, θi terms are MA parameters, ε(t–i)gf terms are prediction errors for taxon g and type f, and εtgf is an error term for taxon g and type f. Collectively, these model terms constituted ARIMA models of the form:
This model structure was modified by adding external regressors to determine how catches of particular fishing types were affected by other fishing types for each taxon:
where Ytgf is catch at time t for taxon g and fishing type f, A is a matrix containing other fishing types for taxon g as external regressors, and other terms are the same as above.
Assumptions of ARIMA modeling were assessed in fisheries catch time series, including stationarity (via augmented Dickey–Fuller tests) and lack of autocorrelation (via Box–Ljung tests). Autocorrelation and partial autocorrelation functions were also used to ensure accurate parameter estimation via maximum-likelihood methods and information-theoretic model selection (Tsitsika et al., 2007). In particular, different constructions of each model—unique combinations of ARIMA parameters [p, d, q] and external regressors—were compared via Akaike’s Information Criterion (AIC) using the Arima function and xreg (external regressor) argument in RStudio Desktop version 1.2.5019 (RStudio, 2019). Measures were taken to avoid including uninformative model parameters (those that do not reduce model deviance). For instance, models with ΔAIC < 2 and one additional parameter relative to top-performing models were excluded from further consideration to ensure that final, most-parsimonious models only included informative parameters while receiving substantial AIC support (Burnham and Anderson, 2002).
For each taxon and fishing type, the ARIMA model that received the lowest AIC score and had random, independent residuals as inferred from a Box–Ljung test was treated as the final, most-parsimonious model. These models were used to evaluate how catches of particular fishing types were affected by other types, and to predict future catches for each fishing type-taxon combination. Models were validated by calculating MASE and NRMSE to compare model-predicted and observed fisheries catches; models with MASE and NRMSE considerably less than 1, indicating good to great performance, were used further. After model validation, interactions among fishing types were assessed for each taxon by referring to external regressor p values and coefficients derived from the A matrix in Equation 6. Only regressors with p-values < 0.05 were treated as significant variables influencing catches of particular fishing types. For each taxon, interactions among fishing types were summarized in conceptual diagrams wherein arrows connecting fishing types indicate significant catch relationships, and arrow width and color are proportional to interaction magnitude and direction (positive, negative). Relationships between total catches (1950–2014) and metacoupling interaction structure were also evaluated. For each taxon, interaction structure encompassed: (1) the relative degree of fishing-type interaction (i.e., sum of fishing-type ARIMA coefficients across types); (2) the number of positive interactions among fishing types; and, (3) the number of negative interactions among fishing types. Relationships were assessed using Pearson product-moment correlation coefficients. All analyses were performed in RStudio Desktop version 1.2.5019 (RStudio, 2019).
Mahi-mahi metacouplings (total catches) amounted to 7.0 × 106 metric tons (MT) in 1950–2014, increasing by an order of magnitude from 2.4 × 104 to 2.4 × 105 MT in this time period (Figure 2A). Type 1 catches exhibited a similar pattern, increasing from 2.3 × 104 to 2.0 × 105 MT and representing a consistently large annual percentage of metacouplings (mean 84%, range 54–97%). Type 3 mahi-mahi catches were relatively substantial in 1987 (39% of metacouplings), 1989 (43%), and 1991 (35%), whereas Type 2 catches were consistently small (mean 4.9 × 103 MT, range 1.4 × 102–2.3 × 104 MT), averaging 4% of metacouplings (Figure 2A).
Figure 2. Fisheries catches in 1950–2014 for (A) mahi-mahi, (B) Atlantic bluefin tuna, and (C) cods (family Gadidae). Catch types are metacouplings (total catch in all exclusive economic zones [EEZs] and the high seas), Type 1 (catches by nations in their own EEZs), Type 2 (catches in adjacent EEZs), and Type 3 (catches in distant EEZs and the high seas).
Tuna metacouplings totaled 2.8 × 106 MT in 1950–2014, but 2014 tonnage (1.8 × 104 MT) was little more than one third of 1950 tonnage (5.2 × 104 MT; Figure 2B). Metacouplings declined by 66% in 1955–1968 (7.8 × 104 to 2.7 × 104 MT), followed by a general increase to 2007 (6.4 × 104 MT) and a rapid fall to 2014. Type 1 tuna catches represented a large annual percentage of metacouplings in 1950–2014 (mean 64%, range 39–80%), although Types 1 and 3 catches were nearly identical in 1999 (40 and 39%, respectively; Figure 2B). Type 2 catches were relatively small in 1950–2014 (mean 7.3 × 103 MT, range 2.8 × 103–1.8 × 104 MT), averaging 17% of metacouplings.
Cod metacouplings totaled 1.6 × 106 MT in 1950–2014, peaking at 5.6 × 104 MT in 1992 but remaining stable overall from 1950 (2.4 × 104 MT) to 2014 (2.5 × 104 MT; Figure 2C). Type 1 catches followed a similar pattern, representing a large annual percentage of metacouplings (mean 63%, range 35–93%). Although Type 3 cod catches were relatively small in 1950–2014 (mean 7.1 × 103 MT), they increased by a factor of eight (4.4 × 103–3.5 × 104 MT) from 1982 to 1992, when they accounted for 61% of metacouplings (Figure 2C). Type 2 catches were consistently small in 1950–2014 (mean 2.9 × 103 MT, range 9.6 × 102–5.6 × 103 MT), averaging 12% of metacouplings.
In 1950–2014, Type 1 catches and associated intranational flows of mahi-mahi were largest in Eastern Asia (1.5 × 106 MT; China, Taiwan, Japan, South Korea), South America (1.2 × 106 MT; primarily Peru and Brazil), and South-central Asia (1.1 × 106 MT; primarily Pakistan and India; Figure 3). Regions where Types 2 and 3 flows were largest included the high seas (4.2 × 105 MT), Oceania (2.8 × 105 MT), Central America (3.8 × 104 MT), and Southeast Asia (3.7 × 104 MT). In the high seas, the largest-tonnage mahi-mahi fishers were from South America (1.8 × 105 MT; primarily Venezuela), Northern America (1.3 × 105 MT; United States), and Eastern Asia (7.4 × 104 MT; primarily Taiwan and Japan; Figure 3). Similarly, the United States, Taiwan, and Japan were the largest-tonnage fishers for Type 2/3 mahi-mahi in Oceania, Central America, and Southeast Asia. Overall, the USA’s Type 2/3 catches of mahi-mahi totaled 4.0 × 105 MT in 1950–2014, 55% of which was derived from Oceania, 32% from the high seas, and 9% from Central America (Figure 3). Likewise, Eastern Asian nations (China, Taiwan, Japan, South Korea) caught 41% and 32% of Type 2/3 mahi-mahi in the high seas and Oceania, respectively. In contrast, South American nations (Venezuela and Brazil) caught 99% of Type 2/3 mahi-mahi in the high seas (Figure 3).
Figure 3. Sankey diagram depicting flows of mahi-mahi (metric tons) within and between world regions from sending systems (left) to receiving systems (right) in 1950–2014. In the left margin, numbers to the left of the divider represent Type 1 fishing—total catches by each region within its own exclusive economic zones. Numbers to the right of the divider represent Type 2/3 fishing—total catches in each region by nations located outside the region. Numbers in the right margin are Type 2/3 catches by each region in other regions.
Type 1 catches and associated intranational flows of tuna in 1950–2014 were largest in Southern Europe (1.2 × 106 MT; primarily Spain and Italy), Northern Africa (3.1 × 105 MT; primarily Morocco and Tunisia), Northern America (1.9 × 105 MT; primarily the United States), and Northern Europe (1.3 × 105 MT; primarily Norway; Figure 4). Regions where Type 2/3 flows were largest included Northern Africa (2.3 × 105 MT), Southern Europe (2.0 × 105 MT), and the high seas (1.6 × 105 MT). In Northern Africa, the largest-tonnage tuna fishers were from Southern Europe (1.5 × 105 MT; primarily Spain and Italy) and Western Europe (5.8 × 104 MT; France and Germany; Figure 4). The largest-tonnage fishers in Southern Europe were from Western Europe (7.7 × 104 MT; France and Germany) and Northern Europe (6.7 × 104 MT; primarily Norway and Denmark). In the high seas, the largest-tonnage tuna fishers were from Northern America (6.3 × 104 MT; primarily the United States), Northern Africa (3.6 × 104 MT; primarily Tunisia and Morocco), and Western Europe (2.8 × 104 MT; France; Figure 4). The three regions that caught the most Type 2/3 tuna in 1950–2014—Western Europe (2.1 × 105 MT), Southern Europe (2.0 × 105 MT), and Northern America (9.0 × 104 MT)—exhibited spatially heterogeneous catch patterns. For instance, Western Europe caught Type 2/3 tuna in multiple regions, including Southern Europe (36% of tuna by tonnage), Northern Africa (27%), the high seas (13%), Middle Africa (13%), and Western Africa (8%; Figure 4). However, in Southern Europe, 74% of Type 2/3 tuna came from Northern Africa. Likewise, 70% of Northern America’s Type 2/3 tuna came from the high seas.
Figure 4. Sankey diagram depicting flows of Atlantic bluefin tuna (metric tons) within and between world regions from sending systems (left) to receiving systems (right) in 1950–2014. In the left margin, numbers to the left of the divider represent Type 1 fishing—total catches by each region within its own exclusive economic zones. Numbers to the right of the divider represent Type 2/3 fishing—total catches in each region by nations located outside the region. Numbers in the right margin are Type 2/3 catches by each region in other regions.
In 1950–2014, Type 1 catches and associated intranational flows of cods were largest at the EEZ level in Japan (main islands; 4.5 × 105 MT), France (Atlantic Coast; 2.4 × 105 MT), and New Zealand (1.2 × 105 MT; Figure 5). In contrast, Type 2/3 flows were largest in New Zealand (3.3 × 105 MT, primarily to Japan, South Korea, and Ukraine), the United Kingdom (1.6 × 105 MT to France), Ireland (6.1 × 104 MT to France), and Norway (2.6 × 104 MT to France). Type 2/3 catches exhibited wide spatial variability in 1950–2014, as France caught Type 2/3 cods in 19 EEZs throughout Northern and Western Europe—most notably the United Kingdom (50% of cods by tonnage), Ireland (19%), and Norway (8%)—but South Korea and Japan caught Type 2/3 cods in New Zealand alone (Figure 5).
Figure 5. Sankey diagram depicting flows of cods (family Gadidae, metric tons) from sending systems (left) to receiving systems (right) in 1950–2014, organized by exclusive economic zones (EEZs). In the left margin, numbers to the left of the divider represent Type 1 fishing—total catches by each nation within its own EEZ(s). Numbers to the right of the divider represent Type 2/3 fishing—total catches in each EEZ by other nations. Numbers in the right margin are Type 2/3 catches by each nation in other EEZs.
Interactions among fishing types occurred for all taxa but often in different ways. For mahi-mahi, catches of various fishing types were generally positively associated except for a negative relationship between artisanal and recreational catches (Figure 6A and Supplementary Table 1). Artisanal fishing was positively associated with subsistence fishing as well as Types 1–3 industrial fishing (p < 0.01). Similarly, mahi-mahi subsistence catches increased with artisanal (p < 0.01) and recreational (p = 0.02) catches; Type 1 industrial catches increased with artisanal catches (p < 0.01); and recreational catches increased with subsistence catches (p < 0.01; Figure 6A and Supplementary Table 1). Fishing types also interacted across spatial scales, as Type 2 industrial fishing was positively associated with Type 1 artisanal fishing (p < 0.01). ARIMA models predicted that future mahi-mahi catches will increase for all fishing types to the year 2030 (Supplementary Figure 1), continuing the increasing trends observed in 1950–2014 (Figure 2A).
Figure 6. Metacoupling relationships for (A) mahi-mahi, (B) Atlantic bluefin tuna, and (C) cods (family Gadidae). Fishing types are organized by spatial scale (Type 1, 2, 3) and sector (artisanal, subsistence, industrial, recreational). Positive interactions are depicted in black and negative interactions in red, with arrow width proportional to effect size (Supplementary Tables 1–3). Statuses of each taxon on the International Union for Conservation of Nature (IUCN) Red List are also included.
Metacoupling interactions were more nuanced and negative for tuna. Artisanal catches increased with subsistence catches but decreased as Type 2 industrial catches grew (p < 0.01; Figure 6B and Supplementary Table 2). Similarly, tuna subsistence catches increased with artisanal catches but decreased as Type 3 industrial catches grew (p < 0.01). In addition, Type 1 industrial fishing was positively associated with artisanal fishing, but—as for mahi-mahi—recreational catches decreased as artisanal catches increased (p < 0.01; Figure 6B and Supplementary Table 2). ARIMA models predicted that future tuna catches will generally decline across fishing types relative to 1950 levels, and decline or remain stable relative to 2014 levels (Supplementary Figure 2).
Similar to tuna, cods exhibited mixed positive and negative metacoupling interactions. Artisanal catches increased with Type 1 industrial catches (p = 0.02) but decreased as subsistence catches grew (p = 0.04; Figure 6C and Supplementary Table 3). Notably, cod subsistence catches were negatively associated with artisanal catches (p < 0.01) as well as Type 2 industrial (p = 0.03) and Type 3 industrial (p = 0.04) catches. In contrast, Type 1 industrial catches increased with artisanal catches and Type 3 industrial catches (p < 0.01), much like Type 3 industrial catches increased with Type 1 industrial catches (p < 0.01; Figure 6C and Supplementary Table 3). Cod recreational fishing did not affect, and was unaffected by, other fishing types. ARIMA models predicted that future cod catches will decrease for subsistence fishing but increase for artisanal and Type 1 industrial fishing relative to 2014 (and 1950) levels (Supplementary Figure 3). Recreational catches and Types 2 and 3 industrial catches were projected to remain consistent near 2014 levels.
Across taxa, the structure of metacoupling interactions among fishing types was related to extinction risk and total catches in 1950–2014. In particular, extinction risk decreased as fishing-type interactions became more positive and less negative. For instance, mahi-mahi, the taxon with the lowest extinction risk (IUCN Least Concern), had four bidirectional positive interactions and nine total positive interactions but only one negative interaction (Figure 6A). In contrast, tuna had the greatest extinction risk (Endangered) with only one bidirectional positive interaction and three total positive interactions, along with three negative interactions. Cod had intermediate extinction risk (Least Concern/Near Threatened/Vulnerable) and intermediate numbers of bidirectional positive interactions (two) and total positive interactions (four) as well as four negative interactions (Figure 6C). Across taxa, total catches in 1950–2014 increased as fishing-type interactions became stronger and more positive (r = 0.99, p = 0.001). However, total catches declined as the number of negative metacoupling interactions increased (r = −0.99, p = 0.04).
Despite growing recognition of the complex ways in which fisheries’ social-ecological linkages span local to global scales (Crona et al., 2015; Österblom and Folke, 2015; Tapia-Lewin et al., 2017), few studies have explored how these multiscalar interactions affect more than one fishery over multidecadal time scales, and no studies have modeled fisheries metacouplings. We helped fill these knowledge gaps by illustrating how marine fisheries are embedded in networks of local, adjacent, and distant human-nature interactions. Our results show how metacouplings are dynamic social-ecological linkages that vary widely within and among taxa such as mahi-mahi, Atlantic bluefin tuna, and cods, whose catch trajectories are increasing, decreasing, and relatively stable, respectively (Pauly et al., 2020), but whose metacouplings have been masked until now. Type 1 fishing predominanted by tonnage for all taxa in 1950–2014, which is predictable as nations routinely fish in their own EEZs. More interesting is that fact that Type 2/3 catches increased substantially for all taxa at certain times, becoming consistently important for tuna and cods after 1980. The latter period coincided with opening of Japanese sushi–sashimi markets, which dramatically increased profitability of tuna exploitation (Fromentin and Powers, 2005), and ongoing cod overexploitation (Finlayson and McCay, 2000)—both of which promoted distant-water fishing resulting in Type 2/3 catches.
Catch landscapes (networks of local, adjacent, and distant fish flows; Figures 3–5) were similar for all taxa in that Type 1 fishing was distributed across many EEZs and world regions, only a few of which had large Type 1 catches (> 2.0 × 105 MT). However, taxa differed with respect to Types 2 and 3 catches, reflecting differences in geographic distributions, the wide-ranging nations (with different fishing capacities) that catch them, and distinct fisheries policies and regulations (Fromentin and Powers, 2005; Aranda et al., 2012). For instance, Type 2/3 mahi-mahi fishing was concentrated in regions overlapping the species’ circumtropical and circumsubtropical distribution (Oceania, high seas; Whoriskey et al., 2011) and carried out by nations from three principal regions (Eastern Asia, Northern America, South America). In contrast, Type 2/3 tuna fishing primarily happened in, and was initiated by nations from, six world regions throughout Africa, Europe, North America, and the high seas. Type 2/3 cod fishing was relatively evenly distributed in EEZs spanning nine world regions, reflecting the wide geographic distribution of gadid fishes such as Atlantic cod (Gadus morhua), Pacific cod (G. macrocephalus), and haddock (Melanogrammus aeglefinus; International Union for Conservation of Nature [IUCN], 2019; Pauly et al., 2020). Even so, cod were primarily caught by France, Japan, and South Korea, nations with vibrant, culturally important fisheries for gadid fishes (Gwak and Nakayama, 2011; Makino, 2013; Neat et al., 2014). The United States caught comparatively little cod, but catches were exclusively intranational and no other countries fished for cod in the USA, reflecting relatively strict cod harvest regulations designed to rebuild overfished stocks (National Oceanic and Atmospheric Administration [NOAA], 2017). These taxonomic differences in spatial catch patterns, reflecting unique biological and socio-political conditions influencing each taxon studied herein, represent metacoupling diversity relevant for fisheries management. By understanding fisheries’ metacoupling similarities and differences, decision-makers can design ecologically, socioeconomically appropriate management and governance programs that optimize local, adjacent, and distant human-nature interactions that are unique to—and shared among—individual fisheries.
Adding social-ecological breadth and depth to fish population analysis, the metacoupling framework and models shed light on reasons for empirically observed catch patterns. For instance, the largely positive relationships among Types 1–3 mahi-mahi fishing—alongside the species’ broad geographic distribution and fast growth (Whoriskey et al., 2011)—provide context for understanding why catches of mahi-mahi were the largest of taxa examined and increased substantially in 1950–2014. Not only are mahi-mahi biologically equipped to produce large, widely distributed populations, their r-selected life-history strategy (short lifespan, early maturation, high reproductive capacity, rapid growth; Winemiller and Rose, 1992) enables largely non-inhibitory interactions among multiple fishing types and explains the increasing 65-year catch trend and low extinction risk (IUCN Least Concern). In comparison, tuna and cod mature later in life and are less prolific, making them less biologically resilient to fishing pressure and helping explain why catch trajectories were stable/declining in 1950–2014, interactions among Types 1–3 fishing were more inhibitory, and extinction risks were higher than for mahi-mahi. Metacouplings warrant further investigation, as biological attributes of fishes interact with socioeconomic, political, and cultural conditions of fisheries, likely influencing the fishing-type relationships we observed. Even so, metacoupling models developed herein represent a methodological advancement for understanding how different fisheries interact within and between adjacent and distant coupled human-natural systems. Likewise, ARIMA models were useful for predicting future catches across taxa and fishing types. Although type-specific catch predictions generally paralleled taxon-specific trends in total catch observed in 1950–2014, important details emerged, including projected Type 1 catch declines (e.g., cod subsistence, tuna artisanal) and increases (e.g., mahi-mahi subsistence and artisanal, cod artisanal) relative to 2014 and earlier catches.
Such trends have implications for food supply and employment, particularly in nations where subsistence and artisanal fishing are common practices and drivers of human health and well-being. For instance, subsistence catches of cods in Malta, South Korea, France, Canada, and the United States totaled 1.2 × 105 MT in 1950–2014 (Supplementary Table 4), supporting food supplies that are rich in protein, vitamins, minerals, and omega-3 fatty acids (Gordon and Roberts, 1977; dos Santos et al., 1993). Projected declines in cod subsistence catches owing to stable Type 2/3 industrial catches may negatively affect subsistence-oriented food supplies in these nations, but all are developed countries where food availability is not a concern. As such, if cod subsistence catches decline below established food-supply goals, alternative food options will be readily available. However, projected declines in tuna artisanal catches owing to stable Type 2 industrial catches may negatively affect income and employment of small-scale fishers in 29 nations throughout Africa, Asia, Europe, and parts of the Caribbean, North America, and South America that collectively caught 2.8 × 105 MT of tuna in 1950–2014 (Supplementary Table 4).
Type 2/3 fishing can negatively affect food supply, food/nutrition security, employment, and livelihoods through illegal fishing—fishing within the EEZs of another country without access permission (Pauly et al., 2020)—which decreases subsistence and artisanal catches, changes species composition, and forces fishers to migrate to new fishing locations (Belhabib et al., 2014). Access agreements may or may not be written, as some arrangements are tacitly based on historical rights to fish in particular EEZs. As such, databases like the Sea Around Us have limited illegal fishing data in most areas of the world, with the exception of West Africa, where poaching is comparatively common (Agnew et al., 2009). Between 1950 and 2010, 3.8 × 107 MT of fish (all taxa) were illegally caught via Type 2/3 fishing in West African EEZs (Supplementary Table 5), more than three times the combined catch of mahi-mahi, tuna, and cods. Given the high profitability of poaching, it is estimated that fines for illegal cod and haddock fishing need to be 38 times larger to make the cost of illegal fishing equal to the gain; values for highly valuable species such as Atlantic bluefin tuna are likely greater (Sumaila et al., 2006). In 1950–2010, 72% of illegal catches in West African EEZs (2.7 × 107 MT) was taken in Mauritania by European nations (Italy, Lithuania, Netherlands, Romania, Spain, Ukraine) and the Russian Federation (Supplementary Table 5; Belhabib et al., 2012; Pauly et al., 2020). Illegal Type 2/3 catches were also relatively large in Senegal (3.9 × 106 MT taken by China and the Russian Federation), Sierra Leone (1.7 × 106 MT, fishers unspecified), Guinea (1.3 × 106 MT taken by China, South Korea, and Senegal), and Liberia (1.3 × 106 MT taken by China and Ghana; Supplementary Table 5). Worldwide, illegal fishers annually catch an estimated 1.1–2.6 × 107 MT valued at US$10–23.5 billion (Agnew et al., 2009), representing a global governance problem involving metacouplings that negatively affect subsistence and artisanal fishers, not to mention marine ecosystem sustainability.
However, projected changes in fisheries catches may also exert positive effects on human health and well-being. Species such as mahi-mahi are important for human diets and livelihoods in developing-country contexts because they are widely distributed (circumtropical, circumsubtropical), mature early, and grow fast, making them resilient to fishing mortality and capable of maintaining productive fisheries (Whoriskey et al., 2011). Predicted increases in mahi-mahi subsistence catches could enhance food/nutrition security in 27 countries (Supplementary Table 4), particularly developing countries where mahi-mahi subsistence catches grew by tens to hundreds of times in 1950–2014 (Saint Kitts and Nevis: 122x; Mayotte: 58x; Samoa: 27x; Turks and Caicos: 13x). Likewise, predicted increases in artisanal catches of mahi-mahi and cod may positively affect income and employment of artisanal fishers in 67 nations that collectively caught 2.9 × 106 MT of mahi-mahi and 8.0 × 104 MT of cods in 1950–2014 (Supplementary Table 4). In addition, projected increases in mahi-mahi artisanal and Type 1 industrial catches may create win-win scenarios for local income and employment due to the mutualistic metacoupling interaction observed herein. However, larger cod artisanal catches could contribute to reduced subsistence catches due to the inhibitory metacoupling relationship between these fishing types.
Sustainable fisheries management requires more than biological understanding; it demands synthesis of biological, economic, political, and cultural information involved in metacouplings across spatial scales. For instance, negative effects of Type 2/3 industrial fishing on artisanal and subsistence catches, as exhibited for tuna and cods across EEZs herein, reflect multiscalar human-nature interactions common to Canada, Italy, Morocco, Liberia, and other nations across the world (Belhabib et al., 2013a, b,c,d,e; Trindade Santos et al., 2013; Piroddi et al., 2014; Belhabib and Pauly, 2015a, b, c;Belhabib et al., 2015; Divovich et al., 2015; Etim et al., 2015; Nunoo et al., 2015; Seto et al., 2015). Likewise, metacoupling relationships among Type 1, small-scale fisheries sectors (artisanal, subsistence, recreational) warrant special consideration due to across-EEZ variability in how these sectors interact as well as methodological differences in how they are defined and quantified. For instance, declining subsistence catches are often linked to increasing artisanal catches due to economic developments and alternative income options within nations over time that make it possible for fishers to transition from subsistence to artisanal fishing, which has been observed in China, Dominica, Morocco, Saint Kitts and Nevis, and other countries (Belhabib et al., 2013b; Krumme et al., 2013; Ramdeen et al., 2014; Pauly and Le Manach, 2015). In addition, increasing artisanal catches can be associated with decreasing subsistence catches due to nationwide human dietary shifts reflecting cultural preferences for protein sources other than fish (e.g., chicken), as in Peru (Mendo and Wosnitza-Mendo, 2014; Carlson et al., 2018a).
Alternatively, artisanal and subsistence catches can be positively related—as observed for mahi-mahi and tuna across EEZs—when artisanal catches are used to estimate data-limited subsistence catches. For example, the take-home portion of artisanal catches—that which fishers bring home to feed themselves and their families—is defined as subsistence-derived fish in catch reconstructions for Guatemala, Malta, Spain, and many other EEZs throughout the world (Coll et al., 2015; Khalfallah et al., 2015; Lindop et al., 2015). Similarly, in Brazil and Honduras, subsistence catches are estimated by multiplying the number of artisanal fishers by their per capita catch of fish for home consumption (Freire et al., 2014; Funes et al., 2015). However, if artisanal catches grow to exceed legal limits, a process that can be driven by cultural beliefs that poaching for food is morally acceptable, resultant overfishing and misreporting can lead to negative associations between artisanal catches and subsistence harvest by fishers unaffiliated with artisanal take-home operations (Divovich et al., 2015), as observed for cods across EEZs.
Recreational fish harvest can also interact with subsistence and artisanal catches. For instance, economic growth within nations over time created job opportunities that enabled fishers to shift from subsistence livelihoods to cash-economy employment that provided disposable income and leisure time for pursuits like recreational fishing (Pauly et al., 2020). Such economic development contributed to subsistence-catch declines and domestic recreational-catch increases in China, Morocco, Saint Kitts and Nevis, and other countries (Belhabib et al., 2013b; Ramdeen et al., 2014; Pauly and Le Manach, 2015). In addition, some nations have tourism-based recreational fisheries, but data on domestic and tourism-based recreational catches are differentially available across EEZs. Hence, further research is needed to identify mechanisms linking recreational and subsistence catches as well as recreational and artisanal catches, which tend to be negatively associated in nations like Brazil, Peru, and the Cayman Islands (Begossi, 2006; Harper et al., 2009; Mendo and Wosnitza-Mendo, 2014).
Overall, it is important to consider heterogeneous linkages among fisheries sectors—particularly small-scale sectors—explaining overall trends in metacoupling interactions observed herein. Indeed, evaluation of mechanisms underlying fishing-sector interactions is a compelling avenue for future metacoupling research that can be facilitated by broader adoption of statistically rigorous, transparent catch recording and reporting systems and use of holistic multi-species, multi-gear catch analysis and reconstruction methods in nations across the world (Belhabib et al., 2013e; Piroddi et al., 2014; Ramdeen et al., 2014). In addition, amid widespread catch underreporting (Divovich et al., 2015; Lindop et al., 2015; Pauly et al., 2020), it is important to improve fisheries governance by enhancing monitoring, regulations, and enforcement systems and fostering better communication between fishers and fisheries management organizations—tasks that encompass multiscalar human-nature interactions and thus would benefit from continued metacoupling research.
Despite the broad applicability of metacoupling models, differential availability and quality of catch data across EEZs and fisheries sectors are limitations of the present study. In general, data are available only if nations regularly report catches to the FAO or produce fisheries research publications containing catch data. Even if catches are reported, underreporting and underestimation are pervasive issues (Pauly and Zeller, 2016) that create a need for catch reconstruction, which varies methodologically across EEZs and sectors. Data on recreational and subsistence catches are often least available and most prone to biased estimation. Even so, rather than treating poorly quantified catches as “zeroes,” it is important to account for them using the best available data and robust estimation procedures, as in the Sea Around Us, which estimates catches using data from an average of 35 publications per reconstruction (Pauly and Zeller, 2016).
Social-ecological fisheries research is increasingly abundant. For instance, researchers have used social-ecological syndromes to understand local-global fisheries interactions (Crona et al., 2015), linked overfishing to marine regime shifts (Österblom and Folke, 2015), and explored how global seafood trade weakens ecosystem signals (Crona et al., 2016). How does the metacoupling framework—particularly metacoupling models like those developed herein—complement and advance previous research? First, whereas most multiscalar fisheries research approaches are social or ecological, and most social-ecological approaches are mono-scalar (Hunt et al., 2013), the metacoupling framework is both social-ecological and multiscalar. Second, the metacoupling framework is a systematic tool for understanding and synthesizing systems, flows, agents, causes, and effects, which are often studied in isolation (Liu, 2017). Third, the metacoupling framework promotes quantitative and qualitative assessments of human-nature interactions, feedbacks, and tradeoffs that both amplify and constrain fisheries ecosystem services, including food, employment, recreation, and culture (Roos et al., 2003; Bennett et al., 2018). Indeed, not all metacouplings are the same—a point clarified by metacoupling models and one that should be actively considered in fisheries policy and management. Finally, the metacoupling framework and models are widely applicable to social-ecological systems within and beyond fisheries, helping decision-makers translate science into policy and management approaches (Schaffer-Smith et al., 2018; Carlson et al., 2019). For example, results from the present study could be used to design decision-support tools to assist fisheries professionals in developing locally and regionally appropriate management and governance approaches that optimize fisheries’ contributions to food supply, employment, and biodiversity conservation.
Overall, the metacoupling framework provides a methodology for quantitatively understanding and predicting multiscalar human-nature interactions in fisheries. Next steps toward actionable metacoupling science include investigating largely unexplored areas such as how metacouplings influence national/international economies as well as food/nutrition security and livelihoods in developing-country contexts, and translating research to real-world fisheries management situations. The metacoupling framework helps bridge science and policy through the concept of metacoupling-informed governance—holistic management of Types 1, 2, and 3 relationships within and among social-ecological systems rather than specific places, issues, etc. (Liu, 2017; Carlson et al., 2018a). The goal of metacoupling-informed governance is social-ecological synthesis across scales, policies, and governance institutions; despite its local, on-the-ground applications (e.g., habitat improvement in one locale, stock enhancement in another), metacoupling-informed governance is social-ecological and multiscalar, distinguishing it from other governance approaches. An essential step in metacoupling-informed governance is identifying and evaluating local, adjacent, and distant social-ecological linkages using the metacoupling framework, as demonstrated herein. Then, metacoupling-informed governance seeks to optimize human-nature interactions by identifying leverage points—places in a system’s structure where small shifts in one social-ecological factor produce large across-system changes—to maximize desirable and minimize undesirable metacouplings, to the extent possible (Liu, 2017; Carlson et al., 2018a). For example, metacoupling-informed cod governance involves designing policies and management strategies that sustain subsistence catches amid competing fishing types (e.g., Type 2/3 industrial) while leveraging desirable interactions such as mutualism between artisanal and Type 1 industrial fishing (Figure 6C). Until recently, these tasks were only achievable in concept through qualitative metacoupling research. Qualitative approaches are valuable for initially investigating metacouplings, but advancements in metacoupling-informed network analysis (Schaffer-Smith et al., 2018) and time series modeling (Herzberger et al., 2019) have helped quantitatively operationalize the metacoupling framework. Our study expands the scope of quantitative metacoupling research to include marine fisheries, thereby broadening and deepening the evidence-based foundation for metacoupling-informed governance of social-ecological systems that previous studies have called for (Hulina et al., 2017; Liu, 2017; Carlson et al., 2018a, 2019).
In conclusion, the metacoupling framework and models are important tools for understanding how multiscalar human-nature interactions influence fisheries throughout the world. Future research directions are numerous, given metacoupling’s infancy and wide applicability across aquatic and terrestrial social-ecological systems (Liu, 2017). In fisheries, metacoupling models can be expanded to include other species (e.g., yellowfin tuna [Thunnus albacares], bigeye tuna [Thunnus obesus]) and broader aspects of marine fishing, including those relevant for human health and well-being such as end use (direct human consumption, fishmeal/fish oil, etc.) and food, nutrition, and livelihood security in developing-country contexts. Trade is another critical metacoupling research area, given that 35% of global fish production is traded internationally at a value of US$143 billion (Food and Agriculture Organization [FAO], 2018). In addition, social-ecological modeling approaches such as network analysis and agent-based modeling (Schaffer-Smith et al., 2018; Dou et al., 2019) promise to deepen understanding and management of fisheries metacouplings. Finally, the metacoupling framework is valuable as a public outreach tool for connecting people and ecosystems at local, regional, and global scales (Carlson et al., 2019). After all, individuals who understand metacouplings that ultimately put fish on their plates are more likely to make sustainable dietary choices than people without metacoupling knowledge. In such a society, “metacoupling” would acquire new meaning, connecting people across the planet in common pursuit of sustainability.
The datasets analyzed in this study can be found at the Sea Around Us (http://www.seaaroundus.org/) and are available on request to the corresponding author.
AC, DR, and SL conceived the research idea. AC obtained and analyzed the data and drafted the manuscript. DR and SL provided text in addition to comments and revisions. All the authors contributed to the writing and editing of the manuscript and approved its submission.
Funding in support of this research was provided by Princeton University’s Dean for Research, Princeton Environmental Institute, Andlinger Center for Energy and the Environment, and the Office of the Provost.
The authors declare that the research was conducted in the absence of any commercial or financial relationships that could be construed as a potential conflict of interest.
We thank associate editor AT and two peer reviewers for constructive comments which helped improve the initial version of this manuscript. We thank members of the Sea Around Us, particularly D. Pauly, D. Zeller, and M. L. D. Palomares, for creating and maintaining an exceptional resource for fisheries science. We thank J. Liu and W. Taylor (Michigan State University) for insights regarding the metacoupling framework and fisheries systems throughout the world. We also thank members of the Rubenstein and Levin labs at Princeton University (especially M. Andrews, J. Bak-Coleman, A. Gersick, S. Hex, J. Kariithi, Y. Li, and E. Krueger) for constructive feedback on initial work leading to this manuscript.
The Supplementary Material for this article can be found online at: https://www.frontiersin.org/articles/10.3389/fmars.2020.00614/full#supplementary-material
Agnew, D. J., Pearce, J., Pramod, G., Peatman, T., Watson, R., Beddington, J. R., et al. (2009). Estimating the worldwide extent of illegal fishing. PLoS One 4:e4570. doi: 10.1371/journal.pone.0004570
Aranda, M., Murua, H., and de Bruyn, P. (2012). Managing fishing capacity in tuna regional fisheries management organisations (RFMOs): development and state of the art. Mar. Policy 36, 985–992. doi: 10.1016/j.marpol.2012.01.006
Arlinghaus, R., and Cowx, I. (2008). “Meaning and relevance of the ecosystem approach to recreational fisheries management: emphasis on the importance of the human dimension,” in Global Challenges in Recreational Fisheries, eds R. Arlinghaus, R. B. Ditton, D. Policansky, and H. L. Schramm Jr. (Oxford: blackwell Publishing), 56–74. doi: 10.1002/9780470697597.ch3
Begossi, A. (2006). Temporal stability in fishing spots: conservation and co-management in Brazilian artisanal coastal fisheries. Ecol. Soc. 11:5. doi: 10.5751/ES-09923-230135
Belhabib, D., Doumbouya, A., Diallo, I., Traore, S., Camara, Y., Copeland, D., et al. (2013a). “Guinean fisheries, past, present and. future?,” in Marine Fisheries Catches in West Africa, 1950–2010, Part I, eds D. Belhabib, D. Zeller, S. Harper, and D. Pauly (Vancouver, BC: The University of British Columbia Fisheries Centre), 91–104.
Belhabib, D., Gascuel, D., Abou Kane, E., Harper, S., Zeller, D., and Pauly, D. (2012). “Preliminary estimation of realistic fisheries removals from Mauritania, 1950-2010,” in Marine Fisheries Catches in West Africa, 1950–2010, Part I, eds D. Belhabib, D. Zeller, S. Harper, and D. Pauly (Vancouver, BC: The University of British Columbia Fisheries Centre), 61–78.
Belhabib, D., Harper, S., Zeller, D., and Pauly, D. (2013b). “Reconstruction of marine fisheries catches from Morocco (north, central and south), 1950–2010,” in Marine Fisheries Catches in West Africa, Part I, eds D. Belhabib, D. Zeller, S. Harper, and D. Pauly (Vancouver, BC: The University of British Columbia Fisheries Centre), 23–40.
Belhabib, D., Kutoub, V., Gueye, N., Mbaye, L., Mathews, C., Lam, V., et al. (2013c). Lots of boats And fewer fishes: catch reconstruction for Senegal, 1950–2010. Paper Presented at the Working Paper Series 2013-03 (Vancouver, BC: The University of British Columbia Fisheries Centre).
Belhabib, D., Kutoub, V., and Pauly, D. (2015). “The marine fisheries of Togo, the ‘heart of West Africa,’ 1950 to 2010,” in Fisheries Catch Reconstructions: West Africa, Part II, eds D. Belhabib and D. Pauly (Vancouver, BC: The University of British Columbia Fisheries Centre), 37–50.
Belhabib, D., Kutoub, V., Sall, A., Lam, V. W. Y., and Pauly, D. (2014). Fisheries catch misreporting and its implications: the case of Senegal. Fish. Res. 151, 1–11. doi: 10.1016/j.fishres.2013.12.006
Belhabib, D., Mendy, A., Zeller, D., and Pauly, D. (2013d). Big fishing for small fishes: six decades of fisheries in The Gambia, ‘The Smiling Coast of Africa. Paper Presented at the Working Paper Series 2013-07 (Vancouver, BC: The University of British Columbia Fisheries Centre).
Belhabib, D., and Pauly, D. (2015a). “Benin’s fisheries: a catch reconstruction, 1950 to 2010,” in Fisheries Catch Reconstructions: West Africa, Part II, eds D. Belhabib and D. Pauly (Vancouver, BC: The University of British Columbia Fisheries Centre), 51–64.
Belhabib, D., and Pauly, D. (2015b). “Côte d’Ivoire: fisheries catch reconstruction, 1950–2010,” in Fisheries Catch Reconstructions: West Africa, Part II, eds D. Belhabib and D. Pauly (Vancouver, BC: The University of British Columbia Fisheries Centre), 17–36.
Belhabib, D., and Pauly, D. (2015c). “Fisheries in troubled waters: a catch reconstruction for Guinea-Bissau, 1950–2010,” in Fisheries Catch Reconstructions: West Africa, Part II, eds D. Belhabib and D. Pauly (Vancouver, BC: The University of British Columbia Fisheries Centre), 1–16.
Belhabib, D., Subah, Y., Broh, N. T., Jueseah, A. S., Nipey, J. N., Boeh, W. Y., et al. (2013e). When ‘reality leaves a lot to the imagination’: Liberian fisheries from 1950 to 2010. Paper Presented at the Working Paper Series 2013-06 (Vancouver, BC: The University of British Columbia Fisheries Centre).
Béné, C., Barange, M., Subasinghe, R., Pinstrup-Andersen, P., Merino, G., Hemre, G., et al. (2015). Feeding 9 billion by 2050—Putting fish back on the menu. Food Secur. 7, 261–274. doi: 10.1007/s12571-015-0427-z
Bennett, A., Patil, P., Kleisner, K., Rader, D., Virdin, J., and Basurto, X. (2018). Contribution of Fisheries to Food and Nutrition Security: Current Knowledge, Policy, and Research. Durham: Duke University, Nicholas Institute for Environmental Policy.
Bjerknes, J. (1969). Atmospheric teleconnections from the equatorial Pacific. Mon. Weather Rev. 97, 163–172. doi: 10.1175/1520-0493(1969)097<0163:atftep>2.3.co;2
Burnham, K. P., and Anderson, D. R. (2002). Model Selection and Multimodel Inference: A Practical Information-Theoretic Approach, 2nd Edn. New York, NY: Springer.
Cardinale, B. J., Duffy, J. E., Gonzalez, A., Hooper, D. U., Perrings, C., Venail, P., et al. (2012). Biodiversity loss and its impact on humanity. Nature 486, 59–67. doi: 10.1038/nature11148
Carlson, A. K., Taylor, W. W., and Liu, J. (2019). Using the telecoupling framework to improve Great Lakes fisheries sustainability. Aquat. Ecosyst. Health 22, 342–354. doi: 10.1080/14634988.2019.1668660
Carlson, A. K., Taylor, W. W., Liu, J., and Orlic, I. (2017). The telecoupling framework: an integrative tool for enhancing fisheries management. Fisheries 42, 395–397. doi: 10.1080/03632415.2017.1342491
Carlson, A. K., Taylor, W. W., Liu, J., and Orlic, I. (2018a). Peruvian anchoveta as a telecoupled fisheries system. Ecol. Soc. 23:35.
Carlson, A. K., Zaehringer, J. G., Garrett, R. D., Silva, R. F. B., Furumo, P. R., Raya Rey, A. N., et al. (2018b). Toward rigorous telecoupling causal attribution: a systematic review and typology. Sustainability 10:4426. doi: 10.3390/su10124426
Cohen, D. M., Inada, T., Iwamoto, T., and Scialabba, N. (1990). FAO Species Catalogue, Volume 10, Gadiform fishes of the world (Order Gadiformes): An Annotated and Illustrated Catalogue of Cods, Hakes, Grenadiers and Other Gadiform Fishes Known to Date. Rome: Food and Agriculture Organization of the United Nations.
Coll, M., Carreras, M., Cornax, M.-J., Massutí, E., Morote, E., Pastor, X., et al. (2015). An estimate of the total catch in the Spanish Mediterranean Sea and Gulf of Cadiz regions (1950–2010). Paper Presented at the Working Paper Series 2015-60 (Vancouver, BC: The University of British Columbia Fisheries Centre).
Collette, B., Acero, A., Amorim, A. F., Boustany, A., Canales Ramirez, C., Cardenas, G., et al. (2015). Common Dolphinfish (Coryphaena hippurus): The IUCN Red List of Threatened Species. Available at: https://www.iucnredlist.org/species/154712/18194682 (accessed June 25, 2020).
Collette, B., Amorim, A. F., Boustany, A., Carpenter, K. E., de Oliveira Leite, N. Jr., Di Natale, A., et al. (2011). Atlantic Bluefin Tuna (Thunnus thynnus): The IUCN Red List of Threatened Species. Available at: https://www.iucnredlist.org/species/21860/9331546 (accessed June 25, 2020).
Collette, B. B. (1999). “Mackerels, molecules, and morphology,” in Proceedings of the 5th Indo-Pacific Fish Conference, Noumea - New Caledonia, eds B. Seret and J. Y. Sire (Paris: Societe Francaise d’ Ichtyologie), 149–164.
Cooke, S. J., and Cowx, I. G. (2004). The role of recreational fishing in global fish crises. Bioscience 54, 857–859.
Crona, B. I., Daw, T. M., Swartz, W., Norström, A. V., Nyström, M., Thyresson, M., et al. (2016). Masked, diluted and drowned out: how global seafood trade weakens signals from marine ecosystems. Fish. Fish. 17, 1175–1182. doi: 10.1111/faf.12109
Crona, B. I., Van Holt, T., Petersson, M., Daw, T. M., and Buchary, E. (2015). Using social-ecological syndromes to understand impacts of international seafood trade on small-scale fisheries. Global Environ. Chang. 35, 162–175. doi: 10.1016/j.gloenvcha.2015.07.006
Divovich, E., Belhabib, D., Zeller, D., and Pauly, D. (2015). Eastern Canada, ‘a fishery with no clean hands’: marine fisheries catch reconstruction from 1950 to 2010. Paper Presented at the Working Paper Series 2015-56 (Vancouver, BC: The University of British Columbia Fisheries Centre).
dos Santos, J., Burklow, I. C., and Jobling, M. (1993). Patterns of growth and lipid deposition in cod (Gadus morhua L.) fed natural prey and fish-based feeds. Aquaculture 110, 173–189. doi: 10.1016/0044-8486(93)90271-Y
Dou, Y., Millington, J. D. A., Silva, R. F. B., McCord, P., Viña, A., Song, Q., et al. (2019). Land-use changes across distant places: design of a telecoupled agent-based model. J. Land Use Sci. 14, 191–209. doi: 10.1080/1747423X.2019.1687769
Dreher, A., Gaston, N., and Martens, P. (2008). Measuring Globalisation: Gauging its Consequences. New York: Springer.
Eriksson, H., Österblom, H., Crona, B., Troell, M., Andrew, N., Wilen, J., et al. (2015). Contagious exploitation of marine resources. Front. Ecol. Environ. 13, 435–440. doi: 10.1890/140312
Etim, L., Belhabib, D., and Pauly, D. (2015). “An overview of the Nigerian marine fisheries and a re-evaluation of its catch data for the years 1950–2010,” in Fisheries Catch Reconstructions: West Africa, Part II, eds D. Belhabib and D. Pauly (Vancouver, BC: The University of British Columbia Fisheries Centre), 66–76.
Finlayson, A. C., and McCay, B. J. (2000). “Crossing the threshold of ecosystem resilience: the commercial extinction of northern cod,” in Linking Social and Ecological Systems: Management Practices and Social Mechanisms for Building Resilience, eds F. Berkes and C. Folke (Cambridge: Cambridge University Press), 311–337.
Food and Agriculture Organization [FAO], (2018). The State of World Fisheries and Aquaculture 2018. Rome: FAO.
Freire, K. M. F., Aragão, J. A. N., Araújo, A. R. R., Ávila-da-Silva, A. O., Bispo, M. C. S., Canziani, G. V., et al. (2014). Revisiting Brazilian catch data for Brazilian marine waters (1950–2010). Paper Presented at the Working Paper Series 2014-23 (Vancouver, BC: The University of British Columbia Fisheries Centre).
Fromentin, J.-M., and Powers, J. E. (2005). Atlantic bluefin tuna: population dynamics, ecology, fisheries and management. Fish. Fish. 6, 281–306. doi: 10.1111/j.1467-2979.2005.00197.x
Funes, M., Zylich, K., Divovich, E., Zeller, D., Lindop, A., Pauly, D., et al. (2015). Honduras, a fish exporting country: preliminary reconstructed marine catches in the Caribbean Sea and the Gulf of Fonseca, 1950–2010. Paper Presented at the Working Paper Series 2015-90 (Vancouver, BC: The University of British Columbia Fisheries Centre).
Godfray, H. C. J., Beddington, J. R., Crute, I. R., Haddad, L., Lawrence, D., Muir, J. F., et al. (2010). Food security: the challenge of feeding 9 billion people. Science 327, 812–818. doi: 10.1126/science.1185383
Gordon, D. T., and Roberts, G. L. (1977). Mineral and proximate composition of Pacific Coast fish. J. Agric. Food Chem. 25, 1262–1268. doi: 10.1021/jf60214a039
Gwak, W. S., and Nakayama, K. (2011). Genetic variation and population structure of the Pacific cod Gadus macrocephalus in Korean waters revealed by mtDNA and msDNA markers. Fish. Sci. 77, 945–952. doi: 10.1007/s12562-011-0403-2
Harper, S., Bothwell, J., Bale, S., Booth, S., and Zeller, D. (2009). “Cayman Islands fisheries catches: 1950–2007,” in Fisheries Catch Reconstructions: Islands, Part I, eds D. Zeller and S. Harper (Vancouver, BC: The University of British Columbia Fisheries Centre), 3–11.
Herzberger, A., Chung, M. G., Kapsar, K., Frank, K. A., and Liu, J. (2019). Telecoupled food trade affects pericoupled trade and intracoupled production. Sustainability 11:2908. doi: 10.3390/su11102908
Hulina, J., Bocetti, C., Iii, H., Hull, V., Yang, W., and Liu, J. (2017). Telecoupling framework for research on migratory species in the Anthropocene. Elementa Sci. Anthrop. 5:5. doi: 10.1525/elementa.184
Hunt, L. M., Sutton, S. G., and Arlinghaus, R. (2013). Illustrating the critical role of human dimensions research for understanding and managing recreational fisheries within a social-ecological system framework. Fish. Manag. Ecol. 20, 111–124. doi: 10.1111/j.1365-2400.2012.00870.x
Hyndman, R. J., and Athanasopoulos, G. (2018). Forecasting: Principles and Practice, 2nd Edn. Melbourne: OTexts.
International Union for Conservation of Nature [IUCN], (2019). Cod: The IUCN Red List of Threatened Species 2011. Available at: https://www.iucnredlist.org/search?query=cod&searchType=species (accessed June 25, 2020).
Kavasseri, R. G., and Seetharaman, K. (2009). Day-ahead wind speed forecasting using f-ARIMA models. Renew. Energ. 34, 1388–1393. doi: 10.1016/j.renene.2008.09.006
Khalfallah, M., Dimech, M., Ulman, A., Zeller, D., and Pauly, D. (2015). Reconstruction of marine fisheries catches for the Republic of Malta (1950–2010). Paper Presented at the Working Paper Series 2015-43 (Vancouver, BC: The University of British Columbia Fisheries Centre).
Kroodsma, D. A., Mayorga, J., Hochberg, T., Miller, N. A., Boerder, K., Ferreti, F., et al. (2018). Tracking the global footprint of fisheries. Science 359, 904–908. doi: 10.1126/science.aao5646
Krumme, U., Wang, T. C., and Wang, D. R. (2013). From food to feed: assessment of the stationary lift net fishery of East Hainan, Northern South China Sea. Cont. Shelf Res. 57, 105–116. doi: 10.1016/j.csr.2012.04.011
Lindop, A., Ixquiac-Cabrera, M., Zylich, K., and Zeller, D. (2015). A reconstruction of marine fish catches in the Republic of Guatemala. Paper Presented at the Working Paper Series 2015-41 (Vancouver, BC: The University of British Columbia Fisheries Centre).
Liu, J. (2017). Integration across a metacoupled world. Ecol. Soc. 22:29. doi: 10.5751/ES-09830-220429
Liu, J., Hull, V., Batistella, M., DeFries, R., Dietz, T., Fu, F., et al. (2013). Framing sustainability in a telecoupled world. Ecol. Soc. 18:26. doi: 10.5751/ES-05873-180226
Makino, M. (2013). Fisheries Management in Japan: Its Institutional Features and Case Studies. Dordrecht: Springer.
Mekonnen, M. M., and Hoekstra, A. Y. (2016). Four billion people facing severe water scarcity. Sci. Adv. 2:e1500323. doi: 10.1126/sciadv.1500323
Mendo, J., and Wosnitza-Mendo, C. (2014). Reconstruction of total marine fisheries catches for Peru: 1950–2010. Paper Presented at the Working Paper Series 2014-21 (Vancouver, BC: The University of British Columbia Fisheries Centre).
National Oceanic, and Atmospheric Administration [NOAA], (2017). 2017 Report to Congress on the Status of U.S. Fisheries. Silver Spring: NOAA.
Neat, F. C., Bendall, V., Berx, B., Wright, P. J., Cuaig, M. Ó, Townhill, B., et al. (2014). Movement of Atlantic cod around the British Isles: implications for finer scale stock management. J. Appl. Ecol. 51, 1564–1574. doi: 10.1111/1365-2664.12343
Nunoo, F., Asiedu, B., Amador, K., Belhabib, D., and Pauly, D. (2015). Reconstruction of marine fisheries catches in Ghana, 1950–2010. Paper Presented at the Working Paper Series 2014-13 (Vancouver, BC: The University of British Columbia Fisheries Centre).
Österblom, H., and Folke, C. (2015). Globalization, marine regime shifts and the Soviet Union. Philos. T. Roy. Soc. B 370:20130278. doi: 10.1098/rstb.2013.0278
Palko, B. J., Beardsley, G. L., and Richards, W. J. (1982). Synopsis of the Biological Data on Dolphin-Fishes, Coryphaena hippurus Linnaeus and Coryphaena equiselis Linnaeus. Silver Spring: National Oceanic and Atmospheric Administration.
Pauly, D. (2006). Major trends in small-scale marine fisheries, with emphasis on developing countries, and some implications for the social sciences. Marit. Stud. MAST 4, 7–22.
Pauly, D., and Le Manach, F. (2015). Tentative adjustments of China’s marine fisheries catches (1950–2010). Paper Presented at the Working Paper Series 2015-28 (Vancouver, BC: The University of British Columbia Fisheries Centre).
Pauly, D., and Zeller, D. (2016). Catch reconstructions reveal that global marine fisheries catches are higher than reported and declining. Nat. Commun. 7:10244. doi: 10.1038/ncomms10244
Pauly, D., Zeller, D., and Palomares, M. L. D. (2020). Sea Around Us Concepts, Design and Data. Available at: http://www.seaaroundus.org/ (accessed June 25, 2020).
Piroddi, C., Gristina, M., Zylich, K., Ulman, A., Zeller, D., and Pauly, D. (2014). Reconstruction of Italy’s marine fisheries catches (1950–2010). Paper Presented at the Working Paper Series 2014-22 (Vancouver, BC: The University of British Columbia Fisheries Centre).
Ramdeen, R., Harper, S., and Zeller, D. (2014). “Reconstruction of total marine fisheries catches for Dominica (1950–2010),” in Fisheries Catch Reconstructions: Islands, Part IV, eds K. Zylich, D. Zeller, M. Ang, and D. Pauly (Vancouver, BC: The University of British Columbia Fisheries Centre), 33–42.
Roos, N., Islam, M. M., and Thilsted, S. H. (2003). Small indigenous fish species in Bangladesh: contribution to vitamin A, calcium and iron intakes. J. Nutr. 133, 4021S–4026S. doi: 10.1093/jn/133.11.4021S
RStudio, (2019). RStudio. Available at: http://www.rstudio.com/. (accessed June 25, 2020).
Schaffer-Smith, D., Tomscha, S. A., Jarvis, K. J., Maguire, D. Y., Treglia, M. L., and Liu, J. (2018). Network analysis as a tool for quantifying the dynamics of metacoupled systems: an example using global soybean trade. Ecol. Soc. 23:3. doi: 10.5751/ES-10460-230403
Seto, K., Belhabib, D., Copeland, D., Vakily, M., Seilert, H., Sankoh, S., et al. (2015). Colonialism, conflict, and fish: a reconstruction of marine fisheries catches for Sierra Leone, 1950–2010. Paper Presented at the Working Paper Series 2015-74 (Vancouver, BC: The University of British Columbia Fisheries Centre).
Silva, R. F. B., Batistella, M., Dou, Y., Moran, E., Torres, S. M., and Liu, J. (2017). The Sino-Brazilian telecoupled soybean system and cascading effects for the exporting country. Land 6, 1–19. doi: 10.3390/land6030053/
Steffen, W., Richardson, K., Rockström, J., Cornell, S. E., Fetzer, I., Bennett, E. M., et al. (2015). Planetary boundaries: guiding human development on a changing planet. Science 347:1259855. doi: 10.1126/science.1259855
Sumaila, U. R., Alder, J., and Keith, H. (2006). Global scope and economics of illegal fishing. Mar. Policy 30, 696–703. doi: 10.1016/j.marpol.2005.11.001
Sun, J., Tong, Y., and Liu, J. (2017). Telecoupled land-use changes in distant countries. J. Integr. Agric. 16, 368–376. doi: 10.1016/S2095-3119(16)61528-9
Tapia-Lewin, S., Vergara, K., De La Barra, C., Godoy, N., Castilla, J. C., and Gelcich, S. (2017). Distal impacts of aquarium trade: exploring the emerging sandhopper (Orchestoidea tuberculata) artisanal shore gathering fishery in Chile. Ambio 46, 706–716. doi: 10.1007/s13280-017-0906-x
Trindade Santos, I., Monteiro, C. A., Harper, S., Zylich, K., Zeller, D., and Belhabib, D. (2013). “Reconstruction of marine fisheries catches for the Republic of Cape Verde, 1950-2010,” in Marine Fisheries Catches in West Africa, 1950–2010, Part I, eds D. Belhabib, D. Zeller, S. Harper, and D. Pauly (Vancouver, BC: The University of British Columbia Fisheries Centre), 79–90.
Tsitsika, E. V., Maravelias, C. D., and Haralabous, J. (2007). Modeling and forecasting pelagic fish production using univariate and multivariate ARIMA models. Fish. Sci. 73, 979–988. doi: 10.1111/j.1444-2906.2007.01426.x
United Nations Statistics Division [UNSD], (2020). Methodology: Standard Country or Area Codes for Statistical Use (M49). Available at: https://unstats.un.org/unsd/methodology/m49/ (Accessed June 25, 2020).
Walther, G.-R., Post, E., Convey, P., Menzel, A., Parmesan, C., Beebee, T. J. C., et al. (2002). Ecological responses to recent climate change. Nature 416, 389–395. doi: 10.1038/416389a
Whoriskey, S., Arauz, R., and Baum, J. K. (2011). Potential impacts of emerging mahi-mahi fisheries on sea turtle and elasmobranch bycatch species. Biol. Conserv. 144, 1841–1849. doi: 10.1016/j.biocon.2011.03.021
Williams, B. M., and Hoel, L. A. (2003). Modeling and forecasting vehicular traffic flow as a seasonal ARIMA process: theoretical basis and empirical results. J. Transp. Eng. 129, 664–672. doi: 10.1061/(asce)0733-947x(2003)129:6(664)
Keywords: fisheries, metacoupling, metacoupling framework, social-ecological systems, sustainability, mahi-mahi, Atlantic bluefin tuna, cods
Citation: Carlson AK, Rubenstein DI and Levin SA (2020) Linking Multiscalar Fisheries Using Metacoupling Models. Front. Mar. Sci. 7:614. doi: 10.3389/fmars.2020.00614
Received: 05 March 2020; Accepted: 06 July 2020;
Published: 28 July 2020.
Edited by:
Athanassios C. Tsikliras, Aristotle University of Thessaloniki, GreeceReviewed by:
Dirk Zeller, The University of Western Australia, AustraliaCopyright © 2020 Carlson, Rubenstein and Levin. This is an open-access article distributed under the terms of the Creative Commons Attribution License (CC BY). The use, distribution or reproduction in other forums is permitted, provided the original author(s) and the copyright owner(s) are credited and that the original publication in this journal is cited, in accordance with accepted academic practice. No use, distribution or reproduction is permitted which does not comply with these terms.
*Correspondence: Andrew K. Carlson, YW5kcmV3a2NAcHJpbmNldG9uLmVkdQ==; YW5kcmV3Y2FybHNvbjQyMkBnbWFpbC5jb20=
Disclaimer: All claims expressed in this article are solely those of the authors and do not necessarily represent those of their affiliated organizations, or those of the publisher, the editors and the reviewers. Any product that may be evaluated in this article or claim that may be made by its manufacturer is not guaranteed or endorsed by the publisher.
Research integrity at Frontiers
Learn more about the work of our research integrity team to safeguard the quality of each article we publish.