- 1National Marine Science Centre, Southern Cross University, Coffs Harbour, NSW, Australia
- 2Commonwealth Scientific and Industrial Research Organisation, Dutton Park, QLD, Australia
- 3New South Wales Department of Primary Industries, National Marine Science Centre, Coffs Harbour, NSW, Australia
Many large coastal sharks are vulnerable to population declines, however, conflict with human activities often results in unselective culls. Successfully and non-destructively, addressing human-wildlife conflicts requires understanding of animal behavior. However, knowledge about white shark (Carcharodon carcharias) behavior near surf zones, where shark bites usually occur, is still limited. We used drones to obtain high-resolution tracks of 108 white sharks directly off coastal beaches on the east coast of Australia. White sharks ranged from 1.9 to 4.0 m (total length) and typically swam parallel to the beach line at an average speed of 0.82 m s−1 (∼3.0 km h−1), with behavior being characteristic of energy conserving motion and foraging. Notably the presence of schools of fish increased mean swim speeds of sharks by 0.33 m s−1 and caused tracks to be more convoluted. White sharks were also observed to swim straighter and faster with time either side of midday, increasing predicted mean swim speeds by 0.11 m s−1, and net travel velocities by 0.25 m s−1. White sharks also displayed inquisitive behavior toward various potential food and non-food items. We demonstrate that white sharks display largely predictable track trajectories and slow movement speeds along coastal beaches, which has utility in further developing non-destructive shark mitigation strategies. Whilst some of the behavior observed might support the exploratory bite hypothesis, there is likely an increased risk of a shark bite to bathers during situations where there are large shark-attracting food sources present.
Introduction
The importance of large sharks for exerting predation influence across marine ecosystems is increasingly recognized (Ferretti et al., 2010; Hussey et al., 2014). However, many are undergoing severe population declines from direct and indirect human exploitation (Dulvy et al., 2014; Roff et al., 2018). Amongst the most vulnerable are large coastal sharks, with many being protected or assigned a conservation status with the International Union for the Conservation of Nature (IUCN; Dulvy et al., 2014; Davidson et al., 2016). However, despite global conservation concerns, the potential for unprovoked shark bites, or “shark attacks,” presents a human-wildlife conflict dilemma that often jeopardizes conservation efforts (Reid et al., 2011; Roff et al., 2018). The occurrence of shark bites often results in reactionary cull-based strategies, which can be largescale, unselective, and long lasting (Cressey, 2013; Roff et al., 2018). Furthermore, common forms of cull-based mitigation can often cause negative consequences for other threatened marine wildlife caught as bycatch (Sumpton et al., 2011).
In addition to ecological considerations, there is mounting social pressure for management agencies to deal with the social and safety issues of shark bites in a non-destructive way (Pepin-Neff and Wynter, 2017; Simmons and Mehmet, 2018). However, to successfully address human-wildlife conflict involving a large predator requires a detailed understanding of the animal’s behavior, which is reflected by the increase of animal movement research (McGowan et al., 2017; Fraser et al., 2018; Suraci et al., 2019). Additionally, increasing the knowledge base on feared animals is also critical for addressing misconceptions and improving social attitudes to support non-destructive management approaches (Dickman, 2010; Draheim et al., 2015).
White sharks (Carcharodon carcharias) are involved in the majority of reported global shark bites and are arguably the most feared ocean predators (Taylor et al., 2019). Although they are susceptible to human-driven population declines and consequently being listed as “vulnerable” on the IUCN red list of threatened species, they are often the driver for cull-based shark mitigation (e.g., Cressey, 2013). Despite tagging and tracking efforts, there is a conceptual understanding of long-range movement patterns of white sharks and their propensity to reside in continental shelf waters (Skomal et al., 2017; Bruce et al., 2019; Watanabe et al., 2019), but little is known about their localized behavior when nearing the surf zone, where unprovoked shark bites are known to occur. Such small-scale movement information has been critical in curbing human-wildlife conflict in terrestrial systems (e.g., McGowan et al., 2017; Suraci et al., 2019).
To capture localized behavior of white sharks, we used drones to obtain fine-scale movement patterns along coastal beaches on the east coast of Australia to determine their movement patterns around the surf zone and their common size ranges. We further quantify track metrics of direction, average (instantaneous) speed, straightness, and net velocity across shark vector (a function of net distance over duration), as well as the potential influence of biological and environmental parameters on track metrics. We illustrate the largely predictable nature of white shark movement patterns at fine scales along coastal beaches.
Methods
Sampling Location and Tracking Procedures
We used <2 kg multirotor drones (DJI Phantom 4) to obtain movement tracks of 108 white sharks off coastal beaches of eastern Australia from September 2016 to October 2018. While over 30 beaches between Brunswick Heads and Anna Bay in New South Wales (NSW) were haphazardly sampled for white sharks, we only encountered them at 10 beaches (Figure 1). To obtain a statistically meaningful quantity of white shark tracks, locations targeted for sampling were a mix of non-probability convenience sampling and haphazardly selecting underrepresented locations along the coastline, whereby around 650–700 targeted drone flights were made. White shark catch and detection information from other shark management strategies were also used as a guide to indicate shark presence, such as from SMART drumlines, VR4G acoustic receivers and aerial surveillance patrols along the NSW coastline. We also used additional opportunistic tracks of two white sharks from drone-based shark surveillance trials operating at Ballina and Evans Head (Colefax et al., 2019; Kelaher et al., 2020a). The majority of targeted search effort and subsequent collected data was from July to October months, coinciding with broad seasonal migrations (Bruce et al., 2019). Due to the nature of sampling, search effort was not recorded as it was not necessarily representative of shark abundance.
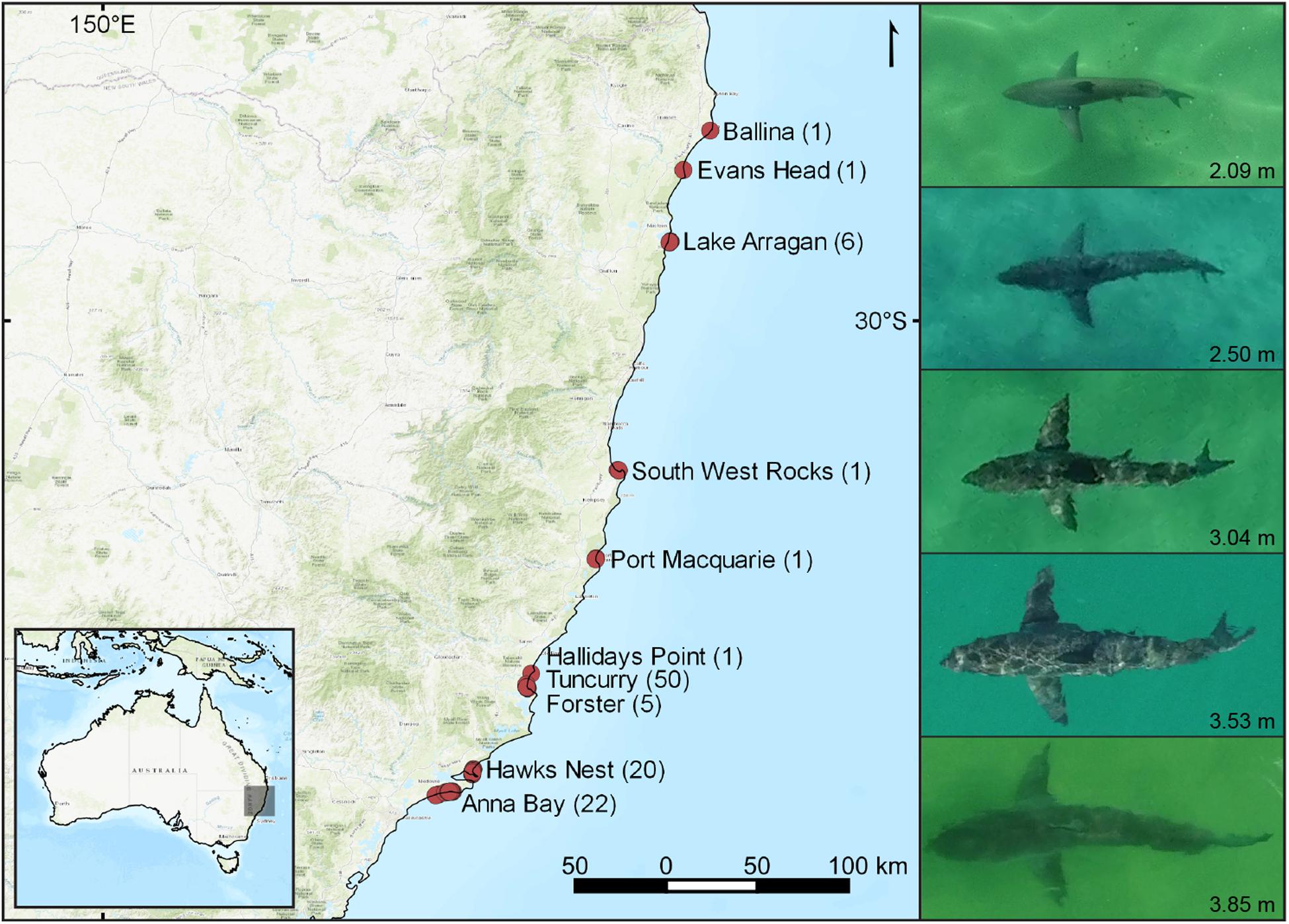
Figure 1. Locations off eastern Australia, where localized movement tracks of white sharks (Carcharodon carcharias) were obtained. Numbers of white sharks tracked at each location are given in parenthesis. The righthand panel shows a scaled comparison of the approximate range of lengths of white sharks observed during this study.
At each beach, the area within and behind the surf break was searched with the drone. Once a white shark was detected, the drone was lowered to 20–25 m for tracking, with the camera pitched at 90°, or nadir (see online Supplementary Material for more information). Smooth course corrections were made so that the shark was maintained in the centre of the screen (marked by digital crosshairs) and the same heading as the aircraft for the entire tracking duration. Video was recorded in UHD 4k resolution (3840 × 2160 pixels) along with active subtitles of time, GPS and altitude information. A track ended when the shark was lost in deep or highly turbid water, traveled beyond regulatory drone “line-of-sight” requirements, or lost between drone battery changes. With each flight, the wind direction and strength were recorded using a Skywatch BL portable weather station. Wind strengths during tracking ranged between 0–11.78 m s−1 with a mean (±SD) of 2.76 m s−1 (±2.05). Tidal data was obtained from the Australian Bureau of Meteorology tide prediction charts relevant to the sampling location, and was later categorized from 1–6 per the stage of the tide. Water clarity index was assessed on a scale from 1–5 with increasing clarity (see Colefax et al., 2019). The median water clarity across all shark tracks was 3.5, indicating good sightability for the majority of tracks, with 25% of tracks in a water clarity of under three where the seabed could not readily be distinguished. Potential sharks that could not reliably be identified as a white shark by the end of a track were excluded from the analysis.
Post flights, we accessed the drone’s flight logs and extracted the GPS coordinate and altitude information pertaining to each shark track (Raoult et al., 2018). This was then averaged to a rate of 1 Hz and plotted in QGIS (QGIS Development Team, 2019). Total length (TL) of each tracked white shark was estimated by extracting multiple still images from corresponding videos at points when the shark was nearest the surface and body straight. The shark in each image was then measured in number of pixels, for the given drone height and estimated depth of the shark. This was then converted to a total length (m) using a calculated equation for the drone’s sensor (see online Supplementary Material for additional information).
Data Analysis
During post analysis of video footage, any shark behavior association was recorded. These included “interactions” with conspecifics and dolphins, schools of fish, and “objects,” such as rays, isolated rocks or reef, and drifting seaweed clumps. For the analysis, drifting seaweed, rays, or isolated rock/reef sections were combined with unclassified objects, which may have been any of the former. Dolphin interactions and exchanges with conspecifics were combined, as were small and large schools of fish. Unattributed behavior, where a reaction or sudden change in behavior was observed but could not be attributed to an object or fish, were disregarded from the analysis. Additionally, a track segment containing boat interactions was removed prior to analysis. We used the trajr package (McLean et al., 2018) in R (R Core Team, 2019) to extract track metrics of mean (instantaneous) swim speed, straightness (Benhamou, 2004), and net velocity across shark vector (net track distance over duration, or rate of movement; Johnson et al., 2009), herein referred to as “net velocity.” Where there were multiple track segments for each shark, a weighted average was calculated, with weights proportional to the track duration, and applied to each shark. A weighted average was also calculated for the frequency of objects encountered along the swim path, as well as interactions with conspecifics and dolphins, and presence of fish schools. Environmental covariates in the analyses included wind strength (m s−1); wind direction, expressed as “nil,” “onshore,” or “offshore” relative to the location; tidal stage, transformed to a sine and cosine scale; water clarity (visibility), as a scale from 1–5; and time (24 h), from the center timepoint in the track, transformed to a sine and cosine scale.
Four statistical models were constructed and fitted using Bayesian inference through the Rstanarm package (Goodrich et al., 2018), which determined if swim direction and each of the track metrics were influenced by shark size or the environmental covariates. For the models of track metrics, we also included white shark interactions with objects, fish schools, and conspecifics and dolphins. Both dependent and independent variables were rescaled to the range [0, 1] prior to analysis to ensure numerical stability of the Markov Chain Monte Carlo (MCMC) methods. All variables and model coefficients were rescaled for use on the natural scale following the MCMC. For each model, location and date were each assigned random intercepts to account for locational variation, and where multiple sharks were tracked on the same day from the same location (see online Supplementary Material for R code and more information). Uninformative priors were assigned, and outputs used 95% Bayesian credible intervals and model diagnostics were examined using shinystan (Stan Development Team, 2017). We visualized model outputs by plotting kernel density estimates of the posterior probability density functions and to further support statistical inferences for the various fixed effects in each model.
In looking for evidence of a fixed-effect having either a positive or negative effect on the response variable, we summarized posterior distributions in two ways. Firstly, we looked at whether there was substantial probability mass concentrated on either positive or negative values of the regression coefficients. Where there was sufficient probability mass (>90%) concentrated on positive (negative) values, we concluded that there was some evidence of a positive (negative) effect on the response variable. Secondly, to summarize the posterior distribution, we constructed Bayesian 95% credible intervals for the parameters. Credible intervals that did not contain zero were considered to show strong evidence of being concentrated on either positive values (indicating an increase on the response variable) or negative values (a decrease in the response variable).
Results
A total of 108 white sharks were tracked (Figure 1), amounting to 26.33 h of footage and associated data (see online Supplementary Material for sample footage). The mean track time (±SD) was 17.1 (±18.3) min, with the longest track being 107.2 min and covering a straight line distance of over 3.47 km. Observed white sharks ranged between 1.9–4.0 m TL, with a mean of 2.7 m, and six sharks being ≥3.4 m.
White shark movement near the surf zone tended to be parallel to the beach line and in constant motion (Figure 2). Mostly, sharks tracked behind the surf zone, but were occasionally observed venturing into the surf zone, particularly when large schools of fish were present. Sometimes, tracks went for a few kilometers along the beachline, before heading back out to deeper water or into the channel waters of an estuarine bar (Figure 3). White sharks often appeared to be inquisitive because they opportunistically investigated fish schools and objects such as isolated patches of reef, rays, and drifting seaweed along their swim paths (Table 1). This was observed as a white shark notably turning toward the object, sometimes circling, before continuing on. In a few cases, white sharks were observed to momentarily increase speed toward fish, often accompanied by a sharp turn, and there were a number of cases where this behavior was observed but no fish could be discerned. There were also few notable instances where large schools of fish caused tracks of white sharks to be more circular and tortuous (Figure 2).
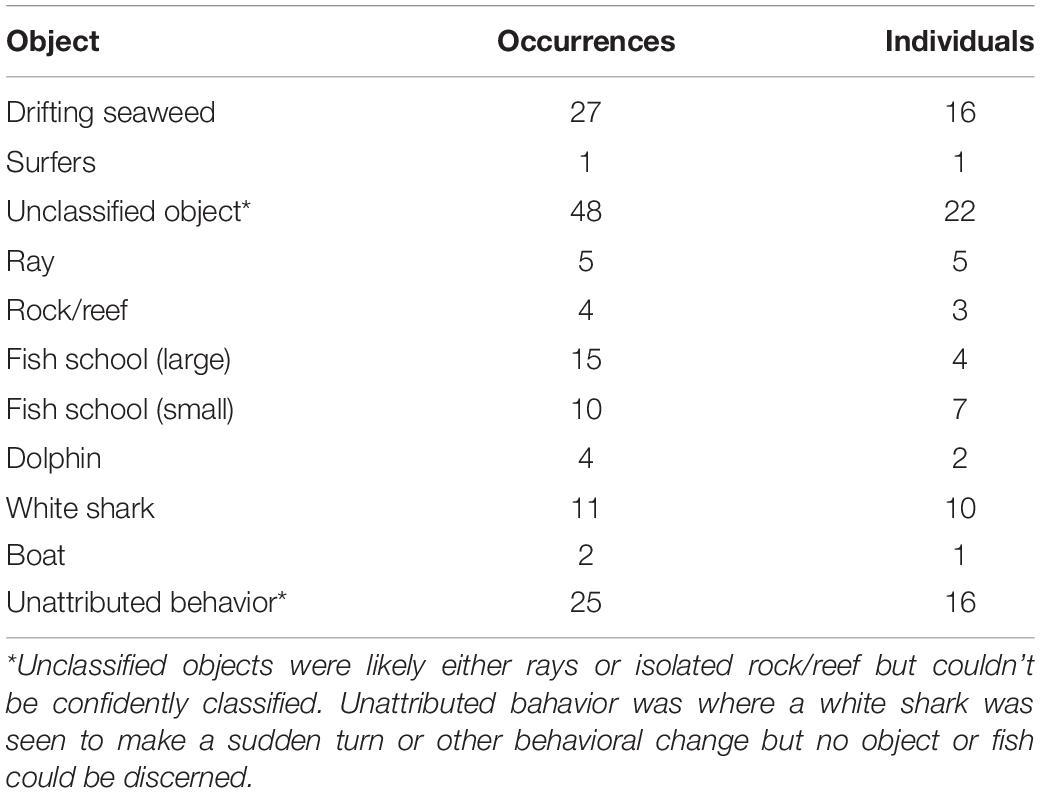
Table 1. Frequency of occurrences by the number of individual white sharks that were visually interpreted to be investigating each object classification.
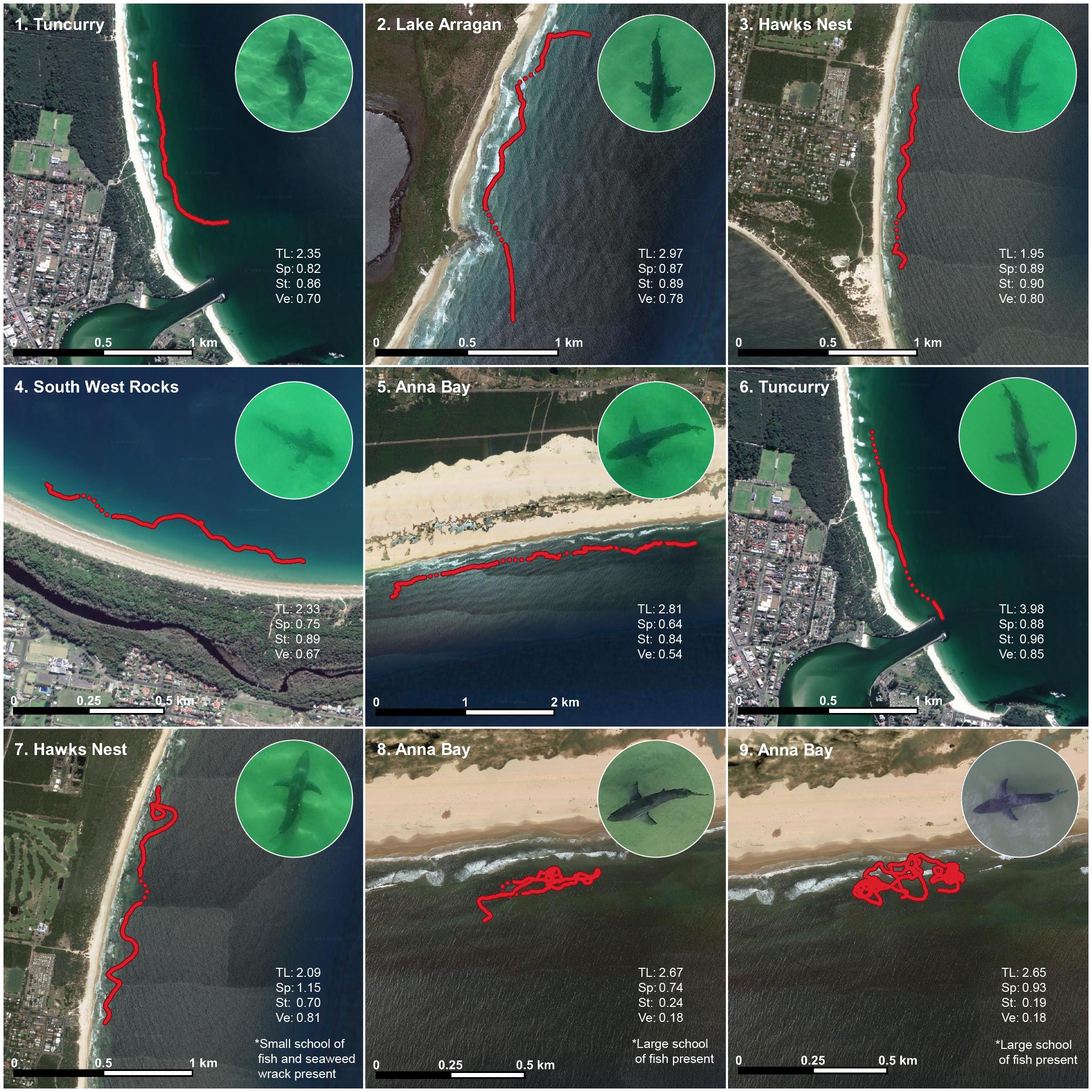
Figure 2. Examples of white shark (Carcharodon carcharias) tracks off some of the coastal beaches from the study. Plots 1 – 6 show common track behavior. Plot 7 shows the effect of a small school of fish and some seaweed rack, and plots 8 and 9 show the effect of large schools of fish on movement tracks. For each of the track plots, an image of the shark indicating direction is shown, along with the location, total length (TL) in m, mean speed (Sp) in m s−1, straightness index (St), and net velocity of shark vector (Ve) in m s−1.
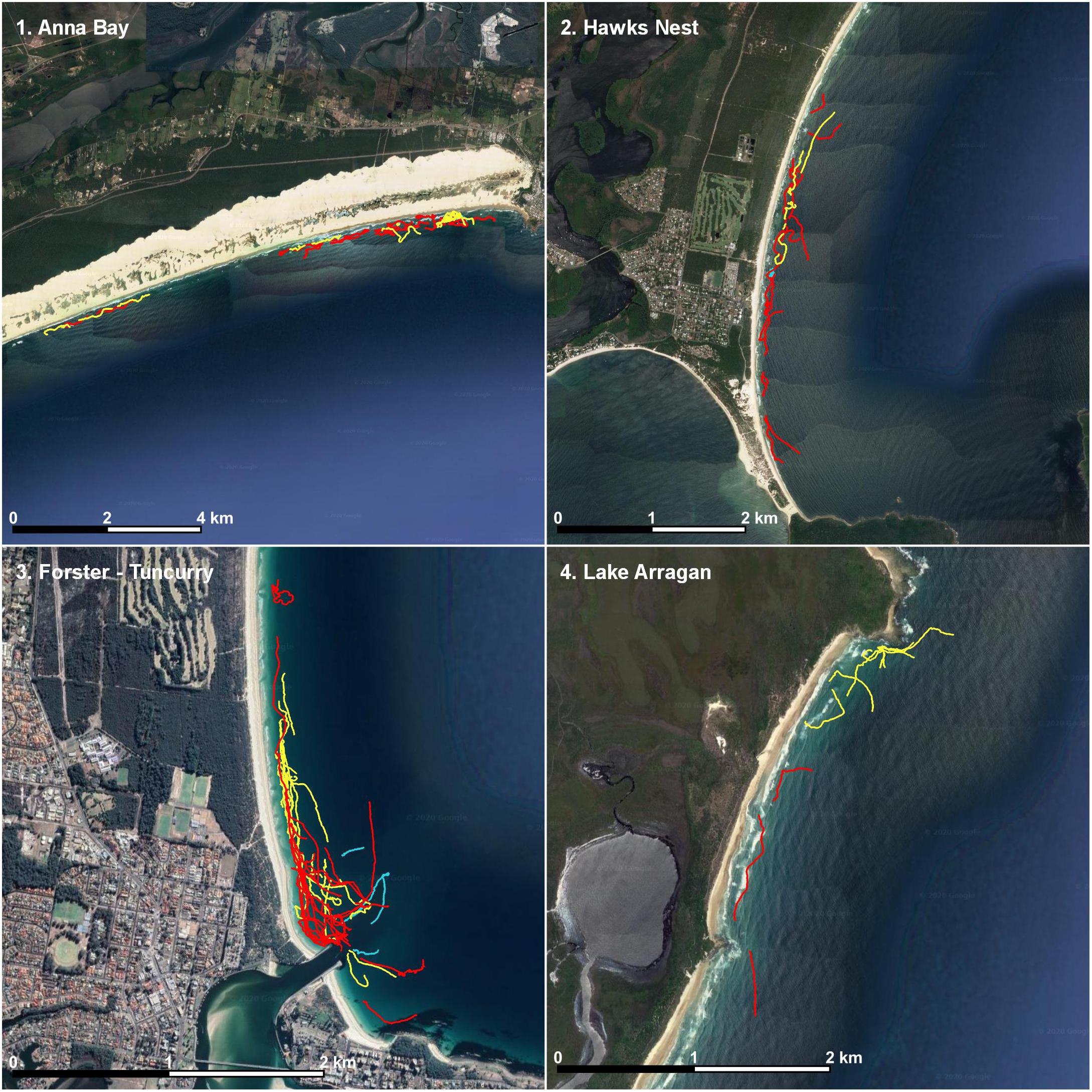
Figure 3. An overview of all white shark (Carcharodon carcharias) tracks from Anna Bay, Hawks Nest, Forster–Tuncurry, and Lake Arragan. Red tracks indicate a southward movement direction, yellow tracks a northward direction, and blue indicate no direction assigned.
Thirty-five white sharks were observed moving in a “northern” direction along coastal beaches compared to 59 moving in a “southern” direction. Seven white sharks were either tracked moving in closer to shore from deeper water or moving offshore away from the beach, and a further seven displayed highly tortuous tracks where no net direction could reliably be discerned (Table 2 and Figure 3). There was no statistical evidence to support hypotheses that swim direction of white sharks along the beach was influenced by any of the fixed model coefficients including environmental parameters of tide, visibility, wind direction and strength, or time of day.
The mean swim speed of observed white sharks was 0.82 m s−1 (±0.15), and ranged between 0.54–1.23 m s−1. From the model, mean swim speed showed statistical evidence for the shark length coefficient to be non-zero, such that the 95% credible intervals did not enclose zero (Table 3). This indicated that the mean swim speed of sharks increased with size, such that for every meter increase in shark length, mean speed increased by 0.10 m s−1 (±0.04). Similarly, the presence of schools of fish increased mean swim speeds by a predicted mean of up to 0.33 m s−1 (±0.12). Interactions with dolphins or conspecifics potentially had a similar effect of increasing average swim speeds by a predicted mean of up to 0.15 m s−1 (±0.10). However, the 95% credible intervals for interactions did not exclude zero (Table 3), but did show 93% probability mass on positive values of the regression coefficient, indicating some evidence to support increased average swim speed. Time of day also influenced mean swim speeds of white sharks. The model showed statistical evidence for mean swim speeds to be faster during morning and afternoon periods compared to around midday, such that swim speed was predicted to increase in the order of 0.11 m s−1 (±0.08) in the morning and afternoon compared with midday. Similarly, the model showed some evidence to suggest a weaker trend of mean swim speeds increasing with time of day by 0.05 m s−1 (±0.03) in the afternoon compared with morning. However, the 95% credible intervals did not exclude zero (Table 1), with 94% probability mass on negative values of the regression coefficient.
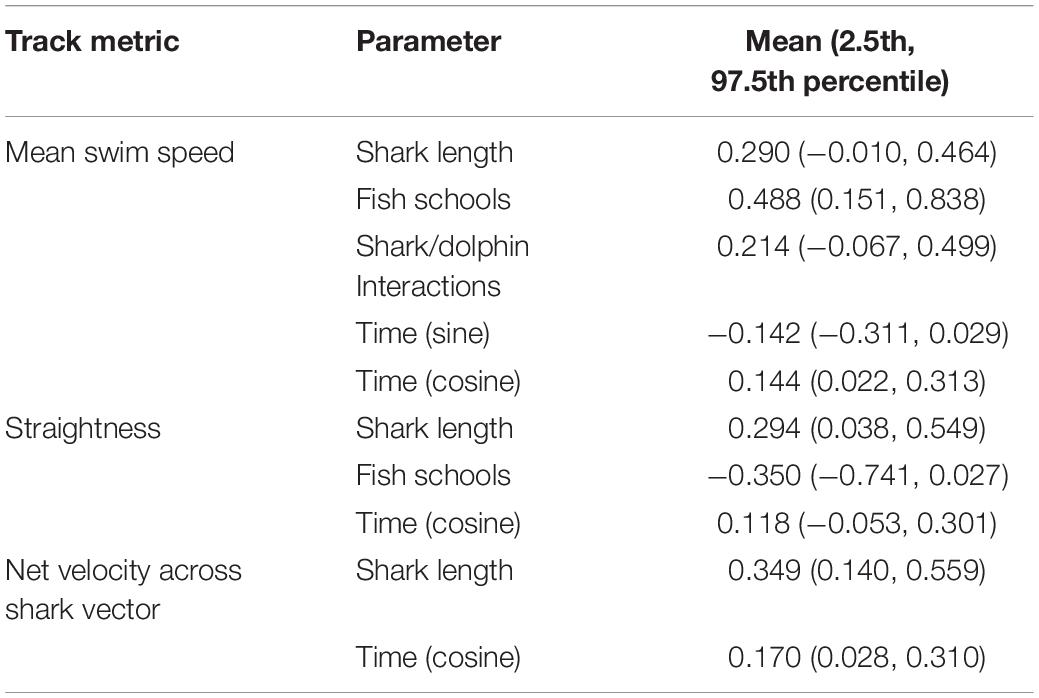
Table 3. Coefficient means and 95% credible intervals (in brackets) for parameters in each modeled track metric where posterior probability density functions had at least 90% probability of being above or below zero.
The mean straightness index across all tracks was 0.74 (±0.24). The model for straightness demonstrated statistical evidence for tracks to become straighter with increasing shark size, such that for every meter increase in shark size, track straightness increased by 0.14 (±0.06). Conversely, the presence of fish schools (overall) along the swim path showed a trend for tracks to be less straight to a predicted mean effect of decreasing straightness by -0.34 (±0.19). Although the 95% credible intervals for fish schools did not exclude zero (Table 3), there was 96% of the probability mass stacked on negative values of the regression coefficient, indicating some evidence for fish schools having a negative effect on track straightness. Time of day may have also affected track straightness, with the model providing some evidence to suggest the straightness of tracks increased by a predicted mean of 0.15 (±0.11) in the morning and afternoon compared with midday. However, only 91% probability mass (for the cosine of time) was stacked on positive values of the regression coefficient.
The mean net velocity of white sharks was 0.61 m s−1 (±0.23), with a maximum net velocity of 1.11 m s−1. The model demonstrated statistical evidence for net velocity to increase with shark size (Table 3), with every meter increase in shark size predicting a 0.16 m s−1 (±0.06) increase in net velocity. The model also showed statistical evidence for net velocities to increase in morning and afternoon periods by a mean increase of up to 0.25 m s−1 (±0.10). There was no evidence to support environmental covariates of wind strength and direction, tide, or water clarity having any influence over the nature of white shark movement patterns along coastal beaches according to the track metrics of mean speed, straightness or net velocity.
Discussion
We demonstrate for the first time the localized movement behavior of white sharks along coastal beaches using drones. From the white sharks sampled, which were not influenced by the presence of whale carcasses or seal colonies, movements observed were typically behind the surf break, relatively parallel to the shoreline at around 0.61 m s−1 (∼2.2 km h−1) for potentially long stretches (up to a few kilometers or more) of beach. This relatively predictable pattern of localized movements is contrary to their long-range spatial movements, where white sharks often take solitary journeys over thousands of kilometers in a largely unpredictable fashion (Bruce et al., 2006, 2019; Skomal et al., 2017). The consistent patterns of nearshore movement has useful implications for the development of non-destructive management approaches that address unprovoked shark bites. These findings could also aid in dispelling some of the public misconceptions and fear around white sharks, which may aid in gaining wider support for mitigation approaches that complement conservation objectives (Pepin-Neff and Wynter, 2017, 2018; Simmons and Mehmet, 2018).
When it came to white sharks potentially detecting objects of interest, which included isolated reef, rays, drifting seaweed, and a surfer, they did not deviate significantly from their predicted swim path. This was also reaffirmed by the high degree of straightness across tracks and the lack of influence on track metrics from investigating objects, which was different from the effect of fish schools. Thus, a white shark traveling parallel to the beach beyond a certain distance from the surf break would unlikely pose a threat to a person in the surf zone. This might have implications for the functionality of shark mitigation methods targeting sharks a distance from the surf break, such as SMART drumlines (Tate et al., 2019). These are often placed ∼500 m from the shore and parallel to the shoreline in a similar fashion to traditional drum lines (Reid et al., 2011; Sumpton et al., 2011). It has been acknowledged that SMART drumlines currently do not prevent bull sharks from entering closer to shore (Guyomard et al., 2019), and it is likely that this is also the case for white sharks. However, the interception rate of white sharks by SMART drum lines might be improved by placing them perpendicular to the shoreline near the ends of the beach or where river mouths and their associated rock training walls are located. This would also apply to traditional drumlines and shark mesh nets, which may explain in part the extremely low capture rates of white sharks from current shark mesh net operations (Reid et al., 2011; Sumpton et al., 2011). In changing the orientation of traditional drumlines and mesh nets, ethical considerations around depleting wide-ranging vulnerable populations, together with communities increasingly opposing cull-based approaches should be carefully considered (Hillary et al., 2018; Pepin-Neff and Wynter, 2018; Roff et al., 2018; Simmons and Mehmet, 2018). There could also be some merit in exploring the potential to exploit the inquisitive nature of white sharks by strategically placing objects providing visual stimuli at the ends of a beach. These could be arranged to incrementally guide sharks back further offshore as they make minor adjustments to their swim path to investigate the objects, acting as passive “shark deflectors.” However, although sharks are known to be highly inquisitive (Strong, 1996), which was reaffirmed in this study, the utility to consistently draw sharks away may be limited and would need thorough research investigation.
Considering white shark movements, being slow and relatively linear along the beach, it would generally allow for more than one surveillance pass with a drone in a transect-based survey procedure, increasing the chance of detection in reasonable water clarity (Colefax et al., 2019; Kelaher et al., 2020a). This utility would be most useful at locations with long stretches of beach and with drone operations not being limited by line of sight aviation restrictions (Colefax et al., 2017). An advantage of drones in such a scenario over manned aircraft would be the ability to make a higher frequency of passes over an area, increasing the chance of intercepting a white shark upon it entering the area (Kelaher et al., 2020b). Additionally, because mean swim speeds and rates of travel were fairly consistent, with tracks broadly linear (in absence of large schools of fish), predictions could generally be made on where a white shark might be along the beach after a given time. This information could be used by surveillance approaches to shark mitigation, where appropriate safe distances between people and white sharks could be better defined and based on how long it would take people to evacuate the water in the context of shark movement speed. This would aid developing better operating protocols for deciding when it might be appropriate to evacuate people from the water.
Water clarity did not influence swim behavior according to track metrics in our study, however, it has been reported to affect the assemblages of large fauna in beach environments from drone-based surveys (Kelaher et al., 2020a). In addition, depleting water clarity reduces the reliability of detections from aerial surveys, which would confound any behavioral associations with water clarity (Pollock et al., 2006; Butcher et al., 2019). Thus, the effect of water clarity might not reduce the chance of a white shark swimming nearshore. In this study, 25% of white sharks were tracked during poor water clarity, with these cases often requiring the animal to be tracked for a few minutes before a reliable classification could be made. There were also a few cases where the track was disregarded due to an inability to classify the animal as a white shark. Consequently, whilst white shark behavior observed in this study may compliment drone-based surveillance approaches to shark mitigation, the reliability of detections during low water clarity would need to be further addressed if people are utilizing the surf during such conditions.
Average swim speeds of sharks off beaches were relatively slow (0.82 m s−1), with even slower net velocities (0.61 m s−1). Other studies have reported similar average speeds and velocities of between 0.52–1.35 m s−1 (Bonfil et al., 2005; Johnson et al., 2009; Watanabe et al., 2019), with faster average speeds of up to 2.91 m s−1 being recorded around seal colonies (Semmens et al., 2013). It has been suggested that white sharks travel at slower speeds to conserve energy and maximize foraging efficiency for locating prey (Johnson et al., 2009; Watanabe et al., 2019), and at faster speeds with associated high energy expenditure when actively patrolling for prey (Johnson et al., 2009; Semmens et al., 2013). Whilst the size classes of white sharks are larger around seal colonies, with an average of 3.6 m TL (Semmens et al., 2013; Watanabe et al., 2019) as opposed to 2.7 m (TL) in this study, the effect of shark size on swim speeds was relatively subtle. Track patterns and behavior were also different to those observed at seal colonies, suggesting that swim speed and associated behavior near the surf break to be characteristic of energy conservation and opportunistic foraging, rather than actively patrolling for prey (Martin et al., 2009; Semmens et al., 2013). However, as this was an observational study limited to relatively short tracking durations and by the numbers of white sharks, it is also plausible that the observed behavior would change with provisional prey items such as seals or whale carcasses.
Time of day influenced white shark behavior in terms of average swim speed, track straightness and net velocity. Notably, the slightly faster swim speeds and higher net velocities, as well as potentially slightly straighter tracks, in morning and afternoon periods. Whilst white sharks were seen to display inquisitive behavior throughout the day, this result could indicate more purposeful foraging during lower light periods outside of our sampling times. White sharks attribute a large portion of their brain for visual processing, and their eye morphology is believed to provide better visibility in low light at the cost of visual resolution (Strong, 1996; Collin, 2018). Hence, higher rates of movement and straighter track trajectories around crepuscular periods has been observed on white sharks, with it being attributed to more directed patrolling activities (Johnson et al., 2009). For example, white sharks predate on seals more effectively in the early mornings, with their hunting effort apportioned accordingly (Martin et al., 2005). Therefore, although white sharks in this study were found to be more representative of inquisitive behavior and opportunistic foraging, it may be plausible that white sharks display more of a patrolling type behavior along coastal beaches around crepuscular periods. Obtaining high resolution behavioral data on white sharks along coastal beaches during low light periods may be logistically difficult, however, it would confirm the assumption of dawn and dusk being higher risk periods for unprovoked shark bites.
White sharks found near the surf zone in this study were juvenile to sub-adult size classes, which is consistent with those reported for nearshore habitats outside of seal colonies where white sharks aggregate (Reid et al., 2011; Bruce et al., 2019; Tate et al., 2019). Ontogenetic feeding preferences of white sharks incorporate elasmobranchs and mammals into their diets at around 3.0 m (TL), with significant dietary trophic shifts measured in vertebrae isotopes between 3.4 and 4.0 m (Estrada et al., 2006). The majority of sharks tracked in this study would still have fish as a major food source (Estrada et al., 2006; French et al., 2017). This was evident by the observed effects of fish schools on behavior, particularly swim speeds and track straightness, and the willingness of similar sized sharks to take fish baits (Bruce et al., 2006, 2019; Tate et al., 2019). It was also observed a few times where white sharks would rapidly accelerate for a feeding attempt on fish. Similar, albeit more aggressive attack strategies have been observed on seals, which resemble a much larger prey (Martin et al., 2005; Ritter and Quester, 2016). Conversely, the observed inquisitive behavior of white sharks to other objects, including non-food items, may lend support to the exploratory bite hypothesis as a causal factor behind unprovoked shark bites given the often superficial wounds inflicted on humans compared to seals (Ritter and Quester, 2016). However, despite the occasional presence of water users (particularly surfers) during the current study, and apart from one minor investigation by a white shark toward a surfer, we did not observe any noticeable interactions with people and so inferences are speculative. Furthermore, there could be individuals that display different behavior than typically observed in the current study (Clua and Linnell, 2018). Whether food sources such as the presence of large schools of fish might alter the behavior of sharks toward humans in the water at the same time is unknown. However, because sharks are likely to exhibit an alteration from “typical” behavior and display more tortuous tracks, they are more likely to remain longer in an area with less relaxed behavior, which may infer increased risk to bathers when large schools of fish are present.
There is an urgent need for effective approaches to shark bite mitigation that can resolve underlying social issues around the conflict, adequately address beach safety, and support conservation values (Dickman, 2010; Reid et al., 2011; Simmons and Mehmet, 2018). In terrestrial settings, relevant animal tracking information has proved invaluable for supporting conservation and alleviating human-wildlife conflict (McGowan et al., 2017; Fraser et al., 2018; Suraci et al., 2019). Likewise, the fairly consistent movement patterns of white sharks, relevant to the area and scale that shark bites occur, will allow further development of non-destructive management approaches. Such developments might include changing the placement and orientation of SMART drumlines to increase catch probabilities of white sharks prone to entering near the surf zone. Similarly, research into visual stimuli that may direct sharks away from the surf zone might provide a new avenue of mitigation research regarding shark bites. Furthermore, the slow swim speeds and largely predictable track trajectories will allow greater chances for detection in transect-based drone-surveillance when water clarity is reasonable. Further investigation into the effect on white shark behavior of bait schools and other large provisional food sources, as well as around crepuscular periods, will provide better resolve regarding potential heightened risk to bathers. However, from the observations of this study, it is likely that such situations present an increased risk. Thus, it might be considered prudent to abstain from water-based activities at coastal beaches when large shark-attracting food sources are present.
Data Availability Statement
The raw data supporting the conclusions of this article will be made available by the authors, without undue reservation.
Ethics Statement
Drone flights were made under New South Wales Department of Primary Industries (NSW DPI) and Office of Environment and Heritage (OEH) scientific permits (Ref. P01/0059; MWL000102746) and animal ethics (16/09 – SIMS).
Author Contributions
AC and PB conceived the idea. All authors contributed to the methodological design. AC collected the data. AC and DP analyzed the data. AC led the writing of the manuscript. All authors contributed critically to intellectual content, the article drafts, and gave final approval for publication.
Funding
Project funding and support was provided by the NSW DPI and associated NSW Shark Management Strategy, Southern Cross University, and the Paddy Pallin Foundation in partnership with the Royal Zoological Society of NSW.
Conflict of Interest
The authors declare that the research was conducted in the absence of any commercial or financial relationships that could be construed as a potential conflict of interest.
Acknowledgments
Additional support was provided by Sci-eye. Further appreciation toward M. Dujmovic (Hover UAV) for assisting with some field sampling and C. Goebel-Try for assisting with some data processing.
Supplementary Material
The Supplementary Material for this article can be found online at: https://www.frontiersin.org/articles/10.3389/fmars.2020.00268/full#supplementary-material
DATA SHEET S1 | Provides additional detail on drone tracking specifications and shark length calculations can be found in the online version.
DATA SHEET S2 | Contains the R code pertaining to the analysis and two sample videos (credit: A. Colefax) of a typical swimming motion of a 2.6 m white shark near the surf break in clear water (Video 1), and an interaction of a different 2.6 m white shark with a large bait school (Video 2).
VIDEO S1 | Typical white shark swim motion observed along coastal beaches.
VIDEO S2 | An example of a white shark interaction with a large school of fish.
References
Benhamou, S. (2004). How to reliably estimate the tortuosity of an animal’s path: straightness, sinuosity, or fractal dimension? J. Theor. Biol. 229, 209–220. doi: 10.1016/j.jtbi.2004.03.016
Bonfil, R., Meyer, M., Scholl, M. C., Johnson, R., O’Brien, S., Oosthuizen, H., et al. (2005). Transoceanic migration, spatial dynamics, and population linkages of white sharks. Science 310, 100–103. doi: 10.1126/science.1114898
Bruce, B. D., Harasti, D., Lee, K., Gallen, C., and Bradford, R. (2019). Broad-scale movements of juvenile white sharks Carcharodon carcharias in eastern Australia from acoustic and satellite telemetry. Mar. Ecol. Prog. Ser. 619, 1–15. doi: 10.3354/meps12969
Bruce, B. D., Stevens, J. D., and Malcolm, H. (2006). Movements and swimming behaviour of white sharks (Carcharodon carcharias) in Australian waters. Mar. Biol. 150, 161–172. doi: 10.1007/s00227-006-0325-321
Butcher, P., Piddock, T., Colefax, A., Hoade, B., Peddemors, V., Borg, L., et al. (2019). Beach safety: can drones provide a platform for sighting sharks? Wildl. Res. 46, 701–712. doi: 10.1071/WR18119
Clua, E. E. G., and Linnell, J. D. C. (2018). Individual shark profiling: an innovative and environmentally responsible approach for selectively managing human fatalities. Conserv. Lett. 12:e12612. doi: 10.1111/conl.12612
Colefax, A. P., Butcher, P. A., and Kelaher, B. P. (2017). The potential for unmanned aerial vehicles (UAVs) to conduct marine fauna surveys in place of manned aircraft. ICES J. Mar. Sci. 75, 1–8. doi: 10.1093/icesjms/fsx100
Colefax, A. P., Butcher, P. A., Pagendam, D. E., and Kelaher, B. P. (2019). Reliability of marine faunal detections in drone-based monitoring. Ocean Coast. Manag. 174, 108–115. doi: 10.1016/j.ocecoaman.2019.03.008
Collin, S. P. (2018). Scene through the eyes of an apex predator: a comparative analysis of the shark visual system. Clin. Exp. Optometry 101, 624–640. doi: 10.1111/cxo.12823
Cressey, D. (2013). Australian shark-cull plan draws scientists’ ire. Nat. News. doi: 10.1038/nature.2013.14373 (accessed December 13, 2013).
Davidson, L. N. K., Krawchuk, M. A., and Dulvy, N. K. (2016). Why have global shark and ray landings declined: improved management or overfishing? Fish Fish. 17, 438–458. doi: 10.1111/faf.12119
Dickman, A. J. (2010). Complexities of conflict: the importance of considering social factors for effectively resolving human-wildlife conflict. Anim. Conserv. 13, 458–466. doi: 10.1111/j.1469-1795.2010.00368.x
Draheim, M. M., Madden, F., McCarthy, J. B., and Parsons, E. C. M. (2015). Human-Wildlife Conflict: Complexity in the Marine Environment. Oxford: Oxford University Press.
Dulvy, N. K., Fowler, S. L., Musick, J. A., Cavanagh, R. D., Kyne, P. M., Harrison, L. R., et al. (2014). Extinction risk and conservation of the world’s sharks and rays. eLife 3:e00590. doi: 10.7554/eLife.00590
Estrada, J. A., Rice, A. N., Natanson, L. J., and Skomal, G. B. (2006). Use of isotopic analysis of vertebrae in reconstructing ontogenetic feeding ecology in white sharks. Ecology 87, 829–834. doi: 10.1890/0012-9658(2006)87[829:uoiaov]2.0.co;2
Ferretti, F., Worm, B., Britten, G. L., Heithaus, M. R., and Lotze, H. K. (2010). Patterns and ecosystem consequences of shark declines in the ocean. Ecol. Lett. 13, 1055–1071. doi: 10.1111/j.1461-0248.2010.01489.x
Fraser, K. C., Davies, K. T. A., Davy, C. M., Ford, A. T., Flockhart, D. T. T., and Martins, E. G. (2018). Tracking the conservation promise of movement ecology. Front. Ecol. Evol. 6:150. doi: 10.3389/fevo.2018.00150
French, G. C. A., Sturup, M., Rizzuto, S., van Wyk, J. H., Edwards, D., Dolan, R. W., et al. (2017). The tooth, the whole tooth and nothing but the tooth: tooth shape and ontogenetic shift dynamics in the white shark Carcharodon carcharias. J. Fish Biol. 91, 1032–1047. doi: 10.1111/jfb.13396
Goodrich, B., Gabry, J., Ali, I., and Brilleman, S. (2018). Rstanarm: Bayesian Applied Regression Modeling Via Stan. R package version 2.5.0.
Guyomard, D., Perry, C., Tournoux, P. U., Cliff, G., Peddemors, V., and Jaquemet, S. (2019). An innovative fishing gear to enhance the release of non-target species in coastal shark-control programs: the SMART (shark management alert in real-time) drumline. Fish. Res. 216, 6–17. doi: 10.1016/j.fishres.2019.03.011
Hillary, R. M., Bravington, M. V., Patterson, T. A., Grewe, P., Bradford, R., Feutry, P., et al. (2018). Genetic relatedness reveals total population size of white sharks in eastern Australia and New Zealand. Sci. Rep. 8:2661. doi: 10.1038/s41598-018-20593-w
Hussey, N. E., Macneil, M. A., McMeans, B. C., Olin, J. A., Dudley, S. F., Cliff, G., et al. (2014). Rescaling the trophic structure of marine food webs. Ecol. Lett. 17, 239–250. doi: 10.1111/ele.12226
Johnson, R., Bester, M. N., Dudley, S. F. J., Oosthuizen, W. H., Meÿer, M., Hancke, L., et al. (2009). Coastal swimming patterns of white sharks (Carcharodon carcharias) at Mossel Bay, South Africa. Environ. Biol. Fish. 85, 189–200. doi: 10.1007/s10641-009-9477-4
Kelaher, B. P., Colefax, A. P., Tagliafico, A., Bishop, M. J., Giles, A., and Butcher, P. A. (2020a). Assessing variation in assemblages of large marine fauna off ocean beaches using drones. Mar. Freshw. Res. 71, 68–77. doi: 10.1071/mf18375
Kelaher, B. P., Peddemors, V. M., Hoade, B., Colefax, A. P., and Butcher, P. A. (2020b). Comparison of sampling precision for nearshore marine wildlife using unmanned and manned aerial surveys. J. Unmanned Vehicle Syst. 8, 30–43. doi: 10.1139/juvs-2018-0023
Martin, R. A., Hammerschlag, N., Collier, R. S., and Fallows, C. (2005). Predatory behaviour of white sharks (Carcharodon carcharias) at Seal Island, South Africa. J. Mar. Biol. Assoc. U. K. 85, 1121–1135. doi: 10.1017/s002531540501218x
Martin, R. A., Rossmo, D. K., and Hammerschlag, N. (2009). Hunting patterns and geographic profiling of white shark predation. J. Zool. 279, 111–118. doi: 10.1111/j.1469-7998.2009.00586.x
McGowan, J., Beger, M., Lewison, R. L., Harcourt, R., Campbell, H., Priest, M., et al. (2017). Integrating research using animal-borne telemetry with the needs of conservation management. J. Appl. Ecol. 54, 423–429. doi: 10.1111/1365-2664.12755
McLean, D. J., Skowron Volponi, M. A., and Tregenza, T. (2018). Trajr: an R package for characterisation of animal trajectories. Ethology 124, 440–448. doi: 10.1111/eth.12739
Pepin-Neff, C., and Wynter, T. (2017). Shark bites and shark conservation: an analysis of human attitudes following shark bite incidents in two locations in Australia. Conserv. Lett. 11:e12407. doi: 10.1111/conl.12407
Pepin-Neff, C. L., and Wynter, T. (2018). Reducing fear to influence policy preferences: an experiment with sharks and beach safety policy options. Mar. Policy 88, 222–229. doi: 10.1016/j.marpol.2017.11.023
Pollock, K. H., Marsh, H. D., Lawler, I. R., and Alldredge, M. W. (2006). Estimating animal abundance in heterogeneous environments: an application to aerial surveys for dugongs. J. Wildl. Manag. 70, 255–262.
QGIS Development Team (2019). QGIS geographic information system. Open Source Geospatial Foundation Project. Available online at: http://qgis.osgeo.org
R Core Team (2019). R: A Language and Environment for Statistical Computing. Vienna: R Foundation for Statistical Computing.
Raoult, V., Tosetto, L., and Williamson, J. (2018). Drone-based high-resolution tracking of aquatic vertebrates. Drones 2:37. doi: 10.3390/drones2040037
Reid, D. D., Robbins, W. D., and Peddemors, V. M. (2011). Decadal trends in shark catches and effort from the New South Wales, Australia, Shark Meshing Program 1950 - 2010. Mar. Freshw. Res. 62:676. doi: 10.1071/mf10162
Ritter, E., and Quester, A. (2016). Do white shark bites on surfers reflect their attack strategies on pinnipeds? J. Mar. Biol. 2016, 1–7. doi: 10.1155/2016/9539010
Roff, G., Brown, C. J., Priest, M. A., and Mumby, P. J. (2018). Decline of coastal apex shark populations over the past half century. Commun. Biol. 1:223. doi: 10.1038/s42003-018-0233-1
Semmens, J. M., Payne, N. L., Huveneers, C., Sims, D. W., and Bruce, B. D. (2013). Feeding requirements of white sharks may be higher than originally thought. Sci. Rep. 3:1471. doi: 10.1038/srep01471
Simmons, P., and Mehmet, M. I. (2018). Shark management strategy policy considerations: community preferences, reasoning and speculations. Mar. Policy 96, 111–119. doi: 10.1016/j.marpol.2018.08.010
Skomal, G. B., Braun, C. D., Chisholm, J. H., and Thorrold, S. R. (2017). Movements of the white shark Carcharodon carcharias in the North Atlantic Ocean. Mar. Ecol. Prog. Ser. 580, 1–16. doi: 10.3354/meps12306
Stan Development Team (2017). shinystan: interactive visual and numerical diagnostics and posterior analysis for Bayesian models. R package version 2.3.0.
Strong, W. R. Jr, (1996). “Shape discrimination and visual predatory tactics in white sharks,” in Great White Sharks: The Biology of Carcharodon Carcharias, eds A. P. Klimley and D. G. Ainley (San Diego: Academic Press), 229–240. doi: 10.1016/b978-012415031-7/50022-7
Sumpton, W. D., Taylor, S. M., Gribble, N. A., McPherson, G., and Ham, T. (2011). Gear selectivity of large-mesh nets and drumlines used to catch sharks in the queensland shark control program. Afr. J. Mar. Sci. 33, 37–43. doi: 10.2989/1814232x.2011.572335
Suraci, J. P., Frank, L. G., Oriol-Cotterill, A., Ekwanga, S., Williams, T. M., and Wilmers, C. C. (2019). Behavior-specific habitat selection by African lions may promote their persistence in a human-dominated landscape. Ecology 100:e02644. doi: 10.1002/ecy.2644
Tate, R., Cullis, B., Smith, S., Kelaher, B., Brand, C., Gallen, C., et al. (2019). White sharks (Carcharodon carcharias) display minimal short-term physiological stress responses after capture on SMART drumlines. Conserv. Physiol. 7:coz042.
Taylor, J., McLean, L., Korner, A., and Glozier, N. (2019). Direct and indirect psychological impacts of shark-bite events. Austral. New Zeal. J. Psychiatry 53, 27–36. doi: 10.1177/0004867418808899
Keywords: animal behavior, drone tracking, human-wildlife conflict, shark behavior, shark mitigation, wildlife conservation
Citation: Colefax AP, Kelaher BP, Pagendam DE and Butcher PA (2020) Assessing White Shark (Carcharodon carcharias) Behavior Along Coastal Beaches for Conservation-Focused Shark Mitigation. Front. Mar. Sci. 7:268. doi: 10.3389/fmars.2020.00268
Received: 12 December 2019; Accepted: 03 April 2020;
Published: 28 April 2020.
Edited by:
Maria Lourdes D. Palomares, The University of British Columbia, CanadaReviewed by:
Vincent Raoult, University of Newcastle Callaghan, AustraliaJane E. Williamson, Macquarie University, Australia
Copyright © 2020 Colefax, Kelaher, Pagendam and Butcher. This is an open-access article distributed under the terms of the Creative Commons Attribution License (CC BY). The use, distribution or reproduction in other forums is permitted, provided the original author(s) and the copyright owner(s) are credited and that the original publication in this journal is cited, in accordance with accepted academic practice. No use, distribution or reproduction is permitted which does not comply with these terms.
*Correspondence: Andrew P. Colefax, YWNvbGVmYXhAc2NpZXllLmNvbS5hdQ==