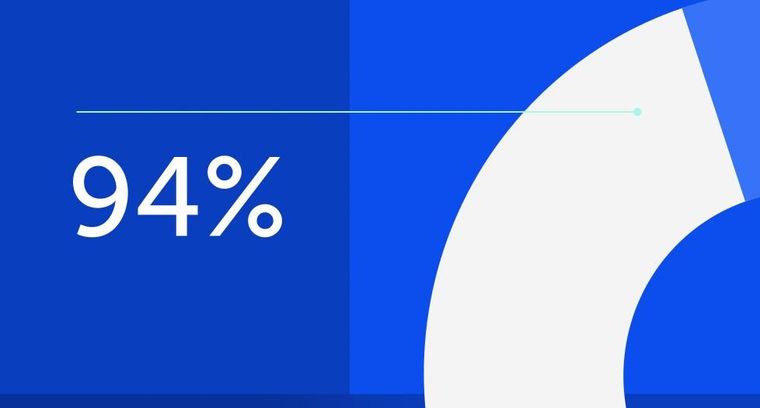
94% of researchers rate our articles as excellent or good
Learn more about the work of our research integrity team to safeguard the quality of each article we publish.
Find out more
ORIGINAL RESEARCH article
Front. Mar. Sci., 04 September 2019
Sec. Coastal Ocean Processes
Volume 6 - 2019 | https://doi.org/10.3389/fmars.2019.00541
Ferromanganese (FeMn) concretions are mineral precipitates found on soft sediment seafloors both in the deep sea and coastal sea areas. These mineral deposits potentially form a three-dimensional habitat for marine organisms, and contain minerals targeted by an emerging seabed mining industry. While FeMn concretions are known to occur abundantly in coastal sea areas, specific information on their spatial distribution and significance for marine ecosystems is lacking. Here, we examine the distribution of FeMn concretions in Finnish marine areas. Drawing on an extensive dataset of 140,000 sites visited by the Finnish Inventory Programme for the Underwater Marine Environment (VELMU), we examine the occurrence of FeMn concretions from seabed mapping, and use spatial modeling techniques to estimate the potential coverage of FeMn concretions. Using seafloor characteristics and hydrographical conditions as predictor variables, we demonstrate that the extent of seafloors covered by concretions in the northern Baltic Sea is larger than anticipated, as concretions were found at ∼7000 sites, and were projected to occur on over 11% of the Finnish sea areas. These results provide new insights into seafloor complexity in coastal sea areas, and further enable examining the ecological role and resource potential of seabed mineral concretions.
Mineral precipitates found on the surface of soft bottom seafloors are ubiquitous in the world’s oceans. Formed through a combination of biogeochemical processes, mineral concretions hold high concentrations of iron, manganese, phosphorus, copper, cobalt, and rare earth elements (Yli-Hemminki et al., 2014; Kuhn et al., 2017). Mineral precipitates come in many shapes and sizes, and according to their shape, they may be referred to in many terms. In the deep sea, common precipitates include polymetallic nodules or crusts, whereas their shallower shelf sea counterparts are often simply referred to as concretions, irrespective of their shape. In many coastal sea areas, the term ferromanganese concretion is established to underline the high concentration of these elements in shallow-water precipitates. Despite the widespread occurrence of shallow-water concretions, considerably more research effort has been invested in studying the distribution of deep-sea nodules (Gazis et al., 2018; Peukert et al., 2018b; Alevizos et al., unpublished), with only scattered observations of shelf sea and coastal concretions (EMODnet Geology, 2019).
Concretions occur on the seafloor as a layer on top of the underlying sediment, or they are covered by thin layer of recent silty-clay muds (Zhamoida et al., 2017). They are formed through chemical reactions driven by differences in redox potential, creating alternating layers of Fe and Mn (Axelsson et al., 2002; Gasparatos et al., 2005). Concretion formation is further driven by microbial reduction, and the diverse bacterial communities associated with concretions affect both their growth and dissolution (Zhang et al., 2002; Yli-Hemminki et al., 2014). As a result of their complex formation mechanisms, the distribution and abundance of mineral concretions on the seafloor is heterogeneous, and they are found in variable shapes and sizes, ranging from < 2 mm buckshot concretions to crusts of >1 m. They are present in a variety of geological settings, and their growth rates vary, depending on environmental conditions, from 0.003 to 0.3 mm a–1 (Zhamoida et al., 2007; Grigoriev et al., 2013). Under oxygen rich conditions, iron and manganese tend to form oxides, contributing to the growth of concretions, while in anoxic conditions concretions are partly dissolved (Zhamoida et al., 2007; Yli-Hemminki et al., 2016).
Previous studies have observed concretions mainly below 40 m depth on ridges and slopes above the depth zone of permanent mud and clay deposition, but less so near the coast in shallower waters (Boström et al., 1982). The major areas of ferromanganese concretions have been concluded to be found on the fringes of seafloor depressions (Glasby et al., 1997), with only scattered occurrences in shallower areas above 40 m depth (Zhamoida et al., 2007). While mineral concretions are known to exist in many parts of coastal sea areas, e.g., in the Black Sea (Baturin, 2010) north-east Atlantic Ocean (González et al., 2010), South China Sea (Zhong et al., 2017), and Kara Sea (Vereshchagin et al., 2019), and the processes affecting their formation have been intensively studied (Ingri, 1985b; Baturin, 2010; González et al., 2010), specific information on their abundance and spatial coverage is still lacking.
Although ferromanganese concretions fields and polymetallic nodules are classified as an underwater habitat type in several habitat classification systems (HELCOM, 2013), their role in marine ecosystems has similarly been left unaddressed. Mineral precipitates form hard substrates on predominantly soft seafloors, and they are therefore suggested to increase habitat complexity (Vanreusel et al., 2016) and to provide shelter from seafloor erosion (Kotilainen et al., 2017). Due to their capacity to absorb heavy metals and phosphorus, FeMn concretions may further be considered as natural filters on the seafloor (Hlawatsch et al., 2002) and they likely act as a buffering system between oxidized and reduced conditions (Zhamoida et al., 2007). Their ability to trap heavy metals and rare earth elements is related to the mineralogy of the concretions, with especially Mn oxides having a high sorption capacity for metal cations (Miyata et al., 2007). In addition, the bacteria associated with the concretions have been found to be able to degrade crude oil and polycyclic aromatic hydrocarbons (Reunamo et al., 2017). Although mineral deposits are partly biogenically formed, it is undecided whether they constitute a biogenic habitat type that should be taken into account in marine conservation.
Due to an increasing demand for critical raw materials, mineral concretions containing commercially exploitable metals are of interest for seabed mineral extraction both in the deep sea and coastal areas (Hannington et al., 2017; Peukert et al., 2018a). Although shallow-water concretions are not being industrially exploited yet, experimental extraction has already taken place in the eastern Gulf of Finland, the Baltic Sea (Zhamoida et al., 2017). In this area, the total weight of ore material concentrated in spheroidal concretions was estimated to be approximately six million tonnes or approximately one million tonnes of manganese metal (Zhamoida et al., 1996). The resource potential of concretions is variable, with the metal concentrations related to the environmental conditions they form in (Menendez et al., 2018; Vereshchagin et al., 2019). However, with technological advances enabling more efficient seabed resource extraction, this increasing interest must be taken into account in spatial planning and governance of marine areas. In order to make informed decisions to support sustainable use of marine space and resources, several knowledge gaps on the spatial extent and ecological importance of concretion fields must be addressed.
Out of the shallow sea areas where FeMn concretions have been observed, concretion fields in the northern Baltic Sea have been deemed particularly abundant (Winterhalter, 1966; Boström et al., 1982). In this study, we examine the distribution of ferromanganese concretions in the Finnish marine areas, and demonstrate how geological and oceanographic data may be used to identify areas suitable for concretion formation. Using an extensive dataset from the seabed mapping by the Finnish Inventory Programme for the Underwater Marine Environment (VELMU), our aim is to quantify the potential area where concretions are found, and to examine the most important environmental drivers of their occurrence. We apply Gradient Boosting Machine (GBM) and its extension Boosted Regression Trees to generalize the relationship between concretions and the environmental conditions in areas where they are found in order to estimate their spatial extent. Identifying the distribution and drivers of FeMn concretion fields in coastal sea areas provides new insights into their potential importance as a habitat type, and enables assessing the extent of marine mineral resources in shelf sea areas.
Our study focuses on the Finnish marine areas (territorial waters and exclusive economic zones) in the northern Baltic Sea, characterized by shallow, brackish water and a salinity gradient from north to south, with heterogeneous seafloor and a myriad of islands (∼100,000). The archipelago areas are generally very shallow, less than 50 m, and the deepest parts (100–300 m) are located in the open sea in the middle of the basins surrounding Finland. Given the limited water exchange only through the Danish straits, having a strong vertical density stratification, and being surrounded by areas of high agricultural discharge (Conley et al., 2009), the Baltic Sea is vulnerable to low oxygen conditions, with frequent and occasional hypoxia occurring in many parts of the basins (Conley et al., 2002, 2011; Virtanen et al., 2019).
The seabed of Finland’s coastal areas, especially in the archipelago areas, is geologically very diverse. Crystalline bedrock areas provide more diverse seabed environments than sedimentary rock areas (Kaskela and Kotilainen, 2017). The main factors that have contributed to the characteristics of the seafloor in the Finnish sea areas include the pre-glacial bedrock surface and processes related to it, glacial erosion and deposition, and post-glacial sedimentary processes (Winterhalter et al., 1981; Kaskela and Kotilainen, 2017).
The bedrock of the Finnish sea areas consists mainly of Paleoproterozoic crystalline basement rocks that are exposed locally along the coast. Sedimentary rocks cover the crystalline bedrock in tectonic depressions in the Åland Sea, and in the central Bothnian Sea and the Bothnian Bay (Winterhalter et al., 1981; Koistinen et al., 2001). The majority of the seabed sediments in the Finnish sea areas have been deposited during or after the last glaciation. The whole sea area was deglaciated by ∼10 cal. ka BP (Svendsen et al., 2004; Stroeven et al., 2016). The glacial sediments consist mainly of diamicton (till), glaciofluvial formations, and glacial (varved) clays and silts, and post-glacial sediments are mainly fine grained sediments. The crystalline bedrock is characterized by several ancient tectonic lineaments and fracture zones that divide the bedrock into blocks, also evident on the seafloor (Härme, 1961; Tuominen et al., 1973; Winterhalter et al., 1981).
The spatial distribution of different substrate types at the seafloor (surface) is very patchy. Factors influencing sediment erosion, transport, and accumulation and thus sediment distribution at the seafloor include, e.g., current velocity, water depth, underwater topography, bedrock, distance from the coast (and river mouths), land uplift, water masses, climate, biological productivity, and benthic fauna (Kaskela et al., 2019). Affected by these spatially and temporally varying processes, the seafloor can be composed of different type and age of substrates, from glacial till to modern mud.
Datasets for our study were derived from the Finnish Inventory Programme for the Underwater Marine Environment (VELMU). During 2004–2018 VELMU has collected information on species, communities and habitats from over 140,000 locations with SCUBA diving and underwater video methods (Virtanen et al., 2018). The visited sites cover all environmental gradients of the Finnish coast, extending from exposed offshore areas to enclosed bays with murky waters and limited water exchange. The data are viewable at the VELMU Map Service: paikkatieto.ymparisto.fi/VELMU_mapservice/.
Data have been collected mostly through random stratified sampling, with targeted inventories (grids of observations) implemented on certain marine habitats, for instance on underwater reefs. Scientific diving focused on collecting data from ∼100 m long dive transects. The coverages (%) of all macrophytes and sessile benthic invertebrates, as well as different bottom substrates, including FeMn concretions, were estimated every horizontal 10 m or vertical 1 m from inspection squares of 1, 2, or 4 m2. Drop-videos, mapping approximately 20 m2 of seabed, were recorded at stratified randomized locations and coverages of species and seabed substrates were analyzed from the videos. Stratified random sampling scheme was based on environmental gradients, such as depth, exposure, salinity and turbidity, and inventories were randomized to cover different environmental gradient combinations.
The concretion coverages were reported on a scale from 0.1 to 100%. For modeling, we produced two main response variables that describe: (1) potential concretion occurrences (0/1), with presence thresholds based on four different percent coverages: >0.1% (all observations and presence observations without information on coverages), >10% (abundant concretions), >50% (substantial cover) and >70% (major concretion fields), and (2) abundances (continuous response variable, 0–100%), where the quantity of FeMn concretions is based on observed coverages. The rationale for using different thresholds is that abundance estimates in the lower end of percentage cover are not very reliable, as detection accuracy may be lowered by water turbidity or heavy sedimentation. Moreover, detection accuracy depends on the observation method, SCUBA diving being a more reliable method than the video. After data pruning, the total dataset available for modeling consisted of 129,873 locations. We included only 10% of sites where concretions were recorded absent, as we wanted to keep the modeling times reasonable. Modeling data, including all presences and the reduced number of absences, resulted in ∼20,000 sites, which is enough for building reliable models. We split the dataset with a ratio of 40:60 for model training and testing the model performance. We kept the model training set smaller to reduce computing times, as we have more than enough data for producing the models.
Model predictors were selected based on previous studies on FeMn concretions (Table 1). For modeling the occurrence distributions and abundances of concretions, we utilized environmental predictors characterizing seabed quality (Virtanen et al., 2018, 2019). We further developed predictors describing hydro-chemical properties of the marine environment, thought to be relevant for ferromanganese concretions. Data on the environmental predictors were derived from the Finnish national environmental monitoring database Hertta and the public bathymetry databases of the Finnish Transport Infrastructure Agency (Väylä).
To generalize the relationship between concretions and the environment where they are found, and to predict the spatial distribution of concretion fields, we used GBM and its extension Boosted Regression Trees (BRT) (Friedman et al., 2000; Breiman, 2017). In BRT, every new tree (i.e., model) is grown by concentrating on the residuals of previously fitted ones (boosting), thus minimizing the prediction error (De’ath and Fabricius, 2000). Ideal parameters for our concretion models were tested with different combinations for interaction depth (2–5), and step-size reduction (0.1, 0.01, 0.005). A bag fraction of 0.7 was set for all models to introduce some stochasticity and to avoid models to follow too closely the data used in training. For the development of models, we used 10-fold cross-validation where training data (∼8000 sites) was partitioned into 10 subsets (without replacement), and each group was left for testing the model, and remaining ones for training the models. After each fold, the optimal number of trees was determined based on minimizing the residual deviance of models. The final best model based on cross-validation was used to predict the concretion distribution probabilities and abundances for the testing data (∼12,000 sites). All models were extrapolated to the full seascape at a resolution of 20 m, and were repeated 10 times with randomly shuffled training datasets (not to be confused with the cross-validation subsets). Thus, we produced 50 model versions, 40 for distributions with different thresholds and 10 for abundances. The same modeling protocol was repeated for all of the five model types (Figure 1). We performed all analyses in R 3.5.0 (R, 2018) with package “gbm” (Greenwell et al., 2018) and custom functions from Elith et al. (2008).
Figure 1. Schematic figure of the modeling approach. The same process was repeated for all of the four models on relative concretion coverages, and the concretion abundance model.
Spatial autocorrelation (SAC), i.e., similarity of observations close to each other, violates the underlying assumptions of statistical models, and may deteriorate the prediction ability (exaggerate or underestimate the predictions) (Legendre, 1993; Legendre et al., 2002). Although tree-based methods have shown to be a useful tool for handling SAC (Crase et al., 2012), all ecological data hold spatial structures that need to be accounted for. We estimated SAC based on spatial correlograms from R package “ncf” (Bjornstad, 2018). Not surprisingly, our data structure showed tendencies to spatial correlation, as grids of observations and transect lines of dives have been sampled close to each other. We handled SAC by introducing a residuals autocovariate (RAC) term to final models, derived from the initial sets of models fitted only with environmental predictors (Crase et al., 2012). We estimated the RAC using inverse distance weighting and focal mean calculations from estimated residuals for each grid cell, with a first-order neighborhood of eight adjacent cells.
Estimation of predictive performances of concretion distribution models was based on the ability to identify an area as such where concretions are most probably found. Models were evaluated with the Area Under the Curve (AUC) and True Skill Statistics (TSS). AUC and TSS are measures of detection accuracies of true positives (sensitivity) and true negatives (specificity), respectively (Allouche et al., 2006; Jiménez-Valverde and Lobo, 2007). Estimation of model fits were based on cross-validated AUC and TSS, whereas predictive performances (AUC, TSS) were estimated from independent data (∼12,000 sites), not included in the model training process. For the concretion abundance models (percent coverages 0.1–100%) we calculated the coefficient of determination (R2) and Mean Absolute Error (MAE).
Finally, we evaluated the potential area (%) of seafloors where concretions are most probably found. Here, we report the minimum and median area of seafloors where concretions are potentially found, based on an agreement between 10 different modeling versions for each model.
Probability predictions were transformed into binary classes of presence/absence. Although classification decreases the information content, it is useful for illustrating the most probable areas of concretion occurrence. There are various approaches for determining presence/absence thresholds that are based on the confusion matrix, i.e., how well the model captures true/false presences or true/false absences. Usually the threshold is defined to maximize the agreement between observed and predicted distributions (Liu et al., 2005), but here we define thresholds objectively based on an agreement between predicted and observed concretion prevalence. This approach probably underestimates areas where concretions are found, and is used here as a conservative estimate for the occurrence of concretions in the study area.
Concretions were observed in all basins of the study area, with the exception of the southern Bothnian Sea (Figure 2). Overall, concretions were observed in 6831 locations. The most abundant deposits are reported from the Gulf of Finland and the Kvarken area in the Gulf of Bothnia. Mineral deposits were observed in depths of 0–75 m, spanning different seafloor types from muddy bottoms to rocky seafloors. In the northern parts of the study area, concretions were generally observed in shallower depths (<25 m) than in the southern parts.
Figure 2. Concretions found along the Finnish marine areas. Larger, light yellow points indicate areas of concretion fields (>70% coverages) and small, orange points of smaller concretion occurrences (0.1–10%).
Concretion models showed excellent performance based on 10-fold cross-validation and when evaluated against independent test data (mean AUC 0.987 ± 0.005) (Figure 3A). A similar pattern was found from TSS, evaluated from the full model on training data (mean TSS 0.960 ± 0.016), and for independent test data (mean TSS 0.888 ± 0.022) (Figure 3B). Models for the whole range of concretion occurrence (>0.1%) were slightly less performant, which supports the hypotheses that concretions at low coverages are less easily observed, and that reliability of observations may vary due to sedimentation and water turbidity. TSS was slightly lower in the independent test data for sensitivity, i.e., identification of true presences, which suggests our models have tendencies to slightly overfit. This does not, however, compromise the prediction ability, as median TSS for all models was above 0.851, which is considered excellent model performance (Figure 3B). Concretion abundance models performed also well, based on 10-fold cross validation across model versions, with a mean R2 of 0.856 ± 0.007, and for independent test data 0.791 ± 0.005 (Supplementary Table S1). Mean absolute errors (MAE) of concretion abundance models were across model versions 13.591 ± 1.963 (%) (Supplementary Table S1).
Figure 3. Model performances based on (A) Area Under the Curve (AUC) and (B) True Skill Statistics (TSS). Models were evaluated based on 10-fold cross-validations (CV), and the resulting final model using the full training data and independent test data, set aside from model development (test).
Depth was found to be the most influential predictor in the concretion models, regardless of the model in question (28.6 ± 5.7%), followed by total phosphorus (19.8 ± 3.4%), occasional hypoxia (7.3 ± 2.3%), frequent hypoxia (4.6 ± 1.2%), and depth-attenuated wave exposure (6.1 ± 3.2%) (Figure 4). Salinity, chlorophyll a, nitrogen, and ruggedness had smaller influences on the occurrence of concretions throughout the models.
Figure 4. Mean environmental predictor influence in different concretions models from the 10-fold cross-validation. Error bars present standard deviation of the runs for each model type. For abbreviations, cf. Table 1.
Partial dependence plots of the predictor variables (Figure 5) show the dependence of concretion occurrence predictions on the values of the predictor variables. Dense concretion occurrences were mostly related to deeper depths and to areas where waves flush the seafloor [high SWM(d)]. This suggests that concretions occur – or are more easily observed – in areas where sedimentation is low, rather than areas where wave exposure is lower and sediment accumulation is higher. Notably, concretions were predicted to occur in areas where there is a high probability for occasional hypoxia (high OH4.6), but low probability for frequent hypoxia (low FH4.6). Furthermore, concretion fields seem to occur on slopes and on edges of larger depressions (high TSI, Slope, and BPI2). Occurrences were related to areas with high total phosphorus (Ptot) content and higher surface salinity (Salsurf). Regarding the substrate, concretions were not predicted to be found on sandy seafloors or in areas where rocky substrates dominate.
Concretions were predicted to occur abundantly in the Gulf of Finland and in the Kvarken area, and over 50% of the models predicted concretions to occur in the central parts of Bothnian Sea and Bothnian Bay (Figure 6 and Supplementary Figures S1–S4).
Figure 6. Concretion prediction maps for concretion occurrences (>0.1%) and concretion fields (>70%).
For areas suitable for concretion fields, we report here only the agreement between different modeling rounds (10 for each model), reflecting the minimum area predicted as suitable for finding concretions according to each threshold criteria (cf. “Modeling”). Each model version was transformed into binary classes with a conservative estimate based on concretion prevalence. We report only areas where similar models agree upon the minimum area suitable for concretions, and the median based on all model versions (cf. Supplementary Figures S1–S4 for all estimates of the spatial distribution).
Concretions were predicted to occur on over 11% of the Finnish marine areas; certain model versions even predicted a coverage of over 20% (Figure 7). Concretion fields, where concretion coverage is >70%, were predicted to exist on ∼2% (minimum) and ∼5% (median) of the seafloor.
Figure 7. Proportional surface area of the potential areas for FeMn concretions in Finnish marine areas.
Detailed knowledge of underwater seascapes is essential for both conservation planning and sustainable use of marine areas and resources. In this work, we have shown that ferromanganese concretions are common and abundant in the northern Baltic Sea, and that topographical and hydrographical parameters provide a solid basis for predicting their occurrence in coastal sea areas. The applied models were successful in projecting the occurrence of FeMn concretions at different relative coverages throughout the Finnish marine areas, which expand over an extensive environmental gradient. To our knowledge, this is the first attempt to quantify the relative importance of environmental drivers in predicting the spatial distribution of biogeochemical seabed formations.
Both the empirical observations and the resulting predictions show a more extensive distribution of ferromanganese deposits in shallow marine areas than previously documented (Glasby et al., 1997). According to even the more conservative predictions of the applied models, FeMn concretions occur in over 11% of the Finnish marine areas. Compared to other underwater habitats in Finnish marine areas, FeMn concretion bottoms cover a substantial area (Kaskela and Kotilainen, 2017). For instance, all marine habitats listed in the EU Habitat Directive Annex I, such as reefs, underwater sandbanks, lagoons, narrow boreal inlets, etc., together only cover 6% of the Finnish sea area (Virtanen et al., 2018). While the role of concretion fields as a biogenic habitat type is not yet established, they potentially form an ecologically meaningful habitat especially in deeper areas, as highlighted in the assessment of threatened habitat types in Finland (Kotilainen et al., 2018). Therefore, it is striking how little attention these mineral deposits have gained thus far from an ecological perspective. It is further notable that concretions mainly occur in oxic sea areas, and are dissolved under permanent anoxia. As hypoxic seafloors may expand due to climate change and the associated increase in sedimentation and oxygen consumption (Meier et al., 2019), concretion fields may become more restricted in the future.
The findings of this study are in line with previous research on the drivers of concretion formation. Dense concretion fields were strongly associated with deeper areas, whereas the overall concretion occurrence was less depth-dependent, as observed by Zhamoida et al. (1996). Our results show that FeMn concretions occur on the fringes of deep basins, and particularly in areas of low sedimentation rates and low primary production. This confirms a large body of literature speculating on the impact of wave exposure and bottom currents in transporting dissolved elements for concretion growth (e.g., Glasby et al., 1997; Winterhalter, 2004; Zhamoida et al., 2007), as well as the impact of organic carbon content in regulating the redox potential of the environment, which enables the formation of mineral precipitates.
While the processes mediating concretion growth take place under oxic conditions, anoxic areas may play an important role in the availability of elements. Lateral transport of dissolved elements from hypoxic and anoxic areas is likely an important mechanism feeding concretion growth (Glasby et al., 1997; Zhamoida et al., 2007). The high relative importance of phosphorus indicates that concretions tend to form within or close to areas with high phosphorus concentrations that contribute to their growth. This may explain why concretions are common in slope areas that are bordering deeper basins, from which phosphorus is released under anoxic conditions. However, it is important to note that high phosphorous concentrations are correlated with high concentrations of other dissolved elements in bottom waters, and phosphorus does not necessarily directly drive concretion growth, but acts as a proxy for the availability of other elements.
The areas with the most notable spatial coverages of concretions span a strong environmental gradient, with salinity ranging from 8 in the Archipelago Sea to <3.5 in the northern parts of the Bay of Bothnia, as well as differing concentrations of dissolved iron and phosphorus across the basins (Leppäranta and Myrberg, 2009). While depth and phosphorus were found to be the key variables controlling concretion occurrence in our models, concretion fields were found in much shallower depths in the Gulf of Bothnia than in the more marine conditions in the Gulf of Finland and the Archipelago Sea, where also the dissolved phosphorus concentrations were higher. The dissolved phosphorus concentrations were not, however, correlated with depth in our data. The abundant deposits in both the Gulf of Finland and the Bay of Bothnia therefore suggest variable processes of concretion formation across the study area, likely depending on their environment of formation (Glasby et al., 1997; Wasiljeff, 2015). Despite the potential variations in concretion formation processes, the created models were successful in predicting the occurrences of concretions across the different northern Baltic Sea sub-basins.
In addition to successfully predicting concretion occurrence within our dataset, the spatial predictions of the concretion distributions from the applied models fit previous estimates based on point observations from previous studies (e.g., Glasby et al., 1997). Forming on the fringes of deeper basins, concretions form distinct belts that span hundreds of kilometers within the Bothnian Sea and the Gulf of Finland, extending from the Finnish marine areas to the Swedish, Estonian and Russian territorial waters (EMODnet Geology, 2019). As several of the predictors used in our models would be relatively easily available with a combination of bathymetric and oceanographic data, the approach may be extended to other basins of the Baltic Sea, and possibly further to other shelf sea areas.
While, we were able to pinpoint several factors that are probably important drivers for concretion formation, other underlying factors may also contribute to concretion growth. In particular, the chemical characteristics of the underlying sediment are likely to be an important driver of concretion formation (Zhamoida et al., 2017). However, detailed data on sediment characteristics are very scarce, and not of sufficient spatial resolution to be included in this approach. Furthermore, using only visual observations of concretion occurrences may undermine model performance in areas where waters are more turbid, or in environments with high sedimentation rates and a thick fluffy layer of sediment, leaving concretions partly or totally buried. As our environmental data on substrate type only accounts for the proportion of sandy or rocky bottoms, areas with mixed sediment types could also be suitable for concretion formation, as previously observed (Kaskela and Kotilainen, 2017). The mixed sediment type refers to substrates covered by a thin (2–20 cm thick) residual sediment (mainly silt/sand and gravel), which often cover the exposed glacial clays at the seabed (Kaskela et al., 2012). The model outputs should thus be interpreted as indications of suitable environments for concretion formation, rather than a description of absolute drivers and presence of the mineral precipitates. While many aspects regarding formation processes of mineral precipitates remain unresolved, our findings provide insights into the large-scale processes related to their distribution and abundance.
The substantial area potentially covered by FeMn concretions puts further pressure on examining the ecological importance of these environments for management purposes. With seabed mineral extraction as one of the priority areas of the European commission’s Blue Growth strategy (European Commission, 2012), there is a pressing need to decide whether these environments form a habitat that needs to be considered in spatial conservation and ecosystem-based maritime spatial planning. While we have reported here extensive areas where FeMn concretions are potentially found, our models cannot be used to assess the absolute quantity of these minerals, i.e., vertical thickness nor density of concretions. This must be considered when examining the mineral resource potential of concretion fields. In order to examine the overall tradeoffs of mineral extraction, the economic resource potential of ferromanganese concretions must be compared with ecosystem functions provided by these underwater environments to estimate the ecological and chemical risks and impacts of possible large-scale extraction activities (Kaikkonen et al., 2018). Only by combining sufficient ecological, geological and technological knowledge can socio-economically and environmentally sustainable marine resource governance be achieved.
The datasets generated for this study are available on request to the corresponding author.
LK, EV, AK, and KK designed the study. EV performed all the analyses with the help from LK. LK and EV wrote the main text and prepared the figures. All authors contributed to the writing and editing of the manuscript.
This work was supported by the Strategic Research Council at the Academy of Finland (Project SmartSea, Grant Nos. 292985 and 314225) and the Finnish Inventory Programme for the Underwater Marine Environment (VELMU), funded by the Finnish Ministry of the Environment.
The authors declare that the research was conducted in the absence of any commercial or financial relationships that could be construed as a potential conflict of interest.
We sincerely thank Tom Jilbert and Markku Viitasalo for their insightful comments that significantly improved this work. We also express our gratitude to the two reviewers whose suggestions helped to clarify this manuscript.
The Supplementary Material for this article can be found online at: https://www.frontiersin.org/articles/10.3389/fmars.2019.00541/full#supplementary-material
Allouche, O., Tsoar, A., and Kadmon, R. (2006). Assessing the accuracy of species distribution models: prevalence, kappa and the true skill statistic (TSS). J. Appl. Ecol. 43, 1223–1232. doi: 10.1111/j.1365-2664.2006.01214.x
Axelsson, M. D., Rodushkin, I., Baxter, D. C., Ingri, J., and Öhlander, B. (2002). High spatial resolution analysis of ferromanganese concretions by LA-ICP-MS. Geochem. Trans. 3:40. doi: 10.1039/b204262m
Baturin, G. N. (2009). Geochemistry of ferromanganese nodules in the Gulf of Finland, Baltic Sea. Lithol. Miner. Resour. 44, 411–426. doi: 10.1134/s0024490209050010
Baturin, G. N. (2010). Element composition of ferromanganese concretions in the Black Sea. Oceanology 50, 83–92. doi: 10.1134/S0001437010010108
Bekkby, T., Isachsen, P. E., Isæus, M., and Bakkestuen, V. (2008). GIS modeling of wave exposure at the seabed: a depth-attenuated wave exposure model. Mar. Geod. 31, 117–127. doi: 10.1080/01490410802053674
Boström, K., Burman, J.-O., and Ingri, J. (1983). A geochemical massbalance for the Baltic. Ecol. Bull. 35, 39–58.
Boström, K., Wiborg, L., and Ingri, J. (1982). Geochemistry and origin of ferromanganese concretions in the Gulf of Bothnia. Mar. Geol. 50, 1–24. doi: 10.1016/0025-3227(82)90058-5
Callender, E., and Böwser, C. J. (1976). “Freshwater ferromanganese deposits,” in Handbook of Strata-Bound and Stratiform Ore Deposits, ed. K. H., Wolf. (Amsterdam: Elesvier) 7, 341–394. doi: 10.1016/b978-0-444-41407-6.50011-8
Conley, D. J., Bjorck, S., Bonsdorff, E., Carstensen, J., Destouni, G., Gustafsson, B. G., et al. (2009). Hypoxia-related processes in the Baltic Sea. Environ. Sci. Technol. 43, 3412–3420.
Conley, D. J., Carstensen, J., Aigars, J., Axe, P., Bonsdorff, E., Eremina, T., et al. (2011). Hypoxia is increasing in the coastal zone of the Baltic Sea. Environ. Sci. Technol. 45, 6777–6783. doi: 10.1021/es201212r
Conley, D. J., Humborg, C., Rahm, L., Savchuk, O. P., and Wulff, F. (2002). Hypoxia in the Baltic Sea and basin-scale changes in phosphorus biogeochemistry. Environ. Sci. Technol. 36, 5315–5320. doi: 10.1021/es025763w
Crase, B., Liedloff, A. C., and Wintle, B. A. (2012). A new method for dealing with residual spatial autocorrelation in species distribution models. Ecography 35, 879–888. doi: 10.1111/j.1600-0587.2011.07138.x
De’ath, G., and Fabricius, K. E. (2000). Classification and regression trees: a powerful yet simple technique for ecological data analysis. Ecology 81, 3178–3192. doi: 10.1890/0012-9658(2000)081%5B3178:cartap%5D2.0.co;2
Elith, J., Leathwick, J. R., and Hastie, T. (2008). A working guide to boosted regression trees. J. Anim. Ecol. 77, 802–813. doi: 10.1111/j.1365-2656.2008.01390.x
EMODnet Geology (2019). EMODnet Geology, 2019. Marine Minerals – Europe © EMODnet Geology, European Commission, 2019. Available at: https://www.emodnet-geology.eu/data-products/ (accessed April 28, 2019).
European Commission (2012). Blue Growth: Opportunities for Marine and Maritime Sustainable Growth: Communication from the Commission to the European Parliament, the Council, the European Economic and Social Committee and the Committee of the Regions. Brussels: Publications Office of the European Union.
Friedman, J., Hastie, T., and Tibshirani, R. (2000). Additive logistic regression: a statistical view of boosting (with discussion and a rejoinder by the authors). Ann. Stat. 28, 337–407. doi: 10.1214/aos/1016120463
Gasparatos, D., Tarenidis, D., Haidouti, C., and Oikonomou, G. (2005). Microscopic structure of soil Fe-Mn nodules: environmental implication. Environ. Chem. Lett. 2, 175–178. doi: 10.1007/s10311-004-0092-5
Gazis, I.-Z., Schoening, T., Alevizos, E., and Greinert, J. (2018). Quantitative mapping and predictive modeling of Mn nodules’ distribution from hydroacoustic and optical AUV data linked by random forests machine learning. Biogeosciences 15, 7347–7377. doi: 10.5194/bg-15-7347-2018
Glasby, G. P., Emelyanov, E. M., Zhamoida, V. A., Baturin, G. N., Leipe, T., Bahlo, R., et al. (1997). Environments of formation of ferromanganese concretions in the Baltic Sea: a critical review. Geol. Soc. Lond. Spec. Publ. 119, 213–237. doi: 10.1144/gsl.sp.1997.119.01.14
Glasby, G. P., Uscinowicz, S., and Sochan, J. A. (1996). Marine ferromanganese concretions from the polish exclusive economic zone: influence of major inflows of North Sea water. Mar. Georesour. Geotechnol. 14, 335–352. doi: 10.1080/10641199609388321
González, F. J., Somoza, L., Lunar, R., Martínez-Frías, J., Rubí, J. A. M., Torres, T., et al. (2010). Internal features, mineralogy and geochemistry of ferromanganese nodules from the Gulf of Cadiz: the role of the mediterranean outflow water undercurrent. J. Mar. Syst. 80, 203–218. doi: 10.1016/j.jmarsys.2009.10.010
Greenwell, B., Boehmke, B., Cunningham, J., and Developers, G. (2018). gbm: Generalized Boosted Regression Models. R Package Version 21 4.
Grigoriev, A. G., Zhamoida, V. A., Gruzdov, K. A., and Krymsky, R. S. (2013). Age and growth rates of ferromanganese concretions from the gulf of Finland derived from 210Pb measurements. Oceanology 53, 345–351. doi: 10.1134/s0001437013030041
Hannington, M., Petersen, S., and Krätschell, A. (2017). Subsea mining moves closer to shore. Nat. Geosci. 10, 158–159. doi: 10.1038/ngeo2897
HELCOM (2013). “HELCOM HUB – technical report on the HELCOM underwater biotope and habitat classification,” in Proceedings of the Baltic Sea Environment Proceedings No. 139, (Helsinki: HELCOM), 96s.
Hlawatsch, S., Garbe-Schönberg, C. D., Lechtenberg, F., Manceau, A., Tamura, N., Kulik, D. A., et al. (2002). Trace metal fluxes to ferromanganese nodules from the western Baltic Sea as a record for long-term environmental changes. Chem. Geol. 182, 697–709. doi: 10.1016/S0009-2541(01)00346-1
Ingri, J. (1985a). Geochemistry of Ferromanganese Concretions and Associated Sediments in the Gulf of Bothnia. Ph.D. thesis, University of Luleå, Luleå.
Ingri, J. (1985b). Geochemistry of ferromanganese concretions in the Barents Sea. Mar. Geol. 67, 101–119. doi: 10.1016/0025-3227(85)90150-1
Jilbert, T., Asmala, E., Schröder, C., Tiihonen, R., Myllykangas, J.-P., Virtasalo, J. J., et al. (2018). Impacts of flocculation on the distribution and diagenesis of iron in boreal estuarine sediments. Biogeosci. Katlenburg-Lindau 15, 1243–1271. doi: 10.5194/bg-15-1243-2018
Jiménez-Valverde, A., and Lobo, J. M. (2007). Threshold criteria for conversion of probability of species presence to either–or presence–absence. Acta Oecol. 31, 361–369. doi: 10.1016/j.actao.2007.02.001
Kaikkonen, L., Venesjärvi, R., Nygård, H., and Kuikka, S. (2018). Assessing the impacts of seabed mineral extraction in the deep sea and coastal marine environments: current methods and recommendations for environmental risk assessment. Mar. Pollut. Bull. 135, 1183–1197. doi: 10.1016/j.marpolbul.2018.08.055
Kaskela, A. M., and Kotilainen, A. T. (2017). Seabed geodiversity in a glaciated shelf area, the Baltic Sea. Geomorphology 295, 419–435. doi: 10.1016/j.geomorph.2017.07.014
Kaskela, A. M., Kotilainen, A. T., Alanen, U., Cooper, R., Green, S., Guinan, J., et al. (2019). Picking up the pieces—harmonising and collating seabed substrate data for european maritime areas. Geosciences 9:84. doi: 10.3390/geosciences9020084
Kaskela, A. M., Kotilainen, A. T., Al-Hamdani, Z., Leth, J. O., and Reker, J. (2012). Seabed geomorphic features in a glaciated shelf of the Baltic Sea. Estuar. Coast. Shelf Sci. 100, 150–161. doi: 10.1016/j.ecss.2012.01.008
Koistinen, T., Stephens, M. B., Bogatchev, V., Nordgulen, Ø, Wennerström, M., Korhonen, J., et al. (2001). Geological Map of the Fennoscandian Shield, Scale 1:2 000 000. Trondheim: Geological Survey of Norway.
Kotilainen, A. T., Kaskela, A. M., Alanen, U., Kiviluoto, S., Kontula, T., Kostamo, K., et al. (2017). “Ferromanganese concretion bottoms as habitats and a renewable resource in the Baltic Sea,” in Proceedings of the 2017 Conference on Program and abstracts of GeoHab, ed. B. J. Todd (Dartmouth, NS: Geological Survey of Canada).
Kotilainen, A. T., Kiviluoto, S., Kurvinen, L., Sahla, M., Ehrnsten, E., Laine, A., et al. (2018). Itämeri. Julk.: Kontula, T. & Raunio, A. (toim.). Suomen luontotyyppien uhanalaisuus 2018. Luontotyyppien punainen kirja – Osa 2: Luontotyyppien kuvaukset (in Finnish, Summary in English). Helsinki: Suomen ympäristökeskus & ympäristöministeriö, 15–98.
Kuhn, T., Wegorzewski, A., Rühlemann, C., and Vink, A. (2017). “Composition, formation, and occurrence of polymetallic nodules,” in Deep-Sea Mining, ed. R. Sharma (Berlin: Springer), 23–63. doi: 10.1007/978-3-319-52557-0_2
Legendre, P. (1993). Spatial autocorrelation: trouble or new paradigm? Ecology 74, 1659–1673. doi: 10.2307/1939924
Legendre, P., Dale, M. R. T., Fortin, M.-J., Gurevitch, J., Hohn, M., and Myers, D. (2002). The consequences of spatial structure for the design and analysis of ecological field surveys. Ecography 25, 601–615. doi: 10.1034/j.1600-0587.2002.250508.x
Leppäranta, M., and Myrberg, K. (2009). Physical Oceanography of the Baltic Sea. Berlin: Springer Science & Business Media.
Liu, C., Berry, P. M., Dawson, T. P., and Pearson, R. G. (2005). Selecting thresholds of occurrence in the prediction of species distributions. Ecography 28, 385–393. doi: 10.1111/j.0906-7590.2005.03957.x
Meier, M., Edman, M., Eilola, K., Placke, M., Neumann, T., Andersson, H., et al. (2019). Assessment of uncertainties in scenario simulations of biogeochemical cycles in the Baltic Sea. Front. Mar. Sci. 6:46. doi: 10.3389/fmars.2019.00046
Menendez, A., James, R., Shulga, N., Connelly, D., and Roberts, S. (2018). Linkages between the genesis and resource potential of ferromanganese deposits in the atlantic, pacific, and arctic oceans. Minerals 8:197. doi: 10.3390/min8050197
Miyata, N., Tani, Y., Sakata, M., and Iwahori, K. (2007). Microbial manganese oxide formation and interaction with toxic metal ions. J. Biosci. Bioeng. 104, 1–8. doi: 10.1263/jbb.104.1
Peukert, A., Petersen, S., Greinert, J., and Charlot, F. (2018a). “Seabed mining,” in Submarine Geomorphology, eds A. Micallef, S. Krastel, and A. Savini (Cham: Springer), 481–502.
Peukert, A., Schoening, T., Alevizos, E., Köser, K., Kwasnitschka, T., and Greinert, J. (2018b). Understanding Mn-nodule distribution and evaluation of related deep-sea mining impacts using AUV-based hydroacoustic and optical data. Biogeosciences 15, 2525–2549. doi: 10.5194/bg-15-2525-2018
Reunamo, A., Yli-Hemminki, P., Nuutinen, J., Lehtoranta, J., and Jørgensen, K. S. (2017). Degradation of crude oil and PAHs in Iron–manganese concretions and sediment from the northern Baltic Sea. Geomicrobiol. J. 34, 385–399.
Sappington, J. M., Longshore, K. M., and Thompson, D. B. (2007). Quantifying landscape ruggedness for animal habitat analysis: a case study using bighorn sheep in the Mojave Desert. J. Wildl. Manag. 71, 1419–1426. doi: 10.2193/2005-723
Savchuk, O. P. (2000). Studies of the assimilation capacity and effects of nutrient load reductions in the eastern Gulf of Finland with a biogeochemical model. Boreal Environ. Res. 5, 147–163.
Stroeven, A. P., Hättestrand, C., Kleman, J., Heyman, J., Fabel, D., Fredin, O., et al. (2016). Deglaciation of fennoscandia. Quat. Sci. Rev. 147, 91–121. doi: 10.1016/j.quascirev.2015.09.016
Svendsen, J. I., Alexanderson, H., Astakhov, V. I., Demidov, I., Dowdeswell, J. A., Funder, S., et al. (2004). Late quaternary ice sheet history of northern Eurasia. Quat. Sci. Rev. 23, 1229–1271. doi: 10.1016/s0277-3791(03)00342-1
Tuominen, H. V., Aarnisalo, J., and Söderholm, B. (1973). Tectonic patterns in the central Baltic Shield. Bull. Geol. Soc. Finl. 45, 205–221.
Vanreusel, A., Hilario, A., Ribeiro, P. A., Menot, L., and Arbizu, P. M. (2016). Threatened by mining, polymetallic nodules are required to preserve abyssal epifauna. Sci. Rep. 6:sre26808. doi: 10.1038/srep26808
Vereshchagin, O. S., Perova, E. N., Brusnitsyn, A. I., Ershova, V. B., Khudoley, A. K., Shilovskikh, V. V., et al. (2019). Ferro-manganese nodules from the Kara Sea: mineralogy, geochemistry and genesis. Ore Geol. Rev. 106, 192–204. doi: 10.1016/j.oregeorev.2019.01.023
Virtanen, E. A., Norkko, A., Nyström-Sandman, A., and Viitasalo, M. (2019). Identifying areas prone to coastal hypoxia – the role of topography. Biogeosciences 16, 3183–3195. doi: 10.5194/bg-16-3183-2019
Virtanen, E. A., Viitasalo, M., Lappalainen, J., and Moilanen, A. (2018). Evaluation, gap analysis, and potential expansion of the Finnish marine protected area network. Front. Mar. Sci. 5:402. doi: 10.3389/fmars.2018.00402
Wasiljeff, J. (2015). Internal Structure and Geochemical Properties of Spheroidal Ferromanganese Concretions of the Baltic Sea. Helsinki: Helsingin yliopisto.
Weiss, A. (2001). “Topographic position and landforms analysis,” in Poster Presentation at ESRI User Conference, (San Diego, CA).
Winterhalter, B. (1966). Pohjanlahden ja Suomenlahden Rauta-Mangaani-Saostumista: Summary: Iron-Manganese Concretions from the Gulf of Bothnia and the Gulf of Finland. Espoo: Geologinen Tutkimuslaitos.
Winterhalter, B. (2004). “Ferromanganese concretions in the Gulf of Bothnia,” in Mineral Resources of the Baltic sea –Exploration, Exploitation and Sustainable Development, eds J. Harff, E. Emelyanov, M. Schmidt-Thome, and M. Spiridonov (Hanover: Zeitschrift fur Angewandte Geologie), 199–212.
Winterhalter, B., Flodén, T., Ignatius, H., Axberg, S., and Niemistö, L. (1981). Geology of the Baltic sea. Amsterdam: Elsevier.
Yli-Hemminki, P., Jørgensen, K. S., and Lehtoranta, J. (2014). Iron–manganese concretions sustaining microbial life in the Baltic Sea: the structure of the bacterial community and enrichments in metal-oxidizing conditions. Geomicrobiol. J. 31, 263–275. doi: 10.1080/01490451.2013.819050
Yli-Hemminki, P., Sara-Aho, T., Jørgensen, K. S., and Lehtoranta, J. (2016). Iron–manganese concretions contribute to benthic release of phosphorus and arsenic in anoxic conditions in the Baltic Sea. J. Soils Sediments 16, 2138–2152. doi: 10.1007/s11368-016-1426-1
Zhamoida, V., Grigoriev, A., Gruzdov, K., and Ryabchuk, D. (2007). The influence of ferromanganese concretions-forming processes in the eastern Gulf of Finland on the marine environment. Geol. Surv. Finl. Spec. Pap. 45, 21–32.
Zhamoida, V., Grigoriev, A., Ryabchuk, D., Evdokimenko, A., Kotilainen, A. T., Vallius, H., et al. (2017). Ferromanganese concretions of the eastern Gulf of Finland–environmental role and effects of submarine mining. J. Mar. Syst. 172, 178–187. doi: 10.1016/j.jmarsys.2017.03.009
Zhamoida, V. A., Butylin, W. P., Glasby, G. P., and Popova, I. A. (1996). The nature of ferromanganese concretions from the Eastern Gulf of Finland, Baltic Sea. Mar. Georesourc. Geotechnol. 14, 161–176. doi: 10.1080/10641199609388309
Zhang, F.-S., Lin, C.-Y., Bian, L.-Z., Glasby, G. P., and Zhamoida, V. A. (2002). Possible evidence for the biogenic formation of spheroidal ferromanganese concretions from the Eastern Gulf of Finland, the Baltic Sea. Baltica 15, 23–29.
Keywords: Baltic Sea, Gradient Boosting Machine (GBM), ferromanganese concretions, marine minerals, polymetallic nodules, seabed habitats, Species Distribution Modeling (SDM)
Citation: Kaikkonen L, Virtanen EA, Kostamo K, Lappalainen J and Kotilainen AT (2019) Extensive Coverage of Marine Mineral Concretions Revealed in Shallow Shelf Sea Areas. Front. Mar. Sci. 6:541. doi: 10.3389/fmars.2019.00541
Received: 11 July 2019; Accepted: 16 August 2019;
Published: 04 September 2019.
Edited by:
Juan Jose Munoz-Perez, University of Cádiz, SpainReviewed by:
Raul Martell-Dubois, National Commission for the Knowledge and Use of Biodiversity (CONABIO), MexicoCopyright © 2019 Kaikkonen, Virtanen, Kostamo, Lappalainen and Kotilainen. This is an open-access article distributed under the terms of the Creative Commons Attribution License (CC BY). The use, distribution or reproduction in other forums is permitted, provided the original author(s) and the copyright owner(s) are credited and that the original publication in this journal is cited, in accordance with accepted academic practice. No use, distribution or reproduction is permitted which does not comply with these terms.
*Correspondence: Laura Kaikkonen, bGF1cmEubS5rYWlra29uZW5AaGVsc2lua2kuZmk=
†These authors have contributed equally to this work
Disclaimer: All claims expressed in this article are solely those of the authors and do not necessarily represent those of their affiliated organizations, or those of the publisher, the editors and the reviewers. Any product that may be evaluated in this article or claim that may be made by its manufacturer is not guaranteed or endorsed by the publisher.
Research integrity at Frontiers
Learn more about the work of our research integrity team to safeguard the quality of each article we publish.