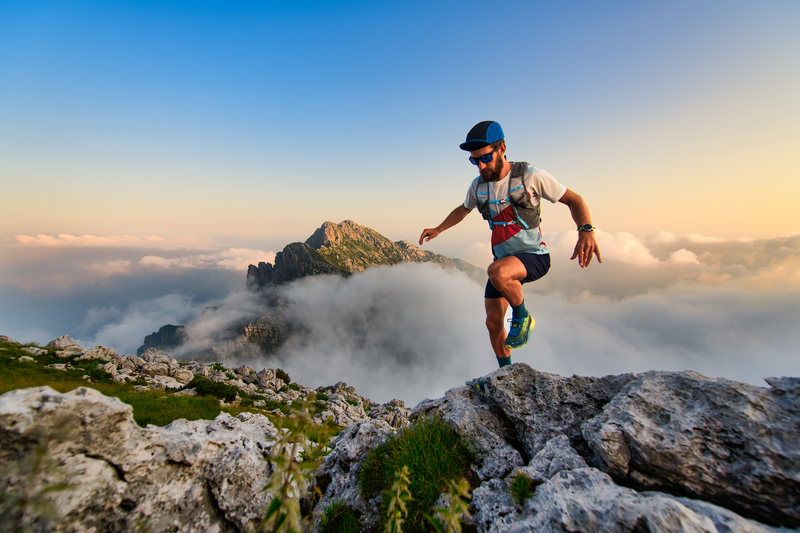
94% of researchers rate our articles as excellent or good
Learn more about the work of our research integrity team to safeguard the quality of each article we publish.
Find out more
ORIGINAL RESEARCH article
Front. Mar. Sci. , 11 July 2019
Sec. Deep-Sea Environments and Ecology
Volume 6 - 2019 | https://doi.org/10.3389/fmars.2019.00401
Vulnerable marine ecosystems (VMEs) are considered hotspots of biodiversity and ecosystem functioning in the deep sea, but are also characterised by a high vulnerability to disturbance and a low recovery potential. Since 2006, a series of United Nations General Assembly (UNGA) resolutions have been developed, attempting to ensure the protection of VMEs in international waters. In the Northwest Atlantic Fisheries Organisation (NAFO) Regulatory Area, large areas of seabed have been closed to bottom-contact fishing to protect VMEs. However, knowledge of the influence of VME-indicator taxa on macrofaunal assemblages, and the appropriateness of current fishery closures for protecting macrofaunal biodiversity in this area is limited. Here we investigate relationships between the prevalence of VME-indicator taxa [poriferans (sponges), gorgonian corals, and pennatulaceans (sea pens)] and an extensive suite of peracarid crustacean biodiversity metrics in the NAFO Regulatory Area. We also examine whether the current NAFO VME closures protect areas of significantly elevated peracarid diversity. Of the VME-indicator taxa analysed, poriferans were found to have by far the greatest influence over peracarid assemblages. Assemblage structure was altered, and peracarid abundance, biomass, richness, diversity, and variability were enhanced in areas of elevated poriferan biomass, whilst assemblage evenness was slightly depressed in these areas. These findings reaffirm the perception of poriferans as crucial components of VMEs. In contrast, gorgonian coral density had little influence over the faunal assemblages investigated, perhaps reflecting their relatively low prevalence in the study area. Similarly, pennatulaceans were found to influence peracarid assemblages only weakly. This too may reflect a moderately low density of Pennatulacea in the study area. Our results highlight that the application of taxon distribution model outputs to ecological investigations and management decisions in data-limited environments should be treated with caution. Finally, our results indicate that the current system of fishery closures in the NAFO Regulatory Area may not be optimal in terms of providing adequate protection to VMEs against the impacts of bottom trawling.
The concept of a “Vulnerable Marine Ecosystem” (VME) centres upon the presence of distinct, diverse benthic assemblages that are limited and fragmented in their spatial extent, and dominated (in terms of biomass and/or spatial cover) by rare, endangered or endemic component species that are physically fragile and vulnerable to damage (or structural/biological alteration) by human activities (Fuller et al., 2008; Rogers et al., 2008; FAO, 2009; Parker et al., 2009; Rogers and Gianni, 2009; Auster et al., 2011; Hansen et al., 2013). Because the component species of VMEs typically exhibit traits of slow growth, late maturity, low fecundity, unpredictable recruitment and high longevity, VMEs are characterised by slow recovery from damage (Fuller et al., 2008; Rogers et al., 2008; FAO, 2009; Parker et al., 2009; Hogg et al., 2010; Auster et al., 2011; Hansen et al., 2013; Clark et al., 2016). Taxa which are considered to be indicative of the presence of a VME include a myriad of deep-sea corals, hydrozoans, cerianthids, poriferans, ophiuroids, stalked crinoids, ascidians, bryozoans, brachiopods, xenophyophores, and deep-water serpulidae and oyster reefs, and chemosynthetic species (Fuller et al., 2008; Parker et al., 2009; Rogers and Gianni, 2009; Gianni et al., 2011; Murillo et al., 2012).
The overarching concept of a VME, and efforts to protect deep-sea VME-indicator taxa [e.g., by spatial closures to destructive fishing practises (Koslow and Gowlet-Holmes, 1998)] have been important aspects of deep-sea ecology and fishery management for at least 20 years. However, formalisation of the term only occurred in the mid-2000s following international efforts to reduce the destruction of VMEs attributable to bottom trawling. Starting in 2004, a series of United Nations General Assembly (UNGA) resolutions of increasing strength and urgency were adopted: UNGA 59/25 (2004), UNGA 61/105 (2006), UNGA 64/72 (2009), UNGA 66/68 (2011), and UNGA 71/123 (2016). These resolutions called for states and Regional Fisheries Management Organisations (RFMOs) to formally recognise the immense importance and value of deep-sea ecosystems and the biodiversity that they contain by adhering to the precautionary principle and ecosystem approach when harvesting biological products from the deep sea. Specifically, these resolutions have called for immediate action to reduce the frequency of Significant Adverse Impacts (SAIs) on VMEs. A SAI is defined as any disturbance to a VME that impairs the ability of affected VME constituent populations to replace themselves, degrades the long-term productivity of the habitat, and causes a significant loss of species richness, habitat diversity and structure whilst occurring at an interval that is, on average, shorter than the recovery time for the habitat in question (Hogg et al., 2010).
In order to achieve the goals of the UNGA resolutions, a number of management tasks have been proposed (FAO, 2009), including the production of thorough impact assessments, the development of “move-on” rules, the spatial closure of all known VME locations to bottom-contact fishing activities, and directed efforts to map VME locations (including the continued monitoring of distributions pending potential change associated with oceanographic variables), this all being bound by appropriate control and surveillance mechanisms (Rogers et al., 2008).
The Northwest Atlantic Fisheries Organisation (NAFO), which is the focal RFMO of this study, has responded to the UNGA resolutions primarily by the mapping of VME locations, the establishment of 21 fishery closures covering a total sea-surface area of over 390,000 km2, and the development of a move-on protocol (Gianni et al., 2016; NAFO, 2017). However, the optimality of the NAFO move-on protocol and fishery closures around known VMEs have been questioned (Gianni et al., 2011, 2016; Wright et al., 2015).
VMEs are considered hotspots of biodiversity and ecosystem functioning in the deep sea, with elevated faunal abundance, biomass, richness, and diversity in VMEs compared to surrounding habitats (Bett and Rice, 1992; Edinger et al., 2007; van Oevelen et al., 2009; Hogg et al., 2010; Barrio Froján et al., 2012; Beazley et al., 2013, 2015). This can largely be attributed to the presence of structure-forming taxa in VMEs, which greatly elevates the physical heterogeneity of VME habitat relative to surrounding soft sediments, providing an enhanced number and diversity of microhabitats to associated metazoans and microbes (Klitgaard, 1995; Thrush et al., 1998; Buhl-Mortensen and Mortensen, 2005; Tissot et al., 2006; Wulff, 2006; Fuller et al., 2008; Rogers et al., 2008; Buhl-Mortensen et al., 2010; Gerovasileiou et al., 2016). The skeletal remains of hexactinellid poriferans represent an important deep-sea habitat, acting to stabilise seafloor sediments and inhibit colonisation by some infaunal taxa. This provides a unique substratum for colonisation by other benthic fauna (Bett and Rice, 1992; Beazley et al., 2013), and the abundance and biomass of macrofaunal taxa may be elevated in proximity to spicule mats (Bett and Rice, 1992). Similarly, scleractinian coral rubble and dead coral skeletons have been shown to host a fauna that is relatively distinct from surrounding sediments (Raes and Vanreusel, 2005; Gheerardyn et al., 2009).
The heterogeneous and complex habitat of VMEs may also provide shelter from predation and adverse hydrological conditions for a multitude of taxa, including commercially important species (Barthel, 1992; Husebø et al., 2002; Krieger and Wing, 2002; Henkel and Pawlik, 2005; Tissot et al., 2006; Amsler et al., 2009), and VMEs may be used as aggregation and spawning sites for fish and other taxa (Freese and Wing, 2003; Auster, 2005; Etnoyer and Warrenchuk, 2007; Baillon et al., 2012; Pham et al., 2015). Further, VME-indicator taxa can act as a direct food source to associated fauna (Krieger and Wing, 2002; Wulff, 2006; Buhl-Mortensen et al., 2010), and may also provide increased access to alternative food sources for some species. For example, VMEs are important forage areas for fish and other predators because of the high abundance and biomass of associated fauna (Husebø et al., 2002; Hogg et al., 2010; Auster et al., 2011), whilst the elevated feeding platforms and increased turbulence created by VME frameworks, affords a raised supply of suspended particles to suspension feeders (Krieger and Wing, 2002; Buhl-Mortensen and Mortensen, 2005; Wulff, 2006; van Oevelen et al., 2009; Buhl-Mortensen et al., 2010). Species living within poriferans may even feed directly on the suspended particles pumped into the poriferan by the action of the choanoderm tissue (Buhl-Mortensen et al., 2010).
Linked to their elevated biodiversity and levels of ecosystem functioning, VMEs are growing in recognition as providers of important ecosystem services. Poriferans and cold-water corals, for example, have been shown to provide a strong link between pelagic and benthic foodwebs (Hentschel et al., 2002; Pile and Young, 2006; van Oevelen et al., 2009; Cathalot et al., 2015), and numerous VME-indicator taxa have been associated with the provision of novel biomolecules (Wright et al., 2004; Reddy et al., 2005; Skropeta, 2008; Jobstvogt et al., 2014). The wider biotechnological potential of poriferans in providing new designs for fibre optics, glass, civil engineering and even semiconductors is also starting to be recognised (Hogg et al., 2010).
In the NAFO Regulatory Area, a large number of VME-indicator taxa have been identified around the Flemish Cap, Flemish Pass and slopes of the Grand Banks [which together form the study area of this investigation, and will herein be referred to as the “study area”; please see Ashford et al. (2018), Figure 1 for a map which places the study area in a global context]. Porifera appear to dominate in terms of abundance and biomass, particularly at upper slope depths (Knudby et al., 2013a), where they can account for up to 90% of invertebrate biomass (Fuller et al., 2008; Murillo et al., 2012). At least 30 poriferan species have been identified, whilst a number of coral taxa (such as zoanthids, antipatharians, gorgonians, pennatulaceans, and scleractinians), hydrozoans, cerianthids, brachiopods, cirripedians, ascidians, stalked crinoids, ophiuroids, holothurians, cidaroids, upright bryozoans such as phidoloporids, and xenophyophores are also found in this region (Fuller et al., 2008; Barrio Froján et al., 2012; Kenchington et al., 2014).
Figure 1. Positions of Northwest Atlantic Fisheries Organisation Vulnerable Marine Ecosystem closures around the Flemish Cap, Flemish Pass and slopes of the Grand Banks (yellow polygons). Locations correct as of December 2016. Contours in metres. SRTM30 bathymetric data used (lighter, shallower; darker, deeper).
Both mapping and modelling approaches have been employed in order to determine the distribution of porifera (Fuller et al., 2008; Murillo et al., 2012; Knudby et al., 2013a; Kenchington et al., 2014) and deep-water corals (Murillo et al., 2010, 2011; Knudby et al., 2013b; Kenchington et al., 2014) in the study area of this investigation. Mapping attempts have been based on data from Spanish and EU groundfish surveys, and attempts to model poriferan and coral distributions in this area (Knudby et al., 2013a,b; Kenchington et al., 2014) suggest their distributions to be more extensive than those reported by Murillo et al. (2011, 2012).
Few studies have investigated the influence of VME-indicator taxa aggregations on surrounding benthic assemblages in the NAFO Regulatory Area. The presence of VME-indicator taxa on the Sackville Spur region of the NAFO Regulatory Area has been shown to be associated with a higher biomass and distinct structure of macrofaunal assemblages (Barrio Froján et al., 2012), whilst higher poriferan abundance has been shown to promote a higher abundance and diversity, and altered assemblage composition, of other megafaunal taxa relative to non-poriferan-dominated habitat (Beazley et al., 2013, 2015).
This study aims to investigate the influence of poriferan grounds, gorgonian forests and pennatulacean fields on macrobenthic peracarid crustacean assemblages, as well as to determine whether or not the current fishery closures enforced by NAFO protect areas of significantly enhanced peracarid biodiversity compared to other areas in the study region. Poriferan, gorgonian and pennatulacean density are estimated both following the approach of Kenchington et al. (2014) and by random forest modelling. Several facets of peracarid assemblages are considered (Table 1), including taxonomic, phylogenetic and functional diversity metrics, and it is hypothesised that these metrics will respond positively to increases in biomass of the investigated VME-indicator taxa.
Peracarid assemblages were sampled by means of 312 box cores (USNEL type, area 0.25 m2) collected from the Flemish Pass, the slopes of the Flemish Cap and the continental slopes of the Grand Banks (Northwest Atlantic Ocean) over spring and summer in 2009 and 2010 as part of the international “” (NEREIDA) programme [see Ashford et al. (2018) for further details]. In total, 20,245 individual peracarids (14,092 amphipods, 2371 isopods, 1985 tanaids, 1774 cumaceans, and 23 mysids) were identified to 177 genera and 74 families (Supplementary Table S2). Multiple studies have demonstrated that higher taxa, particularly genera, can provide an effective proxy for species diversity (Balmford et al., 1996a,b, Balmford et al., 2000; Roy et al., 1996; La Ferla et al., 2002; Villaseñor et al., 2005; Jablonski and Finarelli, 2009).
Peracarid abundance was enumerated as the total number of specimens per sample, and peracarid wet biomass per sample was measured to an accuracy of 0.0001 g. Taxon richness was calculated as the total number of genera per sample, and values of Rao Quadratic Entropy and Shannon Diversity were calculated for each sample using the packages “SYNCSA 1.3.2” (Debastiani, 2015) and “vegan 2.0-9” (Oksanen et al., 2013), respectively, in R 3.0.2 (R Core Team, 2013). Taxon evenness was calculated in R 3.0.2 using Pielou’s Index (Table 1 and Supplementary Table S3).
Phylogenetic diversity metrics [Phylogenetic Variability (PV), Phylogenetic Richness (PR), and Phylogenetic Evenness (PE)] were calculated for each sample following Helmus et al. (2007) using the R package “picante 1.6-2” (Kembel et al., 2014) based on the supertree of Peracarida presented by Ashford et al. (2018) and genus-level assemblage composition data for each sample (Supplementary Table S2). For a full mathematical exploration of these metrics, please see Helmus et al. (2007). Functional diversity metrics [Functional Variability (FV), Functional Richness (FR), and Functional Evenness (FE)] were calculated for each sample based on the functional dendrogram of Peracarida presented by Ashford et al. (2018) and family level composition data for each sample (Supplementary Table S2). Because functional dendrograms and phylogenetic trees are analogous in form, we calculated the values of functional diversity metrics in an identical manner to their phylogenetic equivalents in order to promote consistency in methodology (Table 1 and Supplementary Table S3).
Samples were categorised into being collected from within an area currently closed/open to bottom fishing based on the published locations of closures provided by NAFO1. Visualisation was aided using ESRI ArcGIS 10.1 (Figure 1).
During the identification of specimens, the presence/absence of siliceous poriferan spicules in each sample was recorded (see Figure 2A).
Figure 2. (A) Presence (green)/absence (red) of siliceous poriferan spicules in samples collected as part of the NEREIDA programme. (B) Relative biomass of Porifera across the study area (high, red; low, blue), as determined by random forest regression modelling. (C) Relative biomass of gorgonian corals across the study area (high, red; low, blue), as determined by random forest regression modelling. (D) Relative biomass of Pennatulacea across the study area (high, red; low, blue), as determined by random forest regression modelling. Contours in metres. SRTM30 bathymetric data used (lighter, shallower; darker, deeper).
Poriferan, gorgonian and pennatulacean relative biomass was estimated across the study area via kernel density surface modelling of bycatch data obtained from annual fishery survey trawls conducted by Canada and Spain between 1995 and 2013 (Porifera) and between 2005 and 2013 (Pennatulacea and gorgonian corals) (raw data provided by Dr Ellen Kenchington, Bedford Institute of Oceanography). Consistent identification of poriferans, pennatulaceans and gorgonian corals to species was not achieved during these fishery surveys (Kenchington et al., 2014). However, dominant poriferan families sampled included Geodiidae Gray 1867 and Ancorinidae Schmidt, 1870 (Murillo et al., 2012), pennatulacean species sampled included Anthoptilum grandiflorum (Verrill, 1879), Halipteris finmarchica (Sars, 1851) and Pennatula aculeata Danielssen, 1860 (Murillo et al., 2010; Kenchington et al., 2011), and gorgonian corals sampled included Acanthogorgia Gray, 1857, Keratoisis Wright, 1869, Paragorgia arborea (Linnaeus, 1758), Paragorgia johnsoni Grey, 1862, Paramuricea Kölliker, 1865, and Primnoa resedaeformis (Gunnerus, 1763) (Murillo et al., 2011). Identical methodology to Kenchington et al. (2014) was followed. Kernel density estimation was undertaken using the Kernel Density tool in the Spatial Analyst extension of ESRI ArcGIS 10.1. Poriferan, gorgonian and pennatulacean density at sampling locations was extracted using the Extract Values to Points tool in the Spatial Analyst extension, with local interpolation option selected. Please see Kenchington et al. (2014) for relevant figures.
Point biomass estimates (trawl start positions) for Porifera, gorgonian corals and Pennatulacea were obtained from annual fishery survey trawls conducted by Canada and Spain between 2011 and 2013. Random forest regression models were built for each of the VME-indicator taxa investigated with biomass as the response variable (square root-transformed), and a selection of physical environmental factors as explanatory variables. Following refinement of explanatory variables until all pairwise correlations were less than 0.5 (Pearson correlation coefficient), random forest models were produced in R 3.0.2 (R Core Team, 2013) using the package “randomForest 4.6-12” (Liaw et al., 2015) under default settings and 1000 trees. Predictor variables for each model were selected by application of an iterative permutation procedure using the boruta function in the R package “Boruta 5.1.0” (Kursa and Rudnicki, 2010). The following variables were retained in each model; for Porifera: sediment percent clay/silt, seafloor mean current speed, seafloor temperature variability, seafloor rugosity (225 × 225 m analysis cell size), and bottom trawling intensity; for gorgonian corals: depth below sea level, eastness, northness, sediment percent organic carbon, seafloor rugosity (225 × 225 m analysis cell size) and bottom trawl intensity; for Pennatulacea: bathymetric position index [150 cell (11250 m) outer radius, 1 cell (75 m) inner radius], sediment percent clay, eastness, seafloor minimum temperature, sediment percent organic carbon, and bottom trawling intensity. Please see Ashford et al. (2018) for additional details and methods relating to these environmental factors.
Models were validated based on a training (80% of data) /test (20% of data) routine, using R2 and Normalised Root Mean Squared Error (NRMSE) values. These were calculated using the package “caret 6.0-71” (Kuhn, 2016) in R 3.0.2 (R Core Team, 2013). The models for Porifera and Pennatulacea performed well, with R2 scores of 0.53 and 0.38, respectively, and both achieving mean NRMSE values of 0.10. The model for gorgonian corals performed less well, with an R2 value of 0.05 and mean NRMSE value of 0.08, likely reflecting a high degree of zero inflation in the dataset. The resulting models represent predictions of relative poriferan, gorgonian and pennatulacean biomass in the study area, taking into account the influence of bottom trawling intensity, as well as other environmental variables (see Figures 2B–D). Modelled poriferan, gorgonian and pennatulacean density at sampling locations was extracted using the Extract Values to Points tool in the Spatial Analyst extension of ESRI ArcGIS 10.1, with local interpolation option selected.
High multicollinearity amongst explanatory variables can have severe effects on the estimation of model parameters (Gunst and Webster, 1975). To investigate multicollinearity in the data set, Variance Inflation Factors (VIFs) were calculated for all continuous environmental variables using the package “HH 3.1-32” (Heiberger, 2016) in R 3.0.2 (R Core Team, 2013). No variables were found to have a VIF value greater than 5 - an accepted cut-off (Stine, 1995). A list of all environmental variables analysed is given in Table 2, and data is provided in Supplementary Table S3.
Table 2. Details of the environmental variables retained for analysis following Variance Inflation Factor variable selection.
Because initial data exploration revealed that multiple relationships between dependent and independent variables appeared to be non-linear, Generalised Additive Models (GAMs) were constructed in R 3.0.2 (R Core Team, 2013) using the package “mgcv 1.7-26” (Wood, 2013) for each of the 12 univariate metrics of peracarid assemblage composition (Table 1). Prior to analysis, all VME density estimates obtained based on the methodology of Kenchington et al. (2014) were square root-transformed to reduce high skew in the data. Original measurement scales were retained for all other variables since data distributions were deemed acceptable. Samples with zero or only one peracarid genus present were removed from the dataset.
Initial GAMs consisted of all variables contained within Table 2 as explanatory terms, with smoothers added to all continuous variables. Model performance diagnostics and the Akaike Information Criterion (AIC) were used to select the most appropriate error distributions and link functions (Akaike, 1974; see Supplementary Table S1), and acceptable satisfaction of model assumptions was investigated using the gam.cheque function of the package “mgcv 1.7-26” (Wood, 2013). Where the biological metric was categorical in form (abundance and taxon richness), over-dispersion was checked for by calculation of the dispersion parameter, theta (where theta = residual deviance/residual degrees of freedom), and corrected for as appropriate (see Supplementary Table S1). A penalised thin-plate regression spline was used as the smoothing function, and smoothing parameters were optimised automatically on the basis of the Generalised Cross Validation criterion (Wood, 2013). Moran’s I (Moran, 1950) was used to examine model residuals for the signature of spatial autocorrelation in R 3.0.2 (R Core Team, 2013) using the package “ape 3.2” (Paradis et al., 2004). In no cases could the null hypothesis (that no spatial autocorrelation is present within model residuals) be rejected and hence no further action was taken. The explanatory terms included in each GAM were refined from the full model via backward stepwise selection by consideration of independent variable P-values and model AIC until a minimum AIC value was reached.
Relation of peracarid assemblage structure (identities and relative abundances of genera in each sample) to the presence of VMEs and selection of the most relevant explanatory variables was achieved via Constrained Analysis of Principal coordinates (CAP) (Anderson and Willis, 2003) based on Bray-Curtis dissimilarity (Bray and Curtis, 1957). The model was refined backward based on P-values using the function ordistep (R package: “vegan 2.0-9” (Oksanen et al., 2013); 10000 permutations, P-in = 0.05, P-out = 0.1).
Poriferan density was found to significantly influence all peracarid assemblage metrics considered. The presence of hexactinellid spicules in samples (81% of samples) was found to positively influence peracarid abundance (P < 0.001), biomass (P < 0.001), taxon richness (P < 0.001), functional richness (P < 0.001) (Figure 3A), phylogenetic richness (P < 0.001), Rao Quadratic Entropy (P = 0.015), Shannon diversity (P < 0.001), and functional variability (P = 0.009) (Figure 3B), but negatively influence Pielou’s Index (P = 0.047). The presence/absence of poriferan spicules was also found to significantly alter peracarid assemblage structure (P < 0.001).
Figure 3. Partial relationship between VME presence at sampling locations (n = 312) in the NW Atlantic Ocean, and metrics of peracarid crustacean biodiversity. Solid lines are smoothed lines of best fit as determined by Generalised Additive Modelling. Surrounding bands are 95% confidence intervals. (A) siliceous poriferan spicule presence (blue)/“Functional Richness”; (B) siliceous poriferan spicule presence (blue)/“Functional Variability”; (C) relative biomass of Porifera (random forest model) (blue)/“Functional Variability”; (D) relative biomass of Porifera (random forest model) (blue)/“Phylogenetic Variability”; (E) relative biomass of Porifera (kernel density estimation, √ transformed) (blue)/Shannon Index; (F) relative biomass of Porifera (kernel density estimation, √transformed) (blue)/“Phylogenetic Richness”; (G) relative biomass of Pennatulacea (random forest model) (red)/biomass; (H) relative biomass of Pennatulacea (kernel density estimation, √transformed) (red)/taxon richness; (I) relative biomass of Pennatulacea (kernel density estimation, √transformed) (red)/“Functional Evenness”; (J) sample collected from area subject to NAFO VME closure (yellow)/“Functional Evenness”; (K) sample collected from area subject to NAFO VME closure (yellow)/Pielou’s Index.
In contrast, random forest-modelled poriferan biomass was found to relate negatively with peracarid abundance (P < 0.001), biomass (P < 0.001), taxon richness (P < 0.001), functional richness (P < 0.001), phylogenetic richness (P < 0.001), and possibly Rao Quadratic Entropy (P = 0.057). However, this metric of poriferan biomass was found to positively influence peracarid taxon evenness (P < 0.001), functional evenness (P = 0.004), phylogenetic evenness (P = 0.007), functional variability (P = 0.003) (Figure 3C), and phylogenetic variability (P = 0.001) (Figure 3D). Random forest-modelled poriferan biomass was also found to significantly influence peracarid assemblage structure (P < 0.001).
Poriferan biomass at sampling locations estimated by the kernel density approach of Kenchington et al. (2014) was found to correlate positively with peracarid abundance (P < 0.001), biomass (P < 0.001), taxon richness (P < 0.001), functional richness (P < 0.001), phylogenetic richness (P < 0.001) (Figure 3E), Shannon diversity (P < 0.001) (Figure 3F), and Rao Quadratic Entropy (P = 0.026). Increasing biomass of Porifera was not found to influence phylogenetic or functional variability, but Pielou’s Index (P < 0.001), functional evenness (P = 0.008) and phylogenetic evenness (P = 0.031) were all found to vary, similarly with this metric; this relationship being double-peaked, with assemblage evenness being maximised when relative Porifera biomass is between 0.4 and 2.0, and 14.4 and 17.6. Further, kernel density-estimated poriferan biomass was found to significantly influence the structure of peracarid assemblages (P < 0.001), with the abundance of the amphipod genera Gammaropsis Lilljeborg, 1855, Ampelisca Krøyer, 1842, and, in particular, Haploops Liljeborg, 1856 being especially enhanced in regions of high poriferan biomass.
Based on both the random forest and kernel density approaches to biomass estimation, gorgonian biomass was found to have only a weak influence over peracarid assemblages. Kernel density-estimated gorgonian biomass was not found to influence any of the faunal metrics considered in this analysis, and random forest-modelled biomass was found to relate only to phylogenetic variability (P = 0.008), this relationship being complex and negative overall. However, random forest-modelled gorgonian biomass was found to significantly influence peracarid assemblage structure (P < 0.001).
Pennatulacean biomass was found to relate to peracarid assemblages only weakly. Random forest-modelled pennatulacean biomass was found to have a possible (P = 0.055) effect on peracarid biomass (this relationship taking a complex, undulating form, with no overall directionality; see Figure 3G), and a significant influence on assemblage structure (P = 0.001).
Pennatulacean biomass at sampling locations as estimated by the kernel density approach of Kenchington et al. (2014) was found to relate significantly to a greater number of peracarid assemblage attributes, but these relationships were found to be generally weak and complex in form. Pennatulacean biomass was found to relate negatively with peracarid abundance (P < 0.001) and functional evenness (P = 0.029) (Figure 3H), whilst taxon richness (P = 0.004) (Figure 3I), functional richness (P = 0.009) and phylogenetic richness (P = 0.004) were found to vary in a complex, but overall negative manner with increasing pennatulacean biomass. Based on the results of the constrained ordination, the biomass of pennatulaceans at sampling locations (as estimated by the kernel density approach) was found to significantly influence peracarid assemblage structure (P < 0.001).
Whether or not samples were collected from an area currently closed to bottom trawling by NAFO was found to have only limited influence over the faunal metrics investigated. Peracarid abundance was found to be slightly depressed in cores taken from fishery closures compared to those from regions open to bottom trawling (P = 0.037), whilst Pielou’s Index (P = 0.023) and functional evenness (P = 0.049) were found to be slightly elevated in samples taken from closed areas relative to those from areas where bottom trawling is permitted (Figures 3J,K). Whether or not a sample was taken from an area currently closed to bottom trawling was not found to influence any other of the faunal metrics considered.
The results of this study strongly suggest that, of the three VME-indicator taxa investigated, poriferans have the greatest influence over peracarid assemblages in the NAFO Regulatory Area (Figures 3, 4 and Table 3). Our finding of a positive relationship between hexactinellid spicule presence and peracarid assemblage biodiversity metrics is consistent with our hypothesis, as well as with the conclusions of past studies. Bett and Rice (1992) found macrofaunal abundance and biomass to be elevated in areas of dense hexactinellid spicule mats relative to surrounding sediments, and attributed this to a local enhancement of organic matter and reduction in predation pressure for spicule mat associated macrofauna. Barrio Froján et al. (2012) also presented evidence for altered assemblage structure and enhanced abundance, biomass and taxon richness of benthic macrofauna in the vicinity of dense hexactinellid spicule mats. Further to these investigations, our study documents a positive influence of spicule presence on taxonomic metrics of diversity, phylogenetic and functional metrics of richness, and functional variability (Figure 4 and Table 3), extending knowledge of the influence of hexactinellid spicule mats on deep-sea macrofaunal assemblages.
Figure 4. Summary diagram detailing the influence of VME-indicator taxa on the benthic peracarid fauna of the NAFO Regulatory Area, and whether fishery closures host distinct peracarid assemblages or not. Links between environmental parameters (orange boxes) and biological parameters (purple boxes) are specified. The colour of the arrow represents the form of relationship: blue, positive; red, negative; black, complex (e.g., “U” shaped or uni/multi-modal). Solid arrows represent significant relationships (P < 0.05). Dotted arrows represent marginal relationships (P < 0.1).
Considering poriferan biomass as determined by Kenchington et al. (2014), our findings are consistent with those of previous investigations that highlighted an increased abundance, richness and diversity, and an altered structure of benthic fauna in proximity to poriferan aggregations (Pearse, 1932; Klitgaard, 1995; Beazley et al., 2013, 2015). Such results are most frequently attributed to the beneficial influence of poriferan biomass on three-dimensional habitat space and heterogeneity, reduced predation pressure, and increased feeding opportunities (Cinar et al., 2002; Wulff, 2006; Buhl-Mortensen et al., 2010; Hogg et al., 2010; Beazley et al., 2013). Overall, if only poriferan presence as determined by the presence of hexactinellid spicules and the kernel density biomass estimates of Kenchington et al. (2014) are considered, the results of this study reaffirm the perception of poriferans as important ecosystem engineers in the deep sea.
The contrast in results obtained between hexactinellid spicule data and the poriferan biomass data of Kenchington et al. (2014) on the one hand (both closely linked to ground-truthed observations), and those obtained from the random forest modelling approach on the other (which involves a much larger degree of extrapolation), is conspicuous (Figure 4 and Table 3). If used appropriately and in full awareness of their limitations, Species Distribution Models (SDMs) can provide valuable preliminary information regarding the distribution of species across the deep seafloor (Rengstorf et al., 2013). However, our results indicate that SDMs should not be relied upon in isolation for management and ecological purposes in the NAFO Regulatory Area.
Commonly, poor performance in SDMs leads to the overfitting of model parameters, with resulting predictions being overly sensitive to environmental differences between cells. Poor model performance may be caused by insufficiency and/or bias in the number and spatial spread of biological records, inappropriate weighting of particular biological records, and an inadequacy of available environmental data to fully reflect a taxon’s fundamental niche (Feeley and Silman, 2011).
For the random forest model of Porifera presented here, the following environmental variables were incorporated: sediment percent clay and silt content, average seafloor current speed, variability in annual seafloor temperature, seafloor rugosity, and bottom trawling intensity. However, some environmental variables typically recognised as being important in determining poriferan biomass across space were not incorporated into the model because of data deficiency, including seafloor oxygen concentration (Buhl-Mortensen et al., 2010) and concentration of suspended organic matter (Knudby et al., 2013a). Further, it should be noted that the SDM utilised did not incorporate details of biotic interactions, dispersal and population connectivity, or any biogeographic constraints that may apply to the taxon being modelled. Inclusion of such information may help to improve model performance by reducing instances of false positives of taxon presence in areas which are inaccessible (Boulangeat et al., 2012).
Whilst SDM methods appear to perform consistently well in cases of discrete, geographically limited focal areas that are well sampled, they often struggle where data is limited and/or spatially or temporally biassed (Anderson and Gonzalez, 2011; Feeley and Silman, 2011), as well as when extrapolation to new regions with different prevailing environmental conditions is required (Randin et al., 2006; Anderson and Gonzalez, 2011; Knudby et al., 2013a). In these circumstances, which may apply to the random forest models of this study, poor model performance and over-prediction can occur, resulting in a high rate of false positive and false negative results (Anderson and Gonzalez, 2011; Ashford et al., 2014).
Given these caveats and the results of the present study, we argue that, even considering that the NAFO Regulatory Area is one of the most data-rich deep-sea areas globally, VME distribution modelling in this region is not yet sufficiently developed to be relied upon in isolation for scientific and management applications. Model performance can be improved by field-validation and the collection of additional high-quality biological and environmental data.
Our results suggest that gorgonian corals have a limited influence over peracarid assemblages in the NAFO Regulatory Area (Figure 4 and Table 3). Such findings may reflect a very low prevalence of gorgonians in the study area (Figure 2C), and an inefficacy of box corers in sampling gorgonian coral habitat, rather than an irrelevance of their presence to benthic peracarid communities in general. It appears that at no location from which samples were collected do gorgonian corals reach a sufficient density to dominate the benthic environment and significantly influence peracarid assemblages at the spatial scale investigated.
Like gorgonians, pennatulaceans also appear to have limited influence over peracarid assemblages in the study area (Table 3). Furthermore, as found for Porifera, the results of the analyses presented here contrast depending on which metric of pennatulacean biomass is considered – that provided by Kenchington et al. (2014), or that given by the random forest SDM (Figure 4 and Table 3). Conspicuously, no positive influence of pennatulacean biomass on any of the faunal metrics analysed was recovered. This is surprising since pennatulacean fields are traditionally thought to provide elevated habitat heterogeneity in the deep sea, and can act as a food source for some amphipod species (Buhl-Mortensen et al., 2010).
Two factors may help to explain these relationships. Firstly, like gorgonians, pennatulaceans do not seem to reach particularly high densities in the study area (see Figure 2D). As a result, their densities may never be great enough to strongly shape peracarid communities. Secondly, pennatulacean fields in relative proximity to the sampling regions are known to be utilised extensively by fish (particularly Sebastes spp.) as spawning and nursery grounds (Baillon et al., 2012). We speculate that the above recovered relationships could reflect a signature of top-down control on peracarid assemblages by fish in proximity to pennatulacean fields.
That samples taken from within the NAFO VME closures (Figure 1) did not exhibit elevated peracarid abundance, biomass or diversity (Figure 4 and Table 3) was unexpected since the locations of these closures should correspond closely with the presence of VMEs, and the presence of VMEs should promote elevated macrofaunal biodiversity (Figure 4 and Table 3). In fact, peracarid assemblages sampled from within the fishery closures are statistically indistinguishable [save for slightly lowered abundance and marginally elevated functional and taxon evenness (Figures 3J,K)] from those sampled from surrounding areas, suggesting that VMEs occur in similar densities inside and outside of the closures.
This finding could reflect the simple shapes of the closures (Figure 1); because of this simplicity, the area encompassed by each closure may include a variety of benthic habitats – both VME and non-VME. That a sample was collected from within a closure does not necessarily guarantee that the sample was collected from within a VME habitat, although it should guarantee a certain level of proximity to VME habitats. Further, there is some evidence that not all areas where VMEs are known/are likely to occur are currently protected by fishery closures (NAFO, 2015; Barrio Froján et al., 2016; Gianni et al., 2016). Thus, some samples collected from outside of the fishery closures may also have been collected from VME habitats. There is also evidence that some of the closures currently enforced by NAFO (e.g., the 3O coral closure) describe areas where VME density is unlikely to be significantly higher than surrounding regions, and so could be re-located more appropriately (Rogers and Gianni, 2009). Additional ground-truthing, for example by the use of towed drop-camera surveys, may help to better establish the relative densities of VME indicator taxa inside and outside of fishery closures.
Overall, our results suggest that the current location of fishery closures may not be optimal in terms of providing adequate protection to VMEs across the NAFO Regulatory Area whilst minimising conflict with ongoing fishing activities. A review of the spatial details of current closures in the NAFO Regulatory Area may be warranted to ensure that these closures are as effective as possible.
The results of this study re-emphasise the important influence that structure-forming VME-indicator taxa have over a variety of peracarid assemblage attributes. Particularly striking was the clear dominance of poriferan grounds over the coral (gorgonian and pennatulacean) habitats analysed in terms of their influence on peracarid assemblages. This confirms the status of poriferans as one of the key habitat-forming biological structures (in life and death) in the study region.
Importantly, our results suggest that species distribution models are not yet dependable enough to be applied as the sole source of information for ecological investigations or management decisions in the NAFO Regulatory Area (and, perhaps, the deep sea in general). Field-validation, further biological sampling, and the collection of additional high-quality environmental data, should be promoted.
Lastly, the results of this study suggest that the current fishery closures enforced in the NAFO Regulatory Area may not be optimal in terms of protecting all components of VMEs whilst limiting conflict with current bottom-fishing operations. A review of the details of the current system of VME closures in the NAFO Regulatory Area may be warranted to ensure that these closures are as effective as possible.
OA, AR, AK, and CBF conceived the study. AR, AK, and CBF provided biological specimens, accessed the environmental data, and secured funding. OA and TH identified the biological specimens. OA and A-LD undertook the kernel density surface and random forest modelling. OA, A-LD, and CBF processed the remaining environmental data. OA undertook all other analyses and wrote the manuscript, which was contributed to and edited by AK, CBF, A-LD, TH, and AR.
This research was supported by the Natural Environment Research Council (NERC) Collaborative Awards in Science and Engineering (CASE) studentship (NE/K006886/1), by the Merton College, University of Oxford, United Kingdom, and through the European Union’s Horizon 2020 Research and Innovation Programme under grant agreement no. 678760 (ATLAS). This output reflects only the authors’ views and the European Union cannot be held responsible for any use that may be made of the information contained therein. The funders had no role in study design, data collection and analysis, decision to publish, or preparation of the manuscript.
CBF is employed by company Seascape Consultants Ltd.
The remaining authors declare that the research was conducted in the absence of any commercial or financial relationships that could be construed as a potential conflict of interest.
We thank Dr Ellen Kenchington (Bedford Institute of Oceanography, Canada) for providing the Canadian and Spanish fishery research survey trawl data required for the kernel density estimation analysis of VME-indicator taxa distributions. This study was undertaken as part of the “NAFO potential vulnerable marine ecosystems – impacts of deep-sea fisheries” (NEREIDA) programme, which is supported by Spain’s General Secretary of the Sea, Spain’s Ministry for the Rural and Marine Environment, the Spanish Institute of Oceanography, the Geological Survey of Canada, the Canadian Hydrographic Service, Fisheries and Oceans Canada, the United Kingdom’s Centre for the Environment Fisheries and Aquaculture Science, the Russian Polar Research Institute of Marine Fisheries and Oceanography, and the Russian P.P. Shirshov Institute of Oceanology. We thank the crew and scientists aboard the Spanish research vessel Miguel Oliver who collected the box core samples analysed in this study. We are grateful to the two reviewers and the editor AG for generously providing their time and offering insightful, constructive comments.
The Supplementary Material for this article can be found online at: https://www.frontiersin.org/articles/10.3389/fmars.2019.00401/full#supplementary-material
TABLE S1 | Details of error distribution and link functions used for each optimised Generalised Additive Model (GAM) constructed.
TABLE S2 | Peracarid crustacean genus and family composition per sample.
TABLE S3 | Raw biological and environmental data analysed in this study.
Akaike, H. (1974). A new look at the statistical model identification. IEEE Trans. Automat. Control 19, 716–723. doi: 10.1109/tac.1974.1100705
Amsler, M. O., Mcclintock, J. B., Amsler, C. D., Angus, R. A., and Baker, B. J. (2009). An evaluation of sponge-associated amphipods from the Antarctic Peninsula. Antarct. Sci. 21, 579–589. doi: 10.1017/s0954102009990356
Anderson, M. J., and Willis, T. J. (2003). Canonical analysis of principal coordinates: a useful method of constrained ordination for ecology. Ecology 84, 511–525. doi: 10.1890/0012-9658(2003)084
Anderson, R. P., and Gonzalez, I. Jr. (2011). Species-specific tuning increases robustness to sampling bias in models of species distributions: an implementation with Maxent. Ecolog. Model. 222, 2796–2811. doi: 10.1016/j.ecolmodel.2011.04.011
Ashford, O. S., Davies, A. J., and Jones, D. O. B. (2014). Deep-sea benthic megafaunal habitat suitability modelling: a global-scale maximum entropy model for xenophyophores. Deep Sea Res. Part I 94, 31–44. doi: 10.1016/j.dsr.2014.07.012
Ashford, O. S., Kenny, A. J., Barrio Frojan, C. R. S., Bonsall, M. B., Horton, T., Brandt, A., et al. (2018). Phylogenetic and functional evidence suggests that deep-ocean ecosystems are highly sensitive to environmental change and direct human disturbance. Proc. R. Soc. B Biol. Sci. 285:20180923. doi: 10.1098/rspb.2018.0923
Auster, P. J. (2005). ’Are deep-water corals important habitats for fishes?’, in Cold-Water Corals and Ecosystems, eds A. Freiwald and J. M. Roberts (Heidelberg: Springer), 747–760. doi: 10.1007/3-540-27673-4_39
Auster, P. J., Gjerde, K. M., Heupel, E., Watling, L., Grehan, A., and Rogers, A. D. (2011). Definition and detection of vulnerable marine ecosystems on the high seas: problems with the “move-on” rule. ICES J. Mar. Sci. 68, 254–264. doi: 10.1093/icesjms/fsq074
Baillon, S., Hamel, J.-F., Wareham, V. E., and Mercier, A. (2012). Deep cold-water corals as nurseries for fish larvae. Front. Ecol. Environ. 10, 351–356. doi: 10.1890/120022
Balmford, A., Green, M. J. B., and Murray, M. G. (1996a). Using higher-taxon richness as a surrogate for species richness: I. regional tests. Proc. R. Soc. Lond. Ser. B Biol. Sci. 263, 1267–1274. doi: 10.1098/rspb.1996.0186
Balmford, A., Jayasuriya, A. H. M., and Green, M. J. B. (1996b). Using higher-taxon richness as a surrogate for species richness: II. local applications. Proc. R. Soc. Lond. Ser. B Biol. Sci. 263, 1571–1575. doi: 10.1098/rspb.1996.0230
Balmford, A., Lyon, A. J. E., and Lang, R. M. (2000). Testing the higher-taxon approach to conservation planning in a megadiverse group: the macrofungi. Biol. Conserv. 93, 209–217. doi: 10.1016/s0006-3207(99)00140-8
Barrio Froján, C. R. S., Downie, A.-L., Sacau Cuadrado, M., Kenchington, E., and Kenny, A. (2016). Evaluation of benthic assemblage structure in the NAFO regulatory area with regard to the protection of VME. ICES J. Mar. Sci. 73, 405–419. doi: 10.1093/icesjms/fsv186
Barrio Froján, C. R. S., MacIsaac, K. G., McMillan, A. K., del Mar Sacau Cuadrado, M., Large, P. A., Kenny, A. J., et al. (2012). An evaluation of benthic community structure in and around the Sackville Spur closed area (Northwest Atlantic) in relation to the protection of vulnerable marine ecosystems. ICES J. Mar. Sci. 69, 213–222. doi: 10.1093/icesjms/fss004
Barthel, D. (1992). Do hexactinellids structure antarctic sponge associations? Ophelia 36, 111–118. doi: 10.1080/00785326.1992.10430362
Beazley, L., Kenchington, E., Yashayaev, I., and Murillo, F. J. (2015). Drivers of epibenthic megafaunal composition in the sponge grounds of the Sackville Spur, northwest Atlantic. Deep Sea Res. Part I Oceanogr. Res. Pap. 98, 102–114. doi: 10.1016/j.dsr.2014.11.016
Beazley, L. I., Kenchington, E. L., Murillo, F. J., and del Mar Sacau, M. (2013). Deep-sea sponge grounds enhance diversity and abundance of epibenthic megafauna in the Northwest Atlantic. ICES J. Mar. Sci. 70, 1471–1490. doi: 10.1093/icesjms/fst124
Bett, B. J., and Rice, A. L. (1992). The influence of hexactinellid sponge (Pheronema carpenteri) spicules on the patchy distribution of macrobenthos in the porcupine seabight (bathyal NE Atlantic). Ophelia 36, 217–226. doi: 10.1080/00785326.1992.10430372
Boulangeat, I., Gravel, D., and Thuiller, W. (2012). Accounting for dispersal and biotic interactions to disentangle the drivers of species distributions and their abundances. Ecol. Lett. 15, 584–593. doi: 10.1111/j.1461-0248.2012.01772.x
Bray, J. R., and Curtis, J. T. (1957). An ordination of the upland forest communities of southern Wisconsin. Ecol. Monogr. 27, 325–349. doi: 10.2307/1942268
Buhl-Mortensen, L., and Mortensen, P. B. (2005). “’Distribution and diversity of species associated with deep-sea gorgonian corals off Atlantic Canada,” in Cold-Water Corals and Ecosystems, eds A. Freiwald and J. M. Roberts (Berlin: Springer), 849–879. doi: 10.1007/3-540-27673-4_44
Buhl-Mortensen, L., Vanreusel, A., Gooday, A. J., Levin, L. A., Priede, I. G., Buhl-Mortensen, P., et al. (2010). Biological structures as a source of habitat heterogeneity and biodiversity on the deep ocean margins. Mar. Ecol. 31, 21–50. doi: 10.1111/j.1439-0485.2010.00359.x
Cathalot, C., Van Oevelen, D., Cox, T. J. S., Kutti, T., Lavaleye, M. S. S., Duineveld, G., et al. (2015). Cold-water coral reefs and adjacent sponge grounds: hotspots of benthic respiration and organic carbon cycling in the deep sea. Front. Mar. Sci. 2:1–12.
Cinar, M. E., Katagan, T., Ergen, Z., and Sezgin, M. (2002). Zoobenthos inhabiting Sarcotragus muscarum (Porifera: Demospongiae) from the Aegean Sea. Hydrobiologia 482, 107–117.
Clark, M. R., Althaus, F., Schlacher, T. A., Williams, A., Bowden, D. A., and Rowden, A. A. (2016). The impacts of deep-sea fisheries on benthic communities: a review. ICES J. Mar. Sci. 73, i51–i69. doi: 10.1371/journal.pone.0022588
Debastiani, V. J. (2015). SYNCSA-Analysis of Functional and Phylogenetic Patterns in Metacommunities. Version 1.3.
Edinger, E. N., Wareham, V. E., and Haedrich, R. L. (2007). Patterns of groundfish diversity and abundance in relation to deep-sea coral distributions in Newfoundland and Labrador waters. Bull. Mar. Sci. 81, 101–122.
Etnoyer, P., and Warrenchuk, J. (2007). A catshark nursery in a deep gorgonian field in the Mississippi Canyon, Gulf of Mexico. Bull. Mar. Sci. 81, 553–559.
FAO (2009). International Guidelines for the Management of Deep-Sea Fisheries in the High Seas. Rome: Food and Agriculture Organisation.
Feeley, K. J., and Silman, M. R. (2011). Keep collecting: accurate species distribution modelling requires more collections than previously thought. Divers. Distrib. 17, 1132–1140. doi: 10.1111/j.1472-4642.2011.00813.x
Freese, J. L., and Wing, B. L. (2003). Juvenile red rockfish, Sebastes spp., associations with sponges in the Gulf of Alaska. Mar. Fish. Rev. 65, 38–42.
Fuller, S. D., Murillo, F. J., Wareham, V., and Kenchington, E. (2008). Vulnerable Marine Ecosystems Dominated by Deep-Water Corals and Sponges in the NAFO Convention Area: NAFO SCR Doc. 08/22). Dartmouth: Northwest Atlantic Fisheries Organisation.
Gerovasileiou, V., Chintiroglou, C. C., Konstantinou, D., and Voultsiadou, E. (2016). Sponges as “living hotels” in Mediterranean marine caves. Sci. Mar. 80, 279–290.
Gheerardyn, H., de Troch, M., Vincx, M., and Vanreusel, A. (2009). Harpacticoida (Crustacea: Copepoda) associated with cold-water coral substrates in the Porcupine Seabight (NE Atlantic): species composition, diversity and reflections on the origin of the fauna. Sci. Mar. 73, 747–760. doi: 10.3989/scimar.2009.73n4747
Gianni, M., Currie, D. E. J., Fuller, S., Speer, L., Ardon, J., Weeber, B., et al. (2011). Unfinished Business: a Review of the Implementation of the Provisions of UNGA Resolutions 61/105 and 64/72 Related to the Management of Bottom Fisheries in Areas Beyond National Jurisdiction. Available at: http://www.savethehighseas.org/wp-content/uploads/2011/09/DSCC_review11.pdf (accessed September, 2011).
Gianni, M., Fuller, S. D., Currie, D. E. J., Schleit, K., Goldsworthy, L., Pike, B., et al. (2016). How Much Longer Will it Take? A Ten-Year Review of the Implementation of United Nations General Assembly resolutions 61/105, 64/72 and 66/68 on the Management of Bottom Fisheries in Areas Beyond National Jurisdiction: Deep Sea Conservation Coalition. Available at: http://www.savethehighseas.org/wp-content/uploads/2016/07/DSCC-Review-2016_Launch-29-July.pdf (accessed August, 2016).
Gunst, R. F., and Webster, J. T. (1975). Regression analysis and problems of multicollinearity. Commun. Stat. 4, 277–292. doi: 10.1080/03610927308827246
Hansen, S., Ward, P., and Penney, A. (2013). Identification of Vulnerable Benthic Taxa in the Western SPRFMO Convention Area and Review of Move-on Rules for Different Gear Types, SC-01-09 Presented at the First Meeting of the South Pacific Regional Fisheries Management Organisation (SPRFMO) Scientific Committee (La Jolla, 21-27 September 2013). Canberra: Australian Bureau of Agricultural and Resource Economics and Sciences (ABARES).
Helmus, M. R., Bland, T. J., Williams, C. K., and Ives, A. R. (2007). Phylogenetic measures of biodiversity. Am. Natural. 169, E68–E83. doi: 10.1086/511334
Henkel, T. P., and Pawlik, J. R. (2005). Habitat use by sponge-dwelling brittlestars. Mar. Biol. 146, 301–313. doi: 10.1007/s00227-004-1448-x
Hentschel, U., Hopke, J., Horn, M., Friedrich, A. B., Wagner, M., Hacker, J., et al. (2002). Molecular evidence for a uniform microbial community in sponges from different oceans. Appl. Environ. Microbiol. 68, 4431–4440. doi: 10.1128/aem.68.9.4431-4440.2002
Hogg, M. M., Tendal, O. S., Conway, K. W., Pomponi, S. A., van Soest, R. W. M., Gutt, J., et al. (2010). Deep-Sea Sponge Grounds: Reservoirs of Biodiversity. Cambridge: UNEP-WCMC.
Husebø,Å, Nøttestad, L., Fosså, J. H., Furevik, D. M., and Jørgensen, S. B. (2002). Distribution and abundance of fish in deep-sea coral habitats. Hydrobiologia 471, 91–99.
Jablonski, D., and Finarelli, J. A. (2009). Congruence of morphologically-defined genera with molecular phylogenies. Proc. Natl. Acad. Sci. U.S.A. 106, 8262–8266. doi: 10.1073/pnas.0902973106
Jobstvogt, N., Hanley, N., Hynes, S., Kenter, J., and Witte, U. (2014). Twenty thousand sterling under the sea: estimating the value of protecting deep-sea biodiversity. Ecol. Econom. 97, 10–19. doi: 10.1016/j.ecolecon.2013.10.019
Kembel, S. W., Ackerly, D. D., Blomberg, S. P., Cornwell, W. K., Cowan, P. D., Helmus, M. R., et al. (2014). Picante’ R Tools for Integrating Phylogenies and Ecology. Version.1.6-2.
Kenchington, E., Murillo, F. J., Cogswell, A., and Lirette, C. (2011). Development of Encounter Protocols and Assessment of Significant Adverse Impact by Bottom Trawling for Sponge Grounds and Sea Pen Fields in the NAFO Regulatory Area Ser No 6005. NAFO SCR Doc 11/75. Dartmouth: NAFO.
Kenchington, E., Murillo, F. J., Lirette, C., Sacau, M., Koen-Alonso, M., Kenny, A., et al. (2014). Kernel density surface modelling as a means to identify significant concentrations of vulnerable marine ecosystem indicators. PLoS One 9:e109365. doi: 10.1371/journal.pone.0109365
Klitgaard, A. B. (1995). The fauna associated with outer shelf and upper slope sponges (Porifera, Demospongiae) at the Faroe Islands, northeastern Atlantic. Sarsia 80, 1–22. doi: 10.1080/00364827.1995.10413574
Knudby, A., Kenchington, E., and Murillo, F. J. (2013a). Modeling the distribution of Geodia Sponges and Sponge Grounds in the Northwest Atlantic. PLoS One 8:e82306. doi: 10.1371/journal.pone.0082306
Knudby, A., Lirette, C., Kenchington, E., and Murillo, F. J. (2013b). Species Distribution Models of Black Corals, Large Gorgonian Corals and Sea Pens in the NAFO Regulatory Area: Northwest Atlantic Fisheries OrganisationNAFO SCR Doc. 13/078. Serial No. N6276. Dartmouth: NAFO.
Koslow, J. A., and Gowlet-Holmes, K. (1998). The Seamount Fauna off Southern Tasmania: Benthic Communities, Their Conservation and Impacts of Trawling. Canberra: Environment Australia and the Fisheries Research Development Corporation.
Krieger, K. J., and Wing, B. L. (2002). Megafauna associations with deepwater corals (Primnoa spp.) in the Gulf of Alaska. Hydrobiologia 471, 83–90.
Kursa, M. B., and Rudnicki, W. R. (2010). Feature selection with the Boruta package. J. Stat. Softw. 36, 1–13.
La Ferla, B., Lovett, J. C., Ockwell, D., and Taplin, J. (2002). Continental scale patterns of biodiversity: can higher taxa accurately predict African plant distributions? Botan. J. Linn. Soc. 138, 225–235. doi: 10.1046/j.1095-8339.2002.138002225.x
Liaw, A., Wiener, M., Breiman, L., and Cutler, A. (2015). Package ’Random Forest’ Breiman and Cutler’s Random Forests for Classification and Regression. Version 4.6-12.
Moran, P. A. P. (1950). Notes on continuous stochastic phenomena. Biometrika 37, 17–23. doi: 10.1093/biomet/37.1-2.17
Murillo, F. J., Durán Muñoz, P., Altuna, A., and Serrano, A. (2011). Distribution of deep-water corals of the Flemish Cap, Flemish Pass, and the Grand Banks of Newfoundland (Northwest Atlantic Ocean): interaction with fishing activities. ICES J. Mar. Sci. 68, 319–332. doi: 10.1093/icesjms/fsq071
Murillo, F. J., Kenchington, E., Gonzalez, C., and Sacau, M. (2010). The Use of Density Analyses to Delineate Significant Concentrations of Pennatulaceans from Trawl Survey Data Ser No. N5753. NAFO SCR Doc 10/07). Dartmouth: NAFO.
Murillo, F. J., Muñoz, P. D., Cristobo, J., Ríos, P., González, C., Kenchington, E., et al. (2012). Deep-sea sponge grounds of the Flemish Cap, Flemish Pass and the Grand Banks of Newfoundland (Northwest Atlantic Ocean): Distribution and species composition. Mar. Biol. Res. 8, 842–854. doi: 10.1080/17451000.2012.682583
NAFO (2015). Report of the Scientific Council Meeting, 29 May - 11 June 2015, Halifax, Nova ScotiaSerial No. N6469, NAFO SCS Doc. 15-12. Dartmouth: NAFO.
NAFO (2017). Northwest Atlantic Fisheries Organisation Conservation and Enforcement Measures. Dartmouth: NAFO.
Oksanen, J., Blanchet, F. G., Kindt, R., Legendre, P., Minchin, P. R., O’Hara, R. B., et al. (2013). Vegan: Community Ecology Package. R Package Version 2.0–9.
Paradis, E., Claude, J., and Strimmer, K. (2004). APE: analyses of phylogenetics and evolution in R language (version 3.0-11). Bioinformatics 20, 289–290. doi: 10.1093/bioinformatics/btg412
Parker, S. J., Penney, A. J., and Clark, M. R. (2009). Detection criteria for managing trawl impacts to Vulnerable Marine Ecosystems in high seas fisheries of the South Pacific Ocean. Mar. Ecol. Progr. Ser. 397, 309–317. doi: 10.3354/meps08115
Pham, C. K., Vandeperre, F., Menezes, G., Porteiro, F., Isidro, E., and Morato, T. (2015). The importance of deep-sea vulnerable marine Ecosystems (VMEs) for demersal fish in the Azores. Deep Sea Res. Part I Oceanogr. Res. Pap. 96, 80–88. doi: 10.1016/j.dsr.2014.11.004
Pile, A. J., and Young, C. M. (2006). The natural diet of a hexactinellid sponge: benthic–pelagic coupling in a deep-sea microbial food web. Deep Sea Res. Part I Oceanogr. Res. Pap. 53, 1148–1156. doi: 10.1016/j.dsr.2006.03.008
Raes, M., and Vanreusel, A. (2005). “’The metazoan meiofauna associated with a cold-water coral degradation zone in the Porcupine Seabight (NE Atlantic),” in Cold-Water Corals and Ecosystems, eds A. Freiwald and J. M. Roberts (Berlin: Springer), 821–847. doi: 10.1007/3-540-27673-4_43
Randin, C. F., Dirnböck, T., Dullinger, S., Zimmermann, N. E., Zappa, M., and Guisan, A. (2006). Are niche-based species distribution models transferable in space? J. Biogeogr. 33, 1689–1703. doi: 10.1111/j.1365-2699.2006.01466.x
R Core Team (2013). R: A Language and Environment for Statistical Computing. Vienna: R foundation for statistical computing.
Reddy, N. S., Reed, J. K., Longley, R. E., and Wright, A. E. (2005). Two new cytotoxic linderazulenes from a deep-sea gorgonian of the genus Paramuricea. J. Nat. Prod. 68, 248–250. doi: 10.1021/np040147u
Rengstorf, A. M., Yesson, C., Brown, C., and Grehan, A. J. (2013). High-resolution habitat suitability modelling can improve conservation of vulnerable marine ecosystems in the deep sea. J. Biogeogr. 40, 1702–1714. doi: 10.1111/jbi.12123
Rogers, A. D., Clark, M. R., Hall-Spencer, J. M., and Gjerde, K. M. (2008). The Science Behind the Guidelines: A Scientific Guide to the FAO Draft International Guidelines (December 2007) for the Management of Deep-Sea Fisheries in the High Seas and Examples of How the Guidelines May be Practically Implemented. Switzerland: IUCN.
Rogers, A. D., and Gianni, M. (2009). The Implementation of UN Resolution 61/105 in the Management of Deep-Sea Fisheries on the High Seas. Provisional Report, North Atlantic: Status and Recommendations. London: International Programme on the State of the Ocean.
Roy, K., Jablonski, D., and Valentine, J. W. (1996). Higher taxa in biodiversity studies: patterns from eastern pacific marine molluscs. Philos. Trans. R. Soc. Lond. Ser. B Biol. Sci. 351, 1605–1613. doi: 10.1098/rstb.1996.0144
Skropeta, D. (2008). Deep-sea natural products. Nat. Prod. Rep. 25, 1131–1166. doi: 10.1039/b808743a
Stine, R. A. (1995). Graphical interpretation of variance inflation factors. Am. Stat. 49, 53–56. doi: 10.1080/00031305.1995.10476113
Thrush, S. F., Hewitt, J. E., Cummings, V. J., Dayton, P. K., Cryer, M., Turner, S. J., et al. (1998). Disturbance of the marine benthic habitat by commercial fishing: impacts at the scale of the fishery. Ecol. Appl. 8, 866–879. doi: 10.1890/1051-0761(1998)008
Tissot, B. N., Yoklavich, M. M., Love, M. S., York, K., and Amend, M. (2006). Benthic invertebrates that form habitat on deep banks off southern California, with special reference to deep sea coral. Fish. Bull. 104, 167–181.
van Oevelen, D., Duineveld, G., Lavaleye, M. S. S., Mienis, F., Soetaert, K., and Heip, C. H. R. (2009). The cold-water coral community as a hot spot for carbon cycling on continental margins: a food-web analysis from Rockall Bank (northeast Atlantic). Limnol. Oceanogr. 54, 1829–1844. doi: 10.4319/lo.2009.54.6.1829
Villaseñor, J. L., Ibarra-Manríquez, G., Meave, J. A., and Ortíz, E. (2005). Higher taxa as surrogates of plant biodiversity in a megadiverse country. Conserv. Biol. 19, 232–238. doi: 10.1111/j.1523-1739.2005.00264.x
Wood, S. N. (2013). Mixed GAM Computation Vehicle With GCV/AIC/REML Smoothness Estimation. Version 1.7-26.
Wright, A. E., Chen, Y., Winder, P. L., Pitts, T. P., Pomponi, S. A., and Longley, R. E. (2004). Lasonolides C-G, five new lasonolide compounds from the sponge Forcepia sp. J. Nat. Prod. 67, 1351–1355. doi: 10.1021/np040028e
Wright, G., Ardron, J. A., Gjerde, K., Currie, D. E. J., and Rochette, J. (2015). Advancing marine biodiversity protection through regional fisheries management: a review of bottom fisheries closures in areas beyond national jurisdiction. Mar. Policy 61, 134–148. doi: 10.1016/j.marpol.2015.06.030
Keywords: vulnerable marine ecosystem, Northwest Atlantic Fisheries Organisation, porifera, coral, macrofauna, peracarida, species distribution modelling, deep sea
Citation: Ashford OS, Kenny AJ, Barrio Froján CRS, Downie A-L, Horton T and Rogers AD (2019) On the Influence of Vulnerable Marine Ecosystem Habitats on Peracarid Crustacean Assemblages in the Northwest Atlantic Fisheries Organisation Regulatory Area. Front. Mar. Sci. 6:401. doi: 10.3389/fmars.2019.00401
Received: 27 December 2018; Accepted: 27 June 2019;
Published: 11 July 2019.
Edited by:
Anthony Grehan, National University of Ireland Galway, IrelandReviewed by:
Pedro A. Ribeiro, University of Bergen, NorwayCopyright © 2019 Ashford, Kenny, Barrio Froján, Downie, Horton and Rogers. This is an open-access article distributed under the terms of the Creative Commons Attribution License (CC BY). The use, distribution or reproduction in other forums is permitted, provided the original author(s) and the copyright owner(s) are credited and that the original publication in this journal is cited, in accordance with accepted academic practice. No use, distribution or reproduction is permitted which does not comply with these terms.
*Correspondence: Oliver S. Ashford, b2FzaGZvcmRAdWNzZC5lZHU=
†Present address: Oliver S. Ashford, Scripps Institution of Oceanography, La Jolla, CA, United States
Disclaimer: All claims expressed in this article are solely those of the authors and do not necessarily represent those of their affiliated organizations, or those of the publisher, the editors and the reviewers. Any product that may be evaluated in this article or claim that may be made by its manufacturer is not guaranteed or endorsed by the publisher.
Research integrity at Frontiers
Learn more about the work of our research integrity team to safeguard the quality of each article we publish.