- 1Atmospheric and Oceanic Sciences, University of Colorado Boulder, Boulder, CO, United States
- 2ECMWF, Reading, United Kingdom
- 3Scripps Institution of Oceanography, University of California, San Diego, San Diego, CA, United States
- 4Indian Institute of Tropical Meteorology, Pune, India
- 5Department of Atmospheric Science, Colorado State University, Fort Collins, CO, United States
- 6United States Naval Research Laboratory, Monterey, CA, United States
- 7Meteorological Research Institute, Japan Meteorological Agency, Tsukuba, Japan
- 8National Oceanic and Atmospheric Administration, Earth System Research Laboratory, Physical Sciences Division, Boulder, CO, United States
- 9Bureau of Meteorology, Melbourne, VIC, Australia
- 10Earth Science and Engineering, King Abdullah University of Science and Technology, Thuwal, Saudi Arabia
- 11National Centers for Environmental Prediction, Climate Prediction Center, College Park, MD, United States
- 12Korea Institute of Ocean Science and Technology, Busan, South Korea
- 13Woods Hole Oceanographic Institution, Woods Hole, MA, United States
- 14Center for Computational Sciences, University of Tsukuba, Tsukuba, Japan
- 15School of Earth and Environmental Sciences/Research Institute of Oceanography, Seoul National University, Seoul, South Korea
- 16I.M. Systems Group (IMSG), National Oceanic and Atmospheric Administration, Environmental Modeling Center, College Park, MD, United States
- 17Department of Atmospheric and Oceanic Science, University of Maryland, College Park, College Park, MA, United States
- 18United States Naval Research Laboratory, Stennis Space Center, Hancock, MS, United States
- 19Mechanical Engineering, University of Massachusetts Dartmouth, Dartmouth, MA, United States
- 20Institute of Oceanology, Chinese Academy of Sciences, Qingdao, China
- 21National Oceanic and Atmospheric Administration, Pacific Marine Environmental Laboratory, Seattle, WA, United States
Subseasonal-to-seasonal (S2S) forecasts have the potential to provide advance information about weather and climate events. The high heat capacity of water means that the subsurface ocean stores and re-releases heat (and other properties) and is an important source of information for S2S forecasts. However, the subsurface ocean is challenging to observe, because it cannot be measured by satellite. Subsurface ocean observing systems relevant for understanding, modeling, and forecasting on S2S timescales will continue to evolve with the improvement in technological capabilities. The community must focus on designing and implementing low-cost, high-value surface and subsurface ocean observations, and developing forecasting system capable of extracting their observation potential in forecast applications. S2S forecasts will benefit significantly from higher spatio-temporal resolution data in regions that are sources of predictability on these timescales (coastal, tropical, and polar regions). While ENSO has been a driving force for the design of the current observing system, the subseasonal time scales present new observational requirements. Advanced observation technologies such as autonomous surface and subsurface profiling devices as well as satellites that observe the ocean-atmosphere interface simultaneously can lead to breakthroughs in coupled data assimilation (CDA) and coupled initialization for S2S forecasts. These observational platforms should also be tested and evaluated in ocean observation sensitivity experiments with current and future generation CDA and S2S prediction systems. Investments in the new ocean observations as well as model and DA system developments can lead to substantial returns on cost savings from disaster mitigation as well as socio–economic decisions that use S2S forecast information.
Introduction
Operational centers are undertaking a seamless extension of weather forecasts to Subseasonal-to-Seasonal (S2S) forecasts. There is a growing consensus that coupled atmosphere-ocean modeling will be essential to forecasts on S2S time scales (Penny and Hamill, 2017; Penny et al., 2019). The role of ocean-atmosphere coupling in S2S variability and its representation in models is one of the research foci of the World Meteorological Organization (WMO) S2S Prediction Project. In particular, the initialization and configuration of the ocean and sea ice are key modeling research issues1.
The role of ocean-atmosphere coupling in S2S variability must be well understood not only for improving atmospheric predictions but also for improving predictions relevant to the entire gamut of socio–economic scenarios encompassing everything from disaster mitigation to marine ecosystems and coastal management. Detailed studies to help improve strategies for coupled initialization are underway at various global modeling centers (e.g., ECMWF, NCEP, NRL; Lea et al., 2015; Frolov et al., 2016; Laloyaux et al., 2016).
Innovative observing technology in the subsurface ocean and at the air-sea interface will help us understand air–sea interaction and its role in S2S variability and prediction. S2S forecasts for high- and mid-latitudes can be improved with better observations of sea-ice thickness and concentration and ocean basin boundary currents (current intensity and stratification). Evaluating impacts of new ocean observing technology on ocean analyses and S2S forecasts will contribute to the design and the evolution of oceanic observing systems, such as the Tropical Pacific Observing System (TPOS-2020; Smith et al., 2019), the Atlantic Ocean Observing System (AtlantOS; Foltz et al., 2019), and regional boundary current observing systems (Todd et al., 2019).
Multi-system evaluation of observation impacts based on international collaboration is effective to deliver fair and robust information to society and observational agencies. Coordination by international groups such as CLIVAR and GODAE OceanView aims to support these efforts to make significant progress (see also Fujii et al., 2019). Here, we provide scientific rationales for ocean observations being important for S2S predictions, discuss gaps in observations, and recommend designs of observational and modeling experiments to evaluate the impact of ocean observations on S2S forecasts.
Need for Ocean Observations for S2S Predictions
The top 100 m of the ocean have 40 times the heat capacity of the entire atmosphere. This makes the upper ocean a major reservoir of heat energy for the weather/climate system and a critical component of S2S prediction. In addition to the ocean surface temperatures, several other potential sources of S2S predictability have been identified such as: tropical intraseasonal oscillation (namely, the Madden-Julian Oscillation, MJO; Vitart et al., 2012), subsurface ocean heat anomalies (Alexander et al., 1999; Vimont et al., 2001), and sea ice (Vitart et al., 2012). These phenomena serve as sources of S2S predictability because of their relative persistence or known oscillatory behaviors. Some of them are directly related to ocean conditions. In addition, there are requirements to correctly represent coastal SST, ocean boundary currents, ocean mixed-layer structures, and other oceanic fields relevant to teleconnections and regional variability on S2S timescales.
ENSO is the most dominant mode of climate variability, with a significant influence on S2S variability through global teleconnections (Yeh et al., 2018). The recharge and discharge of warm water volume (WWV) in the Tropical Pacific is closely related to ENSO variability (Jin, 1997; Meinen and McPhaden, 2000). Hence, accurate observations of the evolution of Tropical Pacific WWV can help improve ENSO process understanding and predictions on seasonal timescales. Accurate analysis and initialization of ocean sub-surface states in the equatorial Pacific is of great importance for S2S prediction (Fujii et al., 2015).
Air-sea coupling has been considered a key process that needs to be accurately represented in models for MJO and Monsoon IntraSeasonal Oscillation (MISO) prediction (DeMott et al., 2015 and references therein). MJO prediction skill is higher in coupled models compared to uncoupled atmosphere-only models (e.g., Woolnough et al., 2007; Subramanian et al., 2017). The biases in sea surface temperature in operational forecast models are known to impact the operational predictability of MISOs over Indian region (Sahai et al., 2019). Yet, our understanding of the exact processes of air-sea coupling that are crucial to MJO and MISO prediction is incomplete. The diurnal cycle in air-sea coupling associated with the MJO (Seo et al., 2014) and MISO needs to be observed and understood further. The myriad phenomena that govern the S2S variability in the Tropical region (e.g., Tropical Pacific) are depicted in the schematic shown in Figure 1. Comprehensive observations of these processes will help improve their representation and prediction in the S2S forecasting systems.
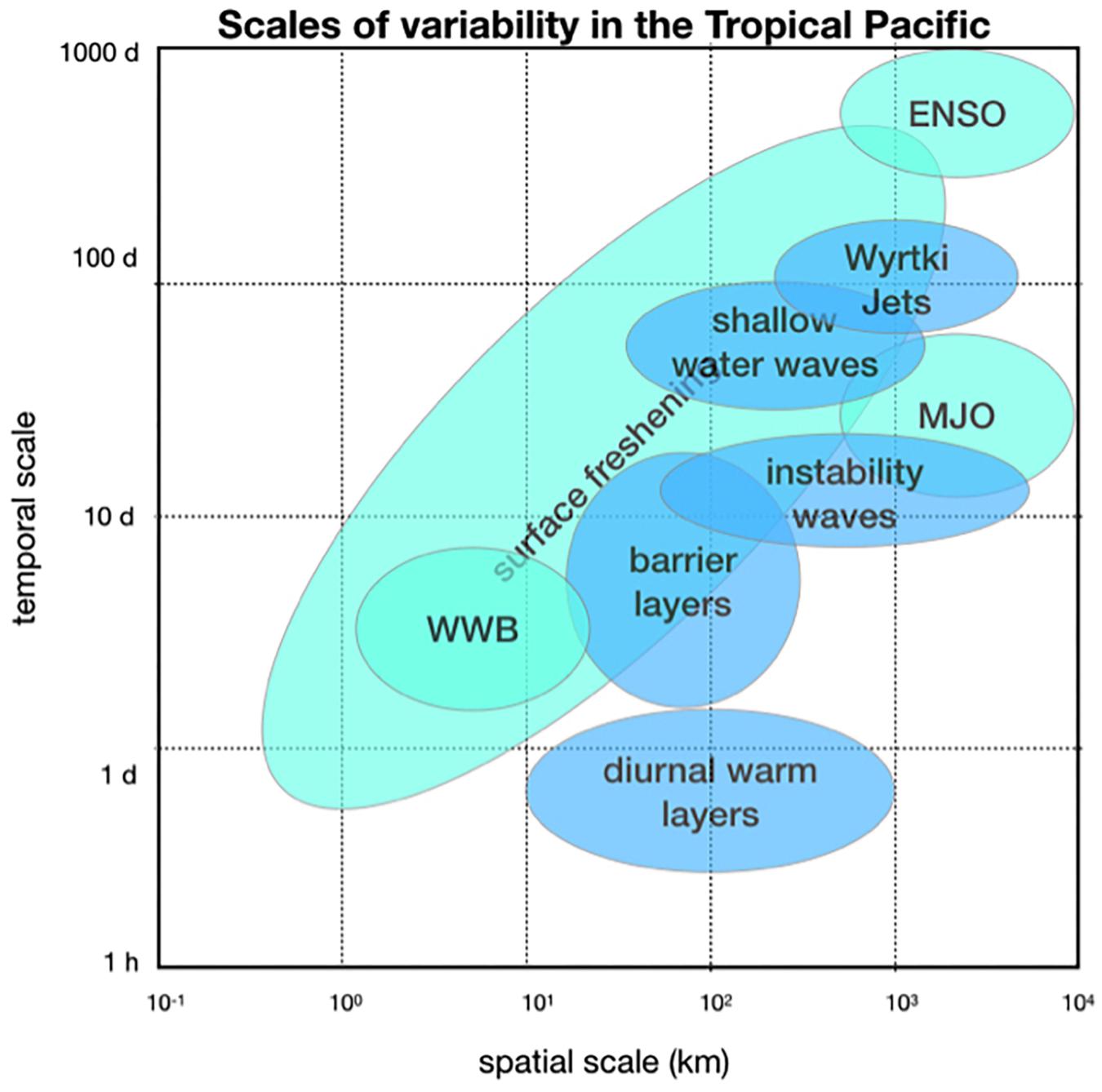
Figure 1. Schematic depicting spatial and temporal scales of phenomena in the ocean-atmosphere coupled system that govern the variability in the Tropical Pacific. Due to limitations in space, we have depicted only a few of the key processes. The blue shaded ovals represent ocean processes and green ovals represent atmospheric processes.
Another outstanding issue related to air-sea coupling is the MJO propagation over the Indo-Pacific Maritime Continent (MC), where some MJO events terminate as consequences of the “barrier effect” of the MC (Zhang and Ling, 2017). This barrier effect is often exaggerated in numerical models, which limits MJO prediction skill. Air–sea interaction in the MC seas plays a key role in determining the MJO propagation through the region. In situ ocean observations are needed for understanding air–sea interaction in the region and their representation in S2S prediction models. The international program Years of the Maritime Continent (YMC, 2017–2020) includes several simultaneous measurements of the upper ocean, atmosphere, and their interactions. These observations provide sorely needed in situ observations from the region.
Predicting sea ice at S2S timescales has also been challenging, but progress is being made (Zampieri et al., 2018). Sea-ice prediction at S2S time scales is challenging in part due to a lack of in situ observations to develop adequate sea-ice models and initialization techniques (Day et al., 2014; Guemas et al., 2014, 2016). The unseasonably early and rapid sea-ice retreat in the Bering Sea in spring 2018 and unprecedented springtime retreat of Antarctic sea ice in 2016 (e.g., Turner et al., 2017) highlight priorities that should be accurately captured by S2S prediction models. Sea-ice-related predictions will not be possible without relevant observations that provide needed initial conditions in terms of upper ocean current and temperature, surface radiation and heat fluxes, surface wind, and wave height in and around the marginal ice zone (MIZ) and underneath sea ice during the sea-ice growing and retreating seasons.
Mid-latitude SST anomalies are a source of predictability on S2S time scales (McKinnon et al., 2016; Saravanan and Chang, 2019 and references therein). Regional warm SST anomalies that persist for 5 days or longer, known as the marine heat waves (Frölicher and Laufkötter, 2018), are being studied as a potential source and target of S2S predictability. Recently, a marine heat wave near the west coast of North America between 2013 and 2015 (Bond et al., 2015; Di Lorenzo and Mantua, 2016; Zaba and Rudnick, 2016) including the 2015–2016 El Niño event persisted for over a season and is being studied as a cause of several regional anomalies in both atmospheric and land conditions. The majority of marine heat waves, however, are not covered by in situ observations. This makes it difficult to understand the physical processes responsible for the formation and termination of the heat waves, let alone their prediction. Representation of marine heat waves requires good observations of air-sea fluxes, as well as the ocean mixed layer.
Need for New Observing Technology for S2S Predictions
The current global ocean observing system comprises a range of in situ and satellite based observing networks, including moored buoys, Argo profiling floats (Roemmich et al., 2019), surface drifters, satellites, ship-based measurement, and gliders (Testor et al., 2019). In addition, emerging observing technology offers the promise of innovative devices [e.g., Autonomous Surface Vehicles (ASVs)] that can supplement the conventional platforms to fill gaps in ocean observations for S2S prediction.
Moored buoys provide high-frequency sampling to resolve the surface diurnal cycle. Argo profiling floats measure oceanic temperature and salinity profiles throughout the top 2000 m of the ocean with near global coverage. Surface drifters (Centurioni, 2018) measure mixed-layer currents while also recording sea surface temperature (SST) and sea-level atmospheric pressure (SLP) globally. These different platforms provide near-real time observations through the Global Telecommunication System for initialization of global prediction models. The SLP data from drifters are known to have a significant and positive impact on numerical weather prediction (Centurioni et al., 2017; Horányi et al., 2017). They are extremely valuable for the global in situ ocean observation network that supports the S2S forecasting systems for initialization and verification. Skillful prediction on S2S timescales demands high temporal (hourly) and vertical resolution (<1 m) observations in the upper ocean (Bernie et al., 2008; Seo et al., 2014).
Current satellites do not provide measurements for all surface variables critical to air-sea fluxes (e.g., surface air temperature, humidity, and downwelling radiation), nor can they measure within the ocean interior. China-France Oceanography SATellite (CFOSAT, launched in 2018) and proposed satellite projects including the European Sea surface KInematics Multiscale monitoring (SKIM) mission for waves and surface currents, as well as a United States concept for a Wind and Currents Mission (WaCM) offer the prospect of enhancing the range of information available from space. One challenge for satellite observations is that most Earth-observing satellites use sun-synchronous orbits, which means that they sample at the same local time (e.g., 6 am and 6 pm) everywhere, and thus multiple satellites with similar objectives but different local sampling times may be needed to capture diurnal variability or the scales of fast-moving synoptic storms.
Autonomous Surface Vehicles have been developed over the last decade to measure air-sea surface fields. Advanced ASVs can measure surface radiation, air pressure, upper-ocean currents, in addition to the standard surface variables. These are variables important to initialize coupled S2S prediction. Collocated ocean-atmosphere observations using ASV are essential for strongly coupled data assimilation (CDA) (Penny et al., 2019). The ASVs can be used to adaptively target special events (e.g., El Niño development) to supplement existing sources of ocean observations. As another example, ASVs are particularly appropriate for observing the diurnal and subsurface structure of marine heat waves. Hence, the advent of the ASVs to gather collocated ocean-atmosphere boundary layer observations, especially in locations where moored buoys are impractical (e.g., near seasonally migrating sea-ice edge) could be an irreplaceable source of information for both improving the models as well as for coupled assimilation for S2S prediction.
Autonomous underwater gliders (Rudnick, 2016; Testor et al., 2019) provide high-resolution, particularly within boundary current systems (Rudnick et al., 2017; Todd et al., 2019). Glider observations resolve the strong gradients that typify coastal and boundary current systems and have demonstrated impact on operational forecast models (e.g., Todd and Locke-Wynn, 2017). Increased utilization of gliders in boundary and coastal regions with strong gradients can help improve our ability to initialize S2S forecasts that are sensitive to upper-ocean gradients.
Some aspects of the MJO genesis and large-scale evolution are captured by the RAMA moored buoy array in the Indian Ocean and by the TAO array in the tropical Pacific, where MJO events interact with and affect ENSO evolution. However, these arrays do not resolve the gradients of temperature and salinity fields or upper ocean currents that potentially influence S2S predictions (Moum et al., 2016). Another example is the eastern edge of the western Pacific warm pool, where strong zonal gradients of temperature with a sharp salinity front at and near the surface migrate in longitude on the S2S timescales. In these two cases, sustained observation platforms complemented with ASVs are needed to cover the gradients in between fixed moorings. Observations in the atmospheric boundary layer are also required as the zonal migration of the salinity front and SST gradient are driven by intraseasonal fluxes of buoyancy, radiation, water vapor, and stress. The deliberate discussion that is taking place as part of TPOS 2020 on the future of the tropical Pacific observing system is essential for improving the skill of S2S predictions.
Western boundary current regions are areas of strong currents and hence are a challenge for Argo and drifting floats to make sustained observations. Moorings, though challenging to maintain in strong current regions, can still act as an important source of measurements of surface fluxes that are useful for initializing S2S forecasts as well as verifying the forecasts (Cronin et al., 2008; Weller et al., 2012; Bigorre et al., 2013). Gliders are able to deliver the data from these moorings via real-time acoustic telemetry to monitoring and assimilation networks (Send et al., 2013). These should ideally be complemented by more spatially integrated transport and surface flux measurements in these regions for forecast validation. Observational efforts should go hand in hand with modeling and data assimilation methods to help improve predictions in these regions.
Although assimilation of sea-ice concentration data into S2S prediction models has started in several operational centers, assimilation of sea-ice thickness is rarely performed due to the limitations of ice thickness data. Sea-ice thickness, while difficult to measure via remote sensing, is an important variable in sea-ice prediction (Blockley and Peterson, 2018). Water and air properties surrounding sea-ice are needed for sea-ice prediction. Currently, there are no routine observations taken near the edge of sea-ice nor in the transition marginal ice zone (MIZ) between sea ice and open water (Lee et al., 2017; Nguyen et al., 2017). Permanent moored subsurface buoys and ice-tethered profilers could be a key source of time-series data in these data sparse regions. However, mobile vehicles (ASVs, and subsurface gliders) are dynamic platforms that can monitor surface and subsurface conditions following the seasonal migration of sea ice. The value of data collected by these mobile vehicles in polar regions to S2S prediction needs to be explored.
Observing System Experiments to Evaluate Observation Networks and S2S Models
Ocean observing system experiments are conducted at ECMWF and other global forecasting centers to evaluate the use of ocean observations in ocean reanalyses (Balmaseda et al., 2007; Fujii et al., 2015; Xue et al., 2017). In these ocean reanalyses produced at ECMWF, specific observing systems are withdrawn in a controlled manner. We call these experiments OSE-ORAs (Observing System Experiments – Ocean ReAnalyses). Differences between OSE-ORAs give information on the possible impact of a given observation type on the mean and variability of the reanalyses. The impact of observations on forecasts could in principle be further quantified by using these OSE-ORAs to initialize coupled forecast, although this assessment may be hindered by low statistical significance and issues related to model biases. This is the approach followed in previous studies targeting seasonal time scales (Balmaseda and Anderson, 2009; Balmaseda et al., 2010; Fujii et al., 2011).
It is important to use a metric with significant level of skill and clearly influenced by the ocean state. Experiments have been conducted to quantify the impact of altimeter-derived sea level anomaly on sub-seasonal forecasts of tropical cyclone activity. The impact is measured by the differences between two sets of sub-seasonal reforecasts spanning the period 1994–2016, which differ only in the ocean initial conditions. The reference experiment uses the operational ocean reanalyses ORAS5 (Zuo et al., 2019), where all the ocean observing systems are used. The second experiment is initialized using a variant of ORAS5 where the altimeter sea level data are withdrawn from the assimilation, keeping everything else the same as in ORAS5. Figure 2 shows that by withdrawing the altimeter sea-level from the ocean initial conditions, the forecast of tropical cyclones is degraded in the 16–45-day time range. Correlations between observed and predicted Accumulated Cyclone Energy (ACE) during the monthly period are lower over the North Atlantic, the eastern North Pacific, the western north Pacific and the South Pacific in the experiment initialized with the assimilation without altimeter data than in the control experiment. The assessment of the adequacy of the current ocean observing system for the sub-seasonal range will be a focus activity of the WWRP/WCRP sub-seasonal to seasonal prediction project (S2S) in the next few years. Hence, the engagement of the ocean observations community with this WMO activity would be very beneficial for all involved.
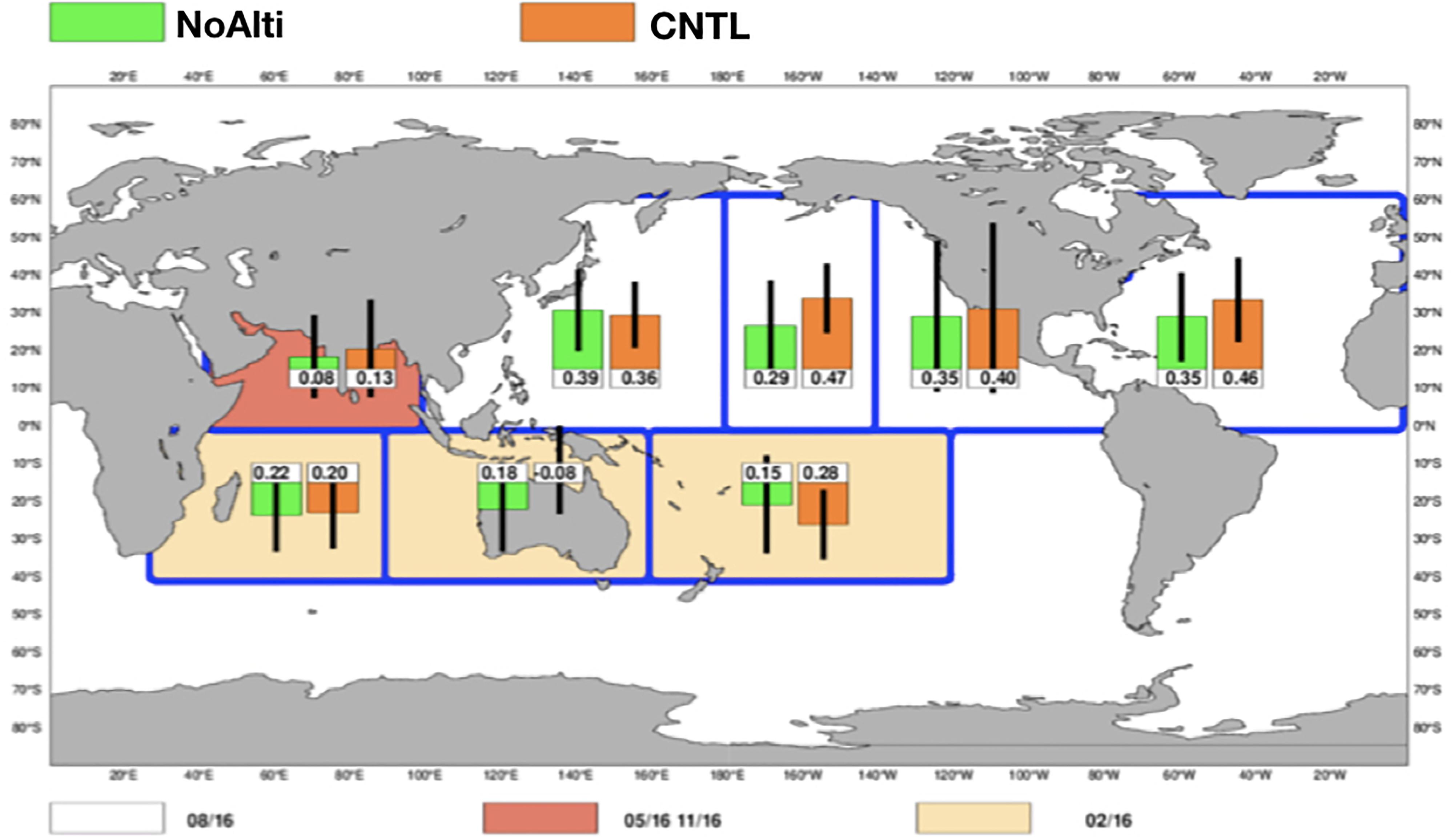
Figure 2. Anomaly correlation skill of forecast of tropical cyclone energy at days 16–45 days from two experiments with different ocean initialization: CNTL (orange) is initialized by ORAS5, which uses all the ocean observing system. In experiment NoAlti (green) the altimeter-derived sea-level has been removed from the ocean initial conditions.
Conclusion
Global S2S forecasting systems are evolving rapidly in centers around the world. Their success critically depends on the development of a CDA capability that demands adequate observations of the upper ocean and ocean-atmosphere interface.
The ocean is under-observed relative to the number of routine observations collected in the atmosphere over land. The subsurface ocean and many variables at the ocean surface are hard, if possible, to observe with satellite remote sensing technologies. The surface and upper-ocean observing systems relevant for understanding, modeling and forecasting on S2S timescales will continue to evolve with the improvement in technological capabilities. Current and future challenges in ocean observing system designs and implementations are complicated by the desire to balance resources for routine ocean observation systems (e.g., moorings, Argo, and satellites) with new and more sophisticated technologies (e.g., ASVs). Hence, the community must focus on designing and implementing cost-effective surface and subsurface ocean observations with a combination of various technologies that provide needed data for initialization and validation of S2S prediction systems as well as advancing understanding of ocean-atmosphere coupling on S2S timescales.
The community can advance the skill of S2S forecasts significantly by focusing next generation ocean observations on higher temporal and spatial resolution observations in the ocean-atmosphere boundary layers in key regions hosting known sources of S2S predictability (Tropics, coastal regions and polar regions) including the diurnal cycle of air-sea coupling and mixed-layer structure as highlighted by studies referenced in the above sections.
Breakthroughs in the accuracy of S2S predictions are finally within reach with the current generation coupled forecasting systems. New tools from CDA and advanced satellite and autonomous technology to observe the ocean-atmosphere boundary layers will make it possible to integrate global observations and local high-resolution simulations in S2S forecasting systems. Scientific, computational, and observation technology challenges need to be addressed to accomplish these required advances in the coming decade. While these challenges are substantial, the revolution in autonomous observing systems and computational breakthroughs focused on addressing high priority S2S prediction problems can help make key progress possible. This will not only benefit the scientific community but also society as a whole by providing climate information that can change the world of climate-data driven decision-making.
Author Contributions
AS led the writing of the manuscript. FV and MB contributed to the analysis and creation of Figure 2. CD and AS contributed to creation of Figure 1. DG contributed to the text of the manuscript. All authors contributed to the text of the manuscript.
Funding
AS was funded by NOAA Climate Variability and Prediction Program (NA14OAR4310276) and the NSF Earth System Modeling Program (OCE1419306). CD was funded by NA16OAR4310094. SG and DG were funded by NASA awards NNX14AO78G and 80NSSC19K0059. DY was supported by NSFC (91858204, 41720104008, and 41421005).
Conflict of Interest Statement
The authors declare that the research was conducted in the absence of any commercial or financial relationships that could be construed as a potential conflict of interest.
Acknowledgments
The authors would like to thank support by a grant from NOAA Climate Variability and Prediction Program (NA14OAR4310276) and the NSF Earth System Modeling Program (OCE1419306). PMEL contribution number 4888. CD was funded by NA16OAR4310094. SG and DG were funded by NASA awards NNX14AO78G and 80NSSC19K0059. DY was supported by NSFC (91858204, 41720104008, and 41421005).
Footnotes
References
Alexander, M. A., Deser, C., and Timlin, M. S. (1999). The reemergence of SST anomalies in the North Pacific Ocean. J. Clim. 12, 2419–2433. doi: 10.1175/1520-0442(1999)012<2419:trosai>2.0.co;2
Balmaseda, M., Alves, O., Awaji, T., Behringer, D., Ferry, N., Fujii, Y., et al. (2010). “Initialization for seasonal and decadal forecasts,” in OceanObs 09 (Paris: European Space Agency), 19–26.
Balmaseda, M., and Anderson, D. (2009). Impact of initialization strategies and observations on seasonal forecast skill. Geophys. Res. Lett. 36:L01701. doi: 10.1029/2008GL035561
Balmaseda, M., Anderson, D., and Vidard, A. (2007). Impact of Argo on analyses of the global ocean. Geophy. Res. Lett. 34:L16605.
Bernie, D. J., Guilyardi, E., Madec, G., Slingo, J. M., Woolnough, S. J., and Cole, J. (2008). Impact of resolving the diurnal cycle in an ocean–atmosphere GCM. Part 2: A diurnally coupled CGCM. Clim. Dyn. 31, 909–925. doi: 10.1007/s00382-008-0429-z
Bigorre, S. P., Weller, R. A., Edson, J. B., and Ware, J. D. (2013). A surface mooring for air–sea interaction research in the Gulf Stream. Part II: analysis of the observations and their accuracies. J. Atmos. Oceanic Technol. 30, 450–469. doi: 10.1175/jtech-d-12-00078.1
Blockley, E. W., and Peterson, K. A. (2018). improving met office seasonal predictions of Arctic sea ice using assimilation of CryoSat-2 thickness. Cryosphere 12, 3419–3438. doi: 10.5194/tc-12-3419-2018
Bond, N. A., Cronin, M. F., Freeland, H., and Mantua, N. J. (2015). Causes and impacts of the 2014 warm anomaly in the NE Pacific. Geophy. Res. Lett. 42, 3414–3420. doi: 10.1002/2015GL063306
Centurioni, L., Horányi, A., Cardinali, C., Charpentier, E., and Lumpkin, R. (2017). A global ocean observing system for measuring sea level atmospheric pressure: effects and impacts on numerical weather prediction. Bull. Am. Meteorol. Soc. 98, 231–238. doi: 10.1175/bams-d-15-00080.1
Centurioni, L. R. (2018). “Drifter technology and impacts for sea surface temperature, sea-level pressure, and ocean circulation studies,” in Observing the Oceans in Real Time, eds R. Venkatesan, A. Tandon, E. D’Asaro, and M. A. Atmanand (Cham: Springer International Publishing).
Cronin, M. F., Meinig, C., Sabine, C. L., Ichikawa, H., and Tomita, H. (2008). Surface mooring network in the Kuroshio Extension. IEEE Syst. J. 2, 424–430. doi: 10.1109/jsyst.2008.925982
Day, J. J., Hawkins, E., and Tietsche, S. (2014). Will Arctic sea ice thickness initialization improve seasonal forecast skill? Geophy. Res. Lett. 41, 7566–7575. doi: 10.1002/2014gl061694
DeMott, C. A., Klingaman, N. P., and Woolnough, S. J. (2015). Atmosphere-ocean coupled processes in the Madden-Julian oscillation. Rev. Geophys. 53, 1099–1154. doi: 10.1002/2014rg000478
Di Lorenzo, E., and Mantua, N. (2016). Multi-year persistence of the 2014/15 North Pacific marine heatwave. Nat. Clim. Change 11, 1042–1047. doi: 10.1038/nclimate3082
Foltz, G. R., Brandt, P., Richter, I., Rodríguez-Fonseca, B., Hernandez, F., Dengler, M., et al. (2019). The tropical Atlantic observing system. textitFront. Mar. Sci. 6:206. doi: 10.3389/fmars.2019.00206
Frölicher, T. L., and Laufkötter, C. (2018). Emerging risks from marine heat waves. Nat. Commu. 9:650. doi: 10.1038/s41467-018-03163-6
Frolov, S., Bishop, C. H., Holt, T., Cummings, J., and Kuhl, D. (2016). Facilitating strongly coupled ocean–atmosphere data assimilation with an interface solver. Mon. Wea. Rev. 144, 3–20. doi: 10.1175/MWR-D-15-0041.1
Fujii, Y., Cummings, J., Xue, Y., Schiller, A., Lee, T., and Balmaseda, M. A. (2015). Evaluation of the Tropical Pacific Observing System from the ocean data assimilation perspective. Quart. J. Roy. Meteor. Soc. 141, 2481–2496. doi: 10.1002/qj.2579
Fujii, Y., Kamachi, M., Nakaegawa, T., Yasuda, T., Yamanaka, G., Toyoda, T., et al. (2011). “Assimilating ocean observation data for ENSO monitoring and forecasting,” in Climate Variability - Some Aspects, Challenges and Prospects, ed. A. Hannachi (Rijeka: InTech Open), 75–98. doi: 10.5772/30330
Fujii, Y., Rémy, E., Zuo, H., Oke, P., Halliwell, G., Gasparin, F., et al. (2019). Observing system evaluationbased on ocean data assimilation and prediction systems: on-going challenges and a future vision for designing and supporting ocean observational networks. Front. Mar. Sci. 6:417. doi: 10.3389/fmars.2019.00417
Guemas, V., Blanchard-Wrigglesworth, E., Chevallier, M., Day, J. J., Déqué, M., Doblas-Reyes, F. J., et al. (2016). A review on Arctic sea-ice predictability and prediction on seasonal to decadal time-scales. Q. J. R. Meteorol. Soc. 142, 546–561. doi: 10.1002/qj.2401
Guemas, V., Doblas-Reyes, F. J., Mogensen, K., Keeley, S., and Tang, Y. (2014). Ensemble of sea ice initial conditions for interannual climate predictions. Clim. Dyn. 43, 2813–2829. doi: 10.1007/s00382-014-2095-7
Horányi, A., Cardinali, C., and Centurioni, L. (2017). The global numerical weather prediction impact of mean-sea-level pressure observations from drifting buoys. Q. J. R. Meteorol. Soc. 143, 974–985. doi: 10.1002/qj.2981
Jin, F. F. (1997). An equatorial ocean recharge paradigm for ENSO. Part I: conceptual model. J. Atmos. Sci. 54, 811–829. doi: 10.1175/1520-0469(1997)054<0811:aeorpf>2.0.co;2
Laloyaux, P., Balmaseda, M., Dee, D., Mogensen, K., and Janssen, P. (2016). A coupled data assimilation system for climate reanalysis. Q. J. Roy. Meteor. Soc. 142, 65–78. doi: 10.1002/qj.2629
Lea, D. J., Mirouze, I., Martin, M. J., King, R. R., Hines, A., Walters, D., et al. (2015). Assessing a new coupled data assimilation system based on the met office coupled atmosphere–land–ocean–sea ice model. Mon. Wea. Rev. 143, 4678–4694. doi: 10.1175/mwr-d-15-0174.1
Lee, C. M., Thomson, J., and The Marginal Ice Zone Team, and Arctic Sea State Teams (2017). An autonomous approach to observing the seasonal ice zone in the western Arctic. Oceanography 30, 56–68. doi: 10.5670/oceanog.2017.222
McKinnon, K. A., Rhines, A., Tingley, M. P., and Huybers, P. (2016). Long-lead predictions of eastern United States hot days from Pacific sea surface temperatures. Nat. Geosci. 9:389. doi: 10.1038/ngeo2687
Meinen, C. S., and McPhaden, M. J. (2000). Observations of warm water volume changes in the equatorial Pacific and their relationship to El Niño and La Niña. J. Clim. 13, 3551–3559. doi: 10.1175/1520-0442(2000)013<3551:oowwvc>2.0.co;2
Moum, J. N., Pujiana, K., Lien, R. C., and Smyth, W. D. (2016). Ocean feedback to pulses of the Madden–Julian oscillation in the equatorial Indian Ocean. Nat. Commun. 7:13203. doi: 10.1038/ncomms13203
Nguyen, A. T., Ocaña, V., Garg, V., Heimbach, P., Toole, J. M., Krishfield, R. A., et al. (2017). On the benefit of current and future ALPS data for improving Arctic coupled ocean-sea-ice state estimation. Oceanography 30, 69–73. doi: 10.5670/oceanog.2017.223
Penny, S. G., Akella, S., Balmaseda, M. A., Browne, P., Carton, J. A., Chevallier, M., et al. (2019). Observational needs for improving ocean and coupled reanalysis, S2S prediction, and decadal prediction. Front. Mar. Sci. 6:391. doi: 10.3389/fmars.2019.00391
Penny, S. G., and Hamill, T. M. (2017). Coupled data assimilation for integrated earth system analysis and prediction. Bull. Am. Meteorol. Soc. 97, ES169–ES172.
Roemmich, D., Alford, M. H., Claustre, H., Johnson, K. S., King, B., Moum, J., et al. (2019). On the future of Argo: a global, full-depth, multi-disciplinary array. Front. Mar. Sci. doi: 10.3389/fmars.2019.00439
Rudnick, D. L. (2016). Ocean research enabled by underwater gliders. Ann. Rev. Marine Sci. 8, 519–541. doi: 10.1146/annurev-marine-122414-033913
Rudnick, D. L., Zaba, K. D., Todd, R. E., and Davis, R. E. (2017). A climatology of the California current system from a network of underwater gliders. Progr. Oceanogr. 154, 64–106. doi: 10.1016/j.pocean.2017.03.002
Sahai, A. K., Chattopadhyay, R., Susmitha, J., Phani, M. K., Pattanaik, D. R., and Abhilash, S. (2019). “Chapter 20 - seamless prediction of monsoon onset and active/break phases,” in Sub-Seasonal to Seasonal Prediction, eds A. W. Robertson, F. Vitart, and A. Robertson (Amsterdam: Elsevier), 421–438.
Saravanan, R., and Chang, P. (2019). “Midlatitude mesoscale ocean-atmosphere interaction and its relevance to S2S prediction,” in Sub-Seasonal to Seasonal Prediction, eds A. W. Robertson and V. Frédéric (Amsterdam: Elsevier), 183–200. doi: 10.1016/b978-0-12-811714-9.00009-7
Send, U., Regier, L., and Jones, B. (2013). Use of underwater gliders for acoustic data retrieval from subsurface oceanographic instrumentation and bidirectional communication in the deep ocean. J. Atmos. Oceanic Technol. 30, 984–998. doi: 10.1175/JTECH-D-11-00169.1
Seo, H., Subramanian, A. C., Miller, A. J., and Cavanaugh, N. R. (2014). Coupled impacts of the diurnal cycle of sea surface temperature on the Madden–Julian oscillation. J. Clim. 27, 8422–8443. doi: 10.1175/jcli-d-14-00141.1
Smith, N., Kessler, W. S., Cravatte, S., Sprintall, J., Wijffels, S., Cronin, M. F., et al. (2019). Tropical Pacific observing system. Front. Mar. Sci. 6:31. doi: 10.3389/fmars.2019.00031
Subramanian, A. C., Vitart, F., Zhang, C., Kumar, A., and Balmaseda, M. A. (2017). Ocean observations for operational subseasonal and seasonal forecasts. (in press).
Testor, P., DeYoung, B., Rudnick, D. L., Glenn, S., Hayes, D., Lee, C., et al. (2019). OceanGliders: A component of the integrated GOOS. Front. Marine Sci. doi: 10.3389/fmars.2019.00422
Todd, R. E., Chavez, F. P., Clayton, S., Cravatte, S. E., Goes, M. P., Graco, M. I., et al. (2019). Global perspectives on observing ocean boundary current systems. Front. Marine Sci. doi: 10.3389/fmars.2019.00423
Todd, R. E., and Locke-Wynn, L. (2017). Underwater glider observations and the representation of western boundary currents in numerical models. Oceanography 30, 88–89. doi: 10.5670/oceanog.2017.225
Turner, J., Phillips, T., Marshall, G. J., Hosking, J. S., Pope, J. O., Bracegirdle, T. J., et al. (2017). Unprecedented springtime retreat of Antarctic sea-ice in 2016. Geophys. Res. Lett. 44, 6868–6875. doi: 10.1002/2017GL073656
Vimont, D. J., Battisti, D. S., and Hirst, A. C. (2001). Footprinting: a seasonal connection between the tropics and mid-latitudes. Geophys. Res. Lett. 28, 3923–3926. doi: 10.1029/2001gl013435
Vitart, F., Robertson, A. W., and Anderson, D. L. (2012). Subseasonal to Seasonal Prediction Project: bridging the gap between weather and climate. WMO Bull. 61, 23–28.
Weller, R. A., Bigorre, S. P., Lord, J., Ware, J. D., and Edson, J. B. (2012). A surface mooring for air–sea interaction research in the Gulf Stream. Part I: Mooring design and instrumentation. J. Atmos. Oceanic Technol. 29, 1363–1376. doi: 10.1175/jtech-d-12-00060.1
Woolnough, S. J., Vitart, F., and Balmaseda, M. A. (2007). The role of the ocean in the Madden–Julian Oscillation: implications for MJO prediction. Q. J. R. Meteorol. Soc. 133, 117–128. doi: 10.1002/qj.4
Xue, Y., Wen, C., Yang, X., Behringer, D., Kumar, A., Vecchi, G., et al. (2017). Evaluation of tropical Pacific observing systems using NCEP and GFDL ocean data assimilation systems. Clim. Dyn. 49, 843–868. doi: 10.1007/s00382-015-2743-6
Yeh, S.-W., Cai, W., Min, S.-K., McPhaden, M. J., Dommenget, D., and Dewitte, B. (2018). ENSO atmospheric teleconnections and their response to greenhouse gas forcing. Rev. Geophy. 56, 185–206. doi: 10.1002/2017RG000568
Zaba, K. D., and Rudnick, D. L. (2016). The 2014–2015 warming anomaly in the Southern California current system observed by underwater gliders. J. Geophy. Res.: Oceans 2016, 1241–1248. doi: 10.1002/2015gl067550
Zampieri, L., Goessling, H. F., and Jung, T. (2018). Bright prospects for arctic sea ice prediction on subseasonal time scales. Geophys. Res. Lett. 45, 9731–9738. doi: 10.1029/2018gl079394
Zhang, C., and Ling, J. (2017). Barrier effect of the Indo-Pacific maritime continent on the MJO: perspectives from tracking MJO precipitation. J. Clim. 30, 3439–3459. doi: 10.1175/jcli-d-16-0614.1
Keywords: subseasonal, seasonal, predictions, air–sea interaction, satellite, Argo, gliders, drifters
Citation: Subramanian AC, Balmaseda MA, Centurioni L, Chattopadhyay R, Cornuelle BD, DeMott C, Flatau M, Fujii Y, Giglio D, Gille ST, Hamill TM, Hendon H, Hoteit I, Kumar A, Lee J-H, Lucas AJ, Mahadevan A, Matsueda M, Nam S, Paturi S, Penny SG, Rydbeck A, Sun R, Takaya Y, Tandon A, Todd RE, Vitart F, Yuan D and Zhang C (2019) Ocean Observations to Improve Our Understanding, Modeling, and Forecasting of Subseasonal-to-Seasonal Variability. Front. Mar. Sci. 6:427. doi: 10.3389/fmars.2019.00427
Received: 01 November 2018; Accepted: 05 July 2019;
Published: 08 August 2019.
Edited by:
Amos Tiereyangn Kabo-Bah, University of Energy and Natural Resources, GhanaReviewed by:
Eric Hackert, National Aeronautics and Space Administration (NASA), United StatesRichard James Matear, Commonwealth Scientific and Industrial Research Organisation (CSIRO), Australia
Copyright © 2019 Subramanian, Balmaseda, Centurioni, Chattopadhyay, Cornuelle, DeMott, Flatau, Fujii, Giglio, Gille, Hamill, Hendon, Hoteit, Kumar, Lee, Lucas, Mahadevan, Matsueda, Nam, Paturi, Penny, Rydbeck, Sun, Takaya, Tandon, Todd, Vitart, Yuan and Zhang. This is an open-access article distributed under the terms of the Creative Commons Attribution License (CC BY). The use, distribution or reproduction in other forums is permitted, provided the original author(s) and the copyright owner(s) are credited and that the original publication in this journal is cited, in accordance with accepted academic practice. No use, distribution or reproduction is permitted which does not comply with these terms.
*Correspondence: Aneesh C. Subramanian, YW5lZXNoY3NAY29sb3JhZG8uZWR1; YWNzdWJyYW1AdWNzZC5lZHU=