- 1School of Environmental Sciences, University of Liverpool, Liverpool, United Kingdom
- 2Laboratoire d'Océanographie et du Climat: Expérimentations et Approches Numériques, IRD/CNRS/MNHN/SU-IPSL, Paris, France
Zooplankton occupy a key place in the ocean ecosystems as they constitute a link between primary producers and upper trophic levels, with many commercially important fisheries relying on the presence of zooplankton to sustain fish stocks. Moreover, zooplankton have an important role in supporting primary production as they can recycle large amounts of micronutrients such as iron, facilitating its retention in the surface ocean and alleviating iron limitation of phytoplankton. Intuitively, one may consider that a large quantity of prey should ensure a healthy zooplankton ecosystem, but the microbial oceanic food web is characterized by a great variability in both the composition and quality of preys. This variability may lead to mismatches between predator and prey stoichiometry, which can in turn affect the growth efficiency of zooplankton. Here we show that variations in food quality are the main drivers of changes in iron assimilation and recycling by zooplankton. Making use of a state-of-the-art biogeochemical model that explicitly accounts for the impact of multiple drivers on the iron assimilation efficiency, we quantify the relative drivers of iron recycling in different ocean regions and across seasons. Our results can be reconciled within a conceptual framework that links the assimilation efficiency of zooplankton to predator-prey stoichiometric mismatch and zooplankton physiological assumptions. If predator and prey stoichiometries are close, then the micronutrient assimilation by zooplankton is optimal and recycling is low. Any departure from this optimal stoichiometry leads to a decrease in assimilation efficiency and a subsequent increase in micronutrient recycling. This framework can be used to understand the impact of variability in prey food quality on iron recycling from previous experiments and generates clear hypotheses about the relative importance of recycling for other micronutrients such as copper, cobalt, manganese, and zinc. Finally, our findings highlight the importance of future changes in prey food quality in driving recycling rates of micronutrients that can amplify or attenuate any climate driven trends in upper ocean nutrient supply.
1. Introduction
Zooplankton are keystone species in the ocean ecosystem (Steinberg and Landry, 2017), and constitute the largest animal biomass on Earth (Richardson, 2008). They form a wide group with several species that graze phytoplankton blooms (Calbet and Landry, 2004), and since zooplankton are themselves grazed upon by fish, they form a key link between the ocean microbial food web and the higher trophic levels (e.g., Jeppesen et al., 1990; Dalpadado et al., 2002). Many predatory zooplankton-fish relationships are species specific, meaning that fish (in particular their larvae) rely on a specific zooplankton species as preys (e.g., Norwegian cod Ghadus morua prey exclusively on Calanus finmarchicus; Beaugrand et al., 2003). Some bird species also depend on zooplankton, as illustrated by the example of Neocalanus being the major food source for Cassin's auklet chicks off the coasts of British Columbia (Bertram et al., 2001). Therefore, understanding the drivers of a healthy zooplankton population is of primary importance to the entire oceanic ecosystem.
From a biogeochemical perspective, zooplankton act as phytoplankton grazers, but they can also recycle nutritive elements such as iron very efficiently (Hutchins and Bruland, 1994; Barbeau et al., 2001; Boyd et al., 2012; Giering et al., 2012; Schmidt et al., 2016). This is important as net primary production is limited by iron over much of the ocean and in such areas, recycled iron is the major iron source for surface primary production for parts of the year (e.g., Hutchins et al., 1993; Boyd et al., 2015; Rafter et al., 2017; Tagliabue et al., 2017). However, the biotic and abiotic drivers of recycling are not well-characterized.
According to Sterner and Elser (2002), nutrient recycling by zooplankton is linked to their assimilation efficiency (AE), with a low assimilation efficiency leading to high recycling (also called excretion in some studies). The assimilation efficiency can itself be directly or indirectly influenced by a suite of biotic and abiotic factors such as zooplankton feeding physiology (Xu and Wang, 2001), prey composition (food quality, Schmidt et al., 1999), diet composition (Steinberg and Landry, 2017; Anderson and Harvey, 2019), and temperature (Richardson, 2008). Temperature affects both phytoplankton and zooplankton growth, with the zooplankton grazing rate (and therefore the amount of ingested food) increasing as a function of temperature (Buitenhuis et al., 2006). As seawater temperature may also influence particle remineralization (Laufkötter et al., 2017), it may indirectly impact assimilation efficiency via its influence on zooplankton grazing rate and on prey abundance and stoichiometry. These different factors have the potential to directly or indirectly affect iron assimilation efficiency and recycling by zooplankton. However, the relative role played by each of these potential drivers and the way they may influence iron assimilation efficiency is unknown. For instance, Montagnes and Fenton (2012) argue that carbon assimilation efficiency may decrease with increased prey abundance through the emergence of sloppy feeding and decreased gut passage time. Therefore, assimilation efficiency becomes lower when preys are more abundant (as also observed by Xu and Wang, 2001 for Cd, Se, and Zn). However, Wang et al. (1996) also show that the assimilation efficiency of different trace metals may be independent of food concentration, but varies with diet composition. The drivers influencing iron assimilation efficiency and recycling are described in Figure 1.
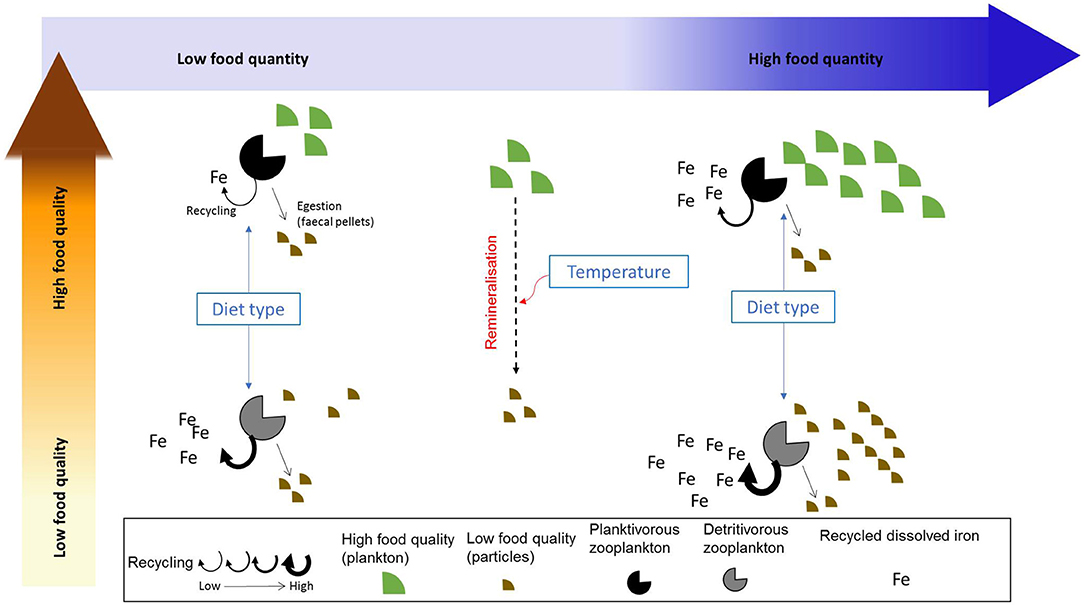
Figure 1. Summarized view of the factors influencing iron recycling by zooplankton. We emphasize the effects of food quality and quantity on iron recycling as well as the indirect influence of zooplankton diet type and temperature.
In this study, we use a modeling approach to explore the characteristics and drivers of iron assimilation and recycling over the global ocean. In particular, our aim is to test whether different drivers (food quantity, food quality, diet composition, defined as the proportion of detritivory in zooplankton diet, and seawater temperature) impact directly or indirectly zooplankton grazing and recycling and to identify which driver has the most influence on iron recycling in the surface ocean. Our results show the global spatial and seasonal variability of iron recycling, and its drivers. We also use a statistical approach to examine the potential for the different drivers to influence iron assimilation efficiency. Finally, we discuss the implications of our results for the study of trace metal biogeochemistry and microbial food webs in the present and future.
2. Characteristics of Iron Recycling
2.1. Model Description
We use the 3D global coupled physical-biogeochemical model NEMO/PISCES (Aumont et al., 2015). This model has been extensively used and evaluated to represent the global macro and micronutrient cycling at global and regional scales (see e.g., Kwiatkowski et al., 2018; Richon et al., 2018; Tagliabue et al., 2018), and to perform future climate projections (Laufkötter et al., 2015; Richon et al., 2019). The specifics of PISCES regarding iron cycling are a variable iron stoichiometry (Fe:C) in phytoplankton cells (between 0 and 80 μmolFe molC−1; e.g., Twining and Baines, 2013), which represents the observed variability in Fe uptake due to iron availability, iron limitation, and assumptions regarding iron allocation and storage (Aumont et al., 2015), and a fixed iron stoichiometry of zooplankton -(Fe:C)zoo- of 10 μmolFe molC−1. Microzooplankton is grazing preferentially on phytoplankton, and more specifically on nanophytoplankton. Mesozooplankton has more potential preys (nanophytoplankton, diatoms, particles, and microzooplankton) and is grazing preferentially on diatoms and microzooplankton. Trace metal recycling of iron by zooplankton is described as:
And iron assimilation efficiency (AEFe):
In Equation (1), (Fe:C)preys and (Fe:C)zoo represent respectively the iron quotas in preys (i.e., phytoplankton, small zooplankton, and particles), and in zooplankton predators. feedingeff represents zooplankton feeding efficiency, which is dependent on the ratio (Fe:C)preys/ (Fe:C)zoo. In this article, we define (Fe:C)zoo/ (Fe:C)preys as “food quality.” According to Mitra et al. (2007), feedingeff decreases with (Fe:C)preys when (Fe:C)preys < (Fe:C)zoo (i.e., food quality > 1). Unass represents the unassimilated fraction of the preys and is assumed constant at 0.3. Grazing by zooplankton (GrazingFe) is temperature dependant and calculated using a Q10 approach [grazing rate ∝exp(0.07608*T)] and proportional to prey biomass (see Aumont et al., 2015, for the complete equations). We refer to Aumont et al. (2018) for an evaluation of zooplankton distribution in PISCES. As a result of Equation (1), iron recycling increases with (Fe:C)prey.
2.2. Modeled Iron Recycling
Figure 2 shows the surface distribution (0–100 m) of dissolved iron (Figure 2A), the ratio of total zooplankton iron recycling to total phytoplankton iron uptake (Figure 2B), and total iron recycling by microzooplankton (Figure 2C), and mesozooplankton (Figure 2D) individually. The iron concentration modeled with PISCES has been previously evaluated and discussed at length by Tagliabue et al. (2016) and displays variability in the surface ocean that is typical of available observations (Figure 2A), with coastal regions and the North Atlantic being iron rich because of the proximity of external iron sources such as rivers, sediments and aerosol deposition. Overall, iron concentration is very low in open ocean regions (below 0.5 μmolFe m−3), except in the North Atlantic, which is broadly consistent with compiled GEOTRACES observations (Schlitzer et al., 2018).
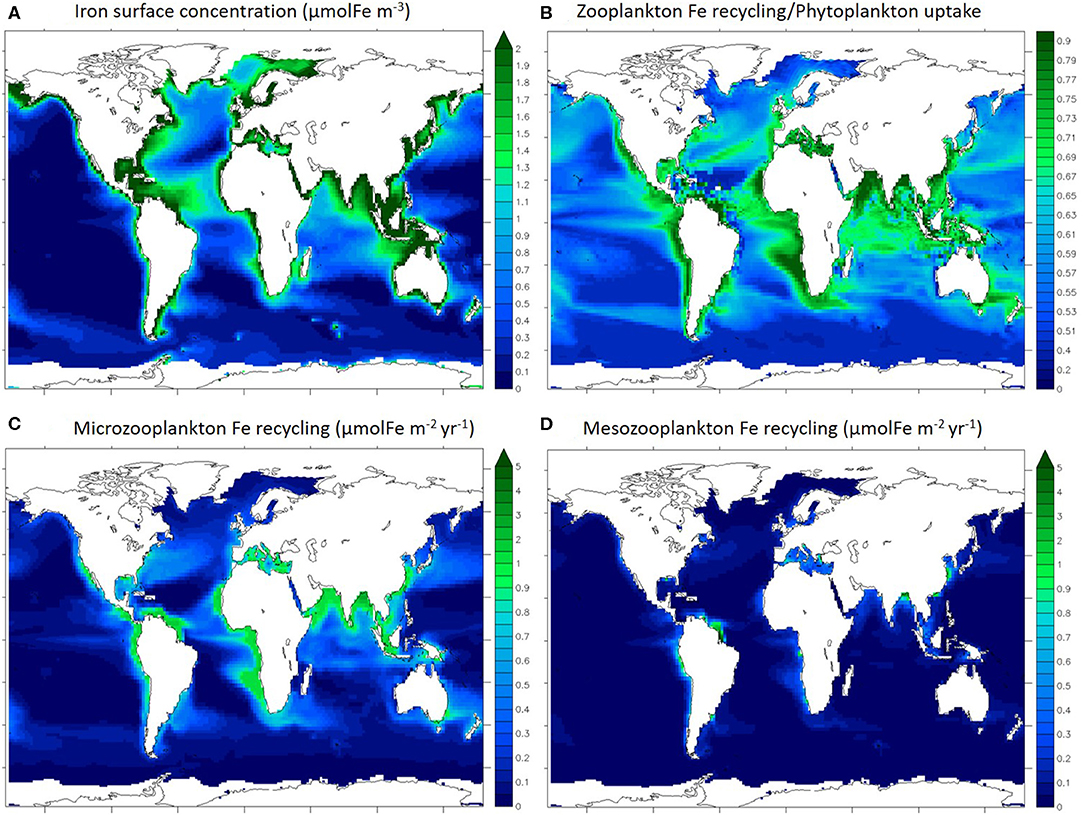
Figure 2. Maps of annually averaged iron concentration in the first 100 m (A), iron recycling/uptake ratio (B), annually averaged microzooplankton (C), and mesozooplankton (D) iron recycling integrated for the first 100 m. Ice covered regions (defined as grid points with an annually averaged ice cover > 50%) are masked out.
In most ocean regions, the iron recycled by zooplankton can be a significant contributor to the iron uptake by phytoplankton (Figure 2B). This is in agreement with studies quantifying the importance of upper ocean iron recycling in supporting primary production (Strzepek et al., 2005; Boyd et al., 2012). In particular, in the most productive regions such as the coastal and upwelling zones, zooplankton recycling/phytoplankton uptake ratio is over 0.70. Thus, zooplankton are able to extend the residence time of iron in the surface ocean via recycling and support phytoplankton iron nutrition. In particular in the Indian Ocean, the Equatorial Atlantic and the eastern Equatorial Pacific regions, the recycling/uptake ratio is high (over 0.65) despite low iron concentrations (Figure 2A), illustrating the phytoplankton reliance on recycled iron. It is important to note that other processes can enhance iron residence time in the surface ocean such as remineralization of particulate iron by bacteria (see Boyd et al., 2010).
Gross rates of iron recycling by micro and mesozooplankton are variable throughout the surface ocean and high gross rates of recycling occur in regions typified by high levels of zooplankton biomass and primary production, such as coastal areas and the equatorial Pacific and Atlantic (Figures 2C,D). According to our model, the integrated Fe recycling by microzooplankton is 1,030 GmolFe yr−1 (in the upper 100 m) and the integrated recycling by mesozooplankton is 240 GmolFe yr−1 (in the upper 100 m). As over 80% of upper 100 m iron recycling is performed by microzooplankton, we will focus the rest of this study on microzooplankton specifically.
2.3. Characterizing the Drivers of Iron Recycling
Iron assimilation efficiency (AE) as well as the major factors driving the recycling of iron are represented in Figure 3. Food quantity is defined as microzooplankton total grazing (Figure 3B). We define detritivory as feeding on particles, which in our model comprises dead phytoplankton and zooplankton, faecal pellets and unassimilated food items, and for iron also includes inorganic particles (scavenged and aggregated colloidal iron). The detritivory proportion in microzooplankton's diet (Figure 3C) is an indication of the diet type described in Figure 1.
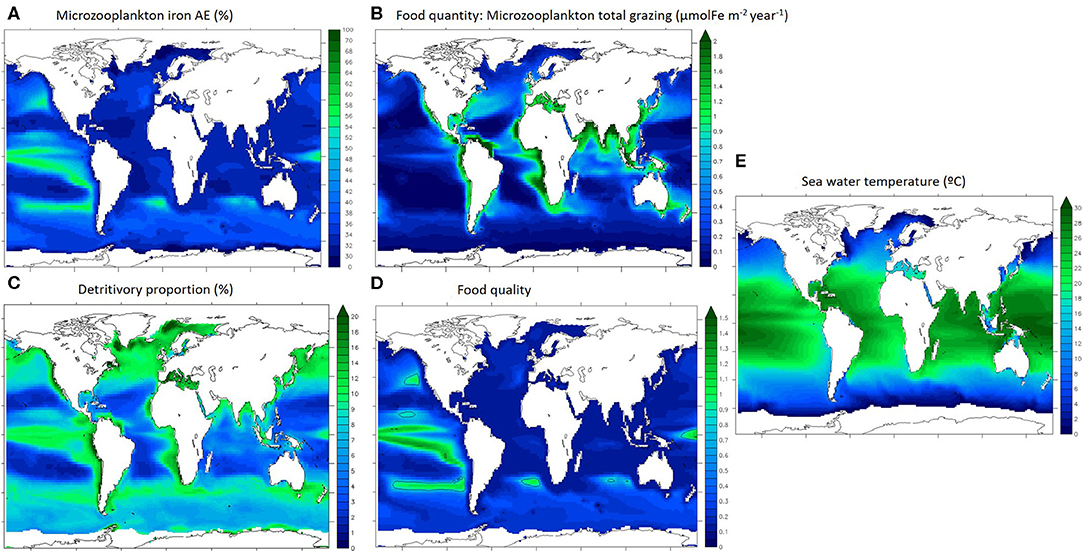
Figure 3. Drivers of microzooplankton recycling in the upper 100 m. (A) iron assimilation efficiency, (B) microzooplankton integrated grazing, (C) proportion of detrivtivory in microzooplankton diet, (D) food quality index, (E) sea water temperature. Ice covered regions (defined as grid points with an annually averaged ice cover > 50%) are masked out.
Annually averaged, the iron assimilation efficiency is highest in the Tropical Pacific and in the mid-latitude gyres (up to 60%) and is lowest in the subtropical oligotrophic gyres (around 30%, Figure 3A). Annually averaged food quantity (total grazing) is variable and maximal in the most productive oceanic regions (over 2 μmolFe m−2 year−1 in coastal seas and mid latitudes and upwelling zones, Figure 3B). Annually averaged detritivory proportion is between 3 and 20% as microzooplankton are mostly herbivorous and the spatial pattern is similar to the overall grazing distributions in the low and mid latitudes (Figures 3B,C). However, detritivory proportion is over 15% in the north Atlantic and Pacific oceans and between 10 and 15% in the Southern Ocean. The food quality index represents the stoichiometric mismatch between zooplankton and their preys (Figure 3D). Food quality is optimal when the index is close to 1, meaning that Fe:C ratios are almost identical for predators and for preys, indicating that zooplankton are feeding on stoichiometrically similar prey. If food quality is lower than 1, prey have a higher Fe stoichiometry than zooplankton. In general, prey stoichiometry is higher than zooplankton stoichiometry in most of the surface ocean (Figure 3D, food quality index <1), except in the tropical Pacific and some mid latitude regions. Overall, the food quality distribution is different than the food quantity distribution, and it appears similar to the iron assimilation efficiency distribution (Figures 3A,D). Temperature (Figure 3E) follows the well understood latitudinal pattern with the hottest temperatures (over 25°C) in the tropical regions and very cold temperatures in the polar seas (around 0°C).
3. Identifying the Driving Factors of Iron Assimilation Efficiency
3.1. Statistical Methods
In order to understand how the drivers we highlighted previously impact iron assimilation efficiency (AE), we performed a statistical analysis using the R package relaimpo (https://cran.r-project.org/web/packages/relaimpo/index.html), resolving the following generalized linear model (GLM):
From this analysis, we can calculate the proportion of the variance in iron assimilation efficiency explained by each factor of Equation (3) and by all of the factors combined, allowing us to examine how each factor impacts iron assimilation efficiency individually and in combination in our biogeochemical model results. An alternative way to perform this assessment would be to conduct a suite of sensitivity experiments with the biogeochemical model where each driving factor (food quality, quantity, etc.) were varied. But due to the central role of zooplankton in the ocean biogeochemical cycles, ad hoc modifications to the rates and extent of grazing would lead to widespread and unrealistic changes in our model. Therefore, we use this statistical framework to diagnose the relative roles played by different factors in the biogeochemical model results. Figure 4 shows the weighted contributions (Groemping, 2006) between iron assimilation efficiency and each driver calculated with our statistical analysis (α, β, γ, and δ from Equation 3).
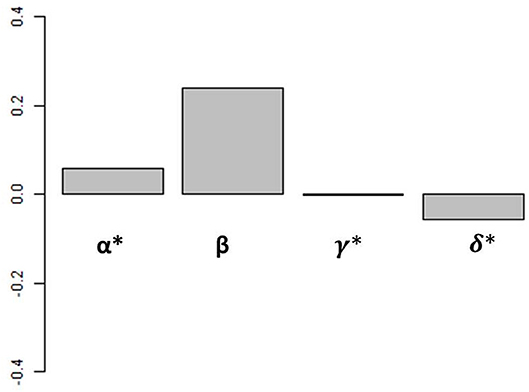
Figure 4. Weighted contribution of each driver to iron assimilation efficiency variance. Values are calculated over the global ocean. Values of α, γ, and δ have been multiplied by 100 for visualization.
Overall, the different drivers have varying influences on iron assimilation efficiency. The weighted contributions of food quantity (α), food quality (β), and % detritivory (γ) are positive, whereas the weighted contribution of temperature (δ) is negative. The effects of temperature and % detrivory on iron assimilation efficiency are indirect and can favor a higher iron assimilation efficiency (i.e., if temperature decreases, grazing decreases i.e., food quantity decreases) or a lower iron assimilation efficiency (i.e., if the proportion of detritivory increases, the food quality is likely to decrease as a result of the high Fe:C in organic and inorganic particles; see Ohnemus and Lam, 2015; Tagliabue et al., 2019). On the global scale, when all spatial and seasonal data is used, food quality is the strongest determinant of the global iron assimilation efficiency and has a weighted contribution 2 orders of magnitude higher than the 3 other factors (Figure 4). Overall, all factors included in Equation (3) are able to explain 84.8% of global surface ocean iron assimilation efficiency variance. Of these 84.8%, food quality explains 97.6%, food quantity 0.3%, diet composition 1.7%, and temperature 0.4%. However, the relationship between iron assimilation efficiency and the drivers may vary on the regional and seasonal scale because of their local variability in space and time.
3.2. Regional Variability of the Driving Factors of Iron Assimilation Efficiency
We repeated our analysis within a set of Longhurst provinces (Longhurst, 2007) and found that our statistical model explains most (over 80%) of iron assimilation efficiency variance in most oceanic regions (except in the Mediterranean and the Atlantic subpolar gyre, Figure 5A).
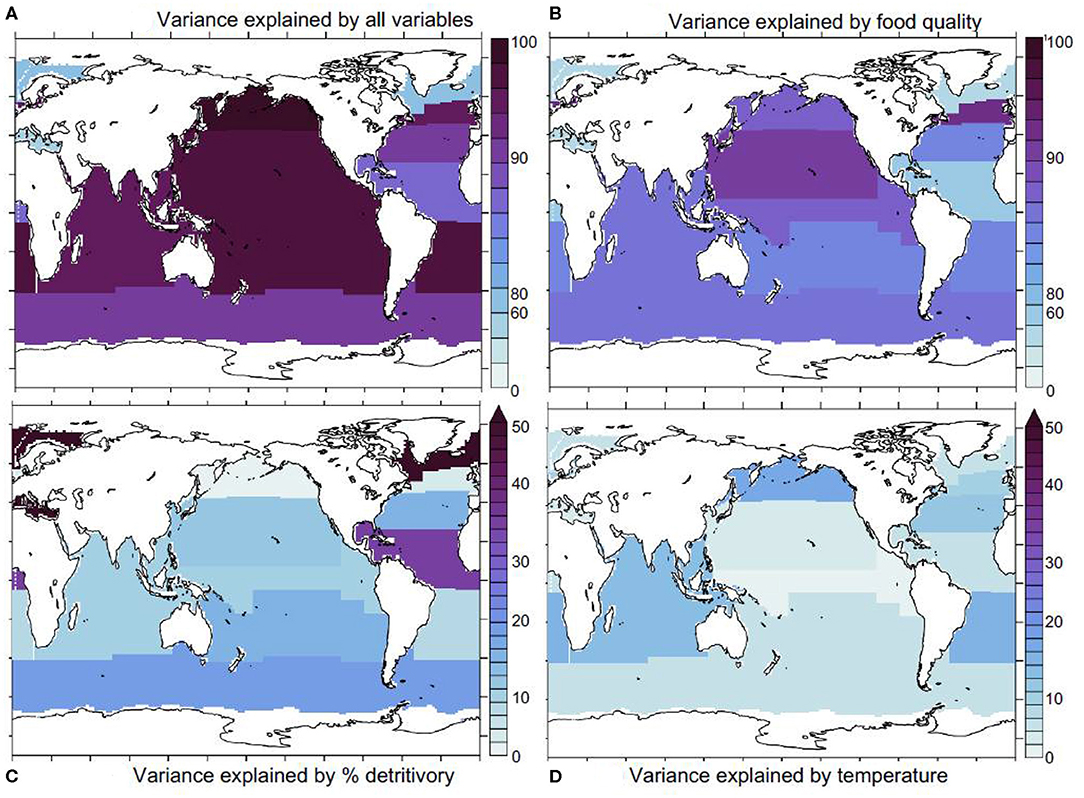
Figure 5. Statistical model results. (A) proportion of iron assimilation efficiency variance explained by all factors (food quality, quantity, and % detritivory), (B) proportion of the explained variance due to food quality, (C) the proportion explained by % detritivory, (D) sea water temperature. Ice covered regions (defined as grid points with an annually averaged ice cover > 50%) are masked out.
This indicates that we are capturing the major drivers of variability in the iron assimilation efficiency. Figure 5B shows that food quality emerges as the main contributor to the variance in the iron assimilation efficiency in almost all oceanic regions. Exceptions are the Arctic and the Equatorial Atlantic, where food quality only explains about 30–40% of the variance in the iron assimilation efficiency. The dominant role for food quality emerges in our model because of the direct link between the iron assimilation efficiency and food quality. Thus, strong spatial and temporal variability in the factors that control the stoichiometric ratios in the zooplankton prey, such as availability of iron and other nutrients, as well as temperature exert a primary control on the iron assimilation efficiency. In contrast, food quantity tends to play a more minor role, despite also showing large spatial and temporal variability (Figure 3B). The iron assimilation efficiency is much less sensitive to food quantity, because, in our model, it is only altered when food quantity becomes very low. Consequently, the variability of iron assimilation efficiency induced by food quantity is much weaker over most of the ocean. The detritivory proportion has a stronger impact on the variance in the iron assimilation efficiency in the Equatorial Atlantic, where it explains over 30% of iron assimilation efficiency variance, in the Southern Ocean where it explains about 20% of iron assimilation efficiency variance, and in the Arctic region where it explain over 50% of assimilation efficiency variance (Figure 5C). Finally, temperature tends to have a minor role, but it can explain about 10–20% of the variance in the iron assimilation efficiency in some mid-latitude regions (Figure 5D).
3.3. Seasonal Variability in Driving Factors of Iron Assimilation Efficiency
We now turn to changes in the iron assimilation efficiency (AE) and its driving factors over the year by focussing on three provinces with distinct seasonalities and drivers: the Southern Ocean because it has a strong seasonal variability and is iron limited, the equatorial Atlantic because of the high levels of dissolved iron availability, grazing and recycling (Figures 2, 3) and the equatorial Pacific because it is a high productivity region with high food quality, but iron limited (Figure 3D). In the Southern Ocean, there is a strong seasonal variation in all the driving variables, with food quantity and quality varying more than three-fold over the year (Figure 6A). However, we find that despite this variability in the drivers, the seasonal variability of iron assimilation efficiency in the Southern Ocean is limited to about 20%. In winter, most of the preys in the Southern Ocean are detritus because algal growth is strongly restricted by light limitation. The large seasonal variations in temperature within the Southern Ocean may lead to stronger feedbacks on food quantity and quality than in the temperate and tropical regions, explaining the higher impact of temperature on iron assimilation efficiency variance.
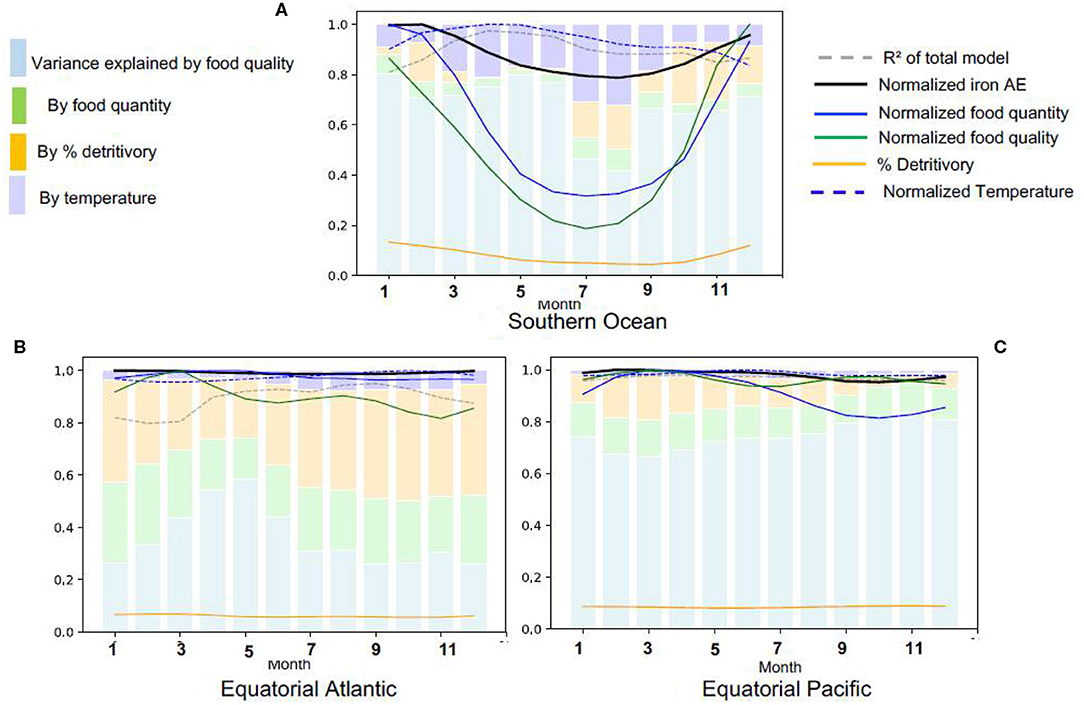
Figure 6. Seasonal variations in the determinants of iron assimilation efficiency in different Longhurst provinces, (A) Southern Ocean, (B) Equatorial Atlantic, (C) Equatorial Pacific. All values are normalized by the seasonal maximum.
In the Equatorial Atlantic and Pacific (Figures 6B,C), the iron assimilation efficiency and its drivers have a low seasonal variability. In the Equatorial Pacific, the variance in the iron assimilation efficiency is mainly driven by food quality year round. In this region, the availability of iron from the prey is relatively stable, which contributes to the maintenance of high food quality throughout the year.
In the Equatorial Atlantic, the detritivory is able to explain about 30–40% of iron assimilation efficiency variance all year long, while food quantity only explains about 20%. In the Equatorial Atlantic, food quantity is relatively high all year long while food quality is relatively poor (Figure 3), leading to a low iron assimilation efficiency and explaining the relatively high recycled iron rates, in comparison to iron uptake (Figure 2B). The large seasonal and spatial variability in the iron supply by dust deposition, especially from the Saharian dust events (Knippertz and Todd, 2012; Mokhtari et al., 2015), leads to a strong variability in the amount of scavenged iron and thus to large variations in the Fe:C of particles. This large variability in particulate iron leads to a large variability in the iron consumed through detritivory, explaining the larger contribution of detritivory to the variance in the iron assimilation efficiency.
To summarize, Figure 6 suggests that the impacts of food quality on iron assimilation efficiency vary regionally due to variability in Fe cycling. Food quality impacts are maximal when prey Fe availability is high and invariant (e.g., in the Equatorial Pacific, Figure 6C). In contrast, the contribution of food quality is minimal when coprophagy is high or in regions typified by high levels of scavenging (e.g., in the Southern Ocean in winter and equatorial Atlantic, Figure 6A).
4. Defining a Generic Relationship Linking Predator-Prey Stoichiometry and Iron Recycling
Our statistical analysis has demonstrated that the relationship between the stoichiometry of zooplankton and the stoichiometry of their preys, which we defined here broadly as “food quality,” is the main driver of iron assimilation efficiency (AE) in all oceanic regions and for most of the year in our model. In order to maximize their iron assimilation efficiency, zooplankton must feed on stoichiometrically similar preys. This result is in agreement with existing theoretical and experimental evidence (Schmidt et al., 1999; Montagnes and Fenton, 2012). Here, we explore the drivers of variations in the iron assimilation efficiency in response to changing iron stoichiometry in both predators and preys.
Iron metabolic demand for zooplankton predators (Fe:Czoo) is fixed in our model, but this value is known to vary depending on multiple factors, including the zooplankton individual size, age, sex, species, and diet (e.g., Sterner and Elser, 2002; Baines et al., 2016). Ratnarajah et al. (2014) report Fe:C ratios ranging between 4 and 69 μmolFe:molC in different species of Antarctic krill. Baines et al. (2016) report a five-fold difference in mesozooplankton Fe:C between the smaller (60–70 μmolFe:molC) and larger (20 μmolFe:molC) size classes they sampled, with a strong day-night variation. They relate this decreasing Fe quota with increasing zooplankton size to respiration rates decreasing with zooplankton size. Finally, Chen et al. (2014) have also shown that some copepod species may adjust their Fe:C ratio in response to food quality. Rearranging Equation (1), we can assess iron recycling as a function of zooplankton stoichiometry and, assuming a fixed prey stoichiometry across a typically observed range of zooplankton Fe:C ratios (Figure 7, Equation 7). In Equation (7), epsher is the maximum growth efficiency of zooplankton (fixed in the model at 0.3 for microzooplankton and 0.35 for mesozooplankton).
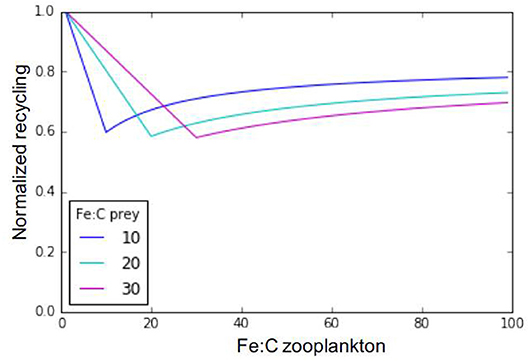
Figure 7. Relationship between zooplankton iron recycling, normalized to its maximum value, and zooplankton iron stoichiometry. The different lines represent different prey stoichiometries.
Setting X = (Fe:C)preys/(Fe:C)zoo:
Our results in Figure 7 highlight three distinctive recycling regimes for any given prey Fe:C stoichiometry. When zooplankton Fe stoichiometry is lower than prey Fe stoichiometry, Fe recycling is high due to excess consumption of Fe. Likewise, when zooplankton Fe stoichiometry is very high in comparison to that of its preys, recycling is also high due to a low feeding efficiency in response to insufficient iron consumption. Finally, if zooplankton and prey stoichiometries are the same, iron recycling is minimal and the iron assimilation efficiency is maximal. In our model, the prey stoichiometry is typically higher than zooplankton stoichiometry in most oceanic regions (Figure 3D), leading to high rates of recycling due to excess iron consumption.
We can further generalize the conceptual model emerging from our analysis to consider all possible combinations of predator and prey stoichiometry towards a more generic understanding of recycling. Figure 8 shows maximum iron assimilation efficiency and minimum recycling for similar predator and prey stoichiometries (close to the 1:1 line). The figure also shows that zooplankton with higher iron stoichiometry display minimal recycling for a larger range of prey stoichiometries). Overall Figure 8 highlights two different phases depending on the value of the food quality factor (Equations 6, 7):
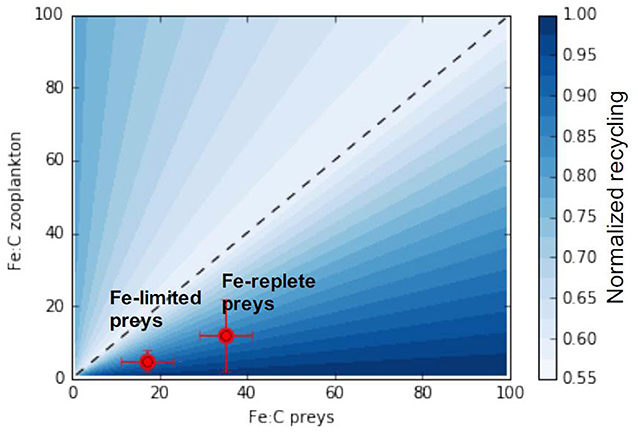
Figure 8. A generic relationship between zooplankton and prey stoichiometries and iron assimilation efficiency. The x and y-axis represent the range of observable zooplankton and prey iron stoichiometries. The black line represents the 1:1 line for which predator and preys stoichiometry are equal. Red points presents the predator prey stoichiometric relationship from the experiments of Schmidt et al. (1999).
If food quality ≤ 1:
If food quality > 1:
As iron recycling increases more rapidly when predator-prey stoichiometries diverge from the 1:1 line in favor of prey becoming richer in iron (food quality towards the right side of Figure 8), the zooplankton assimilation efficiency varies less for an identical shift to prey becoming iron poor.
In the ocean, prey iron stoichiometry, is variable as a consequence of varying nutrient sources and responses of phytoplankton to external nutrient concentrations (Twining and Baines, 2013). Thus as prey change their stoichiometry they may move closer or further from the 1:1 line, depending on the initial match-mismatch with the predator stoichiometry, thus modulating iron recycling rates. In this manner, we can understand the experiments by Schmidt et al. (1999) (illustrated in Figure 8). These experiments showed that, as diatoms switched from being iron replete (Fe:C ≈ 35 μmol:mol) to iron deplete (Fe:C ≈ 17 μmol:mol) at a given predator Fe:C (5–12 μmol:mol), the iron assimilation efficiency increased (and iron recycling decreased) as the system moved leftwards from the lower right hand component of Figure 7 closer to the 1:1 line.
We can use the conceptual framework in Figures 1, 7 to generate hypotheses regarding the potential significance of recycling for other micronutrients. For instance, Mn and Co are known to have very low zooplankton stoichiometries (0.03 μmolCo:molC and ~3 μmolMn:molC; Ratnarajah et al., 2014; Baines et al., 2016) relative to phytoplankton (1–67 μmolCo:molC and 4–40 μmolMn:molC; Ratnarajah et al., 2014; Baines et al., 2016), which would imply large rates of Mn and Co recycling due to overconsumption. Zinc on the other hand, has a zooplankton stoichiometry that is more closely matched to observed phytoplankton Zn levels (zooplankton Zn:C measured between 10 and 100 μmolZn:molC and phytoplankton Zn:C around 66 μmolZn:molC; Twining and Baines, 2013; Ratnarajah et al., 2014; Baines et al., 2016). This suggests that variations in Zn stochiometry of phytoplankton (e.g., from the Zn rich Southern Ocean to the Zn poor low latitudes) will modulate Zn recycling rates.
Zooplankton could lower their sensitivity to any stoichiometric mismatch with their prey, and then increase their iron assimilation efficiency at low prey Fe:C ratio, by increasing their food use efficiency (e.g., by a decrease sloppy feeding). A change in the detritivory proportion may change the unassimilated fraction and change zooplankton tolerance to a stoichiometric mismatch with their preys. Likewise, temperature may influence zooplankton tolerance through variations in iron particle concentration via remineralization. At high prey Fe content, the only option for zooplankton to increase their assimilation efficiency and reduce recycling is to store iron. However, there is, to our knowledge, no evidence that a zooplankton individual can adjust their physiology in this manner. Since the individual iron stoichiometry of zooplankton represents their metabolic demand for iron, it results from evolutionary and developmental conditions and would be expected to vary on longer time scales than the prey stoichiometry.
5. Wider Implications
5.1. Representing Trace Metal Assimilation and Recycling Using Simple Parameters
We have used a relatively complex model of ocean biogeochemical cycling to provide a new perspective on iron assimilation efficiency and recycling by zooplankton in the global ocean, highlighting that the stoichiometric mismatch between zooplankton and its preys is the main driver of iron assimilation efficiency. However, measurements of iron assimilation efficiency and recycling by zooplankton from in situ and laboratory studies are rare. The important role for food quality in driving the iron assimilation efficiency in our model is consistent with the available data. Shifts in prey stoichiometry in the laboratory have induced changes in iron assimilation efficiency consistent with our conceptual model (Schmidt et al., 1999) Figure 8. Carnivorous zooplankton, who consume other zooplankton with similar stoichiometries and metabolic demands (Sterner and Elser, 2002) have been observed to show greater carbon assimilation efficiency (Purcell, 1983). Thus, we can draw a simplified representation of the factors influencing iron recycling in the surface ocean. Figure 1 highlights the direct effects of food quality and quantity on iron recycling (also shown by Equation 1). The figure also highlights how factors such as temperature and zooplankton diet can indirectly influence recycling. Temperature affects zooplankton grazing rates (food quantity) and particle remineralization (Laufkötter et al., 2017), which impacts food quantity and quality through changes in particles stoichiometry. The detritivory proportion may impact food quality since organic and inorganic particulate matter are often iron rich (Ohnemus and Lam, 2015; Tagliabue et al., 2019).
Our results suggest that iron recycling depends directly on food quantity and quality (see Equation 1), and that temperature and diet composition have indirect effects through their influence of food quantity and quality. However, food quantity and quality can be influenced by other factors such as nutrient concentrations and light availability (see Aumont et al., 2015). Moreover, in PISCES, iron recycling is influenced by zooplankton physiological parameters such as feeding efficiency, and the unassimilated fraction of preys. In PISCES, feeding efficiency varies with food quality, with the hypothesis that if food quality is poor (i.e., Fe:Czoo > Fe:Cpreys), it is more difficult to digest and feeding efficiency is low. This was observed by Chen et al. (2014) for iron, however, the relationship between food quality and feeding efficiency is different for macronutrients with some species increasing their feeding efficiency with food quality, and others decreasing it (see Sterner and Elser, 2002). Studies also show that prey unassimilated fraction varies with predator-prey size fraction (Møller, 2007) and zooplankton diet (Steinberg and Landry, 2017). Chen et al. (2014) showed that trace metal assimilation efficiency is correlated to trace metal cytoplasmic content in phytoplankton preys. PISCES does not represent the partition of iron in phytoplankton cells, and prey quality is represented as the averaged Fe:C in phytoplankton cells and particulate matter. Further developments of PISCES to include a variability in zooplankton physiology and iron partitioning in the phytoplankton and zooplankton cells could help better represent the food quality factor and its spatial and temporal variability. However, such developments are at present hindered by the availability of experimental data on phytoplankton and zooplankton trace metal physiology.
Finally, our approach in this study was to consider iron assimilation by zooplankton independently of other nutritive elements. However, zooplankton preys are composed of multiple macro and micronutrients and iron assimilation may also be influenced by the assimilation of other nutrients. Zooplankton growth limitation by another element, or ingestion of toxic elements, may have consequences on zooplankton iron assimilation which are not considered in this study, but are clearly worth investigating.
5.2. Implications for the Future Oceans
The stoichiometric mismatch between zooplankton and its preys (food quality) is the main driving factor of iron assimilation efficiency. Therefore, it is important to understand how the stoichiometries of zooplankton and its preys may vary in the present and future in order to understand how iron assimilation efficiency and recycling may evolve. Changes in nutrient balances in the ocean, driven by changes in nutrient sources and sinks or water circulation, may affect prey stoichiometry (Kwiatkowski et al., 2018). As zooplankton are secondary consumers, we do not know if and on what time scale they may adapt their physiology to changes in prey stoichiometry. Increased water temperature may lead to metabolic and phenotypical changes in zooplankton. For instance, Richardson (2008) highlighted changes in zooplankton distribution and earlier biomass peak of many species in different oceanic regions. These responses to climate change may modify the metabolic demand of zooplankton for iron and the optimal window size. According to Sterner and Elser (2002), zooplankton could respond to a change in prey stoichiometry by changing their food intake (grazing pressure and ingestion) and prey preference or changing their metabolism (Richardson, 2008). If zooplankton species fail to adapt to changes in prey stoichiometry, we may observe in the future a change in the iron recycling rates in the surface ocean, with potential impacts on the global iron biogeochemical cycle.
Moreover, changes in optimal window size of larger species such as mesozooplankton would result from metabolic changes driven by phenotypic and genetic evolutions. These changes may happen on generational to evolutionary timescales. A failure to overcome the stoichiometric mismatch may even lead to zooplankton species disappearance or out competition by different predators, with potential consequences on the entire marine food web. For instance, Chen et al. (2011) show that the naupliar survival of copepods is highly reduced when copepods feed on Fe-limited preys.
Conclusions
Our modeling and statistical approach showed that iron assimilation and recycling by zooplankton is primarily determined by the stoichiometric mismatch between zooplankton and their preys. For zooplankton, food quality is more important than food quantity when it comes to trace metal assimilation efficiency. This is similar to the ecological stoichiometry for macronutrients described by Sterner and Elser (2002), and may be extended to consider other essential trace metals. This relatively simple conclusion may have important impact on the way nutrient cycling in the oceans are studied: zooplankton being important recyclers of trace metals enhancing their bioavailability and residence time in the upper water column, taking into account plankton stoichiometry and not just biomass is key to understanding the current and future micronutrient biogeochemical cycles in the ocean.
Data Availability Statement
Data used for the article are accessible on the Zenodo platform at: https://doi.org/10.5281/zenodo.3706493.
Author Contributions
CR and AT designed the study. CR, OA, and AT contributed to the writing and revision of the manuscript. All authors contributed to the article and approved the submitted version.
Funding
This project has received funding from the European Research Council (ERC) under the European Union's Horizon 2020 research and innovation programme (Grant agreement No. 724289).
Conflict of Interest
The authors declare that the research was conducted in the absence of any commercial or financial relationships that could be construed as a potential conflict of interest.
References
Anderson, S. R., and Harvey, E. L. (2019). Seasonal variability and drivers of microzooplankton grazing and phytoplankton growth in a subtropical estuary. Front. Mar. Sci. 6:174. doi: 10.3389/fmars.2019.00174
Aumont, O., Ethé, C., Tagliabue, A., Bopp, L., and Gehlen, M. (2015). PISCES-v2: an ocean biogeochemical model for carbon and ecosystem studies. Geosci. Model Dev. 8, 2465–2513. doi: 10.5194/gmd-8-2465-2015
Aumont, O., Maury, O., Lefort, S., and Bopp, L. (2018). Evaluating the potential impacts of the diurnal vertical migration by marine organisms on marine biogeochemistry. Glob. Biogeochem. Cycles 32, 1622–1643. doi: 10.1029/2018GB005886
Baines, S. B., Chen, X., Twining, B. S., Fisher, N. S., and Landry, M. R. (2016). Factors affecting Fe and Zn contents of mesozooplankton from the Costa Rica Dome. J. Plankt. Res. 38, 331–347. doi: 10.1093/plankt/fbv098
Barbeau, K., Kujawinski, E. B., and Moffett, J. W. (2001). Remineralization and recycling of iron, thorium and organic carbon by heterotrophic marine protists in culture. Aquat. Microb. Ecol. 24, 69–81. doi: 10.3354/ame024069
Beaugrand, G., Brander, K. M., Alistair Lindley, J., Souissi, S., and Reid, P. C. (2003). Plankton effect on cod recruitment in the North Sea. Nature 426, 661–664. doi: 10.1038/nature02164
Bertram, D. F., Mackas, D. L., and McKinnell, S. M. (2001). The seasonal cycle revisited: interannual variation and ecosystem consequences. Prog. Oceanogr. 49, 283–307. doi: 10.1016/S0079-6611(01)00027-1
Boyd, P. W., Arrigo, K. R., Strzepek, R., and Dijken, G. L. v. (2012). Mapping phytoplankton iron utilization: insights into Southern Ocean supply mechanisms. J. Geophys. Res 117:C06009. doi: 10.1029/2011JC007726
Boyd, P. W., Ibisanmi, E., Sander, S. G., Hunter, K. A., and Jackson, G. A. (2010). Remineralization of upper ocean particles: implications for iron biogeochemistry. Limnol. Oceanogr. 55, 1271–1288. doi: 10.4319/lo.2010.55.3.1271
Boyd, P. W., Strzepek, R. F., Ellwood, M. J., Hutchins, D. A., Nodder, S. D., Twining, B. S., et al. (2015). Why are biotic iron pools uniform across high- and low-iron pelagic ecosystems? Glob. Biogeochem. Cycles 29, 1028–1043. doi: 10.1002/2014GB005014
Buitenhuis, E., Quéré, C. L., Aumont, O., Beaugrand, G., Bunker, A., Hirst, A., et al. (2006). Biogeochemical fluxes through mesozooplankton. Glob. Biogeochem. Cycles 20:GB2003. doi: 10.1029/2005GB002511
Calbet, A., and Landry, M. R. (2004). Phytoplankton growth, microzooplankton grazing, and carbon cycling in marine systems. Limnol. Oceanogr. 49, 51–57. doi: 10.4319/lo.2004.49.1.0051
Chen, X., Baines, S. B., and Fisher, N. S. (2011). Can copepods be limited by the iron content of their food? Limnol. Oceanogr. 56, 451–460. doi: 10.4319/lo.2011.56.2.0451
Chen, X., Fisher, N. S., and Baines, S. B. (2014). Influence of algal iron content on the assimilation and fate of iron and carbon in a marine copepod. Limnol. Oceanogr. 59, 129–140. doi: 10.4319/lo.2014.59.1.0129
Dalpadado, P., Bogstad, B., Gjøsłter, H., Mehl, S., and Skjoldal, H. R. (2002). “Chapter 9: Zooplankton-fish interactions in the Barents Sea,” in Large Marine Ecosystems, Volume 10 of Marine Ecosystems of the North Atlantic, eds K. Sherman and H. R. Skjoldal (Elsevier), 10, 269–291. doi: 10.1016/S1570-0461(02)80061-1
Giering, S. L. C., Steigenberger, S., Achterberg, E. P., Sanders, R., and Mayor, D. J. (2012). Elevated iron to nitrogen recycling by mesozooplankton in the Northeast Atlantic Ocean: Fe:N recycling by mesozooplankton. Geophys. Res. Lett. 39:L12608. doi: 10.1029/2012GL051776
Groemping, U. (2006). Relative importance for linear regression in R: the package relaimpo. J. Stat. Softw. 17, 1–27. doi: 10.18637/jss.v017.i01
Hutchins, D., and Bruland, K. (1994). Grazer-mediated regeneration and assimilation of Fe, Zn and Mn from planktonic prey. Mar. Ecol. Prog. Ser. 110, 259–269. doi: 10.3354/meps110259
Hutchins, D. A., DiTullio, G. R., and Burland, K. W. (1993). Iron and regenerated production: evidence for biological iron recycling in two marine environments. Limnol. Oceanogr. 38, 1242–1255. doi: 10.4319/lo.1993.38.6.1242
Jeppesen, E., Søndergaard, M., Sortkjoær, O., Mortensen, E., and Kristensen, P. (1990). Interactions between phytoplankton, zooplankton and fish in a shallow, hypertrophic lake: a study of phytoplankton collapses in Lake Søbygaard, Denmark. Hydrobiologia 191, 149–164. doi: 10.1007/BF00026049
Knippertz, P., and Todd, M. C. (2012). Mineral dust aerosols over the Sahara: meteorological controls on emission and transport and implications for modeling. Rev. Geophys. 50:RG1007. doi: 10.1029/2011RG000362
Kwiatkowski, L., Aumont, O., Bopp, L., and Ciais, P. (2018). The impact of variable phytoplankton stoichiometry on projections of primary production, food quality, and carbon uptake in the global ocean. Glob. Biogeochem. Cycles 32, 516–528. doi: 10.1002/2017GB005799
Laufkötter, C., John, J. G., Stock, C. A., and Dunne, J. P. (2017). Temperature and oxygen dependence of the remineralization of organic matter. Glob. Biogeochem. Cycles 31, 1038–1050. doi: 10.1002/2017GB005643
Laufkötter, C., Vogt, M., Gruber, N., Aita-Noguchi, M., Aumont, O., Bopp, L., et al. (2015). Drivers and uncertainties of future global marine primary production in marine ecosystem models. Biogeosciences 12, 6955–6984. doi: 10.5194/bg-12-6955-2015
“Longhurst, A. R. (ed). (2010). Chapter 7 - Provinces: the secondary compartments,” Ecological Geography of the Sea (Second Edition) (Academic Press), 103–114. doi: 10.1016/B978-012455521-1/50008-5
Mitra, A., Flynn, K. J., and Fasham, M. J. (2007). Accounting for grazing dynamics in nitrogen-phytoplankton-zooplankton (NPZ) models. Limnol. Oceanogr. 52, 649–661. doi: 10.4319/lo.2007.52.2.0649
Mokhtari, M., Tulet, P., Fischer, C., Bouteloup, Y., Bouyssel, F., and Brachemi, O. (2015). Three-dimensional dust aerosol distribution and extinction climatology over northern Africa simulated with the ALADIN numerical prediction model from 2006 to 2010. Atmos. Chem. Phys. 15, 9063–9082. doi: 10.5194/acp-15-9063-2015
Møller, E. F. (2007). Production of dissolved organic carbon by sloppy feeding in the copepods Acartia tonsa, Centropages typicus, and Temora longicornis. Limnol. Oceanogr. 52, 79–84. doi: 10.4319/lo.2007.52.1.0079
Montagnes, D. J., and Fenton, A. (2012). Prey-abundance affects zooplankton assimilation efficiency and the outcome of biogeochemical models. Ecol. Model. 243, 1–7. doi: 10.1016/j.ecolmodel.2012.05.006
Ohnemus, D. C., and Lam, P. J. (2015). Cycling of lithogenic marine particles in the US GEOTRACES North Atlantic transect. Deep Sea Res. Part II 116, 283–302. doi: 10.1016/j.dsr2.2014.11.019
Purcell, J. E. (1983). Digestion rates and assimilation efficiencies of siphonophores fed zooplankton prey. Mar. Biol. 73, 257–261. doi: 10.1007/BF00392251
Rafter, P. A., Sigman, D. M., and Mackey, K. R. M. (2017). Recycled iron fuels new production in the eastern equatorial Pacific Ocean. Nat. Commun. 8, 1–10. doi: 10.1038/s41467-017-01219-7
Ratnarajah, L., Bowie, A. R., Lannuzel, D., Meiners, K. M., and Nicol, S. (2014). The biogeochemical role of baleen whales and krill in Southern Ocean nutrient cycling. PLoS ONE 9:e0125134. doi: 10.1371/journal.pone.0114067
Richardson, A. J. (2008). In hot water: zooplankton and climate change. ICES J. Mar. Sci. 65, 279–295. doi: 10.1093/icesjms/fsn028
Richon, C., Dutay, J.-C., Bopp, L., Le Vu, B., Orr, J. C., Somot, S., et al. (2019). Biogeochemical response of the Mediterranean Sea to the transient SRES-A2 climate change scenario. Biogeosciences 16, 135–165. doi: 10.5194/bg-16-135-2019
Richon, C., Dutay, J.-C., Dulac, F., Wang, R., Balkanski, Y., Nabat, P., et al. (2018). Modeling the impacts of atmospheric deposition of nitrogen and desert dust-derived phosphorus on nutrients and biological budgets of the Mediterranean Sea. Prog. Oceanogr. 163, 21–39. doi: 10.1016/j.pocean.2017.04.009
Schlitzer, R., Anderson, R. F., Dodas, E. M., Lohan, M., Geibert, W., Tagliabue, A., et al. (2018). The GEOTRACES intermediate data product 2017. Chem. Geol. 493, 210–223. doi: 10.1016/j.chemgeo.2018.05.040
Schmidt, K., Schlosser, C., Atkinson, A., Fielding, S., Venables, H. J., Waluda, C. M., et al. (2016). Zooplankton gut passage mobilizes lithogenic iron for ocean productivity. Curr. Biol. 26, 2667–2673. doi: 10.1016/j.cub.2016.07.058
Schmidt, M., and Zhang, Y. A H. D. (1999). Assimilation of Fe and carbon by marine copepods from Fe-limited and Fe-replete diatom prey. J. Plankton Res. 21, 1753–1764. doi: 10.1093/plankt/21.9.1753
Steinberg, D. K., and Landry, M. R. (2017). Zooplankton and the ocean carbon cycle. Annu. Rev. Mar. Sci. 9, 413–444. doi: 10.1146/annurev-marine-010814-015924
Sterner, R., and Elser, J. (2002). Ecological Stoichiometry: The Biology of Elements from Molecules to the Biosphere. Princeton; Oxford: Princeton University Press. doi: 10.2307/j.ctt1jktrp3
Strzepek, R. F., Maldonado, M. T., Higgins, J. L., Hall, J., Safi, K., Wilhelm, S. W., et al. (2005). Spinning the “Ferrous Wheel”?: the importance of the microbial community in an iron budget during the FeCycle experiment. Glob. Biogeochem. Cycles 19:GB4S26. doi: 10.1029/2005GB002490
Tagliabue, A., Aumont, O., DeAth, R., Dunne, J. P., Dutkiewicz, S., Galbraith, E., et al. (2016). How well do global ocean biogeochemistry models simulate dissolved iron distributions? Glob. Biogeochem. Cycles 30, 149–174. doi: 10.1002/2015GB005289
Tagliabue, A., Bowie, A. R., Boyd, P. W., Buck, K. N., Johnson, K. S., and Saito, M. A. (2017). The integral role of iron in ocean biogeochemistry. Nature 543, 51–59. doi: 10.1038/nature21058
Tagliabue, A., Bowie, A. R., DeVries, T., Ellwood, M. J., Landing, W. M., Milne, A., et al. (2019). The interplay between regeneration and scavenging fluxes drives ocean iron cycling. Nat. Commun. 10:4960. doi: 10.1038/s41467-019-12775-5
Tagliabue, A., Hawco, N. J., Bundy, R. M., Landing, W. M., Milne, A., Morton, P. L., et al. (2018). The role of external inputs and internal cycling in shaping the global ocean cobalt distribution: insights from the first cobalt biogeochemical model. Glob. Biogeochem. Cycles 32, 594–616. doi: 10.1002/2017GB005830
Twining, B. S., and Baines, S. B. (2013). The trace metal composition of marine phytoplankton. Annu. Rev. Mar. Sci. 5, 191–215. doi: 10.1146/annurev-marine-121211-172322
Wang, W.-X., Reinfelder, J. R., Lee, B.-G., and Fisher, N. S. (1996). Assimilation and regeneration of trace elements by marine copepods. Limnol. Oceanogr. 41, 70–81. doi: 10.4319/lo.1996.41.1.0070
Keywords: zooplankton, stoichiometry, recycling, predator, prey, iron
Citation: Richon C, Aumont O and Tagliabue A (2020) Prey Stoichiometry Drives Iron Recycling by Zooplankton in the Global Ocean. Front. Mar. Sci. 7:451. doi: 10.3389/fmars.2020.00451
Received: 12 March 2020; Accepted: 22 May 2020;
Published: 30 June 2020.
Edited by:
Antonio Tovar-Sanchez, Consejo Superior de Investigaciones Científicas (CSIC), SpainReviewed by:
S. Lan Smith, Japan Agency for Marine-Earth Science and Technology (JAMSTEC), JapanPatrick Rafter, University of California, Irvine, United States
Copyright © 2020 Richon, Aumont and Tagliabue. This is an open-access article distributed under the terms of the Creative Commons Attribution License (CC BY). The use, distribution or reproduction in other forums is permitted, provided the original author(s) and the copyright owner(s) are credited and that the original publication in this journal is cited, in accordance with accepted academic practice. No use, distribution or reproduction is permitted which does not comply with these terms.
*Correspondence: Camille Richon, crichon@liverpool.ac.uk