- 1ARDITI – Agência Regional para o Desenvolvimento da Investigação Tecnologia e Inovação, Observatório Oceânico da Madeira (OOM), Funchal, Portugal
- 2UCA – Departamento de Biología, Facultad de Ciencias del Mar y Ambientales, Universidad de Cádiz, Puerto Real, Spain
- 3INMAR – Instituto Universitario de Investigaciones Marinas (INMAR), Campus de Excelencia Internacional del Mar (CEI-MAR), Universidad de Cádiz, Puerto Real, Spain
- 4IDL – Instituto Dom Luiz, Faculdade de Ciências da Universidade de Lisboa, Lisbon, Portugal
Within the Portuguese Exclusive Economic Zone, the Great Meteor and Madeira-Tore complexes are highly productive areas, which are likely to be classified as marine protected areas (MPAs) due to their ecological vulnerability. This was the main focus of the BIOMETORE project and, framed on it, the aim of the present study was to investigate the physical connectivity between both seamount complexes. Using the HYbrid Coordinate Ocean Model coupled with the Connectivity Modeling System (CMS) (a Lagrangian tool), a series of experiments was conducted in order to determine the influence of the main oceanographic phenomena governing the area in: (i) the origin of the particles that reach each complex, (ii) their capacity to capture and retain incoming particles, and (iii) the physical connectivity between them as well as the intra-connectivity within each seamount system. Due to the geographical location of both groups of seamounts, the Azores Current (AzC) and its associated eddies were identified as the main transport pathways, its influence being stronger at intermediate waters and decreasing with depth. Notwithstanding, the Great Meteor and the Madeira-Tore were mainly affected by the AzC southward and eastward branches, respectively, resulting in a non-significant connectivity between the two groups. Meanwhile, the inter-connectivity between seamounts slightly varied with depth at the Great Meteor complex while increasing at Madeira-Tore. In addition, the Plateau, Irving, and Cruiser (PIC) seamounts from the Great Meteor complex and Gorringe and Coral from the Madeira-Tore complex proved to incorporate the regional connectivity routes. Although containing the three smallest seamounts, Madeira-Tore showed the higher capturing capacity per square kilometer, highlighting the influence of the “sticky water effect.” In the Great Meteor complex, the “seamount effect” seems to be the main phenomenon responsible for the greater retention and self-recruitment abilities of these seamounts. The presented results provide valuable information for the design of a MPA to preserve these vulnerable habitats.
Introduction
Seamounts are wide and prominent underwater features of volcanic or tectonic origin that occur mainly around mid-ocean ridges, island-arc areas, and above mantle plumes (Etnoyer et al., 2010; Staudigel and Clague, 2010; Wessel et al., 2010). The size of the seamount is an important factor to consider and is usually defined by their height or relief (Kitchingman et al., 2007). According to Pitcher et al. (2007), elevations higher than 1,000 m are defined as large seamounts, whereas small seamounts are within the range of 100–1,000 m. Recent studies have estimated that the total number of large seamounts (>1,000 m) worldwide varies between 25,000 and 140,000, whereas small ones (100–1,000 m) are more abundant, approximately between 125,000 and 25 million (Wessel et al., 2010; Yesson et al., 2011). Nevertheless, seamount abundances can vary considerably, depending on the methodology applied and the definition of a seamount (Wessel et al., 2010). Despite this uncertainty, most authors highlight seamounts as one of the world’s major (underwater) biomes (Etnoyer et al., 2010).
Seamounts are characterized by high productivity and food availability, being considered hotspots for several benthic and pelagic communities, although this is not true for all seamounts as their distinct morphological and oceanographic features may alter biodiversity and productivity patterns (Morato et al., 2016). The productivity of seamounts is closely related to their shallowness since the interaction between the topographical and the physical features (e.g., internal tides) enhances the vertical movement (upwelling) of nutrients toward the euphotic zone (Tuerena et al., 2019). If the local biomass production is sustained long enough, it will allow the transfer of energy to higher trophic levels. Otherwise, seamounts must rely on outer inputs of organic material to support resident populations (Rogers, 1994; White et al., 2008). Their communities are dominated by suspension feeders, dense aggregations of demersal and benthopelagic fishes, and elevated abundances of zooplankton and micronekton (Clark et al., 2010). Moreover, seamounts are important habitats for species with commercial value since they can create large aggregates that spawn or feed in the vicinity, thus reducing the fishing effort (Clark et al., 2016). However, previous studies showed that seamount-aggregating fishes have higher intrinsic vulnerability to fishing due to their life history attributes, such as slow development, longer lifetime, later sexual maturation, and lower natural mortality (Morato et al., 2006).
Due to the ecological vulnerability of these habitats, the Portuguese government plans to classify the Great Meteor and the Madeira-Tore complexes as marine protected areas (MPAs) under the European Marine Strategy Framework Directive (Jorge et al., 2016). The status of fisheries at Great Meteor seamounts is still unclear, even though evidences of exploratory fishing were found. The fish species most commonly caught in this area are Macroramphosus scolopax, Capros aper, Trachurus picturatus, and Anthias anthias (Fock et al., 2002). At the Madeira-Tore complex, the shallower summits of the Gorringe Bank are the main attraction for fishing fleets. The wreckfish Polyprion americanus and the European conger, Conger conger, are captured during spring and summer, while in autumn and winter the swordfish Xiphias gladius and the black scabbard fish Aphanopus carbo are the main targeted species (Hermida and Delgado, 2016; Campos et al., 2019). Nevertheless, it seems that these fisheries may have reached their limits of geographic expansion and are already overexploited (Hermida and Delgado, 2016). For this reason, the design of these two new MPAs should help balance the exploitation by fisheries and conserve these seamount habitats (Probert et al., 2007).
For the purpose of MPA design, it is necessary to understand population dynamics and the hydrodynamic processes related to seamounts. During the last years, several studies have been focused on describing seamounts biological communities and assessing patterns of benthic biodiversity and marine biogeography (Fock et al., 2002; Wienberg et al., 2013; Christiansen et al., 2015). Samadi et al. (2006) suggested that the genetic exchange between submarine mountains is more frequent than previously assumed and may be related to the dispersal transport induced by ocean currents. The extent to which populations are connected is also determined by the scale, intensity, direction, and frequency of the dispersal pathways (physical factors) and by the post-settlement mortality, growth, and condition from settlement to successful reproduction (biological factors; Pineda et al., 2007; Etter and Bower, 2015). However, the wide variety of interconnected mechanisms that promote or prevent the movement of individuals and their contribution to the gene pool are still poorly known (Shank, 2010). Recent methodological advances in larval tagging, parentage analysis, and otolith microchemistry shed some light on marine connectivity over relatively short spatial and temporal scales. Nonetheless, these methodologies are very expensive since they require intensive spatial and temporal sampling. On the other hand, biophysical numerical models offer the possibility to track virtual particles over longer spatial and temporal scales. This type of approach will contribute to identify the main physical processes that serve to connect or isolate seamount populations (Shank, 2010) as well as to determine their source–sink relationships (Holstein et al., 2014).
In the northeast Atlantic Ocean, several studies focused on comprehending the oceanographic phenomena, as well as the transport and retention mechanisms, around several Portuguese small islands and archipelagos (e.g., Caldeira et al., 2002; Barbosa-Aguiar et al., 2011; Sala et al., 2013, 2016; Caldeira and Reis, 2017). However, none of them focused on the connectivity between seamount complexes. To better understand how persistent oceanographic features affect the recruitment and the connectivity between Madeira-Tore and Great Meteor seamount populations, our study investigates the physical connectivity patterns in the region. For this purpose, a series of numerical experiments was performed using the Connectivity Modeling System (CMS), an off-line Lagrangian tool, attached to an ocean circulation model (as described in detail in section “Data and Methods”). The results of the different numerical experiments are presented in section “Results,” and the general discussion and main conclusions are in section “Discussion and conclusions.”
Materials and Methods
Study Area and Oceanographic Context
Within the northeast (NE) Atlantic region (Figure 1a), the Great Meteor complex is located in the mid-Atlantic ridge, ∼900 km to the south of the Azores, and it is constituted by a series of extinct volcanoes rising from depths greater than 4,500 m to less than 300 m (Beckmann and Mohn, 2002; Geldmacher et al., 2006). The ancient volcanic cones are aligned in a north–south orientation. This assembly includes Atlantis, Plateau, Irving, Cruiser, Tyro, Hyères, and the Great Meteor, as well as the smaller Small-Meteor and the Closs bank (Figure 1b). In turn, Madeira-Tore complex, located ∼700 km off the northwestern African coast, is a northeast-aligned submarine ridge in the central east Atlantic that rises from more than 4,000 m in water depth to ∼35 m below the sea surface (Figure 1b). Several hypotheses have been proposed for its origin (Tucholke and Ludwig, 1982; Morgan, 1983; Peirce and Barton, 1991; Royden, 1993; King and Anderson, 1998), but there is a lack of age and geochemical data (Geldmacher et al., 2006). The complex is composed of the Gorringe bank (with two mountain tops: Gettysburg and Ormonde), Seine, Josephine, Lion, and Unicorn seamounts. The present study is mainly focused on the best-studied groups in this area: Great Meteor, Plateau–Irving–Cruiser (PIC), Tyro, Lion-Josephine, Unicorn-Seine, and Gorringe bank. Additionally, Ampere and Coral seamounts were included due to their proximity to the Madeira-Tore complex.
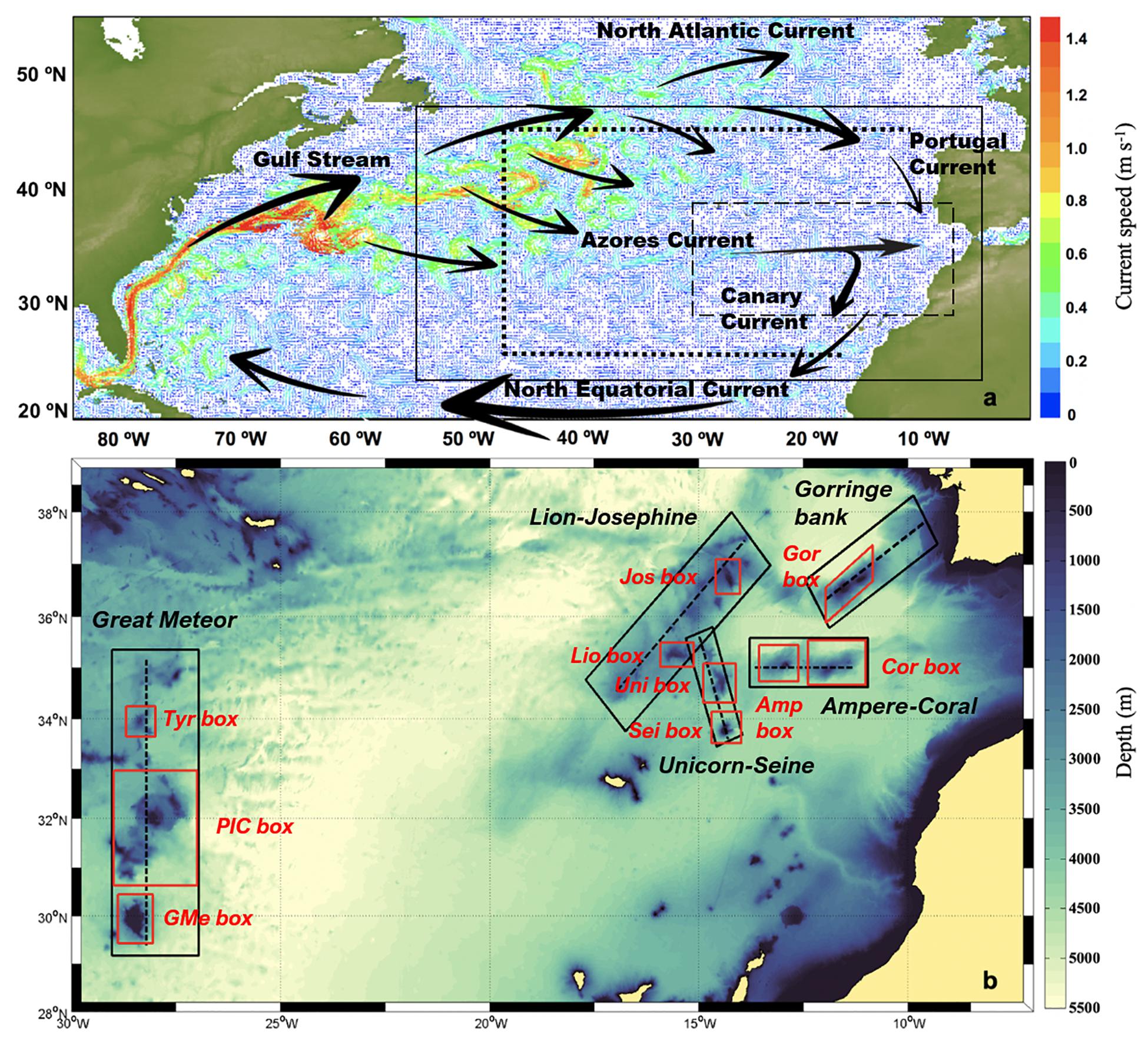
Figure 1. (a) Representative map of the study area that includes the North Atlantic Subtropical Gyre and its associated currents: the Gulf Stream, the North Atlantic Current, the Portugal Current, the Azores Current, the Canary Current, and the North Equatorial Current. The solid line defines the HYCOM domain. The dotted lines represent the release boundaries for the particle retention experiment. (b) Zoomed-in view of the area that contains the seamounts under study [the dashed line on panel (a)]. The dashed lines represent the release lines for the reverse particle experiment. The black boxes represent the sink boxes for the particle retention experiment. The red boxes represent the connectivity polygons defined for the connectivity study.
These deep-sea areas exist in a highly variable oceanographic environment characterized by the prevailing ocean currents and the mesoscale phenomena that result from the interaction of passing ocean flows with the seamount bathymetry. Both complexes, Great Meteor and Madeira-Tore, are affected by the Azores Current (AzC), which is one of the branches of the Gulf Stream (GS) that composes the North Atlantic Subtropical Gyre (Figure 1a; Käse and Siedler, 1982; Gould, 1985; Onken, 1992). The AzC splits in one branch that goes into the Gulf of Cádiz (GoC), toward the Strait of Gibraltar, and another that deviates southward, connecting with the Canary Current (CaC; Johnson and Stevens, 2000). The CaC also receives a small contribution from the Portugal Current (PoC; Barton et al., 2001), which spreads in the north–south direction, connecting the North Atlantic Current, the northeastward branch of the GS, and the AzC (Dietrich et al., 1980). This current regime, along with other phenomena such as ocean eddies, internal tides, and variable meteorological forcing, contributes to mesoscale variability and, ultimately, the different connectivity levels occurring between the seamounts (Clark et al., 2010; Barbosa-Aguiar et al., 2011; Caldeira and Reis, 2017). There are two main processes associated to these features: the (i) “island mass effect” (or in this case “seamount effect”; Doty and Oguri, 1956), which is responsible for the enhanced production occurring around small islands/seamounts in comparison to the surrounding waters, and the (ii) “sticky water effect” (Wolanski, 1994), which generates slower mean currents in the surrounding regions of high-density topography such as a coral reef and, on a different scale, a group of seamounts, leading to an increase of larvae recruitment and trapping in the regions of weaker flow (Clark et al., 2010; Lavelle and Mohn, 2010; Sala et al., 2016).
Ocean Model and Lagrangian Tool
In this study, we applied the HYbrid Coordinate Ocean Circulation Model (HYCOM),1 which is capable of solving the hydrostatic primitive equations in a free surface mode (Bleck, 2002; Chassignet et al., 2007). The US Navy Research Laboratory global solutions (GLBa0.08, experiment 05.8) were used with a horizontal grid resolution of ∼9.25 km (1/12°) and 32 vertical layers. For the off-line Lagrangian simulations, data were extracted from a sub-domain encompassing 22° to 47° N in latitude and 55° to 5° W in longitude (solid line in Figure 1a). A 9-year-solution was considered (2004–2012). This model was previously validated by comparison with Argo (global array of temperature/salinity profiling floats) profiles by Sala et al. (2016), who concluded that the model ensures a good representation of the known water masses and the ocean currents in the region.
The Connectivity Modeling System (CMS v2.0; Paris et al., 2013) was used to track passive particle movements. The CMS runs off-line, applying the 3D velocity fields (u, v, w) of the ocean circulation model to each particle using a 4th order Runge–Kutta numerical discretization method, applied both in space and time (Van Sebille et al., 2012; Paris et al., 2013). To represent the subgrid-scale motion (physical turbulence effects) unresolved by the model, a value of 2 m2 s–1 for horizontal diffusivity was added (following Sala et al., 2016). No vertical diffusivity was considered since these particles traveled in discrete layers while maintaining a constant depth. This Lagrangian tool was especially developed for larval dispersal modeling and to give probability estimates of population connectivity, with multiple modules available for the integration of biotic and abiotic data. Although some modules mimic larval behavior, none was activated in order to provide more accurate representations of the Lagrangian ocean circulation patterns around the Great Meteor and Madeira-Tore complexes. Therefore, the model was applied as a statistical representation of dispersal probabilities rather than as a deterministic study of individual particles and/or larval fates (Ross et al., 2016).
Reverse Particle Tracking Study
This case study was carried out to determine the origin of particles reaching these submarine archipelagos, Great Meteor and Madeira-Tore (also considering the Azorean seamounts), discerning the main mesoscale phenomena that influence the transport dynamics. For each seamount, a line following their longitudinal or meridional axis was considered (depending on their orientation, dashed lines in Figure 1b), with several release points defined at a regular spacing of 0.05° (∼5.55 km): for Great Meteor 117 release points were considered, Lion-Josephine with 63, Unicorn-Seine with 42, and Ampere-Coral and Gorringe bank with 49 release points, adding up to a total of 320 release points. During this experiment, 10 particles were released every month at four different depth layers (5, 150, 500, and 1,000 m) at each release point, summing up to a total of 192,000 particles released at each layer. These release depths represent the common transport pathways previously identified for the northeast Atlantic Ocean by Sala et al. (2013). Trajectories of individual particles were tracked monthly (“backward” in time) from December 31, 2012 until January 1, 2008, considering that 5 years was enough time for the slowest particles to exit the model domain.
Particle Retention Study
This case study was conducted in order to evaluate the capacity of each seamount to capture and retain incoming particles. For these experiments, the particles were released from several release points regularly separated by 1° (∼111.11 km) along the model domain boundaries: the North Boundary (NB) considered 39 release points, the West Boundary (WB) with 21, and the South Boundary (SB) with 32 (dotted lines in Figure 1a). At each release point, 10 particles were released every month at the same depth layers as the reverse particle case study. Therefore, in the NB, a total of 23,400 particles were released, in the WB 11,400 were released, and in the SB 19,200, were released. In this case, the trajectories were monitored (“forward” in time) also at a monthly interval from January 1, 2004 until December 31, 2008.
To assess the “capture capacity” and “retention time interval” of each group of seamounts relative to incoming particles, a set of five “sink boxes” was defined: Great Meteor, Lion-Josephine, Unicorn-Seine, Ampere-Coral, and Gorringe bank (Figure 1b). “Capture capacity” was calculated by counting the number of particles that reached each group of seamounts (i.e., each “sink box”), while the “retention time interval” (in days) was calculated as a frequency distribution, considering the number of days that each particle spent inside a “sink box.” In this experiment, the journey of a particle did not end once this particle enters a “sink box.” Each particle will continue its journey until the end of the 5-year simulation or until it leaves the model domain.
Connectivity Study
This experiment analyzed the degree of connectivity between the different seamounts. For that, particles were released from the inside of 10 different 3D polygons (Figure 1b) at four different depth ranges following Sala et al. (2013): 1–10, 20–200, 300–500, and 600–2,000 m. Those 10 polygons represent the main seamounts that are currently the focus of this study (i.e., Great Meteor, Plateau–Irving–Cruiser, Tyro, Lion, Josephine, Unicorn, Seine, Ampere, Coral, and Gorringe bank). At each polygon, 200 release points, randomly distributed (latitudinally, longitudinally, and in depth) and fixed in time, were considered. At every point, 10 particles were released at a monthly interval for 9 years (2004–2012) and tracked “forward” in time. A particle’s journey ends once it enters a polygon, but they can only settle 160 days after the release event. Connectivity matrices were calculated considering the number of particles released and captured by each polygon, reflecting the probability of a particle released from a given source box (i) to settle in a sink box (j), thus connecting the population i with the population j.
In order to quantify the physical connectivity within the network of seamounts, “source–sink indices” (SS) were calculated as the difference between the total number of particles being exported (Kout) and the total number of particles being imported (Kin) divided by the sum of the two (Holstein et al., 2014):
where K represents the successful settlement “in” (i.e., particles imported) or “out” (i.e., particles exported) of a seamount. Negative index values (SS < 0) indicate that the seamount imports more particles than it exports, acting as a net sink. On the other hand, positive index values (SS > 0) indicate that a seamount exports more particles than it imports, acting as a net source. Meanwhile, a value equal to 0 would indicate that the seamount imports as many particles as it exports.
The “self-recruitment” (selfr) and “subsidy-recruitment” (subr) fractions of each seamount were calculated to identify which seamounts rely most on their own recruitment capacity versus the ones that depend more on the connections with its surroundings to maintain the local populations.
The selfr fraction was determined as the proportion of total recruited particles to a seamount (i) that originated from the seamount itself (Andrello et al., 2013):
where c is the connection probability, i.e., the fraction of particles originating in the seamount i that ended up in the same location (c(i,i)) or in another seamount j (c(i,j)).
Meanwhile, the subr fraction was obtained as the proportion of total recruited particles that came from the surrounding seamounts (j) (Andrello et al., 2013), calculated as:
Thus, if selfr is higher (lower) than 0.5, there are more (less) particles retained by self-recruitment than by subsidy-recruitment.
Sensitivity Tests
Several sensitivity tests were performed in order to examine the limitations of the model. A first test to determine the seasonal and inter-annual variability for the capture and retention capabilities of the seamounts showed a small diversion for the bulk transport pathways. Six commonly used indices were computed to compare the model results and assess its performance: the correlation coefficient, root mean square error, reliability index, average error, average absolute error, and modeling efficiency [for more detailed information, see Stow et al. (2009)]. Notwithstanding, the tests were limited to the most superficial layer where ocean dynamics is more complex, assuming that there are no major changes with increasing depth. For the reverse particle tracking study, all possible particle pathways were recalculated with half of the particles, obtaining very similar dispersal patterns to those obtained with all the particles. For the particle retention and connectivity studies, the capture capability of each group of seamounts was recalculated through the same method, and similar results were obtained (Table 1). Although tens of thousands of particles were released per depth range, these tests proved that the model achieves statistical convergence using all or even half of the number of particles.
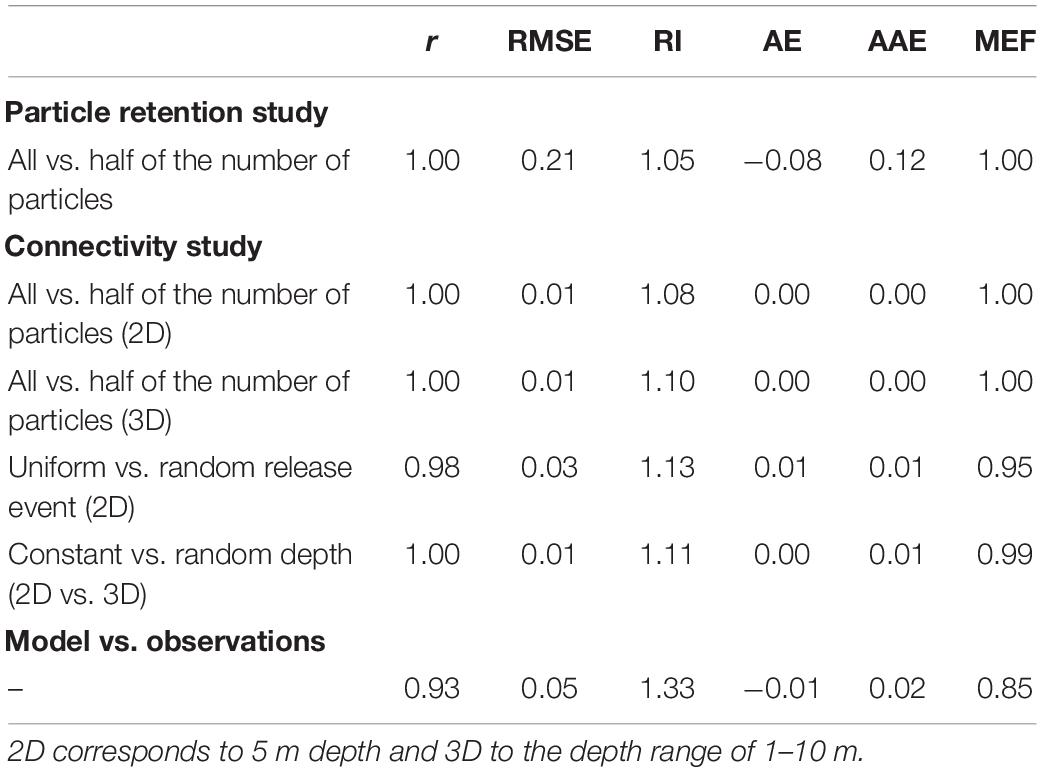
Table 1. Summary statistics, including the correlation coefficient (r), root mean square error (RMSE), reliability index (RI), average error (AE), average absolute error (AAE), and the modeling efficiency (MEF), of the sensitivity tests performed throughout the study.
Additional sensitivity tests were performed for the connectivity study. The connectivity matrices with particles that are uniformly and randomly released (with respect to x and y coordinates) were compared, and the results were similar (Table 1). With matrices in which the release events were set at a constant depth and at a random depth (z-coordinate) within the corresponding depth range (i.e., 1–10, 20–200, 300–500, and 600–2,000 m), the outcomes were also comparable (Table 1). Nevertheless, as this study is not species specific, which are often confined (due to behavior) to a certain depth range, random releases at different depth ranges were considered in order to achieve a generic approach.
Finally, a comparison between the model and surface drifting buoys data was conducted. A total of 772 drifting buoys trajectories were downloaded from the CORIOLIS database,2 taking into account the region (dashed line in Figure 1a) and the study period (2004–2012). Considering the release point of each drifting buoy (i.e., longitude, latitude, and time) and its travel time (31 days maximum), a CMS experiment was performed. The resulting connectivity matrix was compared with the one obtained from the drifters (see Figure 2), revealing a high correlation between the ocean circulation model and the drifting buoys data (see Table 1).
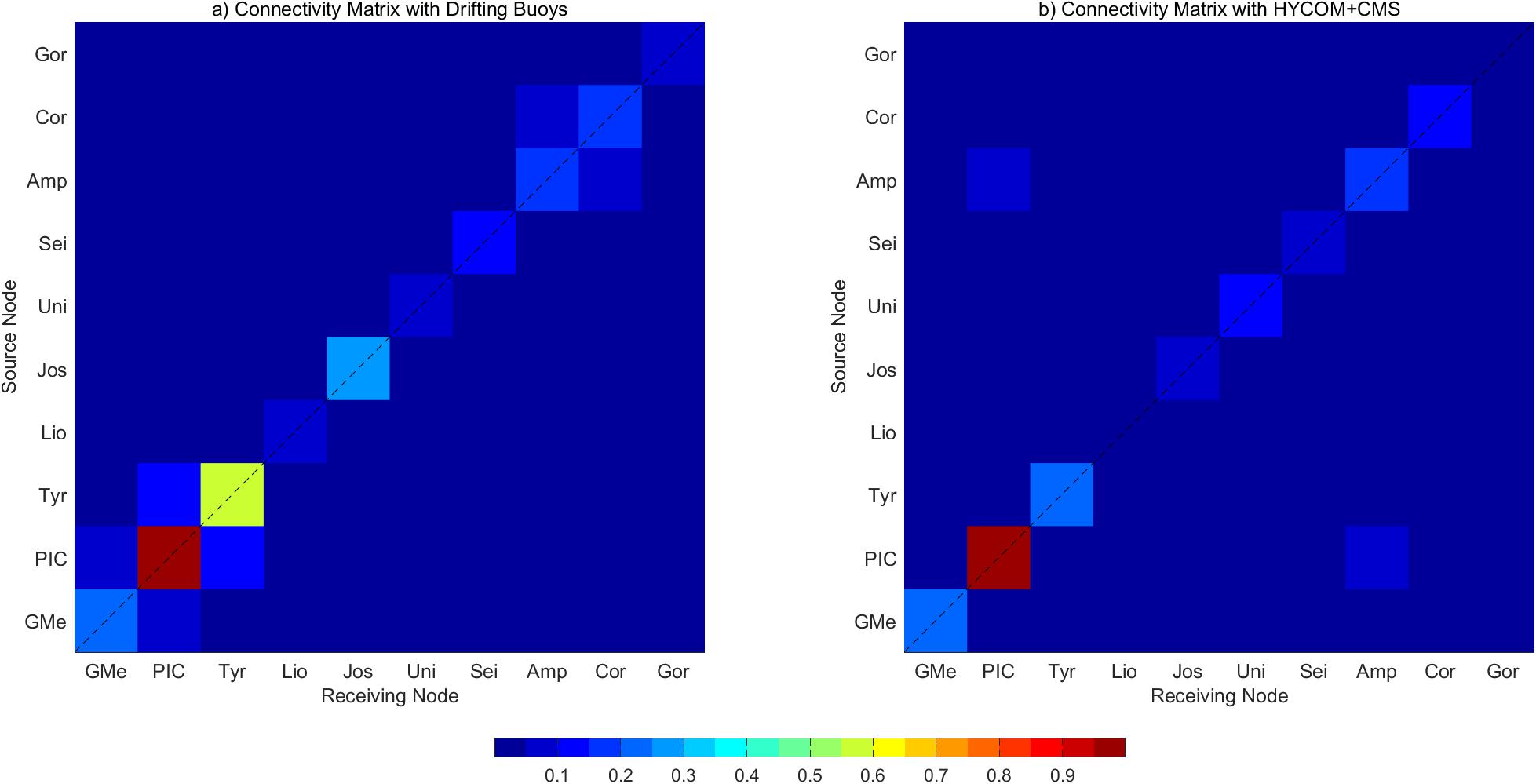
Figure 2. Connectivity matrices computed for the connectivity study area and the time period from 2004 to 2012. (a) Connectivity matrix computed with drifting buoys data from CORIOLIS. (b) Connectivity matrix computed with HYCOM and CMS.
The sensitivity studies, particularly the comparison with observations, gave the authors confidence to pursue a representative study of the physical connectivity between the NE Atlantic seamounts, of which the main findings are detailed in the section below.
Results
Reverse Particle Tracking Study
With regard to the Great Meteor complex located at ∼1,600 km from the nearest continental coast, at 5 m depth, the majority of the particles came from the west/northwest side of the domain (Figure 3a), whereas for the most coastal mountains (i.e., Lion-Josephine, Unicorn-Seine, Ampere-Coral, and Gorringe bank), the particles came mainly from the Iberian Peninsula (IP), GoC, and the northwest African coast (Figures 3b–e). A particularly high-density pathway was observed between the south IP and the Gorringe bank (Figure 3e). The trajectories for particles released at 150 and 500 m exhibited similar results (data not shown).
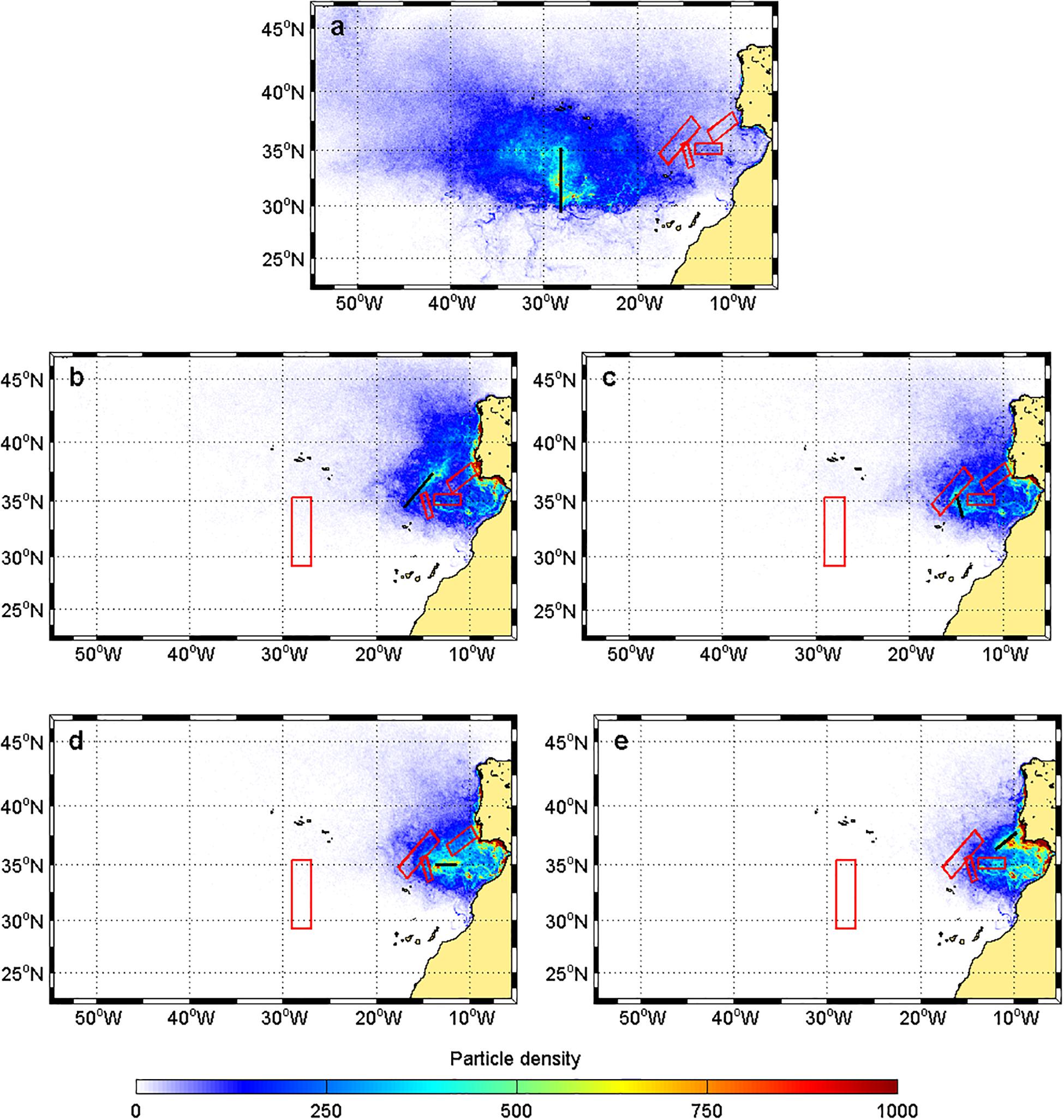
Figure 3. Particle density distribution for the reverse particle tracking study at 5 m depth for particles released at the different seamounts: (a) Great Meteor, (b) Lion-Josephine, (c) Unicorn-Seine, (d) Ampere-Coral, and (e) Gorringe Bank. The black bar corresponds to the final destination of the particles. The red boxes represent the nearby seamounts of interest (as identified in Figure 1b).
At 1,000 m depth, the particles came mostly from the west and the northeast toward the Great Meteor (Figure 4a). At the remaining seamounts, the particles arrived from the nearby regions (Figures 4b,c,e), with a major input from the southwest side (Figures 4b,d).
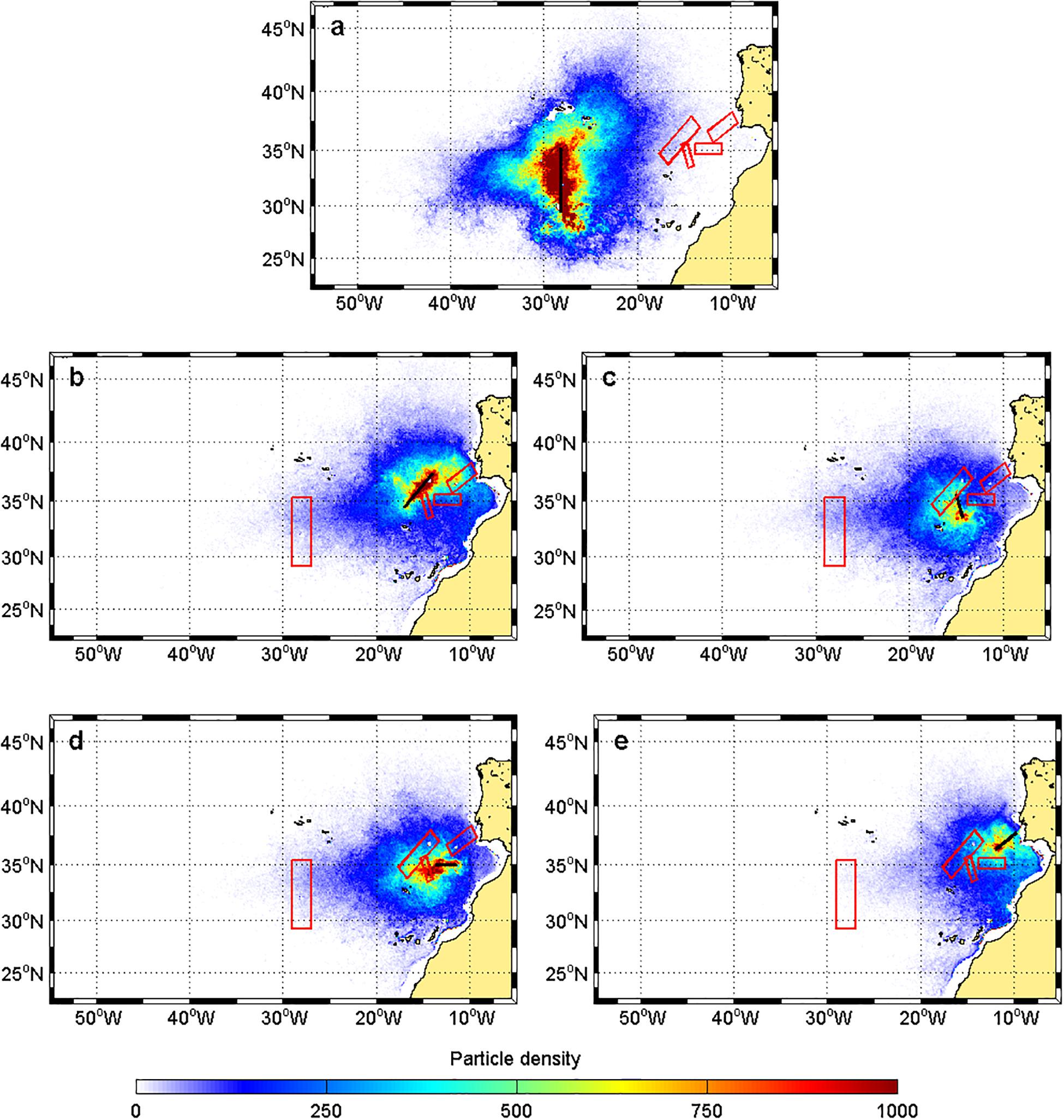
Figure 4. Particle density distribution for the reverse particle tracking study at 1,000 m depth for particles released at the different seamounts: (a) Great Meteor, (b) Lion-Josephine, (c) Unicorn-Seine, (d) Ampere-Coral, and (e) Gorringe Bank. The black bar corresponds to the final destination of the particles. The red boxes represent the nearby seamounts of interest (as identified in Figure 1b).
Particle Retention Capability Study
At Great Meteor, Lion-Josephine, and Unicorn-Seine seamounts, a higher percentage of particles was retained in the most superficial layer (5 m; Table 2). For almost all depths, the main source of particles was the NB, with the exception of the deepest layer where the SB outstood as the main contributor (Table 2). The captured particles spent a minimum of 30 days and a maximum of 250 days inside a box (Table 3). On the other hand, at Ampere-Coral and Gorringe bank, more particles were retained at intermediate waters (500 m; Table 2). The main origin of the total trapped particles for the first two depth layers (5 and 150 m) was the NB, whereas for the remaining two (500 and 1,000 m), the southern contribution exceeded the northern contribution (Table 2). The majority of the retained particles spent a minimum of 40 days and a maximum of 100 days inside one of these boxes (Table 3).
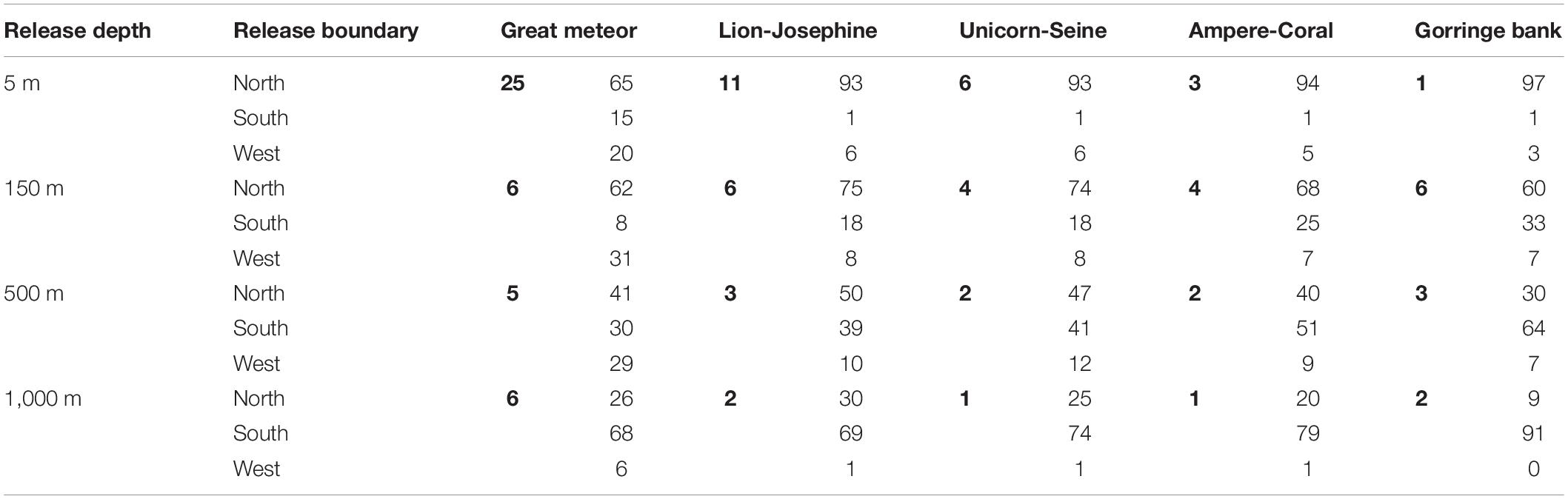
Table 2. Percentage of particles released along the domain boundaries (NB, WB, and SB) that were captured by the different sink boxes (in bold), with the corresponding percentages from each boundary, at each release depth, during the particle retention study.

Table 3. Retention time interval (d) that at least 80% of the captured particles spent inside each sink box, at each release depth, during the particle retention study.
Connectivity Study
The connectivity matrices calculated for each depth range are presented in Figures 5–8. In order to be dimensionless, each element was normalized by the highest number of recruited particles. Therefore, the scale ranges from 0 to 1, with 0 being the lack of connectivity. The X-axis and the Y-axis represent the different seamounts considered in this study, geographically arranged from southwest to northeast, with the main diagonal providing self-recruitment information. It is also important to note that each row represents a release seamount (source), while each column corresponds to a settlement seamount (sink). In addition, the SS index will determine if a seamount is acting as a sink or as a source of particles. Meanwhile, the selfr and subr fractions will distinguish if a seamount relies more on self-recruitment or on subsidy-recruitment, respectively.
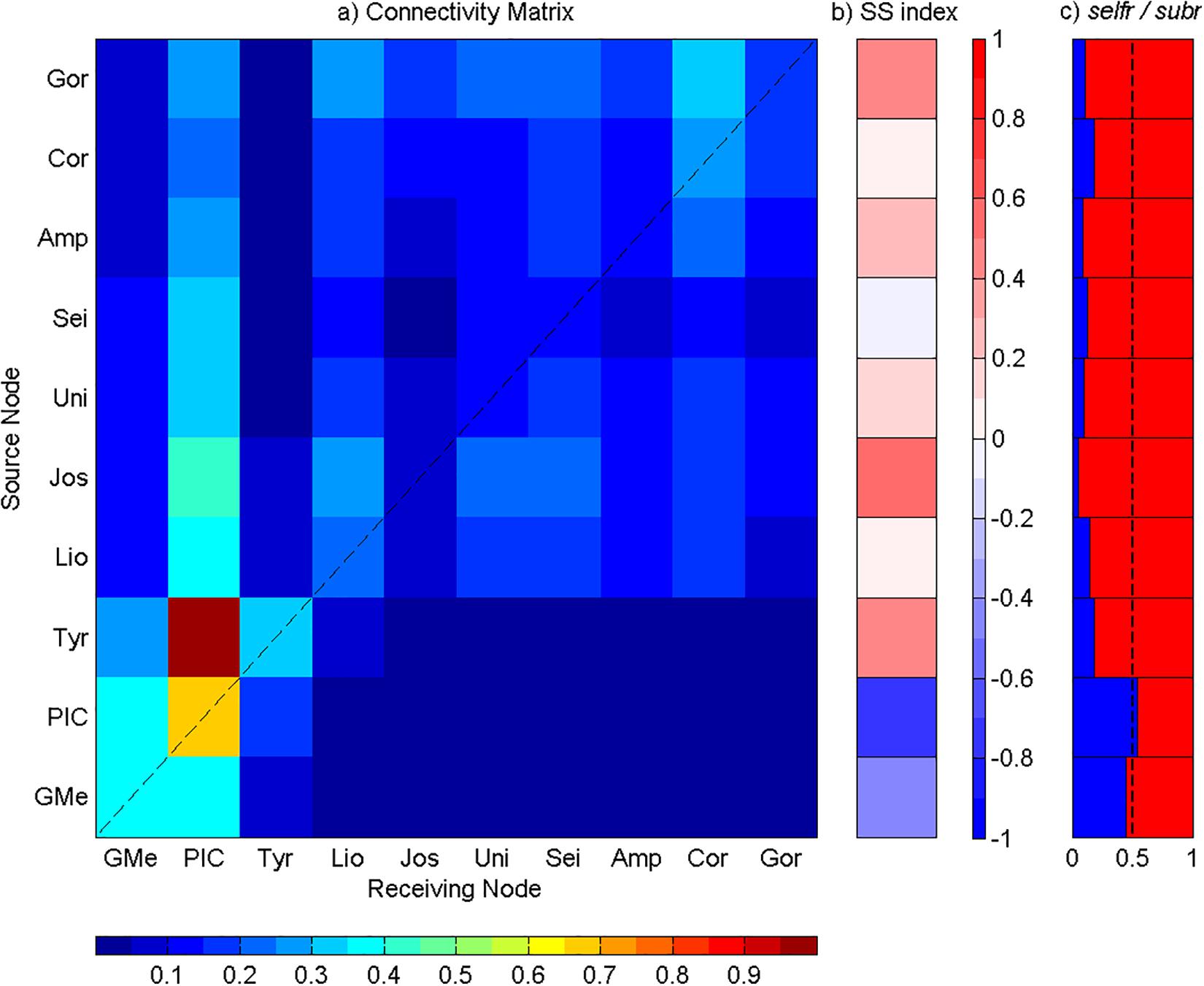
Figure 5. Analysis of the connectivity among Great Meteor and Madeira-Tore complexes at the depth range of 1 to 10 m. (a) Connectivity matrix between the seamounts: GMe – Great Meteor; PIC – Plateau, Irving, and Cruiser; Tyr – Tyro; Lio – Lion; Jos – Josephine; Uni – Unicorn; Sei – Seine; Amp – Ampere; Cor – Coral; and Gor – Gorringe. The y-axis represents seamounts acting as sources and the x-axis represents seamounts acting as sinks. The connectivity degree increases from blue to red. (b) Source–sink indices for each seamount. (c) Self-recruitment (selfr, blue color) and subsidy-recruitment (subr, red color) fractions for each seamount. The dashed line corresponds to the reference value of 0.5.
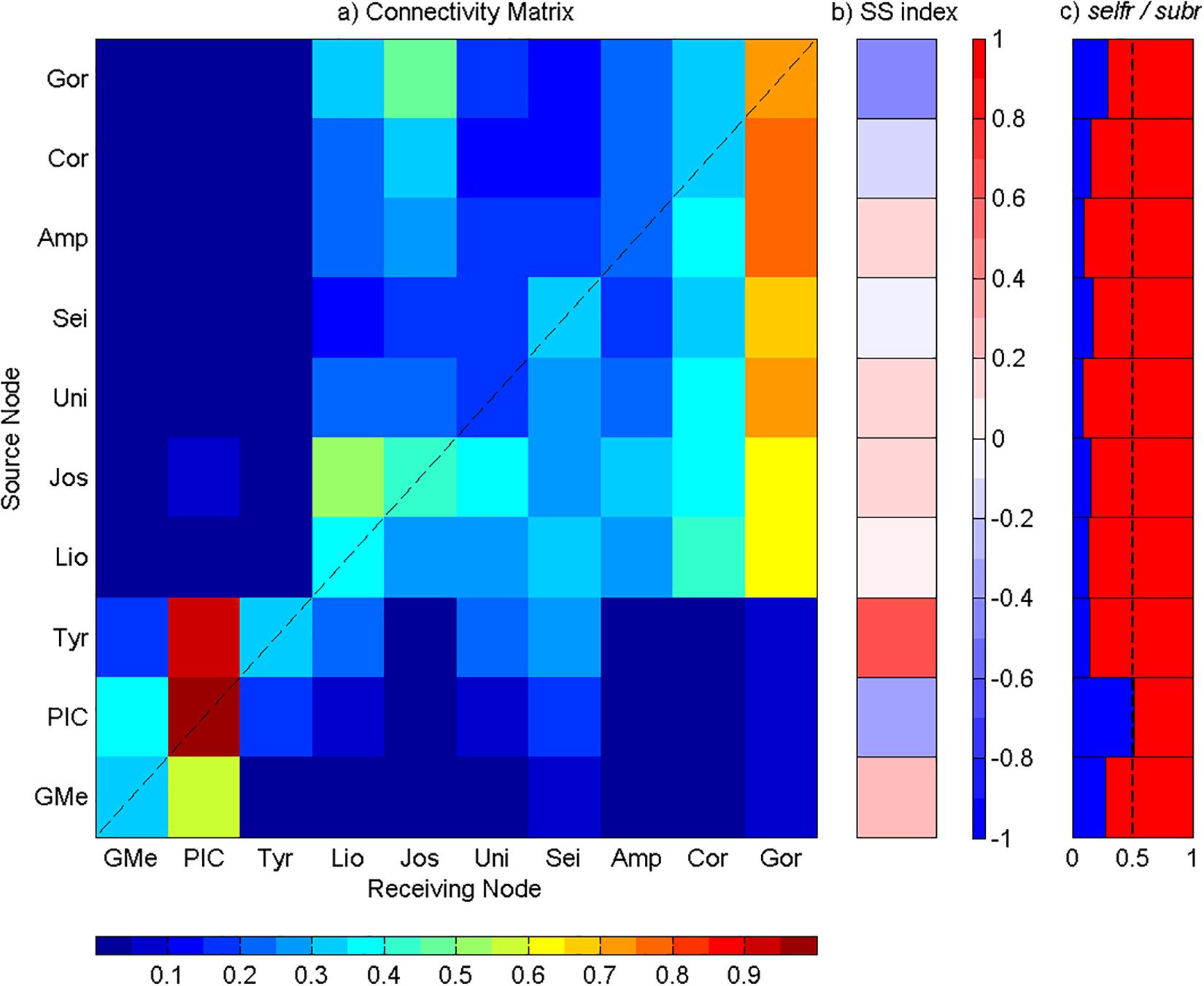
Figure 6. Analysis of the connectivity among Great Meteor and Madeira-Tore complexes at the depth range of 20 to 200 m. (a) Connectivity matrix between the seamounts: GMe – Great Meteor; PIC – Plateau, Irving, and Cruiser; Tyr – Tyro; Lio – Lion; Jos – Josephine; Uni – Unicorn; Sei – Seine; Amp – Ampere; Cor – Coral; and Gor – Gorringe. The y-axis represents seamounts acting as sources and the x-axis represents seamounts acting as sinks. The connectivity degree increases from blue to red. (b) Source–sink indices for each seamount. (c) Self-recruitment (selfr, blue color) and subsidy-recruitment (subr, red color) fractions for each seamount. The dashed line corresponds to the reference value of 0.5.
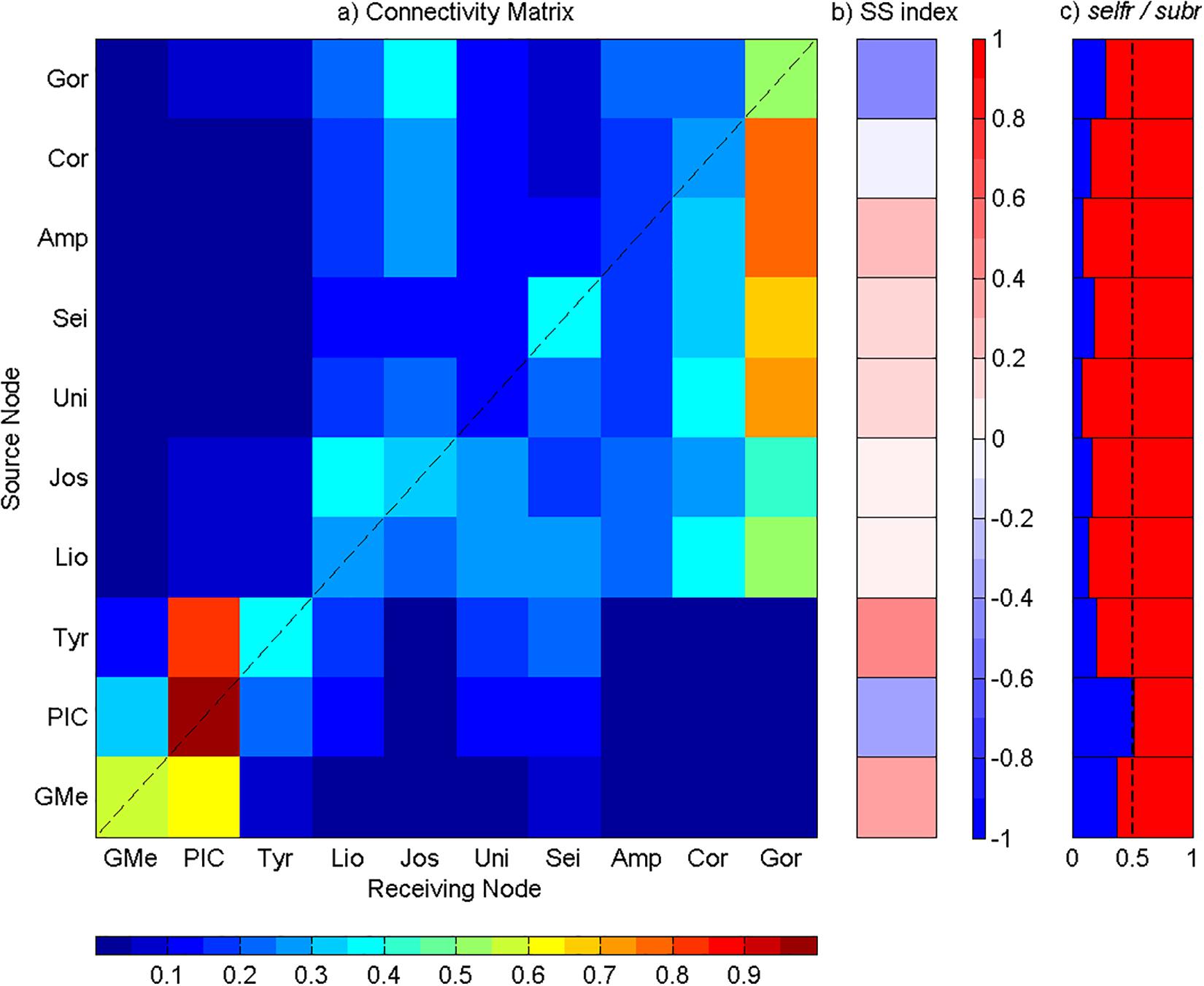
Figure 7. Analysis of the connectivity among Great Meteor and Madeira-Tore complexes at the depth range of 300 to 500 m. (a) Connectivity matrix between the seamounts: GMe – Great Meteor; PIC – Plateau, Irving, and Cruiser; Tyr – Tyro; Lio – Lion; Jos – Josephine; Uni – Unicorn; Sei – Seine; Amp – Ampere; Cor – Coral; and Gor – Gorringe. The y-axis represents seamounts acting as sources and the x-axis represents seamounts acting as sinks. The connectivity degree increases from blue to red. (b) Source–sink indices for each seamount. (c) Self-recruitment (selfr, blue color) and subsidy-recruitment (subr, red color) fractions for each seamount. The dashed line corresponds to the reference value of 0.5.
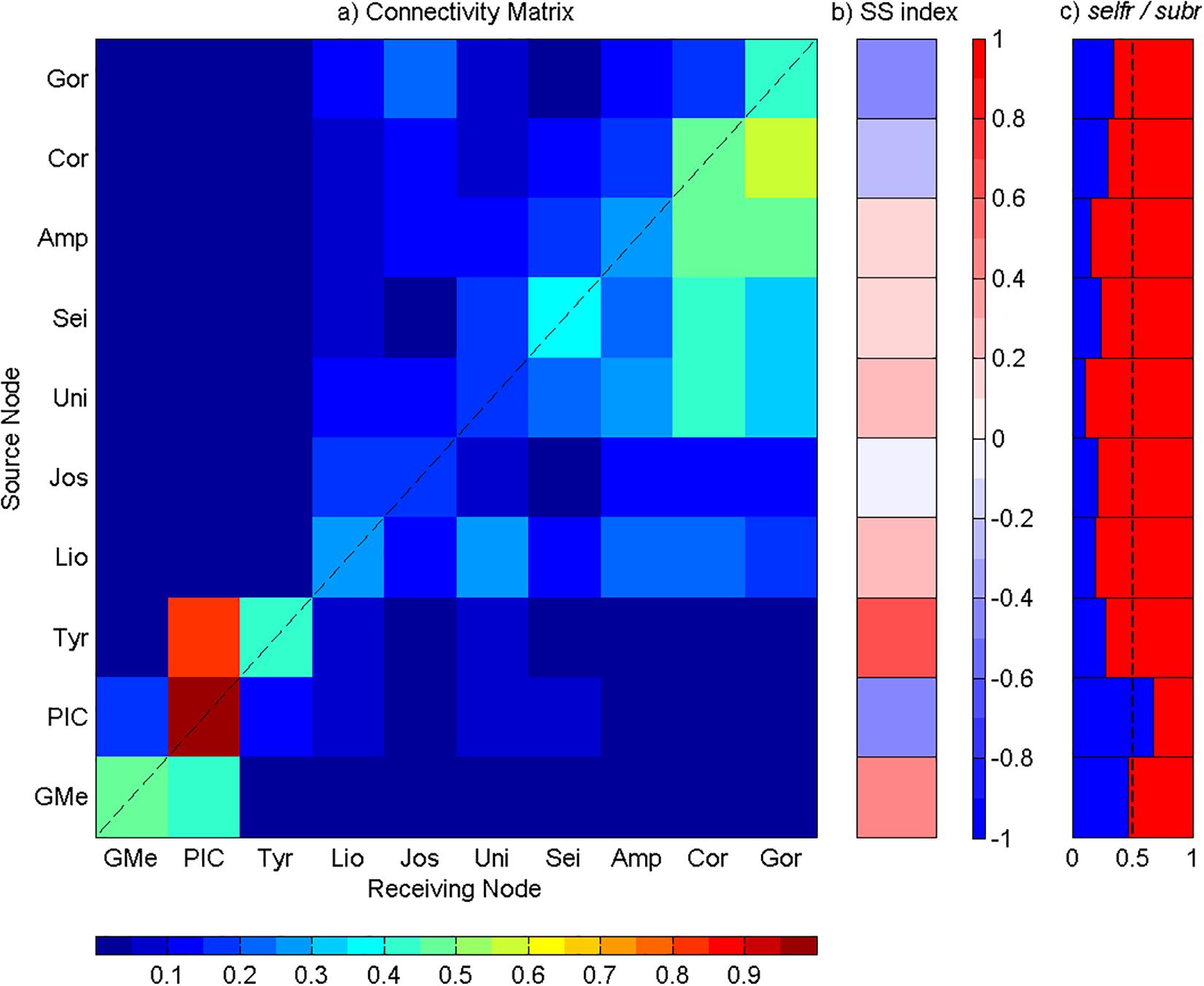
Figure 8. Analysis of the connectivity among Great Meteor and Madeira-Tore complexes at the depth range of 600 to 2,000 m. (a) Connectivity matrix between the seamounts: GMe – Great Meteor; PIC – Plateau, Irving, and Cruiser; Tyr – Tyro; Lio – Lion; Jos – Josephine; Uni – Unicorn; Sei – Seine; Amp – Ampere; Cor – Coral; and Gor – Gorringe. The y-axis represents seamounts acting as sources and the x-axis represents seamounts acting as sinks. The connectivity degree increases from blue to red. (b) Source–sink indices for each seamount. (c) Self-recruitment (selfr, blue color) and subsidy-recruitment (subr, red color) fractions for each seamount. The dashed line corresponds to the reference value of 0.5.
Particles Released Between 1 and 10 m
At this depth range, the intra-seamounts connectivity was stronger in the Great Meteor complex (i.e., Great Meteor, Plateau, Irving, Cruiser, and Tyro) than in the Madeira-Tore complex (i.e., Lion, Josephine, Unicorn, Seine, Ampere, Coral, and Gorringe). A higher self-recruitment was found for the western group of seamounts (i.e., Great Meteor complex; main diagonal, Figure 5a). The Great Meteor and PIC acted as net sinks, with the highest self-recruitment (∼0.37 for both) in comparison to the other seamounts (Figure 5a). These results coincided with the computed SS index < 0 for both mountains, which is indicative of a sink behavior (Figure 5b). For the PIC mountains, the main sources of recruited particles were Tyro, Josephine, Lion, and Great Meteor seamounts. With the exception of Great Meteor, those mountains acted mainly as net sources (SS > 0) and were characterized by low selfr values (Figures 5b,c). Although the PIC seamounts acted as net sinks (SS < 0; Figure 5b), they also presented the highest selfr value, retaining slightly more of their own particles rather than from other seamounts (selfr > 0.5; Figure 5c). Tyro presented the lower value of self-recruitment of the Great Meteor complex (0.31; main diagonal, Figure 5a), which coincides with a positive value of SS index (i.e., acted as source; Figure 5b). Additionally, it revealed a relatively low value of selfr (Figure 5c). On the eastern group of seamounts, where the interaction intra-seamounts was very weak, a stronger connection occurred between the Gorringe bank and Coral patch. For the Coral patch, the highest input of particles came from Gorringe bank, followed by a high self-recruitment pattern, with a low contribution from the remaining sites (Figure 5a). Thus, Gorringe acted as a net source (SS > 0), with a low selfr fraction (Figures 5b,c). As expected, a higher recruitment was observed for seamounts geographically closer to the source (Figure 5a). Moreover, most of the recruited particles were above the main diagonal, evidencing a westward transport.
Particles Released Between 20 and 200 m
At this depth range, the intra-seamounts connectivity from both complexes was intensified, especially in the eastern complex (i.e., Madeira-Tore complex). The exchange of particles between both groups was still present, although most of the recruited particles were below the main diagonal, evidencing an eastward transport (Figure 6a). On the western group, at Great Meteor, the input of particles from PIC slightly exceeded its self-recruitment (Figure 6a). However, Great Meteor acted as a net source (SS > 0), exporting more than it imports (Figure 6b). Moreover, its low selfr fraction proved that more particles from other mountains were retained than its own (Figure 6c). PIC showed the highest self-recruitment value of all mountains (Figure 6a). Besides that, it also received a large contribution from Tyro and Great Meteor (Figure 6b). Thus, PIC showed a selfr fraction value close to 0.5, confirming that the number of particles self-recruited was similar to the number of particles imported from other seamounts (Figure 6c). Meanwhile, Tyro acted as a source, showing a SS index close to 1, which means that the proportion of exported particles was much larger than the imported ones (Figure 6b). At the eastern group of seamounts (Madeira Tore complex), the self-recruitment of almost all mountains was higher at these depths when compared to the most superficial layer. The main source of particles for Lion was Josephine, with a contribution slightly superior than its own self-recruitment (Figure 6a), whereas for Josephine, the input from Gorringe was higher than its own self-recruitment (Figure 6a). Meanwhile, for Unicorn, the greatest input came from Josephine and Lion (0.35 and 0.29, respectively; Figure 6a). For Seine seamount, self-recruitment exceeded inputs from other sources (Figure 6a). Lastly, Gorringe bank acted as the main sink (Figure 6b) for particles released by the surrounding submarine mountains (Figure 6a). Thus, all the eastern group seamounts, with the exception of Gorringe Bank and Coral patch, acted as net sources, albeit the relatively low values (Figure 6b), although at <0.5, Gorringe Bank showed the highest selfr fraction, importing more particles from the surrounding mountains than its own via self-recruitment (Figure 6c).
Particles Released Between 300 and 500 m
The results obtained at this depth-range were very similar to those presented previously. Again, a strong eastern transport was observed. Higher values of connectivity were found intra-seamounts of the western group (i.e., Great Meteor, PIC, and Tyro), while for the eastern group, the strongest connections were established mainly with Gorringe bank (Figure 7a). High self-recruitment was observed (Figure 7a) at the western group, especially in PIC, which continued to act as the main sink (SS < 0) in the Great Meteor complex (Figures 7a,b). PIC also presented similar values of selfr and subr fractions, with the number of self-recruited particles concurrent to the number of imported particles (Figure 7c). At the eastern group of seamounts, Gorringe stood out once again as the main sink for particles released in the surrounding submarine mountains, therefore with a selfr value lower than subr (Figure 7).
Particles Released Between 600 and 2,000 m
Once more the results obtained for this depth range were similar to the previous ranges. However, the intra-seamounts connectivity in both complexes and the eastward connectivity between them were less intense (Figure 8a). All mountains at the western group, particularly PIC stood out, showing a high self-recruitment (Figure 8a), and acting as the main sink (SS < 0) (Figures 8a,b). Nevertheless, the selfr fraction was higher than 0.5, with the number of self-recruited particles greater than the number of imported particles (Figure 8c). At the eastern group, a higher connectivity was observed for Unicorn, Seine, Ampere, Coral, and Gorringe Bank, with the last two seamounts acting mostly as sinks (Figures 8a,b).
Discussion and Conclusions
Recent studies raised concerns on the vulnerability of seamount communities to human impact due to the intrinsic biological factors characteristic of seamount species (e.g., slow growth rates or late maturation; Rogers, 2004; Clark et al., 2010). Of special distress are the consequences of the development of large-scale bottom trawl fishing for sessile fauna (Clark et al., 2010), with coral reefs being particularly vulnerable to damage (Rogers et al., 2007). In the NE Atlantic seamounts, the level of endemism in coral species is very low (<3%), which means that populations rely on external contributions. In addition, the fauna associated to them is significantly different from that recorded at the same depths on the continental slope (Hall-Spencer et al., 2007). Thus, it is necessary to advance in the protection of these (less abundant) native species. In order to further perceive how these species are sustained, understanding the influence of the oceanographic phenomena in the connectivity between the different seamounts located in this region is of special interest. This study proposes a first approximation to pursue this goal using a numerical modeling approach, focusing on the Great Meteor and Madeira-Tore complexes.
Due to the geographical location of Great Meteor and Madeira-Tore, our results highlighted the main role of the AzC and their associated eddies in the transport, dispersion, and retention of particles in this region, as well as their influence in the connectivity among the different seamounts that comprise those complexes (Figure 1). The Great Meteor complex, located offshore ∼1,500 km away from the African coast, is influenced by both branches of the AzC: the one that travels eastward toward the GoC and the one that veers southward near Madeira. The Madeira-Tore complex, located ∼550 km away from the IP coast, is mainly influenced by the eastward branch. When this eastward branch arrives at the GoC, it produces a front characterized by high mesoscale variability, probably being responsible for the transport of particles from the coastal regions of the GoC toward this complex (Figures 3, 4). Moreover, although on a small scale, the PoC also influences the Madeira-Tore dynamics, transporting particles from the western coast of the IP to the open ocean.
The AzC and its associated eddies are the main regional features injecting particles in our study area; thus, the highest input from the north and west boundaries was expected. Our results showed that the NB was the main source of captured particles by all seamounts at the upper layers (i.e., 5 and 150 m; Table 2). However, the contribution from the WB was only evident at the Great Meteor complex (Table 2) since it is located closer to this boundary (∼2,000 km) than Madeira-Tore (∼3500 km). An intensified AzC between 150 and 500 m in depth and 32° and 35° N (Klein and Siedler, 1989; Comas-Rodríguez et al., 2011; Sala et al., 2016) resulted in the increase of captured particles from the WB by all seamounts. Due to the decrease in intensity of the AzC with depth, a lower influence was observed at 1,000 m depth. Moreover, the released particles had a maximum travel time of 5 years that proved to be insufficient to travel a greater distance (Figure 4d and Table 2). While the NB contribution decreased with depth, the contribution from the SB increased due to the intensification of the north-westward and westward transport mediated by the eddy corridors also associated with the AzC (Sangrà et al., 2009; Barbosa-Aguiar et al., 2011; Comas-Rodríguez et al., 2011; Sala et al., 2016).
The capture capacity (i.e., number of particles that reached each sink box) and the retention time interval (i.e., the number of days that each particle spent inside a sink box) were only partially related to seamount size as they depend also on the proximity of each seamount to the different source boundaries and on the predominant oceanic processes determining their oceanic pathways. As expected, the percentage of captured particles decreased with depth (Table 2), while the retention time increased for all boxes except at the Gorringe bank, which did not show a clear pattern of variability (Table 3). In the upper mixed layer (i.e., 5 m), Great Meteor (the largest box) withheld 25% of captured particles, followed by Lion-Josephine (twice as small as the Great Meteor) with 11%. Besides the higher capture capabilities, they also had higher mean retention times. Meanwhile, Unicorn-Seine, the smallest sink box (seven times smaller than Great Meteor), showed the third highest capture capacity (6%; Table 2) but with a shorter retention time interval (0–30 days; Table 3). At the other release depths, the variability between the different sink boxes was lower (Tables 2, 3). Notwithstanding, when the capture capacity per square kilometer is calculated for all the sink boxes, Unicorn-Seine stands out with the highest values at all depths (Figure 9). Great Meteor (the largest box) only exceeds in the most superficial layer. From 150 to 1,000 m deep, the smallest sink boxes (Gorringe bank, Ampere-Coral, and Unicorn-Seine, from large to smaller-sized seamounts) showed a capture capacity (per km2) greater than the larger sink boxes (i.e., Great Meteor and Lion-Josephine). This higher capture capacity of the smallest sink boxes could be related to their location and orientation with respect to the incoming AzC (Figure 1). In addition, these aggregated seamount chains are influenced by the “sticky water effect,” which increases their ability to capture and retain incoming particles, including coral eggs and fish larvae (Andutta et al., 2012). However, as their delimited areas are smaller, the particles stay for a shorter time. So, the particles are likely to be retained longer on seamounts occupying larger areas. While the Madeira-Tore complex is mainly influenced by the “sticky water effect,” the Great Meteor complex, due to its orientation to the incoming AzC, must be stirring the incoming current and causing a “seamount effect,” a process analogous to the “island mass effect”, which enhances productivity in the lee of oceanic islands (see e.g. Dower and Mackas, 1996). Other physical processes, such as lower rates of flow during neap tides, can still induce particle retention (Andutta et al., 2012). Thus, future studies could consider variable flow rates (e.g., tidal currents).
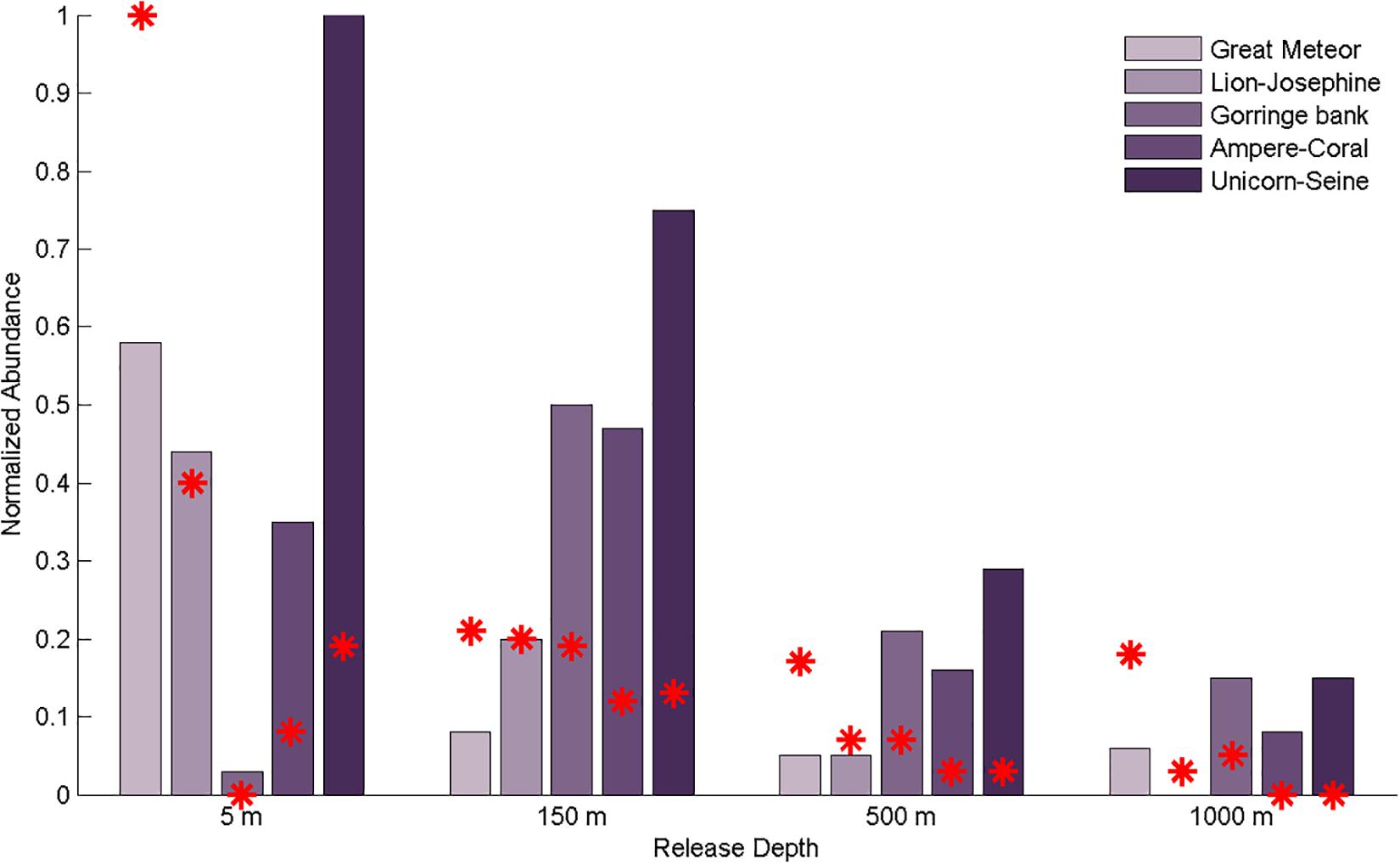
Figure 9. Bar plot representing the captured particles per square kilometer, per sink box, by depth. The red stars represent the total number of captured particles per sink box. The sink boxes are arranged in descending order of sizes (i.e., Great Meteor, Lion-Josephine, Gorringe bank, Ampere-Coral, and Unicorn-Seine). Both datasets were normalized so as to be comparable.
Considering the results, the connectivity between both complexes and the intra-seamount interaction should be stronger following the direction of the predominant stream/flow. Nevertheless, it should be noted that the complexes are ∼1,000 km apart, and assuming a constant speed for the AzC of 50 cm m–1 (Pingree, 1997), a particle would need at least ∼23 days to travel from one complex to another. At the upper mixed layer (5 m depth), a westward connectivity was observed between Great Meteor and Madeira Tore, with PIC seamounts being the main sink (Figure 5). This can be related to the westward flow previously reported by Barbosa-Aguiar et al. (2011) between 18° and 24° W along 32.5° N. This counterflow feeds on a southward recirculation east of Madeira, travels westward, and veers to the south at about 24° W. However, when AzC is intensified (i.e., 20 and 500 m depth), the connectivity seemed to follow an eastward pattern from Great Meteor complex toward Madeira-Tore (Figures 6, 7), whereas in deeper layers (600–2,000 m), where the currents are slower, there was almost no interaction between the two submerged archipelagos (Figure 8).
Regarding the intra-seamount connectivity, this interaction may depend on the submesoscale processes associated with AzC, given the almost perpendicular orientation of the Great Meteor complex (Great Meteor, PIC, and Tyro) relative to the current. In fact, previous observations have shown that the AzC recirculates in westward counterflows located both north and south of the main current, which might enable north–south as well as south–north interactions (Pingree, 1997). This intra-seamount connectivity did not vary substantially with depth. The PIC mountains always stood out with the strongest self-recruitment values, concurrently acting as the main sink for Tyro and Great Meteor, probably because of its larger volume compared with the others (see Figure 1b). The PIC and Great Meteor polygons are closer than PIC and Tyro, allowing a stronger interaction.
Meanwhile, the connectivity within the Madeira-Tore complex should be higher, considering that the seamounts are directly exposed to the AzC flow (Figure 1). At the most superficial layer, the intra-seamount connectivity was very weak. Furthermore, at this depth, all of them acted as net sources. This pattern probably relates with the fact that the particles could only settle 160 days after the release event (Figure 5). However, intra-seamount connectivity increased with depth, being strongest when the AzC is more intense (i.e., 20 and 500 m; Figures 6, 7). Between 20 and 2000 m depth, Gorringe and Coral seamounts, the two polygons closest to the GoC, acted as prime sinks of this complex. Meanwhile, the other mountains (sited further west) continued to act as sources.
The design of MPAs seems an appropriate way to protect the Great Meteor and Madeira-Tore complexes as vulnerable habitats, helping to balance exploitation and conservation (Probert et al., 2007). According to previous studies, their design should (i) assess the necessary degree of conservation, (ii) define the goals that justify the establishment of new MPAs, (iii) integrate the biological information of threatened species, such as dispersal distances and overall distribution, and (iv) select suitable areas to create MPA networks for biodiversity conservation and fishery management (Jones et al., 2007; Clark et al., 2011). Nonetheless, to maximize the success and benefits of these ocean sanctuaries, knowledge about larval retention and connectivity should also be considered (Jones et al., 2007). In this context, our results provide valuable information for this goal. Only physical connectivity was considered in our study, and the particles behaved as passive tracers without any ecological behavior. Therefore, our results will only be relevant for species with at least one planktonic pelagic phase (i.e., unable to swim against a current). Previous studies highlighted the roles of larval behavior and duration in dispersal and connectivity control (Fox et al., 2016; Lett et al., 2019; Taninaka et al., 2019). For instance, vertical larval migration may allow deep populations from nearby seamounts to repopulate shallow areas impacted by overfishing, which might increase the intra-connectivity within each seamount system (Fox et al., 2016). On the other hand, these swimming abilities might disperse larvae over long distances (Majoris et al., 2019), possibly increasing the inter-connectivity between the two systems. Longer-living larvae are expected to travel greater distances, thus enhancing the existing connectivity pathways (Fox et al., 2016). For this reason, future studies including biological factors (i.e., diel vertical migration, reproduction season, pelagic larval duration, and mortality rates) should target a selection of key species with known and/or well-documented behavior. Apart from focusing on a generic first study, the scientific literature on specific species behavior for Great Meteor and Madeira-Tore seamounts is virtually inexistent. On the other hand, considering that our results showed a very low connectivity between the Great Meteor and Madeira-Tore complexes, the connectivity patterns with the surrounding landscape should continue to be further investigated, for instance, in relation to the Macaronesian Islands and the western Iberian and African coasts. This will allow us to better understand the regional dynamics, including the proposed stepping-stone theory whereby the North Atlantic populations expand to the different islands through seamount chains (e.g., Cho and Shank, 2010).
Summary
• The AzC and its associated eddies were identified as the main transport pathways for particles on both groups of seamounts, especially at intermediate waters.
• The Great Meteor complex was mainly affected by the AzC southward branch, while the Madeira-Tore was mainly influenced by its eastward branch.
• Low connectivity was found between the two seamount complexes.
• The inter-connectivity between seamounts increased with depth at Madeira-Tore, while minor variations were found at Great Meteor.
• The PIC seamounts from the Great Meteor complex and Gorringe and Coral from the Madeira-Tore complex led the main connectivity routes.
• Despite their size, the Madeira-Tore seamounts outstood the others with its higher capturing capacity per square kilometer, suggesting a greater influence of the “sticky water effect.”
• In the Great Meteor complex, the “seamount effect” seems to be responsible for the greater retention and self-recruitment capabilities.
• These results provide a valuable first approach, from the (generic) physical point of view, to support MPA design efforts. However, since no coherent biological traits were found and/or considered, strong conclusions cannot be drawn at this stage.
• Future species-specific research including biological factors such as diel vertical migration, reproduction season, pelagic larval duration, and mortality rates are imperative.
Data Availability Statement
The dataset analyzed in this study (HYCOM GLBa0.08, experiment 05.8), from 2004 to 2012, cannot be provided since only the latest data are publicly available. The dataset used for the sensitivity analysis was downloaded from CORIOLIS database (http://www.coriolis.eu.org/Data-Products/Data-Delivery/Data-selection), adapted to our time and spatial domains.
Author Contributions
RC proposed the original ideas which lead to the design of the study. ML and IS were involved in the modeling, data collection, and analysis. All authors were involved in the writing and review process and all authors approved it for publication. An earlier version of this work was part of ML bachelor final project.
Funding
The BIOMETORE Project (PT02-0018) has partially funded this work. ML and RC were financially supported by the Oceanic Observatory of Madeira Project (M1420-01-0145-FEDER-000001-Observatório Oceânico da Madeira-OOM). IS was supported by a grant of the FPI fellowship program.
Conflict of Interest
The authors declare that the research was conducted in the absence of any commercial or financial relationships that could be construed as a potential conflict of interest.
Acknowledgments
We would like to thank Rui Vieira, for the support with computational demands, as well as Margarida Hermida and Cláudio Cardoso, for their useful and constructive recommendations. Special thanks to Luís Nunes and Ana Barbosa for their advice on statistical analysis.
Footnotes
References
Andrello, M., Mouillot, D., Beuvier, J., Albouy, C., Thuiller, W., and Manel, S. (2013). Low connectivity between mediterranean marine protected areas: a biophysical modeling approach for the dusky grouper Epinephelus marginatus. PLoS One 8:e68564. doi: 10.1371/journal.pone.0068564
Andutta, F. P., Kingsford, M. J., and Wolanski, E. (2012). Sticky water’ enables the retention of larvae in a reef mosaic. Estuar. Coast. Shelf Sci. 101, 54–63. doi: 10.1016/j.ecss.2012.02.013
Barbosa-Aguiar, A. C., Peliz, A. J., Cordeiro Pires, A., and Le Cann, B. (2011). Zonal structure of the mean flow and eddies in the Azores Current system. J. Geophys. Res. Ocean. 116, 1–14. doi: 10.1029/2010JC006538
Barton, E. D., Flament, P., Dodds, H., and Mitchelson-Jacob, E. G. (2001). Mesoscale structure viewed by SAR and AVHRR near the Canary Islands. Sci. Mar. 65, 167–175. doi: 10.3989/scimar.2001.65s1167
Beckmann, A., and Mohn, C. (2002). The upper ocean circulation at Great Meteor Seamount. Part II: Retention potential of the seamount-induced circulation. Ocean Dyn. 52, 194–204. doi: 10.1007/s10236-002-0018-3
Bleck, R. (2002). An oceanic general circulation model framed in hybrid isopicnic-Cartesian coordinates. Ocean Model. 4, 55–88. doi: 10.1016/s1463-5003(01)00012-9
Caldeira, R. M. A., Groom, S., Miller, P., Pilgrim, D., and Nezlin, N. P. (2002). Sea-surface signatures of the island mass effect phenomena around Madeira Island. Northeast Atlantic. Remote Sens. Environ. 80, 336–360. doi: 10.1016/S0034-4257(01)00316-9
Caldeira, R. M. A., and Reis, J. C. (2017). The Azores CONFLUENCE Zone. Front. Mar. Sci. 4:37. doi: 10.3389/fmars.2017.00037
Campos, A., Lopes, P., Fonseca, P., Figueiredo, I., Henriques, V., Gouveia, N., et al. (2019). Portuguese fisheries in seamounts of Madeira-Tore (NE Atlantic). Mar. Policy 99, 50–57. doi: 10.1016/j.marpol.2018.10.005
Chassignet, E. P., Hurlburt, H. E., Smedstad, O. M., Halliwell, G. R., Hogan, P. J., Wallcraft, A. J., et al. (2007). The HYCOM (HYbrid Coordinate Ocean Model) data assimilative system. J. Mar. Syst. 65, 60–83. doi: 10.1016/j.jmarsys.2005.09.016
Cho, W., and Shank, T. M. (2010). Incongruent patterns of genetic connectivity among four ophiuroid species with differing coral host specificity on North Atlantic seamounts. Mar. Ecol. 31, 121–143. doi: 10.1111/j.1439-0485.2010.00395.x
Christiansen, B., Vieira, R. P., Christiansen, S., Denda, A., Oliveira, F., and Gonçalves, J. M. S. (2015). The fish fauna of Ampère Seamount (NE Atlantic) and the adjacent abyssal plain. Helgol. Mar. Res. 69, 13–23. doi: 10.1007/s10152-014-0413-4
Clark, M. R., Anderson, O. F., and Doonan, I. J. (2016). “Estimating orange roughy stock size on seamounts: a meta-analysis of physical seamount characteristics,” New Zealand Fisheries Assessment Report 2016/47, Wellington: Ministry of Primary Industries, 5352.
Clark, M. R., Rowden, A. A., Schlacher, T., Williams, A., Consalvey, M., Stocks, K. I., et al. (2010). The ecology of seamounts: structure. function, and human impacts. Ann. Rev. Mar. Sci. 2, 253–278. doi: 10.1146/annurev-marine-120308-081109
Clark, M. R., Watling, L., Rowden, A. A., Guinotte, J. M., and Smith, C. R. (2011). A global seamount classification to aid the scientific design of marine protected area networks. Ocean Coast. Manag. 54, 19–36. doi: 10.1016/j.ocecoaman.2010.10.006
Comas-Rodríguez, I., Hernández-Guerra, A., Fraile-Nuez, E., Martínez-Marrero, A., Benítez-Barrios, V. M., Pérez-Hernández, M. D., et al. (2011). The Azores Current System from a meridional section at 24.5°W. J. Geophys. Res. Ocean. 116:C09021. doi: 10.1029/2011JC007129
Dietrich, G., Kalle, K., Krauss, W., and Siedler, G. (1980). General Oceanography: An Introduction. Hoboken, NJ: John Wiley & Sons.
Doty, M. S., and Oguri, M. (1956). The Island Mass Effect. ICES J. Mar. Sci. 22, 33–37. doi: 10.1093/icesjms/22.1.33
Dower, J. F., and Mackas, D. L. (1996). “Seamount effects” in the zooplankton community near Cobb Seamount. Deep. Res. I 43, 837–858. doi: 10.1016/0967-0637(96)00040-4
Etnoyer, B. P. J., Wood, J., and Shirley, T. C. (2010). How large is the seamount biome? Oceanography 23, 206–209. doi: 10.5670/oceanog.2010.96
Etter, R. J., and Bower, A. S. (2015). Dispersal and population connectivity in the deep North Atlantic estimated from physical transport processes. Deep. Res. Part I Oceanogr. Res. Pap. 104, 159–172. doi: 10.1016/j.dsr.2015.06.009
Fock, H., Uiblein, F., Köster, F., and Von Westernhagen, H. (2002). Biodiversity and species-environment relationships of the demersal fish assemblage at the Great Meteor Seamount (subtropical NE Atlantic), sampled by different trawls. Mar. Biol. 141, 185–199. doi: 10.1007/s00227-002-0804-y
Fox, A. D., Henry, L.-A., Corne, D. W., and Roberts, J. M. (2016). Sensitivity of marine protected area network connectivity to atmospheric variability. R. Soc. Open Sci. 3, 1–18. doi: 10.1098/rsos.160494
Geldmacher, J., Hoernle, K., Klügel, A., Bogaard, P. V. D., Wombacher, F., and Berning, B. (2006). Origin and geochemical evolution of the Madeira-Tore Rise (eastern North Atlantic). J. Geophys. Res. Solid Earth 111:B09206. doi: 10.1029/2005JB003931
Gould, W. (1985). Physical oceanography of the Azores Front. Prog. Ocean. 14, 167–190. doi: 10.1016/0079-6611(85)90010-2
Hall-Spencer, J., Rogers, A. D., Davies, J., and Foggo, A. (2007). Deep-sea coral distribution on seamounts, oceanic islands, and continental slopes in the Northeast Atlantic. Bull. Mar. Sci. 81, 135–146.
Hermida, M., and Delgado, J. (2016). High trophic level and low diversity: Would Madeira benefit from fishing down? Mar. Policy 73, 130–137. doi: 10.1016/j.marpol.2016.07.013
Holstein, D. M., Paris, C. B., and Mumby, P. J. (2014). Consistency and inconsistency in multispecies population network dynamics of coral reef ecosystems. Mar. Ecol. Prog. Ser. 499, 1–18. doi: 10.3354/meps10647
Johnson, J., and Stevens, I. (2000). A fine resolution model of the eastern North Atlantic between the Azores, the Canary Islands and the Gibraltar Strait. Deep Sea Res. Part I 47, 875–899. doi: 10.1016/s0967-0637(99)00073-4
Jones, G. P., Srinivasan, M., and Almany, G. R. (2007). Population connectivity and conservation of marine biodiversity. Oceanography 20, 100–111. doi: 10.5670/oceanog.2007.33
Jorge, R., Sousa, S., Observatory, O., View, P. M., and Ocean, N. A. (2016). BIOMETORE Biodiversity in the Seamounts: The Madeira-Tore and Great Meteor. Report No. Arquivos do DOP, Série Cruzeiros N°2/2016, Portugal.
Käse, R. H., and Siedler, G. (1982). Meandering of the subtropical front south-east of the Azores. Nature 300, 245–246. doi: 10.1038/300245a0
Kitchingman, A., Lay, S., Morato, T., and Pauly, D. (2007). “How many seamounts are there and where are they located?,” in Seamounts: Ecology, Fisheries & Conservation, eds P. J. B. Hart, N. Haggan, R. S. Santos, T. Morato, and M. R. Clark (Hoboken, NJ: John Wiley & Sons).
Klein, B., and Siedler, G. (1989). On the origin of the Azores current. J. Geophys. Res. Atmosph. 94, 6159–6168. doi: 10.1029/JC094iC05p06159
Lavelle, J. W., and Mohn, C. (2010). Motion, commotion, and biophysical connections at deep ocean seamounts. Oceanography 23, 90–103. doi: 10.5670/oceanog.2010.64
Lett, C., Barrier, N., Ourmières, Y., Petit, C., Labonne, M., Bourjea, J., et al. (2019). Modeling larval dispersal for the gilthead seabream in the northwestern Mediterranean Sea. Mar. Environ. Res. 152:104781. doi: 10.1016/j.marenvres.2019.104781
Majoris, J. E., Catalano, K. A., Scolaro, D., Atema, J., and Buston, P. M. (2019). Ontogeny of larval swimming abilities in three species of coral reef fishes and a hypothesis for their impact on the spatial scale of dispersal. Mar. Biol. 166, 1–14. doi: 10.1007/s00227-019-3605-2
Morato, T., Cheung, W. W. L., and Pitcher, T. J. (2006). Vulnerability of seamount fish to fishing: Fuzzy analysis of life-history attributes. J. Fish Biol. 68, 209–221. doi: 10.1111/j.0022-1112.2006.00894.x
Morato, T., Miller, P. I., Dunn, D. C., Nicol, S. J., Bowcott, J., and Halpin, P. N. (2016). A perspective on the importance of oceanic fronts in promoting aggregation of visitors to seamounts. Fish Fish. 17, 1227–1233. doi: 10.1111/faf.12126
Morgan, W. J. (1983). Hotspot tracks and the early rifting of the Atlantic. Tectonophysics 94, 123–139. doi: 10.1016/0040-1951(83)90013-6
Onken, R. (1992). The Azores countercurrent. J. Phys. Oceanogr. 23, 1638–1646. doi: 10.1175/1520-04851993023<1638:TAC<2.0.CO;2
Paris, C. B., Helgers, J., van Sebille, E., and Srinivasan, A. (2013). Connectivity modeling system: a probabilistic modeling tool for the multi-scale tracking of biotic and abiotic variability in the ocean. Environ. Model. Softw. 42, 47–54. doi: 10.1016/j.envsoft.2012.12.006
Peirce, C., and Barton, P. J. (1991). Crustal structure of the Madeira–Tore Rise, eastern North Atlantic—results of a DOBS wide-angle and normal incidence seismic experiment in the Josephine Seamount region. Geophys. J. Int. 106, 357–378. doi: 10.1111/j.1365-246X.1991.tb03898.x
Pineda, J., Hare, J., and Sponaugle, S. (2007). Larval transport and dispersal in the coastal ocean and consequences for population connectivity. Oceanography 20, 22–39. doi: 10.5670/oceanog.2007.27
Pingree, R. D. (1997). The eastern subtropical gyre (North Atlantic): flow rings recirculations structure and subduction. J. Mar. Biol. Assoc. UK 77, 573–624. doi: 10.1017/s0025315400036109
Pitcher, T. J., Morato, T., Hart, P. J. B., Clark, M. R., Haggan, N., and Santos, R. S. (2007). Seamounts: Ecology, Fisheries & Conservation, 1st Edn. Hoboken, NJ: Blackwell Publishing.
Probert, S. P. K., Christiansen, S., Gjerde, K. M., Gubbay, S., and Santos, R. S. (2007). “Management and conservation of seamounts,” in Seamounts: Ecology, Fisheries & Conservation, eds P. J. B. Hart, N. Haggan, R. S. Santos, T. Morato, and M. R. Clark (Hoboken, NJ: John Wiley & Sons).
Rogers, A. D. (1994). The biology of seamounts. Adv. Mar. Biol. 30, 305–350. doi: 10.1016/S0065-2881(08)60065-6
Rogers, A. D. (2004). The Biology, Ecology And Vulnerability Of Seamount Communities, 1st Edn. Switzerland: International Union for Conservation of Nature & Natural Resources.
Rogers, A. D., Baco, A., Griffi, H., and Hart, T. (2007). “Corals on Seamounts,” in Seamounts: Ecology, Fisheries & Conservation, eds P. J. B. Hart, N. Haggan, R. S. Santos, T. Morato, and M. R. Clark (Hoboken, NJ: John Wiley & Sons), 141–169.
Ross, R. E., Nimmo-Smith, W. A. M., and Howell, K. L. (2016). Increasing the depth of current understanding: sensitivity testing of deep-sea larval dispersal models for ecologists. PLoS One 11:1–25. doi: 10.1371/journal.pone.0161220
Royden, L. H. (1993). Evolution of retreating subduction boundaries formed during continental collision. Geology 12, 629–638. doi: 10.1029/92tc02641
Sala, I., Caldeira, R. M. A., Estrada-Allis, S. N., Froufe, E., and Couvelard, X. (2013). Lagrangian transport pathways in the northeast Atlantic and their environmental impact. Limnol. Oceanogr. Fluids Environ. 3, 40–60. doi: 10.1215/21573689-2152611
Sala, I., Harrison, C. S., and Caldeira, R. M. A. (2016). The role of the Azores Archipelago in capturing and retaining incoming particles. J. Mar. Syst. 154, 146–156. doi: 10.1016/j.jmarsys.2015.10.001
Samadi, S., Bottan, L., Macpherson, E., De Forges, B. R., and Marie-Catherine, B. (2006). Seamount endemism questioned by the geographic distribution and population genetic structure of marine invertebrates. Mar. Biol. 149, 1463–1475. doi: 10.1007/s00227-006-0306-4
Sangrà, P., Pascual, A., Rodríguez-Santana, A., Machín, F., Mason, E., McWilliams, J. C., et al. (2009). The canary eddy corridor: a major pathway for long-lived eddies in the subtropical North Atlantic. Deep. Res. Part I Oceanogr. Res. Pap. 56, 2100–2114. doi: 10.1016/j.dsr.2009.08.008
Shank, T. (2010). Seamounts: deep-ocean laboratories of faunal connectivity, evolution, and endemism. Oceanography 23, 108–122. doi: 10.5670/oceanog.2010.65
Staudigel, H., and Clague, D. A. (2010). The geological history of deep-sea volcanoes. Oceanography 23, 58–71. doi: 10.5670/oceanog.2010.62
Stow, C., Jolliff, J., McGillicuddy, D., Doney, S., Allen, J., Friedrichs, M., et al.. (2009). Skill assessment for coupled biological/physical models of marine systems. J. Mar. Syst. 76, 4–15. doi: 10.1016/j.jmarsys.2008.03.011
Taninaka, H., Bernardo, L. P. C., Saito, Y., Nagai, S., Ueno, M., Kitano, Y. F., et al. (2019). Limited fine-scale larval dispersal of the threatened brooding corals Heliopora spp. as evidenced by population genetics and numerical simulation. Conserv. Genet. 20, 1449–1463. doi: 10.1007/s10592-019-01228-7
Tucholke, B. E., and Ludwig, W. J. (1982). Structure and Origin of the J Anomaly Ridge. Western North Atlantic Ocean. J. Geophys. Res. 87:9389. doi: 10.1029/JB087iB11p09389
Tuerena, R. E., Williams, R. G., Mahaffey, C., Vic, C., Green, J. A. M., Naveira-Garabato, A., et al. (2019). Internal tides drive nutrient fluxes into the deep chlorophyll maximum over Mid-ocean ridges. Glob. Biogeochem. Cycles. 33, 995–1009. doi: 10.1029/2019GB006214
Van Sebille, E., England, M. H., Zika, J. D., and Sloyan, B. M. (2012). Tasman leakage in a fine-resolution ocean model. Geophys. Res. Lett. 39, 2–6. doi: 10.1029/2012GL051004
Wessel, P., Sandwell, D., and Kim, S.-S. (2010). The global seamount census. Oceanography 23, 24–33. doi: 10.5670/oceanog.2010.60
White, M., Bashmachnikov, I., Arístegui, J., and Martins, A. (2008). Physical Processes and Seamount Productivity. Seamounts, 62–84. doi: 10.1002/9780470691953.ch4
Wienberg, C., Wintersteller, P., Beuck, L., and Hebbeln, D. (2013). Coral patch seamount (NE Atlantic) - A sedimentological and megafaunal reconnaissance based on video and hydroacoustic surveys. Biogeosciences 10, 3421–3443. doi: 10.5194/bg-10-3421-2013
Wolanski, E. (1994). Physical Oceanographic Processes of the Great Barrier Reef. Boca Raton, FL: CRC Press 194.
Keywords: Lagrangian transport, drifting particles, physical connectivity, seamounts, northeast Atlantic Ocean
Citation: Lima MJ, Sala I and Caldeira RMA (2020) Physical Connectivity Between the NE Atlantic Seamounts. Front. Mar. Sci. 7:238. doi: 10.3389/fmars.2020.00238
Received: 19 December 2019; Accepted: 27 March 2020;
Published: 05 May 2020.
Edited by:
Telmo Morato, University of the Azores, PortugalReviewed by:
Christian Mohn, Aarhus University, DenmarkGeorge Andrew Wolff, University of Liverpool, United Kingdom
Copyright © 2020 Lima, Sala and Caldeira. This is an open-access article distributed under the terms of the Creative Commons Attribution License (CC BY). The use, distribution or reproduction in other forums is permitted, provided the original author(s) and the copyright owner(s) are credited and that the original publication in this journal is cited, in accordance with accepted academic practice. No use, distribution or reproduction is permitted which does not comply with these terms.
*Correspondence: Rui M. A. Caldeira, cnVpLmNhbGRlaXJhQG9vbS5hcmRpdGkucHQ=