- 1NOAA-Fisheries, Woods Hole, MA, United States
- 2Department of Fisheries Oceanography, School for Marine Science and Technology, University of Massachusetts Dartmouth, New Bedford, MA, United States
Ecological and socio-economic indicators are used as proxies for attributes of ecosystems and human communities, respectively. End-to-end models are used to predict how ecosystems will respond to alternative management actions and changing environmental conditions. Despite the importance of these two tools for Ecosystem-Based Management (EBM), there has been limited integration of ecological indicators directly into end-to-end models; the former are typically calculated post hoc with output from the latter. Here we explore how ecological indicators can be better incorporated into end-to-end models and examine the importance of this union with regards to cumulative impacts and indirect effects, setting management objectives, practical indicator selection, and applications to management. We conclude that the inclusion of ecological indicators in end-to-end models is not only feasible, but provides needed guidance on describing ecosystem status relative to strategic as well as tactical ecosystem-level management goals, and will escalate the implementation of EBM.
Introduction
Marine resource management at the ecosystem level is becoming a useful approach to complement single-species, single-sector, and single-impact assessments (Link et al., 2012; Gaichas et al., 2017; Link and Browman, 2017). Given the theoretical advancements in understanding whole ecosystems, there is a growing global imperative to implement ecosystem-based management (EBM) – a management approach that recognizes the full array of interactions within an ecosystem and accounts for multiple human uses, complexity, cumulative impacts, indirect effects, emergent properties, and tipping points (Christensen et al., 1996; McLeod et al., 2005; Link et al., 2015). The rationale and descriptions thereof are replete (e.g., Leslie and McLeod, 2007; Link and Browman, 2014) and not repeated here; the salient point is how to operationally implement EBM. Current strategic policies are actively aiming to use ecosystem-level science to guide decisions in marine management: e.g., the European Union Marine Strategy Framework Directive (MSFD: Palialexis et al., 2014), Commission for the Conservation of Antarctic Marine Living Resources (Constable et al., 2000; Constable, 2011), Australia’s Ocean Policy (Smith et al., 2007), and National Oceanographic and Atmospheric Administration’s Ecosystem-Based Fisheries Management Policy (Noaa-Fisheries, 2016).
The development and uses of both ecological and socio-economic indicators in current ecosystem-level ocean management have greatly increased over the last decade. The importance of ecological indicators to marine ecosystem management has been made evident in global projects such as IndiSeas (Shin and Shannon, 2010), International Council for the Exploration of the Sea working groups (ICES: Tam et al., 2017b) and the Food and Agriculture Organization of the United Nation’s (FAO) approach to sustainable fisheries management (Garcia et al., 2000; Punt et al., 2001). Operationally, ecological indicators have been selected for use directly in marine policy by a number of countries to determine the state of ecosystems (Fulton et al., 2005; Thrush et al., 2011; Levin et al., 2014; Shephard et al., 2015; Jackson et al., 2016). Socio-economic indicators have been used to identify communities vulnerable to fishing collapses and climate change (Pollnac et al., 2015; Colburn et al., 2016). Indicators act as proxies to simplify complicated trends in multiple biological, environmental or anthropogenic variables and are immensely useful to conservation and resource management (Methratta and Link, 2006; Link et al., 2009; Blanchard et al., 2010; Shin and Shannon, 2010; Coll and Lotze, 2016). Ecological indicators can help to reveal overarching patterns in ecosystems, while socio-economic indicators can help to quantitatively define management objectives and determine the achievement of those objectives. By capturing the emergent properties, cumulative impacts, and indirect effects of ecosystems and human communities through indicators (Link et al., 2015; Pollnac et al., 2015) and developing ecosystem-level reference points (ELRPs; Large et al., 2015b; Samhouri et al., 2017a; Tam et al., 2017a), it is possible to avert negative scenarios such as the loss of jobs, overfishing, hypoxia, or stock collapse (Rabalais et al., 2002; Fay et al., 2015).
End-to-end ecosystem models are important tools to collate, understand and predict key features of marine ecosystems (Travers et al., 2007; Fulton, 2010; Rose et al., 2010; Collie et al., 2014; Tittensor et al., 2017; Lotze et al., 2019). The most commonly used marine end-to-end model is Ecopath with Ecosim and Ecospace (Polovina, 1984; Walters et al., 1999; Christensen and Walters, 2004). Ecopath is a mass-balance model of energy flows in an ecosystem, while Ecosim produces time dynamic simulations of the initial Ecopath model, and has been used primarily for fisheries policy exploration. Ecospace allows for the consideration of spatial management by including habitat dependency, migration, and fisheries distributions among other spatially explicit parameters. Large biogeochemical-based models such as Atlantis (Fulton et al., 2011) incorporate human dynamics which have model applications to concentrate on questions spanning all parts of the adaptive management cycle (Jones, 2009). Atlantis connects the biophysical system, human users (primarily industry), monitoring, assessment, management decision processes, and socio-economic drivers of human use and behavior (Fulton et al., 2011). The Atlantis model is capable of addressing policy needs, balancing socio-ecological objectives of human activities and clearly presenting potential trade-offs (Weijerman et al., 2016). Most importantly, Atlantis can test the feasibility of management strategies before they are implemented in reality (Fulton et al., 2011), which is an important step to exploring fisheries, coastal zone, and related ocean-use management actions up to and including full EBM scenarios (e.g., Fulton et al., 2014). Agent-based models such as OSMOSE (Shin and Cury, 2001) and in vitro (McDonald et al., 2006) include individual-based, age-structured fish or predator population and trophic interaction models, biogeochemical plankton production models, hydrodynamic and environmental models, habitat models and representations of human activities. These models use decision algorithms that allow for fluid representation of processes like movement, growth, phenotypic expression, and evolution, making them a useful tool for examining fine scale interactions and responses to the impacts of large scale drivers (Fulton, 2010; Rose et al., 2010).
Many end-to-end models are designed to perform ecosystem-level scenario analysis and Management Strategy Evaluations (MSEs) where ecosystem dynamics can be explored under a variety of plausible management, climate, oceanographic and human use conditions (Fulton et al., 2014; Masi et al., 2018). The MSE process ultimately aims to explore the results from a set of management strategies to compare how well they meet specified management objectives. Other end-to-end models also exist, but the increasing global use of end-to-end models as a common tool is the direct result of public, scientific, and management concerns and interests in examining system-level or indirect effects in multiple use scenarios. Furthermore, the fast pace with which ecosystem level end-to-end models are evolving is strong indication of their importance in furthering EBM.
The importance of both indicators and end-to-end modeling to the future of EBM is clear, yet there has been limited integration of indicators directly into end-to-end models. Currently, ecological indicators are typically calculated as post hoc analyses from end-to-end model simulations (Coll and Steenbeek, 2017; Masi et al., 2017). In doing so, the ability to use information from indicators on cumulative impacts and indirect effects to adjust management actions is lost and not captured within model simulations. Here we aim to describe how indicators and end-to-end models are mutually beneficial to each other, how they can be better integrated to improve the understanding of ecosystem dynamics and, in turn, facilitate more successful management actions. Ultimately, these two tools together will increase the accuracy of end-to-end model predictions to provide operational management advice at the ecosystem-level.
Value of Indicators for End-To-End Models
To execute EBM, ecosystem modeling tools are advisable to collate, synthesize and predict ecosystem dynamics related to cumulative impacts (Korpinen and Andersen, 2016), indirect effects (Crain et al., 2008), emergent properties (Link et al., 2015) and ELRPs (Tam et al., 2017a). There is widespread empirical support that ecosystem-level emergent properties and reference points can be calculated for multiple marine ecosystems using thresholds from empirically derived ecological indicators (Link et al., 2012, 2015; Large et al., 2015a, b; Samhouri et al., 2017a; Tam et al., 2017a). These key fundamental features have been revealed through ecological indicators, adding to strong theoretical support of global ecosystem patterns. Link et al. (2015) found common sigmoidal cumulative biomass-trophic level curves and “hockey stick” cumulative production-biomass curves across 120 marine ecosystems that can help delineate when marine ecosystems are perturbed or recovered. Large et al. (2015a), Samhouri et al. (2017a) and Tam et al. (2017a) have found common multivariate ELRPs for ecological indicators along both anthropogenic and environmental pressure gradients. This comparative work of ecological indicator ELRPs showed that, generally, total landings above ∼2–4 t km–2 yielded significant changes in ecosystem state which was consistent with surplus production models for multiple marine ecosystems (Bundy et al., 2012; Link et al., 2012; Tam et al., 2017a). Friedland et al. (2012) and Tam et al. (2017a) found that there was a notable increase in monthly fishery yield and ecosystem shift, respectively, when primary production was above ∼0.7 mg m–3.
Many of these ecosystem dynamics can be captured with end-to-end models, and thus can be used to help evaluate the consistency and skill of ecosystem model structures (e.g., do indicators from end-to-end models respond to ecosystem drivers similarly as observed). This often requires post hoc processing. For example, the ECOIND plug-in for Ecopath with Ecosim (Coll and Steenbeek, 2017) can calculate a number of indicators (biomass, catch, trophic, size, and species based), but does so after Ecopath, Ecosim or Ecospace has been run. Masi et al. (2017) used the Gulf of Mexico Atlantis model to define indicators that are sensitive to changes in fishing mortality through post hoc calculations of indicators from model outputs under differing fishing scenarios. Olsen et al. (2018) calculated a suite of indicators from the output of a set of Atlantis models and used them to compare ecosystem responses to fishing, spatial management, and ocean acidification both within and between marine ecosystems. In all these examples, the end-to-end model outputs were handled post hoc, not as an integrated part of the modeling and analytical efforts.
While these applications are useful for isolating indicators that are sensitive to particular pressures (e.g., fishing mortality, pollution, etc.), incorporating calculation of these indicators directly into end-to-end models will better reveal overall ecosystem dynamics. Doing so will better facilitate examination of cumulative effects and elucidate indirect impacts, by capturing unintended consequences, exploring synergistic and antagonistic dynamics, and integrating scales and multiple biological features in an end-to-end model setting. This is because calculating indicators post hoc from model output only allows analysts to view indicator snapshots that might not fully detail the way in which values for indicators change over time and space within the model domain. This is not a problem solely with indicators. Essentially having these indicators embedded as part of end-to-end models will enable model users to better account for the second order, non-linear, and indirect effects common in ecosystem models (Kaplan et al., 2010, 2013; Fay et al., 2017). Fay et al. (2017) examined the impacts of ocean acidification on the Atlantis-Northeast US ecosystem model. They determined that impacts to the Northeast US food web extended beyond groups that were thought to be most vulnerable, however, the precise nature of these post hoc analyses were difficult to interpret.
Calculating indicators outside of model simulations captures some of the ecosystem dynamics, but removes the possibility of including feedback loops from indicator values to system dynamics within the model runs, say as the result of management action. Post hoc calculation also negates the ability to track behavioral responses of human activities within the model domain by using ELRPs as part of the model dynamics. Incorporating indicators directly within models will better capture the nuances of these dynamics and allow for the exploration of synergistic management action which has been shown to be more efficient and effective at restoring depleted populations (Crain et al., 2008; Smith et al., 2015; Samhouri et al., 2017b). Embedding indicators as part of end-to-end models allows for “real-time” testing and use of ELRPs for management action. Including these indicators with ELRPs facilitates the ability to test and track their performance, thus advancing the recommended levels and use of ELRPs (Samhouri et al., 2010; Large et al., 2015a, b; Link, 2017; Tam et al., 2017a). This in turn will facilitate the validity and uptake of ecosystem model output by using these standard decision criteria that have been tested and validated.
Indicators also play an important role in evaluating end-to-end model skill (i.e., calibration, validation, and how much confidence to have in the model) and performance. There are numerous methods that can be used to assess end-to-end model skill, but most commonly, model parameters are adjusted to plausible levels (changes made within confidence limits of observed monitoring or assessment data) or are matched to estimates from time series data. This is frequently an iterative process that is unique to each model type, but general guidelines and best practices are documented in the literature (Shin and Cury, 2001; Link et al., 2011; Heymans et al., 2016; Steenbeek et al., 2016). Indicators act as a pathway to assess model skill by additionally including emergent properties that reflect the interactions between model components. Incorporating indicators in the initial development of an end-to-end model would ensure that observed emergent properties and dynamics of the ecosystem will be captured. Olsen et al. (2016) found that indicators were an important consideration when assessing model skill because they could examine emergent properties of ecosystems across a range of spatial levels and metrics (i.e., an indicator of broad system properties is total biomass, an indicator of narrower system properties is charismatic megafauna biomass). By using indicators alongside other data sources (i.e., single species and human use metrics) end-to-end models can be evaluated on their utility for making predictions (hindcast or forecast) for whole socio-ecological systems. Having indicators directly in the models further facilitates this skill evaluation.
A consistent challenge in EBM and MSEs is the task of setting and defining management objectives. While ELRPs for ecological indicators quantitatively define tipping points in ecosystems that translate to avoidance points for managers, setting management objectives for human uses of marine ecosystems beyond a fisheries lens can be difficult. International biodiversity targets such as Aichi or the trade restrictions by the Convention on International Trade in Endangered Species of Wild Fauna and Flora can be used to define ocean policy (CITES, 1973; CBD, 2011; Juffe-Bignoli et al., 2016), but considerations for human coastal community health and well-being are seldom considered in management scenarios. This is not surprising, since measures of well-being have been difficult to quantify (e.g., cultural attachment, job satisfaction, health, and safety) even despite the establishment of specific limits on pollutant concentrations in coastal communities. Recently, however, there has been development of socio-economic indicators that track patterns of community vulnerability and well-being, further elucidating some of the complexity of the human dimension of EBM (Bowen and Riley, 2003; Pollnac et al., 2015; Colburn et al., 2016; Auad et al., 2018). These indicators embedded in end-to-end models will add nuance to the more conventional management considerations (e.g., total allowable catches to commercial fisheries, recreational fishing opportunities) by incorporating patterns of human behavior and overall community health. Furthermore, developing ELRPs for socio-economic indicators will help to quantitatively set management objectives. For example, system-level optimal yields can be calculated as ELRPs for socio-economic indicators that define the amount (or ranges) of resource extraction (for fisheries) needed to maintain community health (avoiding, for example, long-term poverty). With simultaneous explorations of both ecological and socio-economic management objectives within end-to-end models we can begin to quantitatively assess tradeoff spaces (Rockstrom et al., 2009; Link, 2010; Dearing et al., 2014) that avoid ecological regime shifts (e.g., stock collapses) and undesirable shifts to human coastal communities (e.g., increased outmigration).
Value of Using End-To-End Models for Indicators
Ecosystem-Based Management is reliant on the use of ecological indicators to assess ecosystem status. This is evident from the inclusion of ecological indicators in frameworks for a number of EBM programs including the Integrated Ecosystem Assessments (Levin et al., 2009, 2014; Walther and Mollmann, 2014) and the MSFD (Palialexis et al., 2014). Several international efforts have been made to determine a pragmatic set of ecological indicators to assess marine ecosystem status (Fulton et al., 2005; Shin et al., 2010; Tam et al., 2017b; Fu et al., 2019). While much of the development and selection of indicators has been done through time-series and pressure-response relationships with human or environmental pressures (Methratta and Link, 2006; Large et al., 2013), there is much to be gained from using end-to-end models to advance the uses of indicators for ecosystem management.
In many cases, scientists and managers have to work within specified budgets with which to develop research and monitoring to meet specific objectives in EBM (Fulton et al., 2005; Niemeijer and de Groot, 2008). The number of ecological indicators found in the literature to evaluate marine ecosystems can be overwhelming, and determining the “Goldilocks” number of indicators (i.e., not too few, not too many, but just right) can be a challenge. There are numerous methods to reduce the number of indicators needed for practical use in EBM and to limit bias in the representation of an ecosystem attribute (Link et al., 2002; Rice and Rochet, 2005). Tam et al. (2017b) used expert opinion to develop selection criteria to determine a standard set of five food-web indicators from 60 potential indicators. Bundy et al. (2017, 2019) reduced 358 possible indicators used to represent the Scotian Shelf region to a set of 30 indicators through a series of qualitative (selection criteria) and quantitative (redundancy analysis) screening. They also examined these indicators across multiple spatial levels (the strata, regional, and ecosystem level) and determined that different sets of indicators were most effective at detecting changes at each spatial level. To build upon this work, it would be beneficial to adjust end-to-end model parameters to identify which suites of indicators best represent impacts to major concerns or priorities (i.e., maintaining fisheries yields, maximizing biodiversity). Model based approaches to selecting indicators and assessing them against known pressures (human activities, climate, etc.) have a substantial advantage over other methods (e.g., expert opinion, time series trends, multivariate dimension reduction, etc.) as they are not as heavily reliant on up-to-date field data and can be more cost effective.
While there is a wide breadth of indicators that have been vetted for current use in EBM, there is a continuous stream of indicators being conceived and developed. Tam et al. (2017b) identified a number of proposed ecosystem-level food-web indicators that were underdeveloped or lacked necessary data to be considered operational. They suggested that these indicators be re-evaluated for operational use in light of new information. As such, this iterative process to develop indicators is a key step in many EBM frameworks to better understand ecosystem dynamics and ensure that management objectives are met (Levin et al., 2014; Walther and Mollmann, 2014; Queirós et al., 2016). End-to-end models are the perfect platform to test the validity (Which ecosystem attribute is this indicator a proxy for?), sensitivity (What is the capacity of this indicator to detect change in the ecosystem attribute?), and specificity (What is the level of confidence with which the variation of an indicator can be attributed to a particular pressure?) of these un-vetted indicators (Houle et al., 2012; Ortega-Cisneros et al., 2018; Shin et al., 2018). Fulton et al. (2005), Samhouri et al. (2009), Kaplan et al. (2013), Olsen et al. (2018) and Ortega-Cisneros et al. (2018) used end-to-end model simulations to examine the impacts of fishing and climate on indicators. In these studies model simulations were projected at different levels of fishing or environmental variability and indicators were calculated from these outputs and examined against ecosystem attributes (to examine validity and sensitivity) or pressures (specificity). These post hoc analyses of indicators are useful and informative, however, including some of these indicators into existing end-to-end models directly could identify the utility of underdeveloped indicators or to screen for less useful indicators by examining any changes to model performance when adding or subtracting new indicators. This would remove the need for potentially subjective expert opinion from indicator selection processes and allow for more objective, quantitatively based evaluation of indicator performance and selection.
Summary and Conclusion
Successful EBM requires the ability to account for cumulative effects and indirect impacts of human and environmental pressures at the ecosystem level while also accounting for single sector assessments such as fishing mortality from stock assessments or risk analysis for energy exploration (McLeod et al., 2005; Link, 2010; Stelzenmüller et al., 2018). Indicators and end-to-end models are both ecosystem-level management tools already in use that can account for the complexity of interactions within ecosystems alongside single sector assessments that need to be operationalized for EBM (Fulton et al., 2011; Patrick and Link, 2015; Weijerman et al., 2015; Link and Browman, 2017). Evidence from multispecies models using control rules derived from indicator thresholds suggests these models were able to perform better against catch and biodiversity objectives than when harvests were based solely on single-species advice (Fay et al., 2015; Fulton et al., 2019). Kaplan et al. (2013) found that there was a mix of additive and non-additive impacts to fish in model simulations when using indicators as performance measures. Some fleets had a direct impact on target and Bycatch species without extending to other parts of the food web, while other fleets showed unintended impacts on groups beyond the targeted species. These examples of indicators used with end-to-end models reveal that ecosystem-level examinations of pressures on systems necessitates dynamic and mixed approaches that cannot be achieved through single sector management alone.
By integrating indicators into end-to-end models, the patterns, properties and impacts on indicators within simulations can be tracked and also used to help make “virtual” management decisions. Fundamental changes to the model structure and behavior would likely occur through the incorporation of indicators to end-to-end models compared to current versions that operate without. For example, including existing indicator time series in the model fitting process (e.g., Scott et al., 2015) or Monte Carlo routine (e.g., Steenbeek et al., 2018) would constrain model outputs, change simulation results, and potentially improve model uncertainty. Much like the integration of harvest control rules for fisheries into end-to-end model MSE simulations, management actions based on systemic properties through indicators can be used in the simulation process to track management actions based on ELRPs (Figure 1). Fay et al. (2015) describes how the incorporation of ELRPs can improve model management performance using a multispecies model. Incorporating indicators into more complex, end-to-end models would increase the ability to leverage a fuller suite of indicators that span a broader range of objective types. Indicator-based management decisions can be made in “virtual” real-time, thereby better tracking the feedback that such decisions will make on the ecosystem features being monitored and managed. This would give managers and policy makers a tool that incorporates cumulative impacts and indirect effects to fully explore the tradeoffs required to balance the needs of both people and ecosystems, with a better sense of the “non-delayed” (i.e., “real-time”) ramifications of such decisions.
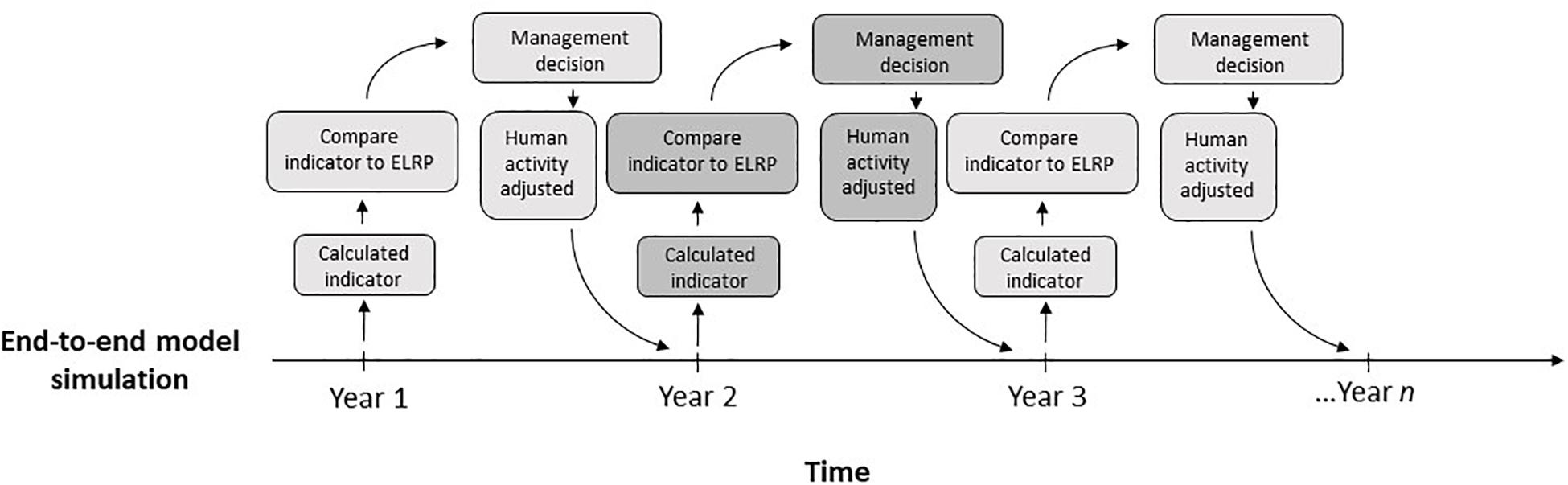
Figure 1. Example of including indicator information into management decisions within end-to-end model simulation where the indicator for each time step is calculated and then compared to ecosystem-level reference points (ELRPs) or thresholds. A management decision is then made, and if necessary human activity is adjusted.
There have been numerous advancements in indicator development and end-to-end modeling over the last decade, with an increasing interest from policy makers and stakeholders to move toward EBM (Patrick and Link, 2015). While there has been increasing joint use of indicators with end-to-end models, there has yet to be a true merger of these two tools. We recommend (1) that direct integration of indicators into end-to-end models should be used to improve model skill and performance, (2) that end-to-end models be used to test un-vetted indicators and to develop suitable indicator suites that effectively represent both ecosystem state and community well-being and (3) that these two tools be used together to develop both strategic and tactical management advice using ELRPs of ecological and socio-economic indicators in addition to testing feasible management strategies. We assert that the benefits of integrating these tools will be greater than the sum of its parts and will further the ability of scientists and managers to implement EBM.
Author Contributions
JT, GF, and JL contributed to the ideas in this manuscript. JT drafted the text. GF and JL contributed considerable edits and input during the writing process.
Funding
JT was supported by a NOAA Postdoctoral Research Fellowship. GF was supported (in part) by the National Oceanic and Atmospheric Administration (NOAA) through the Cooperative Institute for the North Atlantic Region (CINAR) under Cooperative Agreement NA14OAR4320158.
Conflict of Interest Statement
The authors declare that the research was conducted in the absence of any commercial or financial relationships that could be construed as a potential conflict of interest.
Acknowledgments
The ideas in this manuscript grew out of break-out group discussions at the 1st International Summit on the Atlantis end-to-end ecosystem model. The authors thank the attendees of the Atlantis Summit for providing the genesis of this work.
References
Auad, G., Blythe, J., Coffman, K., and Fath, B. D. (2018). A dynamic management framework for socio-ecological system stewardship: a case study for the United States Bureau of ocean energy management. J. Environ. Manage. 225, 32–45. doi: 10.1016/j.jenvman.2018.07.078
Blanchard, J. L., Coll, M., Trenkel, V. M., Vergnon, R., Yemane, D., Jouffre, D., et al. (2010). Trend analysis of indicators: a comparison of recent changes in the status of marine ecosystems around the world. ICES J. Mar. Sci. 67, 732–744. doi: 10.1093/icesjms/fsp282
Bowen, R. E., and Riley, C. (2003). Socio-economic indicators and integrated coastal management. Ocean Coast. Manag. 46, 299–312. doi: 10.1016/S0964-5691(03)00008-5
Bundy, A., Bohaboy, E. C., Hjermann, D. O., Mueter, F. J., Fu, C., and Link, J. S. (2012). Common patterns, common drivers: comparative analysis of aggregate surplus production across ecosystems. Mar. Ecol. Prog. Ser. 459, 203–218. doi: 10.3354/meps09787
Bundy, A., Gomez, C., and Cook, A. M. (2017). Guidance framework for the selection and evaluation of ecological indicators. Can. Tech. Rep. Fish. Aquat. Sci. 3232, 1–26.
Bundy, A., Gomez, C., and Cook, A. M. (2019). Scrupulous proxies: defining and applying a rigorous framework for the selection and evaluation of a suite of ecological indicators. Ecol. Indic. 104, 737–754. doi: 10.1016/j.ecolind.2019.01.031
CBD (2011). Convention on Biological Diversity: Strategic Plan for Biodiversity 2011-2020, including Aichi Biodiversity Targets. Available at: https://www.cbd.int/sp/default.shtml (accessed August 24, 2018).
Christensen, N. L., Bartuska, A. M., Brown, J. H., Carpenter, S., Antonio, D., Francis, R., et al. (1996). The report of the ecological society of america comittee on the scientific basis for ecosystem management. Ecol. Appl. 6, 665–691. doi: 10.2307/2269460
Christensen, V., and Walters, C. J. (2004). Ecopath with ecosim: methods, capabilities and limitations. Ecol. Modell. 172, 109–139. doi: 10.1016/j.ecolmodel.2003.09.003
CITES, (1973). Convention on International Trade in Endangered Species of Wild Fauna and Flora. Washington, DC: CITES.
Colburn, L. L., Jepson, M., Weng, C., Seara, T., Weiss, J., and Hare, J. A. (2016). Indicators of climate change and social vulnerability in fishing dependent communities along the eastern and gulf coasts of the united states. Mar. Policy 74, 323–333. doi: 10.1016/j.marpol.2016.04.030
Coll, M., and Lotze, H. K. (2016). “Ecological indicators and food-web models as tools to study historical changes in marine ecosystems,” in Perspectives on Oceans Past, eds K. Schwerdtner Máñ and B. Poulsen (Dordrecht: Springer), 103–132. doi: 10.1007/978-94-017-7496-3-7
Coll, M., and Steenbeek, J. (2017). Standardized ecological indicators to assess aquatic food webs: the ECOIND software plug-in for ecopath with ecosim models. Environ. Model. Softw. 89, 120–130. doi: 10.1016/j.envsoft.2016.12.004
Collie, J. S., Botsford, L. W., Hastings, A., Kaplan, I. C., Largier, J. L., Livingston, P. A., et al. (2014). Ecosystem models for fisheries management: finding the sweet spot. Fish Fish. 17, 101–125. doi: 10.1111/faf.12093
Constable, A. J. (2011). Lessons from CCAMLR on the implementation of the ecosystem approach to managing fisheries. Fish Fish. 12, 138–151. doi: 10.1111/j.1467-2979.2011.00410.x
Constable, A. J., de la Mare, W. K., Agnew, D. J., Everson, I., and Miller, D. (2000). Managing fisheries to conserve the antarctic marine ecosystem: practical implementation of the convention on the conservation of antarctic Marine living resources (CCAMLR). ICES J. Mar. Sci. 57, 778–791. doi: 10.1006/jmsc.2000.0725
Crain, C. M., Kroeker, K., and Halpern, B. S. (2008). Interactive and cumulative effects of multiple human stressors in marine systems. Ecol. Lett. 11, 1304–1315. doi: 10.1111/j.1461-0248.2008.01253.x
Dearing, J. A., Wang, R., Zhang, K., Dyke, J. G., Haberl, H., Hossain, M. S., et al. (2014). Safe and just operating spaces for regional social-ecological systems. Glob. Environ. Chang. 28, 227–238. doi: 10.1016/j.gloenvcha.2014.06.012
Fay, G., Link, J. S., and Hare, J. A. (2017). Assessing the effects of ocean acidification in the northeast US using an end-to-end marine ecosystem model. Ecol. Modell. 347, 1–10. doi: 10.1016/j.ecolmodel.2016.12.016
Fay, G., Link, J. S., Large, S. I., and Gamble, R. J. (2015). Management performance of ecological indicators in the georges bank finfish fishery. ICES J. Mar. Sci. 72, 1285–1296. doi: 10.1093/icesjms/fsu214
Friedland, K. D., Stock, C., Drinkwater, K. F., Link, J. S., Leaf, R. T., Shank, B. V., et al. (2012). Pathways between primary production and fisheries yields of large marine ecosystems. PLoS One 7:e28945. doi: 10.1371/journal.pone.0028945
Fu, C., Xu, Y., Bundy, A., Grüss, A., Coll, M., and Heymans, J. J. (2019). Making ecological indicators management ready: assessing the specificity, sensitivity, and threshold response of ecological indicators. Ecol. Indic. 105, 16–28. doi: 10.1016/j.ecolind.2019.05.055
Fulton, E. A. (2010). Approaches to end-to-end ecosystem models. J. Mar. Syst. 81, 171–183. doi: 10.1016/j.jmarsys.2009.12.012
Fulton, E. A., Link, J. S., Kaplan, I. C., Savina-Rolland, M., Johnson, P., Ainsworth, C., et al. (2011). Lessons in modelling and management of marine ecosystems: the atlantis experience. Fish Fish. 12, 171–188. doi: 10.1111/j.1467-2979.2011.00412.x
Fulton, E. A., Punt, A. E., Dichmont, C. M., Harvey, C. J., and Gorton, R. (2019). Ecosystems say good management pays off. Fish Fish. 20, 66–96. doi: 10.1111/faf.12324
Fulton, E. A., Smith, A. D. M., and Punt, A. (2005). Which ecological indicators can robustly detect effects of fishing? ICES J. Mar. Sci. 62, 540–551. doi: 10.1016/j.icesjms.2004.12.012
Fulton, E. A., Smith, A. D. M., Smith, D. C., and Johnson, P. (2014). An integrated approach is needed for ecosystem based fisheries management: insights from ecosystem-level management strategy evaluation. PLoS One 9:e84242. doi: 10.1371/journal.pone.0084242
Gaichas, S. K., Fogarty, M., Fay, G., Gamble, R., Lucey, S., and Smith, L. (2017). Combining stock, multispecies, and ecosystem level fishery objectives within an operational management procedure: simulations to start the conversation. ICES J. Mar. Sci. 74, 552–565. doi: 10.1093/icesjms/fsw119
Garcia, S. M., Staples, D. J., and Chesson, J. (2000). The FAO guidelines for the development and use of indicators for sustainable development of marine capture fisheries and an Australian example of their application. Ocean Coast. Manag. 43, 537–556. doi: 10.1016/s0964-5691(00)00045-4
Heymans, J. J., Coll, M., Link, J. S., Mackinson, S., Steenbeek, J., Walters, C. J., et al. (2016). Best practice in ecopath with ecosim food-web models for ecosystem-based management. Ecol. Modell. 331, 173–184. doi: 10.1016/j.ecolmodel.2015.12.007
Houle, J. E., Farnsworth, K. D., Rossberg, A. G., and Reid, D. G. (2012). Assessing the sensitivity and specificity of fish community indicators to management action. Can. J. Fish Aquat. Sci. 69, 1065–1079. doi: 10.1139/f2012-044
Jackson, W. J., Argent, R. M., Bax, N. J., Clark, G. F., Coleman, S., Cresswell, I. D., et al. (2016). Australia State of the Environment Overview. Canberra, ACT: Australian Government.
Jones, G. (2009). “The adaptive management system for the tasmanian wilderness world heritage area-linking management planning with effectiveness evaluation,” in Adaptive Environmental Amangement, eds C. Allan, and G. Stankey (Berlin: Springer), doi: 10.1007/978-1-4020-9632-7
Juffe-Bignoli, D., Harrison, I., Butchart, S. H. M., FIltcroft, R., Hermoso, V., Jonas, H., et al. (2016). Achieving aichi biodiversity target 11 to improve the performance of protected areas and conserve freshwater biodiversity. Aquat. Conserv. Mar. Freshw. Ecosyst. 26, 133–151. doi: 10.1002/aqc.2638
Kaplan, I. C., Gray, I. A., and Levin, P. S. (2013). Cumulative impacts of fisheries in the california current. Fish Fish. 14, 515–527. doi: 10.1111/j.1467-2979.2012.00484.x
Kaplan, I. C., Levin, P. S., Burden, M., and Fulton, E. A. (2010). Fishing catch shares in the face of global change: a framework for integrating cumulative impacts and single species management. Can. J. Fish. Aquat. Sci. 67, 1968–1982. doi: 10.1139/F10-118
Korpinen, S., and Andersen, J. H. (2016). A global review of cumulative pressure and impact assessments in marine environments. Front. Mar. Sci. 3:153. doi: 10.3389/fmars.2016.00153
Large, S. I., Fay, G., Friedland, K. D., and Link, J. S. (2013). Defining trends and thresholds in responses of ecological indicators to fishing and environmental pressures. ICES J. Mar. Sci. 70, 755–767. doi: 10.1093/icesjms/fst067
Large, S. I., Fay, G., Friedland, K. D., and Link, J. S. (2015a). Critical points in ecosystem responses to fishing and environmental pressures. Mar. Ecol. Prog. Ser. 521, 1–17. doi: 10.3354/meps11165
Large, S. I., Fay, G., Friedland, K. D., and Link, J. S. (2015b). Quantifying patterns of change in marine ecosystem response to multiple pressures. PLoS One 10:e0119922. doi: 10.1371/journal.pone.0119922
Leslie, H. M., and McLeod, K. L. (2007). Confronting the challenges of implementing marine ecosystem-based management. Front. Ecol. Environ. 5, 540–548. doi: 10.1890/060093
Levin, P. S., Fogarty, M. J., Murawski, S. A., and Fluharty, D. (2009). Integrated ecosystem assessments: developing the scientific basis for ecosystem-based management of the ocean. PLoS Biol. 7:e14. doi: 10.1371/journal.pbio.1000014
Levin, P. S., Kelble, C. R., Shuford, R. L., Ainsworth, C., Dunsmore, R., Fogarty, M. J., et al. (2014). Guidance for implemenation of integrated ecosystem assessments: a US perspecitve. ICES 71, 1198–1204. doi: 10.1093/icesjms/fst112
Link, J. S. (2010). Ecosystem-Based Fisheries Management: Confronting Tradeoffs, 1st Edn. New York, NY: Cambridge University Press.
Link, J. S. (2017). System-level optimal yeild: increased value, less risk, improved stability and better fisheries. Can. J. Fish. Aquat. Sci. 75, 1–16. doi: 10.1139/cjfas-2017-2250
Link, J. S., Brodziak, J. K. T., Edwards, S. F., Overholtz, W. J., Mountain, D., Jossi, J. W., et al. (2002). Marine ecosystem assessment in a fisheries management context. Can. J. Fish. Aquat. Sci. 59, 1429–1440. doi: 10.1139/f02-115
Link, J. S., and Browman, H. I. (2014). Integrating what? levels of marine ecosystem-based assessment and management. ICES J. Mar. Sci. 71, 1170–1173. doi: 10.1038/nrneurol.2010.205
Link, J. S., and Browman, H. I. (2017). Operationalizing and implementing ecosystem-based management. ICES J. Mar. Sci. 74, 379–381. doi: 10.1093/icesjms/fsw247
Link, J. S., Gaichas, S., Miller, T. J., Essington, T., Bundy, A., Boldt, J., et al. (2012). Synthesizing lessons learned from comparing fisheries production in 13 northern hemisphere ecosystems: emergent fundamental features. Mar. Ecol. Prog. Ser. 459, 293–302. doi: 10.3354/meps09829
Link, J. S., Gamble, R. J., and Fulton, E. A. (2011). NEUS–Atlantis: Construction, Calibration, and Application of An Ecosystem Model With Ecological Interactions, Physiographic Conditions, and Fleet Behavior. Woods Hole, MA: NOAA Central Library.
Link, J. S., Pranovi, F., Libralato, S., Coll, M., Christensen, V., Solidoro, C., et al. (2015). Emergent properties delineate marine ecosystem perturbation and recovery. Trends Ecol. Evol. 30, 1–13. doi: 10.1016/j.tree.2015.08.011
Link, J. S., Yemane, D., Shannon, L. J., Coll, M., Shin, Y.-J., Hill, L., et al. (2009). Relating marine ecosystem indicators to fishing and environmental drivers: an elucidation of contrasting responses. ICES J. Mar. Sci. 67, 787–795. doi: 10.1093/icesjms/fsp258
Lotze, H. K., Tittensor, D. P., Bryndum-buchholz, A., Eddy, T. D., and Cheung, W. W. L. (2019). Global ensemble projections reveal trophic amplification of ocean biomass declines with climate change. Proc. Natl. Acad. Sci. U.S.A. 116, 12907–12912. doi: 10.1073/pnas.1900194116
Masi, M. D., Ainsworth, C. H., and Jones, D. L. (2017). Using a gulf of mexico atlantis model to evaluate ecological indicators for sensitivity to fishing mortality and robustness to observation error. Ecol. Indic. 74, 516–525. doi: 10.1016/j.ecolind.2016.11.008
Masi, M. D., Ainsworth, C. H., Kaplan, I. C., and Schirripa, M. J. (2018). Interspecific interactions may influence reef fish management strategies in the gulf of mexico. Mar. Coast. Fish. Dyn. Manag. Ecosyst. Sci. 10, 24–39. doi: 10.1002/mcf2.10001
McDonald, A. D., Fulton, E. A., Little, L. R., Gray, R., Sainsbury, K. J., and Lyne, V. D. (2006). “Multiple-use management strategy evaluation for coastal marine ecosystems using invitro,” in Complex Science for a Complex World: Exploring Human Ecosystems With Agents, eds P. Perez, and D. Batten (Canberra, ACT: Australian National University), 227–252.
McLeod, K. L., Lubchenco, J., Palumbi, S. R., and Rosenberg, A. A. (2005). Communication Partnership for Science and the Sea (COMPASS); Scientific Consensus Statement on Marine Ecosystem-Based Management. Available at: http://compassonline.org/? q=EBM.
Methratta, E. T., and Link, J. S. (2006). Evaluation of quantitative indicators for marine fish communities. Ecol. Indic. 6, 575–588. doi: 10.1016/j.ecolind.2005.08.022
Niemeijer, D., and de Groot, R. S. (2008). A conceptual framework for selecting environmental indicator sets. Ecol. Indic. 8, 14–25. doi: 10.1016/j.ecolind.2006.11.012
Noaa-Fisheries, (2016). Ecosystem-Based Management Policy of the National Marine Fisheries Service. Silver Spring, MD: NOAA Fisheries.
Olsen, E., Fay, G., Gaichas, S., Gamble, R., Lucey, S., and Link, J. S. (2016). Ecosystem model skill assessment. Yes We Can!. PLoS One 11:e0146467. doi: 10.1371/journal.pone.0146467
Olsen, E., Kaplan, I. C., Ainsworth, C., Fay, G., Gaichas, S., Gamble, R., et al. (2018). Ocean futures under ocean acidification, marine protection, and changing fishing pressures explored using a worldwide suite of ecosystem models. Front. Mar. Sci. 5:23. doi: 10.3389/fmars.2018.00064
Ortega-Cisneros, K., Shannon, L., Cochrane, K., Fulton, E. A., and Shin, Y. J. (2018). Evaluating the specificity of ecosystem indicators to fishing in a changing environment: a model comparison study for the southern Benguela ecosystem. Ecol. Indic. 95, 85–98. doi: 10.1016/j.ecolind.2018.07.021
Palialexis, A., Tornero, V., Barbone, E., Gonzalez, D., Hanke, G., Cardoso, A. C., et al. (2014). In-Depth Assessment of the EU Member States’ Submissions for the Marine Strategy Framework Directive Under Articles 8, 9 and 10. Brussels: Publications Office of the European Union.
Patrick, W. S., and Link, J. S. (2015). Myths that continue to impede progress in ecosystem-based fisheries management. Fisheries 40, 155–160. doi: 10.1080/03632415.2015.1024308
Pollnac, R. B., Seara, T., and Colburn, L. L. (2015). Aspects of fishery management, job satisfaction, and well-being among commercial fishermen in the northeast region of the United States. Soc. Nat. Resour. 28, 75–92. doi: 10.1080/08941920.2014.933924
Polovina, J. J. (1984). Model of a coral reef ecosystem - I. The ECOPATH model and its application to French Frigate Shoals. Coral Reefs 3, 1–11. doi: 10.1007/BF00306135
Punt, A. E., Campbell, R. A., and Smith, A. D. M. (2001). Evaluating empirical indicators and reference points for fisheries management: application to the broadbill swordfish fishery off eastern Australia. Mar. Freshw. Res. 52, 819–832. doi: 10.1071/MF00095
Queirós, A. M., Strong, J. A., Mazik, K., Carstensen, J., Bruun, J., Somerfield, P. J., et al. (2016). An objective framework to test the quality of candidate indicators of good environmental status. Front. Mar. Sci. 3:73. doi: 10.3389/fmars.2016.00073
Rabalais, N. N., Turner, R. E., and Wiseman, W. J. Jr. (2002). Gulf of Mexico HYPOXIA, “The Dead Zone.”. Annu. Rev. Ecol. Syst. 33, 235–263. doi: 10.1146/annurev.ecolsys.33.010802.150513
Rice, J. C., and Rochet, M.-J. (2005). A framework for selecting a suite of indicators for fisheries management. ICES J. Mar. Sci. 62, 516–527. doi: 10.1016/j.icesjms.2005.01.003
Rockstrom, J., Steffen, W., Noone, K., Persson, A., Chapin, F. S. I., Lambin, E. F., et al. (2009). A safe operating space for humanity. Nature 461, 472–475.
Rose, K. A., Allen, J. I., Artioli, Y., Barange, M., Blackford, J., Carlotti, F., et al. (2010). End-to-end models for the analysis of marine ecosystems: challenges. Issues, and next steps. Mar. Coast. Fish. 2, 115–130. doi: 10.1577/C09-059.1
Samhouri, J. F., Andrews, K. S., Fay, G., Harvey, C. J., Hazen, E. L., Hennessey, S. M., et al. (2017a). Defining ecosystem thresholds for human activities and environmental pressures in the California current. Ecosphere 8:1860. doi: 10.1002/ecs2.1860
Samhouri, J. F., Stier, A. C., Hennessey, S. M., Novak, M., Halpern, B. S., and Levin, P. S. (2017b). Rapid and direct recoveries of predators and prey through synchronized ecosystem management. Nat. Ecol. Evol. 1:0068. doi: 10.1038/s41559-016-0068
Samhouri, J. F., Levin, P. S., and Ainsworth, C. H. (2010). Identifying thresholds for ecosystem-based management. PLoS One 5:e8907. doi: 10.1371/journal.pone.0008907
Samhouri, J. F., Levin, P. S., and Harvey, C. J. (2009). Quantitative evaluation of marine ecosystem indicator performance using food web models. Ecosystems 12, 1283–1298. doi: 10.1007/s10021-009-9286-9289
Scott, E., Serpetti, N., Steenbeek, J., and Heymans, J. J. (2015). A stepwise fitting procedure for automated fitting of ecopath with ecosim models. SoftwareX 5, 25–30. doi: 10.1016/j.softx.2016.02.002
Shephard, S., Greenstreet, S. P. R., Piet, G. J., Rindorf, A., and Dickey-collas, M. (2015). Surveillance indicators and thier use in implementation of the marine strategy framework directive. ICES J. Mar. Sci. 72, 2269–2277. doi: 10.1093/icesjms/fsv131
Shin, Y., and Cury, P. (2001). Exploring fish community dynamics through size-dependent trophic interactions using a spatialized individual-based model. Aquat. Living Resour. 14, 65–80.
Shin, Y. J., Houle, J. E., Akoglu, E., Blanchard, J. L., Bundy, A., Coll, M., et al. (2018). the specificity of marine ecological indicators to fishing in the face of environmental change: a multi-model evaluation. Ecol. Indic. 89, 317–326. doi: 10.1016/j.ecolind.2018.01.010
Shin, Y.-J., and Shannon, L. J. (2010). Using indicators for evaluating, comparing, and communicating the ecological status of exploited marine ecosystems. 1. The INDISEAS project. ICES J. Mar. Sci. 67, 686–691. doi: 10.1093/icesjms/fsp273
Shin, Y.-J., Shannon, L. J., Bundy, A., Coll, M., Aydin, K., Bez, N., et al. (2010). Using indicators for evaluating, comparing, and communicating the ecological status of exploited marine ecosystems. 2. setting the scene. ICES J. Mar. Sci. 67, 692–716. doi: 10.1093/icesjms/fsp294
Smith, A. D. M., Fulton, E. A., Hobday, A. J., Smith, D. C., and Shoulder, P. (2007). Scientific tools to support the practical implementation of ecosystem-based fisheries management. ICES J. Mar. Sci. 64, 633–639. doi: 10.1093/icesjms/fsm041
Smith, M. D., Fulton, E. A., and Day, R. W. (2015). Using an atlantis model of the southern benguela to explore the response of ecosystem indicators for fisheries management. Environ. Model. Softw. 69, 23–41. doi: 10.1016/j.envsoft.2015.03.002
Steenbeek, J., Buszowski, J., Christensen, V., Akoglu, E., Aydin, K., Ellis, N., et al. (2016). Ecopath with Ecosim as a model-building toolbox: source code capabilities, extensions, and variations. Ecol. Modell. 319, 178–189. doi: 10.1016/j.ecolmodel.2015.06.031
Steenbeek, J., Corrales, X., Platts, M., and Coll, M. (2018). Ecosampler: a new approach to assessing parameter uncertainty in Ecopath with Ecosim. SoftwareX 7, 198–204. doi: 10.1016/j.softx.2018.06.004
Stelzenmüller, V., Coll, M., Mazaris, A. D., Giakoumi, S., Katsanevakis, S., Portman, M. E., et al. (2018). A risk-based approach to cumulative effect assessments for marine management. Sci. Total Environ. 612, 1132–1140. doi: 10.1016/j.scitotenv.2017.08.289
Tam, J. C., Link, J. S., Large, S. I., Andrews, K., Friedland, K. D., Gove, J., et al. (2017a). Comparing apples to oranges: common trends and thresholds in anthropogenic and environmental pressures across multiple marine ecosystems. Front. Mar. Sci. 4:282. doi: 10.3389/fmars.2017.00282
Tam, J. C., Link, J. S., Rossberg, A. G., Rogers, S. I., Levin, P. S., Rochet, M.-J., et al. (2017b). Towards ecosystem-based management: identifying operational food-web indicators for marine ecosystems. ICES J. Mar. Sci. 74, 2040–2052. doi: 10.1093/icesjms/fsw230
Thrush, S., Hewitt, J. E., Lundquist, C., Townsend, M., and Lohrer, A. M. (2011). A Strategy to Assess Trends IN The Ecological Integrity of New Zealand’s Marine Ecosystems. Prepared for Department of Conservation. Hamilton, New Zealand. Available at: https://www.doc.govt.nz/Documents/conservation/marine-and-coastal/marine-protected-areas/ecological-integrity-marine-ecosystems.pdf (accessed September 2017).
Tittensor, D. P., Eddy, T., Lotze, H. K., Galbraith, E., Cheung, W., Barange, M., et al. (2017). A protocol for the intercomparison of marine fishery and ecosystem models: fish-MIP v1.0. Geosci. Model Dev. 11, 1421–1442.
Travers, M., Shin, Y. J., Jennings, S., and Cury, P. (2007). Towards end-to-end models for investigating the effects of climate and fishing in marine ecosystems. Prog. Oceanogr. 75, 751–770. doi: 10.1016/j.pocean.2007.08.001
Walters, C., Pauly, D., and Christensen, V. (1999). Ecospace: prediction of mesoscale spation patterns in trophi relatiohsips of exploited ecosystem, with empahsis on the impacts of marine protected areas. Ecosystems 2, 539–554. doi: 10.1007/s100219900101
Walther, Y. M., and Mollmann, C. (2014). Bringing integrated ecosystem assessments to real IFE: a scientific framework for ICES. ICES J. Mar. Sci. 71, 1183–1186. doi: 10.1093/icesjms/fst161
Weijerman, M., Fulton, E. A., Janssen, A. B. G., Kuiper, J. J., Leemans, R., Robson, B. J., et al. (2015). How models can support ecosystem-based management of coral reefs. Prog. Oceanogr. 138, 559–570. doi: 10.1016/j.pocean.2014.12.017
Keywords: Atlantis, Ecopath with Ecosim, indicators, emergent properties, ecosystem-based management, cumulative impacts, ecosystem-level reference points
Citation: Tam JC, Fay G and Link JS (2019) Better Together: The Uses of Ecological and Socio-Economic Indicators With End-to-End Models in Marine Ecosystem Based Management. Front. Mar. Sci. 6:560. doi: 10.3389/fmars.2019.00560
Received: 28 February 2019; Accepted: 26 August 2019;
Published: 18 September 2019.
Edited by:
Dorte Krause-Jensen, Aarhus University, DenmarkReviewed by:
Donald F. Boesch, University of Maryland Center for Environmental Science (UMCES), United StatesChristopher James Brown, Griffith University, Australia
Copyright © 2019 Tam, Fay and Link. This is an open-access article distributed under the terms of the Creative Commons Attribution License (CC BY). The use, distribution or reproduction in other forums is permitted, provided the original author(s) and the copyright owner(s) are credited and that the original publication in this journal is cited, in accordance with accepted academic practice. No use, distribution or reproduction is permitted which does not comply with these terms.
*Correspondence: Jamie C. Tam, amFtaWVjdGFtLnBoZEBnbWFpbC5jb20=
†Present address: Jamie C. Tam, Bedford Institute of Oceanography, Fisheries and Oceans Canada, Dartmouth, NS, Canada