- 1Department of Geography, Florida State University, Tallahassee, FL, United States
- 2Department of Biological Science, Florida State University, Tallahassee, FL, United States
Coastal and marine ecosystems characterized by foundation species, such as seagrass beds, coral reefs, salt marshes, oyster reefs, and mangrove forests, are rich in biodiversity and support a range of ecosystem services including coastal protection, food provisioning, water filtration, carbon sequestration, recreational opportunities, cultural value, among others. These ecosystems have experienced degradation and a net loss of total area in regions around the world due to a host of anthropogenic stressors, resulting in declines in the associated ecosystem services they provide. Because of the extensive degradation in many locations, increasing attention has turned to ecosystem restoration of these marine habitats. Restoration techniques for marine and coastal ecosystems are generally more expensive when compared to terrestrial ecosystems, highlighting the importance of carefully selecting locations that will provide the largest return on investment, not only for the probability and magnitude of restoration success, but also for ecosystem service outcomes. However, site selection and spatial planning for marine ecosystem restoration receive relatively little attention in the scientific literature, suggesting a need to better study how spatial planning tools could be incorporated into restoration practice. To the degree that site selection has been formally evaluated in the literature, the criteria have tended to focus more on environmental conditions beneficial for the restored habitat, and less on ecosystem service outcomes once the habitat is restored, which may vary considerably from site to site, or with more complex landscape dynamics and spatial patterns of connectivity. Here we (1) review recent (2015–2019) scientific peer-reviewed literature for several marine ecosystems (seagrass beds, salt marshes, and mangrove forests) to investigate how commonly site selection or spatial planning principles are applied or investigated in scholarly research about marine ecosystem restoration at different spatial scales, (2) provide a conceptual overview of the rationale for applying spatial planning principles to marine ecosystem restoration, and (3) highlight promising analytical approaches from the marine spatial planning and conservation planning literatures that could help improve restoration outcomes. We argue that strategic site selection and spatial planning for marine ecosystem restoration, particularly applied at larger spatial scales and accounting for ecosystem service outcomes, can help support more effective restoration.
Introduction
Coastal and marine ecosystems characterized by foundation species, such as seagrass beds, coral reefs, salt marshes, oyster reefs, and mangrove forests, support a range of ecosystem services important to society including storm protection, food provisioning, water filtration, erosion control, carbon sequestration, recreational opportunities, cultural value, among others (Barbier et al., 2011). All of these ecosystems have experienced degradation and a net loss of total area in regions around the world because of a host of anthropogenic stressors, with associated declines in the ecosystem services that they provide (Valiela et al., 2001; Waycott et al., 2009, IPBES, 2019). Ecosystem degradation is so widespread that is it no longer sufficient to focus just on traditional approaches to ecosystem conservation (e.g., protected areas, threat reduction). In addition to preventing further declines, it will be necessary to restore ecosystems in locations where they have been lost. However, funding for restoration is often inadequate, raising challenging questions about how to best allocate scarce resources.
Traditionally, ecosystem restoration was primarily motivated by protecting biodiversity and restoring the natural character of systems degraded by human development (Hughes et al., 2018). However, in parallel with the growing appreciation for the importance of the ecosystem services provided by natural and properly functioning ecosystems, there is increasing policy focus on restoration efforts that protect or enhance ecosystem services (Bullock et al., 2011). For example, the Convention of Biological Diversity (CBD)’s Aichi Target 14 calls for the restoration and protection of ecosystems that provide essential services by 2020 (CBD, 2010). More recent initiatives, such as the United Nations Sustainable Development Goals (UNGA, 2015) and the zero draft of the CBD post-2020 global biodiversity framework (CBD, 2020), highlight that ecosystem services are a key motivation for ecosystem protection and restoration. Consistent with this policy attention, increasingly many restoration projects focus on ecosystem service objectives (e.g., Allan et al., 2013; Kittinger et al., 2016; Hagger et al., 2017). As pointed out by Bullock et al. (2011), this shift in focus from biodiversity conservation to ecosystem services creates both opportunities and challenges for restoration. A focus on ecosystem services provides an important motivation for funding and executing restoration projects (De Groot et al., 2013; Matzek, 2018). Furthermore, markets for services and specifically payment for ecosystem services schemes could provide an important funding stream to support restoration activities (e.g., Bonn et al., 2014; Hejnowicz et al., 2015; Matzek et al., 2015). However, there could also be tension between these two management goals; although biodiversity and ecosystem services are often positively correlated (Benayas et al., 2009; Nelson et al., 2009), that is not always the case and the relationship between the two can be complex (Tallis et al., 2008).
Given that a key driver of many restoration projects going forward will be recovering the ecosystem services provided by the restored habitat, the impact of many restoration projects is likely to be measured based on the services that are provided. Restoration techniques for marine and coastal ecosystems are generally very expensive (Bayraktarov et al., 2016), particularly compared to terrestrial ecosystem restoration costs, highlighting the importance of selecting locations that will provide the largest return on investment. Poor siting decisions can contribute to failed restoration projects (Fonseca, 2011; Bayraktarov et al., 2016), and indeed many marine and coastal systems have low success rates that contribute to their high average costs (e.g., van Katwijk et al., 2016). Furthermore, return on investment can be assessed as the value that people place on the resulting ecosystem services, suggesting that restoration in areas where people rely on the benefits provided by those systems may be more worthwhile investments.
While restoration projects involve decisions about where to target restoration, it is not clear how often such decisions are based on analyses that explicitly consider alternative sites, or whether such decisions are occurring at appropriate spatial scales and accounting for ecosystem service outcomes. This is surprising given the increasingly wide array of spatial planning tools that are being applied in other fields of conservation and management (such as marine protected area design and marine spatial planning). While the scientific literature on marine ecosystem restoration is rich with studies comparing alternative restoration techniques within a single site, there has been less attention paid to the potentially equally important impacts of decisions about where to conduct restoration in the first place. When site selection is considered, there is often a focus on environmental conditions beneficial for the restored habitat (e.g., Pollack et al., 2012; Valle et al., 2015; Hotaling-Hagan et al., 2017), key threats that need to be avoided or mitigated for restoration to succeed (e.g., Hotaling-Hagan et al., 2017), and/or the historical distribution of the species or habitat as an indicator of whether restoration is likely to be effective (e.g., Braje et al., 2016). For example, Pollack et al. (2012) developed a restoration suitability index model for oysters based on abiotic conditions such as salinity, temperature, turbidity, dissolved oxygen, and depth, and a reef quality index that accounted for the abundance of live oysters, dead shells and spat. These types of approaches are useful, but they overlook the likely spatial variation in ecosystem service outcomes once the habitat is restored.
In this paper we seek to catalyze the wider adoption of quantitative spatial analyses from the closely related fields of marine spatial planning, conservation planning, and spatial ecology into the science and practice of marine ecosystem restoration. Our first step is to present a structured review of the peer-reviewed scientific literature from 2015 through 2019 for three key marine ecosystems, seagrass beds, salt marshes, and mangrove forests. With this comprehensive survey of a limited set of years and ecosystems, we were able to document how site selection is being incorporated into recent restoration research for these representative ecosystems. Examining this literature, we investigate whether and how site selection and spatial planning principles are being applied at different spatial scales. We then provide a conceptual overview of the rationale for applying spatial planning principles to marine ecosystem restoration, highlighting important issues that may not be adequately considered in the current literature. We then discuss specific analytical approaches from other literatures, summarizing how each is used in related conservation and management fields and illustrating how they could improve outcomes in marine ecosystem restoration. Strategic spatial planning for marine ecosystem restoration, using the approaches and tools discussed in this paper, can help support more successful, cost-effective restoration that maximizes desired ecosystem service outcomes.
Literature Review
Methods
We reviewed studies published in the scientific literature between January 2015 and November 2019. Publications were selected based on a topic search in the Web of Knowledge’s Web of Science Core Collection database using specific search terms and keywords for restoration, site selection or spatial planning, and the ecosystem type (Table 1). We focused our search on three ecosystems: seagrass beds, salt marshes, and mangrove forests, evaluating 146, 220, and 206 papers, respectively (see Supplementary Reference Lists). These ecosystems were selected to be illustrative and representative, recognizing that important restoration research and practice is happening in other coastal and marine ecosystems (e.g., oyster reefs, coral reefs). This literature review was more narrowly focused in terms of ecosystem type and publication dates than the rest of the paper.
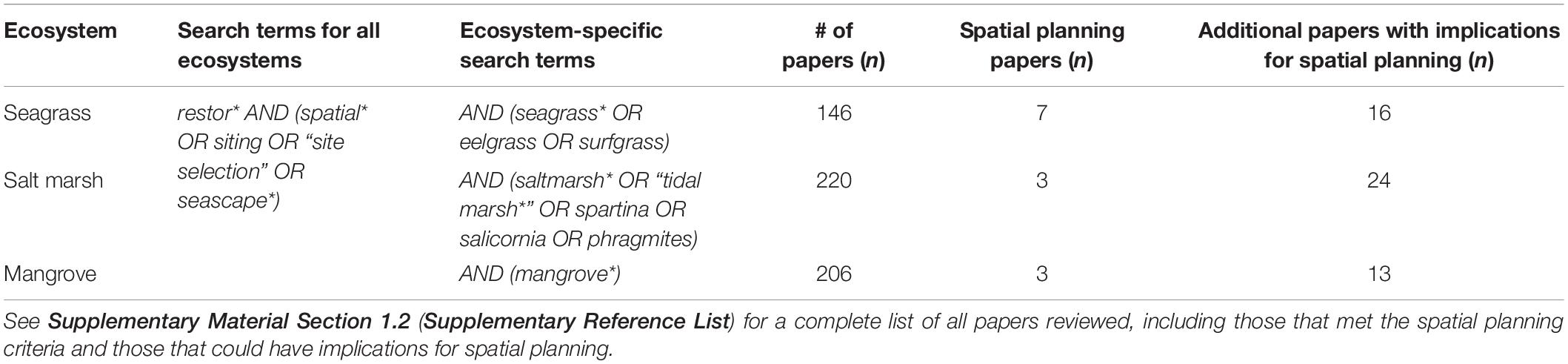
Table 1. Search strings used for each ecosystem type, number of papers published between January 2015 and November 2019 that were returned by each search and thus reviewed, number of papers that met our spatial planning criteria, and number of papers that did not meet our spatial planning criteria but could have implications for spatial planning.
We reviewed all papers returned by our searches to identify those that examined site selection for restoration and/or applied spatial planning principles to ecosystem restoration. We defined spatial planning for restoration as making strategic decisions using available data and/or models about site selection, spatial extent (i.e., size of area to be restored), or spatial configuration (i.e., landscape attributes including patch shape, connectivity, fragmentation). Only studies that examined site selection for restoration or applied spatial planning principles to restoration in the focal systems were analyzed further (Table 1, “Spatial planning papers” and Supplementary Table S1). We did not further analyze articles that did not meet these spatial planning criteria, including those that focused on a different ecosystem or were not about restoration site selection or spatial planning for restoration (e.g., ecology focused papers examining the impact of restoration on species abundance or ecosystem function). In some cases, a paper did not meet our spatial planning criteria because it was not directly about site selection or spatial planning for restoration, but the results of the paper could have implications for spatial planning for restoration, and we noted this (Table 1, “Additional papers with implications for spatial planning”), although it was a more subjective assessment and we did not further analyze these papers.
For those papers that met our spatial planning criteria (n = 7 for seagrass, 3 for salt marsh, and 3 for mangroves; Table 1), we recorded:
(1) Scale of the study, categorized based on the length of the coastline under consideration:
(a) “local” if the study spanned less than 10 km
(b) “regional” if the study was between 10 and 100 km
(c) “larger regional” if the study was between 100 and 1000 km
(d) “large ecosystem” for studies spanning a region longer than 1000 km
(2) Motivation(s) for site selection (not mutually exclusive):
(a) improve restoration success (e.g., identify locations with appropriate environmental conditions or where survival or growth is likely to be higher; identify historical distributions of the target ecosystem; identify areas without stressors that would hinder restoration success)
(b) enhance ecosystem services that could be provided by the restored ecosystem
(c) minimize the financial costs of restoration
(d) minimize conflict with human uses
(3) Analytical technique or approach(es) used for spatial planning (not mutually exclusive):
(a) experimental techniques (e.g., restoration experiments in different locations to inform site selection)
(b) habitat suitability models (HSM) for the habitat targeted for restoration, including statistical models relating vegetation occurrence to environmental factors, and index-based models that rank the relative habitat suitability of different locations
(c) mapping and spatial analysis (including mapping of ecological or social survey data and remotely sensed data, with mapping conducted by hand or using software like ArcGIS)
(d) spatial prioritization algorithms (e.g., Marxan, Zonation)
(e) social surveys (e.g., participatory mapping; stakeholder preferences)
(f) other modeling techniques (e.g., ocean circulation models)
(4) Parameter(s) for site selection used in the analysis (not mutually exclusive):
(a) environmental and biological factors (e.g., light attenuation, water quality, temperature, growth rate)
(b) current or historical presence of the habitat
(c) proximity to the habitat
(d) stressors (e.g., water quality or shoreline alterations)
(e) ecosystem services expected to be enhanced from habitat restoration (e.g., coastal protection, biodiversity enhancement, etc.)
(f) human use (e.g., boating)
We also evaluated whether the study was aiming to inform a real restoration decision or focused on more hypothetical or theoretical aspects of site selection or spatial planning.
Results and Discussion
Our review of scientific literature published between 2015 and 2019 on seagrass, salt marsh, and mangrove restoration suggests that site selection and spatial planning are rarely a research focus. Despite ∼150–220 papers per ecosystem type being returned by the focused searches we conducted, <5% of papers either examined site selection for restoration or applied spatial planning principles to ecosystem restoration (Table 1 and Supplementary Table S1). A somewhat larger number of papers, while not specifically focused on site selection or spatial planning, had potential implications for spatial planning for restoration based on their analysis and findings (Table 1). As examples, some papers conducted experiments to determine the optimal level of a limiting environmental factor(s) (e.g., Sloey et al., 2016; Xu et al., 2016), developed a species distribution model (Adams et al., 2016), or established spatial patterns of population structure and connectivity (Jahnke et al., 2018; Triest et al., 2018), results that could be used to inform site selection. Thus, information that can improve restoration site selection is actively being produced, but most restoration research is not directly evaluating spatial variability in likely restoration outcomes. An additional class of papers mentions the importance of site selection or spatial planning, but do not perform any type of analysis or suggest an approach to make spatial restoration decisions (e.g., Kodikara et al., 2017; Macreadie et al., 2017; Wegscheidl et al., 2017); these papers did not meet any of our criteria, but highlight that there is a need for analytical approaches that can be used to inform spatial planning for restoration.
For the small number of studies that met our spatial planning criteria, motivations for site selection or spatial planning varied across ecosystem type (Table 2). The most common motivation was improving restoration success, an objective of all seagrass and saltmarsh papers, while minimizing conflict with human uses and enhancing ecosystem services were relatively uncommon motivations (Table 2). Minimizing restoration costs was a motivation in about a third of the papers overall, but was not a motivation in any of the seagrass papers, despite the fact that seagrass restoration has a higher per area cost than the other two systems (Bayraktarov et al., 2016). The few papers on salt marsh and mangrove restoration included a variety of motivations, but seemed to have more emphasis on minimizing restoration costs. Enhancing ecosystem services was only mentioned as a motivation for two papers (both about mangrove restoration), a surprising result given that the ecosystem service benefits of all three habitats are a focal point of many management and conservation efforts (Needles et al., 2015; Cullen-Unsworth and Unsworth, 2018; Romañach et al., 2018). In general, social, political and cultural motivations for restoration were often not highlighted explicitly, although these drivers are required for restoration to occur. Over two-thirds of the papers were aiming to inform real restoration decisions, as opposed to studies focused on more hypothetical or theoretical cases.
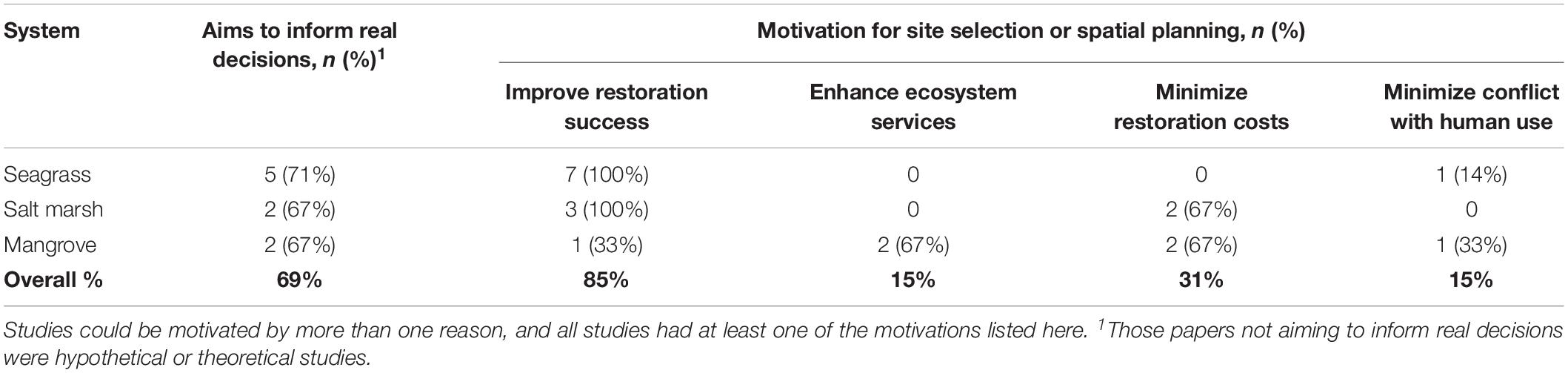
Table 2. Number (and percent) of papers meeting our spatial planning criteria that specified the aims and reasons/motivations for site selection or spatial planning for restoration.
The vast majority (85%) of the studies that met our spatial planning criteria were focused specifically on site selection, as opposed to other aspects of spatial planning, like spatial scale or spatial configuration of restoration areas (Table 3). The two studies that were not focused on site selection (one seagrass study and one salt marsh study) examined spatial scale, specifically determining the minimum spatial scale or patch size required for successful restoration (Adams et al., 2018; Gittman et al., 2018). No studies examined spatial configuration of restored sites or patterns of connectivity, although one study did account for proximity to existing habitat patches (Pirrotta et al., 2015). Furthermore, some of the studies that did not meet our criteria could have implications for approaching restoration at a seascape scale that considers habitat or population connectivity (Howe and Simenstad, 2015; Gillis et al., 2017; Taylor et al., 2017; Jahnke et al., 2018; Olson et al., 2019). Our findings are consistent with those of Gilby et al. (2018) who found, looking across terrestrial and aquatic systems, that landscape context was rarely (<15% of cases) used as a criterion for site selection for habitat restoration aimed at benefitting animal populations. They argue that more consideration of landscape attributes like habitat configuration and population connectivity could help enhance animal populations dependent on the restored habitat because of the importance of colonization dynamics (Gilby et al., 2018). This logic would apply to the valuable nursery habitat function that seagrass, mangrove, and salt marsh systems provide for many marine species, and yet none of the studies that met our spatial planning criteria looked at these types of factors.
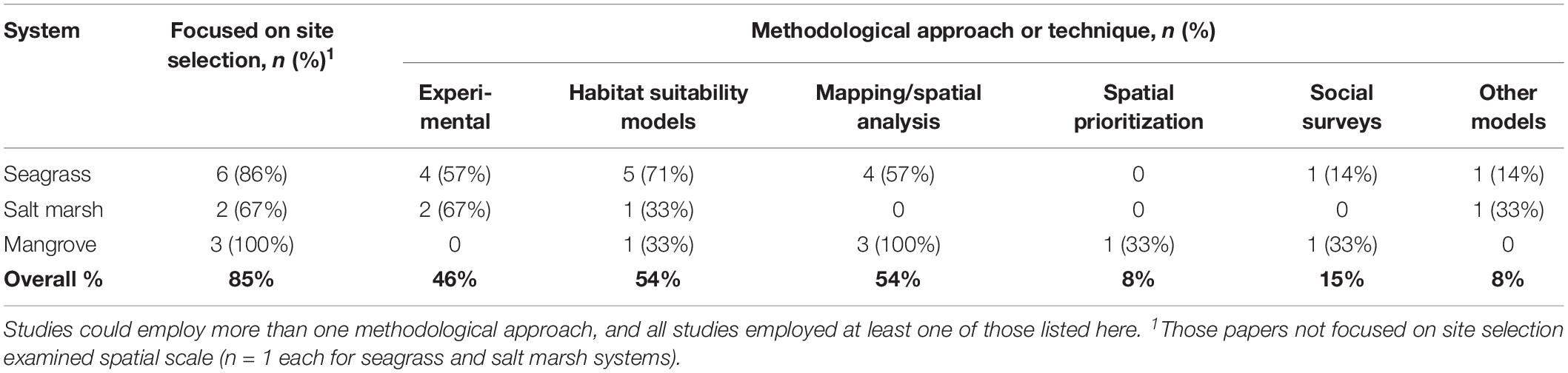
Table 3. Number (and percent) of papers meeting our spatial planning criteria that were focused specifically on site selection (those that did not focused on spatial scale), and methodological approaches used in each study.
The most common methodological approaches employed were habitat suitability models (HSMs) and mapping/spatial analysis (Table 3). Slightly less than half of the studies included an experimental study. Use of spatial prioritization algorithms, such as the conservation planning tools Marxan and Zonation, was rare (<10% of studies) despite the fact that such tools could be directly applicable to site selection analyses. Social surveys or participatory research was also relatively rare, perhaps because the motivations for site selection were often not explicitly focused on the human dimensions of ecosystem restoration (e.g., they were focused on improving restoration success rather than minimizing conflicts with human uses). The parameters most commonly used in site selection and spatial planning (Table 4) largely follow from the methodological approaches and motivations for site selection. Environmental factors predominate (85% of studies), which is consistent with the use of HSMs, experimental approaches, and mapping and spatial analysis. Expected ecosystem services were only used as a site selection parameter in a single study, and stressors and human uses were only used in less than a handful of studies, aligning with the fact that enhancing ecosystem services and reducing conflict with human use were not common motivating factors among these studies.
Across the three systems studied in the spatial planning papers, a majority (67%) examined a “regional” spatial scale (10–100 km along a coastline), with a couple operating at smaller (<10 km) or much larger (>1000 km) spatial scales (Figure 1). The presence of such large scale studies was somewhat surprising given that many actual restoration projects for these habitats are occurring at much smaller scales (Bayraktarov et al., 2016), and given that no study was focused on scales between 100 and 1000 km. On the other hand, site selection and spatial planning become more important and relevant when making decisions over larger scales, and so it makes sense that spatial planning research has a larger scale focus. Finally, the results of our literature search may not reflect the size distribution of actual restoration projects, many of which are not discussed in the peer reviewed scientific literature.
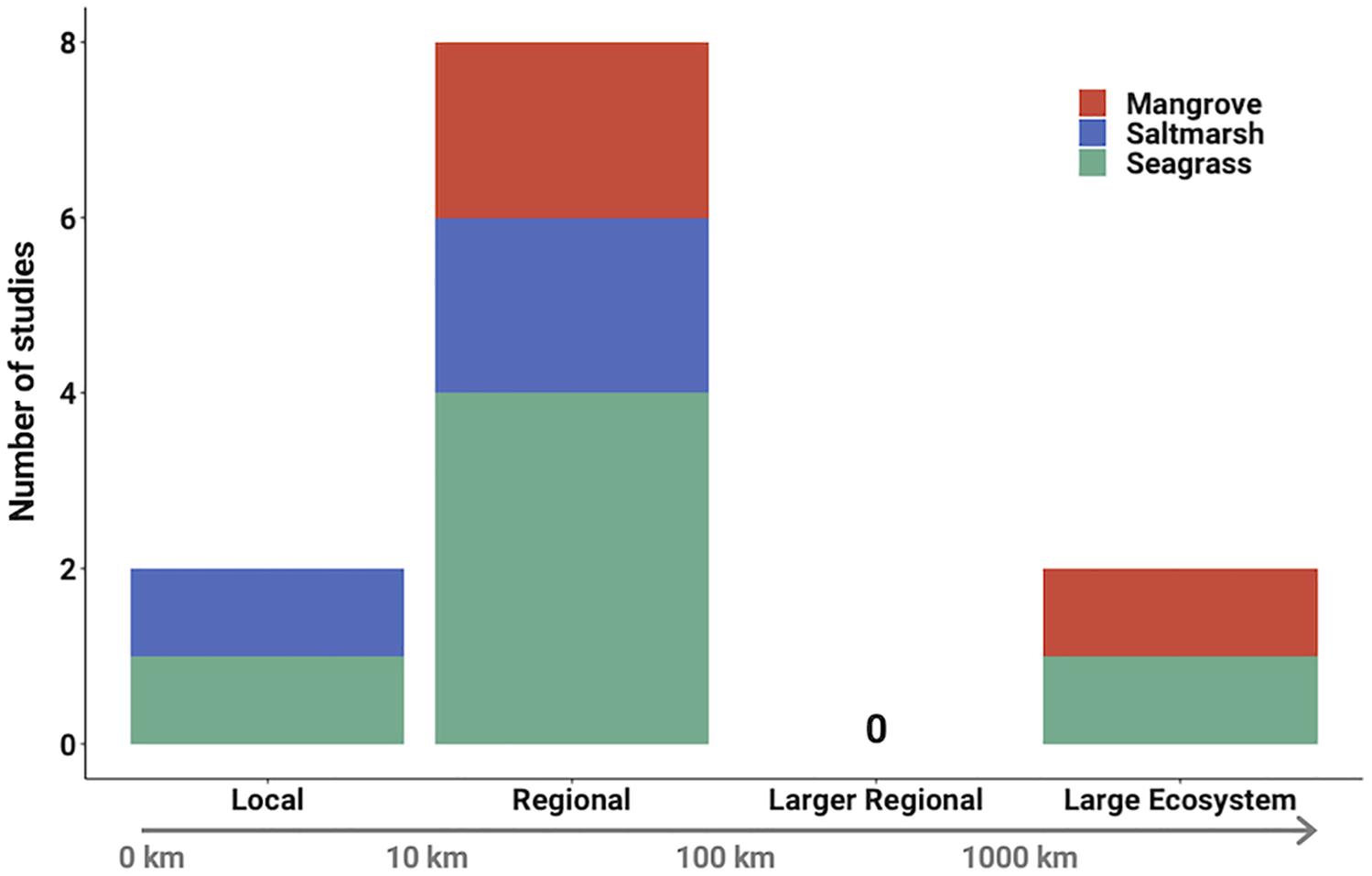
Figure 1. Spatial scale of spatial planning restoration studies (n = 12 because scale was not applicable in one modeling study).
Why Spatial Planning for Marine Ecosystem Restoration?
Spatial planning for restoration – broadly defined here as making strategic decisions using available data and/or models about site selection, spatial extent (i.e., size of area to be restored), or spatial configuration (i.e., landscape attributes including patch shape, connectivity, fragmentation) – has the potential to improve return on investment for marine ecosystem restoration projects (Figure 2). Marine environments are spatially heterogeneous at a range of spatial scales and levels of biological organization; spatial variation in environmental conditions underlies spatial variation at the ecosystem, community, population and individual levels. This creates spatial variability in ecosystem function and processes, and therefore in the ecosystem services that are provided by natural ecosystems (Koch et al., 2009; Townsend et al., 2018). The human communities that depend on marine resources and ecosystem services are also not distributed uniformly along the coast, and anthropogenic impacts can be highly variable across space. Admittedly, in some cases it might be more expedient or practical to implement a restoration project at a site without taking a step back to consider spatial planning or site selection. But in many others, accounting for this tapestry of spatial variation within restoration decision-making could pay important dividends.
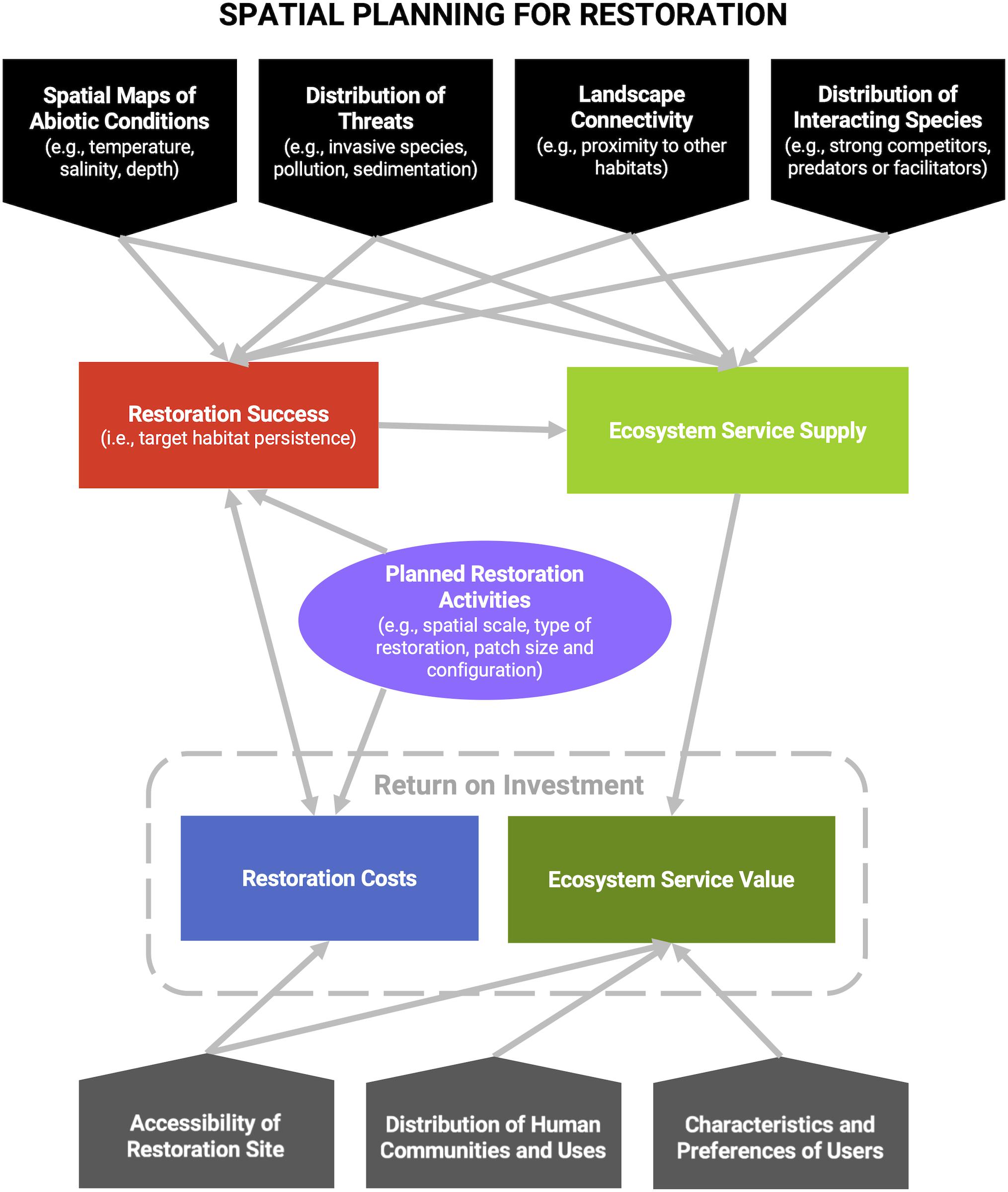
Figure 2. Conceptual figure of sources of spatial variation that influence restoration success, ecosystem service supply and value, and restoration costs. Accounting for these sources of spatial variation in restoration planning and site selection has the potential to improve return on investment, which we use here broadly to refer to the ecological and ecosystem service benefits (i.e., return) and the financial and social costs (i.e., investment) of restoration.
Expected restoration success, whether defined as the target habitat persisting following the restoration intervention for some minimum time, or as germination success, or as survival rate of a foundation species, will vary across the seascape. This is because environmental conditions that influence survival, growth, and reproduction of the target species vary across space. Furthermore, restoration success can be influenced by positive and negative species interactions (e.g., Gómez-Aparicio, 2009; Silliman et al., 2015) and these interacting species are likely patchily distributed. Connectivity with existing areas of the target habitat can also be important for successful establishment of the restored habitat (van Katwijk et al., 2016), either by ensuring supply of recruits undergoing long-distance dispersal or through migration of adults of mobile species. Such connectivity might be inferred from simple proximity or estimated from more sophisticated genetic or oceanographic analyses. Finally, spatial variation in the stressors that lead to losses necessitating restoration can impact success (Allan et al., 2013), as avoiding these stressors minimizes the chance of restoration failure.
There will also be spatial variation in the value of ecosystem services, or the benefits of economic, social or cultural value to people, that are provided by the ecosystem if successfully restored. This variation may not simply mirror the spatial pattern of restoration success. That is because while the supply of a potential service is dictated by ecological functions and biophysical aspects of the ecosystem, the use of that supply by people is what defines it as an actual ecosystem service, and peoples’ preferences for different services further define their value (Tallis et al., 2011). Thus, the supply of potential ecosystem services will vary across the seascape as a function of the factors discussed in the previous paragraph – environmental conditions impacting the growth or productivity of foundation species, species interactions, ecosystem structure and function, and connectivity between and among habitats – in addition to other biophysical aspects of the system (e.g., wave energy for coastal protection services; fish populations for fishery services). But then the use or utility of those potential services will depend on the spatial distribution of human communities along the coast or spatial patterns of human activity (e.g., fishing, recreation), and assessments of service value will further depend on human values which can vary along the coast depending on factors such as social preferences and norms, socioeconomic status, and social vulnerability to environmental threats (Tallis et al., 2011; Townsend et al., 2018).
For example, restoration of mangroves, coral reefs, and oyster reefs can be motivated by the coastal protection service provided by these biogenic habitats dampening wave energy before it reaches the coast (Barbier et al., 2011; La Peyre et al., 2015). The supply of that potential service will vary along the coastline as a function of local oceanographic conditions, wave energy, and characteristics of the ecosystem (e.g., mangrove tree height and density, live coral cover) (Koch et al., 2009). Its value as a service will vary based on where people are living along the coast, and its spatial pattern of value will be further modulated by community preferences and socioeconomic factors, including accessibility to inland shelters during major storm events, ability to rebuild properties following storm and inundation damage, preferences or resources for building artificial protective structures (e.g., seawalls), among others. Service value will not be the same for all people and can be measured for different dimensions of value. For example, the value of coastal protection could be assessed based on property value along the coast or based on the number of people impacted by storm surge, which could yield very different assessments of value (Arkema et al., 2013; Pascal et al., 2016). Collectively, these diverse dimensions of ecosystem services, which couple the social and ecological components of the system, can create rich spatial patterns that could inform restoration priorities across space.
Lastly, there may also be spatial variation in the costs associated with implementing a restoration project. This can include the actual financial costs of the project, but also the social and financial opportunity costs of pre-empting other uses of that space (e.g., restoring a mangrove forest rather than using that area for shrimp farming). Financial costs could depend on the level of ecosystem degradation in different areas, the proximity to existing areas of the ecosystem that could allow for natural recruitment, proximity to ports and other infrastructure, and the existence of stressors that need to be mitigated prior to restoration. For example, Allan et al. (2013) mapped stressors relative to ecosystem services for the Great Lakes and suggested that return on investment should be high where ecosystem services are high and stressors are low or absent because stressor mitigation will not be required. Given the high average cost of restoring even a single hectare of a marine ecosystem, it is crucial that we consider spatial variation in likely restoration success, benefits from ecosystem services, and restoration costs when setting restoration priorities.
The concepts reviewed in this section apply to all coastal and marine ecosystems and to a range of spatial scales. Restoration decision-making includes two scales: the spatial extent of the actual project (i.e., how large of an area will be restored) and the scale of site selection (i.e., how large of an area is under consideration when deciding where to do the project). Weighing the costs and benefits of different scales of restoration projects is useful (Iftekhar et al., 2017), but here we are particularly focused on the benefits from spatial planning and site selection decisions. Although the scale of decision making is often determined by jurisdictional constraints, considering larger areas is likely to include more variation in levels of expected restoration success, predicted ecosystem service benefits, and estimated restoration costs, enhancing the likelihood of identifying sites that provide the best benefit to cost ratio. We thus argue that it is useful to conduct a spatial planning analysis at the largest scale practical within existing constraints.
Approaches and Tools to Support Spatial Planning for Marine Ecosystem Restoration
Spatial planning for marine ecosystem restoration can be advanced by formal quantitative approaches. Fortunately, this does not require the creation of new methodological approaches and analytical tools, but can build on extensive work on spatial planning tools being done in related fields of marine conservation and management. Restoration can adopt existing methods from these fields with rather modest modifications, and can take lessons from how those tools have been applied to similar problems. Here we review several classes of approaches and tools that are particularly applicable to spatial planning for restoration. We borrow heavily from the conservation planning and marine spatial planning literatures, especially the former, with its established history of using spatial analysis to improve the efficiency and biodiversity outcomes of conservation projects. Some tools may fit in more than one of the categories outlined below, and the approaches we describe are not necessarily mutually exclusive. There are examples of all of these approaches being applied to ecosystem restoration as described below, although sometimes just for terrestrial systems, and these examples are often too few and far between. We hope to catalyze more widespread research and adoption of these tools for marine ecosystem restoration.
Mapping and Spatial Analysis
Geographic information systems (GIS) offer powerful and versatile tools for storing, mapping, and analyzing spatial data. As a result, GIS is already used for a variety of purposes associated with spatial planning and site selection for activities like wind farms, aquaculture, and marine protected areas (Gimpel et al., 2015; Brown et al., 2016; St-Pierre and Gagnon, 2020). In these contexts, assembling a GIS database of relevant environmental, biological, and socio-economic data layers is often a critical first step in defining the spatial domain and identifying important information gaps for a study region (Stamoulis and Delevaux, 2015). When key datasets are missing, spatial analyses and models can be used to fill the gaps using analysis platforms like ArcGIS or R (Caldow et al., 2015; Stamoulis and Delevaux, 2015), thereby enabling pixel by pixel comparisons of features across a broad spatial scale. For example, geostatistical approaches (like kriging) are commonly used to interpolate spatial patterns of environmental conditions and potential threats at unsampled locations over various spatial and temporal scales (Gimpel et al., 2015). Classification techniques applied to remote sensing imagery are also widely used to produce habitat maps (e.g., coral reefs, seafloor habitats, and kelp; Roelfsema et al., 2018; St-Pierre and Gagnon, 2020) because direct observation is usually impractical. Furthermore, many HSMs (described below) can be implemented and visualized in a GIS environment, such as those available through the Marine Geospatial Toolbox and the SDM Toolbox1,2. Ecosystem service models (described below) are also frequently implemented and visualized in a GIS platform to map the spatial distribution of potential service outcomes (Guerry et al., 2012). Lastly, participatory mapping in GIS is increasingly used to gain insights into stakeholders’ patterns of use and preferences for different planning options (Brown et al., 2016). All of these types of datasets can be combined, visualized and analyzed in a GIS program. For example, using an overlay analysis, the relative suitability of different locations for restoration can be assessed based on criteria such as environmental and physical conditions (e.g., Hogg et al., 2018), expected species distributions (e.g., Theuerkauf et al., 2019), ecosystem services, fixed constraints (e.g., regulatory boundaries) and stakeholder input.
Many of these GIS mapping and analysis techniques are readily applicable to spatial planning and site selection for marine ecosystem restoration, as demonstrated by studies analyzed in our literature review (Supplementary Table S1). For instance, GIS classification techniques applied to historic and current habitat imagery can be used to identify potential restoration sites, such as areas that previously supported seagrass habitats (Pirrotta et al., 2015) or currently support degraded mangrove habitats (Adame et al., 2015). Further, geostatistical interpolation can be used to create spatial layers for environmental and anthropogenic factors that could become barriers to successful restoration of target species (e.g., light availability and boating disturbance in the case of seagrass beds; Hotaling-Hagan et al., 2017). Habitat maps and interpolated layers can then be used as inputs to HSMs for identifying the best locations for successful restoration of target species (e.g., Pirrotta et al., 2015; Puckett et al., 2018) or can be overlaid with maps of expected ecosystem services (e.g., Adame et al., 2015) or participatory maps indicating preferred restoration sites (e.g., Rakotomahazo et al., 2019) to assess potential restoration options. The reliability of spatial data layers visualized in GIS will depend on the nature and quality of input datasets and on the degree of gap filling or interpolation required, and it is important to account for potential sources of uncertainty and error, as with any modeling and data synthesis exercise (Caldow et al., 2015).
Habitat Suitability Models
Habitat suitability models, sometimes referred to as environmental niche models and including some types of species distribution models, aim to predict the suitability of a location for a species, based on environmental variables that limit its distribution, by using observed relationships between species occurrences and environmental conditions (Rushton et al., 2004; Elith and Leathwick, 2009; Franklin, 2013; Thuiller and Münkemüller, 2017). These models range in sophistication from correlative (e.g., generalized additive models) and machine learning approaches (e.g., MaxEnt; Linhoss et al., 2016), down to simple index models that ask whether locations meet a set of minimum criteria for the species to thrive. In each case, the models generate maps of suitability based on current or predicted environmental conditions. Thus, besides explaining and inferring current distributions, they can be used to predict how suitable habitat for a species could change under different environmental scenarios, such as with predicted climate change (Heikkinen et al., 2006) or threat reduction. Indeed, HSMs have been used to inform marine ecosystem restoration projects, including for seagrass meadows (e.g., Adams et al., 2016), salt marshes (Heuner et al., 2016), mangrove forests (Petrosian et al., 2016), and oyster reefs (Barnes et al., 2007; Theuerkauf et al., 2016; Chowdhury et al., 2019). The reliability of these models, however, depends on the availability and quality of data on species’ distributions and environmental parameters. Furthermore, in heavily degraded systems, current distributions may not accurately reflect where a species could survive if threats were mitigated as part of the restoration project.
The benefit of using HSMs to inform restoration site selection is that they can preemptively identify locations where restoration is likely to be more (or less) successful before costly projects are implemented. For example, for seagrass meadows, HSMs have been used to select locations where restoring is predicted to be successful (Adams et al., 2016; Hotaling-Hagan et al., 2017; Zhou et al., 2017; Thom et al., 2018). Some studies also validate these predictions by performing in situ transplants that resulted in successful seagrass restoration, suggesting that HSMs are useful decision tools for restoration projects (Pirrotta et al., 2015; Valle et al., 2015). Although simple HSMs can be useful, HSMs can also be combined with physical models (e.g., oceanographic modeling projections) and data on species behavior (e.g., animal movement such as dispersal) to provide more dynamic predictions. With these modifications HSMs have been used to identify candidate locations for restoration in oyster and mussel reefs (Barnes et al., 2007; Pollack et al., 2012; Elsäßer et al., 2013). Furthermore, HSMs can also be used to identify locations for restoration that will enhance the provision of ecosystem services. For example, Theuerkauf et al. (2019) combined a habitat suitability index for oysters with abiotic data (e.g., dissolved oxygen, chlorophyll a, water flow) to identify restoration areas that would maximize the water filtration service provided by oyster reefs, while Zellmer et al. (2019) used stacked-species distribution models to identify sites for artificial reefs that would enhance reef fish distributions and richness and the services they support (e.g., fisheries, recreation).
Ecosystem Service Models
Ecosystem service models are increasingly used to describe potential benefits from nature to inform conservation and spatial planning (Nelson et al., 2009; White et al., 2012; Lester et al., 2013; Arkema et al., 2015). These models capture the linkages between ecosystems and people, and assess how service supply and value could be impacted by proposed management actions, human activities, and environmental degradation. Ecosystem service models can range from simplified approaches based on expert opinion or on the assumption that values documented for that ecosystem in one location apply to other locations (e.g., benefit transfer methods; Brander et al., 2012); to complex spatial models that require more data but can account for multiple sources of spatial variation in both service supply and value to people (e.g., production function models; Chan and Ruckelshaus, 2010; Guerry et al., 2012; Townsend et al., 2018).
The development of ecosystem service models for marine systems has lagged behind models for terrestrial and freshwater systems (Townsend et al., 2018), and thus applications to marine ecosystem restoration are somewhat rare. This is understandable given data limitations for marine systems, the complexity of processes controlling the supply and value of ecosystem services, and the uncertainty associated with predicting potential restoration outcomes. Where poor theoretical understanding or data limitations exist, it may be necessary to rely on more simplified approaches (e.g., benefit transfer), knowing there could be greater spatial variation in service outcomes than is captured by these techniques. In this case, relying on generalized ecosystem service models, global databases, and web interfaces for acquiring model parameters and inputs can be useful (like those offered by the InVEST suite of models; Guerry et al., 2012; Sharp et al., 2016). GIS mapping and spatial analysis (described above) can also generate local datasets that can be used in generalized modeling frameworks (e.g., La Peyre et al., 2015; Gilby et al., 2020).
Despite challenges with modeling marine ecosystem services, the growing number of cases where ecosystem service models have been used in spatial planning for conservation illustrate how they could guide marine ecosystem restoration. For example, Arkema et al. (2013) developed a model to quantify coastal protection services associated with different nearshore habitats in the U.S. to evaluate the potential impact of habitat conservation on various measures of social vulnerability to climate change. Arkema et al. (2015) developed models to quantify ecosystems services associated with coral reefs, mangroves, and seagrasses in Belize and used the outputs along with stakeholder preferences to inform a spatial planning process. Both of these analytical approaches could be expanded to inform restoration site selection and planning. Adame et al. (2015) and Theuerkauf et al. (2019) are good examples of this type of approach, showing how ecosystem service models can inform site selection and spatial planning for restoration of oyster reefs and mangroves, respectively.
Spatial Prioritization Analysis
Spatial prioritization is the process of identifying and selecting the best locations to take action. This is perhaps the most well-developed aspect of the field of conservation planning (Groves and Game, 2016), where it is used to select locations for conservation action, most commonly protection (Ban et al., 2013). Spatial prioritization can be done at a range of complexity levels, from applying simple heuristic rules where sites are ranked according to some objective (e.g., species richness or presence of rare species) to using more sophisticated optimization algorithms or approximations (Moilanen et al., 2009). These algorithms, although themselves varying in complexity, essentially aim to identify a site or collection of sites that achieve a set specified objectives (e.g., protecting some percentage of biodiversity, maximizing habitat representation, or minimizing the total amount of area protected) (Camm et al., 1996). There are a variety of software tools available for this purpose, with the most prominent being Marxan (Ball et al., 2011) and Zonation (Lehtomaki and Moilanen, 2013). A common use of these tools in conservation planning is to identify a site or collection of sites that meet specified targets for various conservation objectives while minimizing “costs.” Costs can be actual financial costs or social costs such as prohibiting human activities in areas, and costs are commonly operationalized as the smallest total amount of area, with the implicit assumption that less area protected will be less objectionable to society (Ban and Klein, 2009). Site selection can also demand certain configuration requirements, such as the minimum size of areas, clustering, or site connectivity (Beger et al., 2010). In cases where prioritization tools select sites to meet multiple criteria (e.g., representation of different habitat types while minimizing costs or area protected), they also fall into the tradeoff analysis category described below as they are selecting a site or collection of sites to minimize tradeoffs.
Many of the same fundamental concepts and tools used to prioritize sites for protection can be used to identify and select locations for restoration. In fact, in many cases, spatial prioritization analyses that select areas that are a high priority for conservation do not specify what type of conservation action should take place in those areas (Groves and Game, 2016), and restoration may be critical when ecosystems have been degraded to the point where protection alone will not lead to recovery. There are a few key considerations when applying prioritization analysis to identifying sites for protection versus restoration. For one, in the case of restoration, sites are prioritized based on where the target conservation feature no longer exists or has been severely degraded, typically because of anthropogenic threats, as opposed to prioritizing sites that are often the least impacted locations (Yoshioka et al., 2014). This increases the importance of either historical data, for example to identify potential restoration sites based on differences between current and past distributions (Yoshioka et al., 2014), or predictive models such as species distribution models and HSMs (described above) that can be used to identify sites where restoration is likely to be successful. Costs may also need to be conceptualized differently, as minimizing the area to be restored may not be an objective. Costs can be assessed as the actual financial cost of the restoration project, the feasibility of the project, the cost of removing threats that might hinder restoration success, and the compatibility of other land or sea uses with the target habitat (Yoshioka et al., 2014). Lastly, because restoration projects are increasingly motivated by a desire to protect or enhance ecosystem services, ecosystem service outcomes will often need to be included among the objectives that are being maximized within the optimization algorithm. Again, this requires predictive models because restoration will provide services in new locations, but, as described above, there are spatial ecosystem service models that can be parameterized using hypothetical habitat distributions, such as the array of InVEST models (Sharp et al., 2016).
While it is less common to apply prioritization analyses explicitly to selecting priority restoration sites, particularly at larger spatial scales (Tobon et al., 2017), useful examples do exist (e.g., Crossman and Bryan, 2006; Adame et al., 2015; Vogler et al., 2015; Singh et al., 2019). Tobon et al. (2017) used a heuristic multi-criteria analysis to rank the priority of terrestrial sites for ecosystem restoration throughout Mexico based on the biological importance of sites, the restoration feasibility, and different weightings of the two objectives. Yoshioka et al. (2014) used Marxan to conduct what is known as a complementarity analysis, which assigns high priority to sites with more unrepresented features (in this case, sites that have lost endangered bird species) balanced by costs (in this case, restoration infeasibility measured as the levels of urbanization and farming), for Japan to identify priority restoration sites to benefit birds. There are also a growing number of examples that focus on ecosystem services as key outcomes. For example, Singh et al. (2019) used an optimization algorithm to prioritize locations for wetland restoration at a regional scale using an ecosystem service model of phosphorous retention and restoration costs. Adame et al. (2015), the only study in our literature review analysis that used spatial prioritization, selected priority areas for mangrove restoration in the Mexican Caribbean using Marxan. In this analysis, the authors examined scenarios where they fixed the percentage of area restored, and prioritized sites based on the expected provisioning of three ecosystem services (carbon storage, water depuration, coastal protection) and minimizing costs (assuming costs increase as a function of ecosystem degradation and inaccessibility). This study nicely demonstrates how spatial prioritization tools from conservation planning can be readily adapted to spatial planning for marine ecosystem restoration.
Tradeoff Analysis
Tradeoff analysis is an analytical tool for evaluating alternatives that could be quite useful in spatial planning and site selection for marine ecosystem restoration, although it has not been widely used in this context. The approach borrows from economic theory and multi-objective decision analysis, and provides an explicit and systematic assessment of tradeoffs, for example, among competing management objectives. In the context of spatial planning, where different planning options will have different outcomes for various management objectives, the goal is to identify which planning options best balance those objectives (i.e., maximize a joint objective) (Lester et al., 2013). Therefore, the outcome for each management objective from each possible spatial plan must be quantified, whether through empirical data, quantitative models, or conceptual models. For example, if the goal of a restoration project is to improve water quality and storm protection, and five candidate sites are under consideration, predictive spatial models of water quality and storm damage can be used to determine the outcome for these two objectives under each of the five restoration scenarios. Often the most intuitive way to assess the possible tradeoffs is to visualize them on a multidimensional graph, where the axes represent the objectives and each point corresponds to the outcomes from each spatial plan or restoration scenario. In some cases, there may be obvious win-win options where all objectives are high, and in other cases there will be clear tradeoffs, providing a starting point for a more explicit discussion about how those tradeoffs should be balanced (Lester et al., 2013).
The most useful application of tradeoff analysis assesses all (or many) of the theoretically possible restoration options. In the simple two objective case, the outer bound of all points (those further up and to the right on the graph) is the “efficiency frontier” comprised of “Pareto optimal options,” to use the economics terminology, whereby one objective cannot be further increased without a loss to the other objective. All options on the frontier represent options that differently prioritize objectives across which there are unavoidable tradeoffs. Which option on the frontier is “best” depends on the relative preference for the objectives among society or decision-makers (Lester et al., 2013). The points interior to the frontier are poor options, assuming all of the important management objectives have been included in the tradeoff analysis, because at least one objective could be improved with no cost to the others. The analysis is easiest to visualize with only two objectives, but the logic applies to any number of dimensions. An analytically defined objective function can explicitly evaluate the response of different management objectives to different possible spatial plans, considering different weighting factors for each objective (as not all objectives may be equally important), and specify spatial plans that are on the frontier (e.g., Lester et al., 2018).
This approach has been applied to marine spatial planning and particularly the challenge of siting new ocean uses (White et al., 2012; Lester et al., 2018), and for evaluating tradeoffs in the context of implementing marine protected areas (Brown et al., 2001; Rassweiler et al., 2014), including using prioritization analyses as described above (e.g., Klein et al., 2013). There are also numerous examples of how this approach can be useful in land use planning for evaluating tradeoffs and identifying scenarios that would increase the provisioning of multiple ecosystem services (Polasky et al., 2008; Nelson et al., 2009; Goldstein et al., 2012), which is analogous to the goal of restoring marine habitats to improve ecosystem service outcomes. While there are some examples of tradeoff analysis being applied in the context of restoration (Zheng and Hobbs, 2013; Vogler et al., 2015; Martin et al., 2016; Singh et al., 2019), or for comparing the effects of different types of management actions including restoration (Needles et al., 2015), research that evaluates different site selection options for marine ecosystem restoration using this approach is rare or nonexistent. We argue this approach could be an important tool in spatial planning for restoration, particularly in making site selection decisions that account for spatial variation in restoration costs, likely restoration success, and ecosystem service outcomes. The major challenge with implementing such an approach is access to fine resolution spatial data and spatially explicit models that predict restoration success and ecosystem service delivery if the ecosystem is restored at different locations across the seascape. Nevertheless, when these data and models are available, tradeoff analysis could be used to improve restoration return on investment.
Conclusion and Ways Forward
Because of the high cost and importance of restoration for marine ecosystems, and the limited resources often available for such efforts, pursuing restoration projects that are effective and efficient is essential. Here we have provided a case for how restoration outcomes, particularly for ecosystem services, could be improved by applying spatial planning principles. Despite this strong rationale, our review of recently published (2015–2019) peer-reviewed papers on restoration for three high profile ecosystems (seagrass beds, salt marshes, and mangrove forests) reveals that site selection and spatial planning are rarely incorporated into restoration research. Those studies that do examine site selection or spatial planning for restoration focus most commonly on environmental conditions required for the restored habitat, and rarely include an explicit analysis of ecosystem service outcomes. However, because we focused on peer reviewed literature and did not include gray literature in our search, our results may not capture the current state of restoration practice and we acknowledge that there could be important differences between activities occurring as part of actual restoration efforts and scholarly research about restoration. Nevertheless, we expect that if spatial planning principles were commonly incorporated into restoration practice, these themes would appear more commonly in the scientific literature. Furthermore, their rarity in the literature highlights a missing avenue for their dissemination and adoption. Therefore, to try to facilitate a new wave of research and practice that fills this gap, we provide a conceptual overview of why and how to apply spatial planning principles to marine ecosystem restoration (Figure 2).
Accounting for spatial variation in expected restoration success, the supply and value of ecosystem services, and the cost of restoration projects has the potential to improve return on investment for restoration. While abundant spatial data exist in many cases, the challenge of connecting these data with decision-making may seem daunting or not worth the effort. We aim to dispel this concern by providing an overview of existing analytical tools available to support restoration site selection and spatial planning. These tools, which include GIS and spatial analysis, habitat suitability models (HSMs), ecosystem service models, spatial prioritization algorithms, and tradeoff analyses, have been applied extensively within conservation planning, and more recently, marine spatial planning. There are also examples of all of these approaches being applied to ecosystem restoration, although the scientific literature suggests that applications to coastal and marine ecosystems may still be rare.
The tools described in this paper can be applied for restoration planning at any spatial scale and resolution, and are limited mainly by the availability and quality of spatial data. Although these tools can be employed individually, multiple tools can also be integrated within a single restoration spatial planning analysis. For example, the output of a HSM that makes spatial predictions for restoration success – and thus can identify candidate areas for restoration – could be used within multiple ecosystem service models that predict outcomes if a habitat was restored in those areas. These ecosystem service predictions could then be visualized in a tradeoff analysis that plots different possible siting options for restoration. Alternatively, the ecosystem service predictions can serve as inputs to a prioritization algorithm that suggests the best sites for restoration.
We expect that as spatial planning approaches and principles are applied more frequently to marine ecosystem restoration, there will be improvements made to these tools that allow them to be more readily applied to future restoration projects. Further, as the field matures, more sophisticated planning approaches that account for climatic and other major directional changes will be imperative. As ecosystems move into states for which there is no historical analog, it will be useful to combine climate projections of environmental conditions (e.g., sea level rise, temperature, pH, etc.) and expected changes to human communities (e.g., population density, food insecurity, coastal vulnerability) with predictive models of habitat suitability and ecosystem services to facilitate planning for climate-robust restoration projects. Fortunately, climate model outputs are increasingly available at appropriate spatial resolutions to be integrated into these types of analyses (e.g., Assis et al., 2018). Looking forward, there could also be benefits of including cross-ecosystem connections into spatial planning analyses given that connectivity among adjacent ecosystems, including nutrient exchange, trophic connections, and impacts on physical conditions, can affect restoration success (Gillis et al., 2017). Many marine species use multiple habitat types, either during different life stages or for feeding and shelter over daily time scales, and therefore restoration should account for these linkages. This will require conducting analyses and making coordinated restoration decisions at landscape scales.
Although application of spatial planning tools has substantial potential to improve the probability of restoration success and resulting outcomes for ecosystem services, there are costs to the planning as well. These include the direct costs of doing the planning (salaries for analysts, costs of acquiring or collecting data, etc.), but also the implicit costs associated with a delay in the start of restoration. If a site selection process adds 6 months to the planning stage, this can be viewed as a loss of the ecosystem services that would otherwise have been provided by an earlier start to restoration activities. Analyses of whether formal site selection should be undertaken are vanishingly rare. While the costs of planning can be estimated, the benefits cannot be known until the analysis is undertaken. As a general rule, benefits are likely to be larger when candidate sites are spread across the landscape with relatively high variation either in environmental or social factors, a circumstance which could result from a large potential planning area, or from high local variation within a smaller area. However, even when variation is modest, the high cost of marine restoration projects means that even a small percentage improvement in outcomes is likely to dwarf the direct financial costs of planning, and if the restored habitat has a long expected lifetime, any delay in implementation should be easily outweighed by the improved long-term outcomes.
Marine ecosystem restoration will only become more important as we attempt to halt the degradation of nature and recover the ecosystem services society relies on. As the human population grows and climate change impacts increase, ecosystem restoration projects will increasingly require large scale and costly interventions. By investing some resources and time in strategic spatial planning, restoration can better recover ecosystem function and benefit human communities. Spatial planning can also provide a useful platform for improved outreach and education about restoration aimed at the general public, policy makers and funders, as spatial planning analyses can make important tradeoffs more explicit and can illustrate the benefits of more investment in restoration.
Author Contributions
SL designed the research with input from all co-authors. AD, SL, and GH compiled and analyzed the data. SL, JM, AR, and GH wrote the manuscript.
Funding
This research was supported by the Gulf Research Program of the National Academies of Sciences, Engineering and Medicine under award number 2000009025 to SL and AR.
Conflict of Interest
The authors declare that the research was conducted in the absence of any commercial or financial relationships that could be construed as a potential conflict of interest.
Acknowledgments
We thank C. Walford for assistance with the literature review. We also thank members of the Rasster lab for their helpful feedback on the design of Figure 2.
Supplementary Material
The Supplementary Material for this article can be found online at: https://www.frontiersin.org/articles/10.3389/fmars.2020.00328/full#supplementary-material
Footnotes
References
Adame, M. F., Hermoso, V., Perhans, K., Lovelock, C. E., and Herrera-Silveira, J. A. (2015). Selecting cost-effective areas for restoration of ecosystem services. Conserv. Biol. 29, 493–502. doi: 10.1111/cobi.12391
Adams, M. P., Saunders, M. I., Maxwell, P. S., Tuazon, D., Roelfsema, C. M., Callaghan, D. P., et al. (2016). Prioritizing localized management actions for seagrass conservation and restoration using a species distribution model. Aquat. Conserv. Mar. Freshw. Ecosyst. 26, 639–659.
Adams, M. P., Ghisalberti, M., Lowe, R. J., Callaghan, D. P., Baird, M. E., Infantes, E., et al. (2018). Water residence time controls the feedback between seagrass, sediment and light: implications for restoration. Adv. Water Res. 117, 14–26. doi: 10.1016/j.advwatres.2018.04.004
Allan, J. D., McIntyre, P. B., Smith, S. D. P., Halpern, B. S., Boyer, G. L., Buchsbaum, A., et al. (2013). Joint analysis of stressors and ecosystem services to enhance restoration effectiveness. Proc. Natl. Acad. Sci. U.S.A. 110, 372–377. doi: 10.1073/pnas.1213841110
Arkema, K. K., Guannel, G., Verutes, G., Wood, S. A., Guerry, A., Ruckelshaus, M., et al. (2013). Coastal habitats shield people and property from sea-level rise and storms. Nat. Clim. Change 3, 913–918.
Arkema, K. K., Verutes, G. M., Wood, S. A., Clarke-Samuels, C., Rosado, S., Canto, M., et al. (2015). Embedding ecosystem services in coastal planning leads to better outcomes for people and nature. Proc. Natl. Acad. Sci.U.S.A. 112, 7390–7395. doi: 10.1073/pnas.1406483112
Assis, J., Tyberghein, L., Bosch, S., Verbruggen, H., Serrão, E. A., and De Clerck, O. (2018). Bio-ORACLE v2.0: extending marine data layers for bioclimatic modelling. Glob. Ecol. Biogeogr. 27, 277–284.
Ball, I. R., Possingham, H. P., and Watts, M. (2011). “Marxan and relatives: software for spatial conservation prioritization,” in Spatial Conservation Prioritisation: Quantitative Methods and Computational Tools, eds A. Moilanen, K. A. Wilson, and H. P. Possingham (Oxford: Oxford University Press), 185–195.
Ban, N., Januchowski-Hartley, S., Álvarez-Romero, J., Mills, M., Pressey, R., Linke, S., et al. (2013). “Marine and freshwater conservation planning: from representation to persistence,” in Conservation Planning: Shaping the Future, eds L. Craighead and C. Convis (Redlands, CA: ESRI Press), 175–218.
Ban, N. C., and Klein, C. J. (2009). Spatial socioeconomic data as a cost in systematic marine conservation planning. Conserv. Lett. 2, 206–215. doi: 10.1371/journal.pone.0164869
Barbier, E. B., Hacker, S. D., Kennedy, C., Koch, E. W., Stier, A. C., and Silliman, B. R. (2011). The value of estuarine and coastal ecosystem services. Ecol. Monogr. 81, 169–193.
Barnes, T. K., Volety, A. K., Chartier, K., Mazzotti, F. J., Pearlstine, L., Blvd, N. C., et al. (2007). A habitat suitability index model for the eastern oyster (Crassostrea virginica), a tool for restoration of the Caloosahatchee estuary. Florida 26, 949–959.
Bayraktarov, E., Saunders, M. I., Abdullah, S., Mills, M., Beher, J., Possingham, H. P., et al. (2016). The cost and feasibility of marine coastal restoration. Ecol. Appl. 26, 1055–1074. doi: 10.1890/15-1077
Beger, M., Linke, S., Watts, M., Game, E., Treml, E., Ball, I., et al. (2010). Incorporating asymmetric connectivity into spatial decision making for conservation. Conserv. Lett. 3, 359–368.
Benayas, J. M. R., Newton, A. C., Diaz, A., and Bullock, J. M. (2009). Enhancement of biodiversity and ecosystem services by ecological restoration: a meta-analysis. Science 325, 1121–1124. doi: 10.1126/science.1172460
Bonn, A., Reed, M. S., Evans, C. D., Joosten, H., Bain, C., Farmer, J., et al. (2014). Investing in nature: developing ecosystem service markets for peatland restoration. Ecosyst. Serv. 9, 54–65.
Braje, T. J., Rick, T. C., Erlandson, J. M., Rogers-Bennett, L., and Catton, C. A. (2016). Historical ecology can inform restoration site selection: the case of black abalone (Haliotis cracherodii) along California’s Channel Islands. Aquat. Conserv. Mar. Freshw. Ecosyst. 26, 470–481.
Brander, L. M., Wagtendonk, A. J., Hussain, S. S., McVittie, A., Verburg, P. H., de Groot, R. S., et al. (2012). Ecosystem service values for mangroves in Southeast Asia: a meta-analysis and value transfer application. Ecosyst. Serv. 1, 62–69.
Brown, G., Strickland-Munro, J., Kobryn, H., and Moore, S. A. (2016). Stakeholder analysis for marine conservation planning using public participation GIS. Appl. Geogr. 67, 77–93.
Brown, K., Adger, W. N., Tompkins, E., Bacon, P., Shim, D., and Young, K. (2001). Trade-off analysis for marine protected area management. Ecol. Econ. 37, 417–434. doi: 10.1371/journal.pone.0118764
Bullock, J. M., Aronson, J., Newton, A. C., Pywell, R. F., and Rey-Benayas, J. M. (2011). Restoration of ecosystem services and biodiversity: conflicts and opportunities. Trends Ecol. Evol. 26, 541–549. doi: 10.1016/j.tree.2011.06.011
Caldow, C., Monaco, M. E., Pittman, S. J., Kendall, M. S., Goedeke, T. L., Menza, C., et al. (2015). Biogeographic assessments: a framework for information synthesis in marine spatial planning. Mar. Policy 51, 423–432.
Camm, J. D., Polasky, S., Solow, A., and Csuti, B. (1996). A note on optimal algorithms for reserve site selection. Biol. Conserv. 78, 353–355.
CBD (2010). Aichi Targets: Secretariat of the Convention on Biological Diversity. COP 10 Outcomes. Rio de Janeiro: Convention on Biological Diversity [CBD].
CBD (2020). Zero Draft of the Post-2020 Global Biodiversity Framework. Rio de Janeiro: Convention on Biological Diversity [CBD].
Chan, K. M. A., and Ruckelshaus, M. (2010). Characterizing changes in marine ecosystem services. F1000 Biol. Rep. 2:54. doi: 10.3410/B2-54
Chowdhury, M. S. N., Wijsman, J. W. M., Hossain, M. S., Ysebaert, T., and Smaal, A. C. (2019). A verified habitat suitability model for the intertidal rock oyster, Saccostrea cucullata. PLoS One 14:e0217688. doi: 10.1371/journal.pone.0217688
Crossman, N. D., and Bryan, B. A. (2006). Systematic landscape restoration using integer programming. Biol. Conserv. 128, 369–383.
Cullen-Unsworth, L. C., and Unsworth, R. (2018). A call for seagrass protection. Science 361, 446–448. doi: 10.1126/science.aat7318
De Groot, R. S., Blignaut, J., Van Der Ploeg, S., Aronson, J., Elmqvist, T., and Farley, J. (2013). Benefits of investing in ecosystem restoration. Conserv. Biol. 27, 1286–1293. doi: 10.1111/cobi.12158
Elith, J., and Leathwick, J. R. (2009). Species distribution models: ecological explanation and prediction across space and time. Annu. Rev. Ecol. Evol. Syst. 40, 677–697.
Elsäßer, B., Fariñas-franco, J. M., David, C., Kregting, L., and Roberts, D. (2013). Identifying optimal sites for natural recovery and restoration of impacted biogenic habitats in a special area of conservation using hydrodynamic and habitat suitability modelling. J. Sea Res. 77, 11–21.
Fonseca, M. S. (2011). Addy revisited: what has changed with seagrass restoration in 64 years? Ecol. Restor. 29, 73–81.
Franklin, J. (2013). Species distribution models in conservation biogeography: developments and challenges. J. Conserv. Biogeogr. 19, 1217–1223.
Gilby, B., Olds, A. D., Connolly, R. M., Henderson, C. J., and Schlacher, T. A. (2018). Spatial restoration ecology: placing restoration in a landscape context. Bioscience 68, 1007–1019.
Gilby, B. L., Olds, A. D., Duncan, C. K., Ortodossi, N. L., Henderson, C. J., and Schlacher, T. A. (2020). Identifying restoration hotspots that deliver multiple ecological benefits. Restor. Ecol. 28, 222–232.
Gillis, L. G., Jones, C. G., Ziegler, A. D., van der Wal, D., Breckwoldt, A., and Bouma, T. J. (2017). Opportunities for protecting and restoring tropical coastal ecosystems by utilizing a physical connectivity approach. Front. Mar. Sci. 4:374. doi: 10.3389/fmars.2017.00374
Gimpel, A., Stelzenmüller, V., Grote, B., Buck, B. H., Floeter, J., Núñez-Riboni, I., et al. (2015). A GIS modelling framework to evaluate marine spatial planning scenarios: co-location of offshore wind farms and aquaculture in the German EEZ. Mar. Policy 55, 102–115.
Gittman, R. K., Fodrie, F. J., Baillie, C. J., Brodeur, M. C., Currin, C. A., Keller, D. A., et al. (2018). Living on the edge: increasing patch size enhances the resilience and community development of a restored salt marsh. Estuaries Coast. 41, 884–895. doi: 10.1007/s12237-017-0302-6
Goldstein, J. H., Caldarone, G., Duarte, T. K., Ennaanay, D., Hannahs, N., Mendoza, G., et al. (2012). Integrating ecosystem-service tradeoffs into land-use decisions. Proc. Natl. Acad. Sci. U.S.A. 109, 7565–7570. doi: 10.1073/pnas.1201040109
Gómez-Aparicio, L. (2009). The role of plant interactions in the restoration of degraded ecosystems: a meta-analysis across life-forms and ecosystems. J. Ecol. 97, 1202–1214.
Groves, C., and Game, E. T. (2016). Conservation Planning: Informed Decisions for a Healthier Planet. Greenwood Village, CO: Roberts and Company Publishers.
Guerry, A. D., Ruckelshaus, M. H., Arkema, K. K., Bernhardt, J. R., Guannel, G., Kim, C.-K., et al. (2012). Modeling benefits from nature: using ecosystem services to inform coastal and marine spatial planning. Int. J. Biodivers. Sci. Ecosyst. Serv. Manag. 8, 107–121.
Hagger, V., Dwyer, J., and Wilson, K. (2017). What motivates ecological restoration? Restor. Ecol. 25, 832–843.
Heikkinen, R. K., Luoto, M., Araújo, M. B., Virkkala, R., Thuiller, W., and Martin, T. (2006). Methods and uncertainties in bioclimatic envelope modelling under climate change. Prog. Phys. Geogr. 6, 751–777.
Hejnowicz, A. P., Kennedy, H., Rudd, M. A., and Huxham, M. R. (2015). Harnessing the climate mitigation, conservation and poverty alleviation potential of seagrasses: prospects for developing blue carbon initiatives and payment for ecosystem service programmes. Front. Mar. Sci. 2:32. doi: 10.3389/fmars.2015.00032
Heuner, M., Weber, A., Schroeder, U., Kleinschmit, B., and Schroeder, B. (2016). Facilitating political decisions using species distribution models to assess restoration measures in heavily modified estuaries. Mar. Pollut. Bull. 110, 250–260. doi: 10.1016/j.marpolbul.2016.06.056
Hogg, O. T., Huvenne, V. A. I., Griffiths, H. J., and Linse, K. (2018). On the ecological relevance of landscape mapping and its application in the spatial planning of very large marine protected areas. Sci. Total Environ. 626, 384–398. doi: 10.1016/j.scitotenv.2018.01.009
Hotaling-Hagan, A., Swett, R., Ellis, L. R., and Frazer, T. K. (2017). A spatial model to improve site selection for seagrass restoration in shallow boating environments. J. Environ. Manag. 186, 42–54. doi: 10.1016/j.jenvman.2016.10.005
Howe, E., and Simenstad, C. A. (2015). Using isotopic measures of connectivity and ecosystem capacity to compare restoring and natural marshes in the Skokomish River Estuary, WA, USA. Estuar. Coasts 38, 639–658.
Hughes, A. R., Grabowski, J. H., Leslie, H. M., Scyphers, S., and Williams, S. L. (2018). Inclusion of biodiversity in habitat restoration policy to facilitate ecosystem recovery. Conserv. Lett. 11:e12419.
Iftekhar, M. S., Polyakov, M., Ansell, D., Gibson, F., and Kay, G. M. (2017). How economics can further the success of ecological restoration. Conserv. Biol. 31, 261–268. doi: 10.1111/cobi.12778
IPBES (2019). Global Assessment Report on Biodiversity and Ecosystem Services of the Intergovernmental Science-Policy Platform on Biodiversity and Ecosystem Services. Bonn: IPBES Secretariat.
Jahnke, M., Jonsson, P. R., Moksnes, P.-O., Loo, L.-O., Nilsson Jacobi, M., and Olsen, J. L. (2018). Seascape genetics and biophysical connectivity modelling support conservation of the seagrass Zostera marina in the Skagerrak–Kattegat region of the eastern North Sea. Evol. Appl. 11, 645–661. doi: 10.1111/eva.12589
Kittinger, J. N., Bambico, T. M., Minton, D., Miller, A., Mejia, M., Kalei, N., et al. (2016). Restoring ecosystems, restoring community: socioeconomic and cultural dimensions of a community-based coral reef restoration project. Reg. Environ. Change 16, 301–313.
Klein, C. J., Tulloch, V. J., Halpern, B. S., Selkoe, K. A., Watts, M. E., Steinback, C., et al. (2013). Tradeoffs in marine reserve design: habitat condition, representation, and socioeconomic costs. Conserv. Lett. 6, 324–332.
Koch, E. W., Barbier, E. B., Silliman, B. R., Reed, D. J., Perillo, G. M. E., Hacker, S. D., et al. (2009). Non-linearity in ecosystem services: temporal and spatial variability in coastal protection. Front. Ecol. Environ. 7:126. doi: 10.1890/080126
Kodikara, K. A. S., Mukherjee, N., Jayatissa, L. P., Dahdouh-Guebas, F., and Koedam, N. (2017). Have mangrove restoration projects worked? An in-depth study in Sri Lanka. Restor. Ecol. 25, 705–716. doi: 10.1016/j.jenvman.2015.04.018
La Peyre, M. K., Serra, K., Joyner, T. A., and Humphries, A. (2015). Assessing shoreline exposure and oyster habitat suitability maximizes potential success for sustainable shoreline protection using restored oyster reefs. PeerJ 3:e1317. doi: 10.7717/peerj.1317
Lehtomaki, J., and Moilanen, A. (2013). Methods and workflow for spatial conservation prioritization using Zonation. Environ. Model. Softw. 47, 128–137.
Lester, S. E., Costello, C., Halpern, B. S., Gaines, S. D., White, C., and Barth, J. A. (2013). Evaluating tradeoffs among ecosystem services to inform marine spatial planning. Mar. Policy 38, 80–89.
Lester, S. E., Stevens, J. M., Gentry, R., Kappel, C. V., Bell, T. W., Costello, C., et al. (2018). Marine spatial planning makes room for offshore aquaculture in crowded coastal waters. Nat. Commun. 9:945. doi: 10.1038/s41467-018-03249-1
Linhoss, A. C., Camacho, R., and Ashby, S. (2016). Oyster habitat suitability in the Northern Gulf of Mexico. J. Shellfish Res. 35, 841–849.
Macreadie, P. I., Ollivier, Q. R., Kelleway, J. J., Serrano, O., Carnell, P. E., Ewers Lewis, C. J., et al. (2017). Carbon sequestration by Australian tidal marshes. Sci. Rep. 7:44071. doi: 10.1038/srep44071
Martin, D. M., Hermoso, V., Pantus, F., Olley, J., Linke, S., and Poff, N. L. (2016). A proposed framework to systematically design and objectively evaluate non-dominated restoration tradeoffs for watershed planning and management. Ecol. Econ. 127, 146–155.
Matzek, V. (2018). Turning delivery of ecosystem services into a deliverable of ecosystem restoration. Restor. Ecol. 26, 1013–1016.
Matzek, V., Puleston, C., and Gunn, J. (2015). Can carbon credits fund riparian forest restoration? Restor. Ecol. 23, 7–14.
Moilanen, A., Wilson, K. A., and Possingham, H. P. (2009). Spatial Conservation Prioritization: Quantitative Methods and Computational Tools. Oxford: Oxford University Press.
Needles, L. A., Lester, S. E., Ambrose, R., Andren, A., Beyeler, M., Connor, M. S., et al. (2015). Managing bay and estuarine ecosystems for multiple services. Estuar. Coasts 38, S35–S48.
Nelson, E., Mendoza, G., Regetz, J., Polasky, S., Tallis, H., Cameron, D., et al. (2009). Modeling multiple ecosystem services, biodiversity conservation, commodity production, and tradeoffs at landscape scales. Front. Ecol. Environ. 7:23. doi: 10.1890/080023
Olson, A. M., Hessing-Lewis, M., Haggarty, D., and Juanes, F. (2019). Nearshore seascape connectivity enhances seagrass meadow nursery function. Ecol. Appl. 29:e01897. doi: 10.1002/eap.1897
Pascal, N., Allenbach, M., Brathwaite, A., Burke, L., Le Port, G., and Clua, E. (2016). Economic valuation of coral reef ecosystem service of coastal protection: a pragmatic approach. Ecosyst. Serv. 21, 72–80.
Petrosian, H., Kar, A. D., Ashrafi, S., and Feghhi, J. (2016). Investigating environmental factors for locating mangrove ex-situ conservation zones using GIS spatial techniques and the logistic regression algorithm in mangrove forests in Iran. Polish J. Environ. Stud. 25, 2097–2106.
Pirrotta, M., Tomasello, A., Scannavino, A., Di Maida, G., Luzzu, F., Bellissimo, G., et al. (2015). Transplantation assessment of degraded Posidonia oceanica habitats: site selection and long-term monitoring. Mediterr. Mar. Sci. 16, 591–604.
Polasky, S., Nelson, E., Camm, J., Csuti, B., Fackler, P., Lonsdorf, E., et al. (2008). Where to put things? Spatial land management to sustain biodiversity and economic returns. Biol. Conserv. 141, 1505–1524.
Pollack, J. B., Cleveland, A., Palmer, T. A., Reisinger, A. S., and Montagna, P. A. (2012). A restoration suitability index model for the eastern oyster (Crassostrea virginica) in the mission-aransas Estuary, TX, USA. PLoS One 7:e40839. doi: 10.1371/journal.pone.0040839
Puckett, B. J., Theuerkauf, S. J., Eggleston, D. B., Guajardo, R., Hardy, C., Gao, J., et al. (2018). Integrating larval dispersal, permitting, and logistical factors within a validated habitat suitability index for oyster restoration. Front. Mar. Sci. 5:76. doi: 10.3389/fmars.2018.00076
Rakotomahazo, C., Ravaoarinorotsihoarana, L. A., Randrianandrasaziky, D., Glass, L., Gough, C., Todinanahary, G. G. B., et al. (2019). Participatory planning of a community-based payments for ecosystem services initiative in Madagascar’s mangroves. Ocean Coast. Manag. 175, 43–52.
Rassweiler, A., Costello, C., Hilborn, R., and Siegel, D. A. (2014). Integrating scientific guidance into marine spatial planning. Proc. R. Soc. B Biol. Sci. 281:20132252. doi: 10.1098/rspb.2013.2252
Roelfsema, C., Kovacs, E., Ortiz, J. C., Wolff, N. H., Callaghan, D., Wettle, M., et al. (2018). Coral reef habitat mapping: a combination of object-based image analysis and ecological modelling. Rem. Sens. Environ. 208, 27–41.
Romañach, S. S., DeAngelis, D. L., Koh, H. L., Li, Y., Teh, S. Y., Raja Barizan, R. S., et al. (2018). Conservation and restoration of mangroves: global status, perspectives, and prognosis. Ocean Coast. Manag. 154, 72–82.
Rushton, S. P., Merod, S. J. O. R., and Kerby, G. (2004). New paradigms for modelling species distributions? J. Appl. Ecol. 41, 193–200. doi: 10.1371/journal.pone.0029232
Sharp, R., Tallis, H., Ricketts, T., Guerry, A., Wood, S., Chaplin-Kramer, R., et al. (2016). InVEST 3.7.0 User’s Guide. The Natural Capital Project. Stanford, CA: Stanford University.
Silliman, B. R., Schrack, E., He, Q., Cope, R., Santoni, A., van der Heide, T., et al. (2015). Facilitation shifts paradigms and can amplify coastal restoration efforts. Proc. Natl. Acad. Sci. U.S.A. 112, 14295–14300. doi: 10.1073/pnas.1515297112
Singh, N. K., Gourevitch, J. D., Wemple, B. C., Watson, K. B., Rizzo, D. M., Polasky, S., et al. (2019). Optimizing wetland restoration to improve water quality at a regional scale. Environ. Res. Lett. 14:064006.
Sloey, T. M., Howard, R. J., and Hester, M. W. (2016). Response of Schoenoplectus acutus and Schoenoplectus californicus at different life-history stages to hydrologic regime. Wetlands 36, 37–46.
Stamoulis, K. A., and Delevaux, J. M. S. (2015). Data requirements and tools to operationalize marine spatial planning in the United States. Ocean Coast. Manag. 116, 214–223.
St-Pierre, A. P., and Gagnon, P. (2020). Kelp-bed dynamics across scales: enhancing mapping capability with remote sensing and GIS. J. Exp. Mar. Biol. Ecol. 522, 151246.
Tallis, H., Kareiva, P., Marvier, M., and Chang, A. (2008). An ecosystem services framework to support both practical conservation and economic development. Proc. Natl. Acad. Sci. U.S.A. 105, 9457–9464. doi: 10.1073/pnas.0705797105
Tallis, H., Lester, S. E., Ruckelshaus, M., Plummer, M., McLeod, K. L., Guerry, A., et al. (2011). New metrics for managing and sustaining the ocean’s bounty. Mar. Policy 36, 303–306.
Taylor, M. D., Fry, B., Becker, A., and Moltschaniwskyj, N. (2017). Recruitment and connectivity influence the role of seagrass as a penaeid nursery habitat in a wave dominated estuary. Sci. Total Environ. 584-585, 622–630. doi: 10.1016/j.scitotenv.2017.01.087
Theuerkauf, S. J., Eggleston, D. B., and Puckett, B. J. (2019). Integrating ecosystem services considerations within a GIS-based habitat suitability index for oyster restoration. PLoS One 14:e0210936. doi: 10.1371/journal.pone.0210936
Theuerkauf, S. J., Lipcius, R. N., and Fischer, A. M. (2016). Quantitative validation of a habitat suitability index for oyster restoration. Front. Mar. Sci. 3:64. doi: 10.3389/fmars.2016.00064
Thom, R., Gaeckle, J., Buenau, K., Borde, A., Vavrinec, J., Aston, L., et al. (2018). Eelgrass (Zostera marina L.) restoration in Puget Sound: development of a site suitability assessment process. Restor. Ecol. 26, 1066–1074. doi: 10.1111/rec.12702
Thuiller, W., and Münkemüller, T. (2017). “Habitat suitability modelling,” in Habitat Suitability and Distribution Models: With Applications in R, eds A. Guisan, W. Thuiller, and T. Münkemüller (Cambridge: Cambridge University Press), 513.
Tobon, W., Urquiza-Haas, T., Koleff, P., Schroter, M., Ortega-Alvarez, R., Campo, J., et al. (2017). Restoration planning to guide Aichi targets in a megadiverse country. Conserv. Biol. 31, 1086–1097. doi: 10.1111/cobi.12918
Townsend, M., Davies, K., Hanley, N., Hewitt, J. E., Lundquist, C. J., and Lohrer, A. M. (2018). The challenge of implementing the marine ecosystem service concept. Front. Mar. Sci. 5:359.
Triest, L., Hasan, S., Mitro, P. R., De Ryck, D. J. R., and Van der Stocken, T. (2018). Geographical distance and large rivers shape genetic structure of Avicennia officinalis in the highly dynamic Sundarbans Mangrove Forest and Ganges Delta Region. Estuar. Coasts 41, 908–920.
UNGA (2015). Transforming Our World: The 2030 Agenda for Sustainable Development, A/RES/70/1. New York, NY: United Nations General Assembly [UNGA].
Valiela, I., Bowen, J. L., and York, J. K. (2001). Mangrove forests: one of the world’s threatened major tropical environments. Bioscience 51, 807–815.
Valle, M., Garmendia, J. M., Chust, G., Franco, J., and Borja, A. (2015). Increasing the chance of a successful restoration of Zostera noltii meadows. Aquat. Bot. 127, 12–19.
van Katwijk, M. M., Thorhaug, A., Marbà, N., Orth, R. J., Duarte, C. M., Kendrick, G. A., et al. (2016). Global analysis of seagrass restoration: the importance of large-scale planting. J. Appl. Ecol. 53, 567–578.
Vogler, K. C., Ager, A. A., Day, M. A., Jennings, M., and Bailey, J. D. (2015). Prioritization of forest restoration projects: tradeoffs between wildfire protection, ecological restoration and economic objectives. Forests 6, 4403–4420. doi: 10.1016/j.jenvman.2016.01.033
Waycott, M., Duarte, C. M., Carruthers, T. J. B., Orth, R. J., Dennison, W. C., Olyarnik, S., et al. (2009). Accelerating loss of seagrasses across the globe threatens coastal ecosystems. Proc. Natl. Acad. Sci. U.S.A. 106, 12377–12381. doi: 10.1073/pnas.0905620106
Wegscheidl, C. J., Sheaves, M. I, McLeod, M., Hedge, P. T., Gillies, C. L., and Creighton, C. (2017). Sustainable management of Australia’s coastal seascapes: a case for collecting and communicating quantitative evidence to inform decision-making. Wetl. Ecol. Manag. 25, 3–22.
White, C., Halpern, B. S., and Kappel, C. V. (2012). Ecosystem service tradeoff analysis reveals the value of marine spatial planning for multiple ocean uses. Proc. Natl. Acad. Sci. U.S.A. 109, 4696–4701. doi: 10.1073/pnas.1114215109
Xu, S., Zhou, Y., Wang, P., Wang, F., Zhang, X., and Gu, R. (2016). Salinity and temperature significantly influence seed germination, seedling establishment, and seedling growth of eelgrass Zostera marina L. PeerJ 4:e2697. doi: 10.7717/peerj.2697
Yoshioka, A., Akasaka, M., and Kadoya, T. (2014). Spatial prioritization for biodiversity restoration: a simple framework referencing past species distributions. Restor. Ecol. 22, 185–195.
Zellmer, A. J., Claisse, J. T., Williams, C. M., Schwab, S., and Pondella, D. J. (2019). Predicting optimal sites for ecosystem restoration using stacked-species distribution modeling. Front. Mar. Sci. 6:3. doi: 10.3389/fmars.2019.00003
Zheng, P. Q., and Hobbs, B. F. (2013). Multiobjective portfolio analysis of dam removals addressing dam safety, fish populations, and cost. J. Water Res. Plan. Manag. 139, 65–75.
Keywords: restoration, spatial planning, site selection, conservation planning, spatial prioritization, seagrass, mangrove, salt marsh
Citation: Lester SE, Dubel AK, Hernán G, McHenry J and Rassweiler A (2020) Spatial Planning Principles for Marine Ecosystem Restoration. Front. Mar. Sci. 7:328. doi: 10.3389/fmars.2020.00328
Received: 13 February 2020; Accepted: 21 April 2020;
Published: 20 May 2020.
Edited by:
Brian Silliman, Duke University, United StatesReviewed by:
Carolyn J. Lundquist, National Institute of Water and Atmospheric Research (NIWA), New ZealandKristian Metcalfe, University of Exeter, United Kingdom
Copyright © 2020 Lester, Dubel, Hernán, McHenry and Rassweiler. This is an open-access article distributed under the terms of the Creative Commons Attribution License (CC BY). The use, distribution or reproduction in other forums is permitted, provided the original author(s) and the copyright owner(s) are credited and that the original publication in this journal is cited, in accordance with accepted academic practice. No use, distribution or reproduction is permitted which does not comply with these terms.
*Correspondence: Sarah E. Lester, slester@fsu.edu